- - Google Chrome
Intended for healthcare professionals
- Access provided by Google Indexer
- My email alerts
- BMA member login
- Username * Password * Forgot your log in details? Need to activate BMA Member Log In Log in via OpenAthens Log in via your institution


Search form
- Advanced search
- Search responses
- Search blogs
- Gestational diabetes...
Gestational diabetes mellitus and adverse pregnancy outcomes: systematic review and meta-analysis
- Related content
- Peer review
- Wenrui Ye , doctoral student 1 2 ,
- Cong Luo , doctoral student 3 ,
- Jing Huang , assistant professor 4 5 ,
- Chenglong Li , doctoral student 1 ,
- Zhixiong Liu , professor 1 2 ,
- Fangkun Liu , assistant professor 1 2
- 1 Department of Neurosurgery, Xiangya Hospital, Central South University, Changsha, Hunan, China
- 2 Hypothalamic Pituitary Research Centre, Xiangya Hospital, Central South University, Changsha, China
- 3 Department of Urology, Xiangya Hospital, Central South University, Changsha, Hunan, China
- 4 National Clinical Research Centre for Mental Disorders, Second Xiangya Hospital, Central South University, Changsha, Hunan, China
- 5 Department of Psychiatry, Second Xiangya Hospital, Central South University, Changsha, Hunan, China
- Correspondence to: F Liu liufangkun{at}csu.edu.cn
- Accepted 18 April 2022
Objective To investigate the association between gestational diabetes mellitus and adverse outcomes of pregnancy after adjustment for at least minimal confounding factors.
Design Systematic review and meta-analysis.
Data sources Web of Science, PubMed, Medline, and Cochrane Database of Systematic Reviews, from 1 January 1990 to 1 November 2021.
Review methods Cohort studies and control arms of trials reporting complications of pregnancy in women with gestational diabetes mellitus were eligible for inclusion. Based on the use of insulin, studies were divided into three subgroups: no insulin use (patients never used insulin during the course of the disease), insulin use (different proportions of patients were treated with insulin), and insulin use not reported. Subgroup analyses were performed based on the status of the country (developed or developing), quality of the study, diagnostic criteria, and screening method. Meta-regression models were applied based on the proportion of patients who had received insulin.
Results 156 studies with 7 506 061 pregnancies were included, and 50 (32.1%) showed a low or medium risk of bias. In studies with no insulin use, when adjusted for confounders, women with gestational diabetes mellitus had increased odds of caesarean section (odds ratio 1.16, 95% confidence interval 1.03 to 1.32), preterm delivery (1.51, 1.26 to 1.80), low one minute Apgar score (1.43, 1.01 to 2.03), macrosomia (1.70, 1.23 to 2.36), and infant born large for gestational age (1.57, 1.25 to 1.97). In studies with insulin use, when adjusted for confounders, the odds of having an infant large for gestational age (odds ratio 1.61, 1.09 to 2.37), or with respiratory distress syndrome (1.57, 1.19 to 2.08) or neonatal jaundice (1.28, 1.02 to 1.62), or requiring admission to the neonatal intensive care unit (2.29, 1.59 to 3.31), were higher in women with gestational diabetes mellitus than in those without diabetes. No clear evidence was found for differences in the odds of instrumental delivery, shoulder dystocia, postpartum haemorrhage, stillbirth, neonatal death, low five minute Apgar score, low birth weight, and small for gestational age between women with and without gestational diabetes mellitus after adjusting for confounders. Country status, adjustment for body mass index, and screening methods significantly contributed to heterogeneity between studies for several adverse outcomes of pregnancy.
Conclusions When adjusted for confounders, gestational diabetes mellitus was significantly associated with pregnancy complications. The findings contribute to a more comprehensive understanding of the adverse outcomes of pregnancy related to gestational diabetes mellitus. Future primary studies should routinely consider adjusting for a more complete set of prognostic factors.
Review registration PROSPERO CRD42021265837.

- Download figure
- Open in new tab
- Download powerpoint
Introduction
Gestational diabetes mellitus is a common chronic disease in pregnancy that impairs the health of several million women worldwide. 1 2 Formally recognised by O’Sullivan and Mahan in 1964, 3 gestational diabetes mellitus is defined as hyperglycaemia first detected during pregnancy. 4 With the incidence of obesity worldwide reaching epidemic levels, the number of pregnant women diagnosed as having gestational diabetes mellitus is growing, and these women have an increased risk of a range of complications of pregnancy. 5 Quantification of the risk or odds of possible adverse outcomes of pregnancy is needed for prevention, risk assessment, and patient education.
In 2008, the Hyperglycaemia and Adverse Pregnancy Outcome (HAPO) study recruited a large multinational cohort and clarified the risks of adverse outcomes associated with hyperglycaemia. The findings of the study showed that maternal hyperglycaemia independently increased the risk of preterm delivery, caesarean delivery, infants born large for gestational age, admission to a neonatal intensive care unit, neonatal hypoglycaemia, and hyperbilirubinaemia. 6 The obstetric risks associated with diabetes, such as pregnancy induced hypertension, macrosomia, congenital malformations, and neonatal hypoglycaemia, have been reported in several large scale studies. 7 8 9 10 11 12 The HAPO study did not adjust for some confounders, however, such as maternal body mass index, and did not report on stillbirths and neonatal respiratory distress syndrome, raising uncertainty about these outcomes. Other important pregnancy outcomes, such as preterm delivery, neonatal death, and low Apgar score in gestational diabetes mellitus, were poorly reported. No comprehensive study has assessed the relation between gestational diabetes mellitus and various maternal and fetal adverse outcomes after adjustment for confounders. Also, some cohort studies were restricted to specific clinical centres and regions, limiting their generalisation to more diverse populations.
By collating the available evidence, we conducted a systematic review and meta-analysis to quantify the short term outcomes in pregnancies complicated by gestational diabetes mellitus. We evaluated adjusted associations between gestational diabetes mellitus and various adverse outcomes of pregnancy.
This meta-analysis was conducted according to the recommendations of Cochrane Systematic Reviews, and our findings are reported in accordance with the Preferred Reporting Items for Systematic Reviews and Meta-analyses (table S16). The study was prospectively registered in the international database of prospectively registered systematic reviews (PROSPERO CRD42021265837).
Search strategy and selection criteria
We searched the electronic databases PubMed, Web of Science, Medline, and the Cochrane Database of Systematic Reviews with the keywords: “pregnan*,” “gestatio*” or “matern*” together with “diabete*,” “hyperglycaemia,” “insulin,” “glucose,” or “glucose tolerance test*” to represent the exposed populations, and combined them with terms related to outcomes, such as “pregnan* outcome*,” “obstetric* complicat*,” “pregnan* disorder*,” “obstetric* outcome*,” “haemorrhage,” “induc*,” “instrumental,” “caesarean section,” “dystocia,” “hypertensi*,” “eclampsia,” “premature rupture of membrane,” “PROM,” “preter*,” “macrosomia,” and “malformation,” as well as some abbreviated diagnostic criteria, such as “IADPSG,” “DIPSI,” and “ADIPS” (table S1). The search strategy was appropriately translated for the other databases. We included observational cohort studies and control arms of trials, conducted after 1990, that strictly defined non-gestational diabetes mellitus (control) and gestational diabetes mellitus (exposed) populations and had definite diagnostic criteria for gestational diabetes mellitus (table S2) and various adverse outcomes of pregnancy.
Exclusion criteria were: studies published in languages other than English; studies with no diagnostic criteria for gestational diabetes mellitus (eg, self-reported gestational diabetes mellitus, gestational diabetes mellitus identified by codes from the International Classification of Diseases or questionnaires); studies published after 1990 that recorded pregnancy outcomes before 1990; studies of specific populations (eg, only pregnant women aged 30-34 years, 13 only twin pregnancies 14 15 16 ); studies with a sample size <300, because we postulated that these studies might not be adequate to detect outcomes within each group; and studies published in the form of an abstract, letter, or case report.
We also manually retrieved reference lists of relevant reviews or meta-analyses. Three reviewers (WY, CL, and JH) independently searched and assessed the literature for inclusion in our meta-analysis. The reviewers screened the titles and abstracts to exclude ineligible studies. The full texts of relevant records were then retrieved and assessed. Any discrepancies were resolved after discussion with another author (FL).
Data extraction
Three independent researchers (WY, CL, and JH) extracted data from the included studies with a predesigned form. If the data were not presented, we contacted the corresponding authors to request access to the data. We extracted data from the most recent study or the one with the largest sample size when a cohort was reported twice or more. Sociodemographic and clinical data were extracted based on: year of publication, location of the study (country and continent), design of the study (prospective or retrospective cohort), screening method and diagnostic criteria for gestational diabetes mellitus, adjustment for conventional prognostic factors (defined as maternal age, pregestational body mass index, gestational weight gain, gravidity, parity, smoking history, and chronic hypertension), and the proportion of patients with gestational diabetes mellitus who were receiving insulin. For studies that adopted various diagnostic criteria for gestational diabetes mellitus, we extracted the most recent or most widely accepted one for subsequent analysis. For studies adopting multivariate logistic regression for adjustment of confounders, we extracted adjusted odds ratios and synthesised them in subsequent analyses. For unadjusted studies, we calculated risk ratios and 95% confidence intervals based on the extracted data.
Studies of women with gestational diabetes mellitus that evaluated the risk or odds of maternal or neonatal complications were included. We assessed the maternal outcomes pre-eclampsia, induction of labour, instrumental delivery, caesarean section, shoulder dystocia, premature rupture of membrane, and postpartum haemorrhage. Fetal or neonatal outcomes assessed were stillbirth, neonatal death, congenital malformation, preterm birth, macrosomia, low birth weight, large for gestational age, small for gestational age, neonatal hypoglycaemia, neonatal jaundice, respiratory distress syndrome, low Apgar score, and admission to the neonatal intensive care unit. Table S3 provides detailed definitions of these adverse outcomes of pregnancy.
Risk-of-bias assessment
A modified Newcastle-Ottawa scale was used to assess the methodological quality of the selection, comparability, and outcome of the included studies (table S4). Three independent reviewers (WY, CL, and JH) performed the quality assessment and scored the studies for adherence to the prespecified criteria. A study that scored one for selection or outcome, or zero for any of the three domains, was considered to have a high risk of bias. Studies that scored two or three for selection, one for comparability, and two for outcome were regarded as having a medium risk of bias. Studies that scored four for selection, two for comparability, and three for outcome were considered to have a low risk of bias. A lower risk of bias denotes higher quality.
Data synthesis and analysis
Pregnant women were divided into two groups (gestational diabetes mellitus and non-gestational diabetes mellitus) based on the diagnostic criteria in each study. Studies were considered adjusted if they adjusted for at least one of seven confounding factors (maternal age, pregestational body mass index, gestational weight gain, gravidity, parity, smoking history, and chronic hypertension). For each adjusted study, we transformed the odds ratio estimate and its corresponding standard error to natural logarithms to stabilise the variance and normalise their distributions. Summary odds ratio estimates and their 95% confidence intervals were estimated by a random effects model with the inverse variance method. We reported the results as odds ratio with 95% confidence intervals to reflect the uncertainty of point estimates. Unadjusted associations between gestational diabetes mellitus and adverse outcomes of pregnancy were quantified and summarised (table S6 and table S14). Thereafter, heterogeneity across the studies was evaluated with the τ 2 statistics and Cochran’s Q test. 17 18 Cochran’s Q test assessed interactions between subgroups. 18
We performed preplanned subgroup analyses for factors that could potentially affect gestational diabetes mellitus or adverse outcomes of pregnancy: country status (developing or developed country according to the International Monetary Fund ( www.imf.org/external/pubs/ft/weo/2020/01/weodata/groups.htm ), risk of bias (low, medium, or high), screening method (universal one step, universal glucose challenge test, or selective screening based on risk factors), diagnostic criteria for gestational diabetes mellitus (World Health Organization 1999, Carpenter-Coustan criteria, International Association of Diabetes and Pregnancy Study Groups (IADPSG), or other), and control for body mass index. We assessed small study effects with funnel plots by plotting the natural logarithm of the odds ratios against the inverse of the standard errors, and asymmetry was assessed with Egger’s test. 19 A meta-regression model was used to investigate the associations between study effect size and proportion of patients who received insulin in the gestational diabetes mellitus population. Next, we performed sensitivity analyses by omitting each study individually and recalculating the pooled effect size estimates for the remaining studies to assess the effect of individual studies on the pooled results. All analyses were performed with R language (version 4.1.2, www.r-project.org ) and meta package (version 5.1-0). We adopted the treatment arm continuity correction to deal with a zero cell count 20 and the Hartung-Knapp adjustment for random effects meta models. 21 22
Patient and public involvement
The experience in residency training in the department of obstetrics and the concerns about the association between gestational diabetes mellitus and health outcomes inspired the author team to perform this study. We also asked advice from the obstetrician and patients with gestational diabetes mellitus about which outcomes could be included. The covid-19 restrictions meant that we sought opinions from only a limited number of patients in outpatient settings.
Characteristics of included studies
Of the 44 993 studies identified, 156 studies, 23 24 25 26 27 28 29 30 31 32 33 34 35 36 37 38 39 40 41 42 43 44 45 46 47 48 49 50 51 52 53 54 55 56 57 58 59 60 61 62 63 64 65 66 67 68 69 70 71 72 73 74 75 76 77 78 79 80 81 82 83 84 85 86 87 88 89 90 91 92 93 94 95 96 97 98 99 100 101 102 103 104 105 106 107 108 109 110 111 112 113 114 115 116 117 118 119 120 121 122 123 124 125 126 127 128 129 130 131 132 133 134 135 136 137 138 139 140 141 142 143 144 145 146 147 148 149 150 151 152 153 154 155 156 157 158 159 160 161 162 163 164 165 166 167 168 169 170 171 172 173 174 175 176 177 178 involving 7 506 061 pregnancies, were eligible for the analysis of adverse outcomes in pregnancy ( fig 1 ). Of the 156 primary studies, 133 (85.3%) reported maternal outcomes and 151 (96.8%) reported neonatal outcomes. Most studies were conducted in Asia (39.5%), Europe (25.5%), and North America (15.4%). Eighty four (53.8%) studies were performed in developed countries. Based on the Newcastle-Ottawa scale, 50 (32.1%) of the 156 included studies showed a low or medium risk of bias and 106 (67.9%) had a high risk of bias. Patients in 35 (22.4%) of the 156 studies never used insulin during the course of the disease and 63 studies (40.4%) reported treatment with insulin in different proportions of patients. The remaining 58 studies did not report information about the use of insulin. Table 1 summarises the characteristics of the study population, including continent or region, country, screening methods, and diagnostic criteria for the included studies. Table S5 lists the key excluded studies.

Search and selection of studies for inclusion
Characteristics of study population
- View inline
Associations between gestational diabetes mellitus and adverse outcomes of pregnancy
Based on the use of insulin in each study, we classified the studies into three subgroups: no insulin use (patients never used insulin during the course of the disease), insulin use (different proportions of patients were treated with insulin), and insulin use not reported. We reported odds ratios with 95% confidence intervals after controlling for at least minimal confounding factors. In studies with no insulin use, women with gestational diabetes mellitus had increased odds of caesarean section (odds ratio 1.16, 95% confidence interval 1.03 to 1.32), preterm delivery (1.51, 1.26 to 1.80), low one minute Apgar score (1.43, 1.01 to 2.03), macrosomia (1.70, 1.23 to 2.36), and an infant born large for gestational age (1.57, 1.25 to 1.97) ( fig 2 and fig S1). In studies with insulin use, adjusted for confounders, the odds of an infant born large for gestational age (odds ratio 1.61, 95% confidence interval 1.09 to 2.37), or with respiratory distress syndrome (1.57, 1.19 to 2.08) or neonatal jaundice (1.28, 1.02 to 1.62), or requiring admission to the neonatal intensive care unit (2.29, 1.59 to 3.31) were higher in women with than in those without gestational diabetes mellitus ( fig 3) . In studies that did not report the use of insulin, women with gestational diabetes mellitus had increased odds ratio for pre-eclampsia (1.46, 1.21 to 1.78), induction of labour (1.88, 1.16 to 3.04), caesarean section (1.38, 1.20 to 1.58), premature rupture of membrane (1.13, 1.06 to 1.20), congenital malformation (1.18, 1.10 to 1.26), preterm delivery (1.51, 1.19 to 1.93), macrosomia (1.48, 1.13 to 1.95), neonatal hypoglycaemia (11.71, 7.49 to 18.30), and admission to the neonatal intensive care unit (2.28, 1.26 to 4.13) (figs S3 and S4). We found no clear evidence for differences in the odds of instrumental delivery, shoulder dystocia, postpartum haemorrhage, stillbirth, neonatal death, low five minute Apgar score, low birth weight, and infant born small for gestational age between women with and without gestational diabetes mellitus in all three subgroups ( fig 2, fig 3, and figs S1-S4). Table S6 shows the unadjusted associations between gestational diabetes mellitus and adverse outcomes of pregnancy.

Findings of meta-analysis of association between gestational diabetes mellitus and adverse outcomes of pregnancy after adjusting for at least minimal confounding factors, in studies in patients who never used insulin during the course of the disease (no insulin use). NA=not applicable

