Thank you for visiting nature.com. You are using a browser version with limited support for CSS. To obtain the best experience, we recommend you use a more up to date browser (or turn off compatibility mode in Internet Explorer). In the meantime, to ensure continued support, we are displaying the site without styles and JavaScript.
- View all journals
- My Account Login
- Explore content
- About the journal
- Publish with us
- Sign up for alerts
- Open access
- Published: 25 June 2020

Analyzing trend and forecasting of rainfall changes in India using non-parametrical and machine learning approaches
- Bushra Praveen 1 ,
- Swapan Talukdar 2 ,
- Shahfahad 3 ,
- Susanta Mahato 2 ,
- Jayanta Mondal 2 ,
- Pritee Sharma 1 ,
- Abu Reza Md. Towfiqul Islam 4 &
- Atiqur Rahman 3
Scientific Reports volume 10 , Article number: 10342 ( 2020 ) Cite this article
48k Accesses
221 Citations
19 Altmetric
Metrics details
- Climate sciences
- Natural hazards
This study analyzes and forecasts the long-term Spatio-temporal changes in rainfall using the data from 1901 to 2015 across India at meteorological divisional level. The Pettitt test was employed to detect the abrupt change point in time frame, while the Mann-Kendall (MK) test and Sen’s Innovative trend analysis were performed to analyze the rainfall trend. The Artificial Neural Network-Multilayer Perceptron (ANN-MLP) was employed to forecast the upcoming 15 years rainfall across India. We mapped the rainfall trend pattern for whole country by using the geo-statistical technique like Kriging in ArcGIS environment. Results show that the most of the meteorological divisions exhibited significant negative trend of rainfall in annual and seasonal scales, except seven divisions during. Out of 17 divisions, 11 divisions recorded noteworthy rainfall declining trend for the monsoon season at 0.05% significance level, while the insignificant negative trend of rainfall was detected for the winter and pre-monsoon seasons. Furthermore, the significant negative trend (−8.5) was recorded for overall annual rainfall. Based on the findings of change detection, the most probable year of change detection was occurred primarily after 1960 for most of the meteorological stations. The increasing rainfall trend had observed during the period 1901–1950, while a significant decline rainfall was detected after 1951. The rainfall forecast for upcoming 15 years for all the meteorological divisions’ also exhibit a significant decline in the rainfall. The results derived from ECMWF ERA5 reanalysis data exhibit that increasing/decreasing precipitation convective rate, elevated low cloud cover and inadequate vertically integrated moisture divergence might have influenced on change of rainfall in India. Findings of the study have some implications in water resources management considering the limited availability of water resources and increase in the future water demand.
Similar content being viewed by others
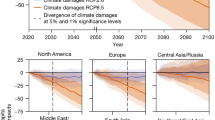
The economic commitment of climate change
Maximilian Kotz, Anders Levermann & Leonie Wenz
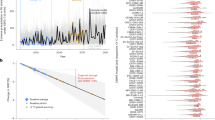
Climate damage projections beyond annual temperature
Paul Waidelich, Fulden Batibeniz, … Sonia I. Seneviratne
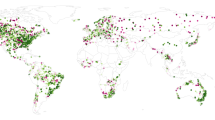
Global prediction of extreme floods in ungauged watersheds
Grey Nearing, Deborah Cohen, … Yossi Matias
Introduction
Rainfall is a key part of hydrological cycle and alteration of its pattern directly affect the water resources 1 . The changing pattern of rainfall in consequence of climate change is now concerning issues to water resource managers and hydrologists 2 . Srivastava et al . 3 and Islam et al . 4 reported that the changes of rainfall quantities and frequencies directly changing the stream flow pattern and its demand, spatiotemporal allocation of run-off, ground water reserves and soil moisture. Consequently, these changes showed the widespread consequences on the water resource, environment, terrestrial ecosystem, ocean, bio-diversity, agricultural and food security. The drought and flood like hazardous events can be occurred frequently because of the extreme changes of rainfall trend 5 . Gupta et al . 6 documented that the amount of soil moisture for crop production is totally determined by the amount of rainfall. The monsoon rainfall plays a vital role for agriculture in India. 68% of cultivated land to the total cultivated land of India is occupying by the rain fed agriculture which supports 60% of livestock population and 40% of human population 7 . Hence, the research on the climate change or most specifically on the changes of rainfall occurrences and its allocation are the most significant way for sustainable water resource management. Therefore, the sustainable development of agriculture in India requires the noteworthy research on the identification and quantification of climate change 7 . Most importantly, a complete understanding of the precipitation pattern in the changing environment will help in better decision making and improve the adapting-capacity of the communities to sustain the extreme weather events.
Nowadays, the water resources have been considered as the key concern for any kinds of development program and planning which includes effective water resource management, food production sector and flood control. The uneven allocation of water supply throughout the country, because of the natural pattern of rainfall occurrence which varies significantly in space and time, is the main hindrance for the effective water resource management in India 8 . The climate change further accelerates this rainfall variability 7 . Consequently, many regions of the country receive huge amounts of rainfall during the monsoon, while others receive very less amount of rainfall and frequently experience the worst reality of water scarcity. Furthermore, the worst consequence of climate change, especially rainfall change, has been experiencing by the agricultural sector of this country. Because the rainfall has not been taking place when it is expected and vice versa 9 . Even, the high winds and hails have been frequently occurred. Consequently, enormous losses to crops have been taken place and the farmers who totally dependent on the agriculture have become devastated 7 . The freakish weather is causing havoc on the farmers community, as a results, the farmers have gone extreme to the committing of suicide. During the period 1995–2014, 300000 farmers have committed suicide in India 10 . Hence, it is noteworthy to evaluate whether there is any trend in rainfall and any pattern in variability 11 .
Therefore, to explore the variability and changes in pattern and existence of trend in rainfall over different spatial horizons have been the key aspects in the study of hydrology, climatology and meteorology worldwide 12 , 13 , 14 , 15 , 16 , 17 . In most studies, researchers have used parametric and non-parametric methods 18 like regression test 19 , 20 , Mann-Kendall test 21 , 22 , Kendall rank correlation test 23 , Sen’s slope estimation 24 , 25 and Spearman rank correlation test 26 . In the present study, the non-parametric test like Mann-Kendall test was applied to detect the trend in rainfall as it is one of the most often applied global methods for trend detection in hydrology, climatology and meteorology 27 , 28 , 29 , 30 , 31 . One of the major reason to use non-parametric tests in the present study is these can be used on independent time series data and are also not much sensitive to outliers 32 . However, the change detection analysis in the climatologic and hydrological data series is the important aspect for the trend analysis throughout the world 33 , 34 .The change detection methods include the Standard Normal Homogeneity Test (SNHT) 35 , Buishand range test 36 and the Pettitt’s test 37 . The trend analysis was carried out before the change point analysis in many researches 32 . This approach can lead to misleading results as the information obtained from change point detection analysis has not been considered for the trend analysis 38 . Hence, Li et al . 36 highly recommended performing the change detection analysis first followed by the trend analysis. The results obtained from this approach are more reasonable and reliable.
In India, several attempts have already been done in the past to detect rainfall trends in regional and national levels 39 , 40 , 41 , 42 , 43 , 44 , 45 , 46 . The research on trend analysis has believed to be a useful tool as it provides the significant information about the possibility of the future changes 4 , 47 , 48 . However, multiple studies have conducted trend analysis using MK test and reported that India as a whole has not recorded significant trend of increase or decrease in annual average rainfall 7 , 46 , 49 . Many researchers identified significant trend in long-term rainfall in India 2 , 44 , 50 , 51 , 52 , 53 , while the significant long-term trend has not been detected in monsoon rainfall on a national scale 7 . Patra et al . 54 studied the trend analysis in monthly, seasonal and annual rainfall over Orissa and reported that long-term insignificant negative trend was detected in annual and monsoonal rainfall, while the positive trend was detected in post- monsoon season. But to the best of authors knowledge, most of the researchers applied non-parametric tests to detect and followed by trend analysis. Therefore, to get reliable and reasonable results of trend, we have obtained both approaches like trend analysis before change point and first change point analysis followed by trend detection.
Sen 55 developed the innovative trend analysis that has been utilized for detecting trend in meteorological, hydrological and environmental variables 56 , 57 , 58 , 59 , 60 . The innovative trend analysis has the worldwide applicability over the non-parametric approach 61 like Mann-Kendal test, spearman’s correlation test and Sen’s slope estimation because these non-parametric test are very much sensitive to the distribution assumptions, serial correlation, size of the time series data and seasonal cycle 62 . Von Storch 63 stated that if the data series have statistically significant serial correlation, the MK test can generate no noteworthy trend existence in the data series. Sen 55 stated that the effective, efficient and optimum water resource management needs to identify the trend not only monotonically over time, but also needs to identify trends separately which have the high, medium and low value which can be possible to identify by the innovative trend. In addition, the innovative trend is not sensitive to serial correlation, non-linearity, and size of the time series data and gives the robust and powerful result with less error. Therefore, researchers prefer the innovative trend analysis for detecting trend in hydrological, meteorological studies 18 , 44 , 55 , 64 , 65 . Therefore, in the present study, apart from Mann-Kendall test, the innovative trend was also utilized for detecting trend. In India, very few studies were conducted to detect trend using innovative trend analysis. But to the best of authors’ knowledge, the application of innovative trend to detect seasonal rainfall for whole India would be the first application.
As we have considered detecting change point using MK test before change point analysis, MK test after change point analysis and innovative trend analysis, the planners of water resource management, environmentalist, and hydrologists can have the chance to compare the result of all these techniques and get high precision trend information in annual and seasonal rainfall. Therefore, in this line of thinking, we can conclude that the study has the quite novelty.
The trend analysis facilitates to understand the present and past climatic changes, but the future forecasting is more useful for the planners to execute the proper planning taking into account future changes in climatic variables 66 . To project future information of the climatic variables, in addition to the complicated climate model which works on global scale, regional forecasting could be carried using statistical techniques and machine learning soft computing techniques 67 , 68 . To execute the physical models, large numbers of database, high configured system, advanced technology, technical expert are required. The physical models are both time and money consuming, although they provide highly reliable results. The statistical techniques like auto regressive (AR), moving average (MA), auto regressive moving average (ARMA) and auto regressive integrated moving average (ARIMA) have several limitations such as AR model regresses past values, while MA model utilizes past error as the explanatory variables and ARMA model can perform for stationary time series data 67 . However, recently, the application of artificial intelligence (AI) models like machine learning techniques have gained attention 69 . Unlike physical models, the AI models perform very well as it does not require huge information, but can handle huge and complicated data sets if provided 70 . It can perform in non-stationary time series data 71 . Darji et al . 67 conducted review survey regarding the rainfall forecasting using neural networks and reported that back propagation based neural network performs well to forecast rainfall. In the present study, we utilized multilayer perceptron (MLP) algorithm based neural network for predicting the rainfall. The MLP based ANN works using back propagation algorithm. However, the application of MLP based artificial neural network (ANN) for predicting and forecasting of multifaceted hydrological and climatic phenomena and has gained popularity across the globe as it provides reliable results 72 , 73 , 74 , 75 , 76 , 77 , 78 , 79 , 80 , 81 . The novelty of the present study is that the application of MLP-ANN is not concentrated on the one or more states or overall India rather the present study considers predicting and forecasting rainfall for thirty-four meteorological sub-divisions of India. Therefore, the study will be highly beneficial as it worked on the whole India in micro level.
Therefore, based on the previous literatures, research gaps, the objectives of the present study are: (1) to analyze the variability and trend analysis in the overall annual and seasonal rainfall for thirty-four meteorological sub-divisions; (2) to detect the change point for all sub-divisions; (3) to explore the change point wise rainfall variability and trend analysis; (4) to detect trend using innovative trend analysis; (5) to predict and forecast rainfall up to 2030 for all sub-divisions; (6) to investigate the causes of the changes of rainfall pattern.
Material and Methods
Study area and data source.
The geographical location of India is between 8.4° and 37.6°N latitude and 68.7° and 97.25°E longitude. The whole India can be categorized into four homogenous climatic regions such as North-West India (NW India), North-East India (NE India), Central India and Peninsular India on the basis of the distribution and occurrence pattern of rainfall and temperature 46 . Although the whole country experiences almost all types of climate due to its physiographic location 59 . However, India observes the four seasons, like summer season (January-February), winter (March-May), monsoon (June-September) and post-monsoon (October-December) 82 . The India receives 117 cm rainfall, out of which 80% of rainfall is observed during monsoon month.
The whole Indian region has been sub-divided into thirty-four meteorological subdivisions by the Indian Meteorological Department (IMD) where the climatic parameters like rainfall, temperature, wind speed, humidity have been recorded since 1901 (Fig. 1a ). In this study, we obtained rainfall data for 115 years (1901–2015) from thirty-four meteorological sub-divisions. The collected data was continuous in nature with no missing values.
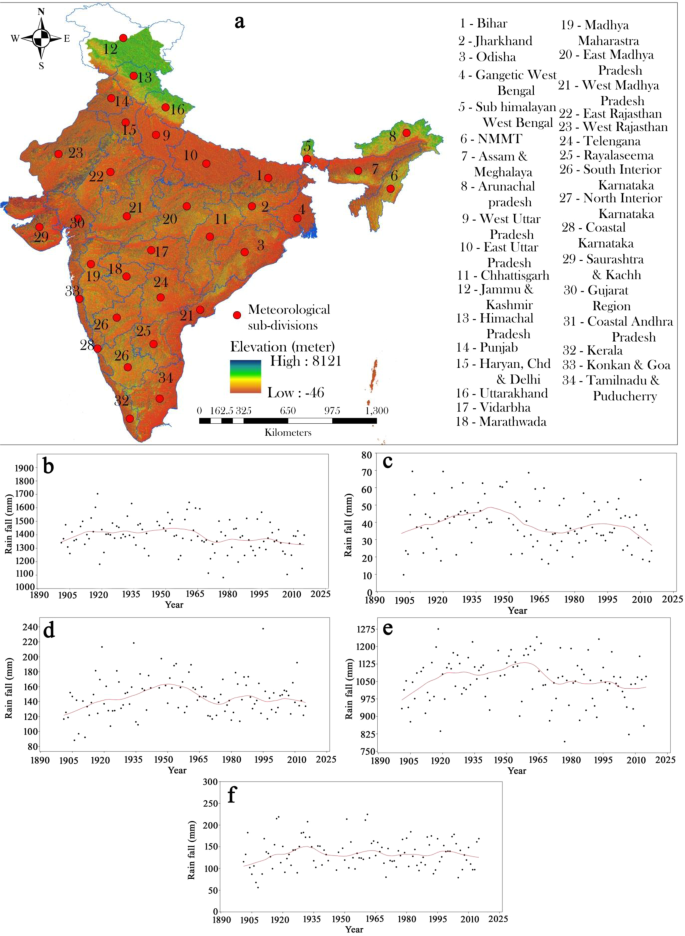
( a ) Geographical location of the study area; LOWESS curve on annual and seasonal rainfall where figure shows ( b ) annual rainfall, ( c ) winter ( d ) summer ( e ) monsoon and ( f ) post monsoon rainfall.
Method for trend analysis
In this paper we used the Mann-Kendal test 18 , a non-parametric statistical test based on rank system, to detect the trend in long-term rainfall data series. The MK test is mainly used for detecting trend in hydro-climatic data series as the lower sensitivity to any sudden change 83 , 84 . To perform this test, it is essential to evaluate the presence of serial correlation within the data series 85 . A positive serial correlation can support the expected number of bogus positive products in the MK test 86 . For this reason, the serial correlation must be excluded prior to applying the MK test. To eliminate the serial correlation, the trend free pre-whitening (TFPW) technique proposed by Yue and Wang 85 was used.
The MK test was calculated using Eq. 1 :
In a time series, K i , i = 1, 2, 3, ……….n , the value of S is supposed to be similar as the normal distribution with a mean 0 and while the discrepancy of statistics S has been computed using Eq. 2 :
The Z MK value is used to find out that the time series information is demonstrating a significant trend or not. The Z MK value is computed using Eq. 3 :
The positive and negative values of Z in a normalized test statistic reflect the increasing and decreasing trend, respectively, while the Z having 0 values reflects a normal distributed data series.
Method for change point detection
In the present study, we utilized Pettitt test proposed by Pettitt 37 and Standard Normal Homogeneity test (SNHT) developed by Alexandersson and Moberg 35 to explore the presence of the abruptly shifting change points in the time series annual and seasonal rainfall dataset for all meteorological sub-divisions.
Pettitt Test
The Pettitt test is a distribution-free rank based test, used to discover noteworthy changes in the mean of the time series. It is more helpful when the hypothesis testing about location of a change point is not necessary. This test has been used extensively to identify the changes observed in climatic and hydrological data series 87 , 88 . When length of a time series is represented by t and the shift take place at m years, the consequential test statistics are expressed as given in Eq. ( 4 ).The statistic is similar to the Mann- Whitney statistic, which characterized by two samples, such as k1, k2…, km and km+1, k2…, kn:
where \(\mathrm{sgn}\) in Eq. 4 is defined by Eq. 5 :
The test statistic Ut,m is calculated from all haphazard variables from 1 to n . The majority of distinctive change points are recognized at the point where the magnitude of the test statistic | Ut,m | is highest (Eq. 6 ).
The probability of shifting year is estimated when | Ut,m | is maximum following Eq. 7 :
If the p- value is less than the significance level α, the null hypothesis is considered to be rejected.
Standard Normal Homogeneity Test (SNHT)
The standard normal homogeneity test is also known as the Alexanderson test. This test is applied to detect sudden shift or presence of change point in time series of climatic and hydrologic datasets. The change point has been detected following Eq. 8 :
The change point refers to the point, when T s attains maximum value in the data series. The T m is derived using Eq. 9 :
where \(\bar{m}\) represents the mean and s represents the standard deviation of the sample data.
Buishand Rang Test
The Buishand range test is also called as Cumulative Deviation test, which is calculated based on the adjusted biased sums or cumulative deviation from mean. The change point using this test is detected following Eqs. 11 and 12 :
The \(S/\sqrt{n}\) is then estimated using the critical values proposed by Buishand 38 .
Methods for innovative trend analysis
The innovative trend analysis, proposed by Şen 55 , was applied to detect the trend in long-term time series rainfall data of India. The innovative trend analysis has greatest advantages over the MK test and other parametric and non-parametric statistical tests, which is that it does not need any assumptions like non-linearity, serial correlation and sample numbers. However, the graph of this test is being plotted on a Cartesian coordinate system depending on a sub-section time series. As per the method’s requirement, the time series rainfall data has been sub-divided in two time series data sets: 1901–1957 and 1958–2015. Both segments were fixed in an ascending order. Typically the first sub-series (x i ) was represented on x axis, while the other sub-series (y i ) was represented on y axis. The data is plotted on the 1: 1 line. If the data is plotted along the 1:1 line, it indicates simply no trend within the time series. If the data is being plotted above the 1:1 line, it will state that the moment series shows an increasing trend, and if the data falls under the 1:1 line, it will indicate the negative trend 56 , 58 . If the scatter plot is more close to the 1: 1 path, the trend alter slope of that time period series will be weaker, along with the farther far from the 1:1 tier it is, the particular stronger the excitement change incline in the time series 55 . Often the straight-line trend slope displayed by the ITA method can be expressed as by the Eq. 13 56 :
Where s is the indicator of trend having the positive values that represent the increasing trend, while the negative values represent the decreasing trend. The \(\bar{{\rm{y}}}\) and \(\bar{{\rm{x}}}\) are the arithmetic average of the first sub-series (xi) and second sub-series (yi) respectively. Furthermore, n is represented by the number of collected data products. The indicator is then multiplied by 10 for comparing with MK test 61 .
Method for analyzing rainfall changes
To calculate atmospheric oscillations on rainfall trend variation, winter and summer precipitations and moisture divergence during 1979–2015 on 1.25° × 1.25° grids were obtained from the European Centre for Medium Range Weather Forecasts (ECMWF), ERA-5 ( http://apps.ecmwf.int/ datasets/data/interim-full-daily). The ERA5-Interim is the most recent ocean-atmospheric changes reanalysis datasets available since 1979 forwards. In addition, low cloud cover dataset was also derived from the ECMWF ERA5 data to assess the effect of cloud cover on rainfall variation 89 . We have quantified the influence of atmospheric circulation changes on the trend patterns in rainfall. At first, we have detected a recent significant change point of annual mean rainfall based on Pettit test for the period 1979–2015 in India and observed that the mean rainfall has a change point after 2000. Then, the change in circulation of the two periods before and afterword the changes are quantified by subtracting 1979–2000 from 2001 to 2015using the ECMWF ERA5 reanalysis data. The GrAdS software ( http://cola.gmu.edu/grads/ ) was used to prepare the spatial maps.
Method for future forecasting