Findings of meta-analysis of association between gestational diabetes mellitus and adverse outcomes of pregnancy after adjusting for at least minimal confounding factors, in studies where different proportions of patients were treated with insulin (insulin use). NA=not applicable
Subgroup, meta-regression, and sensitivity analyses
Subgroup analyses, based on risk of bias, did not show significant heterogeneity between the subgroups of women with and without gestational diabetes mellitus for most adverse outcomes of pregnancy ( table 2 and table 3 ), except for admission to the neonatal intensive care unit in studies where insulin use was not reported (table S7). Significant differences between subgroups were reported for country status and macrosomia in studies with (P<0.001) and without (P=0.001) insulin use ( table 2 and table 3 ), and for macrosomia (P=0.02) and infants born large for gestational age (P<0.001) based on adjustment for body mass index in studies with insulin use (table S8). Screening methods contributed significantly to the heterogeneity between studies for caesarean section (P<0.001) and admission to the neonatal intensive care unit (P<0.001) in studies where insulin use was not reported (table S7). In most outcomes, the estimated odds were lower in studies that used universal one step screening than those that adopted the universal glucose challenge test or selective screening methods ( table 2 and table 3 ). Diagnostic criteria were not related to heterogeneity between the studies for all of the study subgroups (no insulin use, insulin use, insulin use not reported). The subgroup analysis was performed only for outcomes including ≥6 studies.
Subgroup analysis according to country status, diagnostic criteria, screening method, and risk of bias for adverse outcomes of pregnancy in women with gestational diabetes mellitus compared with women without gestational diabetes mellitus in studies with no insulin use
Subgroup analysis according to country status, diagnostic criteria, screening method, and risk of bias for adverse outcomes of pregnancy in women with gestational diabetes mellitus compared with women without gestational diabetes mellitus in studies with insulin use
We applied meta-regression models to evaluate the modification power of the proportion of patients with insulin use when sufficient data were available. Significant associations were found between effect size estimate and proportion of patients who had received insulin for the adverse outcomes caesarean section (estimate=0.0068, P=0.04) and preterm delivery (estimate=−0.0069, P=0.04) (table S9).
In sensitivity analyses, most pooled estimates were not significantly different when a study was omitted, suggesting that no one study had a large effect on the pooled estimate. The pooled estimate effect became significant (P=0.005) for low birth weight when the study of Lu et al 99 was omitted, however (fig S5). We found evidence of a small study effect only for caesarean section (Egger’s P=0.01, table S10). Figure S6 shows the funnel plots of the included studies for various adverse outcomes (≥10 studies).
Principal findings
We have provided quantitative estimates for the associations between gestational diabetes mellitus and adverse outcomes of pregnancy after adjustment for confounding factors, through a systematic search and comprehensive meta-analysis. Compared with patients with normoglycaemia during pregnancy, patients with gestational diabetes mellitus had increased odds of caesarean section, preterm delivery, low one minute Apgar score, macrosomia, and an infant born large for gestational age in studies where insulin was not used. In studies with insulin use, patients with gestational diabetes mellitus had an increased odds of an infant born large for gestational age, or with respiratory distress syndrome or neonatal jaundice, or requiring admission to the neonatal intensive care unit. Our study was a comprehensive analysis, quantifying the adjusted associations between gestational diabetes mellitus and adverse outcomes of pregnancy. The study provides updated critical information on gestational diabetes mellitus and adverse outcomes of pregnancy and would facilitate counselling of women with gestational diabetes mellitus before delivery.
To examine the heterogeneity conferred by different severities of gestational diabetes mellitus, we categorised the studies by use of insulin. Insulin is considered the standard treatment for the management of gestational diabetes mellitus when adequate glucose levels are not achieved with nutrition and exercise. 179 Our meta-regression showed that the proportion of patients who had received insulin was significantly associated with the effect size estimate of adverse outcomes, including caesarean section (P=0.04) and preterm delivery (P=0.04). This finding might be the result of a positive linear association between glucose concentrations and adverse outcomes of pregnancy, as previously reported. 180 However, the proportion of patients who were receiving insulin indicates the percentage of patients with poor glycaemic control in the population and cannot reflect glycaemic control at the individual level.
Screening methods for gestational diabetes mellitus have changed over time, from the earliest selective screening (based on risk factors) to universal screening by the glucose challenge test or the oral glucose tolerance test, recommended by the US Preventive Services Task Force (2014) 181 and the American Diabetes Association (2020). 182 The diagnostic accuracy of these screening methods varied, contributing to heterogeneity in the analysis.
Several studies have tried to pool the effects of gestational diabetes mellitus on pregnancy outcomes, but most focused on one outcome, such as congenital malformations, 183 184 macrosomia, 185 186 or respiratory distress syndrome. 187 Our findings of increased odds of macrosomia in gestational diabetes mellitus in studies where insulin was not used, and respiratory distress syndrome in studies with insulin use, were similar to the results of previous meta-analyses. 188 189 The increased odds of neonatal respiratory distress syndrome, along with low Apgar scores, might be attributed to disruption of the integrity and composition of fetal pulmonary surfactant because gestational diabetes mellitus can delay the secretion of phosphatidylglycerol, an essential lipid component of surfactants. 190
Although we detected no significant association between gestational diabetes mellitus and mortality events, the observed increase in the odds of neonatal death (odds ratio 1.59 in studies that did not report the use of insulin) should be emphasised to obstetricians and pregnant women because its incidence was low (eg, 3.75% 87 ). The increased odds of neonatal death could result from several lethal complications, such as respiratory distress syndrome, neonatal hypoglycaemia (3.94-11.71-fold greater odds), and jaundice. These respiratory and metabolic disorders might increase the likelihood of admission to the neonatal intensive care unit.
For the maternal adverse outcomes, women with gestational diabetes mellitus had increased odds of pre-eclampsia, induction of labour, and caesarean section, consistent with findings in previous studies. 126 Our study identified a 1.24-1.46-fold greater odds of pre-eclampsia between patients with and without gestational diabetes mellitus, which was similar to previous results. 191
Strengths and limitations of the study
Our study included more studies than previous meta-analyses and covered a range of maternal and fetal outcomes, allowing more comprehensive comparisons among these outcomes based on the use of insulin and different subgroup analyses. The odds of adverse fetal outcomes, including respiratory distress syndrome (P=0.002), neonatal jaundice (P=0.05), and admission to the neonatal intensive care unit (P=0.005), were significantly increased in studies with insulin use, implicating their close relation with glycaemic control. The findings of this meta-analysis support the need for an improved understanding of the pathophysiology of gestational diabetes mellitus to inform the prediction of risk and for precautions to be taken to reduce adverse outcomes of pregnancy.
The study had some limitations. Firstly, adjustment for at least one confounder had limited power to deal with potential confounding effects. The set of adjustment factors was different across studies, however, and defining a broader set of multiple adjustment variables was difficult. This major concern should be looked at in future well designed prospective cohort studies, where important prognostic factors are controlled. Secondly, overt diabetes was not clearly defined until the IADPSG diagnostic criteria were proposed in 2010. Therefore, overt diabetes or pre-existing diabetes might have been included in the gestational diabetes mellitus groups if studies were conducted before 2010 or adopted earlier diagnostic criteria. Hence we cannot rule out that some adverse effects in newborns were related to prolonged maternal hyperglycaemia. Thirdly, we divided and analysed the subgroups based on insulin use because insulin is considered the standard treatment for the management of gestational diabetes mellitus and can reflect the level of glycaemic control. Accurately determining the degree of diabetic control in patients with gestational diabetes mellitus was difficult, however. Finally, a few pregnancy outcomes were not accurately defined in studies included in our analysis. Stillbirth, for example, was defined as death after the 20th or 28th week of pregnancy, based on different criteria, but some studies did not clearly state the definition of stillbirth used in their methods. Therefore, we considered stillbirth as an outcome based on the clinical diagnosis in the studies, which might have caused potential bias in the analysis.
Conclusions
We performed a meta-analysis of the association between gestational diabetes mellitus and adverse outcomes of pregnancy in more than seven million women. Gestational diabetes mellitus was significantly associated with a range of pregnancy complications when adjusted for confounders. Our findings contribute to a more comprehensive understanding of adverse outcomes of pregnancy related to gestational diabetes mellitus. Future primary studies should routinely consider adjusting for a more complete set of prognostic factors.
What is already known on this topic
The incidence of gestational diabetes mellitus is gradually increasing and is associated with a range of complications for the mother and fetus or neonate
Pregnancy outcomes in gestational diabetes mellitus, such as neonatal death and low Apgar score, have not been considered in large cohort studies
Comprehensive systematic reviews and meta-analyses assessing the association between gestational diabetes mellitus and adverse pregnancy outcomes are lacking
What this study adds
This systematic review and meta-analysis showed that in studies where insulin was not used, when adjusted for confounders, women with gestational diabetes mellitus had increased odds of caesarean delivery, preterm delivery, low one minute Apgar score, macrosomia, and an infant large for gestational age in the pregnancy outcomes
In studies with insulin use, when adjusted for confounders, women with gestational diabetes mellitus had increased odds of an infant large for gestational age, or with respiratory distress syndrome or neonatal jaundice, or requiring admission to the neonatal intensive care unit
Future primary studies should routinely consider adjusting for a more complete set of prognostic factors
Ethics statements
Ethical approval.
Not required.
Data availability statement
Table S11 provides details of adjustment for core confounders. Supplementary data files contain all of the raw tabulated data for the systematic review (table S12). Tables S13-15 provide the raw data and R language codes used for the meta-analysis.
Contributors: WY and FL developed the initial idea for the study, designed the scope, planned the methodological approach, wrote the computer code and performed the meta-analysis. WY and CL coordinated the systematic review process, wrote the systematic review protocol, completed the PROSPERO registration, and extracted the data for further analysis. ZL coordinated the systematic review update. WY, JH, and FL defined the search strings, executed the search, exported the results, and removed duplicate records. WY, CL, ZL, and FL screened the abstracts and texts for the systematic review, extracted relevant data from the systematic review articles, and performed quality assessment. WY, ZL, and FL wrote the first draft of the manuscript and all authors contributed to critically revising the manuscript. ZL and FL are the study guarantors. ZL and FL are senior and corresponding authors who contributed equally to this study. All authors had full access to all the data in the study, and the corresponding authors had final responsibility for the decision to submit for publication. The corresponding author attests that all listed authors meet authorship criteria and that no others meeting the criteria have been omitted.
Funding: The research was funded by the National Natural Science Foundation of China (grants 82001223 and 81901401), and the Natural Science Foundation for Young Scientist of Hunan Province, China (grant 2019JJ50952). The funders had no role in considering the study design or in the collection, analysis, interpretation of data, writing of the report, or decision to submit the article for publication.
Competing interests: All authors have completed the ICMJE uniform disclosure form at www.icmje.org/disclosure-of-interest/ and declare: support from the National Natural Science Foundation of China and the Natural Science Foundation for Young Scientist of Hunan Province, China for the submitted work; no financial relationships with any organisations that might have an interest in the submitted work in the previous three years; no other relationships or activities that could appear to have influenced the submitted work.
The lead author (the manuscript’s guarantor) affirms that the manuscript is an honest, accurate, and transparent account of the study being reported; that no important aspects of the study have been omitted; and that any discrepancies from the study as planned (and, if relevant, registered) have been explained.
Dissemination to participants and related patient and public communities: The dissemination plan targets a wide audience, including members of the public, patients, patient and public communities, health professionals, and experts in the specialty through various channels: written communication, events and conferences, networks, and social media.
Provenance and peer review: Not commissioned; externally peer reviewed.
This is an Open Access article distributed in accordance with the Creative Commons Attribution Non Commercial (CC BY-NC 4.0) license, which permits others to distribute, remix, adapt, build upon this work non-commercially, and license their derivative works on different terms, provided the original work is properly cited and the use is non-commercial. See: http://creativecommons.org/licenses/by-nc/4.0/ .
- Saravanan P ,
- Diabetes in Pregnancy Working Group ,
- Maternal Medicine Clinical Study Group ,
- Royal College of Obstetricians and Gynaecologists, UK
- O’Sullivan JB ,
- Hartling L ,
- Dryden DM ,
- Guthrie A ,
- Vandermeer B ,
- McIntyre HD ,
- Catalano P ,
- Mathiesen ER ,
- Metzger BE ,
- HAPO Study Cooperative Research Group
- Persson M ,
- Balsells M ,
- García-Patterson A ,
- Simmonds M ,
- Murphy HR ,
- Roland JM ,
- East Anglia Study Group for Improving Pregnancy Outcomes in Women with Diabetes (EASIPOD)
- Magnuson A ,
- Simmons D ,
- Sumeksri P ,
- Wongyai S ,
- González González NL ,
- Bellart J ,
- Guillén MA ,
- Herranz L ,
- Barquiel B ,
- Hillman N ,
- Burgos MA ,
- Pallardo LF
- Higgins JP ,
- Thompson SG ,
- Davey Smith G ,
- Schneider M ,
- Sweeting MJ ,
- Sutton AJ ,
- Hartung J ,
- IntHout J ,
- Ioannidis JP ,
- Alberico S ,
- Montico M ,
- Barresi V ,
- Multicentre Study Group on Mode of Delivery in Friuli Venezia Giulia
- Alfadhli EM ,
- Anderberg E ,
- Ardawi MS ,
- Nasrat HA ,
- Al-Sagaaf HM ,
- Kenealy T ,
- Barakat MN ,
- Youssef RM ,
- Al-Lawati JA
- Ibrahim I ,
- Eltaher F ,
- Benhalima K ,
- Hanssens M ,
- Devlieger R ,
- Verhaeghe J ,
- Berggren EK ,
- Boggess KA ,
- Stuebe AM ,
- Jonsson Funk M
- Korucuoglu U ,
- Aksakal N ,
- Himmetoglu O
- Bodmer-Roy S ,
- Cousineau J ,
- Broekman BFP ,
- GUSTO study group
- Catalano PM ,
- Cruickshank JK ,
- Chanprapaph P ,
- Cheung NW ,
- Lopez-Rodo V ,
- Rodriguez-Vaca D ,
- Benchimol M ,
- Carbillon L ,
- Benbara A ,
- Pharisien I ,
- Scifres CM ,
- Gorban de Lapertosa S ,
- Salzberg S ,
- DPSG-SAD Group
- Zijlmans AB ,
- Rademaker D ,
- Djelmis J ,
- Mulliqi Kotori V ,
- Pavlić Renar I ,
- Ivanisevic M ,
- Oreskovic S
- Domanski G ,
- Ittermann T ,
- Donovan LE ,
- Edwards AL ,
- Torrejón MJ ,
- Ekeroma AJ ,
- Chandran GS ,
- McCowan L ,
- Eagleton C ,
- Erjavec K ,
- Poljičanin T ,
- Matijević R
- Ethridge JK Jr . ,
- Feleke BE ,
- Feleke TE ,
- Forsbach G ,
- Cantú-Diaz C ,
- Vázquez-Lara J ,
- Villanueva-Cuellar MA ,
- Alvarez y García C ,
- Rodríguez-Ramírez E
- Gonçalves E ,
- Gortazar L ,
- Flores-Le Roux JA ,
- Benaiges D ,
- Gruendhammer M ,
- Brezinka C ,
- Lechleitner M
- Hedderson MM ,
- Ferrara A ,
- Hillier TA ,
- Pedula KL ,
- Morris JM ,
- Hossein-Nezhad A ,
- Maghbooli Z ,
- Vassigh AR ,
- Massaro N ,
- Streckeisen S ,
- Ikenoue S ,
- Miyakoshi K ,
- Raghav SK ,
- Jensen DM ,
- Sørensen B ,
- Rich-Edwards JW ,
- Kreisman S ,
- Tildesley H
- Kachhwaha CP ,
- Kautzky-Willer A ,
- Bancher-Todesca D ,
- Weitgasser R ,
- Keikkala E ,
- Mustaniemi S ,
- Koivunen S ,
- Keshavarz M ,
- Babaee GR ,
- Moghadam HK ,
- Kgosidialwa O ,
- Carmody L ,
- Gunning P ,
- Kieffer EC ,
- Carman WJ ,
- Sanborn CZ ,
- Viljakainen M ,
- Männistö T ,
- Kachhawa G ,
- Laafira A ,
- Griffin CJ ,
- Johnson JA ,
- Lapolla A ,
- Dalfrà MG ,
- Ragazzi E ,
- De Cata AP ,
- Norwitz E ,
- Leybovitz-Haleluya N ,
- Wainstock T ,
- Hinkle SN ,
- Grantz KL ,
- Lopez-de-Andres A ,
- Carrasco-Garrido P ,
- Gil-de-Miguel A ,
- Hernandez-Barrera V ,
- Jiménez-García R
- Luengmettakul J ,
- Sunsaneevithayakul P ,
- Talungchit P
- Macaulay S ,
- Munthali RJ ,
- Dunger DB ,
- Makwana M ,
- Bhimwal RK ,
- El Mallah KO ,
- Kulaylat NA ,
- Melamed N ,
- Vandenberghe H ,
- Jensen RC ,
- Kibusi SM ,
- Munyogwa MJ ,
- Patient C ,
- Miailhe G ,
- Legardeur H ,
- Mandelbrot L
- Minsart AF ,
- N’guyen TS ,
- Ratsimandresy R ,
- Ali Hadji R
- Matsumoto T ,
- Morikawa M ,
- Sugiyama T ,
- Knights SJ ,
- Olayemi OO ,
- Mwanri AW ,
- Ramaiya K ,
- Abalkhail B ,
- Nguyen TH ,
- Nguyen CL ,
- Minh Pham N ,
- Nicolosi BF ,
- Vernini JM ,
- Kragelund Nielsen K ,
- Andersen GS ,
- Nybo Andersen AM
- Ogonowski J ,
- Miazgowski T ,
- Czeszyńska MB ,
- Kuczyńska M ,
- Miazgowski T
- Morrish DW ,
- O’Sullivan EP ,
- O’Reilly M ,
- Dennedy MC ,
- Gaffney G ,
- Atlantic DIP collaborators
- Ovesen PG ,
- Rasmussen S ,
- Kesmodel US
- Ozumba BC ,
- Premuzic V ,
- Zovak Pavic A ,
- Bevanda M ,
- Mihaljevic S ,
- Ramachandran A ,
- Snehalatha C ,
- Clementina M ,
- Sasikala R ,
- Redman LM ,
- LIFE-Moms Research Group
- Spanish Group for the Study of the Impact of Carpenter and Coustan GDM thresholds
- Bowker SL ,
- Montoro MN ,
- Lawrence JM
- Kamalanathan S ,
- Saldana TM ,
- Siega-Riz AM ,
- Savitz DA ,
- Thorp JM Jr .
- Savona-Ventura C ,
- Schwartz ML ,
- Lubarsky SL
- Segregur J ,
- Buković D ,
- Milinović D ,
- Cavkaytar S ,
- Shahbazian H ,
- Nouhjah S ,
- Shahbazian N ,
- McElduff A ,
- Sheffield JS ,
- Butler-Koster EL ,
- McIntire DD ,
- Saigusa Y ,
- Nakanishi S ,
- Templeton A ,
- Sirimarco MP ,
- Guerra HM ,
- Lisboa EG ,
- Sletner L ,
- Yajnik CS ,
- Soliman A ,
- Al Rifai H ,
- Soonthornpun S ,
- Soonthornpun K ,
- Aksonteing J ,
- Thamprasit A
- Srichumchit S ,
- Sugiyama MS ,
- Roseveare C ,
- Basilius K ,
- Madraisau S
- Hansen BB ,
- Mølsted-Pedersen L
- Caswell A ,
- Holliday E ,
- Petrović O ,
- Crnčević Orlić Ž ,
- Vambergue A ,
- Nuttens MC ,
- Goeusse P ,
- Biausque S ,
- van Hoorn J ,
- von Katterfeld B ,
- McNamara B ,
- Langridge AT
- Wahabi HA ,
- Esmaeil SA ,
- Alzeidan RA
- Esmaeil S ,
- Mamdouh H ,
- Wahlberg J ,
- Nyström L ,
- Persson B ,
- Arnqvist HJ
- Nankervis A ,
- Weijers RN ,
- Bekedam DJ ,
- Smulders YM
- Bleicher K ,
- Saunders LD ,
- Demianczuk NN
- Homer CSE ,
- Sullivan EA
- American Diabetes Association
- U.S. Preventive Services Task Force
- Tabrizi R ,
- Lankarani KB ,
- Leung-Pineda V ,
- Gronowski AM
- Bryson CL ,
- Ioannou GN ,
- Rulyak SJ ,
- Critchlow C
REVIEW article
Gestational diabetes mellitus—innovative approach to prediction, diagnosis, management, and prevention of future ncd—mother and offspring.
- 1 Mater Research, The University of Queensland, South Brisbane, QLD, Australia
- 2 World Diabetes Foundation, Bagsvaerd, Denmark
- 3 Divakars Specialty Hospital, Bengaluru, India
- 4 Mor Women’s Health Care Center, Tel Aviv, Israel
Gestational diabetes mellitus (GDM) is the commonest medical complication of pregnancy. The association of GDM with immediate pregnancy complications including excess fetal growth and adiposity with subsequent risk of birth trauma and with hypertensive disorders of pregnancy is well recognized. However, the associations with wide ranges of longer-term health outcomes for mother and baby, including the lifetime risks of obesity, pre-diabetes, and diabetes and cardiovascular disease have received less attention and few health systems address these important issues in a systematic way. This article reviews historical and recent data regarding prediction of GDM using demographic, clinical, and biochemical parameters. We evaluate current and potential future diagnostic approaches designed to most effectively identify GDM and extend this analysis into a critical evaluation of lifestyle and nutritional/pharmacologic interventions designed to prevent the development of GDM. The general approach to management of GDM during pregnancy is then discussed and the major final focus of the article revolves around the importance of a GDM diagnosis as a future marker of the risk of non-communicable disease (NCD), in particular pre-diabetes, diabetes, and cardiovascular disease, both in mother and offspring.
On a worldwide basis, both Hyperglycemia in Pregnancy (HIP) and obesity among women of childbearing age are increasing to epidemic proportions ( 1 , 2 ). For our current report, we are following the diagnostic framework for HIP as recommended by the International Federation of Gynecology and Obstetrics (FIGO) ( 3 ) which considers any degree of glucose elevation in pregnancy as part of the umbrella definition of HIP. This broad group is then further divided into those women with either known pre-pregnancy diabetes or markedly elevated glucose levels which would qualify for a diagnosis of diabetes outside pregnancy. This condition is termed “Diabetes in Pregnancy” (DIP). The far larger group of women with elevated glucose levels below these thresholds is classified as “Gestational Diabetes Mellitus” (GDM). Many reports have attempted to dissect the relative importance of HIP and overweight/obesity relating both to pregnancy complications and longer term health of both GDM mothers and their children. Clear determination of causality is challenging, GDM and overweight/obesity frequently affect the same people, and causality is bidirectional—obesity lies on the causal pathway toward hyperglycemia and HIP is causally related to obesity in the offspring. Also, hyperglycemia (pre-diabetes or diabetes) may well exist before pregnancy as demonstrated by large surveys such as NHANES ( 4 , 5 ). However, it is generally asymptomatic at this point and may only be detected when (and if) systematic testing occurs in the pregnancy context.
The Hyperglycemia and Adverse Pregnancy Outcome (HAPO) study ( 6 , 7 ), demonstrated that both maternal BMI and hyperglycemia share similar associations with complications of pregnancy. Both were associated with increased rates of excess fetal growth, primary cesarean birth, clinical neonatal hypoglycemia and fetal adiposity, neonatal hyperinsulinemia, and hypertensive disorder of pregnancy. The association of hyperglycemia with adverse outcomes is generally linear, while that of BMI follows a quadratic pattern with decreasing increments in the highest BMI categories ( 8 ).
HAPO also reported BMI and GDM considered together with pregnancy complications ( 6 ). In HAPO, obesity was present in 13.7% and GDM by IADPSG criteria ( 9 ) in 16.1% of those who remained blinded. Obesity was present in 25% of GDM women but prevalence varied greatly between centers. Compared to women with neither factor, adverse outcomes were increased on both groups. Pre-eclampsia was more frequent in the “obesity/non GDM group”, while excess fetal growth and fetal hyperinsulinemia were slightly more common in the “GDM/no obesity group” than in the “obesity/non GDM group”. The combination of both factors was accompanied by an additive increase in pregnancy complications.
Thus, both maternal BMI and glycemia contribute independently and additively to suboptimal pregnancy outcomes. While not ignoring obesity as a major health problem, our current review will focus primarily on the prediction and diagnosis of GDM and outline optimal management both during and following pregnancy.
Prediction of GDM
As noted above, many women currently considered as “GDM” may actually have undiagnosed hyperglycemia before pregnancy. In high prevalence countries, ideally screening for hyperglycemia would happen as part of well-organized and well- resourced preconception care. However, this approach has limitations as only around 40% of pregnancies worldwide are “intended” ( 10 ). Lack of preconception testing means that we cannot strictly assert that testing in early pregnancy is “predicting” GDM. However, early testing does represent an opportunity to recognize some women as having likely pre-existing abnormalities of glucose metabolism. In India, for example, it has been reported that over 70% of GDM women can be identified at their first antenatal visit ( 11 ).
Further, it may be possible, by using clinical characteristics and biochemical tests, to identify a separate group of pregnant women whose glucose levels are in the normal range in early gestation, but who carry a high risk of progression to “standard GDM”, which is generally diagnosed at around 24 to 28 weeks’ gestation. In pragmatic terms, it appears reasonable to consider women with pre-pregnancy hyperglycemia, those with early GDM and those at high risk of GDM as target groups for early intervention.
GDM is frequently an antecedent of later Type 2 diabetes and a marker for (premature) cardiovascular (CV) disease in women. In addition to their sine qua non of hyperglycemia, GDM and Type 2 diabetes share a range of underlying processes including insulin resistance, chronic metabolic inflammation, changes in adipocytokines, and alterations in many areas of metabolism ( 12 , 13 ).
The simplest models for prediction of GDM involve the use of single or multiple clinical characteristics to stratify GDM risk. The performance of these models has recently been evaluated by van Hoorn et al. ( 14 ), who concluded that models which included both multiple clinical characteristics and early pregnancy glucose measurements performed best in prediction. Recently, machine learning or artificial intelligence methods using demographic variables and previous laboratory results have been applied to improve predictive power ( 15 ).
GDM is also associated with abnormalities of placentation and early pregnancy markers commonly used in aneuploidy prediction such as pregnancy associated plasma protein A (PAPP-A) and free β HCG have also been incorporated into predictive models ( 16 ). Sweeting et al., using stored serum samples from a first trimester screening program in Sydney, have reported that use of multiple biochemical markers in combination with clinical features, is able to predict GDM with high accuracy [area under receiver operator curve (AUROC) 0.91–0.93]. However, these findings have yet to be validated in independent cohorts.
Proteomic screening in early pregnancy has revealed multiple potential protein markers, including a cluster associated with insulin secretion, binding, resistance, and signaling for later GDM ( 17 , 18 ). Ravnsborg et al. have reported that vitronectin, which is also associated with metabolic syndrome outside pregnancy, significantly augments the predictive power of maternal risk factors ( 17 ) and may prove to be a valuable predictor in clinical use. However, proteomic methods are too complex and expensive for routine use and must progress to automated, low cost laboratory tests before they are widely applicable.
Recently, the role of extracellular vesicles (ECVs) as GDM markers has been explored ( 19 , 20 ). These circulating particles, derived in pregnancy primarily from placenta and adipose tissue, “package” multiple potential protein and RNA molecules and transport them to specific sites. James-Allan et al. ( 21 ) have demonstrated that specific small ECVs are associated with GDM and that infusion of human ECVs from GDM women produces both insulin resistance and reduced insulin secretion in rodents, reminiscent of the pathophysiology of GDM.
Micro RNAs are a major component in ECVs and are associated with glucose metabolism. An exploratory case-control study by Yoffe et al. suggested that micro RNA-223 and micro RNA 23a in first trimester blood samples were strongly predictive of later GDM (AUROC 0.91) ( 22 ). Another recent cohort study has confirmed this finding for micro RNA-233 ( 23 ). These results are promising and the overall associations between non coding RNAs and GDM have recently been reviewed in detail ( 24 ). However, as is the case for other biomarkers, these positive findings from small studies need to be validated in independent cohorts. The required assays will also need to be modified to allow low cost, high throughput use in routine diagnostic laboratories.
In summary, cohort studies have revealed multiple potential early pregnancy predictors of later GDM ( 13 ). These range from single or multiple clinical or demographic measures to including early pregnancy glycemic measurement and extending to measurement of complex network of molecular biomarkers. To be valuable for routine clinical practice, molecular biomarkers need both to perform better than clinical risk factors and simple glucose measurements in predicting GDM and pregnancy outcomes and to demonstrate cost-effectiveness. In practical terms, they should also be suited to non-fasting testing at the same time as other routine early pregnancy health screening tests. While many biomarkers have a strong association with later GDM, none have yet been sufficiently developed as automated and low-cost assays to allow their routine clinical use. However, they offer valuable insights into the pathophysiology of GDM and may, in time, be ready for the clinic.
Diagnosis of GDM
GDM is generally diagnosed with an oral glucose tolerance test (OGTT) administered at 24–28 weeks’ gestation. This timing has generally been preferred for routine GDM diagnosis as most of the physiologic insulin resistance of pregnancy will be well established. However, with globally increasing levels of obesity, rising maternal age and other environmental risk factors this assumption may no longer be valid as evidenced by high GDM detection rates in early pregnancy witnessed in recent studies from different parts of the world. The rising prevalence of undiagnosed dysglycaemia (diabetes and pre-diabetes) in reproductive age women enhances the need to rule out pre-existing undiagnosed diabetes at the earliest possible moment, thus bringing into question the old norm of testing between 24 and 28 weeks. The exact process and criteria for OGTT diagnosis of GDM vary widely across the world. The International Association of Diabetes in Pregnancy Study Groups (IADPSG) ( 9 ), World Health Organization (WHO) ( 2 , 25 ) and FIGO ( 3 ) have all endorsed “one step” OGTT testing, using thresholds ≥ 5.1 mmol/L fasting; 10.0 mmol/L at 1 h and 8.5 mmol/L at 2 h following a 75 gram glucose load for diagnosis of GDM. However, the FIGO pragmatic recommendations, recognizing differing health care contexts, allow for alternative diagnostic approaches for China, India, South America, and the United Kingdom ( 3 ). There is a major variance in diagnostic approach in the USA ( 26 ) and Canada ( 27 ) which generally prefer two step testing using a non-fasting, 1 h “glucose challenge” test (GCT), followed by OGTT (100 gram or 75 gram) if the GCT result falls above predefined thresholds. IADPSG, WHO, and FIGO have all also endorsed the need for early testing as well as testing in the traditional 24 to 28 week window.
A further major variation in worldwide testing protocols for GDM is the ongoing debate regarding whether testing should be universal (for all pregnant women) or targeted only toward women with identified risk factors which are associated with a higher risk of a positive test. FIGO ( 3 ), the IADPSG ( 9 ) and the American College of Obstetricians and Gynecologists (ACOG) ( 26 ) all recommend universal testing. The HAPO study ( 7 ) clearly demonstrated that OGTT glucose values are independently associated with adverse pregnancy outcomes, even after correction for multiple additional maternal characteristics including BMI. GDM is almost uniformly asymptomatic, so a testing strategy based on symptoms is clearly untenable. A cost utility analysis for the United Kingdom (UK) concluded that if the population frequency of GDM is >4.2%, then universal OGTT is the most cost effective strategy ( 28 ). No current credible estimates of GDM frequency fall below this threshold. Nonetheless, some authorities, notably the National Institute for Clinical Excellence (NICE) in the UK, still promote risk factor based screening ( 29 ). The most recent Cochrane review ( 30 ) is inconclusive, but a recent systematic review of economic evaluations of GDM screening again concluded that universal screening was the most effective approach ( 31 ). Compliance with officially endorsed risk factor based screening protocols appears to be poor in countries as diverse as Sweden ( 32 ), where only 31% of women received the screening test deemed appropriate for their documented risk factor profile, the UK (61% appropriate screening according to risk factors) ( 33 ) and South Africa ( 34 ), where Adam et al. reported that, although use of risk factors would reduce OGTTs by 46%, this protocol would miss 41% of GDM diagnoses ( 34 ). A further study from Italy reported that 23% of GDM cases would be missed by risk factor based screening ( 34 ). By contrast, a report from Sri Lanka, a country with a high background frequency of diabetes, noted that 80% of women would still require screening if risk factors were used to determine the need for OGTT, but only 13% of GDM cases would be missed ( 35 ). Thus, the effects of implementing risk factor–based screening differ between various populations. However, given that most countries have an increasing prevalence of both GDM and associated risk factors and that correct implementation of risk factor based screening is poor, we favor uniform biochemical testing.
Clearly, the glucose tolerance test is inconvenient, resource intensive and also quite poorly reproducible ( 36 ) and an alternative cheap, reproducible, non-fasting test would be preferable. Self-administered home OGTTs offer increased convenience and appear to perform as well as laboratory testing ( 37 ). For fasting glucose testing, specific meters with tight laboratory based quality control may also provide acceptable accuracy ( 38 ) and this approach has been endorsed by FIGO for use in low resource settings ( 3 ).
Glycosylated haemoglobin (HbA1c) is an obvious alternative and is widely used for diagnosis of diabetes outside pregnancy. However, it performs poorly both in prediction of OGTT diagnosed GDM and in prediction of pregnancy outcomes ( 39 ) and appears to be of limited value except in early pregnancy detection of undiagnosed hyperglycemia ( 40 ).
A variety of other markers of overall glycemia with shorter half-lives (thus more reflective of short term glycemia changes) including fructosamine (FA), glycated albumin (GA) and 1.5 anhydroglucitrol (1.5 AHG) have been evaluated ( 41 ). None of these markers are optimally suited to use in pregnancy. FA is easily measured but is affected by the dilutional anaemia of pregnancy. FA and GA vary in the presence of albuminuria (as in pre-eclampsia) and 1.5 AHG is inaccurate in pregnancy due to reduced renal threshold for glycosuria ( 41 ). More recently, the glycated complement fraction GCD59 ( 42 ) has been shown in retrospective studies to be associated with early GDM and with large for gestational age (LGA) infants ( 43 ) in an obese pregnant population. Larger prospective evaluations are now underway ( 44 ). GCD59 is currently the most promising, non-fasting marker of overall glycemia in the context of pregnancy.
Prevention of GDM—Before and During Pregnancy
Ideally, GDM rates in the population could be reduced by both individual and societal measures designed to promote healthy lifestyle changes including optimal dietary intake and increased physical activity in the general population with a focus on health and fitness of women of reproductive age. Maternal age at conception is an important marker of pregnancy complications including GDM ( 45 ), but is strongly influenced both by individual choices and societal factors and unlikely to be a useful target for preventative measures. Maternal overweight and obesity are also very important risks that need to be addressed pre-pregnancy through lifestyle measures ( 45 ). For marked obesity, bariatric surgery is the best proven means of reducing body weight. Although a full consideration of its effects on pregnancy are beyond the scope of this article, a recent review ( 46 ) showed benefits reduced GDM prevalence, lower rates of LGA infants and reduced prevalence of pregnancy hypertension. However, there was an increase in impaired fetal growth reflected by higher rates of SGA infants. Preterm deliveries were also more frequent. Surgical treatments which cause malabsorption are followed by greater weight reduction and demonstrate lower rates of LGA, but conversely also more SGA, suggesting that clinical decisions must balance potential risks and benefits.
The most recent overview of multiple GDM prevention strategies from the Cochrane group ( 47 ) reports that no single lifestyle or medication-based intervention is of proven benefit and that the overall evidence base is weak, but we shall consider some widely discussed potential options in more detail.
Lifestyle Interventions
Despite the negative Cochrane findings outlined above, which specifically concluded that diet alone or exercise alone had no effect in preventing GDM and that combined diet and exercise had only a “possible” (non-significant) effect in preventing GDM, one systematic review by Song et al. ( 48 ) has suggested that lifestyle intervention before the 15 th gestational week may reduce GDM by 20% [RR 0.80 (95% 9I 0.66–0.97)]. This provides glimmer of hope for lifestyle based interventions, but the weight of evidence is negative for any lifestyle intervention after the first trimester.
Metformin is commonly used in early pregnancy for patients with polycystic ovarian syndrome. It is also used in the second and third trimester as drug treatment for GDM if lifestyle modifications fail to achieve glycemic goals. A recent review has summarized many aspects of metformin use in pregnancy ( 49 ). However, current evidence does not support metformin as a preventative option for GDM. The (EMPOWaR) study randomised 449 obese women with normal baseline glucose tolerance to the addition of metformin of up to 2,500 g per day vs. placebo between 12- and 16-week gestation and continued until delivery of the infant ( 50 ). EMPOWaR noted no difference GDM prevalence, maternal weight gain or maternal lipid metabolism. Birth weight and birthweight SD (Z) score were comparable between the groups. Metformin was associated with more reported diarrhoea (42% vs 19%, P < 0.0001).
Syngelaki et al. randomized women with BMI > 35 kg/m 2 to metformin 3 g per day or placebo from 12- to 18-week gestation until delivery ( 51 ). A total of 202 women on metformin and 198 on placebo completed the study. There was no difference in fetal growth. Maternal GWG was reduced by 1.7 kg (P < 0.001) with metformin. GDM rates were similar between groups and other pregnancy outcomes were also similar.
The GRoW RCT of metformin for overweight and obese women from early pregnancy, conducted by Dodds et al., has also reported negative results, with no reduction in GDM or other pregnancy complications ( 52 ).
Myoinositol
Myoinositol enhances insulin sensitivity outside pregnancy. In GDM, a trial of supplementation of 69 women randomised to myoinositol 4 g/day with folic acid 400mcg daily compared to folic acid alone showed reduced insulin resistance in the myoinositol group ( 53 ). Another study compared myoinositol 2 g with 200 mcg folic acid daily vs. 200 mcg folic acid daily alone in 220 obese women starting at 12- to 13-week gestation and continued throughout pregnancy ( 54 ). GDM prevalence was reportedly reduced from 33.6% to 14% (P = 0.001), in the myoinositol group.
However, another RCT which recruited women with family history of diabetes and compared women randomised to 1,100 mg myoinositol, 27.6 mg D-chiro-inositol plus 400 mcg folic acid, or to 400 mcg folic acid only, from early pregnancy until 24- to 28-week gestation demonstrated no reduction in GDM frequency ( 55 ).
Resveratrol was compared to inositol in another RCT involving overweight pregnant women. The three arms of the study included resveratrol plus inositol, inositol alone and placebo ( 56 ). Resveratrol was associated with reduced lipid and glucose measures. The NiPPeR study plans to compare twice-daily intake of a control nutritional drink, enriched with standard micronutrients, to a twice-daily nutritional drink enriched with additional micronutrients, myoinositol, and probiotics. Results are not yet available ( 57 ).
Probiotics were found reduce GDM in normal weight women in a study from Finland ( 58 ), a result which stimulated great interest in their use as a low risk therapeutic agent in GDM prevention. However, results in women at higher risk due to pre-pregnancy obesity have been less promising. The Probiotics in Pregnancy Study randomised 175 obese women to probiotic vs. placebo capsule for a four week period from 24 until 28 weeks gestation, with the primary outcomes being change in fasting glucose. Treatment groups differed in baseline BMI. After adjustment for BMI, there were no discernible clinical benefits in the major outcome of fasting glucose. Infant birthweight and other metabolic parameters did not improve ( 59 ). More recently, the SPRING study ( 60 ), HUMBA study ( 61 ) and studies from Finland ( 62 ) and Denmark ( 63 ) have reported negative results for probiotic supplementation, so the weight of current evidence is certainly negative.
Dietary fatty acid has also been suggested as a therapy which might reduce GDM and also improve the rates of preterm delivery. The DHA to optimize mother infant outcome (DOMInO) RCT included 2399 women who were randomized before 21-week gestation to either (1) DHA enriched fish oil 800 mg/day or (2) vegetable oil capsules without DHA until the time of birth ( 64 ). GDM and preeclampsia were not reduced and there were no differences in neonatal size or adiposity. Subsequent assessment of the offspring at Age 7 years showed no differences in anthropometry ( 65 ).
Low serum 25 hydroxy vitamin D levels are clearly a risk factor for development of GDM ( 66 ), but the results of therapeutic trials have been mixed. The most recent Cochrane review ( 67 ), including primarily studies from the Middle East, reported that supplementation with Vitamin D alone “probably” reduces the population frequency of GDM [RR 0.51 (95% CI 0.27–0.97)] and pre-eclampsia [RR 0.48 (95% CI 0.30–0.79)]. However, no benefit was noted for Vitamin D + calcium or Vitamin D + calcium + other minerals. The overall quality of available evidence has been considered “low” by the Cochrane Group in their overview of GDM prevention studies ( 47 ). Noting the overall divergence in populations studied, baseline Vitamin D levels and therapeutic Vitamin D doses used in the studies included in/excluded from various reviews, we consider the following conclusion from Corcoy et al. ( 68 ) as a balanced summary: “High dose vitamin D supplementation during pregnancy halves the rate of GDM in pregnant women with baseline Vitamin D < 50 nmol/L”. Toxicity from Vitamin D supplementation up to 5,000 IU/day appears low ( 69 , 70 ). Therefore, in practical terms, Vitamin D therefore appears to be a reasonable option in populations with low baseline levels. Hopefully, future studies will clarify its true therapeutic role.
Management of GDM—During Pregnancy and Post-Partum
Treatment of GDM during pregnancy, centers on dietary modulation, promotion of healthy physical activity and pharmacologic management, primarily with insulin as well as oral hypoglycemic agents (OHA), if glycemic control cannot be achieved with lifestyle measures alone. The details of the therapeutic approach, in particular regarding various dietary approaches and the potential use of OHAs such as metformin and glyburide (glibenclamide) differ widely between and within countries and detailed discussion of these ongoing issues of contention is beyond the scope of our current review, but has recently been covered in detail ( 71 ).
Two landmark prospective RCTs have confirmed that detection of and medical therapy for GDM carries benefits for both mother and baby in terms of immediate pregnancy outcomes ( 72 , 73 ). Women in the intervention arm of the Australian (Crowther) study showed lower rates of fetal macrosomia, reduced frequency of LGA and reduced preeclampsia ( 72 ). In the US (Landon) study, women who received treatment for GDM demonstrated lower GWG and lower rates of pre-eclampsia. Reduced frequency of LGA and macrosomia were noted in infants of treated mothers.
Prevention of Future NCD—Mother and Offspring
Apart from its short term immediate associations with adverse perinatal outcomes, antecedent GDM is the strongest available historical marker for future Type 2 diabetes. GDM women carry an almost tenfold increased risk of progressing to later diagnosis of type 2 diabetes [RR 9.51 (95% CI 7.14–12.67)] ( 74 ). Women with GDM are also at heightened risk of CV disease ( 75 ). This increased risk exists even in those women who do not progress to overt Type 2 diabetes themselves. In the overall population of women with Type 2 diabetes, those with antecedent GDM also appear to carry higher risks than those without such a history ( 76 ). Compared with those who did not have GDM, women with GDM had a twofold higher risk of future CV events [RR 1.98 (95% CI 1.57–2.50)].
Meta-regression analysis demonstrates that this relationship is independent of Type 2 diabetes incidence across various studies (P = 0.34). Even when considering only those women who did progress to overt Type 2 diabetes, GDM was associated with an elevated chance of suffering future CV events [RR 1.56 (95% CI 1.04–2.32)]. Much of this risk occurs early in the life course post pregnancy, with a 2.3-fold increased noted for CV events in the first decade post-partum [RR 2.31 (95% CI 1.57–3.39)] ( 77 ).
The therapeutic opportunity for delaying/preventing type 2 diabetes through post-partum lifestyle modifications and possible use of pharmacotherapy in women identified as being “at risk” due to prior GDM ( 78 ) has been conclusively shown. A German study reported that breastfeeding (BF) was associated with a > 40% reduction in diabetes also appeared to delay the onset of T2DM by a further 10 years. These effects were not dependent on known high risk maternal characteristics such as baseline obesity or a need for insulin treatment during the index pregnancy. The greatest reduction in later diabetes risk was seen in women who breast fed for >3 months ( 79 ). A systematic review and meta-analysis of the association of BF with the post-partum risk of progression from GDM to overt type 2 diabetes reported reduced risks for both pre-diabetes and T2DM. Pre-DM was substantially reduced (OR = 0.66; 95% CI, 0.51–0.86 and T2DM was also less frequent (OR = 0.79; 95% CI, 0.68–0.92). Positive effects were noted among women with longer duration of any BF following a GDM pregnancy. These were even more evident with longer follow-up. Compared with women with shorter duration of BF, longer BF duration was associated with improvement in glucometabolic parameters, including lower fasting glucose and enhanced insulin sensitivity, lower BMI at follow up improved lipid metabolism as demonstrated by lower triglyceride levels. Thus, BF is potentially of great value as a low cost preventative measure in preventing both T2DM and related metabolic derangements in women with a history of GDM, in addition to its other well recognized benefits ( 80 ). Evidence for prevention of CV disease in women with history of GDM through post-partum interventions is currently lacking; but logically breast feeding and improved post-partum lifestyle would be predicted to reduce the risk significantly.
Excessive increase in maternal weight from one pregnancy to the next is often associated with a failure to return to pre-pregnancy weight. This in turn is clearly related to a higher frequency of adverse outcomes in subsequent pregnancies. Documented risks include higher rates of stillbirth ( 81 ). Further this ongoing weight gain adds to the “vicious cycle” of weight gain and heightened risks of development of T2DM and CV disease. However, the precise contribution of interpregnancy weight gain to the overall CV risk profile been quantified in clinical studies.
Offspring developing in a hyperglycemic environment in utero , carry higher risks of obesity developing early in the life course, progression to early impaired glucose tolerance, later development of T2DM and long term risks of development of overt CV disease. These risks are independent of maternal obesity and broadly fall into the conceptual framework of “developmental origins of health and disease” (DoHaD) ( 82 – 85 ). German researchers comparing GDM and non GDM offspring described higher risks for development of childhood disorders, even after adjustment for maternal BMI. They reported adjusted OR of 1.81 (95% CI, 1.23–2.65) for childhood overweight and 2.80 (95% CI, 1.58–4.99) for obesity ( 83 ). Maternal GDM also increased the risk of childhood abdominal adiposity (OR, 1.64; 95% CI, 1.16–2.33). Israeli researchers have reported an association between mild GDM (diet treated) and offspring CV morbidity (relative risk, 1.6; 95% CI, 1.2–2.2) ( 86 ).
Other researchers have suggested a link between GDM and neuro psychiatric disorders in offspring ( 87 ).
The efficacy of current standard GDM interventions during pregnancy in attenuating the risks of obesity and impaired glucose metabolism in children of GDM mothers is much less clear. Existing report suffer from methodologic difficulties related to post hoc design and incomplete cohort follow-up. Specifically designed prospective follow up studies would be of great value, but these are clearly difficult to design, difficult to fund and difficult to conduct. Follow up from the landmark GDM RCTs which demonstrated benefits of GDM treatment during pregnancy have been conducted. Gillman et al. ( 88 ) reported follow up from the Crowther study ( 72 ), but this report was comprised of limited follow up through school based databases rather than direct clinical contact. No benefit was shown for the offspring after maternal treatment of GDM. Landon et al. ( 89 ) reported follow up of their North American study ( 73 ) and despite detailed clinic visits, they again descried no clear offspring benefits from maternal GDM therapy. Landon et al. study did describe some minor improvement in glucometabolic status for girls whose mothers were treated for GDM. This contrasted with their previous findings related to immediate pregnancy outcomes, which had suggested that males were more likely to benefit from GDM treatment ( 90 ). The reason for this disparity remains unclear.
Gunderson et al., describing a cohort based in the USA, have recently reported that breast feeding may reduce some of the offspring risks associated with maternal GDM. They note that offspring weight for length Z score was reduced by 0.36–0.45 standard deviation units at 12 months of Age in those babies of GDM mothers who were intensively breast fed ( 91 ). At this stage, data regarding potential longer term effects is not available.
Post-Partum Glucose Testing
All women with HIP (GDM and overt DIP), should have their glycemic status reassessed with a 75 g OGTT at 6–12 weeks after delivery ( 3 ). However, compliance with this recommendation is poor in most health care systems ( 92 ) and alternative strategies based on testing in the early post-partum period are supported to some extent by physiologic ( 93 ) research demonstrating that changes in maternal insulin sensitivity occur rapidly following delivery. Early testing appears adequate to exclude ongoing Type 2 diabetes at this time point ( 94 ), but appears insufficient to exclude impaired fasting glucose (IFG) or impaired glucose tolerance (IGT) ( 94 ). Diagnosis at 6–12 weeks should conform to the local non-pregnant criteria for diabetes, IFG, and IGT. Those women whose test results fall into the normal range at this point should undergo ongoing surveillance for diabetes, while those with abnormal results should receive structured interventions for pre-diabetes or diabetes depending on their findings ( 95 ).
Irrespective early post-partum glucose results, all women with GDM should be considered to carry higher risks of future diabetes and CV disease. They should be advised to breast feed as long as possible, institute healthy eating patterns and habitual physical activity and seek to achieve a normal body weight. Ideally, they should receive support through consultation and ongoing follow-up with health care professionals knowledgeable about diabetes prevention. It must be recognized that this post-partum care is often the best (and only) opportunity to attempt to improve overall maternal health before the next pregnancy and represents an important opportunity to influence maternal and infant health over the life course.
The biggest stumbling block in the care of GDM mother and her offspring lies in post-partum follow up and inability to set up programs that can help intergenerational prevention of NCDs. There are many barriers in achieving these objectives ( 96 ). Following delivery, women with GDM (usually) no longer have glucose levels in the pre-diabetic or diabetic range. Further, they are no longer pregnancy and thus the maternal health care system rarely provides for ongoing care beyond 6- to 12-week post-partum. Thus, they are less likely to attend for regular follow up of their own health issues. Furthermore, the question of responsibility for ongoing care is often left “open”, without a clear plan for ongoing follow-up ( 97 ).
However, baby health care is often a major priority at this stage and women are generally very diligent in ensuring that their baby receives routine health checks, growth and developmental assessments and scheduled vaccinations. These often occur in a well-organized fashion for at least five years after birth ( 98 ). Therefore, obstetricians, family physicians, internists, and pediatricians must develop systems to link post-partum follow up women with GDM with the recommended routine care of their child as this appears to give the best chance of high quality care and health care engagement for the mother-baby dyad ( 3 ).
All offspring of mothers with HIP are at a heightened risk of glucometabolic and CV disease. However, the female offspring carry the additional risk of developing HIP themselves when they reach reproductive age and thus compounding the intergenerational “vicious cycle” of NCD transmission. Pregnant women with a maternal GDM history themselves are at higher risk of GDM compared to those with such a history from the paternal side ( 99 ). It is therefore important to test these women for hyperglycemia before conception or as early as possible during pregnancy and repeat testing in each trimester.
In summary, GDM represents a major challenge both in the short and long term. In the immediate pregnancy context, GDM detection and treatment are clearly valuable in improving outcomes. The associations with long term health of mothers and babies are also clear, but the optimal approach to treatment remains to be demonstrated. This is a global problem! Prevention and intervention, both during and following pregnancy are urgently needed to reduce the NCD burden of GDM as manifest for affected women and for their offspring. Despite expanding knowledge in this area, practical implementation of proven strategies remains limited. There is great potential for reduction in NCD burden from widespread implementation of relatively simple strategies, to stem the tide of the “slow motion disaster” of obesity and diabetes as identified by Doctor Margaret Chan, Director of the World Health Organization, in 2017 ( 100 ).
Author Contributions
HM wrote the first draft of the manuscript and is guarantor of the work. AK, HD, and MH all reviewed and edited the manuscript and approved the final draft. All authors contributed to the article and approved the submitted version.
Conflict of Interest
The authors declare that the research was conducted in the absence of any commercial or financial relationships that could be construed as a potential conflict of interest.
1. Cho NH, Shaw JE, Karuranga S, Huang Y, da Rocha Fernandes JD, Ohlrogge AW, et al. IDF Diabetes Atlas: Global estimates of diabetes prevalence for 2017 and projections for 2045. Diabetes Res Clin Pract (2018) 138:271–81. doi: 10.1016/j.diabres.2018.02.023
PubMed Abstract | CrossRef Full Text | Google Scholar
2. Colagiuri S, Falavigna M, Agarwal MM, Boulvain M, Coetzee E, Hod M, et al. Strategies for implementing the WHO diagnostic criteria and classification of hyperglycaemia first detected in pregnancy. Diabetes Res Clin Pract (2014) 103:364–72. doi: 10.1016/j.diabres.2014.02.012
3. Hod M, Kapur A, Sacks DA, Hadar E, Agarwal M, Di Renzo GC, et al. The International Federation of Gynecology and Obstetrics (FIGO) Initiative on gestational diabetes mellitus: A pragmatic guide for diagnosis, management, and care. Int J Gynaecol Obstet (2015) 131 Suppl 3:S173–211. doi: 10.1016/S0020-7292(15)30033-3
CrossRef Full Text | Google Scholar
4. Menke A, Casagrande S, Geiss L, Cowie CC. Prevalence of and Trends in Diabetes Among Adults in the United States, 1988-2012. Jama (2015) 314:1021–9. doi: 10.1001/jama.2015.10029
5. Menke A, Casagrande S, Cowie CC. Prevalence of Diabetes in Adolescents Aged 12 to 19 Years in the United States, 2005-2014. Jama (2016) 316:344–5. doi: 10.1001/jama.2016.8544
6. Catalano PM, McIntyre HD, Cruickshank JK, McCance DR, Dyer AR, Metzger BE, et al. The hyperglycemia and adverse pregnancy outcome study: associations of GDM and obesity with pregnancy outcomes. Diabetes Care (2012) 35:780–6. doi: 10.2337/dc11-1790
7. Metzger BE, Lowe LP, Dyer AR, Trimble ER, Chaovarindr U, Coustan DR, et al. Hyperglycemia and adverse pregnancy outcomes. N Engl J Med (2008) 358:1991–2002.
PubMed Abstract | Google Scholar
8. HAPO Study Cooperative Research Group. Hyperglycaemia and Adverse Pregnancy Outcome (HAPO) Study: associations with maternal body mass index. BJOG (2010) 117:575–84. doi: 10.1111/j.1471-0528.2009.02486.x
9. Metzger BE, Gabbe SG, Persson B, Buchanan TA, Catalano PA, Damm P, et al. International association of diabetes and pregnancy study groups recommendations on the diagnosis and classification of hyperglycemia in pregnancy. Diabetes Care (2010) 33:676–82. doi: 10.2337/dc10-0719
10. Sedgh G, Singh S, Hussain R. Intended and unintended pregnancies worldwide in 2012 and recent trends. Stud Fam Plann (2014) 45:301–14. doi: 10.1111/j.1728-4465.2014.00393.x
11. Seshiah V, Balaji V, Balaji MS, Panneerselvam A, Thamizharasi M, Arthi T. Glycemic level at the first visit and prediction of GDM. J Assoc Physicians India (2007) 55:630–2.
12. Lorenzo-Almorós A, Hang T, Peiró C, Soriano-Guillén L, Egido J, Tuñón J, et al. Predictive and diagnostic biomarkers for gestational diabetes and its associated metabolic and cardiovascular diseases. Cardiovasc Diabetol (2019) 18:140. doi: 10.1186/s12933-019-0935-9
13. Powe CE. Early Pregnancy Biochemical Predictors of Gestational Diabetes Mellitus. Curr Diabetes Rep (2017) 17:12. doi: 10.1007/s11892-017-0834-y
14. van Hoorn F, Koster M, Naaktgeboren CA, Groenendaal F, Kwee A, Lamain-de Ruiter M, et al. Prognostic models versus single risk factor approach in first-trimester selective screening for gestational diabetes mellitus: a prospective population-based multicentre cohort study. Bjog (2020). doi: 10.1111/1471-0528.16446
15. Artzi NS, Shilo S, Hadar E, Rossman H, Barbash-Hazan S, Ben-Haroush A, et al. Prediction of gestational diabetes based on nationwide electronic health records. Nat Med (2020) 26:71–6. doi: 10.1038/s41591-019-0724-8
16. Sweeting AN, Wong J, Appelblom H, Ross GP, Kouru H, Williams PF, et al. A first trimester prediction model for gestational diabetes utilizing aneuploidy and pre-eclampsia screening markers. J Matern Fetal Neonatal Med (2018) 31:2122–30. doi: 10.1080/14767058.2017.1336759
17. Ravnsborg T, Svaneklink S, Andersen LLT, Larsen MR, Jensen DM, Overgaard M. First-trimester proteomic profiling identifies novel predictors of gestational diabetes mellitus. PLoS One (2019) 14:e0214457. doi: 10.1371/journal.pone.0214457
18. Zhou T, Huang L, Wang M, Chen D, Chen Z, Jiang SW. A Critical Review of Proteomic Studies in Gestational Diabetes Mellitus. J Diabetes Res (2020) 2020:6450352. doi: 10.1155/2020/6450352
19. Jayabalan N, Lai A, Nair S, Guanzon D, Scholz-Romero K, Palma C, et al. Quantitative Proteomics by SWATH-MS Suggest an Association Between Circulating Exosomes and Maternal Metabolic Changes in Gestational Diabetes Mellitus. Proteomics (2019) 19:e1800164. doi: 10.1002/pmic.201800164
20. Herrera-Van Oostdam AS, Salgado-Bustamante M, López JA, Herrera-Van Oostdam DA, López-Hernández Y. Placental exosomes viewed from an ‘omics’ perspective: implications for gestational diabetes biomarkers identification. Biomark Med (2019) 13:675–84. doi: 10.2217/bmm-2018-0468
21. James-Allan LB, Rosario FJ, Barner K, Lai A, Guanzon D, McIntyre HD, et al. Regulation of glucose homeostasis by small extracellular vesicles in normal pregnancy and in gestational diabetes. FASEB J (2020) 34:5724–39. doi: 10.1096/fj.201902522RR
22. Yoffe L, Polsky A, Gilam A, Raff C, Mecacci F, Ognibene A, et al. Early diagnosis of gestational diabetes mellitus using circulating microRNAs. Eur J Endocrinol (2019) 181:565–77. doi: 10.1530/EJE-19-0206
23. Abdeltawab A, Zaki ME, Abdeldayem Y, Mohamed AA, Zaied SM. Circulating micro RNA-223 and angiopoietin-like protein 8 as biomarkers of gestational diabetes mellitus. Br J BioMed Sci (2020) 1–6. doi: 10.1080/09674845.2020.1764211
24. Filardi T, Catanzaro G, Mardente S, Zicari A, Santangelo C, Lenzi A, et al. Non-Coding RNA: Role in Gestational Diabetes Pathophysiology and Complications. Int J Mol Sci (2020) 21:4020. doi: 10.3390/ijms21114020
25. World Health Organization. Diagnostic Criteria and Classification of Hyperglycaemia First Detected in Pregnancy . Geneva: WHO Press (2013).
Google Scholar
26. A.C.o.P. Bulletins. ACOG Practice Bulletin No. 190: Gestational Diabetes Mellitus. Obstet Gynecol (2018) 131:e49–64. doi: 10.1097/AOG.0000000000002501
27. Canadian Diabetes Association. Canadian Diabetes Association 2008 Clinical Practice Guidelines for the Prevention and Management of Diabetes in Canada. Can J Diabetes (2008) 32:S168–81.
28. Round JA, Jacklin P, Fraser RB, Hughes RG, Mugglestone MA, Holt RI. Screening for gestational diabetes mellitus: cost-utility of different screening strategies based on a woman’s individual risk of disease. Diabetologia (2011) 54:256–63. doi: 10.1007/s00125-010-1881-y
29. NICE guidelines, Diabetes in pregnancy: management from preconception to the postnatal period . London, UK: National Institute for Health and Care Excellence: Clinical Guidelines (2015).
30. Tieu J, McPhee AJ, Crowther CA, Middleton P, Shepherd E. Screening for gestational diabetes mellitus based on different risk profiles and settings for improving maternal and infant health. Cochrane Database Syst Rev (2017) 8:Cd007222. doi: 10.1002/14651858.CD007222.pub4
31. Mo X, Tobe RG, Takahashi Y, Arata N, Liabsuetrakul T, Nakayama T, et al. Economic evaluations of gestational diabetes mellitus screening: A systematic review. J Epidemiol (2020). doi: 10.2188/jea.JE20190338
32. Persson M, Winkvist A, Mogren I. Surprisingly low compliance to local guidelines for risk factor based screening for gestational diabetes mellitus - A population-based study. BMC Pregnancy Childbirth (2009) 9:53. doi: 10.1186/1471-2393-9-53
33. Murphy NM, McCarthy FP, Khashan AS, Myers JE, Simpson NA, Kearney PM, et al. Compliance with National Institute of Health and Care Excellence risk-based screening for Gestational Diabetes Mellitus in nulliparous women. Eur J Obstet Gynecol Reprod Biol (2016) 199:60–5. doi: 10.1016/j.ejogrb.2016.01.044
34. Adam S, Rheeder P. Screening for gestational diabetes mellitus in a South African population: Prevalence, comparison of diagnostic criteria and the role of risk factors. S Afr Med J (2017) 107:523–7. doi: 10.7196/SAMJ.2017.v107i6.12043
35. Meththananda Herath HM, Weerarathna TP, Weerasinghe NP. Is Risk Factor-based Screening Good Enough to Detect Gestational Diabetes Mellitus in High-Risk Pregnant Women? A Sri Lankan Experience. Int J Prev Med (2016) 7:99. doi: 10.4103/2008-7802.188084
36. Bonongwe P, Lindow SW, Coetzee EJ. Reproducibility of a 75G oral glucose tolerance test in pregnant women. J Perinatal Med (2015) 43:333–8. doi: 10.1515/jpm-2014-0208
37. Dunseath GJ, Bright D, Jones C, Dowrick S, Cheung WY, Luzio SD. Performance evaluation of a self-administered home oral glucose tolerance test kit in a controlled clinical research setting. Diabetes Med (2019) 36:862–7. doi: 10.1111/dme.13961
38. Dhatt GS, Agarwal MM, Othman Y, Nair SC. Performance of the Roche Accu-Chek active glucose meter to screen for gestational diabetes mellitus using fasting capillary blood. Diabetes Technol Ther (2011) 13:1229–33.
39. Lowe LP, Metzger BE, Dyer AR, Lowe J, McCance DR, Lappin TR, et al. Hyperglycemia and Adverse Pregnancy Outcome (HAPO) Study: associations of maternal A1C and glucose with pregnancy outcomes. Diabetes Care (2012) 35:574–80.
40. Hughes RC, Moore MP, Gullam JE, Mohamed K, Rowan J. An early pregnancy HbA1c >/=5.9% (41 mmol/mol) is optimal for detecting diabetes and identifies women at increased risk of adverse pregnancy outcomes. Diabetes Care (2014) 37:2953–9.
41. Mendes N, Tavares Ribeiro R, Serrano F. Beyond self-monitored plasma glucose and HbA1c: the role of non-traditional glycaemic markers in gestational diabetes mellitus. J Obstet Gynaecol (2018) 38:762–9.
42. Ghosh P, Luque-Fernandez MA, Vaidya A, Ma D, Sahoo R, Chorev M, et al. Plasma Glycated CD59, a Novel Biomarker for Detection of Pregnancy-Induced Glucose Intolerance. Diabetes Care (2017) 40:981–4.
43. Ma D, Luque-Fernandez MA, Bogdanet D, Desoye G, Dunne F, Halperin JA. Plasma Glycated CD59 Predicts Early Gestational Diabetes and Large for Gestational Age Newborns. J Clin Endocrinol Metab (2020) 105:e1033–40. doi: 10.1210/clinem/dgaa087
44. Bogdanet D, O’Shea PM, Halperin J, Dunne F. Plasma glycated CD59 (gCD59), a novel biomarker for the diagnosis, management and follow up of women with Gestational Diabetes (GDM) - protocol for prospective cohort study. BMC Pregnancy Childbirth (2020) 20:412. doi: 10.1186/s12884-020-03090-9
45. Zhang C, Rawal S, Chong YS. Risk factors for gestational diabetes: is prevention possible? Diabetologia (2016) 59:1385–90. doi: 10.1007/s00125-016-3979-3
46. Kwong W, Tomlinson G, Feig DS. Maternal and neonatal outcomes after bariatric surgery; a systematic review and meta-analysis: do the benefits outweigh the risks? Am J Obstet Gynecol (2018) 218:573–80. doi: 10.1016/j.ajog.2018.02.003
47. Griffith RJ, Alsweiler J, Moore AE, Brown S, Middleton P, Shepherd E, et al. Interventions to prevent women from developing gestational diabetes mellitus: an overview of Cochrane Reviews. Cochrane Database Syst Rev (2020) 6:Cd012394. doi: 10.1002/14651858.CD012394.pub3
48. Song C, Li J, Leng J, Ma RC, Yang X. Lifestyle intervention can reduce the risk of gestational diabetes: a meta-analysis of randomized controlled trials. Obes Rev (2016) 10:960–9. doi: 10.1111/obr.12442
49. Lindsay RS, Loeken MR. Metformin use in pregnancy: promises and uncertainties. Diabetologia (2017) 60:1612–9. doi: 10.1007/s00125-017-4351-y
50. Chiswick C, Reynolds RM, Denison F, Drake AJ, Forbes S, Newby DE, et al. Effect of metformin on maternal and fetal outcomes in obese pregnant women (EMPOWaR): a randomised, double-blind, placebo-controlled trial. Lancet Diabetes Endocrinol (2015) 3:778–86. doi: 10.1016/S2213-8587(15)00219-3
51. Syngelaki A, Nicolaides KH, Balani J, Hyer S, Akolekar R, Kotecha R, et al. Metformin versus Placebo in Obese Pregnant Women without Diabetes Mellitus. N Engl J Med (2016) 374:434–43. doi: 10.1056/NEJMoa1509819
52. Dodd JM, Louise J, Deussen AR, Grivell RM, Dekker G, McPhee AJ, et al. Effect of metformin in addition to dietary and lifestyle advice for pregnant women who are overweight or obese: the GRoW randomised, double-blind, placebo-controlled trial. Lancet Diabetes Endocrinol (2019) 7:15–24. doi: 10.1016/S2213-8587(18)30310-3
53. Corrado F, D’Anna R, Di Vieste G, Giordano D, Pintaudi B, Santamaria A, et al. The effect of myoinositol supplementation on insulin resistance in patients with gestational diabetes. Diabetes Med (2011) 28:972–5. doi: 10.1111/j.1464-5491.2011.03284.x
54. D’Anna R, Scilipoti A, Giordano D, Caruso C, Cannata ML, Interdonato ML, et al. myo-Inositol supplementation and onset of gestational diabetes mellitus in pregnant women with a family history of type 2 diabetes: a prospective, randomized, placebo-controlled study. Diabetes Care (2013) 36:854–7. doi: 10.2337/dc12-1371
55. Farren M, Daly N, McKeating A, Kinsley B, Turner MJ, Daly S. The Prevention of Gestational Diabetes Mellitus With Antenatal Oral Inositol Supplementation: A Randomized Controlled Trial. Diabetes Care (2017) 40:759–63. doi: 10.2337/dc16-2449
56. Malvasi A, Kosmas I, Mynbaev OA, Sparic R, Gustapane S, Guido M, et al. Can trans resveratrol plus d-chiro-inositol and myo-inositol improve maternal metabolic profile in overweight pregnant patients? Clin Ter (2017) 168:e240–7.
57. Godfrey KM, Cutfield W, Chan SY, Baker PN, Chong YS. Nutritional Intervention Preconception and During Pregnancy to Maintain Healthy Glucose Metabolism and Offspring Health (“NiPPeR”): study protocol for a randomised controlled trial. Trials (2017) 18:131. doi: 10.1186/s13063-017-1875-x
58. Isolauri E, Rautava S, Collado MC, Salminen S. Role of probiotics in reducing the risk of gestational diabetes. Diabetes Obes Metab (2015) 17:713–9. doi: 10.1111/dom.12475
59. Lindsay KL, Kennelly M, Culliton M, Smith T, Maguire OC, Shanahan F, et al. Probiotics in obese pregnancy do not reduce maternal fasting glucose: a double-blind, placebo-controlled, randomized trial (Probiotics in Pregnancy Study). Am J Clin Nutr (2014) 99:1432–9. doi: 10.3945/ajcn.113.079723
60. Callaway LK, McIntyre HD, Barrett HL, Foxcroft K, Tremellen A, Lingwood BE, et al. Probiotics for the Prevention of Gestational Diabetes Mellitus in Overweight and Obese Women: Findings From the SPRING Double-blind Randomized Controlled Trial. Diabetes Care (2019) 42:364–71. doi: 10.2337/dc18-2248
61. Okesene-Gafa KAM, Li M, McKinlay CJD, Taylor RS, Rush EC, Wall CR, et al. Effect of antenatal dietary interventions in maternal obesity on pregnancy weight-gain and birthweight: Healthy Mums and Babies (HUMBA) randomized trial. Am J Obstet Gynecol (2019) 221:152.e1–152.e13.
62. Pellonperä O, Mokkala K, Houttu N, Vahlberg T, Koivuniemi E, Tertti K, et al. Efficacy of Fish Oil and/or Probiotic Intervention on the Incidence of Gestational Diabetes Mellitus in an At-Risk Group of Overweight and Obese Women: A Randomized, Placebo-Controlled, Double-Blind Clinical Trial. Diabetes Care (2019) 42:1009–17. doi: 10.2337/dc18-2591
63. Halkjær SI, de Knegt VE, Lo B, Nilas L, Cortes D, Pedersen AE, et al. Multistrain Probiotic Increases the Gut Microbiota Diversity in Obese Pregnant Women: Results from a Randomized, Double-Blind Placebo-Controlled Study. Curr Dev Nutr (2020) 4:nzaa095. doi: 10.1093/cdn/nzaa095
64. Luo X, Ma X, Hu H, Li C, Cao S, Huang L, et al. Kinetic study of pentosan solubility during heating and reacting processes of steam treatment of green bamboo. Bioresour Technol (2013) 130:769–76. doi: 10.1016/j.biortech.2012.12.088
65. Wood K, Mantzioris E, Lingwood B, Couper J, Makrides M, Gibson RA, et al. The effect of maternal DHA supplementation on body fat mass in children at 7 years: follow-up of the DOMInO randomized controlled trial. Prostaglandins Leukot Essent Fatty Acids (2017) 139:49–54. doi: 10.1016/j.plefa.2017.09.013
66. Sadeghian M, Asadi M, Rahmani S, Akhavan Zanjani M, Sadeghi O, Hosseini SA, et al. Circulating vitamin D and the risk of gestational diabetes: a systematic review and dose-response meta-analysis. Endocrine (2020) 70:36–47. doi: 10.1007/s12020-020-02360-y
67. Palacios C, Kostiuk LK, Peña-Rosas JP. Vitamin D supplementation for women during pregnancy. Cochrane Database Syst Rev (2019) 7:Cd008873. doi: 10.1002/14651858.CD008873.pub4
68. Corcoy R, Mendoza LC, Simmons D, Desoye G, Mathiesen ER, Kautzky-Willer A, et al. Vitamin D and gestational diabetes mellitus: a systematic review based on data free of Hawthorne effect. BJOG (2018) 125:1338–9. doi: 10.1111/1471-0528.15278
69. Bokharee N, Khan YH, Wasim T, Mallhi TH, Alotaibi NH, Iqbal MS, et al. Daily versus stat vitamin D supplementation during pregnancy; A prospective cohort study. PLoS One (2020) 15:e0231590. doi: 10.1371/journal.pone.0231590
70. Cannell JJ. Vitamin D and autism, what’s new? Rev Endocr Metab Disord (2017) 18:183–93. doi: 10.1007/s11154-017-9409-0
71. McIntyre HD, Catalano P, Zhang C, Desoye G, Mathiesen ER, Damm P. Gestational diabetes mellitus. Nat Rev Dis Primers (2019) 5:47. doi: 10.1038/s41572-019-0098-8
72. Crowther CA, Hiller JE, Moss JR, McPhee AJ, Jeffries WS, Robinson JS. Effect of treatment of gestational diabetes mellitus on pregnancy outcomes. N Engl J Med (2005) 352:2477–86. doi: 10.1056/NEJMoa042973
73. Landon MB, Spong CY, Thom E, Carpenter MW, Ramin SM, Casey B, et al. A multicenter, randomized trial of treatment for mild gestational diabetes. N Engl J Med (2009) 361:1339–48. doi: 10.1056/NEJMoa0902430
74. Vounzoulaki E, Khunti K, Abner SC, Tan BK, Davies MJ, Gillies CL. Progression to type 2 diabetes in women with a known history of gestational diabetes: systematic review and meta-analysis. BMJ (2020) 369:m1361. doi: 10.1136/bmj.m1361
75. Retnakaran R, Shah BR. Mild glucose intolerance in pregnancy and risk of cardiovascular disease: a population-based cohort study. CMAJ (2009) 181:371–6. doi: 10.1503/cmaj.090569
76. Retnakaran R, Shah BR. Role of Type 2 Diabetes in Determining Retinal, Renal, and Cardiovascular Outcomes in Women With Previous Gestational Diabetes Mellitus. Diabetes Care (2017) 40:101–8. doi: 10.2337/dc16-1400
77. Kramer CK, Campbell S, Retnakaran R. Gestational diabetes and the risk of cardiovascular disease in women: a systematic review and meta-analysis. Diabetologia (2019) 62:905–14. doi: 10.1007/s00125-019-4840-2
78. Bao W, Tobias DK, Bowers K, Chavarro J, Vaag A, Grunnet LG, et al. Physical activity and sedentary behaviors associated with risk of progression from gestational diabetes mellitus to type 2 diabetes mellitus: a prospective cohort study. JAMA Internal Med (2014) 174:1047–55. doi: 10.1001/jamainternmed.2014.1795
79. Much D, Beyerlein A, Roßbauer M, Hummel S, Ziegler AG. Beneficial effects of breastfeeding in women with gestational diabetes mellitus. Mol Metab (2014) 3:284–92. doi: 10.1016/j.molmet.2014.01.002
80. Ma S, Hu S, Liang H, Xiao Y, Tan H. Metabolic effects of breastfeed in women with prior gestational diabetes mellitus: A systematic review and meta-analysis. Diabetes Metab Res Rev (2019) 35:e3108. doi: 10.1002/dmrr.3108
81. Cnattingius S, Villamor E. Weight change between successive pregnancies and risks of stillbirth and infant mortality: a nationwide cohort study. Lancet (2016) 387:558–65. doi: 10.1016/S0140-6736(15)00990-3
82. Lowe WL Jr., Scholtens DM, Kuang A, Linder B, Lawrence JM, Lebenthal Y, et al. Hyperglycemia and Adverse Pregnancy Outcome Follow-up Study (HAPO FUS): Maternal Gestational Diabetes and Childhood Glucose Metabolism. Diabetes Care (2019) 42:372–80. doi: 10.2337/dc18-1646
83. Nehring I, Chmitorz A, Reulen H, von Kries R, Ensenauer R. Gestational diabetes predicts the risk of childhood overweight and abdominal circumference independent of maternal obesity. Diabetes Med (2013) 30:1449–56. doi: 10.1111/dme.12286
84. Dabelea D, Mayer-Davis EJ, Lamichhane AP, D’Agostino RB Jr., Liese AD, Vehik KS, et al. Association of intrauterine exposure to maternal diabetes and obesity with type 2 diabetes in youth: the SEARCH Case-Control Study. Diabetes Care (2008) 31:1422–6. doi: 10.2337/dc07-2417
85. Osgood ND, Dyck RF, Grassmann WK. The inter- and intragenerational impact of gestational diabetes on the epidemic of type 2 diabetes. Am J Public Health (2011) 101:173–9. doi: 10.2105/AJPH.2009.186890
86. Leybovitz-Haleluya N, Wainstock T, Landau D, Sheiner E. Maternal gestational diabetes mellitus and the risk of subsequent pediatric cardiovascular diseases of the offspring: a population-based cohort study with up to 18 years of follow up. Acta Diabetol (2018) 55:10037–42. doi: 10.1016/j.reprotox.2018.03.009
87. Nahum Sacks K, Friger M, Shoham-Vardi I, Abokaf H, Spiegel E, Sergienko R, et al. Prenatal exposure to gestational diabetes mellitus as an independent risk factor for long-term neuropsychiatric morbidity of the offspring. Am J Obstet Gynecol (2016) 215:380.e1–7.
88. Gillman MW, Oakey H, Baghurst PA, Volkmer RE, Robinson JS, Crowther CA. Effect of treatment of gestational diabetes mellitus on obesity in the next generation. Diabetes Care (2010) 33:964–8. doi: 10.2337/dc09-1810
89. Landon MB, Rice MM, Varner MW, Casey BM, Reddy UM, Wapner RJ, et al. Mild gestational diabetes mellitus and long-term child health. Diabetes Care (2015) 38:445–52. doi: 10.2337/dc14-2159
90. Bahado-Singh RO, Mele L, Landon MB, Ramin SM, Carpenter MW, Casey B, et al. Fetal male gender and the benefits of treatment of mild gestational diabetes mellitus. Am J Obstet Gynecol (2012) 206:422.e1–5.
91. Gunderson EP, Greenspan LC, Faith MS, Hurston SR, Quesenberry CP Jr. Breastfeeding and growth during infancy among offspring of mothers with gestational diabetes mellitus: a prospective cohort study. Pediatr Obes (2018) 13:492–504. doi: 10.1111/ijpo.12277
92. Gabbe SG, Landon MB, Warren-Boulton E, Fradkin J. Promoting health after gestational diabetes: a National Diabetes Education Program call to action. Obstet Gynecol (2012) 119:171–6. doi: 10.1097/AOG.0b013e3182393208
93. Waters TP, Minium J, Hagiac M, Schnellinger P, Ryan D, Haugel-de Mouzon S, et al. Does maternal insulin sensitivity improve immediately after delivery or do we need to wait unti six weeks postpartum? Am J Obstet Gynecol (2015) 212:S20. doi: 10.1016/j.ajog.2014.10.074
94. Waters TP, Kim SY, Werner E, Dinglas C, Carter EB, Patel R, et al. Should women with gestational diabetes be screened at delivery hospitalization for type 2 diabetes? Am J Obstet Gynecol (2020) 222:73.e1–73.e11.
95. A.D. Association. 2. Classification and Diagnosis of Diabetes: Standards of Medical Care in Diabetes-2020. Diabetes Care (2020) 43:S14–s31. doi: 10.2337/dc20-S002
96. Nielsen KK, Kapur A, Damm P, de Courten M, Bygbjerg IC. From screening to postpartum follow-up - the determinants and barriers for gestational diabetes mellitus (GDM) services, a systematic review. BMC Pregnancy Childbirth (2014) 14:41. doi: 10.1186/1471-2393-14-41
97. Wilkinson SA, Lim SS, Upham S, Pennington A, O’Reilly SL, Asproloupos D, et al. Who’s responsible for the care of women during and after a pregnancy affected by gestational diabetes? Med J Aust (2014) 201:S78–81. doi: 10.5694/mja14.00251
98. Kapur A. Pregnancy: a window of opportunity for improving current and future health. Int J Gynaecol Obstet (2011) 115:S50–1. doi: 10.1016/S0020-7292(11)60015-5
99. Kragelund Nielsen K, Damm P, Kapur A, Balaji V, Balaji MS, Seshiah V, et al. Risk Factors for Hyperglycaemia in Pregnancy in Tamil Nadu, India. PLoS One (2016) 11:e0151311. doi: 10.1371/journal.pone.0151311
100. Chan M. Obesity and Diabetes: The Slow-Motion Disaster. Milbank Q (2017) 95:11–4. doi: 10.1111/1468-0009.12238
Keywords: gestational diabetes, prediction, diagnosis, prevention, management, pregnancy, non-communicable disease
Citation: McIntyre HD, Kapur A, Divakar H and Hod M (2020) Gestational Diabetes Mellitus—Innovative Approach to Prediction, Diagnosis, Management, and Prevention of Future NCD—Mother and Offspring. Front. Endocrinol. 11:614533. doi: 10.3389/fendo.2020.614533
Received: 06 October 2020; Accepted: 04 November 2020; Published: 03 December 2020.
Reviewed by:
Copyright © 2020 McIntyre, Kapur, Divakar and Hod. This is an open-access article distributed under the terms of the Creative Commons Attribution License (CC BY) . The use, distribution or reproduction in other forums is permitted, provided the original author(s) and the copyright owner(s) are credited and that the original publication in this journal is cited, in accordance with accepted academic practice. No use, distribution or reproduction is permitted which does not comply with these terms.
*Correspondence: H. David McIntyre, [email protected]
Disclaimer: All claims expressed in this article are solely those of the authors and do not necessarily represent those of their affiliated organizations, or those of the publisher, the editors and the reviewers. Any product that may be evaluated in this article or claim that may be made by its manufacturer is not guaranteed or endorsed by the publisher.
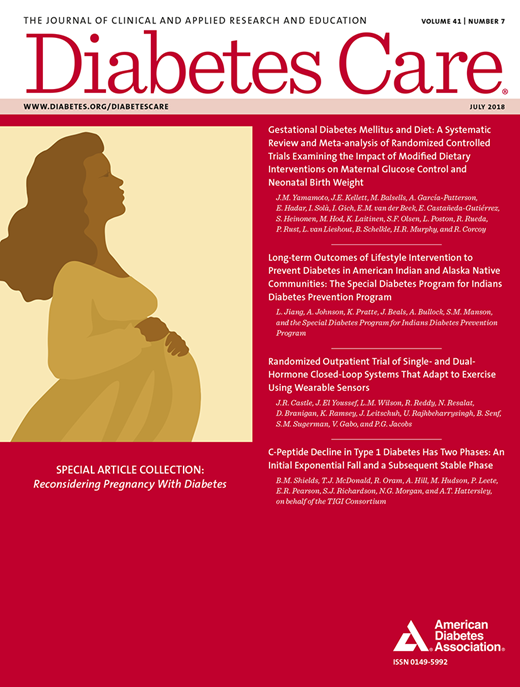
- Previous Article
- Next Article
Introduction
Research design and methods, conclusions, article information, gestational diabetes mellitus and diet: a systematic review and meta-analysis of randomized controlled trials examining the impact of modified dietary interventions on maternal glucose control and neonatal birth weight.

- Split-Screen
- Article contents
- Figures & tables
- Supplementary Data
- Peer Review
- Open the PDF for in another window
- Cite Icon Cite
- Get Permissions
Jennifer M. Yamamoto , Joanne E. Kellett , Montserrat Balsells , Apolonia García-Patterson , Eran Hadar , Ivan Solà , Ignasi Gich , Eline M. van der Beek , Eurídice Castañeda-Gutiérrez , Seppo Heinonen , Moshe Hod , Kirsi Laitinen , Sjurdur F. Olsen , Lucilla Poston , Ricardo Rueda , Petra Rust , Lilou van Lieshout , Bettina Schelkle , Helen R. Murphy , Rosa Corcoy; Gestational Diabetes Mellitus and Diet: A Systematic Review and Meta-analysis of Randomized Controlled Trials Examining the Impact of Modified Dietary Interventions on Maternal Glucose Control and Neonatal Birth Weight. Diabetes Care 1 July 2018; 41 (7): 1346–1361. https://doi.org/10.2337/dc18-0102
Download citation file:
- Ris (Zotero)
- Reference Manager
Medical nutrition therapy is a mainstay of gestational diabetes mellitus (GDM) treatment. However, data are limited regarding the optimal diet for achieving euglycemia and improved perinatal outcomes. This study aims to investigate whether modified dietary interventions are associated with improved glycemia and/or improved birth weight outcomes in women with GDM when compared with control dietary interventions.
Data from published randomized controlled trials that reported on dietary components, maternal glycemia, and birth weight were gathered from 12 databases. Data were extracted in duplicate using prespecified forms.
From 2,269 records screened, 18 randomized controlled trials involving 1,151 women were included. Pooled analysis demonstrated that for modified dietary interventions when compared with control subjects, there was a larger decrease in fasting and postprandial glucose (−4.07 mg/dL [95% CI −7.58, −0.57]; P = 0.02 and −7.78 mg/dL [95% CI −12.27, −3.29]; P = 0.0007, respectively) and a lower need for medication treatment (relative risk 0.65 [95% CI 0.47, 0.88]; P = 0.006). For neonatal outcomes, analysis of 16 randomized controlled trials including 841 participants showed that modified dietary interventions were associated with lower infant birth weight (−170.62 g [95% CI −333.64, −7.60]; P = 0.04) and less macrosomia (relative risk 0.49 [95% CI 0.27, 0.88]; P = 0.02). The quality of evidence for these outcomes was low to very low. Baseline differences between groups in postprandial glucose may have influenced glucose-related outcomes. As well, relatively small numbers of study participants limit between-diet comparison.
Modified dietary interventions favorably influenced outcomes related to maternal glycemia and birth weight. This indicates that there is room for improvement in usual dietary advice for women with GDM.
Gestational diabetes mellitus (GDM) is one of the most common medical complications in pregnancy and affects an estimated 14% of pregnancies, or one in every seven births globally ( 1 ). Women with GDM and their offspring are at increased risk of both short- and longer-term complications, including, for mothers, later development of type 2 diabetes, and for offspring, increased lifelong risks of developing obesity, type 2 diabetes, and metabolic syndrome ( 2 – 6 ). The adverse intrauterine environment causes epigenetic changes in the fetus that may contribute to metabolic disorders, the so-called vicious cycle of diabetes ( 7 ).
The mainstay of GDM treatment is dietary and lifestyle advice, which includes medical nutrition therapy, weight management, and physical activity ( 8 ). Women monitor their fasting and postmeal glucose levels and adjust their individual diet and lifestyle to meet their glycemic targets. This pragmatic approach achieves the glycemic targets in approximately two-thirds of women with GDM ( 8 ). However, despite the importance of medical nutrition therapy and its widespread recommendation in clinical practice, there are limited data regarding the optimal diet for achieving maternal euglycemia ( 8 – 11 ). It is also unknown whether the dietary interventions for achieving maternal glycemia are also effective for reducing excessive fetal growth and adiposity ( 12 ).
Different dietary strategies have been reported including low glycemic index (GI), energy restriction, increase or decrease in carbohydrates, and modifications of fat or protein quality or quantity ( 12 – 14 ). Three recent systematic reviews have been performed examining specific diets and pregnancy outcomes ( 15 – 17 ). Viana et al. ( 16 ) and Wei et al. ( 15 ) concluded that low-GI diets were associated with a decreased risk of infant macrosomia. However, the most recent systematic review from Cochrane, including 19 trials randomizing 1,398 women, found no clear difference in large for gestational age or other primary neonatal outcomes with the low-GI diet ( 17 ). The primary maternal outcomes were hypertension (gestational and/or preeclampsia), delivery by cesarean section, and type 2 diabetes, outcomes for which most trials lacked statistical power, even when dietary subgroups were combined. Remarkably, no systematic reviews examined the impact of modified dietary interventions on the detailed maternal glycemic parameters, including change in glucose-related variables, the outcomes that are most directly influenced by diet.
To address this knowledge gap, we performed a systematic review and meta-analysis of randomized controlled trials to investigate whether modified dietary interventions (defined as a dietary intervention different from the usual one used in the control group) in women with GDM offer improved glycemic control and/or improved neonatal outcomes when compared with standard diets.
In accordance with a published protocol (PROSPERO CRD42016042391), we performed a systematic review and meta-analysis. Reporting is in accordance with the Preferred Reporting Items for Systematic Reviews and Meta-Analyses (PRISMA) guidelines ( 18 ). An international panel of experts was formed by the International Life Sciences Institute Europe. This panel determined the review protocol and carried out all aspects of the review.
Data Sources and Search Strategy
The following databases were searched for all available dates using the search terms detailed in Supplementary Table 1 : PubMed, MEDLINE, Cochrane Central Register of Controlled Trials (CENTRAL), Embase, Cumulative Index to Nursing and Allied Health Literature (CINAHL), Web of Science Core Collection, Applied Social Sciences Index and Abstracts, ProQuest, ProQuest Dissertations & Theses—A&I and UK & Ireland, National Institute for Health and Care Excellence evidence search, Scopus, UK Clinical Trials Gateway, ISRCTN, and ClinicalTrials.gov . The initial search was performed in July 2016. An updated search of MEDLINE, Embase, CENTRAL, and CINAHL was performed on 3 October 2017 using the same search terms.
A hand-search of relevant reviews and all included articles was conducted to identify studies for potential inclusion. As well, experts on the panel were consulted for the inclusion of additional articles. Reference management was carried out using EndNote.
Study Selection
All titles and abstracts were assessed independently and in duplicate to identify articles requiring full-text review. Published studies fulfilling the following criteria were included: randomized controlled trials, evaluated modified dietary interventions on women with GDM, glucose intolerance or hyperglycemia during pregnancy, reported-on primary maternal and neonatal outcomes, included women aged 18–45 years, had a duration of 2 weeks or more, and were published in English, French, Spanish, Portuguese, Italian, Dutch, German, or Chinese. We excluded studies that included participants with type 1 or type 2 diabetes if data for participants with GDM were not presented independently, if dietary characteristics were not available, if the study was in animals, or if the study did not report outcomes of interest. We did not include studies of nutritional supplements such as vitamin D or probiotics as recent reviews have addressed these topics ( 19 , 20 ).
All citations identified after title and abstract assessment were full-text reviewed in duplicate. Reasons for exclusion at the full-text review stage were recorded. Any disagreements between reviewers were resolved by consensus and with consultation with the expert group when required.
Data Extraction
Data from included studies were extracted in duplicate using prespecified data extraction forms. Extracted data elements included study and participant demographics, study design, diagnostic criteria for GDM, glucose intolerance or hyperglycemia, funding source, description of modified dietary intervention and comparator, and maternal and neonatal outcomes. For studies with missing data, inconsistencies, or other queries, authors were contacted. Record management was carried out using Microsoft Excel and RevMan.
For articles providing information on maternal weight, fasting glucose, postprandial glucose, HbA 1c , or HOMA insulin resistance index (HOMA-IR) at baseline and postintervention but not their change, change was calculated as the difference between postintervention and baseline. Standard deviations were imputed using the correlation coefficient observed in articles reporting full information on the variable at baseline and postintervention and its change or a correlation coefficient of 0.5 when this information was not available ( 21 ). As studies differed in postprandial glucose at baseline, glycemic control at study entry was not considered to be equivalent in both arms, and thus continuous glucose-related variables at follow-up are reported as change from baseline.
Data Synthesis
The primary outcomes were maternal glycemic outcomes (mean glucose, fasting glucose, postprandial glucose [after breakfast, lunch, and dinner and combined], hemoglobin A 1c [HbA 1c ], assessment of insulin sensitivity by HOMA-IR, and change in these parameters from baseline to assessment; medication treatment [defined as oral diabetes medications or insulin]) and neonatal birth weight outcomes (birth weight, macrosomia, and large for gestational age).
Data were pooled into relative risks (RRs) or mean differences with 95% CI for dichotomous outcomes and continuous outcomes, respectively. Meta-analysis was performed using random-effects models. A prespecified analysis stratified by type of diet and quality assessment was performed to explore potential reasons for interstudy variation. Heterogeneity was assessed using I 2 statistics. Small study effects were examined for using funnel plots. Analyses were conducted using RevMan version 5.3. Pooled estimation of birth weight in the study and control arms, both overall and according to the specific diet intervention, was performed using Stata 14.0.
Quality Assessment
Methodological quality and bias assessment was completed by two reviewers. Risk of bias was assessed using the Cochrane Collaboration tool, which rates seven items as being high, low, or unclear for risk of bias ( 21 ). These items included random sequence generation, allocation concealment, blinding of participants and personnel, blinding of outcome assessment, incomplete outcome data, selective outcome reporting, and other potential sources of bias ( 21 ). A sensitivity analysis was performed excluding articles with relevant weaknesses in trial design or execution.
The overall quality of the evidence was also assessed using Grading of Recommendations Assessment, Development and Evaluation (GRADE) working group guidelines ( 21 ). GRADE was assessed for all primary and secondary outcomes, both maternal and neonatal, but without subgroup analysis per different dietary intervention for each outcome measure.
We screened 2,269 records for potential inclusion, and 126 articles were reviewed in full ( Supplementary Fig. 1 ). Eighteen studies ( 12 – 14 , 22 – 36 ) were included in the meta-analysis with a total of 1,151 pregnant women with GDM.
Study Characteristics
The types of modified dietary intervention included low-GI ( n = 4), Dietary Approaches to Stop Hypertension (DASH) ( n = 3), low-carbohydrate ( n = 3), fat-modification ( n = 2), soy protein–enrichment ( n = 2), energy-restriction ( n = 1), high-fiber ( n = 1), and ethnic diets (i.e., foods commonly consumed according to participant’s ethnicity) ( n = 1) and behavioral intervention ( n = 1). Details of the study characteristics are included in Table 1 . Most trials were single centered and had small sample sizes (range 12–150). Only two trials (one each from Spain and Australia) included over 100 participants, nine had 50–100 participants, and seven studies had fewer than 50 participants. They were performed in North America, Europe, or Australasia and all had a duration of at least 2 weeks. The ethnicity of participants was reported in seven studies ( 12 , 13 , 26 , 29 , 31 , 32 , 34 ).
Characteristics of studies included
Unless otherwise stated, the units are kcal/day for energy, % for carbohydrate, protein, and fat. OGTT, oral glucose tolerance test.
*Reported actual dietary intake. When not reported, prescribed dietary intake is reported.
†Intervention is defined as dietary intervention different from the usual dietary intervention used in the control group.
‡Indicates prescribed diet.
§The control and intervention groups were reversed for the purpose of meta-analysis so it could be included in the low-carbohydrate group.
Most studies assessed individual dietary adherence using food diaries ( 13 , 23 – 36 ). Although most studies did report an overall difference in dietary composition between the intervention diet and control diet, few studies reported a detailed assessment of dietary adherence. Only five studies used a formal measure of adherence ( 24 , 25 , 29 , 33 , 34 ), and four of them reported data ( 25 , 29 , 33 , 34 ). Adherence ranged from 20% to 76% in the control groups and 60% to 80% in the intervention groups.
Participant Characteristics
When baseline characteristic data were pooled, women in the intervention group were older than women in the control group (pooled mean difference 0.60 years [95% CI 0.06, 1.14]) and had higher postprandial glucose (5.47 [0.86, 10.08]), most influenced by the DASH and ethnic diet studies. There was no overall significant difference between the intervention and control groups for BMI, gestational age at enrollment, fasting glucose, HbA 1c , or HOMA-IR.
Maternal Glycemic Outcomes for All Modified Dietary Interventions
Pooled risk ratios in 15 studies involving 1,023 women demonstrated a lower need for medication (RR 0.65 [95% CI 0.47, 0.88]; I 2 = 55) ( Table 2 ). Thirteen studies ( n = 662 women) reported fasting glucose levels, nine ( n = 475) reported combined postprandial glucose measures, and three ( n = 175) reported post-breakfast glucose measures. Pooled analysis demonstrated a larger decrease in fasting, combined postprandial, and post-breakfast glucose levels in modified dietary interventions (mean −4.07 mg/dL [95% CI −7.58, −0.57], I 2 = 86, P = 0.02; −7.78 mg/dL [−12.27, −3.29], I 2 = 63, P = 0.0007; and −4.76 mg/dL [−9.13, −0.38], I 2 = 34, P = 0.03, respectively) compared with control group. There were no significant differences in change in HbA 1c (seven studies), HOMA-IR (four studies), or in post-lunch or -dinner glucose levels (two studies).
Pooled analyses of primary maternal glycemic and infant birth weight outcomes
Neonatal Birth Weight Outcomes for All Diets
Pooled mean birth weight was 3,266.65 g (95% CI 3,172.15, 3,361.16) in the modified dietary intervention versus 3,449.88 g (3,304.34, 3,595.42) in the control group. Pooled analysis of all 16 modified dietary interventions including 841 participants demonstrated lower birth weight (mean −170.62 g [95% CI −333.64, −7.60], I 2 = 88; P = 0.04) and less macrosomia (RR 0.49 [95% CI 0.27, 0.88], I 2 = 11; P = 0.02) compared with conventional dietary advice ( Table 2 and Fig. 1 ). There was no significant difference in the risk of large-for-gestational-age newborns in modified dietary interventions as compared with control diets (RR 0.96 [95% CI 0.63, 1.46], I 2 = 0; P = 0.85).
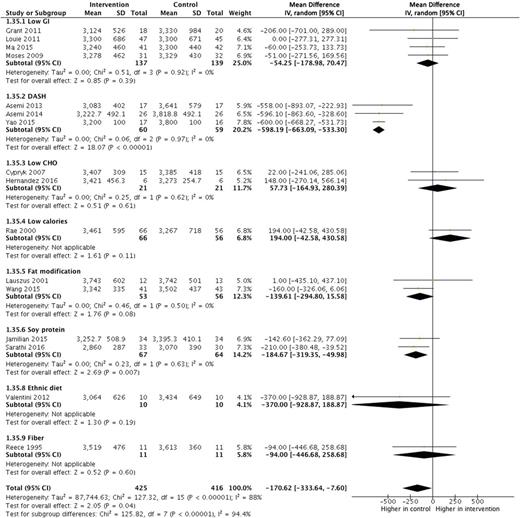
Forest plot of birth weight for modified dietary interventions compared with control diets in women with GDM. Reference citations for studies can be found in Table 1 . CHO, carbohydrate; IV, inverse variance.
Subgroup Meta-analysis by Types of Dietary Interventions
Pooled analysis of low-GI diets showed a larger decrease in fasting ( 26 , 29 , 30 ), postprandial, and post-breakfast glucose compared with control diets ( 26 , 30 ) ( Table 2 ). However, the pooled analysis of the DASH diet showed significant favorable modifications in several outcomes, including change in fasting ( 22 , 36 ) and postprandial glucose ( 22 ), HOMA-IR ( 35 ), HbA 1c ( 22 ), medication need ( 22 , 23 , 36 ), infant birth weight ( 23 , 36 ), and macrosomia ( 23 , 36 ) ( Tables 2 and 3 ). Last, pooled analysis of the soy protein–enriched diet demonstrated a significant decrease in medication use and birth weight ( 14 , 27 ) ( Tables 2 and 3 ). One soy–protein intervention ( n = 68 participants) described significantly lower HOMA-IR ( 27 ) ( Table 2 ).
Sensitivity analysis of primary maternal glycemic and infant birth weight outcomes
Behavioral (one study) and ethnic-specific modified dietary interventions (one study) were included. The behavioral change dietary intervention reported significant differences in change in postprandial glucose and in HbA 1c ( Table 2 ) ( 24 ). The ethnic diet study demonstrated a significantly larger decrease in fasting and postprandial glucose ( Table 2 ) ( 34 ). Fat-modification, low-carbohydrate, and energy-restriction diets were not associated with a significant difference in our primary outcomes in the stratified analysis.
Secondary Outcomes
Weight gain from inclusion was lower for low-carbohydrate diets and cesarean birth for DASH diets ( Supplementary Table 2 ). Specific diet interventions did not show significant between-group differences in maternal gestational weight gain throughout pregnancy, preeclampsia/eclampsia, neonatal hypoglycemia as defined by the authors, preterm birth, neonatal intensive care unit admission, or small-for-gestational-age newborns ( Supplementary Tables 2 and 3 ).
Sensitivity Analysis of Primary Outcomes
Sensitivity analysis was performed to explore reasons for heterogeneity and to assess outcomes when studies with methodological concerns were removed. We were unable to include four studies ( 22 , 23 , 34 , 36 ), including all the DASH diet studies, where clarification of certain aspects of the results could not be obtained, even after a direct approach to the authors. The authors of the ethnic diet study responded to queries but did not provide the required information regarding gestational age at randomization ( 34 ). After these studies are removed, the changes in postprandial glucose (mean −5.90 mg/dL [95% CI −7.93, −3.88], I 2 = 0; P = 0.0001), post-breakfast glucose levels (−4.76 mg/dL [−9.13, −0.38], I 2 = 34; P = 0.03), and birth weight (−74.88 g [−144.86, −4.90], I 2 = 1; P = 0.04) remained significant when all diets were combined ( Table 3 ). Furthermore, the heterogeneity in most primary outcomes decreased after removal of these four studies.
When dietary subgroups were assessed, low-GI diets had significant differences in changes in fasting (mean −5.33 mg/dL [95% CI −6.91, −3.76]) ( 26 , 29 , 30 ), postprandial (−7.08 mg/dL [−12.07, −2.08]) ( 26 , 30 ), and post-breakfast (−8.6 mg/dL [−14.11, −3.09]) glucose ( 26 , 30 ). The soy protein–enriched diet had differences in change of HOMA-IR (mean −2.00 [95% CI −3.17, −0.83]) ( 27 ), required less medication use (RR 0.44 [95% CI 0.21, 0.91]), and had a lower birth weight (mean −184.67 g [95% CI −319.35, −49.98]) ( 14 , 27 ). The behavior modification diet had significant differences in change in postprandial glucose (mean −6.90 mg/dL [95% CI −9.85, −3.95]) and in HbA 1c (−0.19% [−0.26, −0.12]) ( 24 ) ( Table 3 ).
Assessment of Bias and Quality of the Evidence
None of the included studies were assessed as having a low risk of bias in all seven items of the Cochrane Collaboration tool ( Supplementary Fig. 2 ). Most studies were high risk for blinding of participants and personnel and for other sources of bias ( Supplementary Fig. 3 ). Studies scored high risk for other sources of bias for concerns such as baseline differences and industry funding. Most studies had an unclear risk of bias for selective outcome reporting and very few had registered protocols ( Supplementary Fig. 3 ).
GRADE assessment for the outcomes of interest reveals overall low to very low quality of evidence ( Supplementary Table 4 ). Considerations to downgrade quality of evidence involved the entire spectrum, including limitations in the study design, inconsistency in study results, and indirectness and imprecision in effect estimates.
Evaluation for Small Study Effect
Funnel plots of means and RRs of the primary outcomes for the main analysis are shown in Supplementary Figs. 4 and 5 and for the sensitivity analysis in Supplementary Figs. 6 and 7 . Overall, funnel plot asymmetry improves with the sensitivity analysis compared with the main analysis for neonatal birth weight outcomes.
In this meta-analysis, we pooled results from 18 studies including 1,151 women with a variety of modified dietary interventions. Remarkably, this is the first meta-analysis with a comprehensive analysis on maternal glucose parameters. Despite the heterogeneity between studies, we found a moderate effect of dietary interventions on maternal glycemic outcomes, including changes in fasting, post-breakfast, and postprandial glucose levels and need for medication treatment, and on neonatal birth weight. After removal of four studies with methodological concerns, we saw an attenuation of the treatment effect. Nonetheless, the change in post-breakfast and postprandial glucose levels and lowering of infant birth weight remained significant. Given the inconsistencies between the main and sensitivity analyses, we consider that conclusions should be drawn from the latter. These data suggest that dietary interventions modified above and beyond usual dietary advice for GDM have the potential to offer better maternal glycemic control and infant birth weight outcomes. However, the quality of evidence was judged as low to very low due to the limitations in the design of included studies, the inconsistency between their results, and the imprecision in their effect estimates.
Previous systematic reviews have focused on the easier-to-quantify outcomes, such as the decision to start additional pharmacotherapy and glucose-related variables at follow-up, but did not address change from baseline ( 15 – 17 ). The most recently published Cochrane systematic review by Han et al. ( 17 ) did not find any clear evidence of benefit other than a possible reduction in cesarean section associated with DASH diet. The very high-carbohydrate intake (∼400 g/day) and 12 servings of fruit and vegetables in the DASH diet ( 22 , 23 , 36 ) limit its clinical applicability and generalizability to women from lower socioeconomic, inner city backgrounds in Western countries. The Cochrane review shared one of our primary outcomes, large for gestational age ( 17 ). Neither meta-analysis detected a significant difference in risk of large for gestational age because the trials with a larger effect on birth weight (the three DASH studies) did not report on large for gestational age.
Our findings regarding pooled analysis of low-GI dietary interventions are broadly consistent with those of Viana et al. ( 16 ) and Wei et al. ( 15 ). Viana et al. ( 16 ) noted decreased birth weight and insulin use based on four studies of low-GI diet among 257 women (mean difference −161.9 g [95% CI −246.4, −77.4] and RR 0.767 [95% CI 0.597, 0.986], respectively). Wei et al. ( 15 ) also reported decreased risk of macrosomia with a low-GI diet in five studies of 302 women (RR 0.27 [95% CI 0.10, 0.71]). In our analyses of four studies in a comparable number of participants ( n = 276), we found the same direction of these effect estimates, without significant between-group differences. This is most likely due to the different studies included. For example, we were unable to obtain effect estimates stratified by type of diabetes in the study by Perichart-Perera et al. (which included women with type 2 diabetes) and therefore did not include this study ( 37 ). An important difference between our analyses and that of Wei et al. ( 15 ) is that they included DASH diet as a low-GI dietary subtype. We also included a recent study by Ma et al. ( 30 ) not included by the previous reviews.
Our sensitivity analyses highlighted concerns regarding some studies included in previous reviews. Notably, after removal of the studies with the most substantial methodological concerns in the sensitivity analysis, differences in the change in fasting plasma glucose were no longer significant. Although differences in the change in postprandial glucose and birth weight persisted, they were attenuated.
This review highlights limitations of the current literature examining dietary interventions in GDM. Most studies are too small to demonstrate significant differences in our primary outcomes. Seven studies had fewer than 50 participants and only two had more than 100 participants ( n = 125 and 150). The short duration of many dietary interventions and the late gestational age at which they were started ( 38 ) may also have limited their impact on glycemic and birth weight outcomes. Furthermore, we cannot conclude if the improvements in maternal glycemia and infant birth weight are due to reduced energy intake, improved nutrient quality, or specific changes in types of carbohydrate and/or protein.
We have not addressed the indirect modifications of nutrients. For example, reducing intake of dietary carbohydrates to decrease postprandial glucose may be compensated by a higher consumption of fat potentially leading to adverse effects on maternal insulin resistance and fetal body composition. Beneficial or adverse effects of other nutrients such as n-3 long-chain polyunsaturated fatty acid, vitamin D, iron, and selenium cannot be ruled out.
Our study has important strengths and weakness. To our knowledge, ours is the first systematic review of dietary interventions in GDM comprehensively examining the impact of diet on maternal glycemic outcomes assessing the change in fasting and postprandial glucose, HbA 1c , and HOMA-IR from baseline. This is especially important given that groups were not well balanced at baseline. Our review also benefits from the rigorous methodology used as well as the scientific, nutritional, and clinical expertise from an international interdisciplinary panel. However, it also has limitations. Baseline differences between groups in postprandial glucose may have influenced glucose-related outcomes. Furthermore, three of the included trials were pilot studies and therefore not designed to find between-group differences ( 12 , 26 , 34 ). The low number of studies reporting on adherence clearly illustrates that the quality of the evidence is far from ideal. The heterogeneity of the dietary interventions even within a specific type (varied macronutrient ratios, unknown micronutrient intake, and short length of some dietary interventions) and baseline characteristics of women included (such as prepregnancy BMI or ethnicity) may have also affected our pooled results. It should also be noted that the relatively small numbers of study participants limit between-diet comparisons. Last, we were unable to resolve queries regarding potential concerns for sources of bias because of lack of author response to our queries. We have addressed this by excluding these studies in the sensitivity analysis.
Modified dietary interventions favorably influenced outcomes related to maternal glycemia and birth weight. This indicates that there is room for improvement in usual dietary advice for women with GDM. Although the quality of the evidence in the scientific literature is low, our review highlights the key role of nutrition in the management of GDM and the potential for improvement if better recommendations based on adequately powered high-quality studies were developed. Given the prevalence of GDM, new studies designed to evaluate potential dietary interventions for these women should be based in larger study groups with appropriate statistical power. As most women with GDM are entering pregnancy with a high BMI, evidence-based recommendations regarding both dietary components and total energy intake are particularly important for overweight and obese women. The evaluation of nutrient quality, in addition to their quantity, as well as dietary patterns such as Mediterranean diet ( 39 ) would also be relevant. In particular, there is an urgent need for well-designed dietary intervention studies in the low- and middle-income countries where the global health consequences of GDM are greatest.
H.R.M. and R.C. contributed equally to this work.
See accompanying commentary, p. 1343 .
See accompanying articles, pp. 1337 , 1339 , 1362 , 1370 , 1378 , 1385 , 1391 , and e111 .
Funding. H.R.M. was funded by the U.K. National Institute for Health Research (CDF 2013-06-035). This work was conducted by an expert group of the European branch of the International Life Sciences Institute (ISLI Europe). This publication was coordinated by the ISLI Europe Early Nutrition and Long-Term Health and the Obesity and Diabetes task forces. Industry members of these task forces are listed on the ILSI Europe website at www.ilsi.eu . Experts are not paid for the time spent on this work; however, the nonindustry members within the expert group were offered support for travel and accommodation costs from the Early Nutrition and Long-Term Health and the Obesity and Diabetes task forces to attend meetings to discuss the manuscript and a small compensatory sum (honoraria) with the option to decline. The expert group carried out the work, i.e. collecting and analyzing data and information and writing the scientific paper, separate to other activities of the task forces. The research reported is the result of a scientific evaluation in line with ILSI Europe’s framework to provide a precompetitive setting for public-private partnership. ILSI Europe facilitated scientific meetings and coordinated the overall project management and administrative tasks relating to the completion of this work.
The opinions expressed herein and the conclusions of this publication are those of the authors and do not necessarily represent the views of ILSI Europe nor those of its member companies. For further information about ILSI Europe, please email [email protected] or call +32 2 771 00 14.
Duality of Interest. E.M.v.d.B. works part-time for Nutricia Research. E.C.-G. works full-time for Nestec. R.R. works full-time for Abbott Nutrition. No potential conflicts of interest relevant to this article were reported.
Author Contributions. J.M.Y. contributed to data extraction, statistical analyses, and writing the first draft manuscript. J.E.K. contributed to data extraction and writing the first draft summary tables. M.B. and A.G.-P. contributed to literature extraction, statistics, and manuscript revision. E.H. contributed to data extraction and GRADE assessments. I.S. and I.G. contributed to statistics and manuscript revision. E.M.v.d.B., E.C.-G., S.H., and S.F.O. contributed to concept and design, data extraction, and manuscript review. M.H. contributed to concept and design and draft manuscript evaluation. K.L. contributed to concept and design, data extraction, and critical review for intellectual content. L.P. contributed to concept and design and manuscript review. R.R., P.R., and H.R.M. contributed to concept and design, data extraction, and revising the draft manuscript. L.v.L. contributed to data extraction and draft summary tables. B.S. contributed to data extraction and critical review for intellectual content. R.C. contributed to literature extraction, statistical analyses, and revising the draft manuscript. R.C. is the guarantor of this work and, as such, had full access to all the data in the study and takes responsibility for the integrity of the data and the accuracy of the data analysis.
Prior Presentation. Parts of this work were presented at the Diabetes UK National Diabetes in Pregnancy Conference, Leeds, U.K., 14 November 2017, and the XXIX National Congress of the Spanish Society of Diabetes, Oviedo, Spain, 18–20 April 2018.
Supplementary data
Email alerts.
- Gestational Diabetes Mellitus: Does One Size Fit All? A Challenge to Uniform Worldwide Diagnostic Thresholds
- A Tailored Letter Based on Electronic Health Record Data Improves Gestational Weight Gain Among Women With Gestational Diabetes Mellitus: The Gestational Diabetes’ Effects on Moms (GEM) Cluster-Randomized Controlled Trial
- Day-and-Night Closed-Loop Insulin Delivery in a Broad Population of Pregnant Women With Type 1 Diabetes: A Randomized Controlled Crossover Trial
- Gestational Diabetes Mellitus and Renal Function: A Prospective Study With 9- to 16-Year Follow-up After Pregnancy
- Neonatal Hypoglycemia Following Diet-Controlled and Insulin-Treated Gestational Diabetes Mellitus
- The Human Placenta in Diabetes and Obesity: Friend or Foe? The 2017 Norbert Freinkel Award Lecture
- Nutrition Therapy in Gestational Diabetes Mellitus: Time to Move Forward
- Gestational Diabetes Mellitus: Is It Time to Reconsider the Diagnostic Criteria?
- The Sensitivity and Specificity of the Glucose Challenge Test in a Universal Two-Step Screening Strategy for Gestational Diabetes Mellitus Using the 2013 World Health Organization Criteria
- In This Issue of Diabetes Care
- Online ISSN 1935-5548
- Print ISSN 0149-5992
- Diabetes Care
- Clinical Diabetes
- Diabetes Spectrum
- Standards of Medical Care in Diabetes
- Scientific Sessions Abstracts
- BMJ Open Diabetes Research & Care
- ShopDiabetes.org
- ADA Professional Books
Clinical Compendia
- Clinical Compendia Home
- Latest News
- DiabetesPro SmartBrief
- Special Collections
- DiabetesPro®
- Diabetes Food Hub™
- Insulin Affordability
- Know Diabetes By Heart™
- About the ADA
- Journal Policies
- For Reviewers
- Advertising in ADA Journals
- Reprints and Permission for Reuse
- Copyright Notice/Public Access Policy
- ADA Professional Membership
- ADA Member Directory
- Diabetes.org
- X (Twitter)
- Cookie Policy
- Accessibility
- Terms & Conditions
- Get Adobe Acrobat Reader
- © Copyright American Diabetes Association
This Feature Is Available To Subscribers Only
Sign In or Create an Account
- Research article
- Open access
- Published: 07 February 2020
Women’s experiences of a diagnosis of gestational diabetes mellitus: a systematic review
- Louise Craig 1 ,
- Rebecca Sims 1 ,
- Paul Glasziou 1 &
- Rae Thomas ORCID: orcid.org/0000-0002-2165-5917 1
BMC Pregnancy and Childbirth volume 20 , Article number: 76 ( 2020 ) Cite this article
26k Accesses
63 Citations
46 Altmetric
Metrics details
Gestational diabetes mellitus (GDM) - a transitory form of diabetes induced by pregnancy - has potentially important short and long-term health consequences for both the mother and her baby. There is no globally agreed definition of GDM, but definition changes have increased the incidence in some countries in recent years, with some research suggesting minimal clinical improvement in outcomes. The aim of this qualitative systematic review was to identify the psychosocial experiences a diagnosis of GDM has on women during pregnancy and the postpartum period.
We searched CINAHL, EMBASE, MEDLINE and PsycINFO databases for studies that provided qualitative data on the psychosocial experiences of a diagnosis of GDM on women across any stage of pregnancy and/or the postpartum period. We appraised the methodological quality of the included studies using the Critical Appraisal Skills Programme Checklist for Qualitative Studies and used thematic analysis to synthesis the data.
Of 840 studies identified, 41 studies of diverse populations met the selection criteria. The synthesis revealed eight key themes: initial psychological impact; communicating the diagnosis; knowledge of GDM; risk perception; management of GDM; burden of GDM; social support; and gaining control. The identified benefits of a GDM diagnosis were largely behavioural and included an opportunity to make healthy eating changes. The identified harms were emotional, financial and cultural. Women commented about the added responsibility (eating regimens, appointments), financial constraints (expensive food, medical bills) and conflicts with their cultural practices (alternative eating, lack of information about traditional food). Some women reported living in fear of risking the health of their baby and conducted extreme behaviours such as purging and starving themselves.
A diagnosis of GDM has wide reaching consequences that are common to a diverse group of women. Threshold cut-offs for blood glucose levels have been determined using the risk of physiological harms to mother and baby. It may also be advantageous to consider the harms and benefits from a psychosocial and a physiological perspective. This may avoid unnecessary burden to an already vulnerable population.
Peer Review reports
Gestational diabetes mellitus (GDM) is diagnosed by elevated blood glucose in pregnancy though the definition has changed repeatedly since its first description in the 1960’s [ 1 , 2 ]. The most frequently reported perinatal consequence of GDM is macrosomia (usually defined as a neonate weighing over 4 kg) which can increase the risk of caesarean section and shoulder dystocia. For the mother, there are also potential longer-term consequences including an increased risk of type 2 diabetes post-pregnancy and/or in later life [ 3 ]. The investigators of a large international Hyperglycemia and Adverse Pregnancy Outcome (HAPO) study aimed to identify a cut-point in the continuum to decide the blood glucose level (BGL) thresholds that should be used to define GDM [ 4 ]. However, a definitive cut-point was not identified and using the HAPO data the International Association of the Diabetes and Pregnancy Study Groups (IADSPG) consensus panel recommended a BGL threshold associated with the risk of adverse infant outcomes (such as risk of macrosomia, excess infant adiposity and neonatal hyperinsulinemia) [ 5 ]. This change was controversial, and there is currently a lack of an agreed standard for diagnosing high blood glucose in pregnancy.
Pregnancy can be a vulnerable period when a woman is adapting and responding to changes in body perceptions, such as loss of strength or fitness, which can result in reduced self-esteem and depression [ 6 ]. Many women report depression and anxiety during pregnancy which often includes worry for the baby’s wellbeing [ 7 , 8 ]. A diagnosis of a health condition such as GDM could have a detrimental effect on a pregnant woman’s quality of life due to fears that the illness may affect her and/or her baby [ 9 ]. This has potential to convert pregnancy, a natural process, into one associated with risks, ill-health and increased surveillance [ 10 ]. Understanding a women’s response to the GDM diagnosis and its psychological impact has emerged as an important issue [ 11 ]. Some studies report women describing the initial response as one of ‘shock’ [ 12 , 13 ], ‘sadness’ and ‘guilt’ [ 13 ]. A women’s acceptance of risk and fear of complications is likely to influence the acceptability of various interventions. Therefore, it is imperative to amalgamate the findings of these studies to synthesise the array of potential psychosocial consequences of a diagnosis of GDM.
In many countries the prevalence of GDM is rising [ 14 , 15 , 16 ]. Some of this is due to the increasing age at which women are becoming pregnant, an increase in obesity amongst women, more testing during pregnancy, and better recording during pregnancy. However, much of the rise has occurred since 2013 when some countries adopted the new IADPSG criteria and testing regimen for gestational diabetes. This resulted in the anomalous position that two women in two countries with exactly the same glucose levels may or may not be diagnosed with GDM depending on the country’s definition. Caution had been previously raised that the new IADPSG definition would increase prevalence of women diagnosed with GDM by two-to-three-fold [ 17 ].
Despite a significant increase in prevalence of GDM after the introduction of the new IADPSG criteria [ 15 , 16 ], some pre-post studies suggest negligible clinical improvement in the adverse outcomes measured [ 17 , 18 ]. Findings from a qualitative study of 19 women of different cultural backgrounds investigating women’s experiences of a GDM diagnosis reported that the diagnostic criteria itself was viewed as ‘confusing’ by some women and treatment for their ‘borderline’ condition unnecessary [ 19 ].
Although multiple studies have considered the impact of a diagnosis of GDM, a systematic review to synthesise the evidence around the emotional impact of a diagnosis at different stages, i.e. time of diagnosis, after diagnosis, at the delivery of the baby, and post-delivery, is lacking. The findings could inform healthcare clinicians of women’s attitudes and the consequences of a diagnosis and illuminate potential opportunities to provide support and advise. Therefore, in this systematic review, we aim to synthesise the evidence of the psychosocial experiences a diagnosis of GDM has on women during pregnancy and the postpartum period.
We followed the Enhancing Transparency in Reporting the Synthesis of Qualitative Research Guidelines (ENTREQ; Additional file 1 : Table S1) [ 20 ]. We included primary studies published in peer-review journals that:
included pregnant women with a current diagnosis or women with a history of GDM;
provided qualitative data on the psychosocial experiences of a diagnosis of GDM on women across any stage of pregnancy and/or the postpartum period; and
where participants have provided an account of their experience or perspective of living with GDM
No restrictions were placed on country, written language, or year of publication.
Studies were excluded, if:
the primary aim was to identify barriers and/or facilitators to service as these focused on the management of GDM rather than the GDM diagnosis; or
participants were women diagnosed with diabetes before pregnancy
Abstracts, letters, editorials and commentaries were also excluded.
Search methods for identification of studies
The search strategy (MEDLINE version provided in the Additional file 1 ) was developed using a combination of Medical Subject Headings terms centred around three key areas: i) gestational diabetes mellitus ii) diagnostic testing for gestational diabetes mellitus and iii) patient experiences. The Systematic Review Accelerator software was used to translate the search strategy for each of the different databases and to remove duplicated articles [ 21 ]. We searched CINAHL, EMBASE, MEDLINE and PsycINFO databases from inception to April 2018. Forward and backward citation searching of included studies was conducted.
Selection process
A single reviewer (LC) screened the titles and abstracts of retrieved references using Endnote Version X7.7.1. Potentially eligible full-texts were independently reviewed by LC and RS with conflicts resolved via discussion. Two full-text studies published in Portuguese were first translated using Google Translate and then validated by a researcher with both spoken and written Portuguese language skills located within our research network.
Data extraction
All data labelled as results or findings including themes, categories, theories were extracted and imported into NVivo Version 12 by LC. Study characteristics were extracted by LC which included study location, reported research aims, study design, methodology and the analytical approach. Information about the diagnostic criteria used to determine GDM in women was also extracted.
Data synthesis and analysis
To synthesise the findings, we used a thematic synthesis described by Thomas and Harden [ 22 ]. Thematic synthesis has the potential for conclusions to be drawn based on common aspects across otherwise heterogeneous studies and produce findings that directly inform health practitioners [ 22 , 23 ]. Coding was inductive, with codes derived from the data. First, extracted text relevant to patient experiences and perspectives was coded line by line. A subset of studies ( n = 5) were coded independently by LC and RS to develop a coding framework. Disagreements were resolved by discussion. LC and RS coded a further subset ( n = 4) and established an inter-rater reliability of Kappa = 0.87. Following this, LC applied the coding framework to the remaining studies. New codes were iteratively developed as new concepts arose.
Second, relationships between the codes were identified by LC to form the basis of descriptive themes across the studies. Similar codes were grouped to generate themes and less frequently used codes were classified into sub-themes. In the final stage, analytical themes were developed to ‘go beyond’ the primary studies to amalgamate and interpret the findings. The relevant quotes to support each theme were tabulated.
Quality assessment
As recommended by the Cochrane Qualitative Research Methods Group, we assessed the quality of the included studies using the Critical Appraisal Skills Programme Qualitative Checklist (CASP). This tool uses a systematic approach to appraise three key areas: study validity, an evaluation of methodological quality, and an assessment of external validity [ 24 ]. Critical appraisal was conducted by one reviewer (LC) for all studies, with second reviewer appraisal (RS) for a sub-set of included papers. The findings from the two reviewers were compared and any contrasting items were discussed and re-reviewed.
The search identified 840 studies. After deduplication and screening of titles and abstracts 88 full-text articles were assessed (Fig. 1 ). Seven further articles were identified through citation searching. Data were extracted from 41 studies meeting eligibility criteria and were included in the review [ 11 , 12 , 13 , 19 , 25 , 26 , 27 , 28 , 29 , 30 , 31 , 32 , 33 , 34 , 35 , 36 , 37 , 38 , 39 , 40 , 41 , 42 , 43 , 44 , 45 , 46 , 47 , 48 , 49 , 50 , 51 , 52 , 53 , 54 , 55 , 56 , 57 , 58 , 59 , 60 , 61 ].
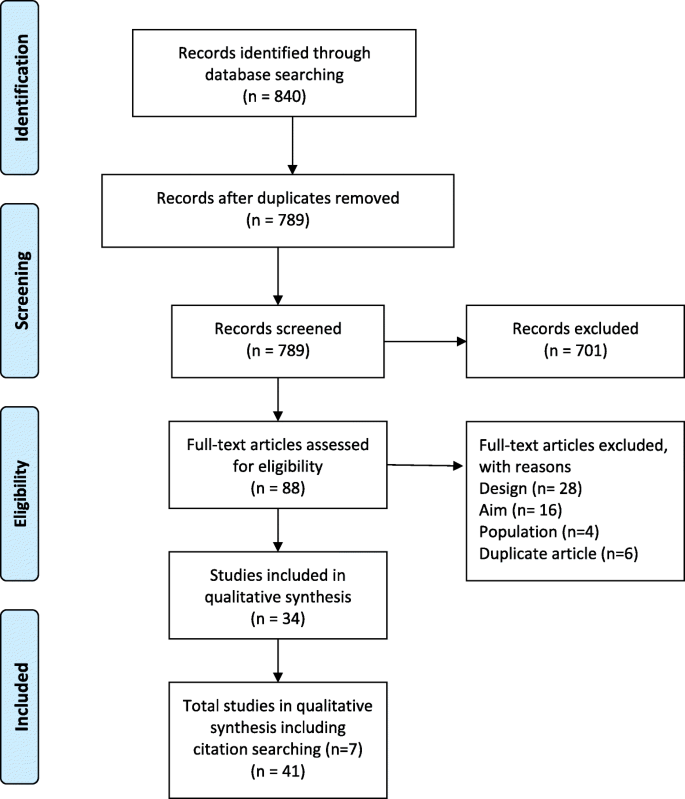
Prima flow diagram
Study characteristics
The studies reflected a variety of sampling methods and data collection methods. For example, interviews were conducted in 34 studies [ 10 , 12 , 13 , 25 , 27 , 28 , 30 , 31 , 32 , 34 , 35 , 36 , 38 , 39 , 40 , 41 , 42 , 43 , 44 , 45 , 46 , 47 , 48 , 49 , 50 , 52 , 53 , 54 , 55 , 56 , 57 , 58 , 60 , 61 ], focus group methods were used in three [ 19 , 32 , 37 ], and interviews and focus groups were used in two studies [ 29 , 51 ]. Two studies used a mixed method approach [ 26 , 59 ]. The sample sizes ranged from 6 to 57 women. Eighteen studies were conducted in Europe, 10 in Australia, 9 in North America, and 2 studies each in Asia and South America. Table 1 details the characteristics of the included studies.
Quality appraisal
Most studies were assessed as high quality (Additional file 1 : Table S2). Study aims were stated in all but one study [ 47 ]. As the purpose of all included studies was to explore or gain knowledge, opinions or attitudes about GDM, the qualitative methods employed in all the studies were appropriate. Different study designs were used and in some cases the lack of reporting details made judgments of the appropriateness of study methods difficult. Data were collected in a way that addressed the research issue, however, a few authors did not discuss or report details such as saturation of data [ 31 , 47 , 56 , 59 ]. The relationship between researcher and participants was considered in only two studies [ 51 , 61 ]. Appropriateness of data analysis was assessed as “unclear” when there was a lack of details about how themes were derived.
Thematic analyses
Eight themes were generated from the data synthesis: 1. initial psychological impact; 2. communicating the diagnosis; 3. knowledge of GDM; 4. risk perception; 5. management of GDM; 6. burden of GDM; 7. social support; and 8. gaining control. The relevant quotes to support each theme are presented in Table 2 .
Initial psychological impact
When initially diagnosed with GDM, most women reported reactions such as self-blame, failure, fear, sadness, concern and confusion. Women often focused on the uncertainty of diagnostic prognosis and some considered it to be a life-altering experience. Some women felt lost and unsure what to do next. Often women felt an overwhelming sense of vulnerability and guilt. In some cases, the diagnosis was received positively and was viewed as an opportunity for lifestyle improvements. For example, some women viewed the diagnosis as a ‘ wake up’ call and were grateful for the chance to intervene and potentially prevent adverse outcomes for their babies and themselves. Some women viewed gaining less weight than expected during their pregnancy as a benefit of having a GDM diagnosis.