Several probabilistic and deterministic methods like ARMA, ARIMA, SARIMA are usually employed to predict the hydrological and climatic datasets. These techniques have many drawbacks like serial correlation, non-linearity, and biasness to predict the non-linear hydro-climatic data sets. Therefore, the newly developed artificial intelligence (AI) models can able to overcome this drawback. Hence, the application of AI models is now widely popular to solve the environmental problems. However, in the present study, we employed artificial neural network, a popular AI model, to predict and forecast the rainfall of thirty-four meteorological sub-divisions. The ANN is a non-linear black-box AI model. This model works like parallel-distributed information processing system which reflecting biological structure of brain as it comprised of simple neurons and links that process information to establish an association between the inputs and outputs. Likewise the function of brain, the ANN model is working using feed-forward multilayer perceptron algorithm. This structure is consisting of three layer, such as an input layer, one or more hidden layer and an output layer. We selected the number of neurons in the hidden layer by trial and error procedure. The ANN learning is based on the structure and functions of biological neural networks i.e. enclosing adjustment to the synaptic links that present in the core of the neurons. The multi-layer perception (MLP) is one of the most extensively employed neural network typologies. The MLP with two hidden layers is the most used classifier for the stationary pattern classification. MLPs are generally functioned using the backpropagation algorithm. The back-propagation principle flourishes the errors from the network and permits adjustment of the unseen units. Two crucial characteristics of multilayer perceptron are:
(1) The non-linear processing elements (PEs) having non-linearity that should be smooth, so that the logistic functions and hyperbolic tangent can be frequently employed; and
(2) Their enormous interconnectivity (any constituent of a given layer feeds all the constituents of the next layer).
In the present study, we divided the whole rainfall datasets into training and testing datasets. Then we normalized the data and applied ANN model for predicting the annual rainfall. We applied ANN model again and again on the same rainfall datasets by changing the model’s parameters like seed, momentum, learning rate until the best ANN model achieved for prediction rainfall. In addition, we evaluated the performance of ANN for predicting using Root Mean Square Error (RMSE) techniques. When we achieved the best ANN model, we fixed the model parameters and applied on the rainfall data to forecast rainfall up to 2030. However, the rainfall prediction and forecasting were performed in Weka software (version 3.9) ( https://weka.informer.com/3.9/ ) and the mappings were done in Arc GIS (version 10.3) software.
Method for change rate calculation in rainfall time series data
The simple statistical method, percentage change is applied to calculate the change rate of the annual and seasonal rainfall for pre change and post change point. This method is very simple but the function of this method is much effective. It is calculated using Eq. 14 .
Spatial mapping using Kriging interpolation method
In this study, the ordinary kriging method was employed to interpolate the statistical data and prepare the spatial rainfall map. The ordinary kriging method works on the data having statistical properties or spatial autocorrelation and this method employed the semi-variogram model to represent the spatial linkage (autocorrelation). The semi-variogram model evaluates the power of spatial association as a function of distance between the data. Kriging is used to estimate the values Z*( x 0 ) at the point x 0 expressed 90 in Eq. 15 and the estimation of error variance \({\sigma }_{k}^{2}({x}_{0})\) , expressed in Eq. 16 .
Where λ i refers to weights; μ refers to LaGrange constant; and ( x 0 − x i ) represents the semi-variogram value equivalent to the distance between x 0 and x i 91 , 92 . Nielsen and Wendroth 93 suggested that a semi-variogram is comprised of the regionalized variable theory and intrinsic hypotheses and it is expressed as follows:
Where, γ ( h ) represents semi-variance, h represent lag distance, Z refers to the rainfall-related parameters, N(h) is the number of couples of locations divided by the lag distance h, \(Z({x}_{i})\) , and \(Z({x}_{i}+h)\) refers to the values of Z at the positions x i and x i + h 94 . The empirical model of semi-variogram generated from collected data which was fitted to the theoretical semi-variogram. It was utilized to create geo-statistical properties which further include nugget structured and the sill variance as well as the distance parameter. In addition, the nugget-sill ratio has been calculated in order to characterize the spatial dependency of the statistical values. A nugget-sill ratio of 75% reflects a weak spatial dependency; or else the dependency is considered moderate.
Furthermore, all mappings in the present work were performed using kriging interpolation method in Arc GIS (version 10.3) software.
Results and Discussion
Descriptive analysis of annual rainfall.
We calculated the descriptive statistics of the annual rainfall since 1901 to 2015 for thirty-four meteorological sub-divisions of India. Results show that the South Indian meteorological divisions i.e. Kerala, Tamil Nadu, and Konkan & Goa have observed the highest average rainfall (3396.64 mm. 2930 mm. and 2974 mm. respectively). While, the minimum average rainfall has been recorded in the sub-divisions of West India meteorological divisions i.e. West Rajasthan (288.74 mm.), Saurashtra and Kutch (494.27 mm.), Haryana (535.47 mm.), Delhi and Chandigarh (596.16 mm.). The standard deviation of rainfall for whole India varies from 1242.04 to 108.99 mm. The highest variation (standard deviation) in rainfall was observed in Arunachal Pradesh meteorological sub-division, followed by Coastal Karnataka (480.98 mm.), Konkan & Goa (478. 49 mm.), while the minimum variation was recorded in Western Rajasthan (108. 99 mm., followed by North Interior Karnataka (135.33 mm), Haryana Delhi and Chandigarh (142. 64 mm). The skewness of the rainfall ranges from −0.81 to 1.05 for all sub-divisions of India. The negative skewness was found in Tamil Nadu (−0.81), Bihar (−0.39), Konkan & Goa (−0.21), Jharkhand (−0.15), West Uttar Pradesh (−0.09), East Madhya Pradesh (−0.02) and Madhya Maharashtra (−0.001). While rest of the meteorological sub-divisions were observed positive skewness.
The arithmetic mean is not significant (robust) to local variations 95 . Therefore, LOWESS regression curve was applied on the seasonal rainfall to minimize the local variation. A cluster of researchers used this statistical method and achieved satisfactory findings over the arithmetic mean 96 , 97 . The findings of LOWESS curve indicate an increasing pattern of annual rainfall upto1965, while a decreasing pattern of rainfall was found after the year 1970. (Fig. 1b ). The findings of LOWESS curve for the winter season (Fig. 1c ) showed that the increasing rainfall pattern was observed for the periods of 1935–1955 and 1980–1998. A sudden decrease in the trend was observed after 1955 and 1998. In the case of summer and monsoon season (Fig. 1d,e ), the curve indicates that the negative trend was observed after the 1960. The identical result was found in the work of 98 . Whilst, in the case of post-monsoon (Fig. 1f ), the LOWESS regression curve indicates the positive trend was found during 1925–1935 and 1955–1965. The negative trend for post monsoon was observed after 1995.
Long Term Pattern and variation of average annual and seasonal rainfall
We used the coefficient of variation techniques to explore the rainfall variation for all meteorological sub-divisions. The Fig. 2a–e showed the spatial mapping of variations of average annual and seasonal rainfall over India using ordinary kriging interpolation method which is geo-statistical approach. The findings of spatial mapping of rainfall variation showed that the meteorological sub-divisions of Western India were recorded highest rainfall fluctuations. The minimum rainfall fluctuation was registered in Assam and Meghalaya (11.35%) metrological divisions, followed by Sub-Himalayan meteorological divisions like West Bengal (12.25%), Orissa (12.92%) and extreme South Indian divisions like Kerala (14.16%), Coastal Karnataka (14.42%) and North Interior Karnataka (14.67%). This result indicates that these states have been experiencing very less inconsistent rainfall trend for 115 years. While, the highest rainfall variation was found in Saurashtra and Kutch (41.14%), followed by Western Rajasthan (37.75%), Arunachal Pradesh (33.23%) and Gujarat region (30.46%) indicating the irregular occurrences of rainfall throughout the year.
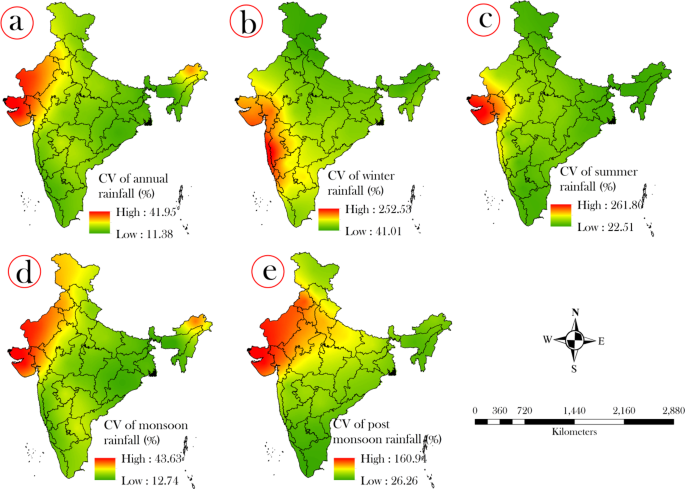
Meteorological subdivision wise spatial variations using the coefficient of variation (CV) in annual and seasonal rainfall where figure ( a ) shows annual, ( b ) winter, ( c ) summer, ( d ) monsoon, and ( e ) post monsoon rainfall pattern.
The analysis of distribution and fluctuation of rainfall over Indian meteorological divisions’ show that the occurrence of winter season rainfall was comparatively less than the other seasons during 1901–2015. The maximum variation of rainfall was reported in Konkan & Goa (253.37%), followed by Coastal Karnataka (187.70%), Gujarat region (185.12%), Saurashtra & Kutch (174.88%) and Madhya Maharashtra (162.06%) indicating the unstable incidence of rainfall in these meteorological units. More consistent rainfall with low variations was observed in Himachal Pradesh (41.89%), followed by Arunachal Pradesh (43.15%), Uttarakhand (48.69%) and Assam & Meghalaya (52.21%) meteorological units (Fig. 2c ). Result show that, the rainfall in summer season had not recorded a significant variation, whilst the maximum variations of rainfall was observed in the meteorological divisions of Western India. The lowest rainfall variation was found in the meteorological divisions of Northeastern India and extreme South India.
The study of diverge distribution of rainfall over India for monsoon season indicates that the maximum rainfall was registered in Saurashtra & Kutch (42.67% and 157.64%), followed by West Rajasthan (39.93% and 145.35%), Arunachal Pradesh. Whereas, the highest rain fall variation in post-monsoon season was found in Punjab (36.18% and 138.65%), followed by Gujarat region (31.38% and 127.66%) indicating unstable rainfall incidences (Fig. 2d,e ). The lowest rainfall fluctuation of monsoon season was recorded in the meteorological divisions of Assam & Meghalaya (12.69%), followed by Orissa (13.34%), Sub Himalayan West Bengal (13.94%) and Coastal Karnataka (15.29%). While, in the post-monsoon season, the lowest rainfall fluctuation was observed in Kerala (26.21%), followed by Tamil Nadu (29.46%) and South Interior Karnataka (36.44%).
Meteorological sub-division wise trends of Annual and Seasonal Rainfall
We calculated the annual and seasonal rainfall trend for thirty-four meteorological sub-divisions using non-parametric Mann-Kendall test (Table 1 ). The Table 1 shows the five shades of brown color based on the intensity of z value of Mann-Kendall test at 0.05 significance level which indicates that darker the shade of brown color, higher the negative z value and vice-versa. Results show that five sub divisions for annual rainfall were found in very dark shade zones (Nagaland Mizoram Manipur & Tripura, East Madhya Pradesh, Jharkhand, East Uttar Pradesh, Chhattisgarh and Kerala) which suggests that these sub-divisions were experienced highly negative trend of rainfall (more than −2 of z value). The z value of MK test ranges from 0 to −2 which could be found in eight meteorological sub-divisions, i.e. Bihar, Orissa, Assam & Meghalaya, West Uttar Pradesh, Uttarakhand, Himachal Pradesh, Vidarbha and East Rajasthan (dark brown color in Table 1 ). The z value having the positive trend varies from 0 to 2 which were observed in the eleven meteorological sub-divisions that implies the increasing nature of rainfall over these divisions, while the six meteorological sub-divisions recorded highly positive trend of more than 2 of z value (very light shade of brown color in Table 1 ), which indicates the significant increase of rainfall over time in these meteorological units.
The two meteorological division (Madhya Maharashtra and North Interior Karnataka) for summer season and seven meteorological divisions (Assam & Meghalaya, Orissa, Bihar, Punjab, East Rajasthan, Chhattisgarh and Kerala) for monsoon season were recorded highly negative trend in rainfall having >2 of z value (very dark shade rows in Table 1 ). On the other hand, no meteorological divisions for winter and post-monsoon season were recorded as highly negative trend (more than >2 of z value) in rainfall, while most of the sub-divisions were detected as negative trend having the z value 0 f 0 to −2 (dark shade rows in Table 1 ).
The Change Point Detection analysis for annual and seasonal rainfall
The above-mentioned analysis (Table 1 ) explained that few meteorological sub-divisions were detected as significant negative trend having the z value of >−2. However, several researchers claimed that the actual trend could not be detected if we apply the MK test on overall hydro-climatic datasets. Therefore, they highly recommended to apply the change detection techniques before the application of MK test. Hence, we utilized change detection methods like Pettitt test, SNHT test and Buishand range test for detecting the abrupt change point in the rainfall datasets in thirty-four meteorological sub-divisions (Supplementary Table 1 ). The Supplementary Table 1 shows that the annual and seasonal rainfall of all meteorological sub-divisions had the abrupt change point which were detected by mentioned three change detection techniques. Furthermore, we selected the change point for annual and seasonal rainfall in each sub-divisions based on the performances (p value) of these tests (Supplementary Table 1 ). Therefore, these abrupt change points suggest that the rainfall datasets had no monotonous trend. The selected change point for seven meteorological sub-divisions (East Uttar Pradesh, West Uttar Pradesh, Punjab, and Gujarat region, Saurastra & Kutch, Coastal Andhra Pradesh and Tamil Nadu) were after 1990s. The nineteen meteorological sub-divisions had the abrupt change point during the period of 1950–1980. The abrupt change point year for the rest of the meteorological divisions were detected before 1940.
Change point wise annual and seasonal variation analysis
We computed the annual and seasonal rainfall variation in pre and post change point wise for all meteorological sub-divisions to explore the dynamics of the intensity of annual and seasonal rainfall variations after the change point. Therefore, we can consider this change point wise rainfall variation analysis as a validating method for the relevancy of uses of the change point methods and further research. Hence, to prove this statement, we computed and prepare the spatial map of annual and seasonal rainfall variation for pre change point (Fig. 3a–e ) and post change point (Fig. 3f–j ) using coefficient of variation method. Results show that highest fluctuation (25% to 239.02%) in annual and seasonal rainfall was observed in the sub-divisions of Western India and North-Western India (3a-e). Whereas, the minimum fluctuation in annual and seasonal rainfall having the CV of 0.36% to 7.76% was observed in the sub-divisions of North Eastern India, Eastern India and Northern India indicating the consistent rainfall occurrence in these region.
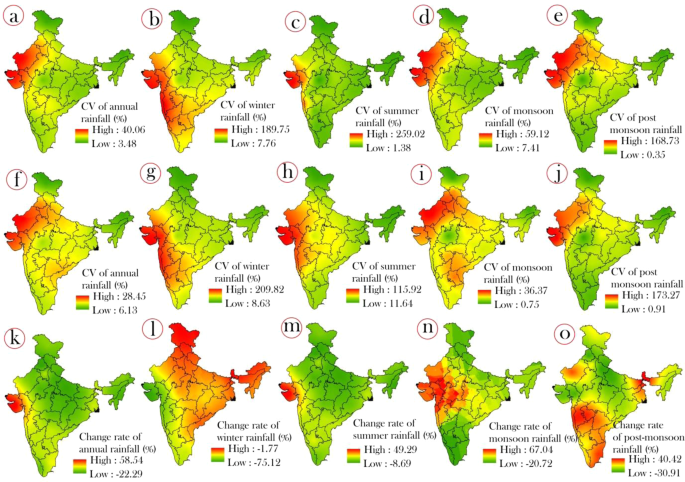
Spatial variation of rainfall measured using the coefficient of variation for pre-change point where figure ( a ) shows annual rainfall, ( b ) winter, ( c ) summer, ( d ) monsoon, and ( e ) post monsoon; post-change point where figure ( f ) shows annual rainfall, ( g ) winter ( h ) summer ( i ) monsoon and ( j ) post monsoon; spatial changes in the rate of rainfall where figure ( k ) shows annual rainfall, ( l ) winter ( m ) summer ( n ) monsoon and ( o ) post monsoon.
The findings of annual and seasonal rainfall analysis in post change point phase show that the large areas of the country like the sub-divisions of Western India, Central India, and South Western India observed high fluctuation having the CV of 22% to 209.82%. In case of monsoon and post monsoon season, the sub-divisions of North Western were experienced by high variation of rainfall suggesting the high tendency of inconsistently rainfall occurrences in these regions. While the lowest rainfall variation having the CV of 0.75% to 11.64% was recorded in sub-divisions of North-Eastern India, North India, East India of winter and summer rainfall and Central India of Post monsoon rainfall. The intensity of minimum rainfall variation range was increased in post change point phase which suggests that inconsistency rainfall events were observed. However, in the post change point phase, the area coverage of lower variation of rainfall incidences were reduced significantly that implies the climate change and validation of the application of change point detection methods.
Rainfall change rate analysis
In the present study, we computed the rainfall change rate for annual and seasonal rainfall in all meteorological sub-divisions based on the calculation between the rainfall data of pre and post change point phase. The Fig. 3k–o shows the spatial mapping of rainfall change rate for annual and seasonal rainfall. Results show that sub-divisions of Western India were observed highest change rate (47% to 58.54%) in annual rainfall, while the sub-divisions of whole country except West India were registered the highest change rate (−0.5% to −1.77%) in winter rainfall. In case of summer and monsoonal rainfall, the sub-divisions of western were observed highest negative change rate (34.22% to 67.04), while the South India and North East India were recorded highest negative change rate in post monsoon rainfall. Furthermore, the North India and Central India were recorded minimum change rate, whereas, the Western India of winter and post-monsoon rainfall. This analysis signifies that the amount of rainfall occurrences was decreased significantly after change point.
Change point wise seasonal rainfall trend analysis
We applied MK test on the datasets of pre and post change point of seasonal rainfall in each of the meteorological sub-divisions as per the recommendation of many scientists. The Table 2 reported the seasonal rainfall trend for pre change point in all sub-divisions Results show that eight meteorological sub-divisions in monsoon, six sub-divisions in post monsoon, fourteen sub-divisions in both summer and winter season were recorded negative trend having the z value of 0 to −2 (dark shade of brown color rows in Table 2 ). While, three sub-divisions in summer and four sub-divisions in winter seasons were recorded highly negative trend having z value of > −2 (very dark shade of brown color rows in Table 2 ). Rests of the meteorological sub-divisions were detected as positive trend (lighter shade of brown color in Table 2 ) except one division (Sub Himalayan West Bengal & Sikkim) which detected has no trend. From the analysis, it can be stated that non-monsoonal seasons were recorded as declining trend.
The Table 3 showed the trend analysis using MK test for post-change point seasonal rainfall. Seventeen meteorological sub-divisions in monsoon, sixteen sub-divisions in post monsoon, seventeen sub-divisions in summer and twenty one sub-divisions in winter seasons were observed the negative trends having the z value of 0 to −2 indicating that huge amount area was recorded declining rainfall than the pre-change point phase (dark shade of brown color rows in Table 3 ). The increasing of meteorological divisions which have the negative trend from the pre change point (Table 3 ). This circumstance implies that the declining trend of rainfall was increased manifold after post change point which shows the sign of climate change. On the other hand, rest of the meteorological sub-divisions were experienced the insignificant positive trend having the z value of <0.5.
Innovative trend analysis for seasonal rainfall
Several researchers suggested that non-parametric statistical techniques like mann-kendall test has many drawbacks like the presence of serial correlation within the data sets, non-linearity and most importantly sample size which could have ability to influence the result. Therefore, Sen 55 developed innovative trend method which can overcome the mentioned drawbacks, especially the problem sample size. Sen 55 reported that innovative trend can effectively able to detect the trend on any numbers of sample size and presence of serial correlation. Hence, we used innovative trend to calculate the trend for seasonal rainfall (winter, summer, monsoon and post monsoon) in thirty-four meteorological sub-divisions (Supplementary Figure 1 – 4 ). We computed D value of innovative trend to compare the intensity of trend achieved by MK test. The spatial mapping using D values of innovative trend for seasonal rainfall was presented in Fig. 4a–d . However, the Supplementary Table 2 showed the slope value of innovative trend for seasonal rainfall in all meteorological sub-divisions. The findings indicate that the negative trend was detected in the sub-divisions of North Eastern, Central and Southern India for summer season. The results were quite identical with the findings of MK test, but the magnitudes of the trend were different which stated that the region was experienced strong negative trend (Fig. 4 ). Although the highly positive trend was detected in the sub-divisions of Rajasthan part and Jammu-Kashmir region which does not imply that the rainfall occurrences was increased, but the regions were received more or less consistent amount of rainfall throughout the time periods, while little amount of rainfall was increased in few years over these regions which is the reason for positive trend. However, in case of monsoon rainfall, the negative slope of innovative trend was detected in the sub-divisions of North Eastern part, Eastern part and some parts of the central India, whereas, rest of the sub-divisions were detected as insignificant positive slope of trend. The sub-divisions of North Eastern states, Bihar, Orissa, Jharkhand, Western Ghat and Punjab regions were experienced very strong negative slope of innovative trend, on the other hand, rest of the part were recorded insignificant positive slope of trend for post monsoon rainfall. In case of winter rainfall, the meteorological sub-divisions of Central part of India, Southern India, Western Ghat regions were observed the negative trend, while the sub-divisions of North Eastern, Western and Eastern part of India were experienced positive slope of trend. Therefore, the findings of MK test and innovative trend analysis were highly identical, however, few states where no significant trend were detected using MK test, but in the case of innovative trend, those region were come under negative trend. However, the findings from both trend detected methods clearly stated that India has been experiencing fewer downpours than the expected rainfall since last 30 years that clearly points out about the climate change. Several researches established that innovative trend can able to detect trend effectively over the others non-parametric test 18 , 99 , 100 , . Therefore, we considered innovative trend as a tool of intensity of trend measurement over the other techniques and results show that the highly negative trend was detected in most of the sub-divisions indicate about the climate change.
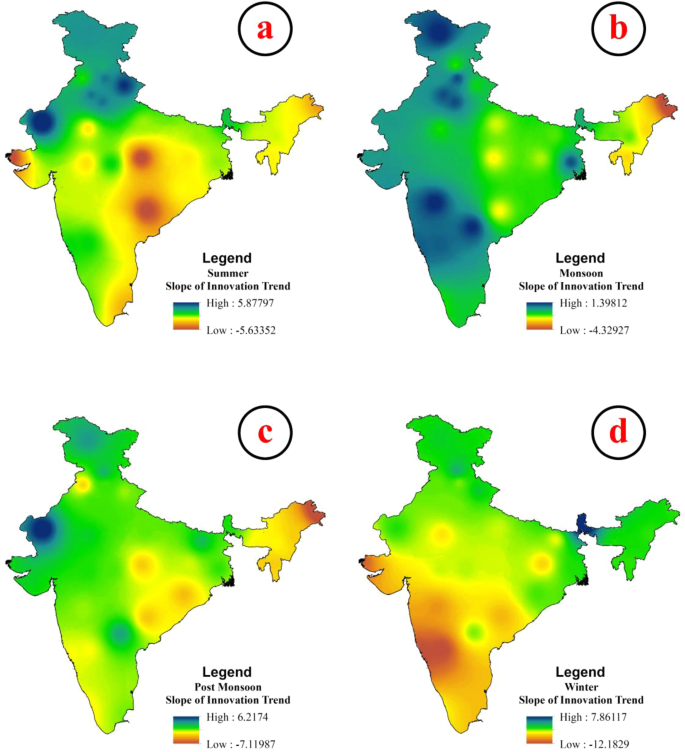
The spatial variation of slope of innovative trend for ( a ) summer, ( b ) monsoon, ( c ) post monsoon and ( d ) winter.