Communicating the diagnosis
Communication with healthcare professionals (HCPs) and their families was a common theme throughout the findings of the included studies. Generally, the level and quality of communication with HCPs was mixed – with some women reporting positive and informative encounters, while others described brief encounters with overly technical language and unsupportive consultations. The main issues were limited time available to spend with the HCP, lack of continuity of care and lack of understanding about the role of the HCP at follow-up. In some instances, women felt that GDM was not a topic that HCPs were keen to discuss - ‘the nurses, they never talked to me about my gestational diabetes’. [ 23 ] The level and quality of information provided was often conflicting, confusing or insufficient. Areas of contention were appropriate foods and the dietary changes that should be made.
Some women formed a dependency on HCPs to know what to do and on the electronic reminders for follow-up appointments and monitoring. Often women reported having no choice in treatment resulting in them feeling threatened and frustrated. Often women were automatically booked in for a caesarean section without consultation or lived in fear of this occurring. One woman referred to GDM as being over medicalised. Receiving limited information also prompted women to independently seek information about the impact and management of GDM from other sources such as the internet. However, some women found the internet limited for specific information or confusing.
Knowledge of GDM
Women had varying levels of understanding of GDM which impacted on their initial reaction to the diagnosis. Those who were able to explain the cause of GDM were able to process and accept the diagnosis more readily than those who had little understanding of GDM, or were confused as to how GDM occurred. Lack of knowledge also extended to and impacted on relatives. Some women stated that they would have preferred to be more prepared to receive the diagnosis by having early knowledge about the testing for diabetes. Women reported being on a steep learning curve, especially the onerous approach of dietary trial and error whereby women learnt what foods would increase their blood glucose level (BGL) and what food to avoid. Women also reported challenges in adopting new habits to manage their GDM, including understanding food labels and nutritional values of food. Often this required a trial and error approach. There was also a lack of understanding about the impact of GDM on their baby with some women believing it would be transmitted to their baby via breastmilk.
Risk perception
Women’s perception of risk were reported before the diagnosis of GDM, after they were diagnosed in pregnancy, and after the delivery. Some women attempted to understand their level of risk in context of family history. Some were very surprised by the diagnosis, especially if they were asymptomatic; and some women found it difficult to come to terms with the diagnosis. There was uncertainty about the severity of the condition. Some women considered the condition to be mild, downplaying the disease and believing that too much ‘ fuss’ was being made about GDM and other women doubted the diagnosis and its seriousness. Women often discussed: the adverse effects that GDM would have on her baby; frustration that the focus was on risks to the baby and less so them; their worry about the consequences for the future; and questioned the impact of insulin on the baby. Women worried that their diet was too restrictive for their growing baby and would not provide the nutrients that the baby required. Some women held the view that GDM was a temporary condition and would disappear once the baby was born, and many women reverted to old eating habits after the baby’s birth. Often women referred to the birth as a ‘ moment of truth ’ or as an endpoint to their GDM. This was also reflected in the level of care that the women received after the birth of their baby.
Managing GDM
Dietary management-related stress was commonly reported amongst interviewed women and was experienced by both insulin and non-insulin users. Stress and frustrations often occurred as a consequence of an unexpected abnormal blood glucose reading following strict adherence to dietary advice. Maintaining stable BGL was an ongoing struggle and in some cases the burden proved too much, with a few women ceasing employment. Insulin users described the process as a ‘ roller coaster ’ as well as the emotional and physical discomfort of injecting, while non-insulin users often became obsessed with a well-controlled diet, with some women viewing this as a way to avoid the use of insulin. Conversely, some women felt relieved when they were transitioned onto insulin, as it reduced the need for dietary restriction.
Making lifestyle changes was considered stringent and restrictive by the majority of women, and for some required ‘ major restructuring’ to their diet and daily routines to incorporate exercise. Some women reported extreme behaviours, including falsifying blood glucose readings, self-starvation and hiding their condition, including from family members. Often the impact of non-adherence to lifestyle changes resulted in guilt and belief that the baby would know they have cheated. Other pregnancy related ailments and the need to care for other children interfered with the ability to make the required changes. Women who had a specific culture-related diet discussed the impact and difficulty of applying or tailoring the dietary recommendations to their diet.
The key motivator to making required lifestyle changes, despite the associated hardships, was to minimise the risks to their unborn baby. Women prioritised the health of the baby over their own health and were willing to do anything to ensure that the health of their baby was not compromised. Over time, management of the GDM became a part of their normal routine for many women. However, some women expressed a desire to have a ‘ normal’ pregnancy similar to their friends, discussing that a diagnosis of GDM made them feel as though their pregnancy was atypical, leading to defining their pregnancy as ‘ abnormal ’ or as an ‘ illness ’. For one woman, it made her feel like an ‘ illegal’ person.
Burden of GDM
Women reported that a diagnosis of GDM came with extra responsibility, which added pressure whilst trying to juggle life commitments such as work, childcare, and daily living responsibilities. Monitoring and treating GDM placed burden on women’s daily routines and most woman agreed that taking BGL measurements was time consuming and disruptive. There was a constant need to prudently plan meals and co-ordinate the attendance at additional hospital appointments, all of which were considered time intensive, especially with travel and wait times. Women expressed that GDM consumed a lot of their thinking time e.g., ‘ I think about diabetes everyday’ and felt that they had to acknowledge GDM all the time and became ‘ super-conscious’ . In some instances, women reported a GDM diagnosis took away some of the ‘ joy of pregnancy ’ . One woman described her pregnancy as a ‘ misfortune’ . Women mentioned the financial burden of buying healthier food – ‘it would take lots of money just because it is so expensive to eat healthy’. [ 25 ] Women also considered the physical burden of GDM such as fatigue and the side effects of treatment such as insulin. There was a longer-term impact on family planning, where in some cases women decided not to have another child because they were fearful of enduring a similar restrictive and stressful pregnancy due to GDM.
Social support
Social support, including family and HCP support, was an important aspect for women during their experience of a GDM diagnosis. Changes in lifestyle often had an overflow effect, with other family members adopting healthier lifestyles. Women not in their country of birth, and without family, often reported feeling isolated and lonely. Disappointment and isolation were also expressed by some women when they perceived a lack of healthcare system support. This often occurred postnatally when the expectations of postpartum care were high, however, in reality, support was absent. In some cases, women were stigmatised by their families and in a few cases received undesirable feedback that they were not doing enough to protect their unborn child.
Gaining control
Control was a frequently used word when women described living with and managing a GDM diagnosis. Initially women reported a lack of control especially over their emotions, however, over time women transitioned from feeling like a victim of diabetes, to being active agents in controlling their GDM. The terms ‘ balance’ and ‘ adjustment’ were used to describe how some women tried to offset the strict compliance and active self-management with reducing their risk to their unborn baby and their own future risk of developing diabetes after pregnancy. Some women reported feeling empowered as their pregnancies progressed, especially when they gained more knowledge about GDM and what action they could take to accept and make sense of the diagnosis. Taking control included realising the changes that were required to their lifestyle, self-initiated care, and self-education. Often investigating alternative options, such as natural remedies outside those recommended by HCPs, provided women with some autonomy in managing their condition and some believed that it was a safer option to medication.
Summary of main findings
This synthesis of the qualitative evidence of women’s experiences of being diagnosed with GDM highlighted the psychosocial consequences a diagnosis of GDM can have on women. The purported benefits of a GDM diagnosis identified from our review, were largely behavioural and included an opportunity to improve health, prevent excessive weight gain, control weight during pregnancy, and prompts to make healthy eating changes. However, the purported harms included the added responsibility (eating regimens, appointments), financial constraints (expensive food, medical bills), and conflicts with their cultural practices (alternative eating, lack of information about traditional food). The psychosocial consequences were wide reaching and often resulted in significant social isolation with women only sharing their diagnosis with partners. Furthermore, there were a few reports of over-medicalisation due to a GDM diagnosis, with the perception that HCPs were often authoritarian, focusing on physiological aspects, with little attempt to involve women in decision making. This is noteworthy considering a non-GDM pregnancy has already come under scrutiny as being over-medicalised with increasing levels of unnecessary intervention [ 62 ].
Women from studies included in our review frequently reported inconsistent information provision. Limited GDM information provision has been identified in another systematic review regarding healthcare seeking for GDM during the postpartum period [ 63 ]. In contrast, findings from another study which aimed to evaluate satisfaction with obtaining a diagnosis of GDM concluded that the majority of women were satisfied with their experience of being diagnosed [ 64 ]. Further, women in the latter study associated poor GDM control with perinatal complications and an increased risk of type 2 diabetes following pregnancy [ 64 ].
Another key finding from this review was low awareness of the potential risks of GDM, particularly in the long-term. Low health literacy levels could be one factor to explain knowledge deficits and understanding of GDM, especially given the sociodemographic diverse population included in this review. One study found that low literacy among disadvantaged women had a significant impact on their understanding of GDM information [ 65 ]. Other research found that women who live in an English-speaking country but primarily speak a non-English language, have lower rates of dietary awareness compared with their English speaking counterparts, and this may affect compliance to dietary interventions [ 66 ]. Therefore, it is important that new educational interventions are developed to target those with lower health literacy as well as cultural factors when diagnosing and managing multi-ethnic populations with GDM [ 66 ].
Interestingly, women with a borderline diagnosis of GDM did not seem as concerned as other women and in some cases were dismissive of the diagnosis and the potential consequences. Similarly, in a study which specifically included women with a borderline diagnosis of GDM, the majority of women reported that they were not worried by the diagnosis [ 67 ]. For some women, the potential transitory nature of GDM was emphasised and some reported that it didn’t seem like a real illness. The diagnostic criteria for GDM has previously been compared with the established criteria used to classify a condition as a disease. This comparison revealed disparity which Goer, in 1996, used to suggest that GDM did not pose a serious health risk, was neither easily nor accurately diagnosed, was not treated effectively and that treatment outweighed the risks of the condition [ 68 ]. Therefore, the levels of heightened psychological distress as reported by the women in our review, may actually be unnecessary and others have gone as far as saying that GDM is an example of ‘obstetric iatrogenesis’ [ 69 ].
The findings of this review did underline a few unmet service needs with recurring themes around the lack of individualised care and its continuity, lack of choice regarding important aspects of care such as birthing options, and the scarcity of comprehensive follow-up. There was a sense of abandonment amongst women after delivery in that they had experienced intensive intervention and then nothing. This could be viewed as a missed opportunity to capitalise on the motivation to make changes during pregnancy. Researchers have previously highlighted that adherence to postpartum screening and continued lifestyle modifications to prevent future diabetes seems to dissipate after birth, possibly because the driver to protect their unborn child is no longer there [ 70 ].
The studies included in our review had participants of varying cultures sampled from countries with different GDM definitions. However, there appeared no difference in the qualitative outcomes between studies/countries. In our review, the experiences of women diagnosed with GDM suggest psychosocial harms appear to outweigh the qualitative benefits. Quantitative studies [ 14 , 15 ] that report prevalence increases in GDM after the IADSPG [ 71 ] definition changed, also report minimal improvements to maternal and infant physical outcomes.
This synthesis of women’s experiences of a GDM diagnosis could be used to inform the content of communication materials both before and after a GDM diagnosis. For example, an awareness of GDM testing and basic information including cultural adaptations to dietary guidelines and addressing misconceptions around breastfeeding. There is also an opportunity for HCPs to use teachable moments with women who have been identified at risk of developing type 2 diabetes post-pregnancy and offer supportive, effective advice about lifestyle changes. This is particularly relevant considering a previous review highlighted a significant time is spent in sedentary behaviour during pregnancy [ 72 ]. A study which examined HCPs views of healthcare provision to women with GDM showed that HCPs themselves perceived that there was a shortfall in GDM education [ 73 ]. There are also signals for service improvement and potential for service redesign, such as increasing community-delivered care for women diagnosed with GDM. This would assist in alleviating the burden on women to attend hospital appointments and potentially offer flexible appointment times. Follow-up appointments post-pregnancy could be made with consideration of other appointments such as maternal and child health milestones and breastfeeding weaning classes, and could also focused on healthy eating for both mother and baby.
Strengths and limitations
This systematic review included studies with women of different demographic characteristics and multicultural samples. The themes identified were represented in the majority of studies which increased the internal validity. The relatively high participation rate in the included studies, and that most studies were conducted during pregnancy or shortly after delivery, contributes to the external validity of our study. Although some participants were interviewed antenatally and some postnatally, this distribution over different gestational stages assists the generalisability of the study findings.
The comparison of coding between authors, discussion of the results and reaching consensus was a robust approach to improve the credibility of the results. Overall, the quality of most studies was good, however, a third of the studies used convenience methods to recruit participants which could contribute to sampling bias and limit the external validity of our findings. Only two studies adequately described the facilitator’s prior experience and the relationship between the participants and the facilitator/researcher. Unfortunately, this review did not capture the perception of HCPs which might be used to explain some of the behaviours and attitudes of the women, particularly in relation to communication of the diagnosis and information provision. Finally, although the data were collected from diverse populations, the majority of the countries in which research were conducted in were high-income countries, which could be considered to have more established and evidence-based healthcare systems than low-income countries.
Further research
A previous study has suggested the need for more research on the benefits and harms of alternative treatment choices for women with GDM [ 33 ]. The findings from this review suggest a need for more investigation around the psychosocial benefits and harms of a diagnosis of GDM. Given some women viewed treatment of ‘borderline GDM’ as unimportant, a new model of care based on stratification or individual level of risk for pregnancy and birth complications could be further explored. This may reduce the need for all women to be labelled as having GDM and negate unnecessary anxiety and burden for those at the lower ‘borderline’ threshold. This would then potentially offer tailored treatment options, improve shared-decision making, and improve women’s knowledge about how a diagnosis of GDM might affect them.
Consequences of a GDM diagnosis are multidimensional and highly contextual. Despite the psychosocial challenges frequently experienced, many women (driven by the innate response to safeguard their unborn baby) were able to gradually adapt to the required lifestyle changes and monitoring regimens. Perhaps a question is whether some of them should have to. There is opportunity to improve lifestyle and to assist the prevention of diabetes after pregnancy, however, this needs to be managed alongside the potential harms of a GDM diagnosis such as the negative psychological impact and social isolation. In the context of rising prevalence [ 14 , 15 , 16 , 17 ], potential minimal clinical [ 14 , 15 , 16 ] improvements, and the wide range of psychosocial experiences identified in this study, the findings of this review highlight the need for HCPs to consider the implications that a GDM diagnosis may have on women. It is essential that women diagnosed with GDM receive consistent evidence-based information and ongoing psychological and social support.
Availability of data and materials
The datasets generated during the current systematic review are available from the lead author upon request.
Abbreviations
Blood glucose level
Critical Appraisal Skills Programme Checklist (Qualitative)
Enhancing Transparency in Reporting the Synthesis of Qualitative Research
- Gestational diabetes mellitus
Hyperglycemia and Adverse Pregnancy Outcomes
Health care professional
International Association of the Diabetes and Pregnancy Study Groups
O'Sullivan JB, Mahan CM. Criteria for the oral glucose tolerance test in pregnancy. Diabetes. 1964;13:278–85.
CAS PubMed Google Scholar
Mishra S, Rao CR, Shetty A. Trends in the diagnosis of gestational diabetes mellitus. Scientifica (Cairo). 2016;2016:5489015.
Google Scholar
Kim C, Newton KM, Knopp RH. Gestational diabetes and the incidence of type 2 diabetes. Syst Rev. 2002;25(10):1862–8. https://doi.org/10.2337/diacare.25.10.1862 .
Article Google Scholar
HAPO Study Cooperative Research Group. Hyperglycaemia and adverse pregnancy outcomes. N Engl J Med. 2008;358:1991–2002.
McIntyre HD, Gibbons KS, Lowe J, Oats JJN. Development of a risk engine relating maternal glycemia and body mass index to pregnancy outcomes. Diabetes Res Clin Pract. 2018;139:331–8.
Article PubMed Google Scholar
Kamysheva E, Skouteris H, Wertheim EH, et al. Examination of a multi-factorial model of body-related experiences during pregnancy: The relationships among physical symptoms, sleep quality, depression, self-esteem, and negative body attitudes. Body Image. 2008;5(2):152–63 https://doi.org/10.1016/j.bodyim.2007.12.005 .
Carolan-Olah M, Barry M. Antenatal stress: an Irish case study. Midwifery. 2014;30(3):310–6. https://doi.org/10.1016/j.midw.2013.03.014 [published Online First: 2013/05/21].
Ferreira CR, Orsini MC, Vieira CR, et al. Prevalence of anxiety symptoms and depression in the third gestational trimester. Arch Gynecol Obstet. 2015;291(5):999–1003. https://doi.org/10.1007/s00404-014-3508-x [published Online First: 2014/10/15].
Dalfra MG, Nicolucci A, Bisson T, et al. Quality of life in pregnancy and post-partum: a study in diabetic patients. Qual Life Res. 2012;21(2):291–8. https://doi.org/10.1007/s11136-011-9940-5 .
Article CAS PubMed Google Scholar
Evans MK, O’Brien B. Gestational diabetes: the meaning of an at-risk pregnancy. Qual Health Res. 2005;15(1):66–81.
Devsam BU, Bogossian FE, Peacock AS. An interpretive review of women's experiences of gestational diabetes mellitus: proposing a framework to enhance midwifery assessment. Women Birth. 2013;26(2):E69–76.
Carolan-Olah M, Duarte-Gardea M, Lechuga J, et al. The experience of gestational diabetes mellitus (GDM) among Hispanic women in a U.S. border region. Sex Reprod Healthc. 2017;12:16–23. https://doi.org/10.1016/j.srhc.2016.11.003 .
Persson M, Winkvist A, Mogren I. ‘From stun to gradual balance’—women’s experiences of living with gestational diabetes mellitus. Scand J Caring Sci. 2010;24(3):454–62. https://doi.org/10.1111/j.1471-6712.2009.00735.x .
Cade TJ, Polyakov A, Brennecke SP. Implications of the introduction of new criteria for the diagnosis of gestational diabetes: a health outcome and cost of care analysis. BMJ Open. 2019;9(1):e023293–e93. https://doi.org/10.1136/bmjopen-2018-023293 .
Article PubMed PubMed Central Google Scholar
Sexton H, Heal C, Banks J, et al. Impact of new diagnostic criteria for gestational diabetes. J Obstet Gynaecol Res. 2018;44(3):425–31. https://doi.org/10.1111/jog.13544 [published Online First: 2018/01/13].
Erjavec K, Poljičanin T, Matijević R. Impact of the implementation of new WHO diagnostic criteria for gestational diabetes mellitus on prevalence and perinatal outcomes: a population-based study. J Pregnancy. 2016;2016:2670912. https://doi.org/10.1155/2016/2670912 .
Feldman RK, Tieu RS, Yasumura L. Gestational diabetes screening the international association of the diabetes and pregnancy study groups compared with carpenter-coustan screening. Obstet Gynecol. 2016;127(1):10–7. https://doi.org/10.1097/aog.0000000000001132 .
Pocobelli G, Yu O, Fuller S, et al. One-step approach to identifying gestational diabetes mellitus: association with perinatal outcomes. Obstet Gynecol. 2018;132(4):859–67. https://doi.org/10.1097/aog.0000000000002780 [published Online First: 2018/08/22].
Draffin CR, Alderdice FA, McCance DR, et al. Exploring the needs, concerns and knowledge of women diagnosed with gestational diabetes: a qualitative study. Midwifery. 2016;40:141–7. https://doi.org/10.1016/j.midw.2016.06.019 .
Tong A, Flemming K, McInnes E, et al. Enhancing transparency in reporting the synthesis of qualitative research: ENTREQ. BMC Med Res Methodol. 2012;12(1):181. https://doi.org/10.1186/1471-2288-12-181 .
Rathbone J, Carter M, Hoffmann T, et al. Better duplicate detection for systematic reviewers: evaluation of systematic review assistant-deduplication module. Syst Rev. 2015;4:6. https://doi.org/10.1186/2046-4053-4-6 [published Online First: 2015/01/16].
Thomas J, Harden A. Methods for the thematic synthesis of qualitative research in systematic reviews. BMC Med Res Methodol. 2008;8(1):45. https://doi.org/10.1186/1471-2288-8-45 .
Lucas PJ, Baird J, Arai L, et al. Worked examples of alternative methods for the synthesis of qualitative and quantitative research in systematic reviews. BMC Med Res Methodol. 2007;7(1):4. https://doi.org/10.1186/1471-2288-7-4 .
Group CCQM. Chapter 4: critical appraisal of qualitative research. In: Noyes J, Booth A, Hannes K, Harden A, Harris J, Lewin S, Lockwood C, editors. Supplementary guidance for inclusion of qualitative research in cochrane systematic reviews of interventions. Version 1; 2011.
Abraham K, Wilk N. Living with gestational diabetes in a rural community. MCN Am J Matern Child Nurs. 2014;39(4):239–45.
Araujo MF, Pessoa SM, Damasceno MM, et al. Gestational diabetes from the perspective of hospitalized pregnant women. Rev Bras Enferm. 2013;66(2):222–7.
Bandyopadhyay M, Small R, Davey MA. Attendance for postpartum glucose tolerance testing following gestational diabetes among south Asian women in Australia: a qualitative study. Int J Gynecol Obstet. 2015;131:E149.
Bandyopadhyay M, Small R, Davey MA, et al. Lived experience of gestational diabetes mellitus among immigrant South Asian women in Australia. Aust N Z J Obstet Gynaecol. 2011;51(4):360–4. https://doi.org/10.1111/j.1479-828X.2011.01322.x .
Carolan M. Women’s experiences of gestational diabetes self-management: a qualitative study. Midwifery. 2013;29(6):637–45. https://doi.org/10.1016/j.midw.2012.05.013 .
Doran F. Gestational diabetes mellitus: perspectives on lifestyle changes during pregnancy and post-partum, physical activity and the prevention of future type 2 diabetes. Aust J Prim Health. 2008;14(3):85–92.
Doran F, Davis K. Gestational diabetes mellitus in Tonga: insights from healthcare professionals and women who experienced gestational diabetes mellitus. N Z Med J. 2010;123(1326):59–67.
PubMed Google Scholar
Eades CE, France EF, Evans JMM. Postnatal experiences, knowledge and perceptions of women with gestational diabetes. Diabet Med. 2018;35(4):519–29.
Figueroa Gray M, Hsu C, Kiel L, et al. “It's a very big burden on me”: Women’s experiences using insulin for gestational diabetes. Matern Child Health J. 2017;21(8):1678–85.
Ge L, Albin B, Hadziabdic E, et al. Beliefs about health and illness and health-related behavior among urban women with gestational diabetes mellitus in the south east of China. J Transcult Nurs. 2016;27(6):593–602.
Ge L, Wikby K, Rask M. ‘Is gestational diabetes a severe illness?’ Exploring beliefs and self-care behaviour among women with gestational diabetes living in a rural area of the south east of China. Aust J Rural Health. 2016;24(6):378–84. https://doi.org/10.1111/ajr.12292 .
Han S, Middleton PF, Bubner TK, et al. Women’s views on their diagnosis and management for borderline gestational diabetes mellitus. J Diabetes Res. 2015;2015:209215. https://doi.org/10.1155/2015/209215 .
Article CAS PubMed PubMed Central Google Scholar
Hirst JE, Tran TS, Do MAT, et al. Women with gestational diabetes in Vietnam: a qualitative study to determine attitudes and health behaviours. BMC Pregnancy Childbirth. 2012;12:81.
Hjelm K, Bard K, Apelqvist J. Gestational diabetes: prospective interview-study of the developing beliefs about health, illness and health care in migrant women. J Clin Nurs. 2012;21(21–22):3244–56.
Hjelm K, Bard K, Apelqvist J. A qualitative study of developing beliefs about health, illness and healthcare in migrant African women with gestational diabetes living in Sweden. BMC Womens Health. 2018;18(1):34.
Hjelm K, Bard K, Berntorp K, et al. Beliefs about health and illness postpartum in women born in Sweden and the Middle East. Midwifery. 2009;25(5):564–75.
Hjelm K, Bard K, Nyberg P, et al. Swedish and middle-eastern-born women’s beliefs about gestational diabetes. Midwifery. 2005;21(1):44–60.
Hjelm K, Berntorp K, Apelqvist J. Beliefs about health and illness in Swedish and African-born women with gestational diabetes living in Sweden. J Clin Nurs. 2012;21(9–10):1374–86. https://doi.org/10.1111/j.1365-2702.2011.03834.x .
Hjelm K, Berntorp K, Frid A, et al. Beliefs about health and illness in women managed for gestational diabetes in two organisations. Midwifery. 2008;24(2):168–82.
Hui AL, Sevenhuysen G, Harvey D, et al. Stress and anxiety in women with gestational diabetes during dietary management. Diabetes Educ. 2014;40(5):668–77.
Kaptein S, Evans M, McTavish S, et al. The subjective impact of a diagnosis of gestational diabetes among ethnically diverse pregnant women: a qualitative study. Can. 2015;39(2):117–22. https://doi.org/10.1016/j.jcjd.2014.09.005 .
Kilgour C, Bogossian FE, Callaway L, et al. Postnatal gestational diabetes mellitus follow-up: Australian women’s experiences. Women Birth. 2015;28(4):285–92. https://doi.org/10.1016/j.wombi.2015.06.004 .
Lawson EJ, Rajaram S. A transformed pregnacy – the psychological consequences of gestational diabetes. Sociol Health Illn. 1994;16(4):536–62.
Lie MLS, Hayes L, Lewis-Barned NJ, et al. Preventing type 2 diabetes after gestational diabetes: women’s experiences and implications for diabetes prevention interventions. Diabet Med. 2013;30(8):986–93.
Neufeld HT. Food perceptions and concerns of aboriginal women coping with gestational diabetes in Winnipeg, Manitoba. J Nutr Educ Behav. 2011;43(6):482–91. https://doi.org/10.1016/j.jneb.2011.05.017 .
Nielsen JH, Olesen CR, Kristiansen TM, et al. Reasons for women’s non-participation in follow-up screening after gestational diabetes. Women Birth. 2015;28(4):e157–63. https://doi.org/10.1016/j.wombi.2015.04.006 .
Parsons J, Sparrow K, Ismail K, et al. Experiences of gestational diabetes and gestational diabetes care: a focus group and interview study. BMC Pregnancy Childbirth. 2018;18:25.
Pennington AVR, O’Reilly SL, Young D, et al. Improving follow-up care for women with a history of gestational diabetes: perspectives of GPs and patients. Aust J Prim Health. 2017;23(1):66–74.
Rafii F, Vasegh Rahimparvar SF, Keramat A, et al. Procrastination as a key factor in postpartum screening for diabetes: A qualitative study of Iranian women with recent gestational diabetes. Iran Red Crescent Med J. 2017;19(5). https://doi.org/10.5812/ircmj.44833 .
Razee H, van der Ploeg HP, Blignault I, et al. Beliefs, barriers, social support, and environmental influences related to diabetes risk behaviours among women with a history of gestational diabetes. Health Promot J Austr. 2010;21(2):130–7.
Salomon IMM, Soares SM. Understanding the impact of gestational diabetes diagnosis. Revista Mineira de Enfermagem. 2004;8(3):349–57.
Svensson L, Nielsen KK, Maindal HT. What is the postpartum experience of Danish women following gestational diabetes? A qualitative exploration. Scand J Caring Sci. 2018;32(2):756–64.
Tang JW, Foster KE, Pumarino J, et al. Perspectives on prevention of type 2 diabetes after gestational diabetes: a qualitative study of Hispanic, African-American and White women. Matern Child Health J. 2015;19(7):1526–34. https://doi.org/10.1007/s10995-014-1657-y .
Tierney M, O'Dea A, Danyliv A, et al. Factors influencing lifestyle behaviours during and after a gestational diabetes mellitus pregnancy. Health Psychol Behav Med. 2015;3(1):204–16. https://doi.org/10.1080/21642850.2015.1073111 .
Trutnovsky G, Panzitt T, Magnet E, et al. Gestational diabetes: women's concerns, mood state, quality of life and treatment satisfaction. J Matern Fetal Neonatal Med. 2012;25(11):2464–6. https://doi.org/10.3109/14767058.2012.683900 .
Wah YYE, McGill M, Wong J, et al. Self-management of gestational diabetes among Chinese migrants: a qualitative study. Women Birth. 2019;32(1):e17–e23.
Whitty-Rogers J, Caine V, Cameron B. Aboriginal women’s experiences with gestational diabetes mellitus: a participatory study with mi'kmaq women in Canada. ANS Adv Nurs Sci. 2016;39(2):181–98. https://doi.org/10.1097/ANS.0000000000000115 .
Johanson R, Newburn M, Macfarlane A. Has the medicalisation of childbirth gone too far? BMJ. 2002;324(7342):892–5.
Van Ryswyk E, Middleton P, Shute E, et al. Women's views and knowledge regarding healthcare seeking for gestational diabetes in the postpartum period: a systematic review of qualitative/survey studies. Diabetes Res Clin Pract. 2015;110(2):109–22.
Goldstein RF, Gibson-Helm ME, Boyle JA, et al. Satisfaction with diagnosis process for gestational diabetes mellitus and risk perception among Australian women. Int J Gynaecol Obstet. 2015;129(1):46–9.
Carolan M. Diabetes nurse educators’ experiences of providing care for women, with gestational diabetes mellitus, from disadvantaged backgrounds. J Clin Nurs. 2014;23(9–10):1374–84. https://doi.org/10.1111/jocn.12421 .
Yuen L, Wong VW. Gestational diabetes mellitus: challenges for different ethnic groups. World J Diabetes. 2015;6(8):1024–32. https://doi.org/10.4239/wjd.v6.i8.1024 [published Online First: 2015/07/25].
Carolan M, Steele C, Margetts H. Knowledge of gestational diabetes among a multi-ethnic cohort in Australia. Midwifery. 2010;26(6):579–88. https://doi.org/10.1016/j.midw.2009.01.006 .
Goer H. Gestational diabetes: the emperor has no clothes. The Birth Gazette Summertown: Second Foundation; 1996. p. 32–5.
Han S, Bubner T, Middleton PF, et al. A qualitative study of women's views on diagnosis and management for borderline gestational diabetes. J Paediatr Child Health. 2013;49:128. https://doi.org/10.1111/jpc.12133 .
Nielsen KK, Kapur A, Damm P, et al. From screening to postpartum follow-up - the determinants and barriers for gestational diabetes mellitus (GDM) services, a systematic review. BMC Pregnancy Childbirth. 2014;14:41. https://doi.org/10.1186/1471-2393-14-41 .
International Association of Diabetes and Pregnancy Study Groups Consensus Panel. International Association of Diabetes and Pregnancy Study Groups Recommendations on the diagnosis and classification of hyperglycemia in pregnancy. Diabetes Care. 2010;33(3):676–82.
Article PubMed Central Google Scholar
Fazzi C, Saunders DH, Linton K, et al. Sedentary behaviours during pregnancy: a systematic review. Int J Behav Nutr Phys Act. 2017;14:32. https://doi.org/10.1186/s12966-017-0485-z .
Van Ryswyk E, Middleton P, Hague W, et al. Clinician views and knowledge regarding healthcare provision in the postpartum period for women with recent gestational diabetes: A systematic review of qualitative/survey studies. Diabetes Res Clin Pract. 2014;106(3):401–11 https://doi.org/10.1016/j.diabres.2014.09.001 .
Download references
Acknowledgements
Not applicable.
LC is supported by a National Health and Medical Research Council Partnership Centre for Health System Sustainability grant (#9100002). RS and RT are supported by a National Health and Medical Research Council Program grant (#1106452) and PG is supported by a NHMRC Research Fellowship (#1080042). The funders had no role in design, data collection, analysis, interpretation or writing of the manuscript.
Author information
Authors and affiliations.
Institute for Evidence-Based Healthcare, Bond University, Gold Coast, Australia
Louise Craig, Rebecca Sims, Paul Glasziou & Rae Thomas
You can also search for this author in PubMed Google Scholar
Contributions
PG, LC and RT conceived the project design, LC conducted the search, extracted, analysed and interpreted the data assisted by RS. RT assisted in data interpretation. All authors contributed to the drafting of the manuscript and approve the final version.
Corresponding author
Correspondence to Rae Thomas .
Ethics declarations
Ethics approval and consent to participate, consent for publication, competing interests.
The authors declare that they have no competing interests.
Additional information
Publisher’s note.
Springer Nature remains neutral with regard to jurisdictional claims in published maps and institutional affiliations.
Supplementary information
Additional file 1:.
Table S1. Enhancing Transparency in Reporting the Synthesis of Qualitative Research Guidelines Checklist. Table S2. Assessment of quality of included studies using the CASP tool.
Rights and permissions
Open Access This article is distributed under the terms of the Creative Commons Attribution 4.0 International License ( http://creativecommons.org/licenses/by/4.0/ ), which permits unrestricted use, distribution, and reproduction in any medium, provided you give appropriate credit to the original author(s) and the source, provide a link to the Creative Commons license, and indicate if changes were made. The Creative Commons Public Domain Dedication waiver ( http://creativecommons.org/publicdomain/zero/1.0/ ) applies to the data made available in this article, unless otherwise stated.
Reprints and permissions
About this article
Cite this article.
Craig, L., Sims, R., Glasziou, P. et al. Women’s experiences of a diagnosis of gestational diabetes mellitus: a systematic review. BMC Pregnancy Childbirth 20 , 76 (2020). https://doi.org/10.1186/s12884-020-2745-1
Download citation
Received : 24 September 2019
Accepted : 15 January 2020
Published : 07 February 2020
DOI : https://doi.org/10.1186/s12884-020-2745-1
Share this article
Anyone you share the following link with will be able to read this content:
Sorry, a shareable link is not currently available for this article.
Provided by the Springer Nature SharedIt content-sharing initiative
- Systematic review
- Qualitative
- Diagnostic impacts
BMC Pregnancy and Childbirth
ISSN: 1471-2393
- Submission enquiries: [email protected]
- General enquiries: [email protected]
Enhancing gestational diabetes mellitus risk assessment and treatment through GDMPredictor: a machine learning approach
Affiliations.
- 1 Department of Laboratory Medicine, The Third Affiliated Hospital of Zhengzhou University, 7 Kangfu Qian Street, Zhengzhou, 450052, Henan, People's Republic of China.
- 2 Zhengzhou Key Laboratory for In Vitro Diagnosis of Hypertensive Disorders of Pregnancy, Zhengzhou, 450052, People's Republic of China.
- 3 Department of Laboratory Medicine, The Third Affiliated Hospital of Zhengzhou University, 7 Kangfu Qian Street, Zhengzhou, 450052, Henan, People's Republic of China. [email protected].
- 4 Zhengzhou Key Laboratory for In Vitro Diagnosis of Hypertensive Disorders of Pregnancy, Zhengzhou, 450052, People's Republic of China. [email protected].
- 5 Department of Laboratory Medicine, The Third Affiliated Hospital of Zhengzhou University, 7 Kangfu Qian Street, Zhengzhou, 450052, Henan, People's Republic of China. [email protected].
- 6 Zhengzhou Key Laboratory for In Vitro Diagnosis of Hypertensive Disorders of Pregnancy, Zhengzhou, 450052, People's Republic of China. [email protected].
- 7 Department of Laboratory Medicine, The Third Affiliated Hospital of Zhengzhou University, 7 Kangfu Qian Street, Zhengzhou, 450052, Henan, People's Republic of China. [email protected].
- 8 Zhengzhou Key Laboratory for In Vitro Diagnosis of Hypertensive Disorders of Pregnancy, Zhengzhou, 450052, People's Republic of China. [email protected].
- PMID: 38460091
- DOI: 10.1007/s40618-024-02328-z
Background: Gestational diabetes mellitus (GDM) is a serious health concern that affects pregnant women worldwide and can lead to adverse pregnancy outcomes. Early detection of high-risk individuals and the implementation of appropriate treatment can enhance these outcomes.
Methods: We conducted a study on a cohort of 3467 pregnant women during their pregnancy, with a total of 5649 clinical and biochemical records collected. We utilized this dataset as our training dataset to develop a web server called GDMPredictor. The GDMPredictor utilizes advanced machine learning techniques to predict the risk of GDM in pregnant women. We also personalize treatment recommendations based on essential biochemical indicators, such as A1MG, BMG, CysC, CO2, TBA, FPG, and CREA. Our assessment of GDMPredictor's effectiveness involved training it on the dataset of 3467 pregnant women and measuring its ability to predict GDM risk using an AUC and auPRC.
Results: GDMPredictor demonstrated an impressive level of precision by achieving an AUC score of 0.967. To tailor our treatment recommendations, we use the GDM risk level to identify higher risk candidates who require more intensive care. The GDMPredictor can accept biochemical indicators for predicting the risk of GDM at any period from 1 to 24 weeks, providing healthcare professionals with an intuitive interface to identify high-risk patients and give optimal treatment recommendations.
Conclusions: The GDMPredictor presents a valuable asset for clinical practice, with the potential to change the management of GDM in pregnant women. Its high accuracy and efficiency make it a reliable tool for doctors to improve patient outcomes. Early identification of high-risk individuals and tailored treatment can improve maternal and fetal health outcomes http://www.bioinfogenetics.info/GDM/ .
Keywords: Gestational diabetes mellitus; Machine learning; Risk prediction; Treatment recommendation.
© 2024. The Author(s).
Grants and funding
- 2021080/The PhD research startup foundation of the Third Affiliated Hospital of Zhengzhou University
- LHGJ20190398/The Medical Science and Technology Joint Construction Project of Henan Province
Gestational Diabetes Research
To mitigate the transgenerational risk of diabetes, research is needed to advance the prevention of gestational diabetes and to properly diagnose and treat it when it occurs. American Diabetes Association (ADA) research supports research projects that address these critical topics. For specific examples of projects currently funded by the ADA, see below.
Erika F. Werner, MD
Women & Infants Hospital of Rhode Island
Project: Assessing and avoiding barriers to post-partum glucose testing among women with gestational diabetes (GDM)
“I was drawn to obstetrics because pregnancy is one of the best screening tests for overall health. Pregnancy outcomes give us a window into the future health of women and the post-partum period gives an opportunity to change this future for the better. There is no better example of this principle than gestational diabetes. If we can appropriately intervene following a diagnosis of gestational diabetes, we can prevent up to 50% of future cases of diabetes in women.”
The Problem: Some women develop high blood sugar levels in pregnancy, a diagnosis known as GDM. A significant percentage of these women will have elevated blood sugars, prediabetes, even after pregnancy. Women with prediabetes are at high risk to develop type 2 diabetes unless they change their lifestyle and/or take medication. Therefore, testing women with GDM for prediabetes post-partum is the first step towards preventing type 2 diabetes. Unfortunately, most women with GDM never get tested for pre-diabetes and instead go on to develop type 2 diabetes in the years after delivery.
The Project: Dr. Werner’s project will determine how we can change post-partum care to better identify women with prediabetes and then intervene to reduce their diabetes risk. Three hundred women with GDM will receive routine care so that we can compare the women who return for post-partum glucose testing to those who do not return. Dr. Werner will compare individual characteristics, barriers to care and attitudes toward health care among the two groups. Additionally, Dr. Werner will determine if the test to determine prediabetes, normally given between 6 and 12 weeks after birth, can be given at 2 days post-birth while the mother is still in the hospital.
The Potential Outcome: The outcomes collected from this project will inform a re-purposing of post-partum care for women with GDM so that we can better prevent type 2 diabetes in a high-risk population.
Reena Oza-Frank, PhD
The Ohio Department of Health
Project: Practical approaches to postpartum weight loss after gestational diabetes (GDM)
“It is important to me to help build an evidence base to broaden public health policy to address the global priority of type 2 diabetes prevention. A healthy family starts with a healthy mother. By empowering women to be able to take the steps towards implementing a healthy lifestyle during the postpartum period, a health-promoting foundation can be created and extend to the family unit, reducing type 2 diabetes risk across generations.”
The Problem: GDM greatly increases risk for subsequent development of type 2 diabetes (T2D). This increased risk is compounded by excess weight retention, a common issue after pregnancy and a major risk factor for developing T2D. Thus, weight loss intervention is critical to prevent and/or delay T2D in these high-risk women and the weeks and months immediately after a GDM-complicated pregnancy present a unique window of opportunity for implementing an intervention. However, asking postpartum women to make lifestyle changes during a time consumed by competing demands has proven to be a major challenge in previous interventions.
The Project: Based on this problem, there is a critical need to test an intervention that addresses postpartum barriers to making lifestyle changes. Dr. Oza-Frank plans to test the effectiveness of a simple, novel, activity-boosting intervention on weight loss through 6 months postpartum among women with GDM. The intervention uses ankle weights (2.5 pounds [1.1 kg]) worn on each ankle during the usual daily activities (e.g., cleaning, cooking, childcare, etc.). Based on her previous work, Dr. Oza-Frank believes women with GDM will adopt an exercise intervention that requires minimal additional time outside of their daily activities.
The Potential Outcome: By adopting this intervention, women in the intervention could experience more, faster weight loss compared with women who were not in the intervention, reducing their long-term risk of T2D. If successful, this intervention program could then be broadly applied to women following a pregnancy with GDM.
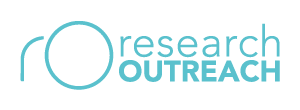
- Publication
- Arts & Humanities
- Behavioural Sciences
- Business & Economics
- Earth & Environment
- Education & Training
- Health & Medicine
- Engineering & Technology
- Physical Sciences
- Thought Leaders
- Community Content
- Outreach Leaders
- Our Company
- Our Clients
- Testimonials
- Our Services
- Researcher App
- Podcasts & Video Abstracts
Subscribe To Our Free Publication
By selecting any of the topic options below you are consenting to receive email communications from us about these topics.
- Behavioral Science
- Engineering & Technology
- All The Above
We are able share your email address with third parties (such as Google, Facebook and Twitter) in order to send you promoted content which is tailored to your interests as outlined above. If you are happy for us to contact you in this way, please tick below.
- I consent to receiving promoted content.
- I would like to learn more about Research Outreach's services.
We use MailChimp as our marketing automation platform. By clicking below to submit this form, you acknowledge that the information you provide will be transferred to MailChimp for processing in accordance with their Privacy Policy and Terms .
Gestational diabetes: A novel detection strategy
Gestational diabetes mellitus is a form of diabetes that may develop during pregnancy. The condition can arise at any time during pregnancy, however it most commonly occurs during the second and third trimesters, and resolves once the pregnancy ends. Gestational diabetes is caused by the diminishing action of insulin (insulin resistance) due to increasing hormone production by the placenta, and is associated with chronic inflammation. Women at risk of developing gestational diabetes are those who are older in age, overweight or obese (with a body mass index [BMI] greater than 30), experience excessive weight gain during pregnancy, or have a family history of diabetes. Other risk factors include previous gestational diabetes, Asian or Middle-Eastern ethnicity and foetal macrosomia (a large baby for gestational age) in previous pregnancies.