Micro level rainfall change rate analysis
In the present study, we attempted to analyze the change rate of annual rainfall for each and every year in thirty-four meteorological sub-divisions. We computed the change rate by calculating the departure of year wise average rainfall from the long-term average rainfall. The heat map was used to show the dynamics of year wise rainfall change rate for all sub-divisions (Fig. 5 ). This heat map was generated from R software (version 3.5.3) ( https://cran.r-project.org/bin/windows/base/old/3.5.3/ ) using the ggplot2 package 101 ( https://cran.r-project.org/web/packages/ggplot2/index.html ). The intensity of change rate was represented by the shades of red and green color indicating the highly negative change rate and vice versa. Results show that the after 1970, all meteorological sub-divisions were observed the negative departure in almost all years from long-term average rainfall by 50 mm.−2000 mm. (Fig. 5 ). While the sub-divisions of North-Eastern India and North India were recorded positive change rate only for few years because of the occurrences of excessive rainfall that was happened occasionally by monsoon burst, ELSO effect (Kripalini et al . 2003) and local climatic effect. However, the highest negative change rate was observed in the sub-divisions like Arunachal Pradesh, Nagaland Manipur, Mizoram & Tripura, Kerala, Western Uttar Pradesh, Rajasthan, Uttarakhand and Himachal Pradesh by more than −2000 mm. While the positive change rate was mainly detected before change detection year or 1970 in all sub-divisions. The positive change rate was varied from 0–2000 mm. However, few sub-divisions like Kerala, Nagaland Manipur Mizoram & Tripura, and Coastal Karnataka.
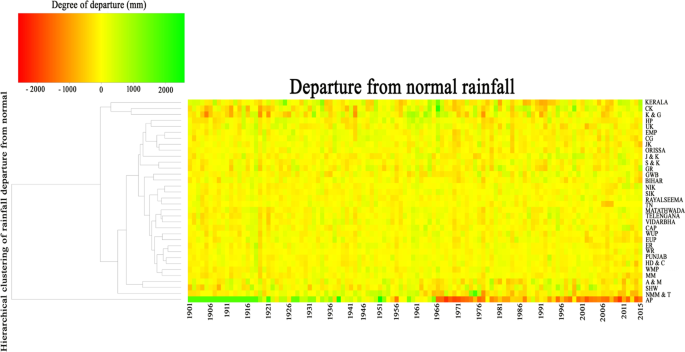
Heatmap represents the departure of rainfall from normal rainfall for all meteorological subdivision. (N.B. CK- Coastal Karnataka, K & G – Konkan and Goa, HP- Himachal Pradesh, UK- Uttarakhand, EMP- Eastern Madhya Pradesh, CG- Chhattisgarh, JK – Jharkhand, J & K – Jammu and Kashmir, S & K- Saurashtra and Kachcha, GR – Gujarat region, GWB – Gangetic West Bengal, NIK – North Interior Karnataka, SIK – South Interior Karnataka, TN – Tamilnadu, CAP – Coastal Andhra Pradesh, WUP – western Uttarpradesh, EUP – Eastern Uttarpradesh, ER – Eastern Rajasthan, WR – Western Rajasthan, HD & C- Haryana Delhi and Chandigarh, WMP – WesternMadhyapradesh, MM – MadhyaMadhyapradesh, A & M- Assam and Meghalaya, SHW – Sub Himalaya West Bengal, NMM & T- Nagaland, Manipur, Mizoram and Tripura, AP- Arunachal Pradesh.
Rainfall prediction and forecasting
The above mentioned analyses show that most of the meteorological sub-divisions of India were experienced significant decrease in rainfall and this decrease was become stronger after the change point. Therefore, the situation of rainfall incidence became critical post change point. Therefore, if the present situation of rainfall occurrences continues with the same intensity, the intensity of rainfall occurrences and their distribution in future will be more worsen. Therefore, the prediction and forecasting of rainfall is become essential for water resource management and planning. Several soft computing machine learning techniques are available for predicting and forecasting rainfall, but MLP based artificial neural network has become widely popular among the researchers as it is easy to compute and generate high quality product 102 , 103 . In this study, we applied MLP-ANN on the annual rainfall data for prediction. We set the parameters of artificial neural network models again and again until the best prediction had not achieved which we evaluated using RMSE techniques. We fixed the model’s parameters and applied on the rainfall of thirty-four meteorological sub-divisions for prediction. In some cases, we changed some of models parameters for predicting in rainfall. However, the Supplementary Table 3 shows the plot between the best MLP-ANN generated model and observed rainfall for thirty four meteorological sub-divisions that indicates that there is close adjacency between predicted and observed rainfall. The error measures like RMSE and MAE (in mm.) were used to quantify the closeness between predicted and observed rainfall. The Supplementary Table 4 reports the 15 years rainfall forecasting up to 2030 for all meteorological sub-divisions. We selected the rainfall of 2020 and 2030 as the representative for spatial mapping (Fig. 6 ) (for the rainfall forecasting data of all years was presented in Supplementary Table 4 ). The findings of spatial mapping of future forecasting show the more declining amount of rainfall.
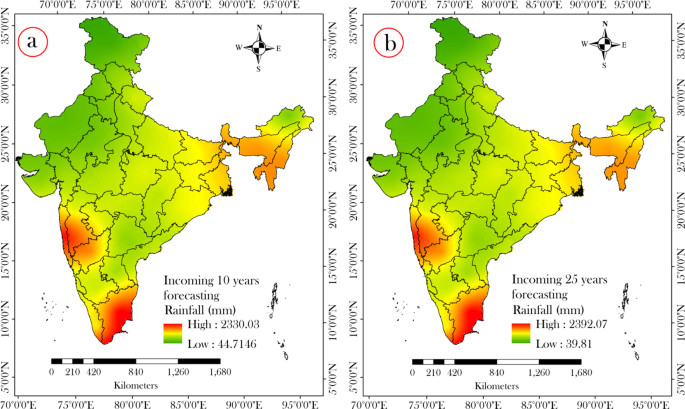
Spatial rainfall forecast for 2020 ( a ) and 2030 ( b ).
In 2020, the maximum rainfall incidence will be observed in the sub-divisions of Coastal Karnataka, Konkan & Goa, Kerala (>2500 mm rainfall) and the minimum rainfall event will be placed on the West Rajasthan, Tamil Nadu, East Rajasthan, Rayalseema (<400 mm rainfall) (Fig. 6a ). On the other hand, in 2030, the highest rainfall will be occurred in the sub-divisions of Coastal Karnataka, Konkan & Goa, Kerala (>2000 mm rainfall), while the lowest rainfall event will be found in the sub-divisions like West Rajasthan, East Rajasthan, Tamilnadu, Rayalseema (<300 mm rainfall) (Fig. 6b ). The findings of future forecasting analysis point out that the occurrences of rainfall will be decreasing gradually for some meteorological stations, while the rainfall will be increased in some meteorological sub-division. The places of rainfall occurrences over India will be remained as like identical pattern of present and earlier rainfall incidences. The findings also show that the extreme rainfall events will be increased in future that will be lead to flooding situation.
Causes of rainfall variation
India encompasses large areas with moderate to high convective precipitation, while low convective rainfall rate occurred which was brought excessive moisture from the Indian Ocean to the in land areas, which is unfavorable for the formation of rainfall (Fig. 7a–f ). This caused a decreased in mean rainfall during monsoon season, which has been distributed in the extreme southern and mid-central divisions of the country (Fig. 7 ). The northeasterly wind was strengthened in the whole country, which reduced the invasions of cooler air, led to decline in rainfall all over India in the winter season. By contrast, most of the divisions had moderate to high mean convective precipitation rate, which increased rainfall to some extent. The low cloud has increased all over the India, and a few cloud covers will enhance the consolidation impact of atmosphere on the solar radiation, and hence it leads to declining rainfall trend. Moreover, high mean total precipitation rate has been influencing across the country except for eastern division, which triggered uneven downdraft pattern and leading to more clear sky days during the recent study period (1979–2017). Most of the regions in India exhibited a declining vertically integrated moisture divergence which triggered by a significant decreasing rainfall changes.
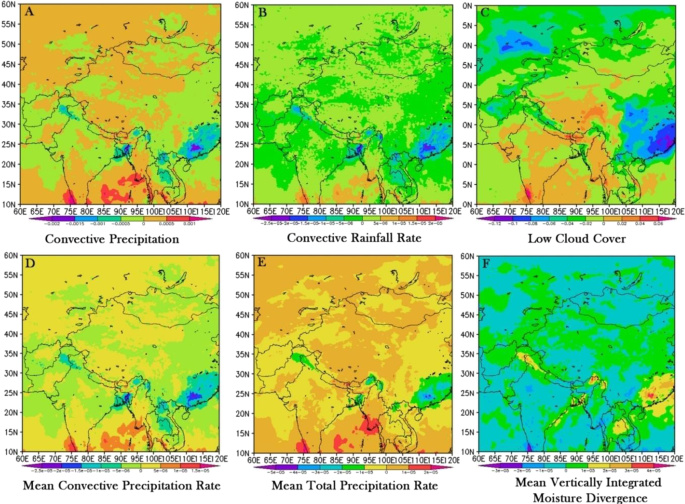
Spatial variations of differences in ( a ) convective precipitation in monsoon season, ( b ) convective rainfall rate in winter season, ( c ) low cloud cover, ( d ) mean convective precipitation rate, ( e ) mean total precipitation rate, and ( f ) mean vertically integrated moisture divergence between the recent period of 2001–2015 and 1979–2000.
Marumbwa et al . 104 suggested that the analysis of historical rainfall trend is very crucial in several fields like water resource management, sustainable agricultural planning, ecosystem management and health sector. The rainfall data of 115 years were used to investigate the variability of annual and seasonal rainfall in very detail and analyzed the trend in several ways for thirty-four meteorological sub-divisions. The findings of 115 years annual and seasonal rainfall variation analysis show that the highest rainfall variation was found in the sub-divisions of Western India of annual and summer, Western India and South Western India of winter, North India and Western India of monsoon and Western, North Western and some parts of Eastern India of post monsoon rainfall. While the lowest variation was found in the sub-divisions of North Eastern and Eastern India. The identical result was reported by several previous studies 32 , 45 , 66 , 71 , 105 . The findings of MK test on annual and seasonal rainfall report that thirteen meteorological sub-divisions were observed negative trend, while rest of the sub-divisions were recorded positive trend. Rajeevan et al . 106 and Guhathakurta and Rajeevan 46 reported that Jharkhand, Chhattisgarh and Kerala were observed negative trend, while eight sub-divisions like Gangetic West Bengal, Coastal Andhra Pradesh, Kanakan & Goa, North Interior Karnataka, Rayalessema, Jammu &Kashmir were recorded decreasing trend over time. These results are totally identical with the findings of the MK test of the present study (Very dark shade of brown color rows in Table 1 ). Mirza et al . 107 reported that the annual and seasonal rainfall was more or less consistent in sub-divisions sub-himalayan Bengal and Gangetic Bengal which was by and large similar with the findings of MK test in the present study (Table 1 ).
Mondal et al . 32 used Pettitt test and SNHT test for detecting change point in the annual rainfall for all meteorological sub-divisions for their study and reported that twenty-one sub-divisions had the change point using Pettitt test between 1950–1966. While we detected eighteen sub-divisions that have change point between 1950–1966 (Supplementary Table 1 ). Goyal 98 documented that the most probable change point was 1959, while in the present study, most of the change points were detected in between 1950–1966 which can conclude that the findings of present work are identical with the work of Goyal. Therefore this study concludes that 115 years annual rainfall had not the monotonous trend and it needs for further research to detect the trend and its magnitude accurately. Based on which planners and scientists can propose the developmental and management plan.
Furthermore, MK test was employed on the annual and seasonal rainfall data of both pre and post change point phase to detect the exact and accurate trend as several scientists recommended to apply MK test based on the analysis of change point to achieve the accurate trend 34 , 38 , 108 . The misleading results could be found if the MK test applies first 38 . However, The findings of MK test on the annual and seasonal rainfall for pre change point phase stated that among the 34 meteorological divisions, 8 sub-divisions of each monsoon and post monsoon, 17 sub-divisions of summer and 18 sub-divisions of winter season were detected insignificant negative but trend (Table 2 ). Parthasarathy and Dhar 109 documented that most of the sub-divisions were recorded positive trend for the period 1901–1970 that was the change point year for the most of the sub-divisions in the present study (Table 2 and Supplementary Table 1 ).
The findings of the MK test for post change point phase showed that the negative significant trend was detected in the 17 meteorological sub-divisions of monsoon, 15 sub-divisions of post monsoon, 19 sub-divisions of summer and 21 sub-divisions of winter season (Table 3 ). Jain and Kumar 110 reported that 4 river basins of India were detected as increasing trend, while 13 river basins were observed significant negative trend in monsoon rainfall. In case of annual rainfall, 15 river basins were recorded negative trend. Therefore, we can state that these findings are supporting the present work. Guhathakurta et al . 111 reported that significant negative trend was found in NW and Central and Peninsular India for the period of 1951–2011. Several studies also reported that the significant amount of rainfall was decreased over time, especially after 1950 44 , 46 , 81 , 105 , 112 .
To the best of authors’ knowledge, the application of recently developed innovative trend analysis to detect rainfall trend was very rare. We found the work of Machiwal et al . 113 who applied innovative trend analysis to detect trend in rainfall and temperature of Indian arid region. Machiwal et al . 113 documented that increasing trend for monsoon rainfall was found in the arid region and this result is identical with the findings of the present study. However, the findings of Innovative trend analysis on annual and seasonal rainfall showed that negative trend was observed in the sub-divisions of NE, E, SE and S part of summer, NE and some parts of E India of monsoon, NE, E India of post-monsoon and W, SW, S India of winter rainfall. The identical finding was found in the work of Mondal et al . 32 . Taxak et al . 96 studied grid wise rainfall trend over India and documented that most of grids were received negative trend except seven grids which were observed positive trend. The findings of this study are totally identical with the present work.
Dash et al . 53 and Kumar et al . 11 documented that frequency of intense rainfall in many parts of Asia has increased, while the amount of rainfall and number of rainy days has decreased significantly. For this reason, the year wise departure study revealed that some of the years were observed positive departure from the long-term average rainfall by more than 1000 mm rainfall (Fig. 5 ). Even the identical findings can be found in the work of Goswami et al . 40 and they reported that the frequency and magnitude of extreme rainfall has amplified significantly, while the frequency and intensity of moderate rainfall has observed noteworthy decreasing trend.
In the present study, the change rate was maximum in the meteorological sub-divisions of Western and Central India in annual and monsoon rainfall, whereas the maximum change rate (%) for winter season was in the sub-divisions of Central, North East and Eastern India. Mondal et al . 32 reported that many parts of Western India and Central India were received very high negative change rate for monsoon rainfall, while Central and North Eastern parts of India were observed highly decreasing change rate. Furthermore, it can be concluding from the analysis that North Eastern part of India has been observed the worst effect of climate change than any other parts of India.
In the present study, the annual rainfall for thirty-four meteorological sub-divisions were predicted and forecasted up to 2030. In addition, the rainfall forecasting for 2030 showed an expected decline of about 5–10% in the overall rainfall of India (Fig. 6 ). However, numbers of studies were conducted to predict and forecast rainfall for India based on the average rainfall and they did not consider studying for all parts of the India. Guhathakurta 114 predicted the rainfall using neural network for Kerala. While Chakraborty et al . 49 predicted the south-west monsoon for India. They used average time series annual rainfall dataset of India. But in the present study, we considered rainfall datasets for thirty-four meteorological sub-divisions. Therefore, the findings of this study would not be generalized; rather it would be more accurate and can be act as the foundation of the developmental planning.
The temporary change in rainfall distribution significantly distresses the agricultural production. It will increase the drought protection and resilience plans under the changing climate conditions. Intergovernmental Panel on Climate change (IPCC) reported that upcoming changes in climate is to be likely to distress agriculture that will amplify the chance of hunger and water paucity, as well as the instructions on quicker thawing of glaciers 115 . Gosain et al . 116 pointed that the amount of freshwater in the river of India is probable to be diminish because of changing climate. This reduction, along with growing population might unfavourably affect a large population in India by 2050.
Large-scale ocean-atmospheric changes derived from the ECMWF ERA5 re-analysis data depicted that compared between 1979–2000 to 2001–2015 period, an increasing/decreasing convective precipitation rate, enhanced low cloud cover and inadequate moisture variance in the Indian ocean being transported to the northwest direction might have highly influenced the rainfall trend in India.Therefore, it is expected that this study will provide an insight for the management and development of agriculture and water resources in India to overcome the possible impacts of the climate change.
The basic and essential requirement for the management and planning of water resource, sustainable agricultural development and other sectors is the exploration of the spatiotemporal distribution and changing pattern of rainfall in any places. Hence, the present study investigated the variability and trend analysis of annual rainfall in several ways like overall data, change point wise (pre and post change point) using 115 years long-term annual and seasonal rainfall data of thirty-four meteorological sub-divisions. The present study shows that the overall annual and seasonal variability of rainfall was highest in the sub-divisions of Western India, while the lowest variability was found in Eastern and North India. The findings of MK test on overall annual and seasonal rainfall reports that the sub-divisions of North-East, South and Eastern India were detected significant negative trend, while the sub-divisions like Sub-Himalayan Bengal, Gangetic Bengal, Jammu & Kashmir, Konkan & Goa, Madhya Maharastra and Marathwada were recorded positive trend. Furthermore, the change detection techniques were utilized and selected the change point based on the performances of the techniques. The most probable change points were detected in between 1950–1966. Based on the change point year, the rainfall variability and trend analysis were again carried out for pre and post change point phase. The rainfall variability was increased significantly in most of the meteorological sub-divisions after post change point and similar kinds of findings were found when the rainfall trend was analyzed for post change point. To get better results of trend analysis, the innovative trend analysis was employed. The finding shows that most of the sub-divisions were recorded significant negative trend. Even some of the sub-divisions were detected as no trend using MK test, but the trend was detected using innovative trend analysis. However, the micro level change rate analysis was used in the present study and the results show that after 1960, most of the meteorological sub-divisions were recorded more than −500mm rainfall departure from the long-term average rainfall in many years, while few years were detected positive departure in few sub-divisions. Therefore, from the detail analysis, it is established that almost all of the sub-divisions were detected the negative trend and high variability after 1970. Even the year wise study also revealed that in which year and how much rainfall amount was departed. Hence, these detail information regarding historical data for whole country are very beneficial for the planning. One of the most striking features of developmental planning in the recent time is the forecast of the incoming event which can be found in any sector like finance, water resource and most importantly climatology. Therefore, in the present study, the rainfalls for all meteorological sub-divisions were forecasted using the advance AI models like artificial neural network. The findings of the rainfall forecasting show that 15% of rainfall will be declined in 2030 that indicates the alarming situations will be appeared for both environment and living world.
The economy of India is totally dependent on the rainfall either it is agriculture or industry. Therefore, the water resource is essential part of the progressive economy of India. But due to climate change, the world rainfall pattern has been disturbed. Therefore, many studies were carried out in the developed countries to quantify the pattern of the rainfall changes and formulate the management plan accordingly. But in case of India, very fewer studies were done to do so. The present study provides information in all aspects like rainfall variability and trend for overall and change point wise for annual and seasonal rainfall, rainfall change rate since change point year, year wise departure, and future rainfall and most importantly this study analyzes the causes of rainfall changes in India. Technically, the present study used several sophisticated techniques which have been admired worldwide by the scientists for providing high precision results. This type of studies has not been conducted for whole India. Therefore, the present study can be the full package and should be very much helpful to the Indian planners to proposing plans for small and large scale regions.
To formulate the management plan for the sustainable development of water resource based sectors and environment, the scientist of others countries can conduct the research like the present study as they need lots of information for developing plan regarding historical, present and future data which can be in any field like hydrology, climatology.
However, in the present study, we considered thirty-four meteorological sub-divisions for the research, but to be more accurate, micro level data like district wise data should be incorporated. Then the very high precision micro level management plan will be achieved. Even, the grid wise rainfall study using very advanced microwave remote sensing technology will be very useful for the planners. The ensemble machine learning techniques, deep learning techniques like long-short-term memory (LSTM) network can be used to achieve very high quality forecasting data.
Islam, T., Rico-Ramirez, M. A., Han, D. & Srivastava, P. K. A Joss–Waldvogel disdrometer derived rainfall estimation study by collocated tipping bucket and rapid response rain gauges. Atmospheric Science Letters 13 , 139–150 (2012).
ADS Google Scholar
Gajbhiye, S., Meshram, C., Singh, S. K., Srivastava, P. K. & Islam, T. Precipitation trend analysis of Sindh River basin, India, from 102‐year record (1901–2002). Atmospheric Science Letters 17 (1), 71–77 (2015).
Google Scholar
Srivastava, P. K., Han, D., Rico-Ramirez, M. A. & Islam, T. Sensitivity and uncertainty analysis of mesoscale model downscaled hydro-meteorological variables for discharge prediction. Hydrological Processes 28 , 4419–4432 (2014).
Islam, T., Rico-Ramirez, M. A., Han, D., Srivastava, P. K. & Ishak, A. M. Performance evaluation of the TRMM precipitation estimation using ground-based radars from the GPM validation network. Journal of Atmospheric and Solar-Terrestrial Physics 77 , 194–208 (2012).
Srivastava, P. K., Mehta, A., Gupta, M., Singh, S. K. & Islam, T. Assessing impact of climate change on Mundra mangrove forest ecosystem, Gulf of Kutch, western coast of India: a synergistic evaluation using remote sensing. Theoretical and Applied Climatology 120 (3–4), 685–700 (2015).
Gupta, M., Srivastava, P. K., Islam, T. & Ishak, A. M. B. Evaluation of TRMM rainfall for soil moisture prediction in a subtropical climate. Environmental Earth Sciences 71 , 4421–4431 (2014).
Meshram, S. G., Singh, V. P. & Meshram, C. Long-term trend and variability of precipitation in Chhattisgarh State, India. Theoretical and Applied Climatology 129 (3–4), 729–744 (2017).
Bandyopadhyay, J., & Perveen, S. A scrutiny of the justifications for the proposed interlinking of rivers in India. Interlinking of rivers in India: Overview and Ken-Betwa link , 23 (2006).
Attri, S. D., & Tyagi, A. Climate profile of India. Environment Monitoring and Research Center, India Meteorology Department: New Delhi, India (2010).
Jain, A. A Study of Trends and Magnitude of Farmer Suicides in India’. International Journal of Advances and Scholarly Research in Allied Education 13 (2), 80–85 (2017).
Kumar, V., Jain, S. K. & Singh, Y. Analysis of long-term rainfall trends in India. Hydrological Sciences Journal–Journal des Sciences Hydrologiques 55 (4), 484–496 (2010).
Yang, P., Ren, G. & Yan, P. Evidence for a strong association of short-duration intense rainfall with urbanization in the Beijing urban area. Journal of Climate 30 (15), 5851–5870 (2017).
Chatterjee, S., Khan, A., Akbari, H. & Wang, Y. Monotonic trends in spatio-temporal distribution and concentration of monsoon precipitation (1901–2002), West Bengal, India. Atmospheric Research 182 , 54–75 (2016).
Tian, Y., Bai, X., Wang, S., Qin, L. & Li, Y. Spatial-temporal changes of vegetation cover in Guizhou Province, Southern China. Chinese Geographical Science 27 (1), 25–38 (2017).
Xia, J., She, D., Zhang, Y. & Du, H. Spatio-temporal trend and statistical distribution of extreme precipitation events in Huaihe River Basin during 1960–2009. J. Geogr. Sci. 22 , 195–208 (2012).
Rao, B. B., Chowdary, P. S., Sandeep, V. M., Rao, V. U. M. & Venkateswarlu, B. Rising minimum temperature trends over India in recent decades: implications for agricultural production. Glob Planet Chang 117 , 1–8 (2014).
Talaee, P. H. Iranian rainfall series analysis by means of nonparametric tests. Theoretical and applied climatology 116 (3–4), 597–607 (2014).
Sonali, P. & Kumar, D. N. Review of trend detection methods and their application to detect temperature changes in India. Journal of Hydrology 476 , 212–227 (2013).
Haan, C. T. Statistical methods in hydrology. Second edition. Iowa State University Press, Ames, Iowa 496pp (2002).
Piao, S. L. et al . The impacts of climate change on water resources and agriculture in China. Nature 467 (2), 43–51 (2010).
ADS CAS PubMed Google Scholar
Mann, H. B. Non-parametric tests against trend. Econometrica 13 , 245–259 (1945).
MathSciNet MATH Google Scholar
Kendall, M. G. Time Series. Charles Griffin and Co. Ltd., London (1973).
Kendall, M. G. Rank correlation methods. Charles Griffin and Co. Ltd., London, U.K. (1975).
Sen, P. K. Estimates of the regression coefficient based on Kendall’s tau. J Am Stat Assoc 63 (324), 1379–1389 (1968).
Pingale, S. M., Khare, D., Jat, M. K. & Adamowski, J. Spatial and temporal trends of mean and extreme rainfall and temperature for the 33 urban centers of the arid and semi-arid state of Rajasthan, India. Atmos Res 138 , 73–90 (2014).
McGhee, J. W. Introductory statistics. West Publishing Co., New York (1985).
Batisani, N. & Yarnal, B. Rainfall variability and trends in semi-arid Botswana: implications for climate change adaptation policy. Applied Geography 30 (4), 483–489 (2010).
Tabari, H., Marofi, S., Aeini, A., Hosseinzadeh Talaee, P. & Mohammadi, K. Trend analysis of reference evapotranspiration in the western half of Iran. Agric For Meteorol 151 , 128–136 (2011).
Du, J. & Shi, C. Effects of climatic factors and human activities on runoff of the Weihe River in recent decades. Quaternary International 282 , 58–65 (2012).
Singh, P., Kumar, V., Thomas, T. & Arora, M. Changes in rainfall and relative humidity in different river basins in the northwest and central India. Hydrol Process 22 , 2982–2992 (2008).
Wang, S., Yan, M., Yan, Y., Shi, C. & He, L. Contributions of climate change and human activities to the changes in runoff increment in different sections of the Yellow River. Quaternary International 282 , 66–77 (2012).
Mondal, A., Khare, D. & Kundu, S. Spatial and temporal analysis of rainfall and temperature trend of India. Theoretical and applied climatology 122 (1–2), 143–158 (2015).
Zarenistanak, M., Dhorde, A. G. & Kripalani, R. H. Trend analysis and change point detection of annual and seasonal precipitation and temperature series over southwest Iran. Journal of earth system science 123 (2), 281–295 (2014).
Li, D., Xie, H. & Xiong, L. Temporal change analysis based on data characteristics and nonparametric test. Water Resour Manage 28 , 227–240 (2014).
Alexandersson, H. & Moberg, A. Homogenization of Swedish temperature data. Part I: Homogeneity test for linear trends. International Journal of Climatology: A Journal of the Royal Meteorological Society 17 (1), 25–34 (1997).
Buishand, T. A. Some methods for testing the homogeneity of rainfall records. Journal of hydrology 58 (1-2), 11–27 (1982).
Pettitt, A. N. A non‐parametric approach to the change‐point problem. Journal of the Royal Statistical Society: Series C (Applied Statistics) 28 (2), 126–135 (1979).
Villarini, G., Serinaldi, F., Smith, J. A. & Krajewski, W. F. On the stationarity of annual flood peaks in the continental United States during the 20th century. Water Resour Res 45 , W08417 (2009).
Sen Roy, S. & Balling, R. C. Jr Diurnal variations in summer season precipitation in India. International Journal of Climatology: A Journal of the Royal Meteorological Society 27 (7), 969–976 (2007).
Goswami, B. N., Venugopal, V., Sengupta, D. & Madhusoodanan, M. S. and xavier, P. K. Increasing Trend of Extreme Rain Events Over India in a Warming Environment. Science 314 (5804), 1442–1445 (2006).
Nikumbh, A. C., Chakraborty, A. & Bhat, G. S. Recent spatial aggregation tendency of rainfall extremes over India. Scientific reports 9 (1), 10321 (2019).
ADS PubMed PubMed Central Google Scholar
Varikoden, H., Revadekar, J. V., Kuttippurath, J. & Babu, C. A. Contrasting trends in southwest monsoon rainfall over the Western Ghats region of India. Climate Dynamics 52 (7–8), 4557–4566 (2019).
Bisht, D. S., Chatterjee, C., Raghuwanshi, N. S. & Sridhar, V. Spatio-temporal trends of rainfall across Indian river basins. Theoretical and applied climatology 132 (1–2), 419–436 (2018).
Sanikhani, H., Kisi, O., Mirabbasi, R. & Meshram, S. G. Trend analysis of rainfall pattern over the Central India during 1901–2010. Arabian Journal of Geosciences 11 (15), 437 (2018).
Paul, S. et al . Weakening of Indian summer monsoon rainfall due to changes in land use land cover. Scientific reports 6 , 32177 (2016).
ADS CAS PubMed PubMed Central Google Scholar
Guhathakurta, P. & Rajeevan, M. Trends in the rainfall pattern over India. International Journal of Climatology: A Journal of the Royal Meteorological Society 28 , 1453–1469 (2008).
Srivastava, P., Singh, R., Tripathi, S. & Raghubanshi, A. S. An urgent need for sustainable thinking in agriculture–An Indian scenario. Ecological indicators 67 , 611–622 (2016).
Swain, S., Verma, M. & Verma, M. K. Statistical trend analysis of monthly rainfall for Raipur District, Chhattisgarh. Int. J. Adv. Engg. Res. Studies/IV/II/Jan.-March 87 , 89 (2015).
Chakraborty, S., Pandey, R. P., Chaube, U. C. & Mishra, S. K. Trend and variability analysis of rainfall series at Seonath River Basin, Chhattisgarh (India). International Journal of Applied Science and Engineering Research 2 (4), 425–434 (2013).
Mondal, A., Lakshmi, V. & Hashemi, H. Intercomparison of trend analysis of multisatellite monthly precipitation products and gauge measurements for river basins of India. Journal of Hydrology 565 , 779–790 (2018).
Bera, S. Trend analysis of rainfall in Ganga Basin, India during 1901-2000. American Journal of Climate Change 6 (1), 116 (2017).
Chandniha, S. K., Meshram, S. G., Adamowski, J. F. & Meshram, C. Trend analysis of precipitation in Jharkhand State, India. Theoretical and Applied Climatology 130 (1-2), 261–274 (2017).
Dash, S. K., Jenamani, R. K., Kalsi, S. R. & Panda, S. K. Some evidence of climate change in twentieth-century India. Climatic Change 85 , 299–321 (2007).
Patra, J. P., Mishra, A., Singh, R. & Raghuwanshi, N. S. Detecting rainfall trends in twentieth century (1871–2006) over Orissa State, India. Clim. Change 111 , 801–817 (2012).
Şen, Z. Innovative trend analysis methodology. Journal of Hydrologic Engineering 17 (9), 1042–1046 (2012).
Sen, Z. Trend identification simulation and application. J. Hydrol. Eng. 19 (3), 635–642 (2014).
Kisi, O. An innovative method for trend analysis of monthly pan evaporations. Journal of Hydrology 527 , 1123–1129 (2015).
Ay, M. & Kisi, O. Investigation of trend analysis of monthly total precipitation by an innovative method. Theoretical and Applied Climatology 120 (3–4), 617–629 (2015).
Martinez-Austria, P. F., Bandala, E. R. & Patiño-Gómez, C. Temperature and heat wave trends in northwest Mexico. Physics and Chemistry of the Earth, Parts A/B/C 91 , 20–26 (2016).
Onyutha, C. Identification of sub-trends from hydro-meteorological series. Stochastic Environmental Research and Risk Assessment 30 (1), 189–205 (2016).
Wu, H. & Qian, H. Innovative trend analysis of annual and seasonal rainfall and extreme values in Shaanxi, China, since the 1950s. International Journal of Climatology 37 (5), 2582–2592 (2017).
Şen, Z. Innovative trend significance test and applications. Theoretical and applied climatology 127 (3-4), 939–947 (2017).
Von Storch, H. Misuses of statistical analysis in climate research. Analysis of climate variability: applications of statistical techniques 2nd edn. In: Proceedings of an Autumn School organized by the Commission of the European Community on Elba from October 30 to November 6, 1993. Springer, Berlin pp 11–26 (1995).
Gedefaw, M. et al . Innovative trend analysis of annual and seasonal rainfall variability in Amhara regional state, Ethiopia. Atmosphere 9 (9), 326 (2018).
Kişi, Ö., Guimaraes Santos, C. A., Marques da Silva, R. & Zounemat-Kermani, M. Trend analysis of monthly streamflows using Şen’s innovative trend method. Geofizika 35 (1), 53–68 (2018).
Pandey, B. K. & Khare, D. Identification of trend in long term precipitation and reference evapotranspiration over Narmada river basin (India). Global and planetary change 161 , 172–182 (2018).
Darji, M. P., Dabhi, V. K. & Prajapati, H. B. Rainfall forecasting using neural network: A survey. IEEE International Conference on Advances in Computer Engineering and Applications Ghaziabad 2015 , 706–713 (2015).
Carter, J. G. et al . Climate change and the city: Building capacity for urban adaptation. Progress in planning 95 , 1–66 (2015).
Pal, S. & Talukdar, S. Modelling seasonal flow regime and environmental flow in Punarbhaba river of India and Bangladesh. Journal of Cleaner Production 252 , 119724 (2020).
Mukherjee, P., Singh, C. K. & Mukherjee, S. Delineation of groundwater potential zones in arid region of India—a remote sensing and GIS approach. Water resources management 26 (9), 2643–2672 (2012).
Chakraverty, S. & Gupta, P. Comparison of neural network configurations in the long-range forecast of southwest monsoon rainfall over India. Neural Comput Appl 17 , 187–192 (2008).
Şahin, M., Kaya, Y. & Uyar, M. Comparison of ANN and MLR models for estimating solar radiation in Turkey using NOAA/AVHRR data. Advances in Space Research 51 (5), 891–904 (2013).
Samantaray, S., Tripathy, O., Sahoo, A., & Ghose, D. K. Rainfall Forecasting Through ANN and SVM in Bolangir Watershed, India. In Smart Intelligent Computing and Applications 767-774 (2020).
Hossain, I., Rasel, H. M., Imteaz, M. A., & Mekanik, F. Long-term seasonal rainfall forecasting using linear and non-linear modelling approaches: a case study for Western Australia. Meteorology and Atmospheric Physics, 1–11 (2019).
Liu, Q., Zou, Y., Liu, X. & Linge, N. A survey on rainfall forecasting using artificial neural network. IJES 11 (2), 240–249 (2019).
Alam, W. et al . Improved ARIMAX modal based on ANN and SVM approaches for forecasting rice yield using weather variables. Indian Journal of Agricultural Sciences 88 (12), 101–105 (2018).
Arabeyyat, O., Shatnawi, N. & Matouq, M. Nonlinear Multivariate Rainfall Prediction in Jordan Using NARX-ANN Model with GIS Techniques. Jordan Journal of Civil Engineering 12 , 3 (2018).
Kala, A., & Vaidyanathan, S. G. Prediction of Rainfall Using Artificial Neural Network. In 2018 International Conference on Inventive Research in Computing Applications 339–342 (2018).
Mishra, S. K., & Sharma, N. Rainfall forecasting using backpropagation neural network. In Innovations in Computational Intelligence 277–288 (2018).
Şenkal, O., Şahin, M. & Peştemalci, V. The estimation of solar radiation for different time periods. Energy Sources, Part A: Recovery, Utilization, and Environmental Effects 32 (13), 1176–1184 (2010).
Sahai, A. K., Soman, M. K. & Satyan, V. All India summer monsoon rainfall prediction using an artificial neural network. Climate dynamics 16 (4), 291–302 (2000).
Das, S., Dey, S., Dash, S. K., Giuliani, G. & Soloman, F. Dust aerosol feedback on the Indian summer monsoon: Sensitivity to absorption property. J. Geophys. Res. Atmos. 120 , 9642–9652 (2015).
Sharma, R., Hooyberghs, H., Lauwaet, D. & De Ridder, K. Urban heat island and future climate change—Implications for Delhi’s heat. Journal of Urban Health 96 (2), 235–251 (2019).
PubMed Google Scholar
Yue, S. & Hashino, M. Long term trends of annual and monthly precipitation in Japan 1. JAWRA Journal of the American Water Resources Association 39 (3), 587–596 (2003).
Yue, S. & Wang, C. Y. Applicability of prewhitening to eliminate the influence of serial correlation on the Mann‐Kendall test. Water resources research 38 (6), 4–1 (2002).
Von Storch, H. Spatial patterns: EOFs and CCA. In Analysis of climate variability (pp. 231–263). Springer, Berlin, Heidelberg (1999).
Gao, P., Mu, X. M., Wang, F., & Gao, P. Changes in streamflow and sediment discharge and the response to human activities in the middle reaches of the Yellow River (2011).
Zhang, S. & Lu, X. X. Hydrological responses to precipitation variation and diverse human activities in a mountainous tributary of the lower Xijiang, China. Catena 77 (2), 130–142 (2009).
Westra, S. et al . Future changes to the intensity and frequency of short‐duration extreme rainfall. Reviews of Geophysics 52 (3), 522–555 (2014).
Kumar, V. Optimal contour mapping of groundwater levels using universal kriging—a case study. Hydrological Sciences Journal 52 (5), 1038–1050 (2007).
Agrawal, R. & Srikant, R. Mining sequential patterns. In icde 95 , 3–14 (1995).
Vauclin, M., Vieira, S. R., Vachaud, G. & Nielsen, D. R. The Use of Cokriging with Limited Field Soil Observations 1. Soil Science Society of America Journal 47 (2), 175–184 (1983).
Nielsen, D. R., & Wendroth, O. Spatial and temporal statistics: sampling field soils and their vegetation. Catena Verlag (2003).
Wang, Y. Q. & Shao, M. A. Spatial variability of soil physical properties in a region of the Loess Plateau of PR China subject to wind and water erosion. Land Degrad. Dev. 24 (3), 296–304 (2013).
Duahn, D. & Pandey, A. Statistical analysis of long term spatial and temporal trends of precipitation during 1901–2002 at Madhya Pradesh, India. Atmospheric Research 122 , 136–149 (2013).
Taxak, A. K., Murumkar, A. R. & Arya, D. S. Long term spatial and temporal rainfall trends and homogeneity analysis in Wainganga basin, Central India. Weather and Climate Extremes 4 , 50–61 (2014).
Helsel, D. R. & Hirsch, R. M. Statistical methods in water resources 323, Reston, VA: US Geological Survey (2002).
Goyal, M. K. Statistical analysis of long term trends of rainfall during 1901–2002 at Assam, India. Water resources management 28 (6), 1501–1515 (2014).
Kisi, O. & Ay, M. Comparison of Mann–Kendall and innovative trend method for water quality parameters of the Kizilirmak River, Turkey. Journal of Hydrology 513 , 362–375 (2014).
ADS CAS Google Scholar
Cui, L. et al . Innovative trend analysis of annual and seasonal air temperature and rainfall in the Yangtze River Basin, China during 1960–2015. Journal of Atmospheric and Solar-Terrestrial Physics 164 , 48–59 (2017).
Wickham, H. ggplot2: elegant graphics for data analysis. Springer (2016).
Du, J., Liu, Y., Yu, Y. & Yan, W. A prediction of precipitation data based on support vector machine and particle swarm optimization (PSO-SVM) algorithms. Algorithms 10 (2), 57 (2017).
Hong, W. C. Rainfall forecasting by technological machine learning models. Applied Mathematics and Computation 200 (1), 41–57 (2008).
Marumbwa, F. M., Cho, M. A. & Chirwa, P. W. Analysis of spatio-temporal rainfall trends across southern African biomes between 1981 and 2016. Physics and Chemistry of the Earth, Parts A/B/C 114 , 102808 (2019).
Sen Roy, S. A spatial analysis of extreme hourly precipitation patterns in India. Int. J. Climatol. 29 , 345–355 (2009).
Rajeevan, M., Bhate, J., Kale, J. D. & Lal, B. High resolution daily gridded rainfall data for the Indian region: Analysis of break and active monsoon spells. Curr. Sci. 91 (3), 296–306 (2006).
Mirza, M. Q., Warrick, R. A., Ericksen, N. J. & Kenny, G. J. Trends and persistence in precipitation in the Ganges. Brahmaputra and Meghna river basins. Hydrol. Sci. J. 43 , 845–858 (1998).
Wang, S. & Zhang, X. Long-term trend analysis for temperature in the Jinsha River Basin in China. Theor Appl Climatol 109 , 591–603 (2012).
Parthasarathy, B. & Dhar, O. N. Secular variations of regional rainfall over India. Q. J. R. Meteorol. Soc. 100 , 245–257 (1974).
Jain, S. K. & Kumar, V. Trend analysis of rainfall and temperature data for India. Current Science 102 (1), 37–49 (2012).
Guhathakurta, P., Rajeevan, M., Sikka, D. R. & Tyagi, A. Observed changes in southwest monsoon rainfall over India during 1901–2011. International Journal of Climatology 35 (8), 1881–1898 (2015).
Xu, C. et al . Decreasing Indian summer monsoon on the northern Indian sub-continent during the last 180 years: evidence from five tree-ring cellulose oxygen isotope chronologies. Climate of the Past 14 (5), 653–664 (2018).
Machiwal, D., Jha, M. K., Singh, V. P. & Mohan, C. Assessment and mapping of groundwater vulnerability to pollution: Current status and challenges. Earth-Science Reviews 185 , 901–927 (2018).
Guhathakurta, P. Long-range monsoon rainfall prediction of 2005 for the districts and sub-division kerala with artificial neural network. Curr Sci 90 , 773–779 (2006).
Khan, N. et al . Performance assessment of general circulation model in simulating daily precipitation and temperature using multiple gridded datasets. Water 10 (12), 1793 (2018).
Gosain, A. K., Rao, S. & Basuray, D. Climate change impact assessment on hydrology of Indian river basins. Current science 90 (3), 346–353 (2006).
Download references
Acknowledgements
The authors of the article would like to thank Indian Meteorological Department for providing the rainfall data of all sub-divisions to conduct this work. The authors also acknowledge the Department of Geography, University of Gour Banga, Malda, West Bengal and Department of Geography and Jamia Milia Islamia, New Delhi for providing laboratory facilities and other supports to carry out the research.
Author information
Authors and affiliations.
School of Humanities and Social Sciences, Indian Institute of Technology Indore, Simrol, Indore, 453552, India
Bushra Praveen & Pritee Sharma
Department of Geography, University of Gour Banga, Malda, India
Swapan Talukdar, Susanta Mahato & Jayanta Mondal
Department of Geography, Faculty of Natural Sciences, Jamia Millia Islamia, New Delhi - 110 025, India
Shahfahad & Atiqur Rahman
Department of Disaster management, Begum Rokeya University, Rangpur, 5400, Bangladesh
Abu Reza Md. Towfiqul Islam
You can also search for this author in PubMed Google Scholar
Contributions
S.T. participated in the design of this study, and performed the statistical analysis, modeling and revise the manuscript. J.M. carried out data acquisition, data processing and some statistical analysis. A.R.M modeled cause of rainfall changes. Shahfahad wrote the initial draft, B.P. collected background information. S.T., Shahfahad, and S.M. carried out literature review. S.T., S.M., A.R.M., A.R., and Shahfahad reviewed and revised the manuscript. P.S. reviewed the manuscript. All authors have read and approved the content of the manuscript.
Corresponding author
Correspondence to Atiqur Rahman .
Ethics declarations
Competing interests.
The authors declare no competing interests.
Additional information
Publisher’s note Springer Nature remains neutral with regard to jurisdictional claims in published maps and institutional affiliations.
Supplemenatry information
Supplemenatry information., rights and permissions.
Open Access This article is licensed under a Creative Commons Attribution 4.0 International License, which permits use, sharing, adaptation, distribution and reproduction in any medium or format, as long as you give appropriate credit to the original author(s) and the source, provide a link to the Creative Commons license, and indicate if changes were made. The images or other third party material in this article are included in the article’s Creative Commons license, unless indicated otherwise in a credit line to the material. If material is not included in the article’s Creative Commons license and your intended use is not permitted by statutory regulation or exceeds the permitted use, you will need to obtain permission directly from the copyright holder. To view a copy of this license, visit http://creativecommons.org/licenses/by/4.0/ .
Reprints and permissions
About this article
Cite this article.
Praveen, B., Talukdar, S., Shahfahad et al. Analyzing trend and forecasting of rainfall changes in India using non-parametrical and machine learning approaches. Sci Rep 10 , 10342 (2020). https://doi.org/10.1038/s41598-020-67228-7
Download citation
Received : 10 March 2020
Accepted : 06 May 2020
Published : 25 June 2020
DOI : https://doi.org/10.1038/s41598-020-67228-7
Share this article
Anyone you share the following link with will be able to read this content:
Sorry, a shareable link is not currently available for this article.
Provided by the Springer Nature SharedIt content-sharing initiative
This article is cited by
Spatio-temporal epidemiology and associated indicators of covid-19 (wave-i and ii) in india.
- Karuppusamy Balasubramani
- Venkatesh Ravichandran
- Praveen Balabaskaran Nina
Scientific Reports (2024)
Long-term trends and spatial variability in rainfall in the southeast region of Bangladesh: implication for sustainable water resources management
- Hrithik Nath
- Sajal Kumar Adhikary
- Ahmed Ali A. Shohan
Theoretical and Applied Climatology (2024)
Linkages and reactions of geomorphic processes in Kerala Flood, 2018
- Satheesh Chothodi
- Sumesh Kuniyil
- Rahul Mishra
Natural Hazards (2024)
Times Series Forecasting of Monthly Rainfall using Seasonal Auto Regressive Integrated Moving Average with EXogenous Variables (SARIMAX) Model
- Shahenaz Mulla
- Chaitanya B. Pande
- Sudhir K. Singh
Water Resources Management (2024)
Modeling and forecasting rainfall patterns in India: a time series analysis with XGBoost algorithm
- Pradeep Mishra
- Abdullah Mohammad Ghazi Al Khatib
- Ramesh Yadav
Environmental Earth Sciences (2024)
By submitting a comment you agree to abide by our Terms and Community Guidelines . If you find something abusive or that does not comply with our terms or guidelines please flag it as inappropriate.
Quick links
- Explore articles by subject
- Guide to authors
- Editorial policies
Sign up for the Nature Briefing: Anthropocene newsletter — what matters in anthropocene research, free to your inbox weekly.