Notably, gestational diabetes is the leading cause of high blood glucose levels (hyperglycaemia) in pregnancy, the effects of which can lead to complications that affect both the mother and child. These complications include foetal macrosomia, preeclampsia, and polyhydramnios (too much amniotic fluid in the womb), all of which can result in premature labour and birth trauma. Babies born to mothers with gestational diabetes also have a higher lifetime risk of obesity and of developing diabetes. It is estimated that hyperglycaemia in pregnancy affects more than 16% of live births, with over 80% of these cases due to gestational diabetes. Also, pregnant women with hyperglycaemia are at higher risk of developing gestational diabetes in subsequent pregnancies and are estimated to be at seven times greater risk of type 2 diabetes development than women with normal pregnancies. Worryingly, mothers with gestational diabetes or with hyperglycaemia in pregnancy are also at high risk for causing other transgenerational effects for their offspring, such as a higher risk of hypertension and kidney disease.

The pre-screening blood test would eliminate the burden and cost of having to perform OGTTs in women at low risk of gestational diabetes.
The treatment of gestational diabetes reduces the risk of associated complications. However, the condition is most commonly diagnosed in late pregnancy, which leaves limited time for any intervention. Overt symptoms of hyperglycaemia during pregnancy are rare and can be easily confused with normal pregnancy symptoms. Consequently, gestational diabetes is universally diagnosed using an oral glucose tolerance test (OGTT) performed between the 24th and 28th week of pregnancy – a procedure that is time-consuming and arduous for both the clinic and the attending pregnant women. Furthermore, the negative impact of maternal hyperglycaemia on the foetus may be underway before conventional late pregnancy gestational diabetes screening is performed. Thus, any intervention given after a diagnosis late in pregnancy may have limited effect. Importantly, many pregnant women experience nausea and discomfort when performing the OGTT, therefore it is of interest to limit the number of women who have to undergo the procedure to only encompass those at higher risk of gestational diabetes. An accurate blood test capable of identifying women at low or high risk for gestational diabetes already in the first trimester of pregnancy would have the potential to reduce the need for universal testing in all pregnant women, decrease costs, and improve outcomes through prevention or treatment.

To meet this challenge, Dr Jonatan Dereke, an assistant researcher and member of the Diabetes Research Laboratory team at the Department of Clinical Sciences Lund, Lund University, Sweden, and his colleagues, Dr Magnus Hillman, Dr Mona Landin-Olsson, Dr Charlotta Nilsson, Dr Helena Strevens, and Birgitte Ekholm, are endeavouring to develop a simple, non-fasting, pre-screening blood test that can be used before the OGTT to identify women at low or high risk of gestational diabetes. With the assistance of funding from the Gorthon Foundation and Skåne University Hospital Funding and Donations, Dr Dereke and his team are currently investigating several proteins present in the blood circulation during early pregnancy that could act as biomarkers, allowing the identification of women at increased or decreased risk of developing gestational diabetes.
One of the key areas of Dr Dereke’s research is the investigation of pregnancy-associated plasma protein-A2 (PAPP-A2), a protease cleaving insulin-like growth factor-binding protein. PAPP-A2 is most abundantly expressed in the placenta, where it is found to regulate foetal-placental growth and placental development. In healthy pregnant women, PAPP-A2 increases as the pregnancy develops, with levels becoming stable during the third trimester. To determine the connection between PAPP-A2 and gestational diabetes, Dr Dereke and his team conducted a case-control study in which circulating PAPP-A2 was analysed in two groups of women in early pregnancy – one group consisting of pregnant women who had been diagnosed with gestational diabetes following an OGTT performed as part of a general screening in southern Sweden, and the other group consisting of pregnant women without diabetes. The women in both groups were matched for age and BMI, to minimise any bias these risk factors may have had on the results. This provided a unique and heterogeneous patient population increasing the validity of the results, as there was no selection bias at recruitment.