- Login To RMS System
- About JETIR URP
- About All Approval and Licence
- Conference/Special Issue Proposal
- Book and Dissertation/Thesis Publication
- How start New Journal & Software
- Best Papers Award
- Mission and Vision
- Reviewer Board
- Join JETIR URP
- Call For Paper
- Research Areas
- Publication Guidelines
- Sample Paper Format
- Submit Paper Online
- Processing Charges
- Hard Copy and DOI Charges
- Check Your Paper Status
- Current Issue
- Past Issues
- Special Issues
- Conference Proposal
- Recent Conference
- Published Thesis
Contact Us Click Here
Whatsapp contact click here, published in:.
Volume 5 Issue 12 December-2018 eISSN: 2349-5162
UGC and ISSN approved 7.95 impact factor UGC Approved Journal no 63975
Unique identifier.
Published Paper ID: JETIREC06132
Registration ID: 229604
Page Number
Post-publication.
- Downlaod eCertificate, Confirmation Letter
- editor board member
- JETIR front page
- Journal Back Page
- UGC Approval 14 June W.e.f of CARE List UGC Approved Journal no 63975
Share This Article
Important links:.
- Call for Paper
- Submit Manuscript online
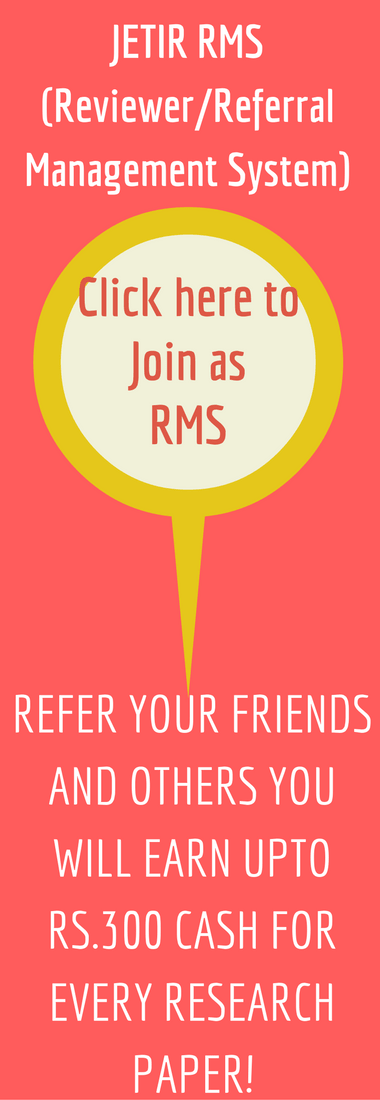
- Salam Suresh Singh
- Abhinay Thakur
- Ashish Kumar
Cite This Article
2349-5162 | Impact Factor 7.95 Calculate by Google Scholar An International Scholarly Open Access Journal, Peer-Reviewed, Refereed Journal Impact Factor 7.95 Calculate by Google Scholar and Semantic Scholar | AI-Powered Research Tool, Multidisciplinary, Monthly, Multilanguage Journal Indexing in All Major Database & Metadata, Citation Generator
Publication Details
Download paper / preview article.