The study revealed that women diagnosed with gestational diabetes in early pregnancy have increased circulating PAPP-A2 levels compared with pregnant women of a similar gestational age not diagnosed with diabetes, independent of BMI, C-peptide (a by-product of insulin production from the pancreas) or adiponectin (an adipocyte-derived protein that enhances insulin sensitivity and is inversely associated with obesity and diabetes risk). The study also demonstrated that if PAPP-A2 is employed as a pre-OGTT screening biomarker, one third of women in early pregnancy, who are at low risk of developing gestation diabetes, will no longer need to receive an initial OGTT. This outcome would eliminate the burden and cost of having to perform OGTTs in women at low risk of gestational diabetes, while minimising the number of women with gestational diabetes missed. Notably, this is the first study to address the topic of pre-screening biomarkers for gestational diabetes. PAPP-A2 might also be able to predict gestational diabetes development in late pregnancy; however, further prospective studies are required to fully evaluate this association.
Notably, this is the first study to address the topic of pre-screening biomarkers for gestational diabetes.
A number of other novel biomarkers in early pregnancy may also serve as potential predictors of gestational diabetes. For example, Dr Dereke and his team have determined that plasma levels of soluble tumour necrosis factor-like weak inducer of apoptosis (sTWEAK) – a protein implicated in numerous cellular processes – are significantly decreased in women with early pregnancy gestational diabetes compared to levels in pregnant women without diabetes. Additionally, Dr Dereke and his colleagues have conducted a study of relaxin-2, an important gestational hormone involved in shaping the endometrium in early pregnancy. Higher levels of relaxin-2 were observed at 12 weeks of pregnancy complicated by gestational diabetes than in pregnant women without diabetes.
Taken collectively, the findings from these studies suggest that, in addition to PAPP-A2, there are several potential biomarker candidates for early pregnancy gestational diabetes that could be detected using a simple blood test. Going forwards, Dr Dereke and his team are planning further investigations to confirm their results.

Personal Response
Please could you provide further details on the next steps for your research and highlight what will be the key focus?
<> Our next steps are to recruit a larger group of pregnant women and measure potential biomarkers for gestational diabetes that are present throughout the whole duration of the pregnancy. This prospective cohort study will give us more insight into the biological events contributing to gestational diabetes development as pregnancy progresses. It will also help us to find even better biomarkers of disease development. We have also started studying the connections between gestational diabetes and the effect on children’s growth development and risk for becoming overweight and obese later in life. Taken together, these studies will help us better understand the underlying pathogenesis for gestational diabetes and its consequences for future generations.
This feature article was created with the approval of the research team featured. This is a collaborative production, supported by those featured to aid free of charge, global distribution.
Want to read more articles like this, sign up to our mailing list and read about the topics that matter to you the most., leave a reply cancel reply.
Your email address will not be published. Required fields are marked *
Neutrophil gelatinase-associated lipocalin (NGAL) levels in twin pregnancy and association with gestational diabetes
- Short Communication
- Published: 20 March 2024
Cite this article
- Angela Silvano 1 na1 ,
- Oumaima Ammar 1 na1 ,
- Noemi Strambi 1 ,
- Giovanni Sisti 2 ,
- Astrid Parenti 3 ,
- Viola Seravalli ORCID: orcid.org/0000-0001-8706-3925 1 na1 &
- Mariarosaria Di Tommaso 1 na1
This is a preview of subscription content, log in via an institution to check access.

Access this article
Price includes VAT (Russian Federation)
Instant access to the full article PDF.
Rent this article via DeepDyve
Institutional subscriptions
Zhu X, Huang C, Wu L et al (2022) Perinatal outcomes and related risk factors of single vs twin pregnancy complicated by gestational diabetes mellitus: meta-analysis. Comput Math Methods Med. https://doi.org/10.1155/2022/3557890
Article PubMed PubMed Central Google Scholar
Springer S, Franz M, Worda K, Gorczyca ME, Haslinger P, Worda C (2022) Neutrophil gelatinase-associated lipocalin and hypertensive disorders of pregnancy: a cohort study in twin pregnancies. J Clin Med 11(14):4163. https://doi.org/10.3390/jcm11144163
Article CAS PubMed PubMed Central Google Scholar
Wang Y, Lam KS, Kraegen EW et al (2007) Lipocalin-2 is an inflammatory marker closely associated with obesity, insulin resistance and hyperglycemia in humans. Clin Chem 53(1):34–41. https://doi.org/10.1373/clinchem.2006.075614
Article CAS PubMed Google Scholar
Sisti G, Rubin G, Zhou C, Orth T, Schiattarella A (2023) Neutrophil gelatinase-associated lipocalin as a predictor of pre-eclampsia: a systematic review and meta-analysis. Int J Gynaecol Obstet 163(1):63–74. https://doi.org/10.1002/ijgo.14777
Lu L, Li C, Deng J, Luo J, Huang C (2022) Maternal serum NGAL in the first trimester of pregnancy is a potential biomarker for the prediction of gestational diabetes mellitus. Front Endocrinol (Lausanne) 16(13):977254. https://doi.org/10.3389/fendo.2022.977254
Article Google Scholar
Download references
Acknowledgements
We would like to thank all subjects, doctors, researchers, and laboratory team for their cooperation and support.
The research reported in this publication was supported by Fondazione CRF Firenze Grant number B15F21003630007 to M.D.T.
Author information
Angela Silvano and Oumaima Ammar, Viola Seravalli and Mariarosaria Di Tommaso have equally contributed to this work.
Authors and Affiliations
Division of Obstetrics and Gynecology, Department of Health Sciences, Careggi Hospital, University of Florence, Florence, Italy
Angela Silvano, Oumaima Ammar, Noemi Strambi, Viola Seravalli & Mariarosaria Di Tommaso
Division of Maternal-Fetal Medicine, Department of Obstetrics and Gynecology, College of Medicine, University of Arizona, Tucson, USA
Giovanni Sisti
Clinical Pharmacology and Oncology Section, Department of Health Sciences, University of Florence, Florence, Italy
Astrid Parenti
You can also search for this author in PubMed Google Scholar
Contributions
AS contributed to Conceptualization, Methodology, Formal analysis, Investigation, Writing—original draft, Writing—review & editing. OA contributed to Methodology, Formal analysis, Investigation, Writing—original draft, Writing—review & editing. VS & AP contributed to Conceptualization, Methodology, Writing—review & editing. NS & GS contributed to Formal analysis, Resources, review & editing. MDT contributed to Conceptualization, Methodology, Supervision, Writing—review & editing.
Corresponding author
Correspondence to Viola Seravalli .
Ethics declarations
Conflict of interest.
The authors declare that they have no conflicts of interest.
Ethical standard statement
The study was approved by the Ethical Committee of Azienda Ospedaliero-Universitaria Careggi (Ref. No.10255/2017). All procedures followed were in accordance with the ethical standards of the responsible committee on human experimentation (institutional and national) and with the Helsinki Declaration of 1975, as revised in 2008.
Informed consent
An informed consent was obtained from all patients for being included in the study.
Additional information
Publisher's note.
Springer Nature remains neutral with regard to jurisdictional claims in published maps and institutional affiliations.
This article belongs to the topical collection Pregnancy and Diabetes, managed by Antonio Secchi and Marina Scavini.
Rights and permissions
Reprints and permissions
About this article
Silvano, A., Ammar, O., Strambi, N. et al. Neutrophil gelatinase-associated lipocalin (NGAL) levels in twin pregnancy and association with gestational diabetes. Acta Diabetol (2024). https://doi.org/10.1007/s00592-024-02240-1
Download citation
Received : 20 October 2023
Accepted : 13 January 2024
Published : 20 March 2024
DOI : https://doi.org/10.1007/s00592-024-02240-1
Share this article
Anyone you share the following link with will be able to read this content:
Sorry, a shareable link is not currently available for this article.
Provided by the Springer Nature SharedIt content-sharing initiative
Advertisement
- Find a journal
- Publish with us
- Track your research

An official website of the United States government
The .gov means it’s official. Federal government websites often end in .gov or .mil. Before sharing sensitive information, make sure you’re on a federal government site.
The site is secure. The https:// ensures that you are connecting to the official website and that any information you provide is encrypted and transmitted securely.
- Publications
- Account settings
Preview improvements coming to the PMC website in October 2024. Learn More or Try it out now .
- Advanced Search
- Journal List
- Int J Environ Res Public Health

Gestational Diabetes: Overview with Emphasis on Medical Management
With the rising trend in obesity, the incidence of gestational diabetes mellitus (GDM) and perinatal complications associated with the condition are also on the rise. Since the early 1900s, much knowledge has been gained about the diagnosis, implications, and management of gestational diabetes with improved outcomes for the mother and fetus. Worldwide, there is variation in the definition of GDM, methods to screen for the condition, and management options. The International Association of Diabetes in Pregnancy Study Groups has published recommendations for a one-step approach to screen pregnant women for GDM, in order to develop outcome-based criteria that can be used internationally. However, management of GDM continues to be varied, and currently several options are available for treatment of hyperglycemia during pregnancy. A review of various aspects of GDM is discussed with a focus on the medical management during pregnancy, as practiced in the United States.
1. Introduction
A worldwide rising trend in obesity has been reported from 1975 to 2016, affecting females and males alike [ 1 ]. In women, the rising obesity has led to an increase in the incidence of gestational diabetes mellitus (GDM) as well as associated pregnancy and perinatal complications. Known non-modifiable risk factors for predisposition to GDM include advanced maternal age, ethnicity, and family history of type 2 diabetes mellitus [ 2 ]. Maternal obesity independently contributes to the development of GDM [ 3 ]. The Center of Disease Control (CDC) estimates that the incidence of GDM in the United States (US) is about 10%. It is reported to be higher in some countries with rates as high as 17.8–41.9% when using the International Association of Diabetes in Pregnancy Study Groups (IADPSG) GDM criteria [ 4 , 5 ]. In Western countries, a body mass index (BMI) of <25 Kg/m 2 is considered normal.
The recommendations for pharmacotherapy in patients with GDM have evolved over the past two decades. In this review, we provide an overview of the condition, with emphasis on the evolution of medical management over the past century. We have included the recent data on the options for medical management, as well as data on the current recommendations based on safety data of these pharmacotherapeutic options during pregnancy.
This review was performed by including studies published in PubMed, Cochrane Library, the national guidelines, and WHO-based guidelines. The search was performed using key words that included “gestational diabetes”, “diabetes management”, and “pregnancy”. Recent publications, randomized control trials, observational studies, review articles, meta-analyses, Cochrane reviews, and current practice guidelines from national organizations were selected. Year of publication was not a limiting factor in this literature search. The publications included in this review were restricted to English language. The diagnosis of GDM in this review is based on the definition recommended by the American College of Obstetrics and Gynecology (ACOG). Articles on pharmacotherapy within the last two decades for treatment of hyperglycemia in GDM are reviewed with emphasis on the recent publications and recommendations.
3. Historical Aspects of Gestational Diabetes
Recognition of diabetes complicating pregnancy dates back to 1873 [ 6 ]. In 1910, it was proposed that patients with gestational glycosuria be grouped into two categories, namely, pregnant women suffering from true and persistent glycosuria and pregnant women who pass sugar in their urine only when their diet contains a large amount of sugar or starch [ 7 ]. Subsequently, in a series of 468 pregnant women, 5 were documented with glycosuria with only one who was known to have pre-pregnancy diabetes [ 8 ]. The author suggested that in cases where glycosuria occurred during pregnancy, true diabetes might be “about to manifest itself”. From these early cases, it was recognized that there is a difference between diabetes diagnosed prior to pregnancy and diabetes diagnosed during pregnancy.
Due to recognition of the possibility of diabetes affecting a pregnancy, studies were conducted to understand the effect of pregnancy on carbohydrate metabolism. The adverse effect of pregnancy on carbohydrate metabolism was first documented in 1946 [ 9 ]. Hoet from Belgium performed animal experiments to study carbohydrate metabolism during pregnancy [ 10 ]. The harmful effects of GDM on pregnancy outcomes were subsequently reported. In 1946, while reporting on the effects of hyperglycemia during pregnancy, the author documented the finding that fetal death can occur even before the woman has symptoms of diabetes [ 11 ]. Subsequently, in 1959, the association of maternal pre-diabetes with fetal macrosomia was published in Italy [ 12 ]. Two years later, O’Sullivan described GDM as “unsuspected and asymptomatic” diabetes, which is elicited in response to a glucose tolerance test during pregnancy [ 13 ]. At that time, the incidence of GDM was reported to be 1 in 116 [ 13 ].
Due to the recognition of the complications associated with GDM, a need for accurate and timely diagnosis was realized [ 14 , 15 ]. In 1924, a 50-g glucose load was administered to a pregnant patient with history of glycosuria, to determine if she had glucose intolerance [ 16 ]. The oral glucose tolerance test (GTT) was described in 1932. In search of an ideal glucose screen, an intravenous glucose tolerance test and a prednisone-glucose tolerance test were studied [ 17 , 18 ]. In 1961, O’Sullivan screened all pregnant patients with a 50-g oral glucose load and when the one-hour venous blood sugar was ≥130 mg/dL, the patient was considered screen-positive. At that time, patients were also classified as screen-positive if the patient had a family history of diabetes, a prior fetal or neonatal death, congenital fetal anomaly, prematurity, toxemia in two or more pregnancies, or a history of a prior baby that weighed 9 pounds or greater. Patients screened as positive were administered a three-hour GTT [ 13 ].
By 1985, glucose screening was routinely being used in patients considered to be at “high risk” for GDM [ 19 ]. Due to the varying definitions, the reported incidence of GDM ranged from 0.31% to 18%. This led to the suggestion of using similar screening criteria by all centers, to help better understand the condition and its impact on pregnancy.
4. Antenatal Glucose Screening
Various organizations attempted to establish population-based protocols to diagnose GDM [ 20 ]. In 1999, the WHO recommended a screening test of a 75-g anhydrous glucose load, following an overnight fasting for 8–14 h, between 24 and 28 weeks’ gestation. The protocols suggested by national and international organizations such as the IADPSG, American Diabetes Association (ADA), and United Kingdom-based National Institute for Health and Care Excellence (NICE) and Canada were recently reviewed [ 21 ]. Both a single-step and a two-step screening process during pregnancy have been described. Based on the Hyperglycemia and Adverse Pregnancy Outcome (HAPO) study, the IADPSG recommended a single-step 2-h glucose tolerance test. After an overnight fast, a 75-g glucose load is administered. The fasting 1-h and 2-h plasma glucose levels are checked.
Despite the ADA endorsing the one-step 75-g glucose load GTT in 2011 based on the IADPSG criteria, the National Institute of Health in the USA recommended the two-step screening for GDM, which has been adopted by the American College of Obstetrics and Gynecology (ACOG) [ 22 , 23 ]. In the two-step approach, women first undergo a 1-h 50-g glucose screen, and if it is abnormal, a 3-h GTT with a 100-g glucose load is performed. If results are abnormal on the 3-h GTT, the patient is diagnosed with GDM. Based on the one-step IADPSG screening criteria, 17.8% of pregnant patients in the USA would test positive for GDM, which would nearly double the incidence of GDM in the USA [ 24 ]. The ACOG and other organizations in the USA have not adopted the one-step process due to a lack of evidence of impact on the pregnancy outcomes. The two-step testing at 24–28 weeks’ gestation starts with an initial screen in a non-fasting state, with an oral 50-g glucose load followed by a 1-h plasma glucose level. The cutoff value for the 1-h glucose screen is 130 to 140 mg/dL. Screen-positive women undergo the 3-h oral GTT. Following an overnight fast, a 100-g oral glucose load is administered. Plasma glucose levels are checked in the fasting state and at 1 h, 2 h, and 3 h following the glucose load [ 22 ]. Details of the different screening protocols are documented in Table 1 .
Protocol-based guidelines for diagnosis of gestational diabetes on oral glucose tolerance test.
Early glucose screening is normally completed at the first prenatal visit in women with risk factors that include obesity with a BMI of ≥30 Kg/m 2 , history of gestational diabetes in a prior pregnancy, known impaired glucose metabolism, hemoglobin A1C of ≥5.7%, first-degree relative with diabetes mellitus, high-risk ethnicity, history of polycystic ovarian syndrome, pre-existing hypertension or cardiovascular disease, or a prior large baby ≥4000 g [ 22 ]. The early screening helps detect patients with pre-pregnancy type II diabetes mellitus. Women who have a normal glucose screen in early pregnancy have the test repeated at 24–28 weeks’ gestation.
Plasma glucose levels drawn in a fasting state, followed by a glucose load. The normal levels are as noted in the table. In the IADPSG, the Canada Diabetes Association, and the NICE guidelines, GDM is diagnosed when one or more plasma glucose levels are elevated above the normal levels. In the ACOG guidelines, patients with a positive 1-h glucose screen undergo the 3-h test and when two or more levels are elevated above the normal levels, GDM is diagnosed.
5. Pathophysiology of Gestational Diabetes
Claes Hellerström has been credited for the early work starting in 1963 on pancreatic changes during pregnancy and lactation in a mouse model (25,28). Insulin requirements physiologically increase during pregnancy. The increase in insulin demand is due to increased maternal caloric intake, maternal weight gain, presence of the placental hormones such as placental growth hormone, and placental lactogen, as well as increased prolactin and growth hormone production. As the pregnancy advances, the pancreatic β-cell mass increases to keep up with the demand for increased insulin. Failure of the β-cell expansion with a relative inadequate rise in insulin secretion leads to GDM.
Maternal glucose is transported across the placenta to the fetus, and this delivery depends on the concentration gradient between the fetus and the maternal glucose levels. In the later part of pregnancy, the fetus diverts an increasing amount of maternal glucose towards itself, which leads to a decrease in maternal glucose levels. In order to maintain the concentration gradient of glucose across the placenta between the mother and the fetus, the maternal insulin resistance increases, as well as the hepatic glucose production [ 25 ]. In turn, the β-cells increase insulin secretion to prevent excessive delivery of glucose to the fetus.
6. Management of Gestational Diabetes
The approach to optimal management of a patient diagnosed with GDM requires a multidisciplinary approach. This includes teaching patient self-monitoring of blood glucose levels, dietary modifications and nutrition monitoring, lifestyle changes, and maternal weight gain management. Up to 70–85% of patients diagnosed with gestational diabetes can be managed with adequate physical activity, and dietary and lifestyle modifications [ 26 ]. In 15–30% of patients, medications will be required. These medications include insulin as well as oral hypoglycemic agents ( Figure 1 ).

Management of hyperglycemia in gestational diabetes.
7. Blood Glucose Monitoring
Most organizations recommend daily self-glucose monitoring at home. Currently, the recommendations include daily self-monitoring with fasting and postprandial blood glucose. The ADA recommends the following target values: a fasting blood glucose of <95 mg/dL and a one-hour postprandial blood glucose of <140 mg/dL or a two-hour postprandial blood glucose of <120 mg/dL. Pre-prandial glucose monitoring is primarily for those with pre-existing diabetes. Monitoring with hemoglobin A1C levels is not as valuable for assessing glucose control in GDM [ 27 ]. Studies have examined alternative testing approaches to self-monitoring, such as healthcare-based monitoring and continuous glucose monitoring. A Cochrane review reported that there were no differences in self-monitoring compared with healthcare-based glucose monitoring for both maternal and neonatal complications. There were also no differences between self-monitoring and continuous glucose monitoring in cesarean section rates, large for gestational age, or neonatal hypoglycemia [ 28 ].
8. Dietary Interventions
Nutritional therapy must be discussed with a patient and, ideally, a diabetes educator counsels the patient regarding dietary modifications. General guidance in pregnancy is for women to consume three meals and two snacks during the day. Meal plans can be developed between the patient and the dietary educator to meet the appropriate daily requirements for pregnant diabetic patients, while trying to incorporate foods that are enjoyed by the patient as well feasible for the patient to follow. In conjunction with diet, women should be advised to write down their meals with their blood glucose values to help them identify foods that may contribute to postprandial hyperglycemia.
There are a variety of dietary approaches that have been described in the literature, including calorie-restricted diets, low-glycemic index diets, the DASH diet (dietary approaches to stop hypertension), low-carbohydrate diets, and low-unsaturated fat diets, high-fiber diets, and soy-based diets. Most of the dietary studies in pregnancy are reported to be of low-quality evidence [ 29 ]. ACOG references a 1993 study suggesting that the daily calorie allotment for gestational diabetics be distributed by percentages between the macronutrients with 40% from carbohydrates, 20% protein, and 40% fat [ 22 ]. However, the ADA’s 2017 review on diabetes care recommended similar dietary guidance for gestational diabetics to women with pre-existing diabetes. Fat consumption guidelines, cited from the Institute of Medicine, state that 20–35% of calories should be from fat. Higher-quality complex carbohydrates with lower glycemic indexes are preferred since they may help reduce the need for insulin as well as decrease postprandial hyperglycemia [ 30 ]. High-protein diets have not been demonstrated to improve health or glycemic control, and some studies suggest that excessive protein intake is associated with low fetal birthweight [ 30 ]. The ADA recommends daily protein requirements of 1–1.5 g/kg or 15–20% of consumed calories. Based on the above resources, dietary education should emphasize a balanced diet with portion control, healthy fats, complex carbohydrates, and 20% protein.
9. Physical Activity
Exercise and physical activity are supported and encouraged during pregnancy, including in women with GDM. Moderate exercise during pregnancy has many beneficial effects including lower risk for development of GDM, lower likelihood of large for gestational age neonates, and being associated with lower hypertensive disorders and preterm birth, without an increase in fetal growth restriction [ 31 , 32 ]. Additionally, lifestyle changes during pregnancy have an influence on the postpartum period by lowering the risk of postpartum depression [ 33 ]. Current recommendations are for 30 min of moderate-intensity exercise, 5 days a week. When unable to perform moderate exercises, women may consider light exercises such as post-meal walks for 10–15 min, which can have a beneficial impact on blood sugar control. Pregnant women should avoid high-impact activities since they could result in abdominal trauma.
10. Pharmacotherapy for Management of Gestational Diabetes
In up to 15–30% of patients with GDM, despite recommended diet and lifestyle modifications, blood glucose control is inadequate, and pharmacotherapy is required [ 34 ]. There are no well-studied protocols to determine the optimal time to initiate pharmacotherapy for glycemic control. Typically, after a period of 10–14 days of dietary and lifestyle modifications, if hyperglycemia persists through the day, pharmacotherapy should be considered. Based on a multicenter randomized trial by the NICHD, if the majority of fasting or postprandial blood glucose measurements are elevated or if there is suspicion of hyperglycemia with a random blood glucose level of ≥160 mg/dL or a fasting level of ≥95 mg/dL, medications should be initiated [ 35 ].
Insulin as well as oral medications has been used for the management of hyperglycemia in GDM patients. During pregnancy, insulin has the safest profile. The oral agents that have been studied include sulfonylurea such as glyburide (also known as glibenclamide), as well as metformin. In the USA, both the ADA and ACOG recommend insulin as the first line for control of hyperglycemia in patients with GDM. Insulin is a large molecule and does not cross the placenta. Metformin and glyburide have been shown to cross the placenta and into the fetus.
11. Insulin Regimens
In patients that require insulin, the dose and timing of administration depend on the patient’s body weight, gestational age, and the time of day at which hyperglycemia is occurring. Once initiated, insulin doses are adjusted frequently throughout the pregnancy based on blood glucose results, symptomatic hypoglycemia, physical activity, dietary consumption, infection, and compliance.
Insulin was discovered in 1922. In 1946, the intermediate-acting NPH insulin was introduced. For a long period of time, NPH was being used. Since then, research in different types of available insulin has evolved significantly. The insulin analogs that are currently available include rapidly acting analogs, such as aspart (Novolog) and lispro (Humalog), short-acting regular insulin, intermediate-acting NPH insulin, or longer-acting insulin analogs such as glargine (Lantus) and detemir (Levemir). In GDM, short-acting insulin is reported to increase the possibility of hypoglycemia and may cause fluctuations in glycemic control. Recent experience with aspart has been reassuring, while lispro has been associated with higher birth weight and increased rates of large for gestational age neonates [ 36 ]. For intermediate- and longer-acting insulin, randomized trials comparing detemir to NPH revealed no difference between the two in regard to glucose control and perinatal outcomes. Detemir has been associated with a lower incidence of hypoglycemia in the non-pregnant diabetic [ 37 ].
Two main approaches to prescribing insulin are based on the specific timing of recurrent hyperglycemia. Insulin can be administered throughout the day in divided doses or be given as a single daily dose depending on the timing of hyperglycemia. In women experiencing hyperglycemia only in the morning fasting state, intermediate insulin, such as NPH, or detemir should be administered at bedtime, as a single dose. In women experiencing postprandial hyperglycemia following specific meals only, rapid-acting insulin should be considered prior to the meal. Women experiencing hyperglycemia throughout the day should be managed with a combination of intermediate- or long-acting and short-acting insulin, with the total daily dose of insulin of 0.7–1.0 unit/Kg divided into rapid-acting insulin given before meals and intermediate- or long-acting insulin in the morning or at bedtime [ 22 ]. When prescribing insulin, close blood glucose monitoring is needed to try to avoid periods of hypoglycemia or hyperglycemia. Patients are advised to bring their self-monitored blood glucose logs to the office so the provider can determine if changes in the insulin regimen are needed.
12. Oral Hypoglycemic Agents
Oral medications have not been adequately studied for possible long-term effects on neonatal outcomes, and therefore they are not recommended as the first choice in treatment for persistent hyperglycemia in GDM patients. These are also reported to fail in controlling hyperglycemia in about a quarter of women with GDM. In instances where patients are unable to get insulin, or refuse insulin, oral medications may be prescribed. When comparing the two oral agents, metformin appears to be safer than glyburide. Glyburide has been associated with neonatal hypoglycemia and higher birth weight, which can increase the risk for shoulder dystocia and need for cesarean delivery [ 38 , 39 ]. Additionally, glyburide is demonstrated to be present in umbilical cord blood samples in concentrations that are 50–70% those of maternal levels which can lead to neonatal hypoglycemia [ 38 ]. Even though metformin is stated to have a lower risk of neonatal hypoglycemia, the umbilical cord blood levels of metformin are reported to be similar to or higher than maternal levels [ 34 ].
Metformin dose starts at 500 mg orally each night or 500 mg twice daily based on glycemic control. Maximum total daily dose is 2500–3000 mg during pregnancy, which is higher than in the non-pregnant state. Glyburide is started at 2.5 mg daily or every 12 h and gradually increased to a maximum of 10 mg twice daily, based on glycemic control [ 22 ]. In pregnancy, the serum concentration of glyburide rises about 30–60 min after oral administration and peaks in 2–3 h, with the peak concentration coinciding with the peak of blood glucose following a meal. Since glyburide peaks at 2–3 h after administration, higher blood glucose values may be observed when checked 1 or 2 h following a meal, with a decrease subsequent to that. This may lead to increasing the glyburide dose but with a negative subsequent effect of hypoglycemia since the peak activity is 2–3 h following a meal [ 40 ]. When optimal glucose control on oral agents cannot be achieved, the option of insulin should be re-addressed with the patient.
13. Maternal and Fetal Complications
Gestational diabetes has a strong impact on maternal and fetal outcomes. Women with GDM are at increased risk for development of pre-eclampsia and increased need for delivery by cesarean section. Much of this risk is related to the degree of glycemic control during the pregnancy, with poor glycemic control leading to higher risk for poor obstetrical outcomes [ 41 ]. These risks include obstetrical and neonatal complications such as preterm delivery, polyhydramnios, macrosomia, shoulder dystocia, admission to the neonatal intensive care unit, neonatal respiratory distress syndrome, fetal hypoglycemia, and hyperbilirubinemia [ 42 ]. There is also an increase in the risk of stillbirth. Aside from pregnancy-related risks, women diagnosed with gestational diabetes are at increased risk of developing type 2 diabetes mellitus later in life.
14. Antepartum Surveillance
The need for fetal assessment is primarily based on maternal glycemic control. In women with good glycemic control on diet alone, fetal testing prior to 40 weeks’ gestation is not indicated, unless there are other indications [ 22 ]. If the patient is not delivered by the estimated due date, fetal testing can be performed weekly or twice weekly beginning at 40 weeks’ gestation.
In women requiring pharmacotherapy, antepartum fetal testing should be initiated by 32 weeks’ gestation, consisting of a biophysical profile and non-stress test. These tests are performed twice weekly, similar to women with pre-existing diabetes, and until delivery [ 43 ]. Presence of other maternal co-morbidities or development of hypertensive disorders may impact the frequency and timing of antepartum testing, with some patients requiring fetal monitoring earlier than 32 weeks [ 22 ]. There is no clear consensus on ultrasound examinations for fetal growth assessment, but it must be performed at least once in the third trimester and closer to delivery to assess fetal growth. In summary, antepartum fetal monitoring is based on glycemic control, the use of medical therapy, and the presence of additional risk factors. Each institution should develop guidelines that are feasible based on the resources and facilities at that institution.
15. Delivery Planning
ACOG published guidelines outlining the timing of delivery in women with gestational diabetes, which is based on glycemic control. When GDM is well controlled with diet and exercise, delivery should be considered starting at 39 weeks. Women requiring medications and with good glucose control are delivered between 39 and 39-6/7 weeks’ gestation. There is less firm evidence on the timing of delivery in women with poorly controlled GDM, which can be considered between 37 and 38-6/7 weeks’ gestation, taking into account the presence of other risk factors. In women with poor glycemic control but reassuring fetal testing, delivery in the preterm period is not recommended. The presence of hypertensive disorders, other co-morbidities, and non-reassuring fetal testing will alter the delivery timing [ 22 ].
In women with GDM and an estimated fetal weight of ≥4500 g on an ultrasound exam, the recommendation for cesarean is not well established [ 44 ]. In GDM cases with suspected fetal macrosomia, along with being counseled regarding the risks of possible shoulder dystocia with vaginal delivery, benefits and risks of cesarean delivery should also be discussed [ 22 , 45 ]. The timing and anticipated route of delivery should be reviewed with a patient during the third trimester.
16. Intrapartum Glucose Management
During labor, women who have required insulin for GDM may have their blood glucose levels checked every hour or less frequently, depending on the antepartum requirements. Women that are diet-controlled can have less frequent blood glucose monitoring during labor, about every 4 h. Based on the findings of a recent randomized controlled trial of GDM patients in labor, blood sugar levels in the range of 60–120 mg/dL are acceptable. In the trial, tight intrapartum blood glucose control with a maternal blood glucose levels of 70–100 mg/dL was compared to more liberal control of 60–120 mg/dL. It was found that there was no difference in neonatal glucose concentrations between the two groups [ 46 ]. Based on the results of the study, when the blood glucose levels during labor are >120 mg/dL, intrapartum insulin must be considered.
The starting dose or rate of intrapartum insulin is generally based on the severity of the elevated blood glucose value. If an insulin infusion is started, hourly blood glucose levels should be checked with the insulin rate adjusted based on the hourly blood glucose level. Insulin is infused in a 5% Dextrose solution or normal saline 0.9% (NS) at 125 mL/hour. The 5% glucose can be exchanged with NS if the blood glucose level is persistently greater than 180 mg/dL. Insulin infusions can be used for women undergoing both vaginal and cesarean delivery; however, in patients undergoing cesarean section, a one-time subcutaneous insulin injection can be considered to achieve euglycemia at the time of delivery. This management is one of many options to ensure euglycemia in the mother prior to delivery. There are a variety of other protocols that have been described in the literature to optimize intrapartum glucose control and the insulin protocol used may vary by institution.
17. Postpartum
Following delivery, insulin and oral agents should be discontinued. In the immediate postpartum period, a fasting blood glucose level can be checked to determine if the patient has persistent hyperglycemia. A fasting plasma glucose level of ≥126 mg/dL or postprandial level of ≥200 mg/dL confirm persistent hyperglycemia. These patients are recommended to continue the diet modifications and lifestyle changes and may need to be treated with pharmacological agents. Insulin or glyburide can be prescribed in the postpartum period even in breast-feeding mothers, without concern for neonatal side effects [ 47 ].
In women with GDM, the risk for subsequent development of type II diabetes mellitus is estimated to be 10 times the control population, at 16.15% when followed up for 10 years. Some studies show the risk to be as high as 60% [ 47 , 48 ]. Postpartum fasting blood glucose often fails to identify patients with impaired glucose tolerance and those with type 2 diabetes mellitus. The Fifth International Workshop on Gestational Diabetes recommends women complete a 75-g 2-h oral glucose tolerance test at 6 to 12 weeks after delivery [ 44 ]. A fasting plasma glucose level of <100 mg/dL and a 2-h post-load plasma glucose level of <140 mg/dL are considered normal. When the fasting plasma glucose level is 100–125 mg/dL or the 2-h post-load glucose level is 140–199 mg/dL, the patient is considered to have impaired glucose tolerance. When the fasting plasma glucose level is ≥126 mg/dL or the 2-h post-load level is ≥200 mg/dL, the patient is diagnosed with diabetes mellitus. Recent data support performing the 2-h glucose tolerance test in the immediate postpartum period while the patient is still hospitalized [ 49 ]. This immediate postpartum glucose screening has not become standardized yet and this approach should be individualized based on the patient population and resources in the community. In conclusion, postpartum women need to be reminded of the importance of postpartum follow-up for the development of type II diabetes mellitus.
There are limitations to this review. Due to the vast amount of literature published on the subject, by various countries, it is possible that some important publications may have not been included in this review. The review was limited to the management of gestational diabetes in the United States, and this may differ in other countries where the incidence of obesity may be lower and resources available to patients may be different.
18. Discussion
Based on the published literature, we have reviewed the history of GDM, screening options, and management, with focus on the management options for persistent hyperglycemia. We report an increasing incidence of diabetes and GDM in association with increasing incidence in maternal obesity.
Prevalence of GDM in the US has increased from <1% in 1961 to about 10%, using the two-step GTT for screening. With the introduction of the one-step 2-h 75-g GTT recommended by the IADPSG, about 18% in the US would qualify as having GDM (13–15). Due to a lack of evidence of improvement in pregnancy outcomes based on the one-step GTT, pregnant patients in the US continue to be screened at 24–28 weeks with the two-step GTT as recommended by the ACOG. Patients not previously diagnosed with diabetes mellitus but with risk factors for developing GDM are recommended to be screened earlier in gestation, and if negative, the screen is repeated at 24–28 weeks.
Patients with GDM are followed closely with self-monitoring of blood glucose levels, and lifestyle changes which include modification in diet and exercise. In those with persistent hyperglycemia despite lifestyle changes, treatment with medications must be considered. Due to potential maternal and perinatal side effects of the oral hypoglycemic agents, insulin is the preferred treatment. Insulin regimens consist of short-, intermediate-, and long-acting insulin. The type, dose, and timing of insulin are determined by the timing and severity of hyperglycemia, and treatment is customized for each patient. Patients are followed closely, and the dose of insulin is adjusted at regular intervals based on the patient’s blood glucose levels.
In patients unable to take insulin, oral hypoglycemic agents can be considered. Metformin is preferred over glyburide due to the risk of possible neonatal hypoglycemia associated with maternal administration of glyburide. Typically, the dose of oral medications is once or twice a day, with increments in doses based on the degree, timing, and persistence of hyperglycemia.
In patients requiring medications for persistent hyperglycemia, due to increased perinatal risks, fetal wellbeing tests are initiated by 32 weeks’ gestation. In patients that are well-controlled GDM on medications, delivery should be planned at 39 weeks and not earlier. However, in cases of maternal complications, poorly controlled hyperglycemia, or non-assuring fetal testing, the delivery may be considered earlier.
Due to the increased incidence of impaired glucose tolerance or type 2 diabetes mellitus in patients with GDM, patient must be screened for diabetes in the postpartum period using the 2-h GTT. Long-term risk for development of type 2 diabetes mellitus is high. For early diagnosis of type 2 DM and to prevent long-term effects of diabetes mellitus, regular follow-up exams and testing, at a minimum of every 1–3 years, are recommended.
Among high-risk patients that test positive for GDM on early GTT, some may indeed have type 2 diabetes mellitus that was previously undiagnosed. The ideal screening test in this population is not known as well as how early it should be administered. Patients diagnosed with GDM prior to 20 weeks are frequently managed similar to those with type 2 diabetes mellitus, but data on the pregnancy outcomes and perinatal outcomes in this group have not been well studied. Future studies on pregnancy outcomes in this population will become increasingly important, as the maternal obesity rates continue to rise. Whether obesity independently compounds the pregnancy risks in patients diagnosed with early onset of hyperglycemia is also an area of research.
Author Contributions
Both authors contributed equally to the methodology, resources, data collection, writing, review and editing. A.R. is the senior author. The literature review, resources, data curation, writing and editing, and visualization were performed by authors A.R. and M.L. No methodology, software, validation, analysis, investigation, and data curation. Supervision of the project was conducted by A.R. No funding acquisition was conducted for this project. All authors have read and agreed to the published version of the manuscript.
This research received no external funding.
Conflicts of Interest
The authors declare no conflict of interest.
Publisher’s Note: MDPI stays neutral with regard to jurisdictional claims in published maps and institutional affiliations.
- Alzheimer's disease & dementia
- Arthritis & Rheumatism
- Attention deficit disorders
- Autism spectrum disorders
- Biomedical technology
- Diseases, Conditions, Syndromes
- Endocrinology & Metabolism
- Gastroenterology
- Gerontology & Geriatrics
- Health informatics
- Inflammatory disorders
- Medical economics
- Medical research
- Medications
- Neuroscience
- Obstetrics & gynaecology
- Oncology & Cancer
- Ophthalmology
- Overweight & Obesity
- Parkinson's & Movement disorders
- Psychology & Psychiatry
- Radiology & Imaging
- Sleep disorders
- Sports medicine & Kinesiology
- Vaccination
- Breast cancer
- Cardiovascular disease
- Chronic obstructive pulmonary disease
- Colon cancer
- Coronary artery disease
- Heart attack
- Heart disease
- High blood pressure
- Kidney disease
- Lung cancer
- Multiple sclerosis
- Myocardial infarction
- Ovarian cancer
- Post traumatic stress disorder
- Rheumatoid arthritis
- Schizophrenia
- Skin cancer
- Type 2 diabetes
- Full List »
share this!
March 21, 2024
This article has been reviewed according to Science X's editorial process and policies . Editors have highlighted the following attributes while ensuring the content's credibility:
fact-checked
trusted source
Preliminary research suggests improving and maintaining heart health after pregnancy may reduce the risk of CVD
by American Heart Association
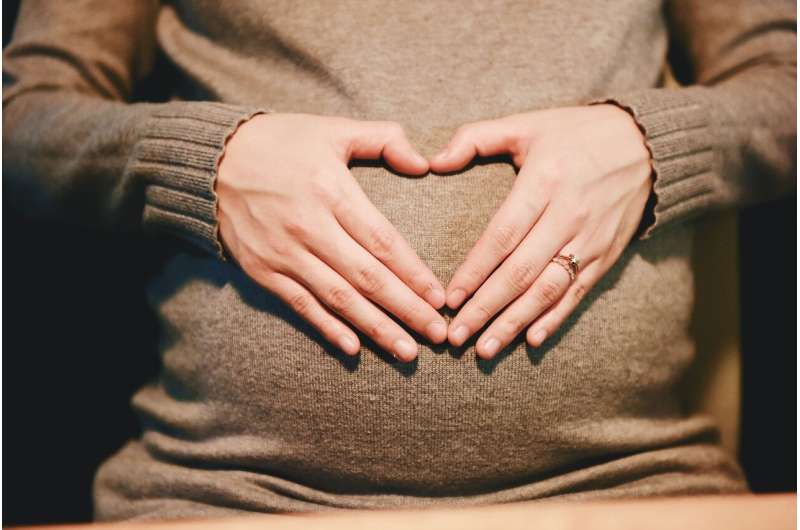
Women with a history of adverse pregnancy complications are at significantly higher risk of developing heart disease but can reduce their risk by maintaining optimal heart health after pregnancy, according to preliminary research presented at the American Heart Association's Epidemiology and Prevention│Lifestyle and Cardiometabolic Scientific Sessions 2024 , held March 18–21, in Chicago.
"Previous studies have shown that women with a history of adverse pregnancy outcomes tend to have a higher risk of developing cardiovascular disease later in life," said lead study author Frank Qian, M.D., M.P.H., a cardiovascular medicine fellow at Boston Medical Center and clinical instructor at the Boston University Chobanian & Avedisian School of Medicine.
"However, it is unknown how much of this increased cardiovascular disease risk can be potentially modified by healthy lifestyle behaviors."
Adverse pregnancy outcomes examined in this study included placental abruption, gestational diabetes, small size for gestational age, pre-term birth and/or hypertensive disorders of pregnancy, defined as preeclampsia or gestational hypertension. Additionally, according to the Association's 2021 statement on Adverse Pregnancy Outcomes and Cardiovascular Disease Risk , many adverse pregnancy outcomes are associated with the future development of CVD risk factors, including hypertension, type 2 diabetes and dyslipidemia.
In this study, researchers reviewed data from the UK Biobank to evaluate the association between Life's Essential 8 scores and the development of cardiovascular disease in women with and without a history of adverse pregnancy outcomes.
Life's Essential 8 are key measures defined by the American Heart Association for improving and maintaining optimal cardiovascular health, including healthy diet, physical activity, smoking cessation, healthy sleep habits and managing weight, cholesterol, blood sugar and blood pressure. Cardiovascular health scores range from 0–100, with higher scores indicating better cardiovascular health.
The analysis found significant interactions among adverse pregnancy outcomes, cardiovascular health scores and cardiovascular events during the 13.5-year follow-up period:
- Women who had better cardiovascular health measures after pregnancy, or Life's Essential 8 scores of 76 or higher, had a 57% lower risk of developing cardiovascular disease compared to women with scores of 67 and below.
- Women who had complications during pregnancy and poor cardiovascular health scores after pregnancy had a 148% increase in the risk of developing cardiovascular disease.
- Among women with a history of pregnancy complications, those who achieved or maintained high heart health after pregnancy were at a similar risk for heart disease as women without adverse pregnancy outcomes and good cardiovascular health.
- Women with intermediate and low Life's Essential 8 scores (scores of 68.2 to 77.5 and below 68.1, respectively) had an elevated risk for heart disease—25% and 81%, respectively—whether or not they had a prior history of adverse pregnancy outcomes.
"We were most surprised to find that women who had a history of pregnancy complications and were able to achieve and maintain a high level of cardiovascular health after pregnancy significantly reduced their risk of future cardiovascular disease. They essentially had an equivalent CVD risk to women without no history of adverse pregnancy outcomes who also had high cardiovascular health," Qian said.
"These findings are important for clinical practice as well as designing public health interventions and policies. We need to identify high-risk women and focus on ensuring they have access to lifestyle or treatment to reduce their long-term risk of cardiovascular disease."
Study background and details:
- The UK Biobank is a large biomedical database and research resource with health records of about 500,000 adults—enrolled from 2006 until 2010—who live in the U.K and received health care through the U.K."s National Health Service. The researchers accessed the data in April 2023.
- The study included 2,263 women with a prior diagnosis of adverse pregnancy outcomes and 107,260 women who had no history of complications during pregnancy. None of the participants had cardiovascular disease at the beginning of the study.
- Mean age of participants at the time of enrollment was 50.2 years among women with a history of adverse pregnancy outcomes and 56.6 years among women without a history of adverse pregnancy outcomes.
- Over the average 13.5 years of follow-up, 197 cardiovascular disease events were documented in women with a history of adverse pregnancy outcomes.
- 95.2% of the participants self-identified as white; 4.8% self-identified as other race, which the researcher combined as one group to meet analytical thresholds. Cardiovascular outcomes were not statistically different between the two groups, Qian said.
- The proportion of women who had an adverse pregnancy outcome and self-identified as non-white was 8.2%, compared to 4.8% of the participants who had no history of an adverse pregnancy outcome.
The study's limitations included that it is an observational analysis, meaning the findings do not confirm cause and effect, and that more than 94% of the study population from the UK Biobank self-identified as white race, meaning the findings may not be generalizable to people of other races or ethnicities.
"Our study did not include enough women from other diverse races or ethnicities to have to be able to understand how cardiovascular health metrics after an adverse pregnancy outcome may influence long-term CVD risk," Qian said.
"That being said, we observed a trend towards lower risk for cardiovascular disease in women who maintained better cardiovascular health after pregnancy, so these improvements in cardiovascular health should also be beneficial to women of other races and ethnicities, though more research is needed to confirm this hypothesis."
Nieca Goldberg, M.D., a clinical associate professor of medicine at NYU Grossman School of Medicine, medical director of Atria NY and an American Heart Association volunteer expert who was not involved in the study, said, "This is an important study because it indicates a role for prevention to lower heart disease risk in women who have pregnancy related disorders of preeclampsia, pre-term birth and gestational diabetes.
"When applying Life's Essential 8 risk scores, those women with the lowest scores were at higher risk for cardiovascular disease . We need to encourage health care professionals to integrate Life's Essential 8 into clinical practice to improve heart health in women with and without pregnancy -related disorders. In addition, we need additional research to evaluate the benefits of Life's Essential 8 in diverse populations of women ."
Explore further
Feedback to editors

Researchers determine underlying mechanisms of inherited disorder that causes bone marrow failure
2 hours ago

Intervention after first seizure may prevent long-term epilepsy

New genomic method offers diagnosis for patients with unexplained kidney failure

Researchers uncover protein interactions controlling fertility in female mice

Scientists close in on TB blood test that could detect millions of silent spreaders

New study reveals preventable-suicide risk profiles
3 hours ago

Anti-inflammatory molecules show promise in reducing risks of further heart damage

A boost to biomedical research with statistical tools: From COVID-19 analysis to data management
4 hours ago

UK study provides insights into COVID-19 vaccine uptake among children and young people

Researchers describe tools to better understand CaMKII, a protein involved in brain and heart disease
Related stories.

Complicated pregnancies increase the risk of dying of cardiovascular diseases, finds study
Mar 5, 2024

Pregnancy complications tied to higher risk of later hypertension
Oct 23, 2019

Adverse pregnancy outcomes may help identify future heart disease risk
Feb 27, 2023

New study finds that pregnancy complications can also affect child's health later in life
Feb 12, 2024

Maternal obesity predicts heart disease risk better than pregnancy complications, finds study
Oct 10, 2023

High blood pressure, pregnancy complications may greatly raise moms' future heart risks
May 12, 2023
Recommended for you

Risk of adverse pre-eclampsia outcomes accurately identified through new AI model
5 hours ago

A bioelectronic mesh capable of growing with cardiac tissues for comprehensive heart monitoring
Mar 21, 2024

Cardiac amyloidosis: New AI system developed for early diagnosis

Researchers create real-time view of placental development in mice
Let us know if there is a problem with our content.
Use this form if you have come across a typo, inaccuracy or would like to send an edit request for the content on this page. For general inquiries, please use our contact form . For general feedback, use the public comments section below (please adhere to guidelines ).
Please select the most appropriate category to facilitate processing of your request
Thank you for taking time to provide your feedback to the editors.
Your feedback is important to us. However, we do not guarantee individual replies due to the high volume of messages.
E-mail the story
Your email address is used only to let the recipient know who sent the email. Neither your address nor the recipient's address will be used for any other purpose. The information you enter will appear in your e-mail message and is not retained by Medical Xpress in any form.
Newsletter sign up
Get weekly and/or daily updates delivered to your inbox. You can unsubscribe at any time and we'll never share your details to third parties.
More information Privacy policy
Donate and enjoy an ad-free experience
We keep our content available to everyone. Consider supporting Science X's mission by getting a premium account.
E-mail newsletter
Thank you for visiting nature.com. You are using a browser version with limited support for CSS. To obtain the best experience, we recommend you use a more up to date browser (or turn off compatibility mode in Internet Explorer). In the meantime, to ensure continued support, we are displaying the site without styles and JavaScript.
- View all journals
- My Account Login
- Explore content
- About the journal
- Publish with us
- Sign up for alerts
- Open access
- Published: 22 March 2024
Diet, lifestyle and gut microbiota composition among Malaysian women with gestational diabetes mellitus: a prospective cohort study
- Thubasni Kunasegaran 1 ,
- Vinod R. M. T. Balasubramaniam 1 ,
- Valliammai Jayanthi Thirunavuk Arasoo 1 ,
- Uma Devi Palanisamy 1 ,
- Yen Ker Tan 1 , 2 &
- Amutha Ramadas 1
Scientific Reports volume 14 , Article number: 6891 ( 2024 ) Cite this article
Metrics details
- Applied microbiology
- Risk factors
The study addressed a significant gap in the profiling and understanding of the gut microbiota’s influence on Malaysian Malay women with gestational diabetes mellitus (GDM). This prospective cohort study aimed to explore the intricate relationship between gut microbiota, dietary choices, and lifestyle factors among Malay women, both with and without GDM. The research specifically focused on participants during the second (T0) and third (T1) trimesters of pregnancy in Johor Bahru, Malaysia. In Part 1 of the study, a diverse pool of pregnant women at T0 was categorized into two groups: those diagnosed with GDM and those without GDM, with a total sample size of 105 individuals. The assessments encompassed demographic, clinical, lifestyle, and dietary factors at the T0 and T1 trimesters. Part 2 of the study delved into microbiome analysis, targeting a better understanding of the gut microbiota among the participants. Stool samples were randomly collected from 50% of the individuals in each group (GDM and non-GDM) at T0 and T1. The collected samples underwent processing, and 16s rRNA metagenomic analysis was employed to study the microbial composition. The results suggested an association between elevated body weight and glucose levels, poor sleep quality, lack of physical activity, greater intake of iron and meat, and reduced fruit consumption among women with GDM compared to non-GDM groups. The microbiome analysis revealed changes in microbial composition over time, with reduced diversity observed in the GDM group during the third trimester. The genera Lactiplantibacillus , Parvibacter , Prevotellaceae UCG001 , and Vagococcus positively correlated with physical activity levels in GDM women in the second trimester. Similarly, the genus Victivallis exhibited a strong positive correlation with gravida and parity. On the contrary, the genus Bacteroides and Roseburia showed a negative correlation with omega-3 polyunsaturated fatty acids (PUFAs) in women without GDM in the third trimester. The study highlighted the multifaceted nature of GDM, involving a combination of lifestyle factors, dietary choices, and changes in gut microbiota composition. The findings emphasized the importance of considering these interconnected elements in understanding and managing gestational diabetes among Malaysian Malay women. Further exploration is essential to comprehend the mechanisms underlying this relationship and develop targeted interventions for effective GDM management.
Introduction
Gestational diabetes mellitus (GDM) is defined as glucose intolerance with the first onset or recognition during pregnancy and is associated with adverse maternal and neonatal outcomes 1 , 2 . Approximately 1 in 6 live births are affected by diabetes in pregnancy, 84% of which are diagnosed as GDM 1 , 2 . The global prevalence of GDM has been rising, including in Malaysia, where it contributes to approximately 23.2% of cases 1 . This increase raises significant concerns due to the potential adverse consequences for mothers and their offspring.
Understanding GDM and its root factors is essential for effective prevention and management strategies. Previous research has provided evidence suggesting a potential connection between the gut microbiome and the development and progression of GDM 3 , 4 , 5 . The gut microbiome refers to the community of microorganisms residing in the gastrointestinal tract. It is crucial in various physiological processes, including metabolism and immune function 6 . Furthermore, clinical characteristics, diet intake, lifestyle habits, and alterations in the gut microbial composition have been associated with metabolic disorders and pregnancy-related complications 7 , 8 .
While a growing body of research explores the relationship between the gut microbiome and GDM, most studies have focused on populations in other regions, and limited research has been conducted on the Malaysian population 9 . The Malaysian government has prioritized research in nutrition, precision medicine, and the promotion of maternal and neonatal health, emphasizing the need to address GDM in the country. Abdullah et al. 9 found no significant variations in the gut microbiota composition between the first and third trimesters among pregnant women in Malaysia. However, the study lacked detailed dietary and lifestyle information, making it impossible to establish correlations between Malaysian pregnant women's gut microbiota profile, dietary intake, and lifestyle factors. Additionally, the study design did not involve comparing women with medical conditions, such as GDM, with healthy pregnant women.
Given the unique ethnic and cultural context of the Malaysian population, particularly among Malays, who constitute about 70% of women diagnosed with GDM 10 , there is an urgent need for targeted and culturally relevant research. This study addressed existing knowledge gaps by investigating the associations between diet, lifestyle, gut microbiota composition, and GDM among Malaysian Malay women in the second (T0) and third (T1) trimesters.
The research question that guided this study was: How did dietary habits, lifestyle factors, and gut microbiota composition vary between Malaysian Malay women with GDM and those without GDM during the T0 and T1 trimesters? The hypothesis underlying this study posited that specific dietary patterns and lifestyle factors contributed to distinct variations in gut microbiota composition, which, in turn, might have influenced the development and progression of GDM among Malaysian Malay women.
Patient recruitment
This prospective cohort study was conducted in two government clinics, Klinik Kesihatan Tampoi and Klinik Kesihatan Abdul Samad, in Johor Bahru, Malaysia. By performing the study in these government clinics, the research aimed to capture a diverse population of pregnant women from various demographic backgrounds within Johor Bahru. Ethical approval to conduct the study was obtained from the Malaysian Research Ethics Committee (MREC) (NMRR-19-4186-52297) and Monash University Human Research Ethics Committee (MUHREC) (28975). All protocols in the study were performed following relevant guidelines and regulations. Written informed consent was obtained from participants before the recruitment.
The targeted participants were pregnant women of Malay ethnicity with and without GDM in their T0 trimester who had done oral glucose tolerance tests (OGTT). The participants in the study were identified and approached through a collaborative effort with the nurses at the two government clinics. The study objectives and criteria for participation were communicated to the clinic nurses. The involvement of nurses not only facilitated the identification of eligible participants but also helped establish a sense of trust and credibility among the potential participants, increasing the likelihood of their willingness to participate in the study. Both parties identify potential participants based on their medical records and clinical assessments. The records of pregnant women attending the clinics for routine antenatal visits were reviewed to determine their eligibility for the study.
The exclusion criteria were women who are below 18 years and above 45 years old, mixed parentage and non-Malay ethnicity, unable to provide consent due to impairments or severe mental illness as provided in the medical history, diagnosed with other types of diabetes (Type 1, Type 2, Monogenic or secondary) or other morbidities such as gestational hypertension, cardiovascular diseases, gastrointestinal diseases, anemia, and preeclampsia before and during recruitment into the study, having multiple pregnancies and undergoing other therapies or consuming medications (insulin, antibiotics, probiotics, glucose-lowering drugs, or glucose-increasing drugs during recruitment or at any phase after recruitment which could affect the gut microbial profile or study outcomes.
Sample size calculation
For part 1 of the study, the minimum sample size calculation was done using the web-based EPItools 11 sample size calculator for the cohort study. The findings by Bowers et al. 12 reported Asian GDM mothers with high weight gain to have a 4.42 higher likelihood of having a baby born larger than gestational age (LGA). Based on this, a minimum number of 39 participants will be required in each group to give the study 80% power with 95% confidence. Considering possible 20% dropouts due to the study’s prospective design and extreme values, the sample size has been increased to a minimum of 50 pregnant women in each study group (GDM and non-GDM). It is essential to note that the outcome (large gestational age babies) was factored into the calculation as part of objective 4 of the study, which has been excluded in this article.
For Part 2, the sample size for gut microbial analysis was determined based on statistical considerations and feasibility. The minimum sample size for this study was determined using a web-based web application for sample size and power calculation in microbiome studies 13 . Using an operational taxonomic unit (OTU) of 8 as recommended by Fernandez et al. 14 , standard overdispersion of 0.1, and stratification, a minimum sample size of 16 in each group is sufficient to achieve a minimum power of 80%. Additionally, it is prudent to anticipate the possibility of participant dropouts or incomplete data in research studies. By incorporating a slightly larger sample size, this study sought to mitigate the potential impact of such occurrences on the statistical power of study analysis. Therefore, this study opted for a minimum sample size of 25 to guarantee a standard statistical power of at least 80%.
Besides statistical considerations, feasibility was crucial in determining the sample size. Factors such as time constraints, available resources, and the recruitment capacity of the clinics were considered to ensure a realistic and achievable sample size. Hence, for the part 1 study, 105 participants were recruited, with 50 individuals in the GDM group and 55 individuals in the non-GDM group, to ensure an adequate sample size for the overall analysis and to account for potential dropouts or exclusions during the study period. From this sample, 25 participants from each group were randomly selected for stool collection at two different time points (T0 and T1) for the part 2 study. This subset of participants was chosen to obtain prospective data and assess changes in the gut microbial profile over time. The random selection process ensured unbiased representation from both groups and minimized selection bias.
Data and sample collection
Part 1 of the study recruited pregnant women at T0 and divided them into GDM (n = 50) and non-GDM (n = 55). They completed a questionnaire in the Malay language (Supplementary Table 1 ) consisting of demographic, clinical, lifestyle, and dietary assessments at the T0 and third trimesters (T1). The dietary intake was assessed using three-day 24-h dietary recalls (2 weekdays and one weekend), and the DietPLUS ® 15 was utilized to analyze the energy and nutrient intakes from these recalls. The nutritional data collected from the participants underwent processing to yield energy-adjusted nutrient intakes and detect food groups. This step involved adjusting the nutrient intakes for energy content to account for individual differences in caloric intake. Furthermore, food group analysis was performed to assess the number of servings per day consumed based on different food groups. In addition to the dietary data, the participants' physical activity levels were evaluated using METs (Metabolic Equivalents) calculated from the Global Physical Activity Questionnaire 16 , administered as part of the study instruments. The METs provided a standardized measure of the intensity of physical activity, enabling a comparison of activity levels between the groups. A well-structured questionnaire was used to collect data on participants’ sleeping habits. The questionnaire included specific questions about bedtime and wake-up times during weekdays and weekends, nighttime sleep quality, reasons for waking up during the night, daytime nap duration, and nighttime ambient lighting conditions. Participants rated their nighttime sleep quality on a Likert scale, choosing from “Good,” “Moderate,” or “Not satisfied”. They also selected reasons for waking up during the night from a predefined list. The questionnaire inquired about the duration of daytime naps and the darkness or lightness of the room during nighttime sleep. This structured questionnaire helped collect standardized and detailed information about participants' sleep patterns.
Part 2 of the study involved microbiome analysis. The stool samples were randomly collected from 50% of pool participants from each group: GDM (n = 25) and non-GDM (n = 25). They gave stool samples twice in total, each at T0 and T1. Participants were provided with sterile collection containers (Norgen's Stool Nucleic Acid Collection and Preservation System). Prior to sample collection, participants received a brief explanation of using collection containers. The collected stool samples are frozen at − 80 °C before DNA extraction.
DNA extraction
Approximately 400 µl of liquid samples were processed using the RNEASY PowerMicrobiome kit from QIAGEN (QIAGEN, Hilden, Germany), following the manufacturer's instructions. The extracted DNA was further assessed for concentration and purity using a SpectraMax QuickDrop Micro-Volume Spectrophotometer from Molecular Devices, USA. The ratio of sample absorbance at 260 and 280 nm was employed to assess DNA purity, with a typical high-quality DNA sample having a 260/280 ratio close to 1.8. The extracted DNA was safely stored at − 20 °C, pending sequencing analysis.
V3–V4 16s rRNA sequencing: PCR amplification
Barcoded amplicon libraries spanning the V3-V4 hypervariable region of the 16s rRNA gene were prepared using the 341F-805R primer set 17 . Samples were sequenced using the Illumina MiSeq v2 platform, employing the 2 × 250 bp sequencing mode at the Genomics Facility, Monash University Malaysia. Raw sequence reads were subjected to quality control and processing using the DADA2 R package 18 . This package leverages error profiles to precisely define Amplicon Sequence Variants (ASVs). ASVs were subsequently assigned to taxonomy using a pre-trained naïve Bayes classifier trained on the curated 16s rRNA gene database Greengenes v13_8 (99% OTUs). To maintain data quality, ASVs present in fewer than 5% of samples or with fewer than 100 observations and ASVs accounting for less than 0.01% of total reads were filtered from the final dataset before downstream analysis. Sequence depth analyses and rarefaction were also performed using the Phyloseq R package 19 .
Statistical analyses
All the questionnaire variables were analyzed using IBM SPSS Statistics (version 25). The RStudio software (V.4.2.2) was utilized for gut microbial analysis and its statistical analyses. Initially, the number of reads for each taxon was divided by the total number of reads from all taxa within each sample, enabling the calculation of the relative abundance at different taxonomic levels, including phylum, class, order, family, and genus. The 20 most abundant or frequently observed classes were selected from the data for further analysis.
The relative abundance of a significant class across different conditions was compared using Kruskal–Wallis’s non-parametric test, which is suitable for comparing multiple groups. The resulting p-values were adjusted for multiple comparisons using the False Discovery Rate (FDR) method. Alpha diversity analysis was performed to evaluate the diversity of microbial taxa within each group. Two metrics were used: richness, representing the number of different taxa, and Shannon's index, which accounts for both the richness and evenness of taxa. The cross-sectional difference in alpha diversity between GDM and non-GDM groups at T0 and T1 was assessed using Student's t -test.
Additionally, the change in alpha diversity within pregnancy was determined using a repeated measures ANOVA test, comparing GDM status and the different time points. For beta diversity analysis, the significant differences in microbial community composition between GDM and non-GDM women at T0 and T1 were calculated using permutational Multivariate Analysis of Variance (pMANOVA) based on weighted UniFrac distances. The UniFrac distances were determined using the Bray–Curtis distance method.
The “LDA Effect Size (LEfSe)” method was employed to identify specific biomarkers within groups and time points. This method, implemented using the MicrobiomeMarker R package 20 , incorporates the Kruskal–Wallis test, Wilcoxon-Rank Sum test, and Linear Discriminant Analysis (LDA) to generate the LEfSe. Spearman’s rank correlation test was applied to examine the associations between gut microbial profiles and the other variables of interest (clinical characteristics, dietary intake, and lifestyle habits) at the given time points (T0 and T1) in Malaysian Malay women with and without GDM.
Part 1: study questionnaires’ findings
This section summarizes the results of the study questionnaires, providing insights into demographic, clinical, lifestyle, and dietary factors among the participants.
Characteristics of the study population
The demographic and clinical characteristics of the recruited participants are shown in Table 1 . The median maternal age for all participants was 31 (IQR = 8), and one-third (66.7%) were between 25 and 34 years. Most pregnant women with at least higher secondary-level education (94.3%) were employed (53.3%). Women with GDM exhibited significantly higher fasting blood glucose (FBG) and 2-h postprandial glucose (2-HPP) compared to the women without GDM (p < 0.001, respectively). Women with GDM also experienced higher weight gain at T0 (p = 0.031) with a median weight of 4 (IQR = 4.8) and 6 (IQR = 3.0) at T1 (p = 0.044) compared to women without GDM. Lastly, the mean blood pressure was higher among women with GDM.
Comparison of lifestyle and dietary factors between study groups at second and third trimesters
Table 2 compares lifestyle factors between the study groups at T0 and T1. A higher proportion of the women with GDM (22.5%) were not satisfied with their sleep quality compared to pregnant women without GDM at T1. Conversely, the darkness level during sleep was associated with the study groups only at T0 (p = 0.018). The level of physical activity was associated with the distribution of the participants according to study groups at T0 but not at T1. The proportion of women with GDM with high physical activity at T0 appears lower than those without GDM (p = 0.035).
Table 3 compares the dietary intake between T0 and T1 trimesters study groups. Interestingly, the GDM group reported a lower median intake of carbohydrates (129.1 g/1000 kcal vs 139.2 g/1000 kcal, p = 0.005) and total fats (7.5 g/1000 kcal vs 41.9 g/1000 kcal, p = 0.001) compared to the non-GDM group at T1. No differences can be observed in any macronutrient intake in T0.
Women with GDM exhibited significantly higher median intake of iron (8.1 mg/1000 kcal vs 6.8 g/1000 kcal, p = 0.012) and retinol (538.5 mg/1000 kcal vs 343.4 mg/1000 kcal, p = 0.033) compared to women without GDM at T0. However, such differences were not seen at T1. Median vitamin C intake was reported to be significantly lower in the GDM group compared to the non-GDM group at T1 (29.6 mg/1000 kcal vs 43.5 mg/1000 kcal, p = 0.009). While the median sugar intake was significantly lower in the GDM group, the median cholesterol and omega 3 PUFA intake was higher.
The median intake of vegetables (1.1 servings/day vs. 0.7 servings/day, p = 0.034), meats (2.3 servings/day vs 1.1 servings/day, p < 0.001), and fats and oils (15.3 servings/day vs 12.0 servings/day, p < 0.001) were significantly higher in the GDM group, compared with the non- GDM group at T0. While the overall fats and oils intake was reduced in T1, the consumption was still relatively higher in the GDM group (p < 0.001). However, the median intake of fruits was lower in the GDM group than in the non-GDM group at T1 (0.1 servings/day vs 0.4 servings/day, p = 0.013).
Part 2: microbiome analysis findings
This section presents microbiome analysis results, highlighting changes in microbial composition over time and any significant correlations with the participants' characteristics, such as dietary habits and lifestyle factors.
Comparison of alpha diversity of gut microbiota between groups in the second and third trimesters
The findings indicated no significant differences in alpha diversity at T0 between groups (Fig. 1 a). In contrast, notable differences were observed at T1 between pregnant women with gestational diabetes mellitus (GDM) and those without GDM (Fig. 1 b). The beta diversity analysis also demonstrated relatively similar microbial community compositions between the non-GDM and GDM groups at T0 and T1 (data not shown).
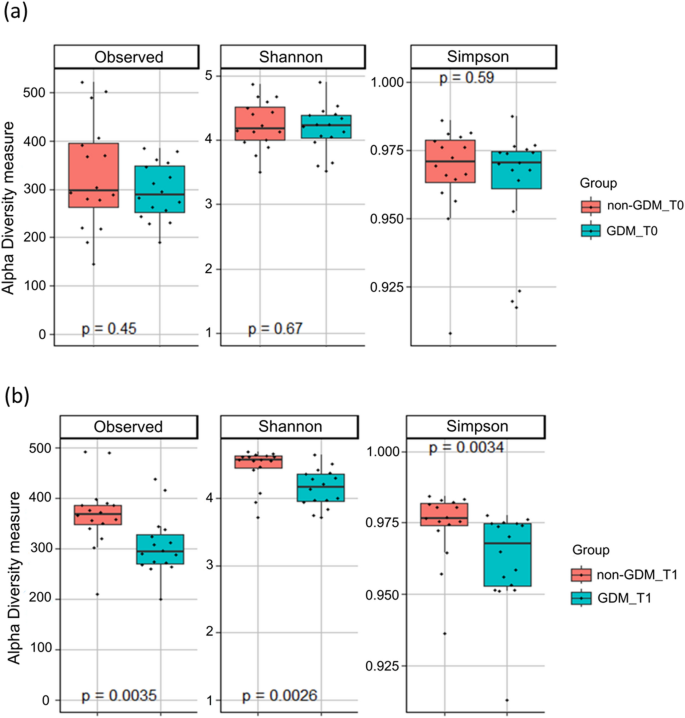
Alpha diversity measures for non-GDM and GDM groups in the second trimester (T0) ( a ) and in the third trimester (T1) ( b ).
Taxonomic analysis of microbial communities at the genus level for GDM and non-GDM groups at second and third trimesters
The taxonomic analysis conducted at the genus level revealed significant differences in the relative abundance of specific microbial genera among different groups (Fig. 2 ). Firstly, the abundance of Bilophila showed a significant difference between the non-GDM group in the T0 and T1 trimesters. It suggests that the relative abundance of Bilophila may change as pregnancy progresses. Similarly, the Lachnospiraceae ND3007 group exhibited a significant difference (p = 0.024) in abundance between the non-GDM group at T0 and T1 (Table 4 ), indicating that this specific group of bacteria may undergo compositional changes during pregnancy. Furthermore, the abundance of Oscillibacter showed a significant difference (p = 0.003) between the non-GDM group at T0 and T1, suggesting that the progression of pregnancy may influence the relative abundance of Oscillibacter .
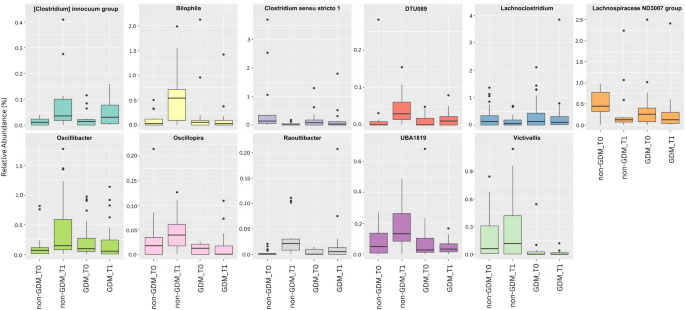
Taxonomic analysis of microbial communities at the genus level.
Additionally, the abundance of Victivallis displayed a significant difference (p = 0.009) between the non-GDM group at T1 and the GDM group at T1. It suggests a potential association between the presence or abundance of Victivallis and GDM during the mid-third trimester. Lastly, the [Clostridium] innocuum group exhibited a significant difference (p = 0.027) in abundance between the non-GDM group at T0 and T1, indicating that the relative abundance of this group may also change as pregnancy progresses.
Identification of marker abundance
In the non-GDM group at T0, three microbial taxa, species Oscillospira , Elusimicrobium , and Terrisporobacter , showed enrichment compared to other groups (Fig. 3 ). In the non-GDM group at T1, several microbial taxa, including genus and species Ruminococcaceae , Bacteroides_caccae , Eggerthellaceae , Incertae Sedis, and species Roseburia , wadsworthia , Oscillibacter , Lachnospiraceae UCG010 , UBA1819 , Flavonifractor , [Clostridium] innocuum group , butyriciproducens , Raoultibacter_timonensis , Phocea_massiliensis , [Eubacterium] fissicatena group , DTU089 , exhibited enrichment compared to other groups.
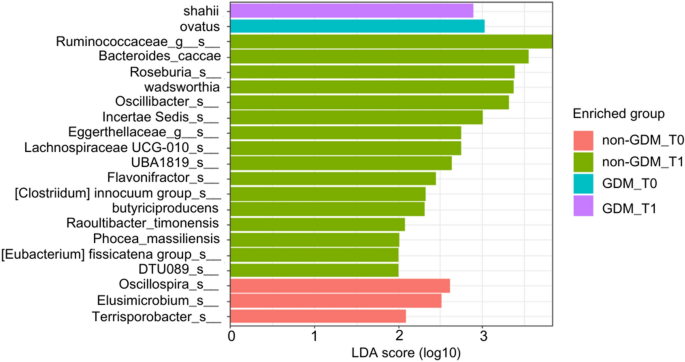
Bar plot showing the LDA score (log 10) for the abundance of potential biomarker taxa identified using the LEfSe approach for the two groups (non-GDM and GDM) at T0 and T1.
In the GDM group at T0, the species Ovatus showed enrichment to other groups. In the GDM group at T1, the species Shahii belonging to the genus Alistipes showed enrichment compared to other groups. Figure 3 presents the bar plot for the Linear Discriminant Analysis (LDA) score (log 10). Supplementary Table 2 provides information on the enrichment of specific microbial features in two groups (non-GDM and GDM at T0 and T1) and their corresponding effect sizes linear discriminant analysis (ef_lda), p-values, and FDR.
Correlation between demographic, clinical, lifestyle, and nutrient intake factors to genus taxonomic rank for GDM and non-GDM groups in the second and third trimesters
Several significant correlations were observed in the correlation analysis between clinical, lifestyle, and nutrient intake factors and the genus-level taxonomic rank for the GDM group in the T0. Specifically, four genera, namely Lactiplantibacillus , Parvibacter , Prevotellaceae UCG001 , and Vagococcus , positively correlated with physical activity level (Supplementary Table 3 ). It indicates that a higher level of physical intensity was associated with an increased abundance or presence of these genera in the microbial communities of the GDM group at T0. Additionally, the genus Victivallis exhibited a strong positive correlation with gravida (number of pregnancies) and parity (number of previous live births). These correlations suggest that a higher number of pregnancies and previous live births were associated with an increased abundance or presence of Victivallis in the microbial communities of the GDM group at T0. These findings highlight potential associations between specific clinical and lifestyle factors and the composition of microbial communities in individuals with GDM at T0. Figure 4 a represents the plot of Spearman’s correlation coefficient values between gut microbial taxa and lifestyle and clinical factors in the GDM group at T0. Supplementary Table 3 presents the detected significant correlation values for the GDM group at T0. Conversely, no significant correlations were observed in the analysis of the correlation between clinical, lifestyle, and nutrient intake factors and genus taxonomic rank for the non-GDM group at T0 (results not shown).
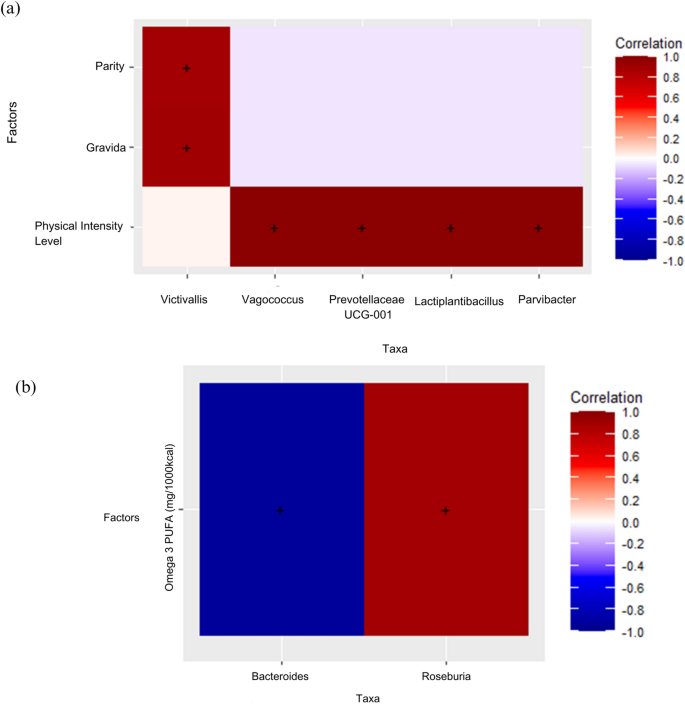
Correlation plot showing Spearman’s correlation coefficient values between gut microbial taxa and lifestyle and clinical factors in the GDM group in the second trimester (T0) ( a ) and correlation plot between gut microbial taxa and Omega-3 PUFA intake (mg/1000 kcal) in the non-GDM group in the third trimester (T0) ( b ). ( a ) Red color represents stronger positive correlations. The X-axis represents the microbial taxa, and the Y-axis represents the parity, gravida, and physical intensity level. ‘+’ signs represent observations with a significant correlation at p-value < 0.05. ( b ) Darker colors represent stronger positive correlations. The X-axis represents the microbial taxa, and the Y-axis represents the nutrient factor. ‘+’ signs represent observations with a significant correlation at p-value < 0.05. Omega3 PUFA (mg/1000 kcal) refers to omega-3 polyunsaturated fatty acids intake per 1000 kcal.
The findings were contradictory in the T1 (Fig. 4 b). No significant correlations were found in the correlation analysis between clinical, lifestyle, and nutrient intake factors and the genus-level taxonomic rank for the GDM group at T1. It suggests no strong associations between these factors and the abundance or presence of specific genera in the microbial communities of individuals with GDM at T1. However, there are two significant correlations for the non-GDM group at T1.
First, the genus Bacteroides showed a strong negative correlation with omega-3 polyunsaturated fatty acids (PUFAs). It suggests that higher levels of omega-3 PUFA intake were associated with decreased abundance or presence of Bacteroides in the microbial communities of the non-GDM group at T1 (Supplementary Table 4 ). Bacteroides is a commonly found genus in the gut microbiota and plays a role in various metabolic processes.
On the other hand, the genus Roseburia exhibited a strong positive correlation with omega-3 PUFAs. It indicates that higher levels of omega-3 PUFA intake were associated with increased abundance or presence of Roseburia in the microbial communities of the non-GDM group at T1. Roseburia is known for its ability to produce short-chain fatty acids, which benefit gut health. These findings suggest a potential link between the intake of omega-3 PUFAs and the composition of microbial communities in individuals without GDM at T1. Figure 4 b represents the plot of Spearman’s correlation coefficient values between gut microbial taxa and lifestyle and clinical factors in the non-GDM group at T1. Supplementary Table 4 presents the detected significant correlation values for the non-GDM group at T1.
In addressing the study's first objective, we sought to discern differences in clinical factors, dietary intake, and lifestyle habits between Malaysian Malay women with and without GDM at the T0 and T1 trimesters. The investigation revealed distinct patterns between the two groups at both time points. Participants with GDM exhibited elevated FBG levels, 2-HPP levels, and increased body weight. Furthermore, they were more likely to fall into the overweight and obese BMI categories. Notable variations in lifestyle habits, including sleep quality, darkness during sleep, and physical activity levels, were observed. Dietary intake also differed, with variations in iron, meat, fats/oils, carbohydrates, and fruit consumption.
In comparison, the current study aligns with systematic reviews 21 , 22 that have consistently reported higher FBG and postprandial levels in GDM groups, indicating poorer glucose tolerance. Notable changes in weight and BMI categories were observed among Malaysian Malay women with and without GDM in the T1, compared to the earlier T0 stage. The higher weight and increased likelihood of being overweight or obese in the GDM group align with previous studies that have reported an association between GDM and higher BMI 23 , 24 , 25 . Changes in weight and BMI during pregnancy, particularly in the context of GDM, require careful consideration. It is expected that women will experience weight gain during pregnancy due to factors such as fetal growth, increased blood volume, and expansion of maternal tissues 26 . Monitoring weight gain and ensuring it aligns with recommended guidelines based on pre-pregnancy BMI is crucial.
Women with GDM had higher total fat intake than those without GDM at T0. This higher fat intake could contribute to increased insulin resistance and impaired glucose metabolism, factors associated with GDM development 27 , 28 . Additionally, the higher iron intake observed in the GDM group aligns with systematic reviews reporting higher iron requirements during pregnancy, particularly in women with GDM 29 . Iron is crucial for maintaining hemoglobin levels and preventing iron deficiency anemia, which can negatively affect maternal and fetal health 30 , 31 , 32 . However, it is essential to note that pregnant women, especially those with other known GDM risk factors, should avoid excessive heme iron-enriched food 29 . Further research is needed to explore the relationship between iron-enriched foods and GDM within the Malaysian Malay population.
Moving to the T1 trimester, the GDM group showed increased meat and fats/oils consumption compared to the non-GDM group. It aligns with studies suggesting a potential association between GDM and a higher intake of animal-based foods and unhealthy fats 33 , 34 . While lean meat sources can provide essential nutrients, excessive red or processed meat intake may increase saturated fat and cholesterol levels, potentially exacerbating insulin resistance 28 , 35 . Moreover, the higher intake of fat/oils in the GDM group could contribute to excessive calorie intake and impact glycemic control 36 . Carbohydrate intake, another crucial aspect in GDM management, exhibited variations between the two groups. The GDM group had higher carbohydrate intake at T0, highlighting the importance of monitoring carbohydrate consumption to prevent rapid blood glucose spikes and challenges in glycemic control 37 , 38 . At T1, both groups showed a decrease in carbohydrate intake, reflecting dietary modifications aimed at managing GDM through carbohydrate monitoring and control. Fruit consumption, often recommended for its valuable nutrient content and fiber, was lower in the GDM group at T0 and T1. Furthermore, lower fruit consumption may be associated with limited nutrient diversity and inadequate fiber intake 39 , 40 , potentially impacting GDM management. This finding raises concerns as fruits provide essential vitamins, minerals, and dietary fiber that support overall health and glycemic control.
The lower satisfaction with sleep quality reported by the GDM group aligns with previous research suggesting a relationship between poor sleep quality and an increased risk of GDM 41 . Disrupted sleep patterns and inadequate sleep duration have been linked to insulin resistance and impaired glucose metabolism, critical factors in GDM pathogenesis 42 , 43 . Interventions aimed at improving sleep quality and promoting healthy sleep habits may have implications for preventing and managing GDM. The difference in darkness during sleep is an intriguing finding. The higher proportion of participants in the GDM group reporting complete darkness during sleep may indicate an association between melatonin secretion and GDM. Melatonin, a hormone released during the night, has been shown to play a role in glucose homeostasis and insulin sensitivity 44 , 45 . Further research is needed to understand the underlying mechanisms and potential interventions related to light exposure during sleep in the context of GDM.
Physical activity levels also differed between the two groups, with the GDM group having a lower proportion of individuals with high physical activity levels. This finding is consistent with a systematic review suggesting an inverse relationship between higher physical activity and GDM risk 46 . Regular physical activity has improved insulin sensitivity, glucose metabolism, and cardiovascular health 47 , 48 . Encouraging pregnant women, including those with GDM, to engage in appropriate physical activities under healthcare guidance can improve glycemic control and overall health outcomes.
The study revealed noteworthy insights in addressing the second objective centered on discerning differences in gut microbial profiles between Malaysian Malay women with and without GDM at the T0 and T1 trimesters. The analysis of gut microbial profiles revealed significant disparities in the abundance of specific microbial groups at different taxonomic levels between the non-GDM and GDM groups. Surprisingly, at T0, no substantial differences in microbial diversity emerged between the two groups. However, by T1, the GDM group demonstrated a marked reduction in alpha diversity compared to the non-GDM group. These outcomes substantiate the initial hypotheses, implying that GDM may influence the composition of the gut microbiome. The unexpected absence of diversity differences at T0 and the subsequent decline in alpha diversity at T1 underscore the dynamic nature of the gut microbiome during pregnancy, with the impact of GDM becoming more apparent in later stages.
Furthermore, the present findings are consistent with previous research suggesting significant differences in the gut microbial diversity and composition between women with and without GDM during different stages of pregnancy. For instance, Koren et al. conducted a study on 91 pregnant women with varying pre-pregnancy BMI and gestational diabetes status and their infants to understand their role in pregnancy better 49 . They found that the gut microbiota underwent significant changes from the first to the third trimester of pregnancy. These changes included a remarkable expansion of microbial diversity among mothers, an overall increase in the abundance of Proteobacteria and Actinobacteria, and a reduction in overall microbial richness. These findings highlight the dynamic nature of the gut microbiota during pregnancy and suggest that the third trimester is a critical period of microbial community remodeling. Similarly, Crusell et al. examined the gut microbiota of pregnant women with (n = 50) and without GDM (n = 157) and found significant differences in microbial diversity and abundance 3 and observed distinct microbial profiles associated with GDM. The diagnosis of GDM during the third trimester of pregnancy is associated with an altered composition of the gut microbiota compared to pregnant women with normal blood sugar levels 3 . The present study and previous investigations 3 , 49 , 50 provide compelling evidence for the influence of GDM on gut microbiome composition. It suggests that GDM, as a metabolic disorder, can impact the diversity and abundance of microbial taxa in the gut as pregnancy progresses. It supports the concept that metabolic dysregulation associated with GDM may extend beyond glucose metabolism and affect other physiological processes, including the gut microbiota.
Furthermore, in the present study, the taxonomic analysis at the genus level revealed a difference in the abundance of Victivallis between the non-GDM and GDM groups. It is significantly reduced in the GDM group than non-GDM at T1. This finding suggests a potential association between the reduced abundance of Victivallis and the development of GDM. However, there is a lack of existing literature specifically exploring the abundance and activities of Victivallis in disease development. Victivallis is a recently described genus, and its role in the gut microbiome, diseases, and complete characterization is yet to be fully understood 51 , 52 . Further investigations are necessary for the species Victivallis due to its recent discovery and limited understanding of its role in the gut microbiome and its potential implications for diseases such as GDM. Understanding the functional significance of Victivallis in GDM pathophysiology is crucial to gain insights into its potential involvement in metabolic regulation, inflammation, and other relevant processes. Identifying reduced Victivallis abundance in Malaysian Malay pregnant women with GDM compared to non-GDM during the third trimester highlights the need for further research to validate and expand upon these findings.
The analysis provided valuable insights in exploring the final objective to reveal associations between clinical factors, dietary intake, lifestyle habits, and gut microbial profiles during pregnancy (T0 and T1) in Malaysian Malay women with and without GDM. Positive correlations were observed between Bacteroides and omega-3 PUFAs in Malaysian Malay pregnant women without GDM at T1. Negative correlations were observed between Roseburia and omega-3 PUFAs. It suggests a potential link between dietary intake of omega-3 PUFAs and gut microbiota composition during the later stages of pregnancy. Omega-3 PUFAs are known for their anti-inflammatory properties and have been associated with various health benefits. Previous studies have also reported associations between dietary factors, particularly omega-3 PUFAs, and gut microbiota composition 53 , 54 , 55 , 56 , 57 . However, further research is needed to understand the underlying mechanisms and their implications for maternal and fetal health.
The study also identified positive correlations between specific microbial taxa ( Lactiplantibacillus , Parvibacter , Prevotellaceae UCG001 , Vagococcus , and Victivallis ) and physical activity levels in Malaysian Malay pregnant women with GDM at T0. Physical activity has been recognized as a modulator of the gut microbiome, influencing microbial diversity and composition. The findings suggest that physical activity level during the T0 may be associated with specific microbial taxa in GDM individuals, highlighting the potential impact of exercise on the gut microbiome in the context of GDM 58 , 59 , 60 .
Furthermore, correlations were observed between microbial taxa and gravida and parity. Gravida refers to the number of pregnancies a woman has had, while parity refers to the number of births. Previous research has shown that parity influences the gut microbiota of offspring 61 , indicating its impact beyond the mother. These associations suggest that the reproductive history of GDM individuals, as indicated by gravida and parity, may contribute to variations in the gut microbial composition during the T0 among Malaysian Malay women.
Indeed, the observed microbial variations in Malaysian Malay women with and without GDM raise exciting questions about the potential role of gut microbiota in developing and progressing GDM. While the exact mechanisms are still being investigated, several hypotheses can be proposed based on the current findings.
Firstly, the gut microbiota has been implicated in regulating glucose metabolism and insulin sensitivity 62 , 63 . Disruptions in the composition and diversity of gut microbiota in Malaysian Malay pregnant women with GDM may lead to impaired glucose metabolism and insulin resistance, both critical factors in the development of GDM.
Secondly, imbalances in gut microbiota can trigger an inflammatory response and affect immune regulation 64 , 65 . Chronic low-grade inflammation contributes to insulin resistance and the pathogenesis of GDM. Changes in the gut microbial composition in Malaysian Malay pregnant women with GDM may influence the production of inflammatory markers and modulate the immune response, thereby influencing the risk of GDM.
Thirdly, the current study's findings suggest that specific bacterial markers associated with butyrate production exist in non-GDM individuals but not those with GDM. These markers include taxa such as Ruminococcaceae , Roseburia species, Oscillibacter species, Lachnospiraceae UCG010 , Flavonifractor, Butyriciproducens , [Clostridium] innocuum group , and Bacteroides_caccae . Butyrate, a short-chain fatty acid (SCFA), is produced by certain bacteria in the gut through the fermentation of dietary fibers 66 , 67 . It has been recognized as an essential energy source for colonocytes and has shown the ability to regulate glucose and lipid metabolism 68 . The correlation between butyrate-producing bacteria and metabolic homeostasis suggests that these bacteria may be crucial in maintaining metabolic health. The current findings imply that a lack of abundance of butyrate-producing bacteria, as observed in GDM individuals, may have implications for glucose and lipid metabolism regulation. Consequently, alterations in the gut microbiota, precisely the absence or reduced abundance of specific butyrate-producing bacteria in the GDM group, may contribute to the development or progression of GDM.
Lastly, dysbiosis in the gut microbiota can increase gut permeability, allowing the translocation of bacterial products such as lipopolysaccharides (LPS) into the bloodstream 69 , 70 . This phenomenon, called endotoxemia, may trigger an inflammatory response and contribute to insulin resistance and metabolic dysfunction in Malaysian Malay pregnant women with GDM.
It is important to note that the observed microbial variations may not solely be a cause but rather a consequence or marker of GDM. Further research is needed to establish causality and elucidate the complex interplay between gut microbiota, metabolic processes, and GDM development in Malaysian Malay pregnant women with GDM.
Study’s strengths
Overall, the study design and methodology possess several strengths that contribute to the quality and credibility of the findings. The prospective cohort design, inclusion of control and condition groups, comprehensive assessment of variables, utilization of advanced molecular techniques, and use of validated measurement tools collectively enhance the scientific rigor of the study. These strengths increase the reliability and generalizability of the findings, making them valuable contributions to the existing knowledge on the gut microbiome, GDM, and associated factors during pregnancy.
Study’s limitations and potential sources of bias
While this study has provided valuable insights into the gut microbiome to GDM and pregnancy outcomes, it also acknowledged and explored the limitations and potential sources of bias that may have influenced the results. One potential limitation is the relatively small sample size of the study. The sample size in both the GDM and non-GDM groups may limit the generalizability of the findings to larger populations. A larger sample size would allow for more robust statistical analyses and uncover additional associations. Furthermore, the study was conducted at only two clinics in the southern part of Malaysia, which may introduce some site-specific biases and limit the generalizability of the findings to another setting or population within Malaysia and worldwide. Secondly, the study relied on self-reported measures for various variables, including dietary intake, physical activity, and lifestyle factors. Self-reported data are subject to recall bias and social desirability bias, which may impact the accuracy and reliability of the information collected. Thirdly, the study's findings are based on associations and correlations rather than mechanistic explanations. The underlying mechanisms remain unclear, but the associations between the gut microbiome and various factors have been identified.
In conclusion, the study delved into the nuanced landscape of GDM among Malaysian Malay women, revealing significant disparities in clinical factors, dietary patterns, and lifestyle habits during pregnancy. Participants with GDM exhibited elevated glucose levels, increased body weight, and distinct lifestyle variations. The exploration of gut microbial profiles highlighted a dynamic shift in diversity, particularly a reduction in alpha diversity in the GDM group during the third trimester. The findings highlight the intricate interplay between GDM, maternal health parameters, and the evolving gut microbiome.
Recommendations
Future research should focus on elucidating the underlying mechanisms, exploring longitudinal changes, investigating the impact of dietary interventions, studying the gut-brain axis, considering the interplay with host genetics, and examining environmental factors in the context of GDM and the gut microbiome. Addressing these knowledge gaps will advance our understanding of the complex interactions between the gut microbiome, GDM, and maternal and neonatal outcomes and pave the way for targeted interventions and improved clinical management strategies for Malay Malaysian pregnant women.
Data availability
The datasets generated and analyzed during the study will not be publicly available due to patient confidentiality rules.
International Diabetes Federation. IDF Diabetes Atlas 10th edn. (International Diabetes Federation, 2021).
Google Scholar
Cho, N. H. et al. IDF Diabetes Atlas: Global estimates of diabetes prevalence for 2017 and projections for 2045. Diabetes Res. Clin. Pract. 138 , 271–281 (2018).
Article CAS PubMed Google Scholar
Crusell, M. K. W. et al. Gestational diabetes is associated with change in the gut microbiota composition in third trimester of pregnancy and postpartum. Microbiome 6 , 89 (2018).
Article PubMed PubMed Central Google Scholar
Ferrocino, I. et al. Changes in the gut microbiota composition during pregnancy in patients with gestational diabetes mellitus (GDM). Sci. Rep. 8 , 12216 (2018).
Kuang, Y. S. et al. Connections between the human gut microbiome and gestational diabetes mellitus. GigaScience 6 , 1–12 (2017).
Thursby, E. & Juge, N. Introduction to the human gut microbiota. Biochem. J. 474 , 1823–1836 (2017).
Ponzo, V. et al. Diet-Gut microbiota interactions and gestational diabetes mellitus (GDM). Nutrients 11 , 330 (2019).
Article CAS PubMed PubMed Central Google Scholar
Hasain, Z. et al. Gut microbiota and gestational diabetes mellitus: A review of host-gut microbiota interactions and their therapeutic potential. Front. Cell. Infect. Microbiol. 10 , 188 (2020).
Abdullah, B., Daud, S., Aazmi, M. S., Idorus, M. Y. & Mahamooth, M. I. J. Gut microbiota in pregnant Malaysian women: A comparison between trimesters, body mass index and gestational diabetes status. BMC Pregnancy Childbirth 22 , 152 (2022).
Logakodie, S. et al. Gestational diabetes mellitus: The prevalence, associated factors and foeto-maternal outcome of women attending antenatal care. Malays. Family Physician 12 , 9–17 (2017).
CAS Google Scholar
Sergeant, E. Epitools Epidemiological Calculators. Ausvet. http://epitools.ausvet.com.au . (2018).
Bowers, K. et al. Gestational diabetes, pre-pregnancy obesity and pregnancy weight gain in relation to excess fetal growth: Variations by race/ethnicity. Diabetologia 56 , 1263–1271 (2013).
Mattiello, F. et al. A web application for sample size and power calculation in case-control microbiome studies. Bioinformatics (Oxford, England) 32 , 2038–2040 (2016).
CAS PubMed Google Scholar
Fernández, M. F. et al. Breast cancer and its relationship with the microbiota. Int. J. Environ. Res. Public Health 15 , 1747 (2018).
Tony Ng, K. W. Jr. DietPLUS—A user-friendly ‘2 in 1’ food composition database and calculator of nutrient intakes. Malays. J. Nutr. 16 , 125–130 (2010).
PubMed Google Scholar
Armstrong, T. & Bull, F. Development of the world health organization global physical activity questionnaire (GPAQ). J. Public Health 14 , 66–70 (2006).
Article Google Scholar
Takahashi, S., Tomita, J., Nishioka, K., Hisada, T. & Nishijima, M. Development of a prokaryotic universal primer for simultaneous analysis of Bacteria and Archaea using next-generation sequencing. PLoS One. 9 , e105592. https://doi.org/10.1371/journal.pone.0105592 (2014).
Callahan, B. J. et al. DADA2: High-resolution sample inference from Illumina amplicon data. Nat. Methods 13 , 581–583 (2016).
McMurdie, P. J. & Holmes, S. phyloseq: An R package for reproducible interactive analysis and graphics of microbiome census data. PLoS One 8 , e61217 (2013).
Cao, Y. et al. microbiomeMarker: An R/Bioconductor package for microbiome marker identification and visualization. Bioinformatics 38 , 4027–4029 (2022).
Lee, K. W. et al. Prevalence and risk factors of gestational diabetes mellitus in Asia: A systematic review and meta-analysis. BMC Pregnancy Childbirth 18 , 494 (2018).
Kunasegaran, T., Balasubramaniam, V. R. M. T., Arasoo, V. J. T., Palanisamy, U. D. & Ramadas, A. Gestational diabetes mellitus in southeast asia: A scoping review. Int. J. Environ. Res. Public Health 18 , 1272 (2021).
Martin, K. E., Grivell, R. M., Yelland, L. N. & Dodd, J. M. The influence of maternal BMI and gestational diabetes on pregnancy outcome. Diabetes Res. Clin. Pract. 108 , 508–513 (2015).
Article PubMed Google Scholar
Miao, M. et al. Influence of maternal overweight, obesity and gestational weight gain on the perinatal outcomes in women with gestational diabetes mellitus. Sci. Rep. 7 , 305 (2017).
Kouhkan, A. et al. Gestational diabetes mellitus: Major risk factors and pregnancy-related outcomes: A cohort study. Int. J. Reprod. Med. 19 , 827–836 (2021).
Institute of Medicine (US) and National Research Council (US) Committee to Reexamine IOM Pregnancy Weight Guidelines Composition and components of gestational weight gain: Physiology and metabolism. In Weight Gain During Pregnancy: Reexamining the Guidelines (eds Rasmussen, K. M. & Yaktine, A. L.) (National Academies Press, 2009).
Mizgier, M., Jarzabek-Bielecka, G. & Mruczyk, K. Maternal diet and gestational diabetes mellitus development. J. Maternal-fetal Neonatal Med. 34 , 77–86 (2021).
Article CAS Google Scholar
Schoenaker, D. A., Mishra, G. D., Callaway, L. K. & Soedamah-Muthu, S. S. The role of energy, nutrients, foods, and dietary patterns in the development of gestational diabetes mellitus: A systematic review of observational studies. Diabetes Care 39 , 16–23 (2016).
Zhao, L. et al. Dietary intake of heme iron and body iron status are associated with the risk of gestational diabetes mellitus: A systematic review and meta-analysis. Asia Pac. J. Clin. Nutr. 26 , 1092–1106 (2017).
Feng, Y. et al. The relationship between iron metabolism, stress hormones, and insulin resistance in gestational diabetes mellitus. Nutr. Diabetes 10 , 17 (2020).
Zhang, C. & Rawal, S. Dietary iron intake, iron status, and gestational diabetes. Am. J. Clin. Nutr. 106 , 1672s–1680s (2017).
Kataria, Y., Wu, Y., Horskjær, P. H., Mandrup-Poulsen, T. & Ellervik, C. Iron status and gestational diabetes-a meta-analysis. Nutrients 10 , 621 (2018).
Saraf-Bank, S., Tehrani, H., Haghighatdoost, F., Moosavian, S. P. & Azadbakht, L. The acidity of early pregnancy diet and risk of gestational diabetes mellitus. Clin. Nutr. 37 , 2054–2059 (2018).
Shin, D., Lee, K. W. & Song, W. O. Dietary patterns during pregnancy are associated with risk of gestational diabetes mellitus. Nutrients 7 , 9369–9382 (2015).
Shiraseb, F. et al. Red, white, and processed meat consumption related to inflammatory and metabolic biomarkers among overweight and obese women. Front. Nutr. 9 , 1015566 (2022).
Stubbs, R. J. Impact of carbohydrates, fat and energy density on energy intake. Nat. Med. 27 , 200–201 (2021).
Bradley, P. Refined carbohydrates, phenotypic plasticity and the obesity epidemic. Med. Hypotheses 131 , 109317 (2019).
Vlachos, D., Malisova, S., Lindberg, F. A. & Karaniki, G. Glycemic Index (GI) or Glycemic Load (GL) and dietary interventions for optimizing postprandial hyperglycemia in patients with t2 diabetes: A Review. Nutrients 12 , 1561 (2020).
Liao, Y. P. et al. Fruit, vegetable, and fruit juice consumption and risk of gestational diabetes mellitus: A systematic review and meta-analysis. Nutr. J. 22 , 27 (2023).
Xu, Q. et al. Dietary fiber intake, dietary glycemic load, and the risk of gestational diabetes mellitus during the second trimester: A nested case-control study. Am. J. Clin. Nutr. 30 , 477–486 (2021).
Zhang, X. et al. The effect of sleep impairment on gestational diabetes mellitus: A systematic review and meta-analysis of cohort studies. Sleep Med. 74 , 267–277 (2020).
Briançon-Marjollet, A. et al. The impact of sleep disorders on glucose metabolism: Endocrine and molecular mechanisms. Diabetol. Metab. 7 , 25 (2015).
Gooley, J. J., Mohapatra, L. & Twan, D. C. K. The role of sleep duration and sleep disordered breathing in gestational diabetes mellitus. Neurobiology 4 , 34–43 (2018).
Owino, S. et al. Nocturnal activation of melatonin receptor type 1 signaling modulates diurnal insulin sensitivity via regulation of PI3K activity. J. Pineal Res. 64 , e12462 (2018).
Peschke, E. & Mühlbauer, E. New evidence for a role of melatonin in glucose regulation. Best Pract. Res. Clin. Endocrinol. Metab. 24 , 829–841 (2010).
Aune, D., Sen, A., Henriksen, T., Saugstad, O. D. & Tonstad, S. Physical activity and the risk of gestational diabetes mellitus: A systematic review and dose–response meta-analysis of epidemiological studies. Eur. J. Epidemiol. 31 , 967–997 (2016).
Onaade, O. et al. Physical activity for blood glucose control in gestational diabetes mellitus: Rationale and recommendations for translational behavioral interventions. Eur. J. Epidemiol. 7 , 7 (2021).
van Poppel, M., Ruchat, S. M. & Mottola, M. Physical activity and gestational diabetes mellitus. Med. Sport Sci. 60 , 104–112 (2014).
Koren, O. et al. Host remodeling of the gut microbiome and metabolic changes during pregnancy. Cell 150 , 470–480 (2012).
Kunasegaran, T., Balasubramaniam, V., Arasoo, V. J. T., Palanisamy, U. D. & Ramadas, A. The modulation of gut microbiota composition in the pathophysiology of gestational diabetes mellitus: A systematic review. Biology 10 , 1027 (2021).
Derrien, M., Cho, J. & Hedlund, B. P. Family I. Victivallaceae fam. nov.. Bergey’s Manual Syst. Bacteriol. 2 , 791 (2011).
Zoetendal, E. G., Plugge, C. M., Akkermans, A. D. L. & de Vos, W. M. Victivallis vadensis gen. nov., sp. Nov., a sugar-fermenting anaerobe from human faeces. Int. J. Syst. Evol. Microbiol. 53 , 211–215 (2003).
Vijay, A., Astbury, S., Le Roy, C., Spector, T. D. & Valdes, A. M. The prebiotic effects of omega-3 fatty acid supplementation: A six-week randomised intervention trial. Gut Microbes 13 , 1–11 (2021).
Kaliannan, K., Wang, B., Li, X.-Y., Kim, K.-J. & Kang, J. X. A host-microbiome interaction mediates the opposing effects of omega-6 and omega-3 fatty acids on metabolic endotoxemia. Sci. Rep. 5 , 11276 (2015).
Robertson, R. C. et al. Deficiency of essential dietary n-3 PUFA disrupts the caecal microbiome and metabolome in mice. Br. J. Nutr. 118 , 959–970 (2017).
Menni, C. et al. Omega-3 fatty acids correlate with gut microbiome diversity and production of N-carbamylglutamate in middle aged and elderly women. Sci. Rep. 7 , 11079 (2017).
Bellenger, J., Bellenger, S., Escoula, Q., Bidu, C. & Narce, M. N-3 polyunsaturated fatty acids: An innovative strategy against obesity and related metabolic disorders, intestinal alteration and gut microbiota dysbiosis. Biochimie 159 , 66–71 (2019).
Bressa, C. et al. Differences in gut microbiota profile between women with active lifestyle and sedentary women. PLoS One 12 , e0171352 (2017).
Tzemah Shahar, R. et al. Attributes of physical activity and gut microbiome in adults: A systematic review. Int. J. Sports Med. 41 , 801–814 (2020).
Valeriani, F. et al. Are nutrition and physical activity associated with gut microbiota? A pilot study on a sample of healthy young adults. Ann. Ig. 32 , 521–527 (2020).
Berry, A. S. F. et al. Remodeling of the maternal gut microbiome during pregnancy is shaped by parity. Microbiome 9 , 146 (2021).
Jang, H. R. & Lee, H. Y. Mechanisms linking gut microbial metabolites to insulin resistance. World J. Diabetes 12 , 730–744 (2021).
Utzschneider, K. M., Kratz, M., Damman, C. J. & Hullar, M. Mechanisms linking the gut microbiome and glucose metabolism. J. Clin. Endocrinol. Metab. 101 , 1445–1454 (2016).
Yoo, J. Y., Groer, M., Dutra, S. V. O., Sarkar, A. & McSkimming, D. I. Gut microbiota and immune system interactions. Microorganisms 8 , 7618 (2020).
Al Bander, Z., Nitert, M. D., Mousa, A. & Naderpoor, N. The gut microbiota and inflammation: An overview. Int. J. Environ. Res. Public Health 17 , 7618 (2020).
Fu, J., Zheng, Y., Gao, Y. & Xu, W. Dietary fiber intake and gut microbiota in human health. Microorganisms 10 , 1587 (2022).
Bellamy, L., Casas, J.-P., Hingorani, A. D. & Williams, D. Type 2 diabetes mellitus after gestational diabetes: A systematic review and meta-analysis. Lancet 373 , 1773–1779 (2009).
He, J. et al. Short-chain fatty acids and their association with signalling pathways in inflammation, glucose and lipid metabolism. Int. J. Mol. Sci. 21 , 6356 (2020).
Guo, S., Al-Sadi, R., Said, H. M. & Ma, T. Y. Lipopolysaccharide causes an increase in intestinal tight junction permeability in vitro and in vivo by inducing enterocyte membrane expression and localization of TLR-4 and CD14. Am. J. Pathol. 182 , 375–387 (2013).
Raetz, C. R. & Whitfield, C. Lipopolysaccharide endotoxins. Annu. Rev. Biochem. 71 , 635–700 (2002).
Download references
Acknowledgements
The study was funded by the Jeffrey Cheah School of Medicine and Health Sciences, Monash University Malaysia’s Seed Grant 2020 (SCH SEED/2020/005) and ECR Seed Grant 2021 (ECR-000016). The authors acknowledge Monash University Malaysia Genomics Platform (MUMGP) for the 16sRNA sequencing service. The authors also thank Amili Pte Ltd Singapore for their guidance in gut microbial analysis using the RStudio software.
Author information
Authors and affiliations.
Jeffrey Cheah School of Medicine and Health Sciences, Monash University Malaysia, 47500, Bandar Sunway, Malaysia
Thubasni Kunasegaran, Vinod R. M. T. Balasubramaniam, Valliammai Jayanthi Thirunavuk Arasoo, Uma Devi Palanisamy, Yen Ker Tan & Amutha Ramadas
Mackay Base Hospital, Mackay, QLD, 4740, Australia
Yen Ker Tan
You can also search for this author in PubMed Google Scholar
Contributions
T.K. and A.R. conceptualized and designed the study; T.K. analyzed and processed the data; T.K., Y.K.T. conducted the data and sample collection; T.K. responsible for DNA extraction; carried out the bioinformatics analyses and generated the manuscript figures; A.R. supervised the data analysis; T.K., Y.K.T. enrolled and followed-up the patients; T.K. drafted the manuscript, which was critically revised by A.R., U.P., V.R.M.T.B., V.J.T.A., Y.K.T. All authors read and approved the final manuscript and had final responsibility for the final content.
Corresponding author
Correspondence to Amutha Ramadas .
Ethics declarations
Competing interests.
The authors declare no competing interests.
Additional information
Publisher's note.
Springer Nature remains neutral with regard to jurisdictional claims in published maps and institutional affiliations.
Supplementary Information
Supplementary table 1., supplementary table 2., supplementary table 3., supplementary table 4., rights and permissions.
Open Access This article is licensed under a Creative Commons Attribution 4.0 International License, which permits use, sharing, adaptation, distribution and reproduction in any medium or format, as long as you give appropriate credit to the original author(s) and the source, provide a link to the Creative Commons licence, and indicate if changes were made. The images or other third party material in this article are included in the article's Creative Commons licence, unless indicated otherwise in a credit line to the material. If material is not included in the article's Creative Commons licence and your intended use is not permitted by statutory regulation or exceeds the permitted use, you will need to obtain permission directly from the copyright holder. To view a copy of this licence, visit http://creativecommons.org/licenses/by/4.0/ .
Reprints and permissions
About this article
Cite this article.
Kunasegaran, T., Balasubramaniam, V.R.M.T., Thirunavuk Arasoo, V.J. et al. Diet, lifestyle and gut microbiota composition among Malaysian women with gestational diabetes mellitus: a prospective cohort study. Sci Rep 14 , 6891 (2024). https://doi.org/10.1038/s41598-024-57627-5
Download citation
Received : 18 January 2024
Accepted : 20 March 2024
Published : 22 March 2024
DOI : https://doi.org/10.1038/s41598-024-57627-5
Share this article
Anyone you share the following link with will be able to read this content:
Sorry, a shareable link is not currently available for this article.
Provided by the Springer Nature SharedIt content-sharing initiative
By submitting a comment you agree to abide by our Terms and Community Guidelines . If you find something abusive or that does not comply with our terms or guidelines please flag it as inappropriate.
Quick links
- Explore articles by subject
- Guide to authors
- Editorial policies
Sign up for the Nature Briefing newsletter — what matters in science, free to your inbox daily.


IMAGES
COMMENTS
One of the main forms of diabetes is gestational diabetes mellitus (GDM), which is recognized as glucose intolerance, and is diagnosed initially during pregnancy. ... authors declare that there is no conflict of interest that could be perceived as prejudicing the impartiality of the research reported. Acknowledgements. This study was registered ...
Current challenges and research gaps in relation to gestational diabetes. Whilst pregnancy only represents a short time window in the life course of a woman, it represents a particularly important window of opportunity to address the risk of diabetes and other NCDs, especially among women affected by gestational diabetes mellitus.
Abstract. Gestational diabetes mellitus (GDM) is a serious pregnancy complication, in which women without previously diagnosed diabetes develop chronic hyperglycemia during gestation. In most cases, this hyperglycemia is the result of impaired glucose tolerance due to pancreatic β-cell dysfunction on a background of chronic insulin resistance.
Objective To investigate the association between gestational diabetes mellitus and adverse outcomes of pregnancy after adjustment for at least minimal confounding factors. Design Systematic review and meta-analysis. Data sources Web of Science, PubMed, Medline, and Cochrane Database of Systematic Reviews, from 1 January 1990 to 1 November 2021. Review methods Cohort studies and control arms of ...
Gestational diabetes is a complex metabolic condition thought to have a strong genetic predisposition. A large genome-wide association study of participants from Finland sheds light on the genetic ...
Gestational diabetes (GDM) is the most common pregnancy complication, occurring in 3-25% of pregnancies globally 1.GDM is associated with short- and long-term risks to both mothers and babies ...
Gestational diabetes mellitus (GDM) is the most common complication in pregnancy and has short-term and long-term effects in both mother and offspring. This Primer discusses the definitions of GDM ...
On repeat OGTT at 24 to 28 weeks' gestation, gestational diabetes was diagnosed again in 67.0% of the women in the control group. A greater percentage of women in the immediate-treatment group ...
Gestational diabetes mellitus (GDM) is a condition associated with pregnancy that engenders additional healthcare demand. A growing body of research includes empirical studies focused on pregnant women's GDM healthcare experiences. The aim of this scoping review is to map findings, highlight gaps and investigate the way research has been conducted into the healthcare experiences of women ...
1 Mater Research, The University of Queensland, South Brisbane, QLD, Australia; 2 World Diabetes Foundation, Bagsvaerd, Denmark; 3 Divakars Specialty Hospital, Bengaluru, India; 4 Mor Women's Health Care Center, Tel Aviv, Israel; Gestational diabetes mellitus (GDM) is the commonest medical complication of pregnancy. The association of GDM with immediate pregnancy complications including ...
vity and dietary changes remain the hallmark of treatment, with insulin becoming the medication of choice if further intervention is needed. This article reviews the steps clinicians can take to screen for patients with gestational diabetes, recommendations for future type 2 diabetes screening, and how to manage gestational diabetes during the course of a patient's pregnancy based on the ...
GDM was diagnosed based on a combination of fasting glucose < 7.8 mmol/L (140 mg/dL) and 2-hour postload glucose 7.8 to 11.0 mmol/L (140-199 mg/dL), respectively, using the 75-g 2-hour OGTT between 24 and 34 weeks' gestation, following screening with either positive clinical risk factors or the GCT ( 28 ).
Gestational diabetes mellitus (GDM) is one of the most frequent medical complications of pregnancy, affecting 8% of births.1 GDM increases the risk for adverse pregnancy outcomes, including fetal macrosomia, cesarean delivery, and preeclampsia, along with imparting a lifetime risk for adverse metabolic outcomes in the mother and child.1 Current guidelines recommend universal screening for GDM ...
Gestational diabetes mellitus (GDM) is one of the most common medical complications in pregnancy and affects an estimated 14% of pregnancies, or one in every seven births globally ().Women with GDM and their offspring are at increased risk of both short- and longer-term complications, including, for mothers, later development of type 2 diabetes, and for offspring, increased lifelong risks of ...
Gestational diabetes mellitus (GDM) - a transitory form of diabetes induced by pregnancy - has potentially important short and long-term health consequences for both the mother and her baby. There is no globally agreed definition of GDM, but definition changes have increased the incidence in some countries in recent years, with some research suggesting minimal clinical improvement in outcomes.
Gestational diabetes (GDM) is the most common metabolic complication of pregnancy with an increasing prevalence consistent with the concomitant global increase in obesity and diabetes 1.
The definition of gestational diabetes mellitus (GDM) is any degree of glucose intolerance with onset or first recognition during pregnancy. GDM can classify as A1GDM and A2GDM. Gestational diabetes managed without medication and responsive to nutritional therapy is diet-controlled gestational diabe ….
Background: Gestational diabetes mellitus (GDM) is a serious health concern that affects pregnant women worldwide and can lead to adverse pregnancy outcomes. Early detection of high-risk individuals and the implementation of appropriate treatment can enhance these outcomes. Methods: We conducted a study on a cohort of 3467 pregnant women during their pregnancy, with a total of 5649 clinical ...
Gestational Diabetes Research. To mitigate the transgenerational risk of diabetes, research is needed to advance the prevention of gestational diabetes and to properly diagnose and treat it when it occurs. American Diabetes Association (ADA) research supports research projects that address these critical topics.
Gestational diabetes - a type of diabetes where blood glucose levels are higher than normal during pregnancy - is a common condition that is estimated to affect between 2% and 10% of pregnancies. ... Metformin as a potential first-line treatment for gestational diabetes. Recently published research, ...
Gestational diabetes mellitus (GDM) is a state of hyperglycemia (fasting plasma glucose ≥ 5.1 mmol/L, 1 h ≥ 10 mmol/L, 2 h ≥ 8.5 mmol/L during a 75 g oral glucose tolerance test according to IADPSG/WHO criteria) that is first diagnosed during pregnancy [ 1 ]. GDM is one of the most common medical complications of pregnancy, and its ...
Screening and treatment for early gestational diabetes should begin before 20 weeks' gestation for optimal pregnancy outcomes, say authors of a study finding a greater risk for neonatal complications.
Gestational diabetes is a complication arising in pregnancy that can lead to serious and lifelong health concerns for the mother and child. Currently all pregnant women undergo screening for gestational diabetes, a time-consuming and demanding procedure. However, diagnosis often comes late in pregnancy, leaving limited time for intervention.
Gestational diabetes mellitus (GDM), a state of hyperglycemia due to insufficient insulin secretion and/or insulin resistance that occurs during pregnancy, is the most common metabolic disorder of ...
Gestational diabetes mellitus (GDM) is the most common metabolic complication of pregnancy, affecting up to 4-8% of pregnancies. The incidence of GDM in twin pregnancies is higher compared to singleton pregnancies [].The pathogenesis of GDM remains largely unknown, but it is associated with changes in metabolism during pregnancy such as maternal body weight gain, hyperglycemia ...
Gestational diabetes mellitus should be diagnosed at any time in pregnancy if one or more of the following criteria are met. Fasting plasma glucose. 5.1-6.9 mmol/L (92-125 mg/dL) 1-h plasma glucose following a 75 g oral glucose load. ≥ 10.0 mmol/L (180 mg/dL) 2-h plasma glucose following a 75 g oral glucose load.
Feature papers represent the most advanced research with significant potential for high impact in the field. A Feature Paper should be a substantial original Article that involves several techniques or approaches, provides an outlook for future research directions and describes possible research applications. ... S. Gestational Diabetes and the ...
6. Management of Gestational Diabetes. The approach to optimal management of a patient diagnosed with GDM requires a multidisciplinary approach. This includes teaching patient self-monitoring of blood glucose levels, dietary modifications and nutrition monitoring, lifestyle changes, and maternal weight gain management.
The UK Biobank is a large biomedical database and research resource with health records of about 500,000 adults—enrolled from 2006 until 2010—who live in the U.K and received health care ...
Gestational diabetes mellitus (GDM) is defined as glucose intolerance with the first onset or recognition during pregnancy and is associated with adverse maternal and neonatal outcomes 1,2. ...