Download Paper
Preview this article, download pdf, print this page.

Climate Change In Manipur In Context Of COP 26 Of UNFCCC
Resolution of the consultation on climate change in manipur in context of cop 26 of unfccc.
The participants of the consultation on “Climate Change in Manipur in context of COP 26 of UNFCCC”, organized by the Centre for Research and Advocacy, Manipur and the Youth Forum for Human Rights in Manipur at the Manipur Press Club on 8 November 2021, hereby:
Express deep concern with the worsening Climate Change impacts in Manipur: viz, record high temperatures, unpredictable flood, drought, diseases, crop failure, drying of water sources, biodiversity loss etc, that unleashed suffering, livelihood loss, displacement among indigenous communities.
Noted the Inter-Governmental Panel on Climate Change report of 2021 that reported alarming impacts of climate change, rapid temperature rise, increased in disasters, biodiversity loss, rapid sea level rises etc.
Alarmed with unsustainable development processes in Manipur amidst India’s neoliberal development targeting peoples’ land, forest, water and resources. Mega dams, mining, oil exploration, roads, railways, transmission lines pursued as part of India’s Act East Policy worsened land loss and climate change.
Express concern that large dams such as 66 MW Loktak Downstream Project, 190 MW Pabram Dam, 70 MW Nungleiband dam etc will destroy forest, agriculture land and threaten indigenous peoples livelihood, culture and identity while deepening climate crisis and impacts on indigenous peoples’ rights.
Concerned with increased land grabbing in mega infrastructure projects, Trans Asian Railway and Asian Highway projects, Imphal Town Ring Road etc, that will cause destroy agriculture land and forest areas.
Are you getting our free newsletter?
Considered with increased financing by the Asian Development Bank, World Bank, JICA etc in energy, infrastructure project with climate change and human rights implications.
Concerned with the push for palm oil and large dams in Manipur to increase forest cover and as renewable energy as part of India’s Intended Nationally Determined Contributions (INDC) to the UNFCCC.
Condemn the false climate change solutions pursued in Manipur and efforts of corporate bodies trying to seek profit by plundering natural resources of Manipur. The National Hydroelectric Power Corporation tried to seek carbon credits and profits from its controversial 105 MW Loktak Hydroelectric Project in Manipur from the CDM of UNFCCC despite violations.
Concerned that countries used the COP26 of UNFCCC to craft neoliberal policies through false climate solutions like Net Zero Emissions, carbon trades, carbon sequestration etc to respond to climate crisis. The COP 26 will reinforce business as usual for corporations to profit by exploiting nature and people.
Hereby Resolved that
1) The Government of India, corporations and international financial institutions should protect and promote indigenous peoples self-determined rights over their land and resources and should take their free, prior and informed consent before targeting their land and resources.
2) All MoUs for mega dams, oil exploration, mining, agri-business (viz. Palm Oil) pursued without their consent should be revoked for possible adverse impacts on environment, people and climate in Manipur. The MOUs granted to Roukela Private Ltd, Sarvesh Refractories, Gulf Natural Resources for mining and to Jubilant Oil and Gas Pvt Ltd and to Oil India Limited for oil exploration in Manipur should be revoked.
3) All neoliberal policies, such as Manipur Hydro Power Policy, 2012, North East hydrocarbon vision 2030, Manipur Loktak Lake Protection Act, 2006, the Mining and Minerals (Development and Regulation) Amendment Bill 2015 etc that facilitated the plunder of peoples’ land and resources should be repealed.
4) Dam building companies, viz, National Hydroelectric Power Corporation (NHPC) should stop building 66 MW Loktak Downstream Hydroelectric project and other dams that will destroy forest, agriculture land, river and peoples’ livelihood. All MoUs with NHPC and NEEPCO for dams should be revoked.
5) The Government should review and rescind its plan to promote palm oil in Manipur and North East given its documented adverse impacts on people, environment and climate in Mizoram, Indonesia, Malaysia etc.
6) The government should stop counting mega dams and palm oil as renewable energy and as solutions for climate change, in its effort to fulfil its INDC.
7) International Financial Institutions and corporates should stop financing unsustainable development projects and false climate solutions with social, environment and climate impacts. Climate finance should desist funding social and environmentally damaging projects.
8) We reject all false climate change solutions that violate indigenous peoples’ human right and their self-determination over their land and resources.
9) All climate change mitigation and adaptation related policies should ensure compliance with indigenous peoples’ rights as per UN Declaration on Indigenous Peoples Rights, 2007.
10) COP 26 of UNFCCC should desist from any decisions that serve corporate bodies interest for profiting by plundering peoples land and resources.
11) Indigenous peoples’ traditional sustainable management of land and natural resources, including role of women, youths etc and their low consumption and carbon oriented way of life must be promoted in all development decision making processes that can affect their land, lives and future.
12) The Manipur State Action Plan on Climate Change and other climate policies should be reviewed to ensure its compliance with human rights and sustainable development goals in its implementation.#
References: – Resolution: Climate Change in Manipur in Context of COP 26 of UNFCCC – Consultation on Climate Change in Manipur in Context of COP 26 – Revoke MoUs for mega dams, oil exploration, mining, agri-business in Manipur

Did you know Scoop has an Ethical Paywall?
If you're using Scoop for work, your organisation needs to pay a small license fee with Scoop Pro. We think that's fair, because your organisation is benefiting from using our news resources. In return, we'll also give your team access to pro news tools and keep Scoop free for personal use, because public access to news is important! Go to Scoop Pro Find out more
UN News: Green Light For New Cholera Vaccine, Ukraine Attacks Condemned, Action Against Racism
A new oral vaccine for cholera has been given the green light for manufacture by the UN health agency allowing for the massive scale-up of lifesaving immunisation in the world’s most vulnerable communities.
Laureus: Grand Slam Champion Garbiñe Muguruza Announces Retirement Ahead Of Laureus World Sports Awards
The 30-year-old initially stepped back from tournament tennis in 2023 and ahead of the Laureus World Sports Awards – taking place in Madrid on Monday, April 22 – officially ended her competitive career and revealed that her future will include a role as a Laureus Ambassador.
Carbon Market Watch: Going For Green - Is The Paris Olympics Winning The Race Against The Climate Clock?
The 2024 Paris Olympics promises a gold-winning climate performance and to set the pace for future games. Our in-depth assessment reveals that, despite improvement, the carbon footprint of the Olympics remains far too high to be sustainable. This calls for a radical rethinking of the games.
New Zealand Defence Force: NZDF Working With Pacific Neighbours To Support Solomon Islands Election
A New Zealand Defence Force (NZDF) contingent of more than 200 personnel has been providing logistical support to Solomon Islands, on behalf of the Ministry of Foreign Affairs and Trade, as the country geared up for today’s general election.
UN News: Ceasefire The Only Way To End Killing And Injuring Of Children In Gaza
Advocating for a ceasefire is the best way to support the people of Gaza, including children in the north who are dying of hunger, a Spokesperson for the UN Children’s Fund (UNICEF) said on Thursday.
ICHRP: US-Japan-Philippines Trilateral Summit Makes The Philippines A Battlefield For US-China Conflict
The “Independence” of the Philippines in the immediate postwar period institutionalized US military, economic and political control through unequal treaties and trade agreements.
LATEST HEADLINES
- European Union Funds Film Raro2 To Save Culture In The Pacific. 2:17 PM | Film Raro2
- Reverse The Massive Increase In World Military Spending 12:52 PM | Sydney Anti-AUKUS Coaliti...
- The False Diversity Of Identity, Part Two 10:26 AM | Martin LeFevre - Meditations
- International Workers’ Memorial Day 2024: Action For Climate-Related Workplace H... 7:52 AM
- Telling The ‘Untold’ Stories Of Palestinian Lives, Dreams, And Hopes—in Gaza And... 5:36 AM
- South Korea’s Election Outcome: Opposition Party Triumphs, President Yoon Faces ... 5:08 AM
- Grand Slam Champion Garbiñe Muguruza Announces Retirement Ahead Of Laureus World... 21/04/24
- Pacific.Scoop
- Cafe Pacific
- Three Strikes Targets Those ‘too Brown To Be White... 4:20 PM | admin
- European Union Funds Film Raro2 To Save Culture In... 2:17 PM | admin
- New Diplomatic Appointments 1:11 PM | admin
- NZDF Māori Cultural Group Helps Samoan Christchurc... 11:52 AM | admin
- Have Your Say On The Restoring Citizenship Removed... 18 Apr | admin
- Palestine’s Status At The UN Explained 18 Apr | admin
- New Zealanders Flock To Fiji: Figures Reveal Highe... 18 Apr | admin
- Café Pacific blog has now expanded into a new ind... | cafe pacific
- The 'death' of journalism â may its memory be a ... | cafe pacific
- Two countries, two kidnappings â Port Moresby sh... | cafe pacific
- West Papuan cat-and-mouse over NZ pilot taken capt... | cafe pacific
- Papuan journalist award-winner Victor Mambor targe... | cafe pacific
- Fijiâs media veterans recount intimidation under... | cafe pacific
- What the resignation of New Zealand's inspirationa... | cafe pacific
- VIDEO: BBC refuses apology to Fiji after documenta... | niusedita
- AUDIO: Ex-detective calls on Fiji police to speed ... | niusedita
- Auckland Polyfest opens out to all cultures | niusedita
- Pasting content into a rich text editor field | david
- Pacific Media Centre style guide | Tony Murrow
- Toktok Issue 17 (Winter): Key speakers lining up f... | david
- Malcolm Evans cartoons | david
World Section
- Oilprice.com
- Democracy Now
- Google News
- Guardian Newspaper
- The Independent
- Washington Post
- New York Times
- The Huffington Post
- Council on Hemispheric Affairs
- Green Peace
Other Links
- Powershop.co.nz: Electricity Supplier - Electricity Prices
- GPS tracking
- Property Management South Auckland
- NZ On Screen: NZ Television - NZ Film - NZ Documentary
Scoop Newsagent
- Media Monitoring
Scoop Techlab Smartphone Reviews :
- Nokia Lumia 920 Smartphone - Nokia Lumia 920
- Samsung Galaxy Note II a.k.a. Galaxy Note 2
Join Our Free Newsletter
- Women’s Resilience to Disasters (WRD) Programme
- Policy Tracker
- WRD Community of Practice
- WRD Expert Register
- Recommended Platforms
Accessibility menu
Content for the offcanvas goes here. You can place just about any Bootstrap component or custom elements here.
India: In rural Manipur, women feel the heat of climate change
By Ninglun Hanghai
Women farmers in Manipur are struggling to grow crops in an increasingly hostile environment in north-east India
It is that time of the year when the weather is dry and windy. Hmuoki has to work even harder than usual to water and fertilise her four acres of farmland on the banks of the Khuga River in Churachandpur district of Manipur, north-east India.
Hmuoki struggles to ensure her family has enough food to eat during the dry season, which begins in November. From November till March, she plants crops like mustard, pumpkin, varieties of peas, beans, ginger and turmeric. She also supplements her food by working on farms in the hills surrounding the village, by going to Jhum fields or to collect wild fruits or herbs.
Women like Hmuoki play a major role in agriculture in Manipur. There are a large number of women farmers working in the state, suffering from protracted conflictbetween authorities and armed groups. These women are also on the front line of climate change in the north-eastern state, where erratic rainfall, floods and higher temperatures are making daily life more difficult for rural farmers.
In mid February, a small group of women farmers gathered at Hmuoki’s home with a team from the civil society organisation Rural Women Upliftment Society (RWUS) to talk about their experiences in the fields and the changes they have witnessed in recent years. Hmuoki lives in Saidan village, 5 kilmetres from Lamka, the main town in the district.
February is the time when women prepare for Jhum cultivation – a traditional slash and burn agriculture technique used widely in the hills of Manipur. The women are also seeing more random forest fires, set off by people who want to hunt wild animals. This causes huge damage to the land. Burning for Jhum preparation, however, does not damage soil fertility or cause environment degradation, argued the women. For Jhum preparation a small forest area is cleared, and twigs, branches and waste from the clearings are dried and eventually burned. The ash actually helps the make the soil more fertile.
Forest fires also affect their water sources – natural springs, which are slowly shrinking and drying up.
For many years now certain areas in the forest surrounding the village have been declared a “forest reserve” by the village authority (under the Chief) to preserve its resources, mostly for fuel and wood. “Due to this we had to even go farther for our Jhum cultivation,” said Thiengi, a woman who dropped in to the meeting on her way to the Jhum fields. “Now our Jhum cultivation field is very far from our village,” she said. There is no time to rest, as she goes to Jhum fields about an hour’s walk away, to get herbs and other edible leaves and plants.
Too little, too much water
The women dread the summer when water will be really scarce. Saidan village, with over 100 households, has three public water reservoirs, but the women say this is insufficient. Rainfall, the main source of water for domestic use, has become sporadic and this sometimes leads to drought.
The women have also seen more frequent floods over the past decade. Floods become a major hazard during the monsoon. “My field/ farm was frequently destroyed by flood, damaging all crops in monsoon,” said Hmuoki. This is one reason why she works persistently during the winter months, struggling hard to keep her farm in good condition, so as to extend the crop-growing season.
Even if there is no heavy rain, water released from behind the Khuga dam sometimes inundates the whole river downstream, damaging crops, and houses around the river. Khuga Dam is a multipurpose hydroelectric project located in south Churachandpur. During the monsoon, water is released from behind the dam to relieve pressure. The dam was completed in 2010.
Water for domestic use and for agriculture and irrigation is a key concern. The burden of collecting water falls on women. Besides the drying of springs, one of the major causes of water scarcity is the lack of snowfall. “Ten or twenty years back we had snowfall in winter. There is no snowfall now,” explained Hmuoki. “Snowmelt makes the soil wet and fertile”.
Therefore women farmers like Hmuoki have to put even more effort into raising their crops, which is their main livelihood. At the moment, her farm is on the banks of the Khuga River, which provides irrigation. But Hmuoki is worried. The Lanva, a tributary of the Khuga that runs through Lamka town, has completely dried up during winter – though it still floods causing havoc during the monsoon.
Feeling the heat
The summer months are increasingly hot, said the women from Saidan village. February, once upon a time a cool, breezy month, was already warm and sultry this year. Though rural villages in Churachandpur have to bear the summer heat without amenities, in Lamka ceiling fans and standing fans have become a necessity. In peak summer, temperatures in Churachandpur have reached over 30 degrees Celsius in recent years. Record temperatures in Manipur reached 35.6 degrees Celsius in April 2014. Many families in Manipur have also fitted air conditioners at home. These changes have been distinct in the past few years, compared to the usually pleasant and moderate climatic conditions in Manipur.
Rising temperatures have made it more difficult to preserve seeds. Traditionally, seeds were preserved by drying them out in the sun or above fireplaces and keeping them in bamboo containers. In past generations, seeds would be planted directly in the soil. Now seeds have to be germinated first with manure and water in nurseries at home before being planted in the soil, to ensure crops grow well.
The hard work doesn’t end there. Once the crops grow, they come under attack from a growing number of insects and other pests. “These days we buy and use pesticides and fertilizers, our homemade manure is not sufficient,” said Hmuoki.
It’s not only crops. Mangoes, one of the common fruit plants in Churachandpur, for instance, are vulnerable to infection, said Thiengi. “Crops like peas are impossible to grow now without insecticides and pesticides,” she said.
Plants and fruits that were once delicacies for the locals have slowly disappeared. One example is the zawngtah or “stink bean” (Parkia speciosa). The bean trees have slowly dried up and been infected by pests and the price of the beans has gone up in recent years. The plant used to grow in the wild as well as being cultivated. Now the beans have to be imported from Moreh, a town and trade hub 270 kilometres away from Lamka on the Indian-Myanmar border.
Uphill struggle
Farming has become a real struggle for these women in Manipur. They want to take advantage of recent developments in technology and modern agricultural techniques to boost their productivity and experiment new ways of farming. They also hope to find alternative ways to earn income. For instance, introducing new varieties of climate-resistant seeds, or new varieties of crops, such as pulses.
It is rural women, particularly farmers, who see and experience climate change, said Mary Beth Sanate, secretary of the Rural Women Upliftment Society. They are the ones who are preserving natural resources, but unfortunately, policymakers do not consider their knowledge and skills. “Women are not part of the decision making bodies, be it at the local level, or in traditional or state bodies,” said Sanate.
Creative Commons BY-ND 2.0
Read the original story here
Related News
Empowering resilience: wrd and partners forge inclusive disaster risk reduction strategies in the pacific.
Civil society partners, women’s organizations and organizations for people with disabilities came together for a Talanoa Session on Gender, Disability and Social Inclusion in Disaster Risk Reduction, affirming resilience building that leaves no one behind.
Latinas for Climate: meet the young Latinas championing climate justice
As climate-related disasters escalate in frequency and intensity, Latinas for Climate has emerged as a beacon of hope for young Latinas to elevate their voices to influence climate justice at the country, regional and global levels and make a meaningful impact.
Accelerating action for gender responsive disaster risk reduction
At a time of growing risks and vulnerabilities, UN Women recognizes the urgent need to optimize the work of the next seven years of the Sendai Framework’s implementation to achieve gender-responsive disaster risk reduction.
The Women's Resilience to Disasters programme launches in the Solomon Islands
A new programme to bolster women’s resilience and leadership for sustainable, secure and thriving communities in the Solomon Islands, in the face of disasters, was launched today.
ED's Speech: Accelerating action for gender-responsive disaster risk reduction
Opening speech by UN Women Executive Director Sima Bahous to the Risk Reduction Hub, a series of meetings held on the margins of the High-Level Meeting on the Midterm Review of the Sendai Framework for Disaster Risk Reduction, 17 May 2023.
UN Women and the Youth Leaders and Young Professionals (YLYP) Network host event highlighting the role of youth and digital technology for women’s resilience
In the lead up to CSW67, youth activists advanced their key asks related to digital technology to build women's and girls' resilience to disasters
Three asks on gender equality to COP27
On the Gender Day at COP27, the Executive Director of UN Women lays out what the UN Climate Conference must deliver to advance gender equality.
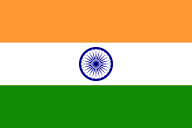
Manipur weather in April
Planning a journey to Manipur this April and curious over the weather conditions to await? Your essential details are found on this page, answering your query:
What is the weather like in Manipur in April?
Manipur is a region in India . April in Manipur generally has warm temperatures, with moderate precipitation.
You can expect warm maximum daytime temperatures of around 28°C and nighttime temperatures around 17°C in Imphal .
April is a moderate rainy month with an average rainfall of around 90 mm in Imphal .
To learn more about the conditions in specific areas of Manipur in April, check out the map below. For more details on the yearly weather, visit our Manipur climate page .
Where to go in Manipur in April
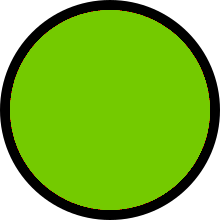
Popular destinations in Manipur
What is the weather like in April for the most popular destinonation in Manipur?
Average weather in Imphal
In April Imphal generally has high temperatures with maximum daytime temperatures around 28°C , minimum nighttime temperatures around 17°C and moderate monthly rainfall. So on average the conditions are perfect that month.
Is your destination not in the list? Have a look at other destinations in Manipur , in India .
Is April the best time to visit Manipur?
We believe that April is one of the best times to visit Manipur with overall perfect weather in Imphal . However, the weather conditions may vary depending on your destination.
On average, the best time to visit Manipur is in January , February , March , April , November and December with generally perfect weather in Imphal . In contrast, June and July tend to have poor weather conditions.
Interested in more climate information?
- January
- February
- March
- April
- May
- June
- July
- August
- September
- October
- November
- December
Weather and climate has detailed information for every country in the world. Are you going to India? Get detailed historical weather averages for many cities in India .
Not sure yet where to go? We now have a tool which recommends destinations that suit your ideal climate conditions. Find out where to go with our weather planner .

ICAR RESEARCH DATA REPOSITORY FOR KNOWLEDGE MANAGEMENT (An Institutional Publication and Data Inventory Repository)
- KRISHI Publication and Data Inventory Repository
- Natural Resource Management A8
- ICAR-Research Complex for NEH Region N2
- NRM-RCNR-Publication
Items in KRISHI are protected by copyright, with all rights reserved, unless otherwise indicated.

Weather in Manipur, Manipur, India
Partly sunny.
Feels Like: 36 °C Forecast: 32 / 18 °C Wind: 4 km/h ↑ from Northwest
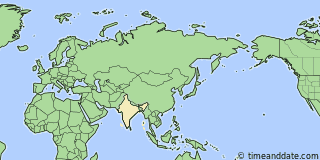
Upcoming 5 hours
See more hour-by-hour weather
Forecast for the next 48 hours
14 day forecast, day-by-day Hour-by-hour forecast for next week
Yesterday's weather
Fog. 30 / 20 °C Humidity: 82%. Wind: 2 km/h ↑ from Northwest
More weather last week
Currently at nearby stations
Sylhet: (214 km).
Scattered clouds. (1 hour ago)
Tezpur: (217 km)
North lakhimpur: (249 km).
Fog. (1 hour ago)
More weather in India
Forecast for the next 2 weeks
Detailed forecast for 14 days
Need some help?
ENVIS Centre, Ministry of Environment, Forest & Climate Change, Govt. of India
Agriculture.
Agriculture is the main occupation of the people of Manipur. Agriculture sector contributes a major share to the total state domestic product and provides employment to about 22.13 percent (according to 2011 census) of the total workers in Manipur. Out of the total geographical area of the state, only 7.41 percent is used for cultivation. Of this total cultivated area, 52% is confined to the valley. Therefore, half of the total valley area, which accommodates 67% of the total population, is occupied for agriculture purposes. Thus, the pressure on land in the valley is quite conspicuous.
The agriculture practices in the state can be broadly categorized into two distinct types, viz., settled farming practiced in the plains, valleys, foothills, terraced slopes, etc. and shifting cultivation(Jhum) practiced on the hill slopes. The shifting cultivation leads to possible forest degradation in the foot hills and reduc the total sink potential across the state.
There are 18 (eighteen) main crops which are cultivated during the two seasons in the state. Rice cultivation dominates all others crops. With the increase of the population, the requirement or demand of all crops has been increased tremendously. However the production of the crops has been decreased. Therefore there has been the great requirement of adaptation of the high yielding varieties of seed over the traditional seeds. The adoption of high-yielding varieties (HYV) of different crops except paddy has been very slow in Manipur, especially in the hill districts.
Some of the commercial crops grown in Manipur are cotton, kabrangchak, oilseeds and sugarcane which is very essential for enhacing the growth of agro-based industries in the state of Manipur.
Common horticulture crops grown in the State are Kharif vegetables (French bean, Cucurbits, Tomatoes, Brinjal, Bhindi, Colocecia, Alocacia), Rabi vegetables (Cabbage, Cauliflower, Potato, Pea, Broad bean, Radish, Carrot, Broccoli, lettuce, Capsicum), Spices (Onion, Garlic, Chilli, Ginger, Turmeric, Hatkora) Fruits and Plantation Crops (Litchi, Cashew nuts, Wall nuts, Orange, Lemon, Banana, Pineapple, Passion fruit, Peach, Pear, Plum). The state has the scope and potential to grow various horticulral crops because of varied agro- climatic condition.
The main categories of livestock reared in Manipur are cattle, buffalo, sheep, goat, pig, etc. Cattles and buffaloes provide motive power in wet cultivation.Rearing of pigs and poultry farming are found to be very important sources of income generating activities. The farmers in the state perform livestock rearing with a view of sustainable development & self sufficiency in livestock products. But there is still a huge gap between demand and supply of these livestock products in the state.
Due to the irregularity and erratic behaviour of monsoon accompanied by inadequate irrigation facilities have resulted in severe fluctuations in agricultural production. Agriculture plays a very crucial role in the state’s economy.
Pattern of Land Utilisation :
Due to un cadastrally surveyed of Hill areas, the Land Utilisation statistics for the entire State of Manipur are not available. The plains of Manipur occupies about 2,238 sq. kms. which accounts for about 10 percent of the total geographical area. As per the land utilization statistics of 2010-11, out of the total geographical area, the reporting land available for agriculture is about 231.19 sq. km.The total crop area of Manipur during 2012-13 are 239.43 hectares. The land use classification (Total crop Area) of Manipur for the year 2011-12 to 2012-13 are presented below:
Table: Land use Classification (Total Crop Area) of Manipur, 2011-12 to 2012-13
Area in ‘000 hectares
Source: Department of Agriculture, Manipur
Agricultural Production :
Permanent cultivation is generally practised in the valley districts, while terrace cultivation is practised in some of the hills where jhuming or shifting cultivation is widely adopted in most of the hill districts. Rice is the staple food of Manipur and is grown in both the hill and plain areas. Cultivation is almost entirely mono-crop with rice accounting about 98 percent of food-grains production and about 72 percent of the total cropped area is grown with paddy. The following table presents the districtwise area, production and average yield of cereal crops in Manipur during 2014-15:
Table: Districtwise Area, Production and Average yield of cereal crops in Manipur, during 2014-15
Area:’000 hectares
Yield rate: kg./hectares
Production:’000 tonnes
Usage of Fertilizer and Pesticide :
Chemical fertilizers play an important role in increasing the agricultural production particularly when used with the high yielding varieties which are responsive to recommended doses of fertilizers. The increase in agricultural production was possible as a result of adoption of quality seeds, appropriate doses of fertilizers and plant protection chemicals, coupled with assured irrigation. The improved and certified seeds have been the catalyst for making inputs cost effective. The fertilizer consumption was 59.05 thousand tonnes in 2003- 04 and it decreased to 17.12 thousand tonnes in 2011-12 and 23.40 thousand tonnes in 2012-13. The trend in the consumption of fertilizers and pesticides can be seen from the following Tables:
Table: Consumption of Fertilizers in Manipur during 2002-03 to 2012-13
(in ‘000 tonnes)
- Not available
Source : Agriculture Department, Govt. of Manipur
Table: List of the pesticide consumption in Manipur are given below:
Source: Directorate of Agriculture Govt. of Manipur
Fishery Resources in Manipur:
Fish is the most important item and easily digestible protein food of the Manipuri. It is the only source of animal protein acceptable to all the people of the state..The State has vast potential of fisheries resources such as ponds, tanks, natural lakes, marshy areas, swampy areas, rivers, submerged cropped land, low lying paddy fields etc. The largest source of fish is the Loktak Lake.The swamp areas can be profitably utilized for culture of various indigenous natural fishes like Ukabi(Anabas tesdudineus), Ngamu(Lata fish), Ngaton(Labeo bata),Ngakrijou(Lepidodephalichthys SPP), Sareng Khoibi(Botia SPP), Nganap (Pengia SPP), Ngatin (Labeo Pangusia), Ngakra (Barbus tor), Ngasang (Esomus denricus), Phabounga (Puntius SPP), Ngamhai (Chanda SPP), Pengba (Osteobrama belangeri) etc. Event though many trained fish farmers are producing large nos. of fishes, the State still requires large nos. of fishes. So, a large quantities of fishes are being imported from outside the state every year to fill the gaps.
Effect on agriculture due to climate change:
Agricultural production is highly sensitive to climate change and weather variability. Extreme weather events such as droughts, floods, tropical cyclones, heavy precipitation events, heat and cold waves significant negative impacts on agricultural production and livelihood of the farmers as agriculture forms the backbone of state economy.The main source of water for agriculture is rain water. The productivity of agriculture are largely dependent in the rainfall because the irrigation system in Manipur is not fully developed.

Manipur Weather And Best Time To Visit Manipur
The pride of northeast India , Manipur is a state filled with infinite natural beauty, rich vibrant culture and overall, the most ideal tourist destination for every kind of traveller. The name of the state literally translates to ‘jewelled state’; a befitting moniker for a place so exquisite. Famous gems like the Loktak Lake and the Ima Keithel women’s market have helped strengthen Manipur’s identity as a top holiday spot that flaunts the best of both worlds in terms of natural wonders and cultural heritage. While the weather of Manipur rarely gets too harsh for comfort, the early winter months are usually considered the most welcoming time of the year. Read on to find out the weather conditions and the best time to visit Manipur.
Winter (October - March)

Winters are, by far, the best time to visit Manipur. It does get quite cold and the temperature can go as low as zero degrees, especially during the coldest month of January. But the snow-covered peaks of the hilly regions and the chilly yet cosy climate will definitely make your trip worthwhile. The entire ambience of Manipur is laid-back during these months, and under the winter sun, the afternoons often get warm enough to shed your heavy winter-gear and enjoy the local hotspots of the region. The skies remain mostly clear, but the weather can occasionally get foggy as well.
Summer (April - July)

Summers in Manipur are not scorching like those in the rest of northern India . However, the afternoons can often get hot and humid most days, with the average temperature ranging between 16⁰C to 36⁰C during the daytime. The early morning and late evening hours are considered the most pleasant times of the day, with the climate remaining sunny and clear during the afternoons. The lush green beauty of Manipur is scintillating during these months, and city excursions and sightseeing tours can be very enjoyable on good weather days. All in all, early summers can be a wonderful time to visit Manipur.
Monsoon (June - September)

The monsoon months in Manipur are associated with moderate rainfall, which is somewhere around 1500 mm annually. Tourists usually prefer to avoid this season for holiday trips though, mainly due to the unpredictability of the weather and travel connectivity issues. Those who love rainy weather may still be interested in visiting Manipur during these months; the vibrant beauty of the region that comes alive after the rain is sure to be irresistible to anyone who gets to witness it. The temperature hovers around a range of 23-30⁰C, with June being the rainiest month of the season.

Before You Go... Got Questions?
Find the best manipur packages from top travel agents.
Manipur, Haryana
Around the globe, hurricane tracker.
Severe Weather
Radar & Maps
News & features, today's weather.
Mon, Apr 22
Sunny to partly cloudy and very warm Hi: 99°
Tonight: Mainly clear Lo: 68°
Current Weather
Manipur weather radar & maps.
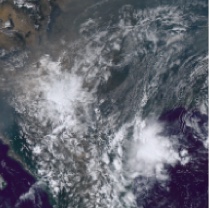
Hourly Weather
10-day weather forecast.
Mostly sunny and very warm
Night: Mainly clear
Mostly cloudy and very warm
Sunny and very warm
Very hot with some sun
Partly cloudy
Partly sunny and very warm
Mostly sunny and very hot
A couple of showers
Mainly clear
Sunny and very hot
Sun & Moon
Air quality.
The air quality is generally acceptable for most individuals. However, sensitive groups may experience minor to moderate symptoms from long-term exposure.
Allergy Outlook
Top Stories
Winter Weather
Frosty mornings will mean trouble for plants in the Midwest and East
55 minutes ago

Multiday severe storm, tornado risk to unfold in central US late week

Meteor shower drought to end as Lyrids peak this weekend

Weather News
Maui wildfire report: Wind-swept smoke trapped residents

Weather Forecasts
Warmest weather since 2023 to build over Southwest into this week

Featured Stories
AccuWeather Ready
Top 3 tips on lightning safety from a leading safety expert

Some bumblebees can survive underwater for up to a week, study shows

More than 100 killed across Pakistan and Afghanistan as flash floods a...

Camels evolved from a cold-weather ancestor

5 ways the Columbia disaster changed spaceflight forever

Weather Near Manipur:
- Chhachhrauli , Haryana
- Sadaura , Haryana
- Yamunanagar , Haryana
We have updated our Privacy Policy and Cookie Policy .
Get AccuWeather alerts as they happen with our browser notifications.
Notifications Enabled
Thanks! We’ll keep you informed.

COMMENTS
74 °F. 21:30. Manipur. History & Climate. Climate (modelled) meteoblue Ad-free 9 €. The meteoblue climate diagrams are based on 30 years of hourly weather model simulations and available for every place on Earth. They give good indications of typical climate patterns and expected conditions (temperature, precipitation, sunshine and wind ...
Climate & Weather Averages in Manipur, Manipur, India. Weather Today Weather Hourly 14 Day Forecast Yesterday/Past Weather Climate (Averages) Currently: 74 °F. Fog. (Weather station: Imphal Tulihal, India). See more current weather.
Weather MIS of 9 (nine) districts of Manipur. ENVIS Centre, Manipur has developed and maintaining a database managemnet system of Weather MIS of 9 (nine) districts of Manipur. - This Weather MIS provide daily, monthly and yearly district level climate data for some basic parameters viz. Max/Min Temp, Total Rainfall, Max/Min Relative Humidity ...
Title: 15.Analytical Report on Weather Data for the state of Manipur during January 2023 ,DoE&CC,IMPHAL-EIACP,MANIPUR Year of Publication: 2023 Month of Issue: April Published by: DoE&CC, Imphal-EIACP, Manipur Printed Year: 2023 Reference: Indian State Level Basic Environment Information Database (ISBEID) Details: Analytical Report on Weather Data for the state of Manipur during January 2023 ...
This group contain data of Manipur state. Weather & Climate. Data and Resources. Manipur Temperature Map 2021 wms. View in Geoportal. Field Value; ... Weather & Climate. Purpose of Creating Data: To provide information on monthly temperature in district-wise. Access Constraints:
Study Area and data source. The geographical location of India is between 8.4° and 37.6°N latitude and 68.7° and 97.25°E longitude. The whole India can be categorized into four homogenous ...
Climate change is a global phenomenon and one of the burning issues in the present scenario. This article provides a description of the impacts of climate change on Manipur's agricultural, woodland, and wetland ecosystems; a north-eastern Indian state. Some major findings in the correlation with the effects of climate change on different ecosystems have been discussed however the more ...
Climate change is becoming top threat to biodiversity and it is the fastest-growing cause of species loss in the world. The rising sea level and increased extreme weather events of climate change---fires, floods, pestilence and drought---have already caused widespread harm to biodiversity. India has continuously demonstrated its responsibility towards acknowledging the emerging threats from ...
22.2 Climate Profile of The Manipur 15 2.2.1 Data Used 15 ... 3.5 Climate Change Impact on Water Resources in Manipur 29 3.6 Climate Change Impact on Forests & Its Ecosystem and Biodiversity 30 ... drought, flood, severe weather events sea-level rise etc. are likely to result in food shortage, increase in vector-borne diseases, ...
The participants of the consultation on "Climate Change in Manipur in context of COP 26 of UNFCCC", organized by the Centre for Research and Advocacy, Manipur and the Youth Forum for Human ...
level. It has sub-tropical temperate climate. The rainfall of Manipur in 2019, as recorded by ICAR, Lamphelpat is 1137.1 mm. The State has a salubrious climate. 1.1 Manipur, though tiny in size, has a distinct history of its own. The recorded history of Manipur can be obtained from the State Royal chronicle called the Cheitharol Kumpaba
Record temperatures in Manipur reached 35.6 degrees Celsius in April 2014. Many families in Manipur have also fitted air conditioners at home. These changes have been distinct in the past few years, compared to the usually pleasant and moderate climatic conditions in Manipur.
ENVIS Centre, Manipur has developed and maintaining a database managemnet system of Weather MIS of 9(nine) districts of Manipur. - This Weather MIS provide daily, monthly and yearly district level climate data for some basic parameters viz. Max/Min Temp, Total Rainfall, Max/Min Relative Humidity, Wind speed, wind direction, solar radiation ...
Manipur is a region in India . April in Manipur generally has warm temperatures, with moderate precipitation. You can expect warm maximum daytime temperatures of around 28°C and nighttime temperatures around 17°C in Imphal . April is a moderate rainy month with an average rainfall of around 90 mm in Imphal . To learn more about the conditions ...
Manipur has seen maximum net warming during winter. A new analysis from Climate Central, a US-based organisation of climate scientists and researchers, explains how temperatures in February have ...
About 80% of the state population depends on agriculture for livelihood. However, agriculture sector in Manipur is facing the consequences of climate change. Climate change is a reality and an increasing trend in temperature, precipitation and emission of greenhouse gases has been observed in Manipur.
Manipur 14 Day Extended Forecast. Weather Today Weather Hourly 14 Day Forecast Yesterday/Past Weather Climate (Averages) Currently: 82 °F. Passing clouds. (Weather station: Imphal Tulihal, India).
Weather in Manipur, Manipur, India. Time/General; Weather . Weather Today/Tomorrow ; Hour-by-Hour Forecast ; 14 Day Forecast ; Yesterday/Past Weather; Climate (Averages) ... Weather Today Weather Hourly 14 Day Forecast Yesterday/Past Weather Climate (Averages) Now. 84 °F. Haze. Feels Like: 91 °F Forecast: 88 / 61 °F Wind: 3 mph ...
Table: Consumption of Fertilizers in Manipur during 2002-03 to 2012-13 ... Agricultural production is highly sensitive to climate change and weather variability. Extreme weather events such as droughts, floods, tropical cyclones, heavy precipitation events, heat and cold waves significant negative impacts on agricultural production and ...
Winters are, by far, the best time to visit Manipur. It does get quite cold and the temperature can go as low as zero degrees, especially during the coldest month of January. But the snow-covered peaks of the hilly regions and the chilly yet cosy climate will definitely make your trip worthwhile. The entire ambience of Manipur is laid-back ...
In addition to having a sub-humid climate and a lot of rainfall (1600 mm annually), Manipur has geological formations that are prone to landslides and a topography that is generally rocky.
Current Weather. 5:06 AM. 77° F. RealFeel® 82°. Air Quality Fair. Wind ENE 6 mph. Wind Gusts 15 mph. Partly cloudy More Details.
This group contain data of Manipur state. Data Extent . Weather & Climate. Data and Resources. Manipur Temperature Map 2014 wms. View in Geoportal. Field Value; ... Weather & Climate. Purpose of Creating Data: To provide information on monthly temperature in district-wise. Access Constraints: