Taking a systems thinking approach to problem solving
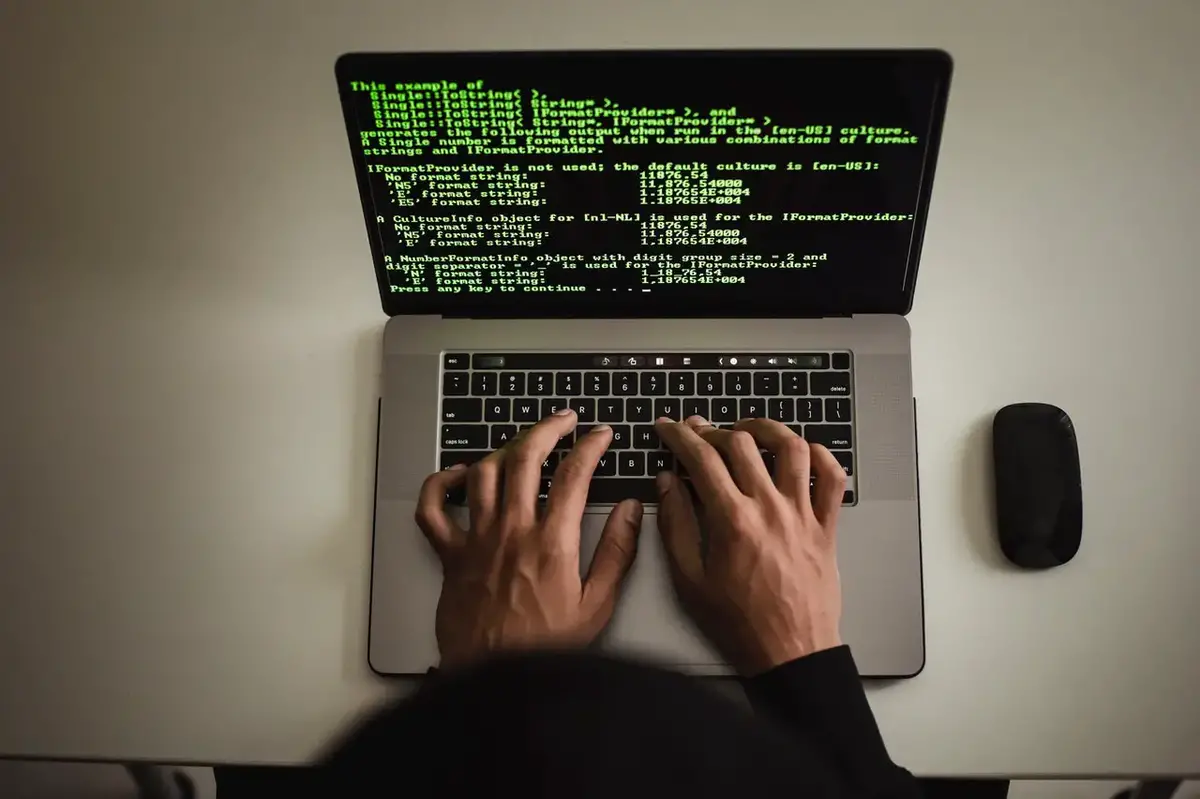
Systems thinking is an approach that considers a situation or problem holistically and as part of an overall system which is more than the sum of its parts. Taking the big picture perspective, and looking more deeply at underpinnings, systems thinking seeks and offers long-term and fundamental solutions rather than quick fixes and surface change.
A systems thinking approach might be the ideal way to tackle essentially systemic problems. Our article sets out the basic concepts and ideas.

What is systems thinking?
When we consider the concepts of a car, or a human being we are using a systems thinking perspective. A car is not just a collection of nuts, bolts, panels and wheels. A human being is not simply an assembly of bones, muscles, organs and blood.
The history of systems thinking is itself innately complex, with roots in many important disciplines of the 20th century including biology, computing and data science. As a discipline, systems thinking is still evolving today.
How can systems thinking be applied to problem solving?
A popular way of applying a systems thinking lens is to examine the issue from multiple perspectives, zooming out from single and visible elements to the bigger and broader picture (e.g. via considering individual events, and then the patterns, structures and mental models which give rise to them).
Systems thinking is best applied in fields where problems and solutions are both high in complexity. There are a number of characteristics that can make an issue particularly compatible with a systems thinking approach:
Areas where systems thinking is often useful include health, climate change, urban planning, transport or ecology.
What is an example of a systems thinking approach to problem solving?
Beneath the waterline and invisible, lie deeper and longer-term trends or patterns of behavior. In our example this might be internal fighting in the political party which overshadows and obstructs its public campaigning and weakens its leadership and reputation.
The electoral system in the country may also be problematic or unfair, making the party so fearful and defensive against losing its remaining support base, that it has no energy or cash to campaign on a more positive agenda and win new voters.
Mental models
At the very base of the iceberg, deepest under the water, lie the mental models that allow the rest of the iceberg to persist in this shape. These include the assumptions, attitudes, beliefs and motivations which drive the behaviors, patterns and events seen further up in the iceberg.
When is a systems thinking approach not helpful?
If you are looking for a quick answer to a simple question, or an immediate response to a single event, then systems thinking may overcomplicate the process of solving your problem and provide you with more information than is helpful, and in slower time than you need.
A final word…
The biggest problems in the real world are rarely simple in nature and expecting a quick and simple solution to something like climate change or cancer would be naive.
Whether you think of it as zooming out to the big picture while retaining a focus on the small, or looking deeper under the water at the full shape of the iceberg, systems thinking can be a powerful tool for finding solutions that recognize the interactions and interdependence of individual elements in the real world.
You may also like
Systems thinking vs. linear thinking: understanding the key differences, systems thinking for school leaders: a comprehensive approach to educational management, what is systems thinking, 5 ways to apply systems thinking to your business operations: a strategic guide, download this free ebook.
- Increase Font Size
10 Systems Approach to Problem Solving
Dr. Ashish Saihjpal
1. Learning Outcome:
After completing this module the students will be able to:
- Understand the concept of a system.
- Systems Approach to Problem Solving
- Understand how Systems Approach can be applied to Information Systems.
- Understand the concept of Systems Approach with practical examples.
2. Introduction
Today’s problems cannot be solved by the same level of thinking that once created them. ‑Albert Einstein
This statement by Einstein has put forward a strong argument for the need of such an approach that makes us to address a problem as a whole rather than breaking it into parts. Prima Facie, we need to know what exactly we mean by problem in any business organization. Defining a problem depends on its objectives. This can be explained with the help of an example. If a person has a headache, he will need a medicine. However, if he has no medicine at home then it becomes a problem. In the opposite case, if a person is not having any headache then the non-availability of the medicine doesn’t create any problem. Therefore, a problem is closely attached with its purpose and whenever, we have a problem we need a solution to fix it. The systems approach to problem solving involves a systems route to explain the meaning of problems and opportunities and also to develop the correct feasible solutions for the same. The term ‘system’ signifies the different features, elements, merits and demerits of a particular subject that may be biological or commercial. Each and every system has certain targets to be attained. To attain these, a system has certain elements like input, output and processing devices. A system functions as per different rules, regulations, principles and policies. Therefore, systems approach is a well- organized, focused structure that consists of interrelated and interdependent elements (components, entities, factors, members, parts etc.). These elements influence one another continuously either in a direct manner or in an indirect manner to maintain their activity and the existence of the system so as to attain the targets of the system.
In simple words, a system is composed of parts or elements that are connected to each other directly or indirectly otherwise there shall be two or more distinct systems. A system has a definite limit and has a limitation of space and time. A system can be a subset of a bigger system or function in accordance with another system.
A system can be broken into smaller subsystems depending upon the nature and need of the system. The behavior of a system in an environment depends upon its interactions. This can be explained with the help of an example. In an organization, its sales function can be one system (Exhibit 1). If the problem is of poor sales performance which is the output of the business, it might be caused by lack of effort on the part of the sales team (input) or due to the obsolete methods of sales (processing) or incorrect information on sales (feedback) or improper sales management (control). Through the systems approach, such a problem can be addressed specifically and solution can be provided.
Another example can be the implementation of enterprise resource planning software. It acts as a system in which we put together the different functional departments of the firm to manage day to day business operations (Exhibit 2). The integration of these functions is optimized as per business needs concerning people, processes and technology.
- Defining Systems Approach to Problem Solving
- The Scientific Method vs. Systems Approach
The Scientific Method – the scientific approach is based on the established problem-solving methodology i.e. a logical explanation of the observed events. A step by step approach is followed which includes a generally recognized and ordered series of steps. These are given below:
- Recognizing and defining a problem
- Formulating a hypothesis
- Conducting experiments to test the formulated hypothesis
- Interpreting the results of the experiments
- Drawing conclusion about the hypothesis.
The Systems Approach – In a systems approach, the problem is viewed holistically with inherent elements and their interrelationships. Such a detailed analysis facilitates in identifying the actual problem and its symptoms for developing the actual solutions.
- Problem Recognition Using System Thinking
Under the systems approach, recognition of the problem and opportunities is the first task. Problems are occurrences that create hurdles in the attainment and fulfillment of the desired objectives whereas opportunities throw up the potential for attaining the desired objectives.
- Evaluation of Alternatives.
The next task of the expert is to identify the different systems, sub-systems and the different elements of the systems. Therefore, the systems approach takes into consideration the inputs that are used by the organization, the processing of these inputs and the resultant outputs. This also includes the reviews and the control components. These are the different systems and the sub-systems. The interactions between these are also covered. To solve particular problem, the expert needs to ascertain if all these systems are working effectively.
Once, the different solutions have been framed by the expert, the next step is to compare the different solutions. The expert has to keep in mind the past experience of the organization, the options that have been tried earlier, the cost of the different solutions etc.
- Formulating the Solution
The expert then starts the process of selecting the best solution. He/she can make criteria on the basis of which evaluation is done.
- Solution Designing
When the expert finalizes the solution for the problem then he/she starts the work of designing that solution. This may require the help from the technical support staff and other departments. The objective here is to design and describe each and every specification of the solution. Another objective is to design the complete stages of implementation. This involves specifying the work that has to be done by the different participants of the system, sub-systems etc. This then calls for assigning the work as per the capabilities, changes in the work allocation, specifying the different technical and physical resources needed and specifying the time limit of the attainment of the objectives.
- Implementation and Evaluation
The last stage includes implementation. The detailed and specified plan is implemented with utmost care. This may again require the collaboration of the different departments. All the different resources or inputs needed are also garnered. Certain cautions are required to be followed. One that there might be overlapping between the different stages and two, sometimes, the expert might have to start the process all over again. By going back, one learns what one has missed out.
These activities undertaken in a systems approach may be interdependent and interrelated. This happens when resources are integrated. Some activities may occur one time while some could be repeated. Also, an overlap of some activities is likely to occur.
- Applying the Systems Approach to Information Systems.
Systems approach is applied to the development of information systems solution in addressing business problems. This is called as information systems development or application development. In any business enterprise when the systems approach is applied to resolve the problems related with information systems then the model is called as Systems Development Life Cycle (SDLC).
- Investigation Stage
The first step is called as investigation stage. In this stage, the problem is identified in any business. In this stage, several issues are addressed like what are the different repercussions of a problem. The experts also explore the information systems solutions in this stage.
- Feasibility Studies
In the second stage, the experts find out the cost and benefits of the proposed project. This also includes the detailed preparation of the different resource requirement in terms of men, equipment, infrastructure and limiting areas are identified and agreed upon.
Feasibility studies cover the following elements:
- Organizational Level – It implies that the aims and objectives of the company are well aligned with the proposed solution of the information system to be implemented.
- Client Level – The demands and preferences of the client are to be kept at the locus of the whole planning process. The transformation system to be implemented should be so as to meet business requirements and apply to business use cases.
- Economic Level –The economic feasibility of the project is also a significant consideration. Also, parameters such as multiplication of revenues, lesser investments, reduced response time and cost cuttings are essential parameters to be met.
- Operational Level – The implementation of the systems approach through the information systems is effective when not only the top management is involved but all the management levels directly associated with it are involved. Also, the customers and suppliers are also involved.
- Technical Level – The system to be implemented is developed through the use of interoperable, scalable and robust hardware. It can be either developed or acquired by the business organization. This may also need financial investments and technical expertise.
3. Systems Analysis
Systems analysis requires the detailed study of the wants and requirements that are critical for implementing the information system in an organization. The detailed analysis regarding information needs, resources, activities and products is carried out. The shortfall in these and the additional requirements are then estimated.
- System Design
In this stage, all the specifications studied in the systems analysis stage are put into the designing of systems approach. These specifications helps in the designing of the software, hardware and other activities involved with the implementation.
The stage of system design is made up of three individual stages. The first being the User Interface design stage followed by the data design and process design stage. The user interface design lays emphasis on making the implementation more user-friendly and easily understandable. It deals with images, text, reports, forms and dialogues. In the data design stage, files and database structure is worked upon. In the last stage (process design) procedures and layout of programs and codes is done.
There are two steps involved:
Logical Systems Design – In this step, designing of the specifications required for the Information System tool to satiate the end user requirements.
Physical System Design – This step involves the development of the software, hardware and human resources. All these integrations should be well in agreement to the specifications of the logical systems design.
- Systems Implementation
After the systems design stage, the next stage is the systems implementation stage. Therefore, in this stage the acquirement of software and hardware, procedure testing, appointment of new personnel, system and service documentation and training to enable the system to become operative is done.
- Computer-Aided Systems Engineering
The SDLC Cycle is a traditional, complex and time consuming systems. These days, a new tool has emerged which is more flexible, quick and more efficient. This is called as Computer-aided systems engineering (or computer-aided software engineering) or CASE. CASE assists effectively with project management, interface design, database design, and software development. CASE as the name suggests, uses software packages or CASE tools, to perform many of the activities of the systems development life cycle. CASE packages facilitate tools both for the planning, analysis, and design of the systems development life cycle as well as for implementation and maintenance. CASE packages also include a system repository component that expands the role of the data catalogue of data definitions.
A central repository provides all the details and descriptions related with the initial stages of planning and systems analysis activities and also of design, implementation, and maintenance of the system. Thus, the repository has become a bigger database for all the details of a system generated with other systems development tools.
- Prototyping
It refers to the understanding of system proposals prior to the actual implementation. Prototyping is like a mini working model of the system. It is reviewed by the different decision makers and stakeholders. The feedback given by these can be incorporated before the actual implementation.
5. Exemplifying the Systems Approach
A systems approach refers to viewing the big picture. It does not study the system merely in fragments but in the holistic sense. It also highlights context and situations where necessary action is to be taken. Given below are the different examples that highlight the significance of the systems approach:
In a city the pollution levels are high so the problem is to estimate the pollutants. In such a system, the input is the fuel consumption and the output is the pollution generated. In the systems approach, this problem is observed as a whole without finding as to who was responsible for creating the pollution and to what extent. Such a process uses the Black Box model as it is not known what happens inside or the interactions that take place within the black box. On the other hand if the system is transparent and the interactions can be seen then it refers to the ‘white box’.
Many a times, the examination of the human body by the doctors also follows the black box approach. A doctor may not always require an internal test, an x-ray or an ultrasound to intricately observe what happens inside the body. Through the physical examination of the body, a doctor is able to treat the disease. In this system, the medicine prescribed is the input; the impact of this drug is the output. However, the chemical reaction that occurs inside the human body that causes this reaction is not analysed.
If, ABC is the coach of the Indian cricket team and the Australian cricket team is touring India. The objective of the coach is to make the Indian team win over the visiting team. The coach then may use a systems approach to achieve the objective. He collects information about the Indian team. This is a system. The sub-systems are the bowlers, batsmen, fielders. The external environment includes the regulators, umpires, the coach, the audience, media and the opponent’s team. There is another sub-system which includes the supporting staff i.e. the doctor, coach and physiotherapist of the team. All these sub-systems have an independent and distant role to play yet together these have a direct impact on the outcome of the game. In this case, the following steps are involved in the application of the systems approach:
Problem definition – In this situation, the problem is that the Indian the team win over Australian team. The coach studies the past performances of the teams and these set a benchmark.
Data Collection – The coach does and extensive research. He studies the performance of both the Indian team and the Australian team. For this purpose, he reviews the videos of the previous matches of both the teams. He identifies the strengths and weaknesses of the both the teams. He also takes note of the other factors affecting the team’s performance, directly or indirectly, for example, the weather conditions. He then frames his team’s strategy which may also include certain corrective measures. If the team plays in its home country, it not only is a reason to motivate the team but the expectations rise higher.
Identifying alternatives – Based on the collected data, the coach outlines more than one strategy for the players. He might suggest weakening the rivals’ players by sledging or other tactics. Making his bowlers to attack on the rival batsmen’s weak areas. The coach also frames strategies for its own opening batsmen. They have to be aggressive yet give stability to the wicket. Sledging needs to be avoided and keep the best attacking bowlers during the power play.
Evaluating alternatives- The coach, now, compares the different strategies. He has to select one. He compares the different alternatives. The first alternative may focus more on the competitive ability of the openers. However, it is important to team up players in a way that both interpersonal differences and sledging is avoided. The second alternative may give more stability to the mid wicket if the run rate is not as per expectations. However, this should not be affecting the opening and the aggressive play of the openers by neutralizing their efforts. The second strategy provides a safer option in the sense that it will neutralize the aggressive game of the openers but there is limited chance of getting breakthroughs. The third option of employing aggressive close-in fielders to play upon the internal personal differences of the openers and at the same. Time employing the best bowlers may lead to breakthroughs and may also restrict the aggressive openers.
Selecting the best alternative- The coach has to select the best solution to make sure a competitive lineup is formed and the aggressive players are kept in the middle to push up the performance.
Implementing and monitoring- Communicate between the coach, the team and the various stakeholders is much needed. Therefore, the coach must effectively convey the chosen strategy to them. This should be kept in mind and practiced during the trials and practice sessions.
- Applying Systems Approach for Problem Solving
1. Partitioning the system into Black Boxes – This stage requires the division of the system into different black boxes. Essentially, ‘black box’ entails that nothing is known about the structure of the system. The construction of the black box requires the knowledge of the input that goes into the process; the output that comes out. For the construction of the black box, the internal knowledge of the processing and functionality is not required. Hence, it can be said that a Black Box Model is a computer program where the information is entered by the users and the pre programmed system gives the output to the end user. These black boxes have the advantage as these need limited knowledge for construction.
- Organizing the Black Boxes into Hierarchies – This is the second stage, where the so constructed black boxes are organized into hierarchies so that the relationships among them are easily established. This establishes the hierarchy between them. Once, a hierarchy of the black boxes is established, the system becomes easier to understand as it makes the internal working of the system clearer.
Systems, processes and structures are the basic foundations of developing an Information Systems. This approach lays emphasis on the relationships between different things. When a system is considered as a whole, rather than laying focus on its component parts separately, the composite system is said to have properties which cannot be found from the properties of the component parts. The systems approach emphasizes that to solve a problem one needs to understand the interdependency between the different functional departments. This requires that the firms and organizations are organized in a more integrated way. It may be incorrect to say that a system approach implies a single ‘right’ or ‘ideal’ solution. Different approaches are measured weighed against each other so as to find one path or solution which can best suited to serve the objective of the business organization.
- Laudon Kenneth C, Laudon Jane P, Management Information Systems, Managing the Digital Firm , Pearson Education South Asia, 2013
- O’Brien A James, Marakas M George, Behl Ramesh, “Management Information Systems. ” 9th Edition, Tata Mc Graw Hill Education Pvt. Ltd.
Web Resources
- https://en.wikipedia.org/wiki/Systems_development_life_cycle
- https://scholar.harvard.edu/files/waldo/files/ps-2006-6.pdf
Lean Six Sigma Training Certification

- Facebook Instagram Twitter LinkedIn YouTube
- (877) 497-4462
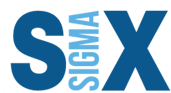
Systems Thinking: A Holistic Approach to Solving Complex Problems
February 22nd, 2024
Everything has become so interconnected, comprising of multi-pronged challenges. We still try to tackle modern problems with linear thinking approaches that isolate problems and reduce complexity.
But, more often than usual, they fall short of providing a viable solution.
Here, systems thinking offers an alternative perspective to understand how things influence one another within embedded contexts.
This holistic approach proves uniquely capable of untangling thorny issues like sustainability , inequality, or emerging diseases and making decision making effective.
As systems thinking gains wider traction, questions arise about what exactly it entails and why it matters. This article maps out the fundamental principles of systems thinking, dynamic tools used, diverse applications across sectors, and the overall benefits of adopting a systems view, especially in 2024.
The systemic perspective holds special relevance for problem solvers and changemakers currently grappling with twisted challenges and complex systems fundamentally shaped by interdependence.
By revealing hidden connections and patented patterns, systems thinking empowers interventions well-matched to our intricately networked world.
From classrooms to boardrooms, systems tools meet teams in any field to support analysis, communication, planning, or evaluation through an inter-relational lens geared to 22nd-century dynamics shaping our existence.
What is Systems Thinking?
Systems thinking is an approach to understanding how things influence one another within a whole entity. Systems thinking studies connections between key parts to see the collective behaviors that result. Expanding perspectives brings clarity to complex situations.
Systems thinking provides a framework for seeing relationships and patterns to explain how systems function. The key concepts include recognizing the interconnected and interdependent nature of systems and shifting from linear to circular causality.
Reductionist Thinking vs. Systems Thinking
In systems thinking, systems behave as integrated wholes in which elements dynamically impact each other over time.
This contrasts with traditional forms of reductionist thinking that isolate parts to understand systems.
Reductionism breaks systems down into discrete elements, rather than examining the fuzzy system boundaries, complex interactions, and unintended consequences that arise within intricate open systems in the real world.
Systems thinking offers a new perspective focused on the linkages, relationships, emergence, and feedback processes underlying systems functioning. By mapping reinforcing and balancing loops, systems thinking can identify behavior-over-time patterns for a system. This helps explain the whole picture better than reductionism.
Key Concepts of Systems Thinking
Several principles form the foundation of systems thinking. First, systems thinking recognizes the importance of feedback loops in driving system behavior. Feedback loops capture how the output of one part of a system impacts the input to another part, creating causal chains.
Reinforcing feedback loops amplify change exponentially while balancing loops counteract the change.
By mapping these feedback loops, system archetypes emerge – common patterns like limits to growth, escalation, and tragedy of the commons. These system archetypes help diagnose systemic issues, revealing core interrelating dynamics.
System archetypes function as conceptual models for understanding challenges like sustainability, urban decay, and organizational change.
In complex systems, leverage points serve as places to intervene for substantial impact. The goal is to identify where minimal effort shifts the system, through changes to parameters, feedback loops, or paradigm-shifting transformations at the level of goals or mindsets. This contrasts with incidental low-leverage tweaks.
Changing social or ecological systems often involves unintended consequences. However each system has interconnections, time delays, and complex human motivations at play. These can undermine change efforts when not adequately mapped and anticipated.
Systems thinking aims to reveal these unintended ripple effects so they can be weighed when leveraging change.
So, while unintended consequences often limit pure design, systems thinking provides insights to navigate reform more wisely. By elevating awareness of inter-dependencies and causal loops, one can recognize patterns, structures, boundaries, and relationships fundamental to systems insights.
Using Systems Thinking Approaches
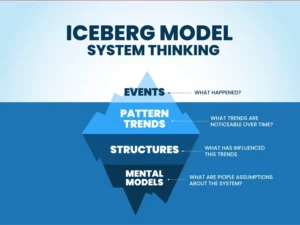
Systems thinkers employ various conceptual tools to understand systems, communicate about them, and guide interventions.
Causality mapping visually depicts variables in a system, their connections, and the direction of causal influence. This illumination of causal links reveals chains of systemic connectivity not otherwise apparent. It supports the analysis of cascading effects and feedback dynamics.
Systems mapping outlines key system components, their attributes and functions, and interrelationships. This structural perspective clarifies the organization of various elements into an integrated whole.
Systems mapping tools can also overlay dynamic processes like information flows and decision pathways to evaluate systemic leverage points.
Mental models strongly shape how people perceive systems and strategic choices within them. Two people can have divergent understandings of the same system. Reflecting critically on how mental models influence thinking is crucial for expanding limited mindsets that bound perspectives on addressing systemic issues.
More detailed system dynamics computer simulations help model system behavior by mapping dynamic complexity. This computational modeling integrates time delays, feedback processes, stocks, and flows to run long-term scenario forecasts for deeper analysis of complex systems like ecosystems, markets, or hospitals.
Adaptive systems thinkers also recognize that models have limits and that systems change over time in nonlinear ways. Rather than attempting precise prediction and control, adaptive approaches use feedback to dynamically adjust interventions according to emergent system patterns.
This flexibility to meet systems in flux is well-suited for catalyzing change in complex contexts.
Applying Systems Thinking
Systems thinking has powerful and diverse applications across sectors:
In business, systems thinking helps managers gain perspective on organizational challenges and identify root causes of problems like low morale or stagnant sales. By mapping reinforcing loops, leaders can find intervention points to shift momentum.
Systems thinking offers analytic tools to rethink structures, decision processes, and feedback channels for organizational change.
Government policymakers similarly utilize systems approaches to craft robust public policies able to balance social, environmental and , outcomes. Methodologies like group model building bring together diverse stakeholders to map out key system relationships as part of the policy design process.
This systems perspective enables policies attuned to ripple effects.
Nonprofit organizations working on social change also apply systems thinking to guide advocacy and programming. For instance, systems tools like behavior over time graphs and connection circles help groups explicitly map the structural causes perpetuating social problems like homelessness at a community level.
This equips nonprofits to pursue systemic intervention points.
More broadly, systems thinking skills help strengthen collaborative problem solving in teams. Facilitating activities that surface mental models, unpack complex dynamics, and scan for unintended consequences builds shared systemic understanding to transform discussion and explore structural solutions.
Overall, systems thinking fosters paradigm shifts towards interconnected, ecological, and holistic thinking in any problem solving context. This empowers more responsible decision-making.
Benefits of Systems Thinking
Adopting a systems thinking perspective carries many advantages:
Systems thinking allows one to see situations more completely rather than getting lost in details. By focusing on interconnections and processes that link system elements, systems thinking provides a “big picture” orientation. This expanded framework reveals areas of critical linkage within the messiness of complex contexts.
A systems view also aids in identifying types of high-leverage interventions amidst complexity. For instance, by mapping system archetypes like limits to growth or tragedy of the commons, one can pinpoint potent areas to reroute damaging feedback loops.
A systems lens highlights openings for targeted changes to cascade through interconnected subsystems.
Systems thinking also anticipates longer-term consequences of potential actions. By tracing causal threads through a system, secondary and tertiary effects are revealed that may otherwise go unseen.
This equips better foresight for the unintended impacts that might ripple across time and space from well-intentioned interventions.
Additionally, systems thinking brings order and coherence to complexity. By surfacing the organic patterning at play, systems tools decode complex dynamics in understandable yet nuanced ways.
Conceptual frameworks like stocks and flows clarify the structural forces driving issues like urban brain drain or suburban sprawl without oversimplifying.
In all of these ways, systems thinking empowers solutions better aligned to real-world complexity while still providing transformational direction. It permeates analysis with key principles of inter-relationship, temporality, perspectival flexibility, and buried connectivity – allowing insight into predicaments otherwise overwhelming.
Systems Thinking in Practice
For those first learning systems thinking, frustrations can arise. Ingrained linear thinking patterns clash with the new multidimensional perspective. Beginners also face cognitive overload wrestling with interconnections between system elements. However, skills gradually build from foundational concepts towards adept systems analysis.
With consistent practice, systems thinkers progress to parsing dynamics of specialized contexts like public health, smart grids, or supply chains. These domain experts learn to rapidly orient to unfamiliar systems through a systemic lens to ask probing questions.
Expanding one’s toolkit with advanced skills like system dynamics modeling and group facilitation extends capabilities to address complex settings.
Some systems thinkers like Donella Meadows significantly advance the field through groundbreaking applications. Meadows demonstrated deep systems wisdom over her career with The Limits to Growth and pioneering system dynamics methodologies.
These mentors develop strong systemic intuition after internalizing inter-relational patterns for decades. Their capacity to shift mental models in themselves and others unlocks societal transformation.
Ultimately, accomplished systems thinkers heed the call to teach others systemic perspectives that spread. Skills-building workshops on causal loop mapping, systems archetypes, communication tactics, and facilitation techniques proliferate systems literacy.
Outreach occurs across diverse communities given universal relevance. Each effort to cultivate systems thinking and broaden capacity for recognizing systemic leverage sustains movement toward positive change.
SixSigma.us offers both Live Virtual classes as well as Online Self-Paced training. Most option includes access to the same great Master Black Belt instructors that teach our World Class in-person sessions. Sign-up today!
Virtual Classroom Training Programs Self-Paced Online Training Programs
SixSigma.us Accreditation & Affiliations
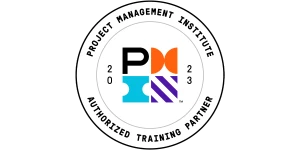
Monthly Management Tips
- Be the first one to receive the latest updates and information from 6Sigma
- Get curated resources from industry-experts
- Gain an edge with complete guides and other exclusive materials
- Become a part of one of the largest Six Sigma community
- Unlock your path to become a Six Sigma professional
" * " indicates required fields
- Skip to main content
- Skip to primary sidebar
- Skip to secondary sidebar
- Skip to footer
Computer Notes
- Computer Fundamental
- Computer Memory
- DBMS Tutorial
- Operating System
- Computer Networking
- C Programming
- C++ Programming
- Java Programming
- C# Programming
- SQL Tutorial
- Management Tutorial
- Computer Graphics
- Compiler Design
- Style Sheet
- JavaScript Tutorial
- Html Tutorial
- Wordpress Tutorial
- Python Tutorial
- PHP Tutorial
- JSP Tutorial
- AngularJS Tutorial
- Data Structures
- E Commerce Tutorial
- Visual Basic
- Structs2 Tutorial
- Digital Electronics
- Internet Terms
- Servlet Tutorial
- Software Engineering
- Interviews Questions
- Basic Terms
- Troubleshooting
Header Right
Systems approach to problem solving.
By Dinesh Thakur
Systems approach is widely used in problem solving in different contexts. Researchers in the field of science and technology have used it for quite some time now. Business problems can also be analyzed and solved using this approach. The following steps are required for this:
We’ll be covering the following topics in this tutorial:
Defining the Problem
This is the step when the problem has to be defined. Sometimes one may confuse the symptoms or the exhibition of a behavior to be a problem but actually it may only be a symptom of a larger malaise. It may just exhibit the behavior of a larger phenomenon. It is vital to drill deep into an issue and clearly understand the problem rather than having a superficial understanding of the problem. One must appreciate that this in the initial stage of problem solving and if the problem itself is not correctly diagnosed then the solution will obviously be wrong. Systems approach is therefore used to understand the problem in granular detail to establish requirement and objectives in-depth. By using the systems approach the problem will be analyzed in its totality with inherent elements and their interrelationships and therefore this detailed analysis will bring out the actual problem and separate out the symptom from it.
Developing Alternative Solutions
This the logical next step in the systems approaches for problem solving. In this stage alternative solutions are generated. This requires creativity and innovation. In this stage-the analyst uses creativity to come up with possible solutions to the problem. Typically in this stage only the outline of solutions are generated rather than the actual solutions.
Selecting a Solution
In this step, the solution that suits the requirement and objectives in the most comprehensive manner is selected as the ‘best’ solution. This is done after evaluating all the possible solutions and then comparing the possible set of solutions to find the most suitable solution lot of mathematical, financial and technical models is used to select the most appropriate solution.
Designing the Solution
Once the most appropriate solution is chosen, it is then made into a design document to give it the shape of an actionable solution, as in the evaluation stage, only the outline of the solution is used. At this stage the details of the solution are worked out to create the blueprint for the solution. Several design diagrams are used to prepare the design document. At this stage the requirement specifications are again compared with the solution design to double check the suitability of the solution for the problem.
Implementing the Solution
It is the next step in the process. The solution that has been designed is implemented as per the specifications -laid down in the design document. During implementation care is taken to ensure that there are no deviations from the design.
Reviewing the Solution
This is the final step in the problem solving process where the review of the impact of the solution is noted. This is a stage for finding out if the desired result has been achieved that was set out.
A Systems Approach Example
Let us assume that A is the coach of the Indian cricket team. Let us also assume that the objective that A has been entrusted with is to secure a win over the touring Australian cricket team. The coach uses a systems approach to attain this objective. He starts by gathering information about his own team.
Through systems approach he views his own Indian team as a system whose environment would include the other team in the competition, umpires, regulators, crowd and media. His system, i.e., team itself maybe conceptualized as having two subsystems, i.e., players and supporting staff for players. Each subsystem would have its own set of components/entities like the player subsystem will have openers, middle order batsmen, fast bowlers, wicket keeper, etc. The supporting staff subsystem would include bowling coach, batting coach, physiotherapist, psychologist, etc. All these entities would indeed have a bearing on the actual outcome of the game. The coach adopts a systems approach to determine the playing strategy that he will adopt to ensure that the Indian side wins. He analyses the issue in a stepwise manner as given below:
Step 1: Defining the problem-In this stage the coach tries to understand the past performance of his team and that of the other team in the competition. His objective is to defeat the competing team. He realizes that the problem he faces is that of losing the game. This is his main problem.
Step 2: Collecting data-The coach employs his supporting staff to gather data on the skills and physical condition of the players in the competing team by analyzing past performance data, viewing television footage of previous games, making psychological profiles of each player. The support staff analyses the data and comes up with the following observations:
- Both teams use an aggressive strategy during the period of power play. The competing Australian team uses the opening players to spearhead this attack. However, recently the openers have had a personal fight and are facing interpersonal problems.
- The game is being played in Mumbai and the local crowd support is estimated to be of some value amounting to around fifty runs. Also the crowd has come to watch the Indian team win. A loss here would cost the team in terms of morale.
- The umpires are neutral and are not intimidated by large crowd support but are lenient towards sledging.
Step 3: Identifying alternatives-Based on the collected data the coach generates the following alternate strategies:
- Play upon the minds of the opening players of the competitors by highlighting their personal differences using sledging alone.
- Employ defensive tactics during power play when the openers are most aggressive and not using sledging.
- Keep close in fielders who would sledge and employ the best attacking bowlers of the Indian team during the power play.
Step 4: Evaluating alternatives-After having generated different alternatives, the coach has to select only one. The first alternative may lead to loss of concentration on the part of openers and result in breakthroughs. However, there is a chance that the interpersonal differences between the two openers may have already been resolved before they come to the field and in such a case this strategy will fail. The second strategy provides a safer option in the sense that it will neutralize the aggressive game of the openers but there is limited chance of getting breakthroughs. The third option of employing aggressive close in fielders to play upon the internal personal differences of the openers and at the same time employing the best bowlers may lead to breakthroughs and may also restrict the aggressive openers.
Step 5: Selecting the best alternative-The coach selects the third alternative as it provides him with the opportunity of neutralizing the aggressive playing strategy of the openers as well as increases the chances of getting breakthrough wickets.
Step 6: Implementing and monitoring-The coach communicates his strategy to his players and support staff, instructs support staff to organize mock sessions and tactics to be employed to make the strategy a success. The players and support staff performance is monitored by the coach on a regular basis to ensure that the strategy is employed perfectly.
Simplifying a System or Applying Systems Approach For Problem Solving
The easiest way to simplify a system for better understanding is to follow a two-stage approach.
Partitioning the System into Black Boxes
This is the first stage of the simplification process, in this stage the system is partitioned into black boxes. Black boxes need limited knowledge to be constructed. To construct a black box one needs to know the input that goes into it, the output that comes out of it and its function. The knowledge of how the functionality is achieved is not required for constructing a black box. Black box partitioning helps in the comprehension of the system, as the entire system gets broken down into granular functionalities of a set of black boxes.
Organizing the Black Boxes into Hierarchies
This is the second stage of the simplification process, in this stage the black boxes constructed in the earlier phase are organized into hierarchies so that the relationships among the black boxes is easily established. Once, a hierarchy of the black boxes is established, the system becomes easier to understand as the internal working of the system becomes clearer.
You’ll also like:
- What is Systems Approach? Definition and Meaning
- Basic Concepts of the Systems Approach
- Database Approach
- Types of Systems
- Information Systems Planning
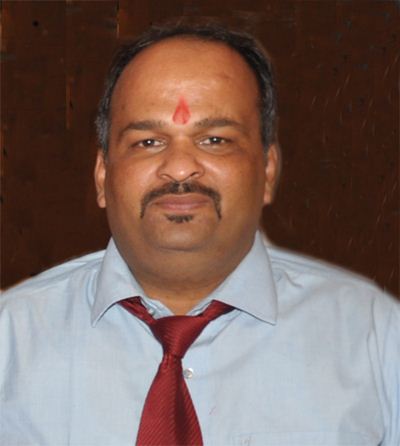
Dinesh Thakur is a Freelance Writer who helps different clients from all over the globe. Dinesh has written over 500+ blogs, 30+ eBooks, and 10000+ Posts for all types of clients.
For any type of query or something that you think is missing, please feel free to Contact us .
Basic Course
- Database System
- Management System
- Electronic Commerce
Programming
- Structured Query (SQL)
- Java Servlet
World Wide Web
- Java Script
- HTML Language
- Cascading Style Sheet
- Java Server Pages
MBA Knowledge Base
Business • Management • Technology
Home » Management Information Systems » Systems Approach to Problem Solving
Systems Approach to Problem Solving
The systems approach to problem solving used a systems orientation to define problems and opportunities and develop solutions. Studying a problem and formulating a solution involve the following interrelated activities:
- Recognize and define a problem or opportunity using systems thinking.
- Develop and evaluate alternative system solutions.
- Select the system solution that best meets your requirements.
- Design the selected system solution.
- Implement and evaluate the success of the designed system.
1. Defining Problems and Opportunities
Problems and opportunities are identified in the first step of the systems approach. A problem can be defined as a basic condition that is causing undesirable results. An opportunity is a basic condition that presents the potential for desirable results. Symptoms must be separated from problems. Symptoms are merely signals of an underlying cause or problem.
Symptom: Sales of a company’s products are declining. Problem: Sales persons are losing orders because they cannot get current information on product prices and availability. Opportunity: We could increase sales significantly if sales persons could receive instant responses to requests for price quotations and product availability.
2. Systems Thinking
Systems thinking is to try to find systems, subsystems, and components of systems in any situation your are studying. This viewpoint ensures that important factors and their interrelationships are considered. This is also known as using a systems context, or having a systemic view of a situation. I example, the business organization or business process in which a problem or opportunity arises could be viewed as a system of input, processing, output, feedback, and control components. Then to understand a problem and save it, you would determine if these basic system functions are being properly performed.
The sales function of a business can be viewed as a system. You could then ask: Is poor sales performance (output) caused by inadequate selling effort (input), out-of-date sales procedures (processing), incorrect sales information (feedback), or inadequate sales management (control)? Figure illustrates this concept.
3. Developing Alternate Solutions
There are usually several different ways to solve any problem or pursue any opportunity. Jumping immediately from problem definition to a single solution is not a good idea. It limits your options and robs you of the chance to consider the advantages and disadvantages of several alternatives. You also lose the chance to combine the best points of several alternative solutions.
4. Evaluating Alternate Solutions
Once alternative solutions have been developed, they must be evaluated so that the best solution can be identified. The goal of evaluation is to determine how well each alternative solution meets your business and personal requirements. These requirements are key characteristics and capabilities that you feed are necessary for your personal or business success.
Then you would develop evaluation criteria and determine how well each alternative solution meets these criteria. The criteria you develop will reflect how you previously defined business and personal requirements. For example, you will probably develop criteria for such factors as start-up costs, operating costs, ease of use, and reliability. Criteria may be ranked or weighted, based on their importance in meeting your requirements.
5. Selecting the Best Solution
Once all alternative solutions have been evaluated, you can being the process of selecting the best solution. Alternative solutions can be compared to each other because they have been evaluated using the same criteria.
Alternatives with a low accuracy evaluation (an accuracy score less than 10), or a low overall evaluation (an overall score less than 70) should be rejected. Therefore, alternative B for sales data entry is rejected, and alternative A, the use of laptop computers by sales reps, is selected.
6. Desingning and Implementing Solution
Once a solution has been selected, it must be designed and implemented. You may have to depend on other business end users technical staff to help you develop design specifications and an implementation plan. Typically, design specifications might describe the detailed characteristics and capabilities of the people, hardware, software, and data resources and information system activities needed by a new system. An implementation plan specifies the resources, activities, and timing needed for proper implementation. For example, the following items might be included in the design specifications and implementation plan for a computer-based sales support system:
- Types and sources of computer hardware, and software to be acquired for the sales reps.
- Operating procedures for the new sales support system.
- Training of sales reps and other personnel.
- Conversion procedures and timetable for final implementation.
7. Post Implementation Review
Related posts:.
- Operations Research approach of problem solving
- Systems Approach to Management
- How Creativity Helps in Problem Solving Process?
- Case Study on Information Systems: Cisco Systems
- System – Concept, Meaning, Definition and Characteristics
- 4 Important Types of Systems
- 11 Types of Information Systems Used in Business
- Strategic Information Systems
- Business Benefits of ERP Systems
- Role of a Systems Analyst in Organizations
Leave a Reply Cancel reply
Your email address will not be published. Required fields are marked *

Reqi Systems Engineering Articles
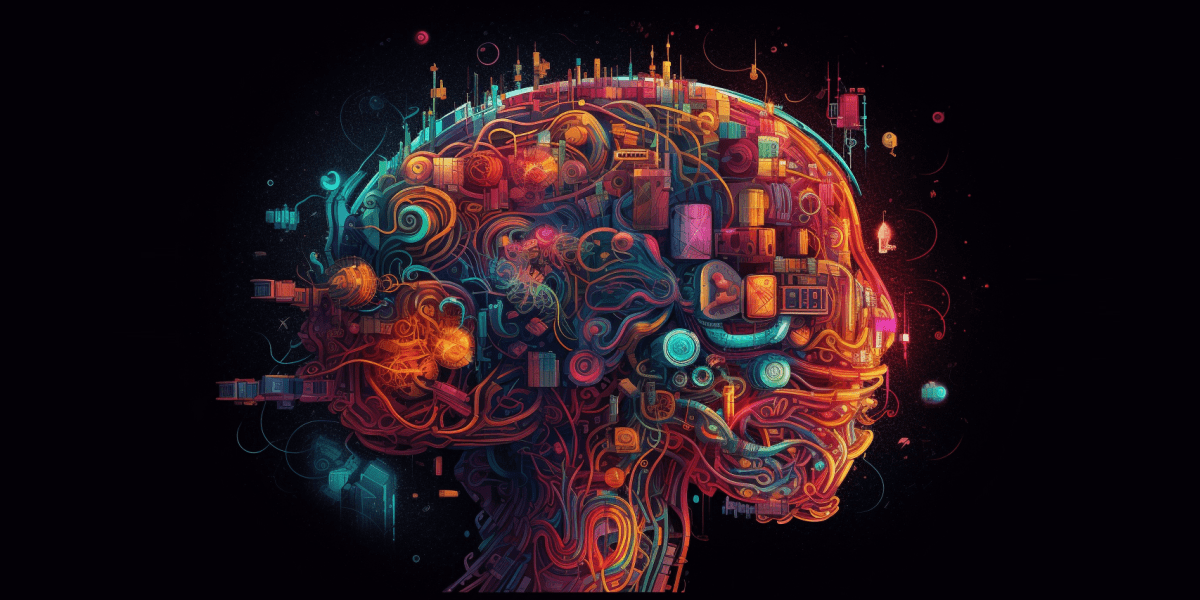
Understanding Systems Thinking: A Path to Insightful Problem-Solving
In today’s dynamic and complex business landscape, traditional problem-solving approaches often fall short in addressing persistent challenges. Enter systems thinking, a powerful methodology that offers a fresh perspective by considering the interconnectedness of various elements within a system. In this article, we delve into the fundamentals of systems thinking, exploring its principles, benefits, and practical tips for beginners. Whether you’re eager to introduce this approach in your organisation or looking to enhance your problem-solving skills, let’s embark on a journey of understanding the intricacies of systems thinking.
Table of Contents
Understanding Systems Thinking
Practical tips for beginners, the benefits of systems thinking, when to apply systems thinking, getting started, utilising systems thinking tools, indicators of progress in systems thinking.
Systems thinking encompasses a broad range of principles, tools, and a philosophical mindset. It involves understanding the circular nature of the world we live in, recognising the role of structures in shaping the conditions we face, and acknowledging the existence of powerful laws governing systems. By adopting a systems thinking approach, we gain a deeper understanding of the consequences of our actions, allowing us to make more informed decisions.
- Study Archetypes: Dive into the classic stories and patterns to enhance your understanding.
- Practice Frequently: Analyse real-world scenarios, such as newspaper articles and current headlines, through a systems lens.
- Apply Systems Thinking Everywhere: Extend your application of systems thinking beyond the workplace to gain a holistic perspective.
- Embrace Different Perspectives: Use systems thinking to explore alternative viewpoints and understand how others perceive a system.
- Accept the Learning Curve: Recognise that becoming skilled in utilising systems thinking tools takes time and practice. Embrace the journey!
Systems thinking offers several compelling reasons to adopt its principles in problem-solving endeavours. By broadening our thinking and enabling us to articulate problems in novel ways, it expands the range of choices available for resolving complex issues. Furthermore, systems thinking emphasises the importance of considering the interconnectedness of various elements, highlighting that every decision has ripple effects throughout the system. By anticipating these impacts, we can make informed choices and minimise unintended consequences.
Ideally, systems thinking is suited for problems with the following characteristics:
- Importance: The issue at hand holds significant significance.
- Chronicity: The problem persists over time, rather than being a one-time event.
- Familiarity: The problem has a known history, indicating previous attempts at resolution.
- Previous Failures: Past efforts to solve the problem have been unsuccessful.
When approaching a problem through systems thinking, it’s crucial to foster a blame-free environment. Instead of focusing on assigning blame, encourage curiosity within the team. Prompt discussions by asking thought-provoking questions like, “What aspects of this problem are we failing to comprehend?”
To ensure a comprehensive analysis, employ the iceberg framework. Encourage the team to describe the problem by examining its events, patterns, and underlying structures. Additionally, diverse perspectives are essential. Involve individuals from various departments or functional areas to capture a comprehensive range of mental models.
One of the fundamental tools in systems thinking is the causal loop diagram. When using this tool, remember that simplicity is key. Start with a small and straightforward diagram, gradually adding elements as necessary. The diagram should reflect the story your group aims to depict accurately. Don’t fret about creating a diagram that includes every variable; focus on capturing the causal relationships that matter most.
Another valuable resource in systems thinking is the use of archetypes. These classic stories serve as powerful illustrations of systems behaviour. Keep the application of archetypes simple and relatable, allowing individuals to draw parallels between the archetypes and their own problems.
As you progress in your journey of applying systems thinking, it’s essential to gauge your proficiency and recognise when you have truly grasped its principles. Here are some indicators that can help you determine if you’re on the right track:
- Asking Different Kinds of Questions: A hallmark of systems thinking is a shift in the types of questions you ask. Instead of focusing solely on immediate causes and effects, you start exploring the underlying systemic structures and interconnections. You find yourself inquiring about feedback loops, dependencies, and unintended consequences, seeking a more holistic understanding of the system at play.
- Recognising Cautionary Flags: With a growing understanding of systems thinking, you become attuned to catchphrases that may oversimplify complex problems. For instance, when someone suggests, “The problem is we need more (sales staff, revenue),” you instinctively recognise the need to delve deeper. You redirect the discussion towards systemic factors, understanding that increasing staff or revenue alone may not address the root causes.
- Detecting Archetypes and Balancing Processes: As you deepen your knowledge of systems thinking, you begin to identify recurring patterns or archetypes in stories and real-world situations. These archetypes, such as “The Tragedy of the Commons” or “Shifting the Burden,” illustrate common systemic behaviours. Recognising these archetypes enables you to spot imbalances and reinforcing processes within a system, facilitating a more comprehensive analysis of complex issues.
- Surfacing Mental Models: Systems thinking invites a deep exploration of mental models—the deeply held beliefs, assumptions, and perspectives that shape our understanding of the world. As you progress, you become adept at recognising and challenging your own mental models and those of others. By surfacing and examining these mental models, you can uncover potential biases and broaden your perspective, enabling more robust problem-solving.
- Identifying Leverage Points: Leverage points are strategic areas within a system where interventions can have a significant and lasting impact. With increasing proficiency in systems thinking, you start recognising these leverage points, understanding which actions can create meaningful change. This heightened awareness empowers you to identify leverage points in classic systems stories and apply them creatively to real-world challenges.
Systems thinking is a transformative approach to problem-solving, offering a powerful lens through which to understand complex issues. By embracing these principles and utilising its tools, you can unlock fresh insights and uncover interconnected patterns. Whether you’re just beginning your journey or seeking to refine your skills, systems thinking empowers you to tackle challenges more comprehensively, paving the way for effective and sustainable solutions.
Remember, systems thinking is not just a method; it’s a lifelong practice that cultivates curiosity, clarity, compassion, choice, and courage. Embrace this holistic approach, and you’ll witness a paradigm shift in the way you perceive the world and address complex problems.
Related Posts
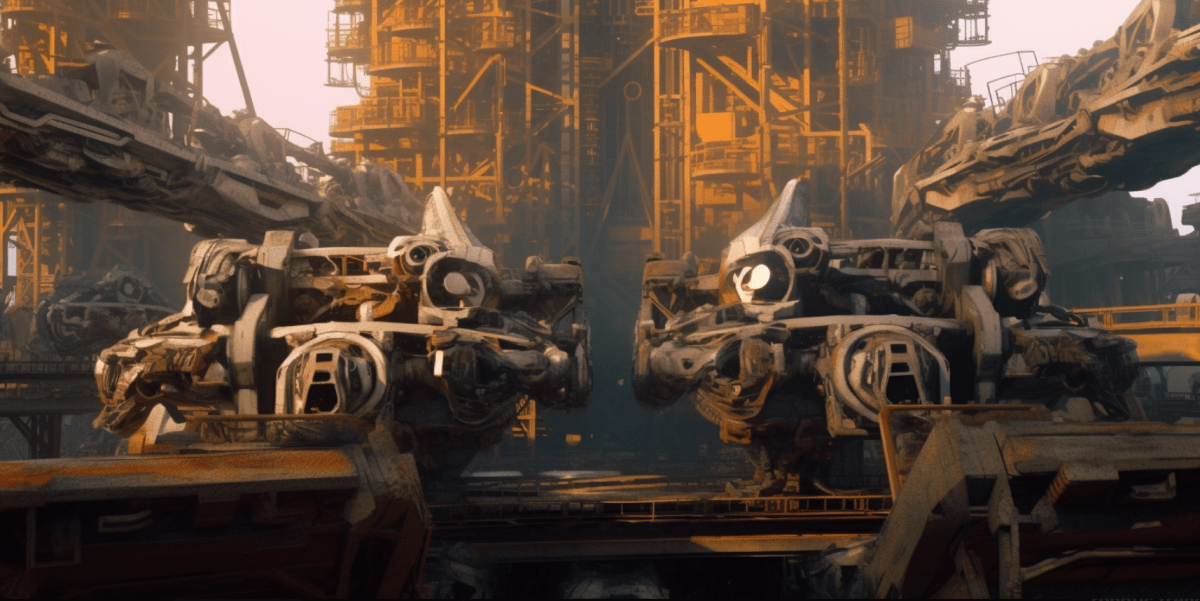
Leave a Reply Cancel reply
You must be logged in to post a comment.
View or edit this activity in your CPD log.
What is systems thinking?
Systems thinking is an approach to problem solving which takes into account the overall system as well as its individual parts. According to Peter Senge, it’s “a framework for seeing interrelationships rather than things, for seeing patterns rather than static snapshots. It is a set of general principles spanning fields as diverse as physical and social sciences, engineering and management” [ Peter Senge, The Fifth Discipline, 2nd Ed 2006 ].
Why is it important?
Complex projects need both technical and managerial leaders who understand each other’s needs and requirements, and who consequently can work in an integrated way. There is substantial evidence that an integrated project management and systems engineering approach adds value by reducing the need for re-planning and rework, and optimising the risk margin, allowing projects to fulfill their objectives both on time, and to budget.
Read more on the Systems Thinking resource page .
You can also find more through the Systems Thinking Specific Interest Group (SIG), which has a valuable resource area with links to material including books, papers, presentations and webinars. The aim of the Systems Thinking SIG is: “To promote systems thinking as a methodology to improve delivery of complex change initiatives“ and welcomes collaboration and input. To read more about the origin of the SIG, have a look at the article published on the integration of P3M and systems engineering .
John McGlynn’s blog also explains more about the development and aim of the SIG and encourages involvement.
According to new research from APM, conventional project management relying on prescribed systems and processes leaves no room for flexibility. Even agile methods are not enough as projects increase in complexity.
The research was awarded funding from APM’s research fund in 2017 to gain a better insight into the current level of understanding, application and recognition of the potential benefits of systems thinking. Read the report to discover how systems thinking can be used in project management.
People who think about systems thinking in projects argue that when you have complex interactions between people or other variables on a project it becomes very difficult to plot a straight linear path towards the defined outcome. One solution to dealing with uncertainty and ambiguity is to use a programme approach to organising the work – a framework that expects the outcomes to be created in iterative tranches or chunks of activity. Read more in this blog by Ruth Murray-Webster.
Finally, the excerpt below explains how project managers can benefit from a systems thinking approach:
The management of change in projects that are building or modifying complex systems is challenging. We define systems as complicated if they consist of many elements of different types, although, individually, each may have characteristics that can be described in simple terms. We consider systems as complex if some of the relationships and interactions between subsystems are not easily understood, and thus difficult to manage.
But project managers can borrow from engineering systems thinking, which evolved as a consequence of technical systems becoming too complicated to analyse and manage by means of conventional engineering approaches. Systems thinking enables managers to analyse the components of systems and to handle their interactions.
Systems thinking can be applied not only to technical systems, but also to projects. In this sense, projects can be considered as complex and complicated systems, where tasks and activities are the equivalent of subsystems.
Read more about this in the recent Project Journal article, Chess or dominoes .
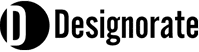
Designorate
Design thinking, innovation, user experience and healthcare design
The Six Systems Thinking Steps to Solve Complex Problems
A quick overview of common problem solving techniques indicates that most of these methods focus on the problem rather than the whole eco-system where the problem exists. Along with the challenges of global economy , problems turn out to be more complicated and sometimes awakening problems. Climate change, traffic problems, and organizational problems that have developed through the years are all complex problems that we shouldn’t look at the same way as simple or linear problems. Part of the problem of thinking about a complex problem is the way we approach it, which may contribute to making the problem even more complex. As stated by Albert Einstein, “The problems cannot be solved using the same level of thinking that created them.” Systems thinking tends to focus on the broader ecosystem rather than the problem itself.
Systems thinking was developed by Jay Forrester and members of the Society for Organizational Learning at MIT. The idea is described in his book, The Fifth Discipline , as follows: “Systems thinking is a discipline for seeing wholes. It is a framework for seeing interrelationships rather than things, for seeing patterns of change rather than static ‘snapshots.’” A common example of the systems thinking method is the life around us where multiple systems interact with each other and are affected by each other. This wide perspective of systems thinking promotes it to solve complex problems that are dependent on external factors. Below are some of the stations that system thinking may contribute to solve.
- Complex problems that involve different factors, which require understanding the big picture in order to be efficiently solved
- Situations that are affecting, are being affected by, or affect the surrounding systems
- Problems that have turned more complicated by previous attempts to solve them
Concepts of Systems Thinking
In order to understand systems thinking, a number of concepts should be highlighted in order to define the relation between the problem and the other elements in the system and how to observe this relation in order to reach an effective solution. These principles include the following.
- All systems are composed of interconnected parts, and changing one part affects the entire system, including other parts.
- The structure of a system determines its behavior, which means that the system depends on the connection between parts rather that the part themselves.
- System behavior is an emergent phenomenon. System behavior is hard to predict due its continuously changing, non-linear relations and its time delay. It can’t be predicted by simply inspecting its elements or structure.
- Feedback loops control a system’s major dynamic behavior. The feedback loop is a number of connections causing an output from one part to eventually influence input to that same part. The number of feedback loops are larger than the system parts, which contributes to increasing system complicity.
- Complex social systems exhibit counterintuitive behavior. Solving complex problems can’t be achieved through everyday problem solving methods. They can be solved only through analytical methods and tools. Solving complex problems can be achieved through systems thinking, a process that fits the problem, and system dynamics , which is an approach to model systems by emphasizing their feedback loops.
Systems Thinking in Six Steps
In their paper Six Steps to Thinking Systemically , Michael Goodman and Richard Karash introduced six steps to apply systems thinking principles while solving complex problems. These steps were part of their case study to Bijou Bottling company’s problem of getting their orders shipped on time.
Set 1: Tell the Story
The first step in solving the problem is to understand it, and this can be achieved through looking deeply at the whole system rather than individual parts. This step requires meeting with the stakeholders to share their vision about the situation. One of the common tools to build this understanding is to utilize Concept Maps, which are graphical tools used to represent the organization or a structure of knowledge. Concept Maps visually present the system’s elements, concept links, proposition statements, cross-links, and examples.
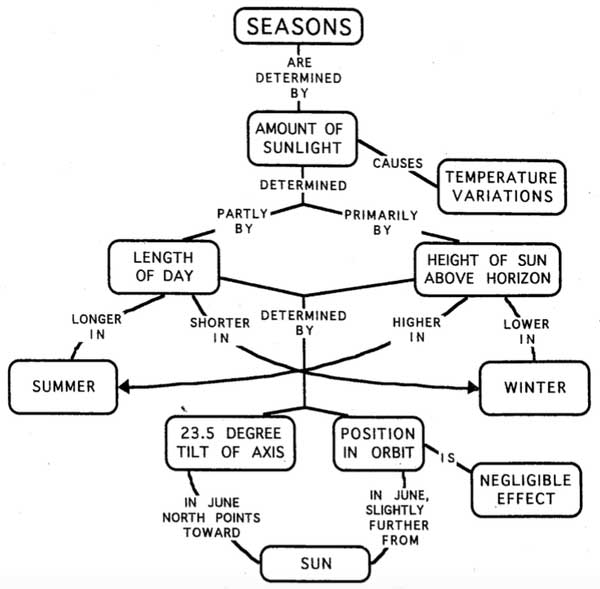
Step 2: Draw Behavior Over Time (BOT) Graphs
When thinking about a problem, we are influenced with the current situation that is reflected in our analysis, yet the problem follows a time dimension, which means that it should be tracked through the time. The Behavior Over Time graph draws a curve that presents a specific behavior (Y) through the time (X). This graph helps us to understanding whether or not the current solution is effective.
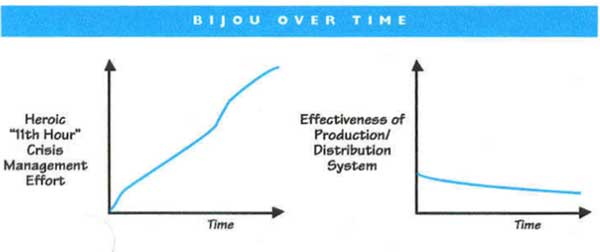
Step 3: Create a Focusing Statement
At this point, there should be a clear vision about the problem solving process, which is defined in the from of a statement that indicates the team’s target and why the problem occurs.
Step 4: Identify the Structure
After having clear vision about the problem through the proposed statement, the system structure should be described, including the behavior patterns. Building these patterns helps in understanding more about the problem, and it can be formed as a system archetype.
Step 5: Going Deeper into the Issues
After defining the problem and the system structure, this step tends to understand the underlying problems through clarifying four items: the purpose of the system (what we want), the mental models, the large system, and personal role in the situation.
Set 6: Plan an Intervention
The previously collected information is used to start the intervention phase, where modifications to the current problem relate parts to connections. This intervention attempts to reach the desirable behavior.
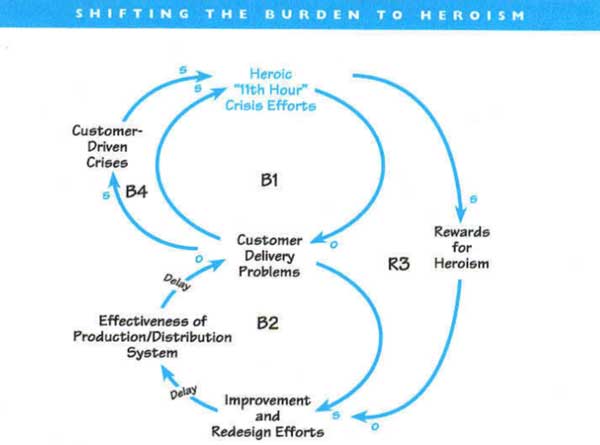
Practice Example of Systems Thinking
One of the direct examples of adopting the systems thinking method was presented by Daniel Aronson highlighting insects who caused damage crops. Traditional thinking to solve crop damage is to apply more pesticides to reduce the number of insects and subsequently reduce the crop damage. However, this solution solves the problem for a short term. In the long run, the problem isn’t truly solved, as the original insect eating the crops are controlling the population of another species of insect in the environment either by preying on it or competing with it. Subsequently, the crop damage increases again due to the increasing numbers of other insect species.
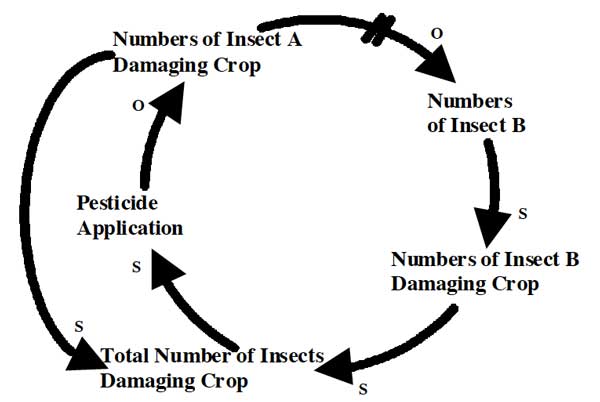
Observing the ecosystem that includes both the insects and the crops, systems thinking suggests exploring a solution that ensures reducing the crop damage in the long run without affecting the environmental balance, such as deploying the Integrated Pest Management that has proven success based on MIT and the National Academy of Science. This solution tends to control the number of an insect species by introducing its predators in the area.
Unlike everyday problems, complex problems can’t be solved using traditional problem solving methods due to the nature of the problems and their complexity. One of the theories that attempts to understand complex problems is systems thinking, which is defined by a number of characters. Six steps are to be used to explore and solve complex problems under the umbrella of systems thinking, which help us to observe and think in a whole eco-system rather than individual parts. Systems thinking can be deployed in multiple domains to solve organization problem, or global problems such as energy, pollution, and poverty.
Wait, Join my Newsletters!
As always, I try to come to you with design ideas, tips, and tools for design and creative thinking. Subscribe to my newsletters to receive new updated design tools and tips!
Dr Rafiq Elmansy
As an academic and author, I've had the privilege of shaping the design landscape. I teach design at the University of Leeds and am the Programme Leader for the MA Design, focusing on design thinking, design for health, and behavioural design. I've developed and taught several innovative programmes at Wrexham Glyndwr University, Northumbria University, and The American University in Cairo. I'm also a published book author and the proud founder of Designorate.com, a platform that has been instrumental in fostering design innovation. My expertise in design has been recognised by prestigious organizations. I'm a fellow of the Higher Education Academy (HEA), the Design Research Society (FDRS), and an Adobe Education Leader. Over the course of 20 years, I've had the privilege of working with esteemed clients such as the UN, World Bank, Adobe, and Schneider, contributing to their design strategies. For more than 12 years, I collaborated closely with the Adobe team, playing a key role in the development of many Adobe applications.
You May Also Like
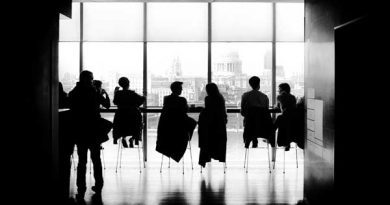
What Kills Design Thinking?
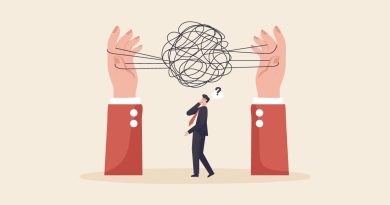
How to Create the Systems Thinking Diagrams
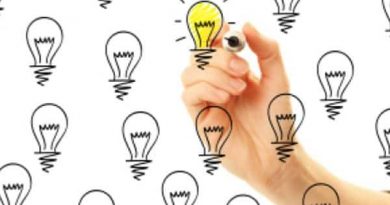
How to Evaluate Design Ideas
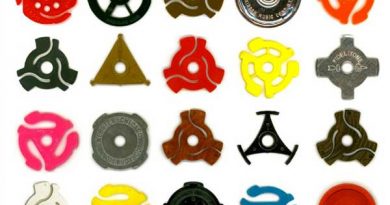
Top Resources to Learn Design Thinking Online
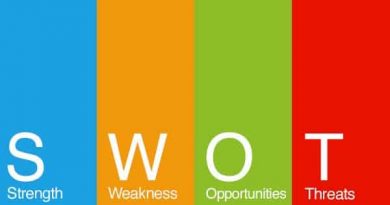
SWOT Analysis: Exploring Innovation and Creativity within Organizations
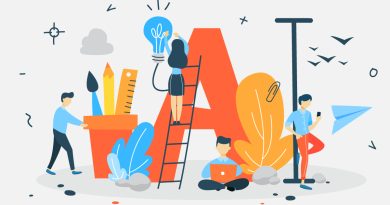
What are Design Research Types and Applications?
3 thoughts on “ the six systems thinking steps to solve complex problems ”.
“Systems thinking was developed by Jay Forrester and members of the Society for Organizational Learning at MIT. The idea is described in his book, The Fifth Discipline, as follows:” Peter Senge is the author of The Fifth Discipline
Thank you so much Misi for the helpful information.
Thank you for the valuable information. I believe that systems thinking can be applied to every aspect of our lives. When you teach yourself to spot patterns, cycles, and loops instead of individuals elements. You see behind the scenes. Understand what actually needs addressing to move forward and make progress faster with less damage.
Leave a Reply Cancel reply
Your email address will not be published. Required fields are marked *
Sign me up for the newsletter!
How it works
Transform your enterprise with the scalable mindsets, skills, & behavior change that drive performance.
Explore how BetterUp connects to your core business systems.
We pair AI with the latest in human-centered coaching to drive powerful, lasting learning and behavior change.
Build leaders that accelerate team performance and engagement.
Unlock performance potential at scale with AI-powered curated growth journeys.
Build resilience, well-being and agility to drive performance across your entire enterprise.
Transform your business, starting with your sales leaders.
Unlock business impact from the top with executive coaching.
Foster a culture of inclusion and belonging.
Accelerate the performance and potential of your agencies and employees.
See how innovative organizations use BetterUp to build a thriving workforce.
Discover how BetterUp measurably impacts key business outcomes for organizations like yours.
Daring Leadership Institute: a groundbreaking partnership that amplifies Brené Brown's empirically based, courage-building curriculum with BetterUp’s human transformation platform.

- What is coaching?
Learn how 1:1 coaching works, who its for, and if it's right for you.
Accelerate your personal and professional growth with the expert guidance of a BetterUp Coach.
Types of Coaching
Navigate career transitions, accelerate your professional growth, and achieve your career goals with expert coaching.
Enhance your communication skills for better personal and professional relationships, with tailored coaching that focuses on your needs.
Find balance, resilience, and well-being in all areas of your life with holistic coaching designed to empower you.
Discover your perfect match : Take our 5-minute assessment and let us pair you with one of our top Coaches tailored just for you.
Find your coach
-1.png)
Research, expert insights, and resources to develop courageous leaders within your organization.
Best practices, research, and tools to fuel individual and business growth.
View on-demand BetterUp events and learn about upcoming live discussions.
The latest insights and ideas for building a high-performing workplace.
- BetterUp Briefing
The online magazine that helps you understand tomorrow's workforce trends, today.
Innovative research featured in peer-reviewed journals, press, and more.
Founded in 2022 to deepen the understanding of the intersection of well-being, purpose, and performance
We're on a mission to help everyone live with clarity, purpose, and passion.
Join us and create impactful change.
Read the buzz about BetterUp.
Meet the leadership that's passionate about empowering your workforce.

For Business
For Individuals
What’s systems thinking? The secret to a future-minded organization
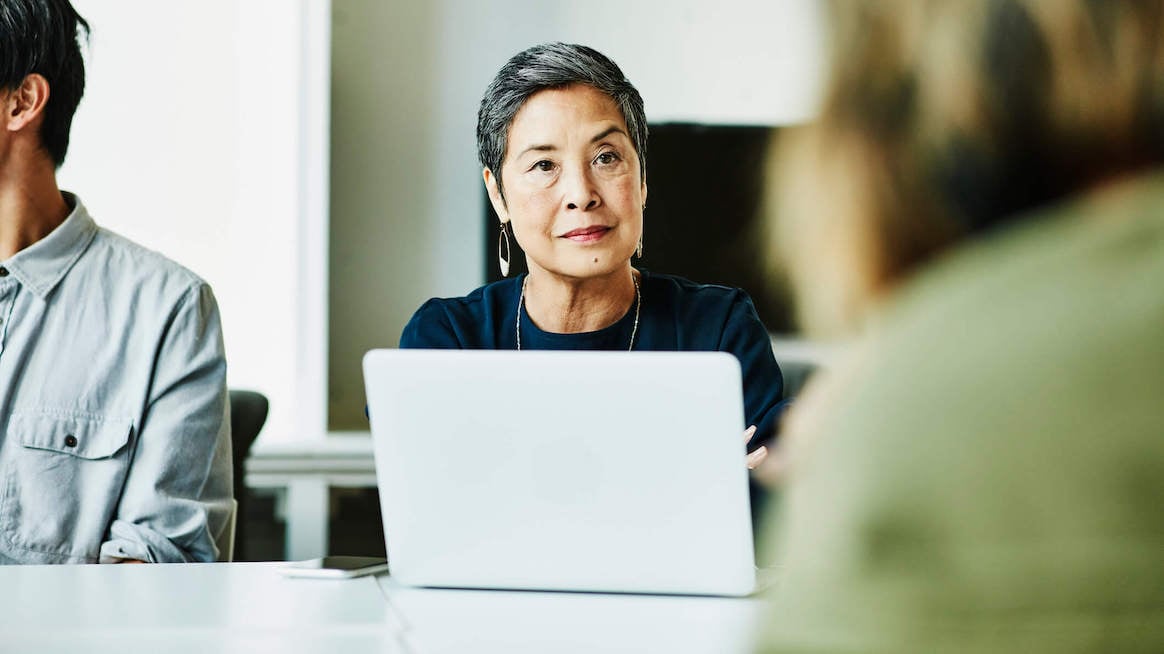
I’ve been working on widening my aperture. What does that mean? In photography, zooming out. Seeing the forest for the trees.
As a writer, I find that I often get bogged down in the details. Sometimes, I look too closely at a topic or an idea without considering the complexities, relationships, and implications.
It’s easy to see things when we’re close to them. But it takes a concerted effort to step back and look at the bigger picture. It requires a different type of mindset, strategic thinking, and perspective on problem-solving .
We probably can all think of people who approach the world as system thinkers. You probably can name a few off the top of your head: Ruth Bader Ginsburg, Steve Jobs, Stacey Abrams, Bill Gates, Malala Yousafzai, Barack Obama, and many more.
They’re big-picture thinkers , dreamers, and strategists. They all share curiosity, courage , and the willingness to challenge the status quo. They see the problem at hand in a network of complex systems, and they aren’t afraid to prod at the larger ecosystem. Systems thinking might sound like a clunky, corporate jargon phrase. And in some ways, by definition, it is complex. But at its heart, systems thinking is about seeing things through a wide lens, recognizing how interconnected we are, and acting with empathy and innovation.
Actions have consequences, not always the ones intended. While it can be about solving wicked problems, systems thinking can also be about getting stuff done in ways that are beneficial to the whole organization, not just your little piece of it. A system can be a company, a school, a community, a region, or even a family.
In the context of today’s world of work, systems thinking can help you to be more strategic and better prepared for what the future has in store. Applying systems thinking to our current climate can help us look ahead with a more strategic lens.
Especially when things are constantly changing — and uncertainty looms overhead — systems thinking helps organizations be better prepared to solve complex problems. Let’s break down what systems thinking is. We’ll also talk about what it takes to become a systems thinker — and how applying systems thinking can help your organization thrive.
What is systems thinking?
Before we go any further, let’s pause to understand what we mean by systems thinking .
Systems thinking is the ability that an individual or organization has to solve tough problems. With systems thinking, individuals use strategic, big-picture thinking to make sense of a complex system.
For example, at BetterUp we talk about how optimizing for the company typically means sub-optimizing for individual teams. But it holds true for any large organization.
Without systems thinking, a team might set its goals very narrowly and pursue them. Sometimes, those pursuits result in strategies that are detrimental to another team or the bigger company objectives.
Companies that want to be more than the sum of their parts need managers who can think systemically and with enough transparency that people can understand the system.
Systems thinking is a holistic approach to problem-solving. It’s a way of looking at how systems work, what that system’s perspective is, and how to better improve system behaviors.
The systems thinking methodology isn’t necessarily formulaic. It takes some understanding of key concepts to be able to take a systems approach to today’s most challenging problems.
Systems thinking in leadership
As we mentioned, many of today’s most notable strategic leaders lean on their systems thinking skills to drive change. It requires a deep understanding of mental models with the goal of improving them to optimize organizational performance . And while you might not know it, many leaders have applied system thinking tools to help come to new conclusions.
Systems thinking in leadership, however, isn’t a one-size-fits-all approach. Every problem is different with its own set of system dynamics. Let’s break down what some of this could look like in leadership.
- A future-mindedness. At BetterUp, we’ve studied future-minded leaders . It’s the idea that a leader looks ahead with a sense of pragmatism and optimism. Leaders who use the future-minded lens say they spend 147% more time planning in their lives and 159% more time planning in their work than those with low future-minded leadership skills. The result of all this planning? Future-minded leaders have higher-performing teams. increased agility, team engagement, innovation, risk-taking, performance, and resilience.

- Strategy and planning. As you could’ve guessed, strategic thinking and strategic planning are big components of adopting a systems perspective. Leaders are able to zoom out to see the whole system, then zoom in to see how the system works.
- A growth mindset. If we really strip down systems thinking, it’s about problem-solving. This means leaders don’t know everything. They need to learn — and be willing to learn — new things. Leaders who adopt a growth mindset are better equipped to see how the system works because of this perspective.
- The willingness to be wrong. We’ve probably all had managers who are unwilling to be wrong. Even if the data and science back it up, there’s some excuse as to why their theory, strategy, or process will still work. It’s a fixed mindset that won’t let go. But with system thinkers in leadership, they’re willing to be wrong. They can see when a systems theory isn’t working. And they embrace that vulnerability of admitting they need to re-think what they originally thought.
“We learn more from people who challenge our thought process than those who affirm our conclusions. Strong leaders engage their critics and make themselves stronger. Weak leaders silence their critics and make themselves weaker. This reaction isn’t limited to people in power. Although we might be on board with the principle, in practice we often miss out on the value of a challenge network.” Adam Grant, BetterUp Science Board Member, organizational psychologist, author, Think Again
What are examples of systems thinking?
To better understand systems thinking, let’s look at these three examples. Each example demonstrates the innovation that arises when you see the potential for a whole new board game rather than just swapping out one piece of the puzzle.
- Smartphones. I grew up in a house where phones were plugged into the wall and computers took over phone lines. When I wanted to call a friend, I dragged the landline — cord still plugged in — into my bedroom. If I wanted to look something up on the internet, I had to make sure no one in my household was using the phone. Why? Well, because the internet required dialed-in access to the phone line. Fast forward a couple of decades and now, we have tiny, little computers that fit into our pockets. Smartphones allow you to access the internet virtually everywhere you go, so long as there’s a signal or a WiFi log-in. Smartphones didn’t come about just to change where and how we could make a phone call. They evolved because system thinkers like Steve Jobs anticipated how connectivity could change the bigger system of how we consume and interact. Systems thinkers see what could be instead of what is.
- Cryptocurrency. When is the last time you had cash in your wallet? If you’re like me, you rarely carry any cash anymore. Though just twenty years ago, I made sure I had at least $10 in cash with me at all times. But soon, the world evolved with plastic cards that somehow, became much more valuable than any number of bills you could carry in your wallet. Debit and credit cards replaced weekly bank withdrawals. But system thinkers took currency one step further: crypto . Money now moves in networks that securely transfer different types of digital property over the Internet. This technology reimagines how the world does business, but it also has implications for larger monetary, regulatory, and political systems.
- Renewable energy. With climate change , we’re living on the brink of irreversible damage. With global temperatures rising faster than before, system thinkers had to find a way to power the world that doesn’t harm the planet. Enter: renewable energy. Renewable energy sources (like solar and wind power) have reimagined how we run businesses, travel, and even produce goods. This system-of-systems approach is helping to shape a low-carbon economy . According to Deloitte, slowing the accelerating pace at which the climate crisis is progressing requires overhauling how systems work.
Push a little further on these examples and you might also see that each also shows the failure to fully imagine the impact on the broader systems they touch.
Smartphones and crypto-currency each have environmental effects, increasing demand for energy and rare materials. Shifts in demand can create new supply chains and new companies as well as shortages and power imbalances. Systems thinking is recognizing that there are no simple answers.
Complex adaptive systems are just that: adaptive. They’re dynamic systems that hinge on feedback loops, innovation, and collaboration . And it’s with systems thinking that we’re able to evolve and innovate to find better solutions to today’s modern challenges.

6 important concepts of systems thinking
For your organization, adopting concepts of systems thinking can help your business stay a step ahead. Especially in a fast-changing world, it’s critical that organizations stay agile and strategic to stay relevant. Here are six important concepts of systems thinking to help your organization stay resilient, agile, and relevant for the future.
1. Systems mapping
To understand how to solve a problem, you need to understand the ecosystems in which the problem lives. This is called systems mapping: getting to know the systems where a problem lives to better take it apart.
Once you’ve mapped out the systems to help solve your problem, you can do some systems modeling to help understand how they’re connected. Which leads us to …
2. Interconnectedness
Interconnectedness. If we know anything about the world, it’s much smaller than we think. And after you’ve mapped out the systems for the problem you’re trying to solve, it’s time to figure out how the systems are connected.
Sometimes, it may seem nonlinear or non-consequential. But if you dig deep enough, you’ll likely find some fibers connected between specific systems.
For example, let’s use the pandemic. COVID-19 illuminated that our systems are more connected than we think. The impacts of COVID-19 disproportionately impacted communities of color and those of lower socioeconomic status. On its face, it might not have been readily apparent that a public health crisis would bleed into a different system, our economy.
3. Synthesis
This concept is synthesizing. Essentially, it’s making sense of things in the context of the problem you’re trying to solve. Opposite to analysis, synthesis usually is when you combine ideas or things to create something new.
4. Emergence
Let’s look at the solar system. We know that the solar system is a large, abstract, and complex system. It’s made up of planets, stars, galaxies, and many other things that we likely have yet to discover.
But that’s the point of emergence: larger things emerge from smaller things. And when it comes to figuring out how synthesizing (or how you’re putting together different parts), emergence is critical.
5. Feedback loops
Feedback is critical to understanding if something is working. More importantly, feedback helps us understand when things aren’t working.
If you’re adopting systems thinking in your organization, consider how you’re implementing feedback loops into the process.
For example, let’s say you’re rolling out a new performance management software. Your HR teams are working with managers across the business to adequately train folks on how to use the platform. However, you realize that some managers are missing key milestones, like annual performance reviews .
You set up some focus groups and office hours with your managers. In these sessions, you learn that your managers are missing out on performance review milestones in the system because they don’t know how to navigate the software. After gathering feedback , you realize that your organization requires more support.
6. Causality
Causality is the idea that there’s a cause and effect. It’s pretty simple: your actions impact the outcome. And so when you’re looking at a part of the system to solve, it’s important to test the cause and effect pieces of your systems.
Let’s go back to our example from above. Because you’ve implemented regular feedback checkpoints within manager office hours, your HR team can better adjust their communication strategy. With help from the internal communication team, your HR team put together some guides on how to best use the software. This helped improve the number of “missed” performance reviews by 30%.
How to apply systems thinking to the workplace
If you’re ready to apply systems thinking to the workplace, here are four things to keep in mind.

Practice future-minded thinking
Future-mindedness can keep organizations prepared for the future. Of course, we know the future is unknown. Especially now, there’s plenty of uncertainty and change looming.
But with future-mindedness, your organization can be better equipped for what the future holds. Training your leaders to build their future-minded skills can help to keep your organization agile, resilient, and relevant for whatever the future holds. With future-mindedness , the impact speaks for itself:
- Individual performance and well-being increases
- Team performance increases with more agility, resilience, and risk-taking
- Teams are more innovative, creative, and collaborative
- Employee retention increases by 33%
Promote a growth mindset
Organizations, now more than ever, need to adopt a growth mindset. Learning is a lifelong journey for any person. Why wouldn’t organizations adopt the same sort of mindset?
Think about how you can cultivate a growth mindset within your workplace. For example, how are you encouraging professional development ? Are you promoting from within and encouraging career mobility ? In what ways are you creating career advancement opportunities? Do your employees invest in upskilling or reskilling?

Create space for feedback
The success of any organization hinges on the ability to provide — and receive — feedback . At BetterUp, we see feedback as a gift. It’s a way to identify what’s working. But more importantly, it’s how we evolve and grow.
Are you creating spaces for feedback? How are you keeping a pulse on your employees’ engagement ? Are you encouraging upward feedback or 360-degree feedback ?
Use coaching
We all need guidance. Especially when we’re tasked with solving some of the toughest problems, it helps to have an outside perspective.
That’s where coaching comes in. With BetterUp, you can pair your employees with personalized support to help crack tough problems. A coach can help your employees tap into parts of themselves that they didn’t know existed. In turn, it will help improve your organizational effectiveness .
Try BetterUp. Together, we can build a future better equipped to solve tomorrow’s problems.
Understand Yourself Better:
Big 5 Personality Test
Madeline Miles
Madeline is a writer, communicator, and storyteller who is passionate about using words to help drive positive change. She holds a bachelor's in English Creative Writing and Communication Studies and lives in Denver, Colorado. In her spare time, she's usually somewhere outside (preferably in the mountains) — and enjoys poetry and fiction.
Does dotted line reporting work? 9 pros and cons of blurring the line
6 big picture thinking strategies that you'll actually use, 4 reasons to promote an employee into a new role, management isn’t for everyone: here’s what to do instead, 15 tips for your end-of-year reviews, developing individual contributors: your secret weapon for growth, 17 career development questions for managers to ask, from service member to civilian: unlock this key talent pool with successful transitions, meet the future-minded leader: your organization’s answer to uncertainty, thinking outside the box: 8 ways to become a creative problem solver, 3 ways to solve your performance management problems, 5 candidate sourcing tactics that’ll put your company a step ahead, what’s convergent thinking how to be a better problem-solver, how to use strategic foresight to stay ahead of the curve, 3 essential traits for leaders in turbulent times, outsmarting the innovator's bias: where future-mindedness and innovation meet, stay connected with betterup, get our newsletter, event invites, plus product insights and research..
3100 E 5th Street, Suite 350 Austin, TX 78702
- Platform Overview
- Integrations
- Powered by AI
- BetterUp Lead™
- BetterUp Manage™
- BetterUp Care®
- Sales Performance
- Diversity & Inclusion
- Case Studies
- Why BetterUp?
- About Coaching
- Find your Coach
- Career Coaching
- Communication Coaching
- Personal Coaching
- News and Press
- Leadership Team
- Become a BetterUp Coach
- BetterUp Labs
- Center for Purpose & Performance
- Leadership Training
- Business Coaching
- Contact Support
- Contact Sales
- Privacy Policy
- Acceptable Use Policy
- Trust & Security
- Cookie Preferences
Hey! You are using an outdated browser. Please upgrade your browser to improve your experience.
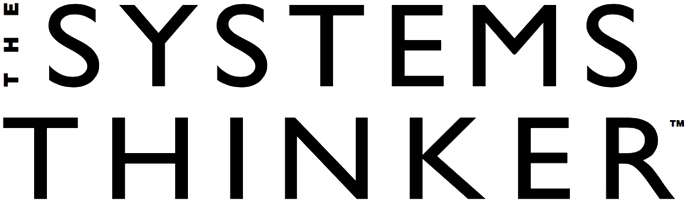
Six Steps to Thinking Systemically
B ijou Bottling Company is a fictitous beverage bottler with an all too real problem: chronic late shipments. Its customers—major chain retailers—are looking for orders shipped complete and on time. About five years ago, in a U. S. region covering about six states, this problem reached crisis proportions…
In the face of day-to-day pressures, groups often leap to solutions after only a modest amount of brainstorming. A systemic approach, however, provides a structured problem-solving process for digging deeper into our most vexing problems.
To get a sense for how systems thinking can be used for problem identification, problem solving, and solution testing, we have outlined a six-step process. To use this process on a problem in your workplace, try the worksheet on page 9.
1. Tell the Story
The starting point for a systems thinking analysis is to get your head above water enough to start thinking about the problem instead of just acting on it. An effective way to do this is to gather together all of the important players in the situation and have each one describe the problem from his or her point of view.
At Bijou Bottling Company, the problem was usually a customer complaint: “Where were the 40 cases of 2-litre Baseball tie-in product that were ordered last week?!” Somehow Bijou would get the goods there on time, whatever it took—including air shipping heavy soda in glass bottles at enormous costs. But this crisis management led to a culture where people built their careers on coming in at the 11th hour and turning around a customer complaint.
2. Draw “Behavior Over Time” Graphs
In the storytelling stage, most of the energy is focused on the pressures of the current moment. When we move to “Behavior Over Time” (BOT) graphs, however, we begin to connect the present to the past and move from seeing events to recognizing patterns over time.
Draw only one variable per graph on a Post-it™ note so it can be easily moved around in the steps that follow. The time frame should span from past up to the present—but it can also include future projections (see “Bijou Over Time”).
3. Create a Focusing Statement
At this point, you want to create a statement that will help channel energy during the rest of the process. This statement may involve a picture of what people want, or a question about why certain problems are occurring. At Bijou, for example, the focusing statement was: “We’re pretty good at solving each problem as it arises. But why are these problems recurring with greater frequency and intensity? What is causing them?”
BIJOU OVER TIME
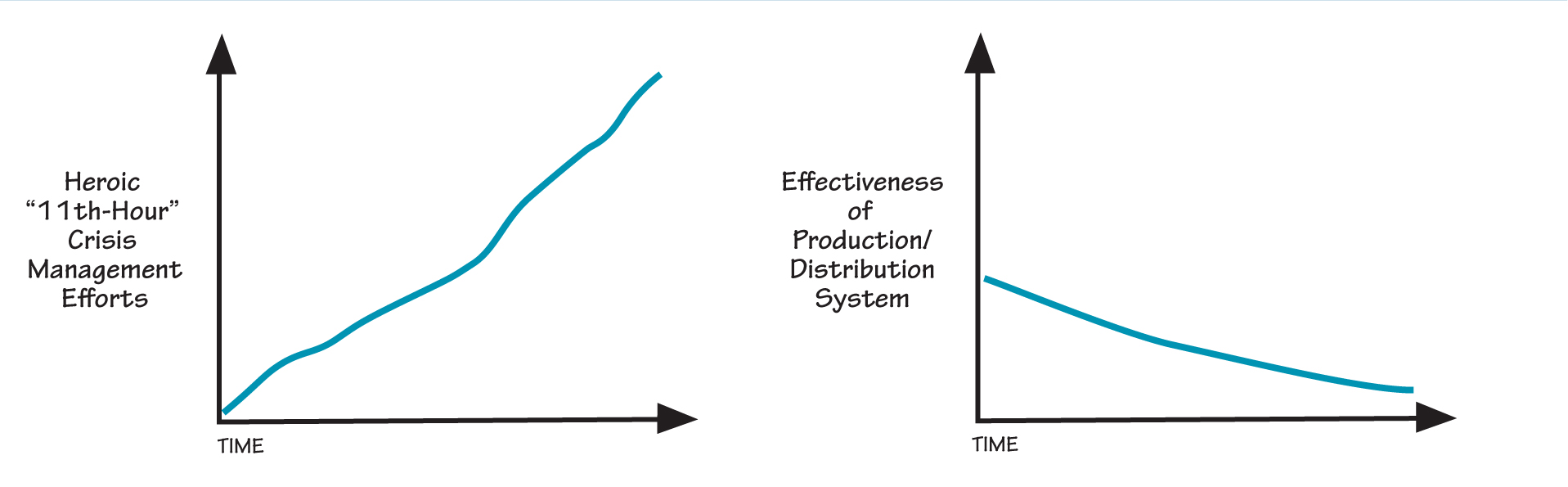
At Bijou, crisis management efforts had increased over time, while the effectiveness of the production/distribution system had decreased.
4. Identify the Structure
You now want to describe the systemic structures that are creating the behavior patterns you identified. The systems archetypes are an easy way to begin building a theory of why and how things are happening (see “Systems Archetypes at a Glance,” V22N6, August 2011).
Begin by reviewing the story, graphs, and focusing statement to see if they follow the storyline of an archetype. If so, draw the loop diagram for that archetype, place the Post-its of the variables in the diagram, and move them around on a flip chart until you have a diagram that seems to capture what is going on.
The group at Bijou decided that their problem matched the “Shifting the Burden” storyline, in which a problem is “solved” by applying a short-term solution that takes attention away from more fundamental improvements. They identified a balancing loop that described how customer problems were solved with heroic “11th-Hour” efforts (the symptomatic solution) at the expense of improvement and redesign of the production/distribution system (the fundamental solution). As people “learned” over time that heroism is rewarded, their willingness and ability to address system-wide problems decreased (see “Shifting the Burden to Heroism”).
SHIFTING THE BURDEN TO HEROISM
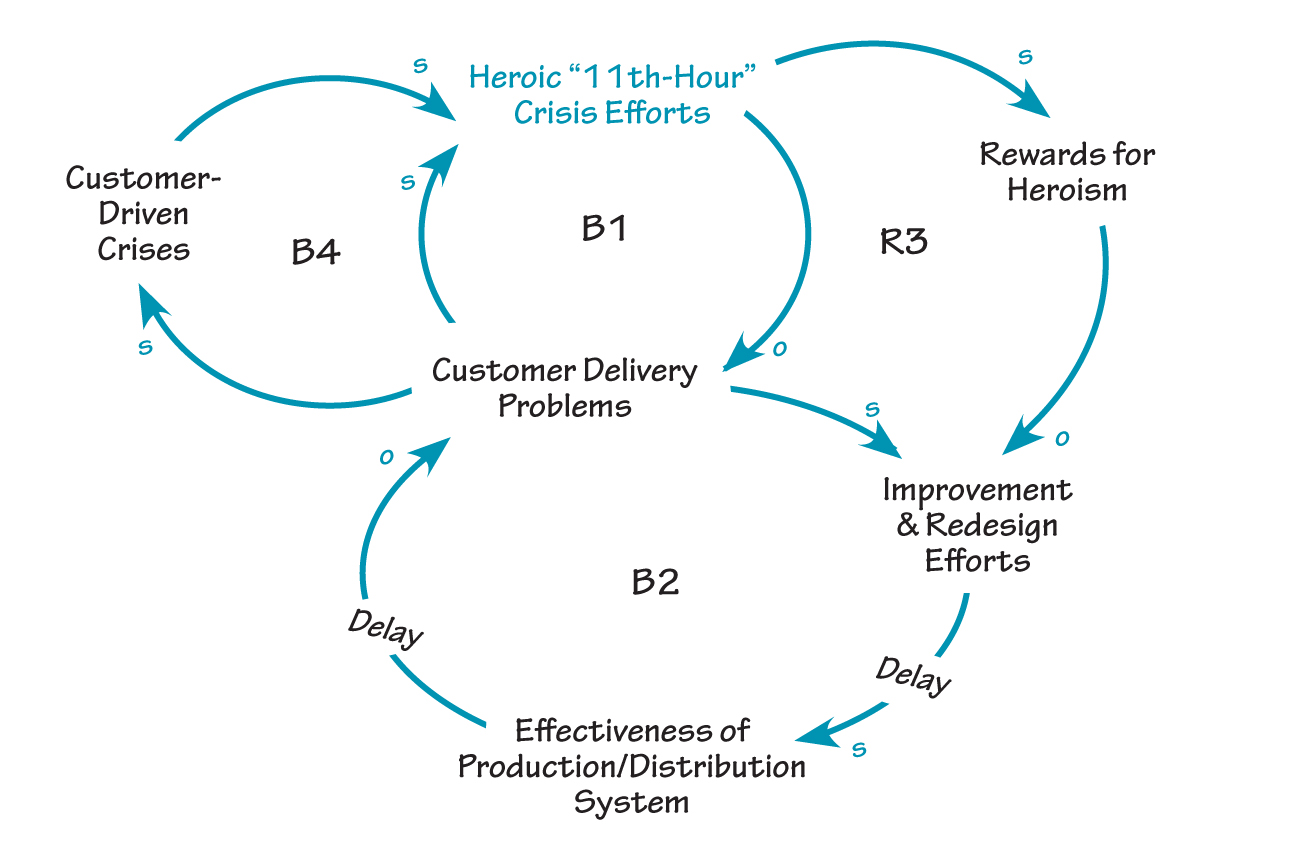
At Bijou, customer problems were solved with heroic “11th-Hour” efforts (B1) rather than with improvements in the production/distribution system (B2). Over time, people at Bijou “learned” that heroism is rewarded, which reduced their willingness and ability to address system-wide problems and increased the company’s dependence on heroic efforts (R3). One negative side-effect of Bijou’s “heroism” attitude was that customers were taking problem situations and escalating them to crises in order to get the company’s attention (B4).
5. Going Deeper™ into the Issues
Once you have a reasonably good theory of what is happening, it is time to take a deeper look at the underlying issues in order to move from understanding to action. There are four areas you should clarify:
- Purpose of the System. Ask yourself, “In the larger context, what do we really want here?”
- Mental Models. Begin the exploration of mental models by adding “thought bubbles” to those links in the diagram that represent choices being made (see “Mental Models and Systems Thinking: Going Deeper into Systemic Issues,” V23N5, June/July 2012).
- The Larger System. Add links and loops to enrich the story and connect the relationships to the larger system.
- Personal Role. Acknowledge and clarify your own role in the situation.
For example, when the people at Bijou looked at the larger system, they wondered what role their customers played in the system. They theorized that customers were taking problem situations and escalating them into crises in order to get the company’s attention (B4).
6. Plan an Intervention
When planning an intervention, use your knowledge of the system to design a solution that will structurally change it to produce the results you want. This might take the form of adding a new link or loop that will produce desirable behavior, breaking a link or loop that produces undesirable behavior, or a combination of the two. The most powerful interventions often involve changing the thinking of the people involved in the system.
At Bijou, the key to change was realizing that the problems were largely self-inflicted. They realized that they had to make progress on production/distribution system improvements while still doing enough fire-fighting to keep things afloat. In the longer term, they would need to change the reward systems that promoted heroic behavior. They also recognized the need to sustain the improvement efforts even when the pressure came off—otherwise the problems would be back again soon.
Part of a Cycle
Even as systems thinkers, it is easy to fall back into a linear process. But learning is a cycle—not a once-through process with a beginning and an end. Once you have designed and tested an intervention, it is time to shift into the active side of the learning cycle. This process includes taking action, seeing the results, and then coming back to examine the outcomes from a systemic perspective.
Michael Goodman is an internationally recognized speaker, author, and practitioner in the fields of systems thinking, organizational learning and change, and leadership.
Richard Karash is a founding trustee of the Society for Organizational Learning, a founding member of the SoL Coaching Community of Practice, and a co-creator of “Coaching from a Systems Perspective.”
Editorial support for this article was provided by Colleen Lannon.
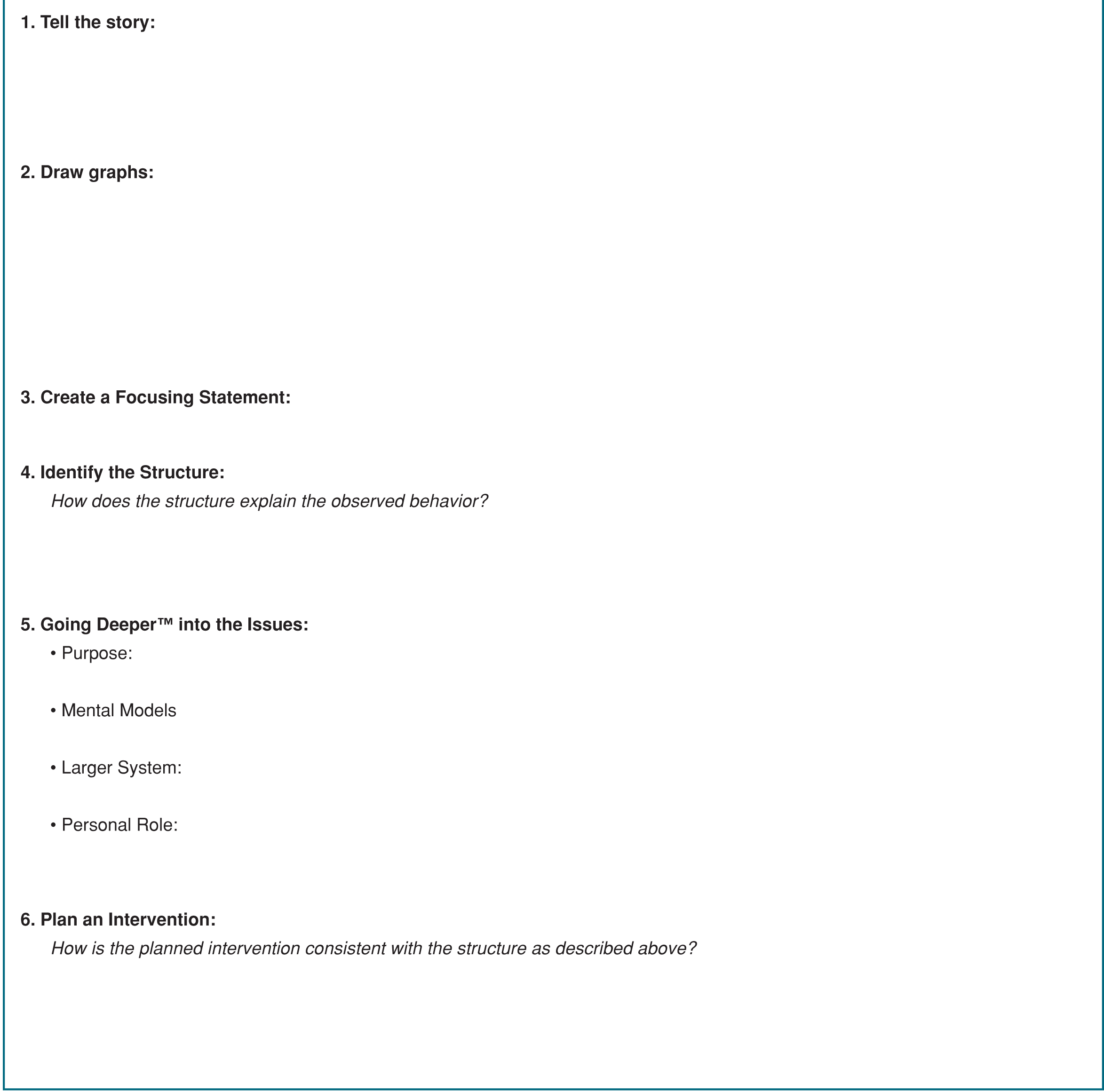
Related Articles
Moving from blame to accountability.
When something goes wrong in an organization, the first question that is often posed is, “Whose fault is…
The “Thinking” in Systems Thinking: How Can We Make It Easier to Master?
Despite significant advances in personal computers and systems thinking software over the last decade, learning to apply systems…
Systems Thinking: What, Why, When, Where, and How?
If you’re reading The Systems Thinker®, you probably have at least a general sense of the benefits of…
From Key Success Factors to Key Success Loops
Many of us are familiar with the following drill: Corporate pushes a new program, and each department must…
Sign up to stay in the loop
Receive updates of new articles and save your favorites..
- First Name *
- Last Name *
- Password * Enter Password Confirm Password
How to master the seven-step problem-solving process
In this episode of the McKinsey Podcast , Simon London speaks with Charles Conn, CEO of venture-capital firm Oxford Sciences Innovation, and McKinsey senior partner Hugo Sarrazin about the complexities of different problem-solving strategies.
Podcast transcript
Simon London: Hello, and welcome to this episode of the McKinsey Podcast , with me, Simon London. What’s the number-one skill you need to succeed professionally? Salesmanship, perhaps? Or a facility with statistics? Or maybe the ability to communicate crisply and clearly? Many would argue that at the very top of the list comes problem solving: that is, the ability to think through and come up with an optimal course of action to address any complex challenge—in business, in public policy, or indeed in life.
Looked at this way, it’s no surprise that McKinsey takes problem solving very seriously, testing for it during the recruiting process and then honing it, in McKinsey consultants, through immersion in a structured seven-step method. To discuss the art of problem solving, I sat down in California with McKinsey senior partner Hugo Sarrazin and also with Charles Conn. Charles is a former McKinsey partner, entrepreneur, executive, and coauthor of the book Bulletproof Problem Solving: The One Skill That Changes Everything [John Wiley & Sons, 2018].
Charles and Hugo, welcome to the podcast. Thank you for being here.
Hugo Sarrazin: Our pleasure.
Charles Conn: It’s terrific to be here.
Simon London: Problem solving is a really interesting piece of terminology. It could mean so many different things. I have a son who’s a teenage climber. They talk about solving problems. Climbing is problem solving. Charles, when you talk about problem solving, what are you talking about?
Charles Conn: For me, problem solving is the answer to the question “What should I do?” It’s interesting when there’s uncertainty and complexity, and when it’s meaningful because there are consequences. Your son’s climbing is a perfect example. There are consequences, and it’s complicated, and there’s uncertainty—can he make that grab? I think we can apply that same frame almost at any level. You can think about questions like “What town would I like to live in?” or “Should I put solar panels on my roof?”
You might think that’s a funny thing to apply problem solving to, but in my mind it’s not fundamentally different from business problem solving, which answers the question “What should my strategy be?” Or problem solving at the policy level: “How do we combat climate change?” “Should I support the local school bond?” I think these are all part and parcel of the same type of question, “What should I do?”
I’m a big fan of structured problem solving. By following steps, we can more clearly understand what problem it is we’re solving, what are the components of the problem that we’re solving, which components are the most important ones for us to pay attention to, which analytic techniques we should apply to those, and how we can synthesize what we’ve learned back into a compelling story. That’s all it is, at its heart.
I think sometimes when people think about seven steps, they assume that there’s a rigidity to this. That’s not it at all. It’s actually to give you the scope for creativity, which often doesn’t exist when your problem solving is muddled.
Simon London: You were just talking about the seven-step process. That’s what’s written down in the book, but it’s a very McKinsey process as well. Without getting too deep into the weeds, let’s go through the steps, one by one. You were just talking about problem definition as being a particularly important thing to get right first. That’s the first step. Hugo, tell us about that.
Hugo Sarrazin: It is surprising how often people jump past this step and make a bunch of assumptions. The most powerful thing is to step back and ask the basic questions—“What are we trying to solve? What are the constraints that exist? What are the dependencies?” Let’s make those explicit and really push the thinking and defining. At McKinsey, we spend an enormous amount of time in writing that little statement, and the statement, if you’re a logic purist, is great. You debate. “Is it an ‘or’? Is it an ‘and’? What’s the action verb?” Because all these specific words help you get to the heart of what matters.
Want to subscribe to The McKinsey Podcast ?
Simon London: So this is a concise problem statement.
Hugo Sarrazin: Yeah. It’s not like “Can we grow in Japan?” That’s interesting, but it is “What, specifically, are we trying to uncover in the growth of a product in Japan? Or a segment in Japan? Or a channel in Japan?” When you spend an enormous amount of time, in the first meeting of the different stakeholders, debating this and having different people put forward what they think the problem definition is, you realize that people have completely different views of why they’re here. That, to me, is the most important step.
Charles Conn: I would agree with that. For me, the problem context is critical. When we understand “What are the forces acting upon your decision maker? How quickly is the answer needed? With what precision is the answer needed? Are there areas that are off limits or areas where we would particularly like to find our solution? Is the decision maker open to exploring other areas?” then you not only become more efficient, and move toward what we call the critical path in problem solving, but you also make it so much more likely that you’re not going to waste your time or your decision maker’s time.
How often do especially bright young people run off with half of the idea about what the problem is and start collecting data and start building models—only to discover that they’ve really gone off half-cocked.
Hugo Sarrazin: Yeah.
Charles Conn: And in the wrong direction.
Simon London: OK. So step one—and there is a real art and a structure to it—is define the problem. Step two, Charles?
Charles Conn: My favorite step is step two, which is to use logic trees to disaggregate the problem. Every problem we’re solving has some complexity and some uncertainty in it. The only way that we can really get our team working on the problem is to take the problem apart into logical pieces.
What we find, of course, is that the way to disaggregate the problem often gives you an insight into the answer to the problem quite quickly. I love to do two or three different cuts at it, each one giving a bit of a different insight into what might be going wrong. By doing sensible disaggregations, using logic trees, we can figure out which parts of the problem we should be looking at, and we can assign those different parts to team members.
Simon London: What’s a good example of a logic tree on a sort of ratable problem?
Charles Conn: Maybe the easiest one is the classic profit tree. Almost in every business that I would take a look at, I would start with a profit or return-on-assets tree. In its simplest form, you have the components of revenue, which are price and quantity, and the components of cost, which are cost and quantity. Each of those can be broken out. Cost can be broken into variable cost and fixed cost. The components of price can be broken into what your pricing scheme is. That simple tree often provides insight into what’s going on in a business or what the difference is between that business and the competitors.
If we add the leg, which is “What’s the asset base or investment element?”—so profit divided by assets—then we can ask the question “Is the business using its investments sensibly?” whether that’s in stores or in manufacturing or in transportation assets. I hope we can see just how simple this is, even though we’re describing it in words.
When I went to work with Gordon Moore at the Moore Foundation, the problem that he asked us to look at was “How can we save Pacific salmon?” Now, that sounds like an impossible question, but it was amenable to precisely the same type of disaggregation and allowed us to organize what became a 15-year effort to improve the likelihood of good outcomes for Pacific salmon.
Simon London: Now, is there a danger that your logic tree can be impossibly large? This, I think, brings us onto the third step in the process, which is that you have to prioritize.
Charles Conn: Absolutely. The third step, which we also emphasize, along with good problem definition, is rigorous prioritization—we ask the questions “How important is this lever or this branch of the tree in the overall outcome that we seek to achieve? How much can I move that lever?” Obviously, we try and focus our efforts on ones that have a big impact on the problem and the ones that we have the ability to change. With salmon, ocean conditions turned out to be a big lever, but not one that we could adjust. We focused our attention on fish habitats and fish-harvesting practices, which were big levers that we could affect.
People spend a lot of time arguing about branches that are either not important or that none of us can change. We see it in the public square. When we deal with questions at the policy level—“Should you support the death penalty?” “How do we affect climate change?” “How can we uncover the causes and address homelessness?”—it’s even more important that we’re focusing on levers that are big and movable.
Would you like to learn more about our Strategy & Corporate Finance Practice ?
Simon London: Let’s move swiftly on to step four. You’ve defined your problem, you disaggregate it, you prioritize where you want to analyze—what you want to really look at hard. Then you got to the work plan. Now, what does that mean in practice?
Hugo Sarrazin: Depending on what you’ve prioritized, there are many things you could do. It could be breaking the work among the team members so that people have a clear piece of the work to do. It could be defining the specific analyses that need to get done and executed, and being clear on time lines. There’s always a level-one answer, there’s a level-two answer, there’s a level-three answer. Without being too flippant, I can solve any problem during a good dinner with wine. It won’t have a whole lot of backing.
Simon London: Not going to have a lot of depth to it.
Hugo Sarrazin: No, but it may be useful as a starting point. If the stakes are not that high, that could be OK. If it’s really high stakes, you may need level three and have the whole model validated in three different ways. You need to find a work plan that reflects the level of precision, the time frame you have, and the stakeholders you need to bring along in the exercise.
Charles Conn: I love the way you’ve described that, because, again, some people think of problem solving as a linear thing, but of course what’s critical is that it’s iterative. As you say, you can solve the problem in one day or even one hour.
Charles Conn: We encourage our teams everywhere to do that. We call it the one-day answer or the one-hour answer. In work planning, we’re always iterating. Every time you see a 50-page work plan that stretches out to three months, you know it’s wrong. It will be outmoded very quickly by that learning process that you described. Iterative problem solving is a critical part of this. Sometimes, people think work planning sounds dull, but it isn’t. It’s how we know what’s expected of us and when we need to deliver it and how we’re progressing toward the answer. It’s also the place where we can deal with biases. Bias is a feature of every human decision-making process. If we design our team interactions intelligently, we can avoid the worst sort of biases.
Simon London: Here we’re talking about cognitive biases primarily, right? It’s not that I’m biased against you because of your accent or something. These are the cognitive biases that behavioral sciences have shown we all carry around, things like anchoring, overoptimism—these kinds of things.
Both: Yeah.
Charles Conn: Availability bias is the one that I’m always alert to. You think you’ve seen the problem before, and therefore what’s available is your previous conception of it—and we have to be most careful about that. In any human setting, we also have to be careful about biases that are based on hierarchies, sometimes called sunflower bias. I’m sure, Hugo, with your teams, you make sure that the youngest team members speak first. Not the oldest team members, because it’s easy for people to look at who’s senior and alter their own creative approaches.
Hugo Sarrazin: It’s helpful, at that moment—if someone is asserting a point of view—to ask the question “This was true in what context?” You’re trying to apply something that worked in one context to a different one. That can be deadly if the context has changed, and that’s why organizations struggle to change. You promote all these people because they did something that worked well in the past, and then there’s a disruption in the industry, and they keep doing what got them promoted even though the context has changed.
Simon London: Right. Right.
Hugo Sarrazin: So it’s the same thing in problem solving.
Charles Conn: And it’s why diversity in our teams is so important. It’s one of the best things about the world that we’re in now. We’re likely to have people from different socioeconomic, ethnic, and national backgrounds, each of whom sees problems from a slightly different perspective. It is therefore much more likely that the team will uncover a truly creative and clever approach to problem solving.
Simon London: Let’s move on to step five. You’ve done your work plan. Now you’ve actually got to do the analysis. The thing that strikes me here is that the range of tools that we have at our disposal now, of course, is just huge, particularly with advances in computation, advanced analytics. There’s so many things that you can apply here. Just talk about the analysis stage. How do you pick the right tools?
Charles Conn: For me, the most important thing is that we start with simple heuristics and explanatory statistics before we go off and use the big-gun tools. We need to understand the shape and scope of our problem before we start applying these massive and complex analytical approaches.
Simon London: Would you agree with that?
Hugo Sarrazin: I agree. I think there are so many wonderful heuristics. You need to start there before you go deep into the modeling exercise. There’s an interesting dynamic that’s happening, though. In some cases, for some types of problems, it is even better to set yourself up to maximize your learning. Your problem-solving methodology is test and learn, test and learn, test and learn, and iterate. That is a heuristic in itself, the A/B testing that is used in many parts of the world. So that’s a problem-solving methodology. It’s nothing different. It just uses technology and feedback loops in a fast way. The other one is exploratory data analysis. When you’re dealing with a large-scale problem, and there’s so much data, I can get to the heuristics that Charles was talking about through very clever visualization of data.
You test with your data. You need to set up an environment to do so, but don’t get caught up in neural-network modeling immediately. You’re testing, you’re checking—“Is the data right? Is it sound? Does it make sense?”—before you launch too far.
Simon London: You do hear these ideas—that if you have a big enough data set and enough algorithms, they’re going to find things that you just wouldn’t have spotted, find solutions that maybe you wouldn’t have thought of. Does machine learning sort of revolutionize the problem-solving process? Or are these actually just other tools in the toolbox for structured problem solving?
Charles Conn: It can be revolutionary. There are some areas in which the pattern recognition of large data sets and good algorithms can help us see things that we otherwise couldn’t see. But I do think it’s terribly important we don’t think that this particular technique is a substitute for superb problem solving, starting with good problem definition. Many people use machine learning without understanding algorithms that themselves can have biases built into them. Just as 20 years ago, when we were doing statistical analysis, we knew that we needed good model definition, we still need a good understanding of our algorithms and really good problem definition before we launch off into big data sets and unknown algorithms.
Simon London: Step six. You’ve done your analysis.
Charles Conn: I take six and seven together, and this is the place where young problem solvers often make a mistake. They’ve got their analysis, and they assume that’s the answer, and of course it isn’t the answer. The ability to synthesize the pieces that came out of the analysis and begin to weave those into a story that helps people answer the question “What should I do?” This is back to where we started. If we can’t synthesize, and we can’t tell a story, then our decision maker can’t find the answer to “What should I do?”
Simon London: But, again, these final steps are about motivating people to action, right?
Charles Conn: Yeah.
Simon London: I am slightly torn about the nomenclature of problem solving because it’s on paper, right? Until you motivate people to action, you actually haven’t solved anything.
Charles Conn: I love this question because I think decision-making theory, without a bias to action, is a waste of time. Everything in how I approach this is to help people take action that makes the world better.
Simon London: Hence, these are absolutely critical steps. If you don’t do this well, you’ve just got a bunch of analysis.
Charles Conn: We end up in exactly the same place where we started, which is people speaking across each other, past each other in the public square, rather than actually working together, shoulder to shoulder, to crack these important problems.
Simon London: In the real world, we have a lot of uncertainty—arguably, increasing uncertainty. How do good problem solvers deal with that?
Hugo Sarrazin: At every step of the process. In the problem definition, when you’re defining the context, you need to understand those sources of uncertainty and whether they’re important or not important. It becomes important in the definition of the tree.
You need to think carefully about the branches of the tree that are more certain and less certain as you define them. They don’t have equal weight just because they’ve got equal space on the page. Then, when you’re prioritizing, your prioritization approach may put more emphasis on things that have low probability but huge impact—or, vice versa, may put a lot of priority on things that are very likely and, hopefully, have a reasonable impact. You can introduce that along the way. When you come back to the synthesis, you just need to be nuanced about what you’re understanding, the likelihood.
Often, people lack humility in the way they make their recommendations: “This is the answer.” They’re very precise, and I think we would all be well-served to say, “This is a likely answer under the following sets of conditions” and then make the level of uncertainty clearer, if that is appropriate. It doesn’t mean you’re always in the gray zone; it doesn’t mean you don’t have a point of view. It just means that you can be explicit about the certainty of your answer when you make that recommendation.
Simon London: So it sounds like there is an underlying principle: “Acknowledge and embrace the uncertainty. Don’t pretend that it isn’t there. Be very clear about what the uncertainties are up front, and then build that into every step of the process.”
Hugo Sarrazin: Every step of the process.
Simon London: Yeah. We have just walked through a particular structured methodology for problem solving. But, of course, this is not the only structured methodology for problem solving. One that is also very well-known is design thinking, which comes at things very differently. So, Hugo, I know you have worked with a lot of designers. Just give us a very quick summary. Design thinking—what is it, and how does it relate?
Hugo Sarrazin: It starts with an incredible amount of empathy for the user and uses that to define the problem. It does pause and go out in the wild and spend an enormous amount of time seeing how people interact with objects, seeing the experience they’re getting, seeing the pain points or joy—and uses that to infer and define the problem.
Simon London: Problem definition, but out in the world.
Hugo Sarrazin: With an enormous amount of empathy. There’s a huge emphasis on empathy. Traditional, more classic problem solving is you define the problem based on an understanding of the situation. This one almost presupposes that we don’t know the problem until we go see it. The second thing is you need to come up with multiple scenarios or answers or ideas or concepts, and there’s a lot of divergent thinking initially. That’s slightly different, versus the prioritization, but not for long. Eventually, you need to kind of say, “OK, I’m going to converge again.” Then you go and you bring things back to the customer and get feedback and iterate. Then you rinse and repeat, rinse and repeat. There’s a lot of tactile building, along the way, of prototypes and things like that. It’s very iterative.
Simon London: So, Charles, are these complements or are these alternatives?
Charles Conn: I think they’re entirely complementary, and I think Hugo’s description is perfect. When we do problem definition well in classic problem solving, we are demonstrating the kind of empathy, at the very beginning of our problem, that design thinking asks us to approach. When we ideate—and that’s very similar to the disaggregation, prioritization, and work-planning steps—we do precisely the same thing, and often we use contrasting teams, so that we do have divergent thinking. The best teams allow divergent thinking to bump them off whatever their initial biases in problem solving are. For me, design thinking gives us a constant reminder of creativity, empathy, and the tactile nature of problem solving, but it’s absolutely complementary, not alternative.
Simon London: I think, in a world of cross-functional teams, an interesting question is do people with design-thinking backgrounds really work well together with classical problem solvers? How do you make that chemistry happen?
Hugo Sarrazin: Yeah, it is not easy when people have spent an enormous amount of time seeped in design thinking or user-centric design, whichever word you want to use. If the person who’s applying classic problem-solving methodology is very rigid and mechanical in the way they’re doing it, there could be an enormous amount of tension. If there’s not clarity in the role and not clarity in the process, I think having the two together can be, sometimes, problematic.
The second thing that happens often is that the artifacts the two methodologies try to gravitate toward can be different. Classic problem solving often gravitates toward a model; design thinking migrates toward a prototype. Rather than writing a big deck with all my supporting evidence, they’ll bring an example, a thing, and that feels different. Then you spend your time differently to achieve those two end products, so that’s another source of friction.
Now, I still think it can be an incredibly powerful thing to have the two—if there are the right people with the right mind-set, if there is a team that is explicit about the roles, if we’re clear about the kind of outcomes we are attempting to bring forward. There’s an enormous amount of collaborativeness and respect.
Simon London: But they have to respect each other’s methodology and be prepared to flex, maybe, a little bit, in how this process is going to work.
Hugo Sarrazin: Absolutely.
Simon London: The other area where, it strikes me, there could be a little bit of a different sort of friction is this whole concept of the day-one answer, which is what we were just talking about in classical problem solving. Now, you know that this is probably not going to be your final answer, but that’s how you begin to structure the problem. Whereas I would imagine your design thinkers—no, they’re going off to do their ethnographic research and get out into the field, potentially for a long time, before they come back with at least an initial hypothesis.

Want better strategies? Become a bulletproof problem solver
Hugo Sarrazin: That is a great callout, and that’s another difference. Designers typically will like to soak into the situation and avoid converging too quickly. There’s optionality and exploring different options. There’s a strong belief that keeps the solution space wide enough that you can come up with more radical ideas. If there’s a large design team or many designers on the team, and you come on Friday and say, “What’s our week-one answer?” they’re going to struggle. They’re not going to be comfortable, naturally, to give that answer. It doesn’t mean they don’t have an answer; it’s just not where they are in their thinking process.
Simon London: I think we are, sadly, out of time for today. But Charles and Hugo, thank you so much.
Charles Conn: It was a pleasure to be here, Simon.
Hugo Sarrazin: It was a pleasure. Thank you.
Simon London: And thanks, as always, to you, our listeners, for tuning into this episode of the McKinsey Podcast . If you want to learn more about problem solving, you can find the book, Bulletproof Problem Solving: The One Skill That Changes Everything , online or order it through your local bookstore. To learn more about McKinsey, you can of course find us at McKinsey.com.
Charles Conn is CEO of Oxford Sciences Innovation and an alumnus of McKinsey’s Sydney office. Hugo Sarrazin is a senior partner in the Silicon Valley office, where Simon London, a member of McKinsey Publishing, is also based.
Explore a career with us
Related articles.
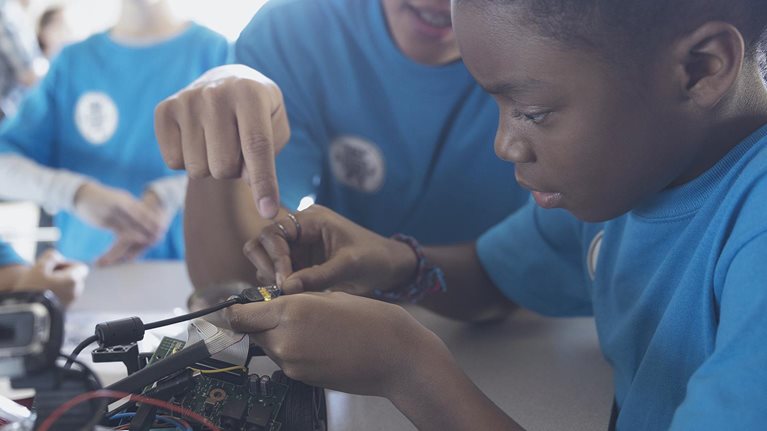
Strategy to beat the odds
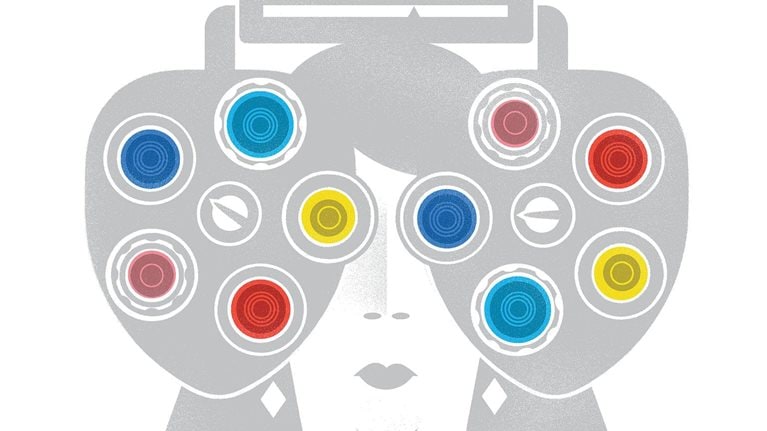
Five routes to more innovative problem solving
Academics & Programs
Faculty & research, ulmer career management center, alumni & friends, news & events.
- Resources for:
- Current Students
- Staff & Faculty Resources
- Recruiters & Employers
- Press & Media
About the College of Business
The UofL College of Business enhances the intellectual and economic vitality of our city, the region, and the broader business world through our academic programs, research, and community outreach activities. We strongly believe that lives improve through entrepreneurship, innovation, critical and rigorous thinking, diverse ideas, and people.
The University of Louisville College of Business offers a wide variety of degree programs to help you accelerate your success and achieve your professional goals. Whether you’re laying a strong foundation for your business career, taking the next big step on your professional journey, or building specialized industry expertise, we’ve got you covered.
UofL College of Business faculty pride themselves on real-world experience and depth of research. They value innovation, critical thinking, and the exchange of new ideas. Our distinguished faculty will help you achieve your goals — before and after graduation.
The Ulmer Career Management Center is a state-of-the-art resource connecting local, regional, and international employers with high-potential College of Business students and alumni.
The UofL College of Business continues to succeed thanks to the commitment and resources of alumni and friends. Learn about our latest initiatives, the impact of our alumni on our mission, and how you can stay involved.
Get up-to-date on the latest news from the College of Business community.
- Staff & Faculty
Systems Thinking: How to Solve Problems So They Stay Solved
From production to customer service and marketing, organizations are made up of a series of interconnected parts. While each function may appear to operate efficiently on its own, a change in just one cog can throw the whole system out of whack. The problems that arise in interconnected organizations can be difficult to solve.
Systems thinking is problem-solving approach that examines the relationships between functions in an organization. Systems thinking is powerful because it enables you to predict the consequences of a potential change. This problem-solving method can also help you eliminate silos, see different viewpoints, and remain focused on the big picture.
Ultimately, systems thinking empowers you to solve problems so that they stay solved. Instead of offering quick-fix solutions that work only in the short term, systems thinking helps you make decisions that benefit your organization in the long run.
You will learn how to:
- Apply systems thinking in the workplace in ways that benefit you and your organization: encouraging innovation, learning from mistakes, and enhancing leadership and management skills.
- Apply the tools of systems thinking to solve a problem.
- Minimize the unintended consequences of major decisions.
Seminar Fee
$430 (includes instruction, seminar manual, refreshments, certificate of completion and parking)
Stay Notified of Upcoming Classes
Interested to learn about our other programs? Check out our other Seminars . Or sign up and we will keep you informed about future educational offerings.
- Search all Louisville
- Search College of Business
Thank you for visiting nature.com. You are using a browser version with limited support for CSS. To obtain the best experience, we recommend you use a more up to date browser (or turn off compatibility mode in Internet Explorer). In the meantime, to ensure continued support, we are displaying the site without styles and JavaScript.
- View all journals
- Explore content
- About the journal
- Publish with us
- Sign up for alerts
- Open access
- Published: 23 August 2024
An integrated coupled oscillator network to solve optimization problems
- Markus Graber ORCID: orcid.org/0000-0002-6736-121X 1 &
- Klaus Hofmann ORCID: orcid.org/0000-0002-6675-0221 1
Communications Engineering volume 3 , Article number: 116 ( 2024 ) Cite this article
58 Accesses
1 Altmetric
Metrics details
- Electrical and electronic engineering
Solving combinatorial optimization problems is essential in scientific, technological, and engineering applications, but can be very time and energy-consuming using classical algorithms executed on digital processors. Oscillator-based Ising machines offer a promising alternative by exploiting the analog coupling between electrical oscillators to solve such optimization problems more efficiently. Here we present the design and the capabilities of our scalable approach to solve Ising and quadratic unconstrained binary optimization problems. This approach includes routable oscillator connections to simplify the time-consuming embedding of the problem into the oscillator network. Our manufactured silicon chip, featuring 1440 oscillators implemented in a 28 nm technology, demonstrates the ability to solve optimization problems in 950 ns while consuming typically 319 μW per node. A frequency, phase, and delay calibration ensures robustness against manufacturing variations. The system is evaluated with multiple sets of benchmark problems to analyze the sensitivity for parameters such as the coupling strength or frequency.
Similar content being viewed by others
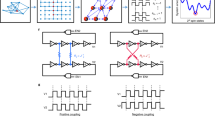
A 1,968-node coupled ring oscillator circuit for combinatorial optimization problem solving

Analog Coupled Oscillator Based Weighted Ising Machine
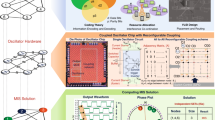
Using synchronized oscillators to compute the maximum independent set
Introduction.
The scaling of semiconductor technologies to smaller, more advanced nodes to increase computing power and energy efficiency is getting more and more difficult with each semiconductor technology step. In addition, the demand for computing power in combination with improved energy efficiency is increasing, particularly due to advances in artificial intelligence training 1 . A part of bridging the gap between desired and available computing power are Ising machines 2 . Those specialized hardware implementations focus on solving optimization problems formulated as the Ising model, which are non-deterministic polynomial-time (NP)-hard and thus require intensive computation power to solve 3 . Researchers are exploring different physical implementations such as quantum computing 4 , optics 5 , 6 , memristors 7 , and spintronics 8 , 9 , 10 to directly employ them for computation in the form of a highly efficient Ising machine. However, such systems are difficult to implement physically and are not yet ready for mass production compared to competing approaches using well-established complementary metal-oxide-semiconductor (CMOS) technologies. Besides specialized digital processors 11 , 12 the class of oscillator-based Ising machines (OIMs), which is usually implemented in CMOS as well, is very promising due to their high speed and energy efficiency. Systems capable of solving problems as fast as 50 ns at a low total power consumption of just 42 mW for a 1968 node system have already been reported 13 . Other systems with varying network topologies have been presented as well 13 , 14 , 15 , 16 , 17 , 18 , 19 . The efficiency and effectiveness of oscillator-based computing are also highlighted by the closely related oscillatory neural networks, which are used for machine learning tasks 20 , 21 , 22 .
Well-known examples of NP-complete optimization problems are the traveling salesman, the knapsack problem, the maximum cut, and the remaining of Karp’s 21 NP-complete problems 23 . Those can be converted within polynomial time to the Ising model 24 . A few exemplary applications for the Ising model are routing 25 , image segmentation 26 , and collaborative filtering 27 . There are many more practical applications in finance, economics, traffic management, computer-aided design, and machine scheduling in a QUBO form 28 , which can be transformed into the Ising model. The Ising model, which was initially introduced to describe the spins of ferromagnetic material 29 , is defined as shown in Eq. ( 1 ). The Hamiltonian H, which is a function of the discrete spins σ i ∈ {+1, −1} shall be minimized. \({J}_{ij} \,\in\, {\mathbb{R}},i\ne j\) are the coefficients for the interaction between spins, while h i acts on just a single spin and gives a bias to the discrete state. In the following, we will refer to the term ∑ 〈 i 〉 h i ⋅ σ i as ‘bias’. The similar QUBO problem form is shown in Eq. ( 2 ), where f Q with \({Q}_{ij}\in {\mathbb{R}}\) shall be minimized by the discrete vector x with binary variables x i ∈ {0, 1}. Both models can be transformed into each other as shown by conversion between x i and σ i in Eq. ( 3 ), where a Q i j x i x j QUBO term gets transformed into the Ising coefficients J i j , h i , and h j . Consequently, we focus on the Ising model in the following since it is more commonly used in the field of OIMs.
The concept of computation using OIMs is outlined in Fig. 1 a. An optimization problem from an application must first be converted into the Ising form. This problem, consisting of discrete variables and weights linking those, is then embedded into the physical oscillator network. Each discrete variable must be mapped to a physical oscillator, which represents the binary states of a discrete variable in its phase angle, being either in-phase or anti-phase ( π phase shifted). Physical coupling circuits between the oscillators realize the weights of the optimization problem. After the successful embedding, the oscillators run and mutually influence their phases, which forms a solution to the optimization problem. Since phases are continuous while combinatorial optimization problems have only discrete states, a sub-harmonic injection locking (SHIL) is used before readout. The SHIL perturbs the oscillators and forces alignment of their phases in discrete groups. Details about the phase dynamics can be found in 30 , 31 , 32 . The phases are read out to assign the discrete states of the corresponding variables σ i based on their position in one of the two by π separated distinct groups. An exemplary problem showing the letter ‘G’, which was computed on the actual chip, is shown in Fig. 1 b.
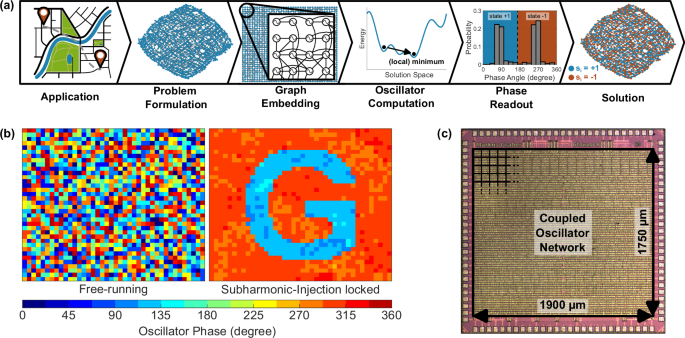
a Conceptual step-by-step procedure of computing with OIM systems. The application problem is formulated in the Ising form. After embedding this problem graph into the OIM-system network, the coupled oscillators naturally strive towards a (local) minimum state in hardware. The solution is finally obtained by reading out the phases. b Exemplary outcome of the computing process in the phase domain for a created illustration problem showing the letter ‘G’ of the chip codename ‘Glowworm’. c Die micrograph of the 1440 coupled oscillator network implemented on a core area of 3.325 mm 2 . The power supply distribution using the topmost layers hides the circuitry below.
Unfortunately, the graph embedding of the problem graph into a hardware connectivity network can be NP-hard as well 33 , 34 . Creating another NP-hard problem to solve the target NP-hard problem is one of the major drawbacks of such computing systems and can become a serious bottleneck of the overall computation. However, the embedding is a general issue of such hardware-based Ising machines with a sparse network, independent of the underlying physical devices. In general, it is sufficient to find just a single valid graph embedding into the available hardware network. Consequently, the hardware network should be designed to simplify the problem embedding to prevent it from becoming the bottleneck of the computation. While all-to-all network connectivity does not require time-consuming embedding, the number of connections \(\frac{n}{2}(n-1)\) scales quadratically with the network size n , making it challenging for large networks. Besides the immense area, the coupling can get difficult. For example, the delay and capacitive load introduced by the numerous connections poses a serious challenge. Embedding approaches often combine multiple physical nodes to form a single discrete variable to increase the connectivity 33 , 35 . However, this adds substantial overhead from the additionally needed physical nodes and their connections and can reduce the solution accuracy 15 , 35 . Hence, we propose a configurable, routable network with strong local connectivity as a trade-off. The idea is, that most of the graph edges should be embedded into the local connectivity. The routing network is just used for the remaining edges, which cannot be embedded locally. While the first edges can usually be easily mapped to the hardware connectivity graph, it gets increasingly difficult to find valid mappings for the remaining edges the more nodes and edges are already mapped to a hardware component. By using the flexible routing connections on demand for these remaining challenging connections, the difficulty can be reduced. Hence, the addition of such routable channels simplifies the embedding and can increase the success rate compared to a local network 36 .
A very similar configurable topology is the field programmable Ising machine (FPIM) proposed by Jagielski et al. 37 . They map boolean satisfiability problems (SATs) to a hardware representation and use a modified open-source field-programmable gate array (FPGA) tool flow to embed such problems in the hardware. This flow enables them to precisely analyze the needed hardware connectivity to embed a given set of optimization problems.
The contribution of this work is as follows: We present a scalable architecture of an OIM system designed for solving Ising and QUBO problems with high accuracy and discuss the analog computing circuitry as well as the routing concept. We show extensive benchmarks solving maximum cut problems of the prototype, which is illustrated in Fig. 1 c. Further experimental measurements highlight the importance of critical parameters like the coupling strength, SHIL strength, and system frequency for a high solution accuracy. Furthermore, the routable connections are evaluated and the calibration methods to compensate for unavoidable manufacturing mismatch as well as varying operating conditions like voltage, process, and temperature are discussed. Although abstract oscillator simulation models are available 38 , 39 , 40 , 41 , they might not consider all non-idealities of real circuits. A more precise transistor-level simulation of the whole network is not feasible because of the excessive simulation runtime. Hence, this work is an experimental approach to investigate and verify the operation of OIMs.
The connectivity of the system network is shown in Fig. 2 a. Each oscillator has a local connectivity to 11 neighbors forming a fixed, sparse network. It connects to the horizontal, vertical, and diagonal neighbors in a 2-dimensional grid, but also to the neighbor of the horizontal and vertical neighbor. The configurable routing network (blue) with the Wilton-style switch blocks 42 provides 4 connections per oscillator to any other. To implement the bias term h i of the Ising model the oscillators can be coupled to a globally distributed phase reference (red). Our 4.6 mm 2 prototype manufactured in a 28 nm technology of a world-leading foundry contains a total of 1440 oscillators and 11,724 coupling elements. 2584 of these connections are freely routable across the whole chip, while the remaining have predefined local connectivity.

a Simplified excerpt of the oscillator network. The local connectivity of a single node is highlighted in black, the routing network with the switch blocks (SB) is blue, and the reference connections for the external bias term are red. The local connectivity of other nodes is shown in gray for better visibility. b Simplified block diagram of an internal node, which represents one discrete variable of the optimization problem. CPL: Coupler Circuit; D2S: Differential-to-single ended converter; DAC: Digital-to-analog converter; PDC: Phase-to-digital converter; ref clk: reference clock; SHIL: Sub-harmonic injection locking c 4-stage differential ring oscillator. d The delay cell of the oscillator including a coarse and fine-tuning for the frequency calibration. e Simplified schematic of an unidirectional coupler stage, which is used back-to-back to establish the bidirectional coupling connection. Each differential pair has a drive (drv) and modulation (mod) input. f Current summation circuit, which combines the currents of each coupler and injects the sum I i n j into the oscillator. g Overall computing and test concept, which is managed by a custom Matlab application, consisting of an field-programmable gate array (FPGA), external clock generation using phase-locked loops (PLLs), delay line ( Δ T), and optional oscilloscope. h Photograph of the test board with the pluggable chip carrier board in the center.
Node Implementation
The block diagram of an oscillator node representing a discrete variable σ i is shown in Fig. 2 b. The central element is the ring oscillator shown in Fig. 2 c operating at a frequency of 100 MHz, which keeps signal propagation delays to neighboring oscillators still small compared to the oscillation period. Due to the necessary graph embedding and data transmission for the OIM-system configuration, we think that higher oscillation frequencies will have limited benefit for the overall computation. We use a differential delay cell design shown in Fig. 2 d, which was empirically optimized for its high sensitivity to coupling currents. The small number of 4 delay cells provides a good sensitivity but still sustains oscillation at strong injected currents reliably. The differential architecture provides phase shifts of π by swapping both differential signals. This is used to implement in-phase (0-phase difference) and anti-phase ( π -phase difference) coupling. Additionally, the higher power supply rejection of differential designs reduces unwanted coupling via the power supply. However, a differential to single-ended converter provides the oscillator signal for the all-digital phase-to-digital converter (PDC) 43 . Although just a binary phase measurement would be sufficient for the Ising model, a 4-bit resolution is implemented to investigate the OIM computation in more detail. The sensitive oscillator waveform is buffered to avoid loading effects 44 . The configurable coupler circuits convert the phase differences between the oscillators into a current, which is summed up and injected into the oscillator to change its phase. An all-transistor coupler design is proposed in this work to avoid large resistors or capacitors. As shown in Fig. 2 e it consists of two differential pairs to modulate the injected current based on the phase difference. Swapping the input signals implements the sign of the weights by coupling in-phase or anti-phase. By setting the bias current via an individual 4-bit digital-to-analog converter (DAC) for each coupler, the weight coefficients of the optimization problem are set. The current summation circuit as shown in Fig. 2 f accumulates the current of all couplers for injection into the oscillator, while its self-biasing stabilizes the common mode voltage level. In combination with the output buffer of the oscillator, which mitigates the capacitive loading caused by the connected couplers, several coupling connections can be simply added to achieve the desired connectivity. To implement the bias term h i , we couple the oscillators to the already available reference clock, which adds just a small area overhead. The SHIL-injector gradually applies the perturbating SHIL signal to gently freeze the oscillator phase and therefore discretize the oscillator phases in the two spin groups. This ensures, that the coupled oscillators try to minimize the Ising Hamiltonian 30 . Based on the experimental evaluation of our previous generation with adjustable ramps, we decided to ramp up the SHIL in 8 linear steps, which are increased after each nominal oscillation period.
The routing enables the connection of two arbitrary oscillators on the chip together if suitable routing tracks are not already occupied. When transmitting a signal through the routing network, it gets inevitably delayed by a few hundred picoseconds up to a few nanoseconds depending on the distance. This delay becomes particularly relevant at the oscillation period of 10 ns. The received time-delayed signal creates a perceived phase lag at the distant oscillator, that does not match the actual phase of the receiver. When coupled, the oscillators will react based on this perceived phase potentially causing a runaway situation and a frequency shift. To address this, we have introduced a delay compensation scheme, the design and simulation of which are discussed in our previous work 45 . This method essentially transmits the oscillator signal of the previous stage, which can be seen as the oscillator waveform with a “negative” delay. An adjustable delayline supplements the propagation delay through the routing network to match that “negative” delay so that the received oscillator waveform is time synchronous with the actual transmitting oscillator. So, the perceived phase at the receiving oscillator matches the phase of the transmitter. However, the phase dynamics of the coupling are altered, because any response is perceived at the receiver with a full period delay. Because the propagation delay heavily depends on the traveled distance but also on temperature, process, and supply voltage, the optimal delayline setting is obtained using a calibration, which is discussed later in the calibration section.
Computing & benchmark setup
The overall system concept of our computing setup is shown in Fig. 2 g and its physical realization is depicted in Fig. 2 h. The OIM chip just requires external clocks and an FPGA, which could be in general integrated on the same die as well. The data transfer and generation of the timing signals are handled by that FPGA, which interfaces with a computer. Matlab scripts handle the test management, OIM configuration, timing scheduling, and analysis of the results. Four off-chip drivers allow us to investigate and verify the behavior of selected oscillators using an external oscilloscope, which is not needed for normal computing operation. External bias voltages are used to adjust the oscillator frequency, SHIL-injection strength, and global coupling strength. A single computation takes in total 950 ns. The first 200 ns are used to let the oscillators couple. Then the SHIL strength is ramped up to gradually force the coupled oscillators into the two phase groups, which takes 182 ns including a small margin for stabilization. Lastly, the phase measurement to obtain the computed result takes 568 ns. The energy consumption per computed problem varies between 404 nJ and 466 nJ across the individual problems of the later discussed ‘difficult’ benchmark set. A single oscillator including its periphery has a measured power consumption of 113.3 μW and a coupling connection needs approximately 23 μW.
To evaluate the accuracy, we solve randomly generated benchmark problems and compare them against the best-known solution. Multiple network topologies of varying difficulty are tested, which are sketched together with the histograms in Fig. 3 . The outcome of an OIM computation using our chip depends on the random initial states and might be affected by noise, so that the computed solution varies randomly when computing the same problem again. As shown in Fig. 3 a, we solved each problem 500 times and noted the average (red) and best (blue) solution. The computed cut is normalized with the best-known solution, which was obtained using the commercially available software Gurobi 46 . Each of the 500 runs per problem exhibits a unique solution, where at least 6.4% of states are different compared to every other solution of the same problem. When running the oscillators without any mutual interaction, only 0.18% of oscillators show a weak correlation between their neighbors. Furthermore, no auto-correlation between the previous phases of an oscillator can be detected after roughly 1200 ns.
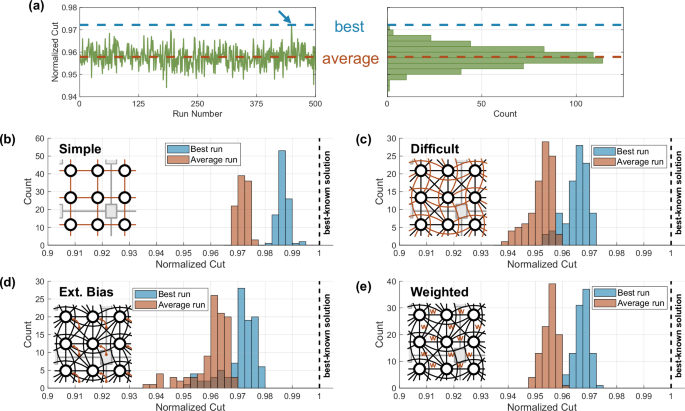
Each problem set contains 100 problems, which are repeatedly solved 500 times each. a Example of solving the same problem repeatedly 500 times to record the best and average cut. b ‘Simple’ 47 benchmark set with horizontal and vertical neighbors. c ‘Difficult’ 48 benchmark set using all 11 available local neighbors. d ‘Bias’ 49 benchmark set which additionally includes the external bias term h i respectively Q i i . e ‘Weighted’ 50 benchmark set, which additionally uses weights with random 4-bit resolution for all edges.
The ‘simple’ 47 set shown in Fig. 3 b contains only connections to vertical and horizontal neighbors on a two-dimensional grid (0.27% of all-to-all connectivity). Since all weights of a maximum cut problem have a positive weight of 1, a checkerboard pattern is a maximum solution, with the cut being the sum of all edge weights. While such a problem might be simple for an iterative algorithm, the parallel nature of OIM systems does not benefit much from this structure. However, the optimum solution of a checkerboard pattern satisfies all coupling connections, which makes this problem less difficult for OIMs than the others. The average cut reaches more than 96.8% of the best-known solution. The ‘difficult’ 48 set in Fig. 3 c uses all available local connections (0.73% of all-to-all connectivity) while keeping the weights at positive 1. The denser local connectivity makes it more difficult to solve since there is no contention-free solution anymore, which satisfies all coupling connections. To emphasize the higher difficulty, computing the reference solution using the commercial software Gurobi 46 for the ‘difficult’ set took on average 10,000x longer than the ‘simple’ set. However, this is just an indication and no proper measurement of difficulty. As expected, the solution accuracy is worse with an average normalized cut of better than 94%. The ‘bias’ 49 set in Fig. 3 d adds the bias terms h i to the structure of the ‘difficult’ problem set, which shows no accuracy degradation from the added bias term. While the previous problems have a fixed weight of +1, the ‘weighted’ 50 set tests the same topology with the full 4-bit weight resolution in Fig. 3 e. The performance is very similar to the ‘bias’ benchmark set. Interestingly the spread between individual problems is reduced. The parameters like the coupling strength were individually tuned for all four shown benchmark sets to achieve the best accuracy.
Experiments & optimization
Figure 4 a illustrates the impact of the coupler strength, which can be globally scaled using an external bias voltage, on the computing performances by evaluating the ‘simple’, ‘difficult’, and ‘weighted’ benchmark sets at different coupling strengths. A distinct range for the coupling strength exists, which achieves the best performance but depends on the benchmark problem set respectively problem connectivity. Figure 4 b graphically illustrates the impact of the coupling strength using the already known example from Fig. 1 b. A weak coupling strength causes multiple local areas, which are solved in themselves correctly, but exhibit a distinct boundary to other such local areas. This effect is much less pronounced at higher coupling strengths, but still relevant around the best coupling strength. At higher coupling strengths, the forming of such local areas is less likely, but faulty spots appear, which couple in an undesired phase despite the clear coupling inputs from the neighbors. So, the coupling strength and SHIL can be thought of as the equivalent of an annealing schedule, which is frequently used in simulated annealing 51 . We suppose, that similar mechanisms considerably reduce the accuracy of the OIM for most problems. It should be emphasized, that the choice of coupling strength is very system and problem-specific. Therefore, we recommend that those parameters are not fixed by design, but remain adjustable during operation. Figure 4 c shows a sweep over the reference clock frequency, where the SHIL frequency is exactly double that value. The best results are achieved for a frequency between 80 and 90 MHz, which is 10% to 20% lower than the actual oscillator free-running frequency. To assess the effectiveness of routable connections, which have slightly different phase dynamics due to their inevitable routing delay with compensation scheme 45 , a comparison is provided in Fig. 4 d. A reduced 10 × 10 network size ‘simple’ benchmark set was solved with routable connections and for comparison using local connections. While the local couplings find the best-known solution for all problems, the routing only for 52 out of the 100 problems. The spread between the problems widens as well, where the worst problem achieves just 85% using the routing network compared to 95% for the local routing.
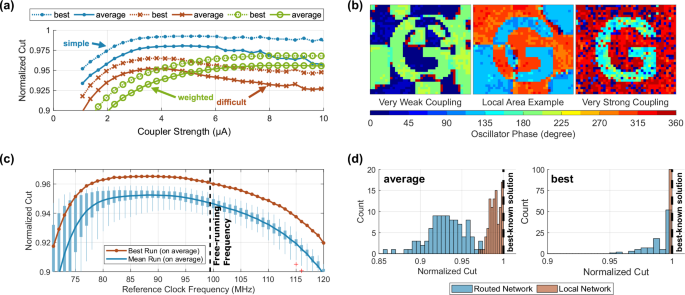
a The measured impact of the coupling strength on the solution accuracy. b Visual example of the impact of weak and strong coupling as well as remaining formation of local areas at the optimal coupling strength. c The experimental measured impact of the reference clock frequency on the solution accuracy. The boxplots indicate the minimum, 25% percentile, 75% percentile, and maximum of the individual problems of the ‘difficult’ set. d Comparison of the solution accuracy between an identical problem solved with a purely local connection and a purely with the routing network. The average accuracy is shown on the left and the best run for each problem is on the right.
Calibration routines
The OIM system is a mixed signal design, where the analog components are responsible for the actual computation. The digital circuitry configures and supports the analog computing core. Unfortunately, random manufacturing mismatches and imperfections influence the sensitive analog circuitry. For example, some transistors might have a higher conductivity than others, causing differences in the frequencies. We employ an on-chip frequency calibration, a Matlab-assisted phase calibration, and a Matlab-assisted routing delay calibration to compensate for such manufacturing mismatch.
Frequency calibration
The frequency of the oscillators is approximately Gaussian distributed with a spread between 75.6 MHz and 119.1 MHz within a silicon die as shown in Fig. 5 a. Since such free-running frequency differences cause phase offsets in the coupled state, which can affect the computation 44 , a fine and coarse calibration adjusts the individual frequencies to the target 100 MHz. After calibration, the standard deviation of the oscillator frequency is reduced from 6.7 MHz to just 0.33 MHz, which improves the computing accuracy on average by approximately 0.7 percentage points. According to our previous chip generation, any frequency deviation less than approximately 1 MHz yields negligible performance gains.
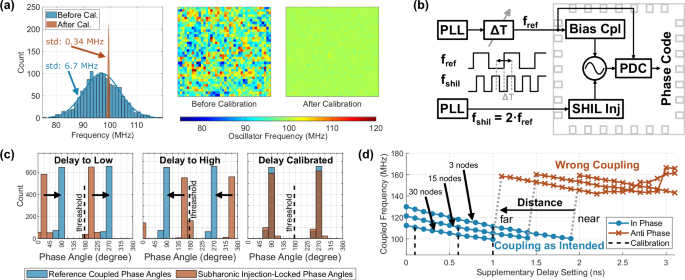
a Distribution of the oscillator free-running frequencies across the chip before and after the calibration. The color-coded maps show the spatial distribution across the whole chip. b Principle of the external delay adjustment for the phase calibration. Bias Cpl: Coupler for spin bias; PDC: phase-to-digital converter; PLL: Phase-locked loop; SHIL Inj: Sub-harmonic injector; Δ T : delay-line; c Alignment procedure of the reference and SHIL-clock by adjusting the external clock delayline Δ T. d Frequency to delay relationship of the routing connections, which is the basis of the routing calibration.
Reference phase calibration
While the weights between two discrete variables ( J i j ⋅ σ i σ j ) just depend on the difference between two discrete states, the bias terms ( h i ⋅ σ i ) depend on just one state, which requires an absolute phase reference to define the actual states. The calibration principle is shown in Fig. 5 b, which adjusts the external delayline Δ T to change the phase between the reference clock and the SHIL clock. The distribution of the reference coupled oscillators, with one half coupled in-phase and the other half anti-phase, is shown in Fig. 5 c (blue). The distribution is only slightly influenced by the operating frequency and coupling strength, as well as supply voltage, temperature, and process. The phase in the SHIL locked states (red) is then adjusted using the delayline Δ T to match the reference coupled phase distribution (blue). Without calibration, the oscillators might not couple as intended by the bias term ( h i ⋅ σ i ) leading to poor accuracy. The phase threshold can be determined by finding the center of both reference phase (blue) groups. The phase angle of the +1 spin group can be clearly identified by the phase of the in-phase coupled oscillators.
Routing delay calibration
Any signals, that are transmitted through the routing network, experience a propagation delay. This is greatly affected by the spatial distance between the connected oscillators as well as supply voltage, temperature, and process variations. Unfortunately, the coupled oscillators are sensitive to that delay leading to changes in their frequency and phase. They either couple as intended or settle in the wrong, opposite phase (shifted by π ). This observed behavior is a probability function depending on the delay instead of a sharp transition. The measurements in Fig. 5 d show the impact of the routing network on the frequency and phase for a pair of coupled oscillators at three different distances. The delay is changed using a delayline with a 100 ps step size, which is implemented for the calibration scheme. The plot is simplified for better clarity by showing the behavior of the majority of oscillators instead of the actual probability density, which is the reason for the distinct transition. While the frequency shows a declining trend with rising delay, the oscillators couple in the wrong phases and increase their frequency by approximately 50% when exceeding a certain threshold. For calibration, we choose a delay setting leading to a coupled frequency closest to the free-running frequency, which still couples as intended with a probability greater than 70%. This delay value, which is separately obtained for all possible routing distances, is stored in a lookup table. Without the calibration (e.g., setting the delaylines to 0 for their minimum delay), the routing channels essentially fail to function, achieving a similar performance as a random number generator. However, the delay setting has a roughly 400 ps tolerance range, which exhibits very similar performance.
Comparison with Other Works
A comparison with other integrated CMOS OIMs is provided in Table 1 . This work offers a versatile trade-off and introduces routable connections between oscillators. It has a competitive number of nodes and edges. Together with Lo et al. 15 , it is the only system to provide the bias term of the Ising model. However, our approach can be used for any network topology instead of requiring an all-to-all connected oscillator. While all-to-all connectivity is superior, they are limited to very small systems with just 30 16 and 48 15 nodes. The routing channels in this work add great flexibility to the network. In combination with the local connectivity, it is well-suited for large, sparse problems. In comparison to our predecessor 19 , we managed to maintain the high solution accuracy despite increasing the number of nodes and edges while reducing the power and area per node.
We presented a scalable approach for OIMs capable of solving optimization problems in Ising as well as QUBO formulation. The critical characteristic of such OIM systems is the network connectivity. For solving real-world optimization problems, the network should be as large as possible having tens of thousands of nodes with as many connections as possible. However, the quadratic increase of connections in all-to-all networks makes a circuit implementation unfeasible for large systems due to the immense area demand. Our approach unites these two opposing requirements by introducing flexible, routable connections between oscillators. This is a good trade-off for sparse optimization problems as it provides every possible connection between nodes while avoiding hardwiring every connection to save area. Additionally, the flexibility of the routing simplifies the computationally intensive embedding of the optimization problem into the OIM network, which can potentially exceed the computation time of the actual problem.
Our fabricated chip demonstrates the effectiveness of OIMs by solving max-cut problems within 950 ns while consuming an average power of 460.3 mW. The computed solutions reach on average at least 94% of the optimum and even more when selecting the best runs. The two major design challenges are area efficiency and random device variation from manufacturing. To be as compact as possible, we proposed a fully transistor-based coupler circuit with weights set by simple current DACs to avoid large, area-intensive resistors and capacitors. A time-multiplexing of the DACs, which occupy 12.8% of the core area, could further save area. To deal with process variation and mismatch of individual devices, we use calibration schemes and fine-tune parameters such as coupling strength and SHIL frequency. Our experiments emphasize that good performance is reached in just a narrow range of the coupling strength, which, however, shifts with the optimization problem topology. The oscillator frequency calibration increases the accuracy by approximately 0.7 percentage points. A software-based phase angle calibration ensures correct operation of the bias term ‘ h i ’ of the Ising model, which is area-efficiently implemented utilizing the already distributed reference clock for the phase measurement. The calibration of the routing delay is crucial. Without it, the network is not usable and just as effective as a random number generator. Unfortunately, despite the compensation scheme including calibration, the routing connections exhibit lower performance due to the unavoidable propagation delay than native local connections between neighboring oscillators.
A calibration scheme for the coupling weights might further increase the accuracy of OIMs. Additionally, a digital post-processing of the measured phases could improve the performance as well. While our approach is well suited for large, sparse optimization problems, we think that large and dense problems might likely not be feasible for OIMs due to the high amount of needed oscillators and coupling links. Instead, hybrid approaches, which could partition a large graph into smaller sections to be solved by OIMs, might combine the advantages of OIMs with digital processors.
Chip design
The chip was designed in a 28 nm node of a world-leading semiconductor foundry. A 4.6 mm 2 silicon chip was manufactured using the mini@sic program of the Europractice IC service. The latest process design kit from the foundry was utilized, providing compact models for simulation. The design uses regular core devices operating at 0.9 V with different thresholds. Cadence Virtuoso version IC6.1.8 was used for schematic and layout entry. Cadence Spectre 21.1.0 was used for transistor-level circuit simulations. Siemens Calibre nmDRC, nmLVS, and PEX version v2020.4_15.9 are used for layout rule checking and parasitic extraction. The digital control logic was synthesized utilizing the foundry-provided standard cells with Synopsys Design Version R-2020.09-SP2 and Cadence Innovus 21.10 for the place-and-route. More details about the overall system implementation can be found in our previous work 45 , 52 .
Delay-line calibration
The delayline calibration is explained in detail in our separate paper 45 . For convenience, the principle is outlined in Fig. 6 . As shown in Fig. 6 a the internal waveforms of the oscillator are phase-shifted. So the output at ‘4’ is a delayed version of the previous stage at ‘3’. The output of this previous stage is transmitted through the routing network as shown in Fig. 6 b. The unavoidable delay T r o u t i n g is supplemented with the adjustable delayline Δ T . So, the received phase at oscillator B (point ‘3’) can be aligned with the actual output of oscillator A (point ‘4’) to minimize the skew T s k e w . Since T s k e w cannot directly be measured and varies with the operating conditions, the discussed calibration is performed.
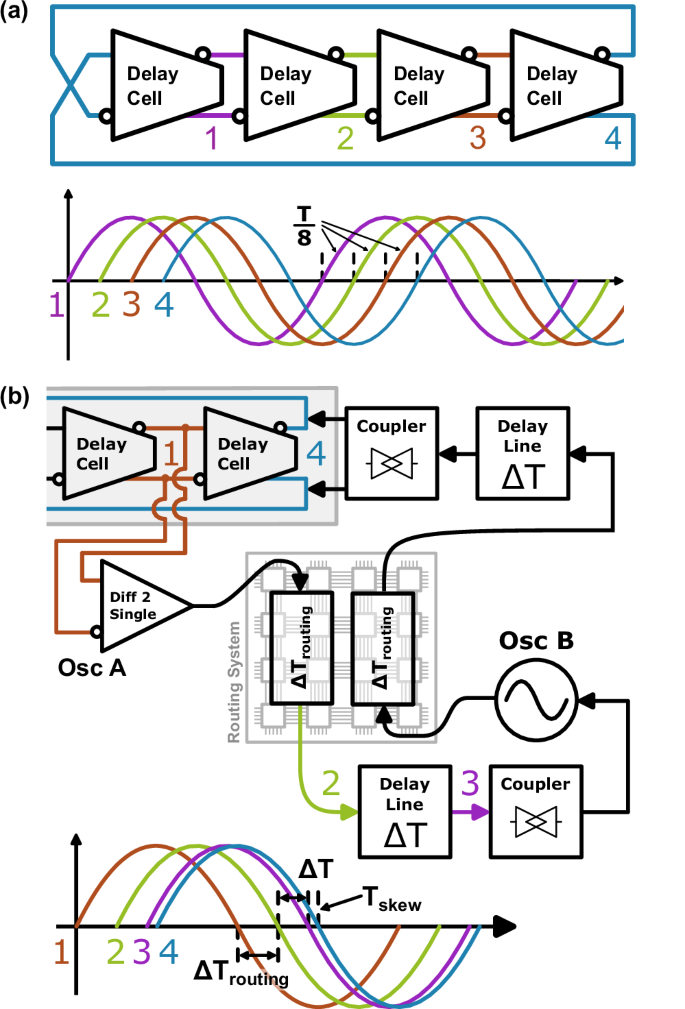
a Phase shift internal of the oscillator. b Compensation scheme showing the transmitting of a signal from oscillator (Osc) A to B. The unavoidable routing delay T r o u t i n g is supplemented by the adjustable delayline Δ T so that a small skew T s k e w is reached at the receiving oscillator B.
Chip reconfigurability & external adjustments
Every local coupling connection on the chip can be configured independently. Every weight is set using a 4-bit DAC value and an additional bit to select between in-phase and anti-phase coupling. The same applies to the routing connections, which have additionally a 5-bit word for the delayline with approximately 100 ps steps. The coupler for the bias term h i is similar, but has 5-bit resolution and has four times the maximum strength of the regular J i j connections. Every switch of the switch block and the switches to connect the oscillator with the routing network can be individually enabled/disabled. The algorithm to configure these switches is mentioned below in section ‘Path Routing’.
External analog bias voltages are internally converted into bias currents using resistors. So, the coupling strength, the SHIL-strength, and the oscillator frequency can be externally set. Furthermore, the SHIL frequency can be set with the external phase-locked loop (PLL). The timing to control the operating states (enable oscillator couple, apply the SHIL signal, phase measurement, oscillator measurement, frequency calibration) is triggered by the external FPGA (Fig. 2 g) on the test printed circuit board (PCB) (Fig. 2 h).
Experimental results
The dies with 108 pads, whereof 67 pads are for power supply and bias voltages, were wire-bonded into a QFN-64 package and soldered on a pluggable carrier board. The presented results are from die number 1, the first one we have soldered on a carrier board without any selection. The benchmarks were conducted with the other 9 chips as well. The test PCB contains all circuitry for the experiments, just an external power supply was used additionally. A Matlab script coordinates the testing by selecting optimization problems, generating the configuration bitfiles for loading the problem into the OIM chip, and configuring the test PCB. The interface between the computer and FPGA limits the maximum data throughput to 12 Mbit in our experiments. Therefore, the OIM system is disabled during the configuration data transfer from the computer to the chip. During transmission of the measured phase data, the oscillators are kept frozen in SHIL-lock and stay in the same phase for sufficient SHIL strengths, which was validated regularly. We have no control over the initial phases at the start of the computation. All experiments were conducted at room temperature between 20 and 25 °C. The presented calibration data is averaged over 500 samples per oscillator. The routing frequency-delay behavior in Fig. 5 d is the averaged behavior of 34 oscillator pairs sampled 500 times each.
G-Letter example problem
The example of the ‘G’ is generated from a black-and-white image of the letter G with identical resolution as the OIM network (40 × 36), so that each pixel corresponds to one oscillator. It is iterated through all pixels in the image and the coupling connections are set based on the color of the direct horizontal, vertical, and diagonal pixel neighbors. If the color of the neighbor pixel is identical, a connection between the corresponding oscillators to couple in-phase is added. If the color of the corresponding neighbor pixel is the opposite, then an anti-phase coupling connection is inserted. Overall, the underlying problem is of a king’s graph structure with all connections enabled, where the sign is determined by the color of the root image. By construction, all oscillators can couple in phases as intended, which is the optimum solution.
Phase Threshold
The threshold to distinguish between the spins σ i = +1 and σ i = − 1 state for all shown results was determined by testing all 16 possible thresholds (resolution of the phase angle by the 4-bit PDC) and selecting the threshold with the best Ising Hamiltonian. We have chosen this brute-force method because it ensures a repeatable and reliable calculation of the Ising Hamiltonian independent of any prior calibration or results. The test PCB generates the SHIL clock f s h i l and reference clock f r e f from the same clock signal (see Fig. 2 g) using Analog Devices ADF4351 PLLs. Since the internal oscillator feedback loop within the PLL is used for better jitter performance, we do not have control of the phase between f s h i l and f r e f . Any time the PLL setting is changed, that phase difference and hence the phase threshold changes. For non-experimental usage, one could either determine the threshold just once based on the phase values or use the reference calibration.
Calibrations
The on-chip frequency calibration is conducted at every start of our test system because the previous calibration is lost after a power cycle. The calibration mainly compensates for random manufacturing mismatch and stays similar between calibration cycles. The phase calibration is conducted once at the start-up of the system and when the operating parameters are notably changed. This is the case, if either the PLL configurations are altered or the SHIL strength/coupling strength is considerably changed. The delay settings of the routable connections were calibrated once and the necessary delays depending on the length stored in a lookup table. This table was then used to get the delay settings in all following experiments.
The benchmark problems were randomly generated by a custom Matlab script tailored for our OIM chip network. To ensure that the generated problems follow the intended network topology, a list with all allowed and physically available edges is generated first. Then edges are randomly deleted until the desired network density is reached, which is randomly selected between 30% and 70% of all edges of that chosen topology (‘simple’: 841 to 1963 edges, ‘difficult’: 2285 to 5331 edges, ‘bias’: 2717 to 6339 edges, ‘weighted’: as difficult). No checks for the existence of isolated sub-graphs were applied. The edge weights (if applicable) were randomly chosen from a uniform distribution. We interpreted the problem as a maximum cut similar to other works, which closely follows the Ising Model. We extended the maximum cut to include a bias term, which can be interpreted as an edge from a node to a fixed set. The edge weights of the maximum cut problem are limited to positive only values (transformed into negative J i j coefficients of the Ising model) for better comparability of the obtained cut. Consequently, the obtained cut is limited to the range between 0 and the best-known solution, which we normalize to 1. The sign of the weights in the maximum cut is simply inverted for transformation into the Ising model, so the OIM operates with just anti-phase coupling. Due to the differential design, where the in-phase and anti-phase coupling is achieved by just swapping the inputs to the coupler circuits in its multiplexer, the general coupling behavior stays identical. The best-known solution for each generated problem was obtained using the commercial tool Gurobi 46 . Gurobi version 10.0.3 was executed on an AMD Threadripper Pro 5965WX 24-core processor and 256 GB RAM without any time restriction, which took up to 4.67 hours per problem. The solution was guaranteed to be the optimal solution.
Path routing
The connections through the routing network were configured using Matlab. It is based on a graph representation of the available hardware network, which includes every physical track and switch of the switch block. The ‘shortestpath’ function in Matlab, which internally uses the Dijkstra algorithm, calculates a path to connect the two oscillators. The returned path includes all physical tracks and switches of the switch blocks. These used elements are then marked as occupied so that they are avoided for the following paths. The obtained path is checked to be physically possible and verified to not contain any already occupied tracks. All routing connections of an optimization problem are applied in a sequential order, where the path for each connection only considers the occupied tracks from previous connections. For the routing problems in Fig. 4 d, this approach connects the oscillators in the shortest, direct way.
Power measurement
The test PCB (Fig. 2 h) contains onboard current shunts for every supply and bias voltage rail, which are placed within the feedback loop of the onboard voltage regulator to compensate for the voltage drop over those shunts. A Keysight DAQ970A sequentially scans the voltage drop over the shunt resistors of the bias rails, analog supply, and digital supply. The separate supply rails for the I/O-ring and off-chip driver are excluded from the measurement. The operating state of the coupled as well as SHIL-locked oscillators were extended for multiple seconds to precisely measure the (average) power consumption. The power consumption during the SHIL ramping and phase measurement could not be directly measured due to their short duration but was approximated by the power consumption under SHIL lock. Additionally, the time and energy for writing the configuration data in the chip and sending the phase data out of the chip are omitted. The power consumption varies substantially with the optimization problem since they influence the amount of enabled oscillators and couplers. Thus, we recorded the power consumption for each problem of the ‘difficult’ set. Additionally, the total power consumption depends on the frequency and the global coupling strength, where the optimal parameters for the optimization problem were used.
Data availability
The used benchmark problems ‘simple’ 47 , ‘difficult’ 48 , ‘weighted’ 49 , and ‘bias’ 50 are available at figshare.
Sevilla, J. et al. Compute trends across three eras of machine learning. In 2022 International Joint Conference on Neural Networks (IJCNN) , 1–8 https://doi.org/10.1109/IJCNN55064.2022.9891914 (2022).
Mohseni, N., McMahon, P. L. & Byrnes, T. Ising machines as hardware solvers of combinatorial optimization problems. Nat. Rev. Phys. 4 , 363–379 (2022).
Article Google Scholar
Kalinin, K. P. & Berloff, N. G. Computational complexity continuum within ising formulation of np problems. Commun. Phys. 5 , 20 (2022).
Johnson, M. W. et al. Quantum annealing with manufactured spins. Nature 473 , 194–198 (2011).
Honjo, T. et al. A coherent ising machine based on a network of 100,000 degenerate optical parametric oscillator pulses. In 2022 IEEE 22nd International Conference on Nanotechnology (NANO) , 405–408 https://doi.org/10.1109/NANO54668.2022.9928658 (2022).
Honjo, T. et al. 100,000-spin coherent ising machine. Sci. Adv. 7 , eabh0952 (2021).
Jiang, M., Shan, K., He, C. & Li, C. Efficient combinatorial optimization by quantum-inspired parallel annealing in analogue memristor crossbar. Nat. Commun. 14 , 5927 (2023).
Yun, C. et al. Electrically programmable magnetic coupling in an ising network exploiting solid-state ionic gating. Nat. Commun. 14 , 6367 (2023).
Dutta, S., Khanna, A. & Datta, S. Understanding the continuous-time dynamics of phase-transition nano-oscillator-based ising hamiltonian solver. IEEE J. Exploratory Solid-State Computational Devices Circuits 6 , 155–163 (2020).
Vaidya, J., Surya Kanthi, R. S. & Shukla, N. Creating electronic oscillator-based ising machines without external injection locking. Sci. Rep. 12 , 981 (2022).
Yamamoto, K. et al. Statica: A 512-spin 0.25m-weight annealing processor with an all-spin-updates-at-once architecture for combinatorial optimization with complete spin-spin interactions. IEEE J. Solid-State Circuits 56 , 165–178 (2021).
Zhang, T., Tao, Q., Liu, B. & Han, J. A review of simulation algorithms of classical ising machines for combinatorial optimization. In 2022 IEEE International Symposium on Circuits and Systems (ISCAS) , 1877–1881 https://doi.org/10.1109/ISCAS48785.2022.9937767 (2022).
Moy, W. et al. A 1,968-node coupled ring oscillator circuit for combinatorial optimization problem solving. Nat. Electron. 5 , 310–317 (2022).
Wang, T., Wu, L. & Roychowdhury, J. Late breaking results: New computational results and hardware prototypes for oscillator-based ising machines. In 2019 56th ACM/IEEE Design Automation Conference (DAC) , 1–2 https://doi.org/10.1145/3316781.3322473 (2019).
Lo, H., Moy, W., Yu, H., Sapatnekar, S. & Kim, C. H. An ising solver chip based on coupled ring oscillators with a 48-node all-to-all connected array architecture. Nat. Electron. 6 , 771–778 (2023).
Bashar, M. K. et al. Experimental demonstration of a reconfigurable coupled oscillator platform to solve the max-cut problem. IEEE J. Exploratory Solid-State Computational Devices Circuits 6 , 116–121 (2020).
Ahmed, I., Chiu, P.-W., Moy, W. & Kim, C. H. A probabilistic compute fabric based on coupled ring oscillators for solving combinatorial optimization problems. IEEE J. Solid-State Circuits 56 , 2870–2880 (2021).
Mallick, A., Bashar, M. K., Truesdell, D. S., Calhoun, B. H. & Shukla, N. Overcoming the accuracy vs. performance trade-off in oscillator ising machines. In 2021 IEEE International Electron Devices Meeting (IEDM) , 40.2.1–40.2.4 https://doi.org/10.1109/IEDM19574.2021.9720612 (2021).
Graber, M. & Hofmann, K. A coupled oscillator network to solve combinatorial optimization problems with over 95% accuracy. In 2023 IEEE International Symposium on Circuits and Systems (ISCAS) , 1–5 https://doi.org/10.1109/ISCAS46773.2023.10181365 (2023).
Hoppensteadt, F. C. & Izhikevich, E. M. Oscillatory neurocomputers with dynamic connectivity. Phys. Rev. Lett. 82 , 2983–2986 (1999).
Delacour, C. & Todri-Sanial, A. Mapping hebbian learning rules to coupling resistances for oscillatory neural networks. Front. Neurosci. 15 , 694549 (2021).
Delacour, C. et al. A mixed-signal oscillatory neural network for scalable analog computations in phase domain. Neuromorphic Comput. Eng. 3 , 034004 (2023).
Karp, R. M. Reducibility among Combinatorial Problems , 85–103 (Springer US, Boston, MA, 1972). https://doi.org/10.1007/978-1-4684-2001-2_9
Lucas, A. Ising formulations of many np problems. Front. Phys. 2 , 5 (2014).
Harwood, S. et al. Formulating and solving routing problems on quantum computers. IEEE Trans. Quantum Eng. 2 , 1–17 (2021).
Qin, P. & Zhao, J. A polynomial-time algorithm for image segmentation using ising models. 2011 Seventh Int. Conf. Nat. Comput. 2 , 932–935 (2011).
Google Scholar
Liu, Z. et al. Ising-cf: A pathbreaking collaborative filtering method through efficient ising machine learning. In 2023 60th ACM/IEEE Design Automation Conference (DAC) , 1–6 https://doi.org/10.1109/DAC56929.2023.10247860 (2023).
Kochenberger, G. et al. The unconstrained binary quadratic programming problem: a survey. J. Combinatorial Optim. 28 , 58–81 (2014).
Article MathSciNet Google Scholar
Ising, E. Beitrag zur theorie des ferromagnetismus. Z. f.ür. Phys. 31 , 253–258 (1925).
Wang, T., Wu, L., Nobel, P. & Roychowdhury, J. Solving combinatorial optimisation problems using oscillator based ising machines. Nat. Comput. 20 , 287–306 (2021).
Wang, T. Novel Computing Paradigms using Oscillators . Ph.D. thesis, EECS Department, University of California, Berkeley http://www2.eecs.berkeley.edu/Pubs/TechRpts/2020/EECS-2020-12.html (2020).
Wang, T. & Roychowdhury, J. Oscillator-based ising machine Preprint at https://doi.org/10.48550/arXiv.1709.08102 (2017).
Lobe, E., Schürmann, L. & Stollenwerk, T. Embedding of complete graphs in broken chimera graphs. Quantum Inf. Process. 20 , 234 (2021).
Sugie, Y. et al. Minor-embedding heuristics for large-scale annealing processors with sparse hardware graphs of up to 102,400 nodes. Soft Comput. 25 , 1731–1749 (2021).
Date, P., Patton, R., Schuman, C. & Potok, T. Efficiently embedding qubo problems on adiabatic quantum computers. Quantum Inf. Process. 18 , 117 (2019).
Graber, M., Wesp, M. & Hofmann, K. A fast graph minor embedding heuristic for oscillator based ising machines. In 2022 Austrochip Workshop on Microelectronics (Austrochip) https://doi.org/10.1109/Austrochip56145.2022.9940722 (2022).
Jagielski, T., Manohar, R. & Roychowdhury, J. Fpim: Field-programmable ising machines for solving sat Preprint at https://doi.org/10.48550/arXiv.2306.01569 (2023).
Izhikevich, E. & Kuramoto, Y. Weakly coupled oscillators. In Françoise, J.-P., Naber, G. L. & Tsun, T. S. (eds.) Encyclopedia of Mathematical Physics , 448–453 (Academic Press, Oxford, 2006). https://www.sciencedirect.com/science/article/pii/B0125126662001061 .
Bhansali, P. & Roychowdhury, J. Gen-adler: The generalized adler’s equation for injection locking analysis in oscillators. In 2009 Asia and South Pacific Design Automation Conference , 522–527 https://doi.org/10.1109/ASPDAC.2009.4796533 (2009).
Graber, M., Angeli, N. & Hofmann, K. An efficient modeling approach for large ring oscillator based ising machines. In SMACD / PRIME 2021; International Conference on SMACD and 16th Conference on PRIME , 1–4 (2021).
Ochs, K. & Beattie, B. A. Towards wave digital modeling of neural pathways using two-port coupling networks. In 2022 IEEE International Symposium on Circuits and Systems (ISCAS) , 809–812 https://doi.org/10.1109/ISCAS48785.2022.9937250 (2022).
Wilton, S. J. Architectures and Algorithms for Field-Programmable Gate Arrays with Embedded Memory . Ph.D. thesis, University of Toronto https://hdl.handle.net/1807/10686 (1997).
Angeli, N. & Hofmann, K. A scalable fully synthesized phase-to-digital converter for phase and duty-cycle measurement of high-speed clocks. In 2018 IEEE International Symposium on Circuits and Systems (ISCAS) , 1–5 https://doi.org/10.1109/ISCAS.2018.8351118 (2018).
Graber, M. & Hofmann, K. Analysis and design of oscillator coupling for solving combinatorial optimization problems. In 2022 29th IEEE International Conference on Electronics, Circuits and Systems (ICECS) , 1–4 https://doi.org/10.1109/ICECS202256217.2022.9970974 (2022).
Graber, M. & Hofmann, K. Flexible routing to overcome the embedding bottleneck of oscillator-based ising machines. In 2023 30th IEEE International Conference on Electronics, Circuits and Systems (ICECS) , 1–4 https://doi.org/10.1109/ICECS58634.2023.10382841 (2023).
Gurobi Optimization, LLC. Gurobi Optimizer Reference Manual https://www.gurobi.com (2023).
Graber, M. Simple benchmark set. figshare https://doi.org/10.6084/m9.figshare.25018898 (2024).
Graber, M. Difficult benchmark set. figshare https://doi.org/10.6084/m9.figshare.25018892 (2024).
Graber, M. Bias benchmark set. figshare https://doi.org/10.6084/m9.figshare.25018862 (2024).
Graber, M. Weighted benchmark set. figshare https://doi.org/10.6084/m9.figshare.25018952 (2024).
Kirkpatrick, S., Gelatt, C. D. & Vecchi, M. P. Optimization by simulated annealing. Science 220 , 671–680 (1983).
Graber, M. & Hofmann, K. An enhanced 1440 coupled cmos oscillator network to solve combinatorial optimization problems. In 2023 IEEE 36th International System-on-Chip Conference (SOCC) , 1–6 https://doi.org/10.1109/SOCC58585.2023.10256945 (2023).
Download references
Acknowledgements
We want to thank the German Research Foundation (DFG) for supporting this work with grant number 496307198. Furthermore, the authors want to thank Tim Lukas Kessel and Malte Nilges (both Technical University of Darmstadt) for their help and support in the development of the printed circuit board (PCB) for testing. Large language models were used to improve the language of the manuscript. Grammarly by Grammarly, Inc. was used for spell checking. ChatGPT by OpenAI and DeepL by Deepl SE were used to enhance the clarity of the writing.
Open Open Access funding enabled and organized by Projekt DEAL.
Author information
Authors and affiliations.
Technical University of Darmstadt, Integrated Electronic Systems Lab, Darmstadt, Germany
Markus Graber & Klaus Hofmann
You can also search for this author in PubMed Google Scholar
Contributions
M.G. conducted the circuit design, simulation, and layout for the manufactured chip. M.G. built the test setup, ran the experiments, and was the primary author of the manuscript. K.H. supervised the work, provided guidance, and contributed to the writing and revision process. All authors reviewed the manuscript.
Corresponding author
Correspondence to Markus Graber .
Ethics declarations
Competing interests.
The authors declare no competing interests.
Peer review
Peer review information.
Communications Engineering thanks Aida Todri, Louis Hutin, Jérémie Laydevant for their contribution to the peer review of this work. Primary Handling Editors: Damien Querlioz and Anastasiia Vasylchenkova. A peer review file is available.
Additional information
Publisher’s note Springer Nature remains neutral with regard to jurisdictional claims in published maps and institutional affiliations.
Supplementary information
Peer review file, rights and permissions.
Open Access This article is licensed under a Creative Commons Attribution 4.0 International License, which permits use, sharing, adaptation, distribution and reproduction in any medium or format, as long as you give appropriate credit to the original author(s) and the source, provide a link to the Creative Commons licence, and indicate if changes were made. The images or other third party material in this article are included in the article’s Creative Commons licence, unless indicated otherwise in a credit line to the material. If material is not included in the article’s Creative Commons licence and your intended use is not permitted by statutory regulation or exceeds the permitted use, you will need to obtain permission directly from the copyright holder. To view a copy of this licence, visit http://creativecommons.org/licenses/by/4.0/ .
Reprints and permissions
About this article
Cite this article.
Graber, M., Hofmann, K. An integrated coupled oscillator network to solve optimization problems. Commun Eng 3 , 116 (2024). https://doi.org/10.1038/s44172-024-00261-w
Download citation
Received : 08 February 2024
Accepted : 05 August 2024
Published : 23 August 2024
DOI : https://doi.org/10.1038/s44172-024-00261-w
Share this article
Anyone you share the following link with will be able to read this content:
Sorry, a shareable link is not currently available for this article.
Provided by the Springer Nature SharedIt content-sharing initiative
Quick links
- Explore articles by subject
- Guide to authors
- Editorial policies
Sign up for the Nature Briefing newsletter — what matters in science, free to your inbox daily.

Failure Analysis of Automotive Drive System: A Six Sigma DMADV Approach
- Tools and Techniques
- Published: 24 August 2024
Cite this article
- Shivagond Teli 1 ,
- Vinod Surange ORCID: orcid.org/0000-0002-3388-9489 2 &
- Lokpriya Gaikwad 3
Explore all metrics
This study outlines the application of the Six Sigma methodology to rectify oil seal leaks in an automobile company's hollow drive shafts. Persistent issues with oil seepage through the shaft's oil seal led to clutch malfunctions, power failures, and subsequent breakdowns, resulting in high warranty costs, which negatively impacted the status of a firm in the market and the level of fulfillment experienced by its clients. Projects are crucial for translating an organization's strategic goals into achievable outcomes, serving as the cornerstone of success. We implemented a quality-driven effort using Six Sigma and the Theory of Inventive Problem Solving (TRIZ) to foster a culture of continuous improvement. Utilizing the procedures-oriented DMADV (Define, Measure, Analyze, Design, and Verify) technique, the oil seal leakage issue was addressed and resolved successfully from the gearbox. The Pugh matrix was used to choose a new design from various alternatives, a KANO model to evaluate customer demands, and a cause-and-effect diagram to analyze possible failure situations. The overall aim was to determine the failure situations that were most likely to occur. Through its deployment, we intended to improve both the product's dependability and the production process's efficiency. Following the design improvements, the defects observed in the assemblies dropped to 58, with no occurrences of oil seal leakage. It is plausible to conclude that these techniques can significantly enhance product quality. The study demonstrates the efficacy of Six Sigma methodologies in tackling intricate situations.
This is a preview of subscription content, log in via an institution to check access.
Access this article
Subscribe and save.
- Get 10 units per month
- Download Article/Chapter or eBook
- 1 Unit = 1 Article or 1 Chapter
- Cancel anytime
Price includes VAT (Russian Federation)
Instant access to the full article PDF.
Rent this article via DeepDyve
Institutional subscriptions
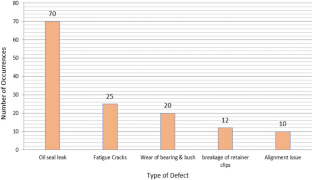
Similar content being viewed by others
Application of 7-Step Problem-Solving Methodology for Defect Elimination: A Case Study in an Automotive Industry
Advanced RCM Industry Case—Modeling and Advanced Analytics (ELMAS) for Improved Availability and Cost-Efficiency
Integrating Reliability, Availability, and Maintainability Issues for Analyzing Failures in Fuel Injection Pump
Explore related subjects.
- Artificial Intelligence
S. Thawani, Six Sigma—strategy for organizational excellence. Total Qual. Manag. Bus. Excell. 15 (5–6), 655–664 (2004). https://doi.org/10.1080/14783360410001680143
Article Google Scholar
S.D.R. Perera, Failure on an automobile rear axle shaft. J. Fail. Anal. Prev. 22 (3), 934–939 (2022). https://doi.org/10.1007/s11668-022-01412-x
I. Daniyan, A. Adeodu, K. Mpofu, R. Maladzhi, M.G. Kana-Kana Katumba, Application of lean Six Sigma methodology using DMAIC approach for the improvement of bogie assembly process in the railcar industry. Heliyon. 8 (3), e09043 (2022). https://doi.org/10.1016/j.heliyon.2022.e09043
Article PubMed PubMed Central Google Scholar
L.M. Gaikwad, S.N. Teli, V.S. Majali, U.M. Bhushi, An application of Six Sigma to reduce supplier quality cost. J. Inst. Eng. Ser. C. 97 (1), 93–107 (2016). https://doi.org/10.1007/s40032-015-0200-2
M. Singh, R. Rathi, Investigation of critical success factors associated with Lean Six Sigma implementation in MSMEs using best worst method. Int. J. Six Sigma Compet. Advant. 12 (2/3), 209–233 (2020). https://doi.org/10.1504/IJSSCA.2020.110976
K.M. Suresh, P. Asokan, S. Vinodh, Application of design for Six Sigma methodology to an automotive component. Int. J. Six Sigma Compet. Advant. 10 (1), 1–23 (2016). https://doi.org/10.1504/IJSSCA.2016.080446
D.S. Jiju Antony, J.J. Dahlgaard, Lean Six Sigma and innovation: an exploratory study among UK organisations. Total Qual. Manag. Bus. Excell. 27 (1–2), 124–140 (2016). https://doi.org/10.1080/14783363.2014.959255
V.G. Surange, Implementation of Six Sigma to reduce cost of quality: a case study of automobile sector. J. Fail. Anal. Prev. 15 (2), 282–294 (2015). https://doi.org/10.1007/s11668-015-9927-6
L.M. Gaikwad, U. Bhushi, S.N. Teli, Implementation of Six Sigma methodologies to gain a competitive advantage: a case study approach (2022). https://doi.org/10.1109/ASET53988.2022.9735103
A. Trubetskaya, O. McDermott, P. Durand, D.J. Powell, Improving value chain data lifecycle management utilising design for Lean Six Sigma methods. TQM J. 36 (9), 136–154 (2024). https://doi.org/10.1108/TQM-01-2024-0020
S.G. Bidikar, S.B. Rane, P.R. Potdar, Product development using design for Six Sigma approach: case study in switchgear industry. Int. J. Syst. Assur. Eng. Manag. 13 (1), 203–230 (2022). https://doi.org/10.1007/s13198-021-01199-4
F.-K. Wang, C.-T. Yeh, T.-P. Chu, Using the design for Six Sigma approach with TRIZ for new product development. Comput. Ind. Eng. 98 , 522–530 (2016). https://doi.org/10.1016/j.cie.2016.06.014
D.M. Wu, C.K.P. Luk, W.Z. Fei, Quality control of low-cost electric machines for electric vehicles by DOE assisted six sigma DMADV method, in 2017 7th International Conference on Power Electronics Systems and Applications: Smart Mobility, Power Transfer & Security (PESA) (2017), pp. 1–8. https://doi.org/10.1109/PESA.2017.8277740
K. Guler, D.M. Petrisor, A Pugh Matrix based product development model for increased small design team efficiency. Cogent Eng. 8 (1), 1923383 (2021). https://doi.org/10.1080/23311916.2021.1923383
Download references
Author information
Authors and affiliations.
Bharati Vidyapeeth College of Engineering, Navi Mumbai, India
Shivagond Teli
Symbiosis Institute of Business Management, Nagpur, Symbiosis International (Deemed University), Pune, India
Vinod Surange
SIES Graduate School of Technology, Navi Mumbai, India
Lokpriya Gaikwad
You can also search for this author in PubMed Google Scholar
Corresponding author
Correspondence to Vinod Surange .
Additional information
Publisher's note.
Springer Nature remains neutral with regard to jurisdictional claims in published maps and institutional affiliations.
Rights and permissions
Springer Nature or its licensor (e.g. a society or other partner) holds exclusive rights to this article under a publishing agreement with the author(s) or other rightsholder(s); author self-archiving of the accepted manuscript version of this article is solely governed by the terms of such publishing agreement and applicable law.
Reprints and permissions
About this article
Teli, S., Surange, V. & Gaikwad, L. Failure Analysis of Automotive Drive System: A Six Sigma DMADV Approach. J Fail. Anal. and Preven. (2024). https://doi.org/10.1007/s11668-024-02003-8
Download citation
Received : 21 March 2024
Revised : 06 July 2024
Accepted : 21 July 2024
Published : 24 August 2024
DOI : https://doi.org/10.1007/s11668-024-02003-8
Share this article
Anyone you share the following link with will be able to read this content:
Sorry, a shareable link is not currently available for this article.
Provided by the Springer Nature SharedIt content-sharing initiative
- Automotive manufacturing
- TRIZ methodology
- Critical-to-Quality (CTQ)
- Find a journal
- Publish with us
- Track your research

COMMENTS
A systems thinking approach to problem solving recognizes the problem as part of a wider system and addresses the whole system in any solution rather than just the problem area. A popular way of applying a systems thinking lens is to examine the issue from multiple perspectives, zooming out from single and visible elements to the bigger and ...
The systems approach to problem solving involves a systems route to explain the meaning of problems and opportunities and also to develop the correct feasible solutions for the same. The term 'system' signifies the different features, elements, merits and demerits of a particular subject that may be biological or commercial. ...
Systems thinking is an approach to understanding how things influence one another within a whole entity. Systems thinking studies connections between key parts to see the collective behaviors that result. Expanding perspectives brings clarity to complex situations. Systems thinking provides a framework for seeing relationships and patterns to ...
Simplifying a System or Applying Systems Approach For Problem Solving. The easiest way to simplify a system for better understanding is to follow a two-stage approach. Partitioning the System into Black Boxes. This is the first stage of the simplification process, in this stage the system is partitioned into black boxes.
The systems approach to problem solving used a systems orientation to define problems and opportunities and develop solutions. Studying a problem and formulating a solution involve the following interrelated activities: Recognize and define a problem or opportunity using systems thinking. Develop and evaluate alternative system solutions.
4.2 Systems analysis: a model of a problem-solving cycle 4.2.1 Overview The general methodology of systems analysis has probably been the most widely used systems approach to problem-solving. It has been used in many of the subject areas listed in section 4.1, including, of course, the area of manufac
A systemic approach to "problem solving," however, offers systemic (holistic rather than piecemeal) and/or systematic (step-by-step rather than intuitive) guidelines. Systems methodologies are thus systematic in the way that a "problem" is tackled and/or systemic when holistic thinking is adopted.
The Benefits of Systems Thinking. Systems thinking offers several compelling reasons to adopt its principles in problem-solving endeavours. By broadening our thinking and enabling us to articulate problems in novel ways, it expands the range of choices available for resolving complex issues. Furthermore, systems thinking emphasises the ...
The Systems Thinking Approach. Systems thinking is a response to the perceived need to understand phenomena in terms of the whole rather than simply the parts, as a dynamic of interactions between elements over time, and as being based upon a purposeful and subjective world view. Systems thinking is a framework whereby some form of systems ...
Systems thinking is, literally, a system of thinking about systems. As discussed later in this paper, this highlights the problems with the definitions available in the literature. These definitions tend to analyze systems thinking through a reductionist approach â€" generally considered a non-systems-thinking approach.
2. Synthesis. In general, synthesis refers to the combining of two or more things to create something new. When it comes to systems thinking, the goal is synthesis, as opposed to analysis, which ...
Systems thinking is an approach to problem solving which takes into account the overall system as well as its individual parts. According to Peter Senge, it's "a framework for seeing interrelationships rather than things, for seeing patterns rather than static snapshots. It is a set of general principles spanning fields as diverse as ...
Solving complex problems can't be achieved through everyday problem solving methods. They can be solved only through analytical methods and tools. Solving complex problems can be achieved through systems thinking, a process that fits the problem, and system dynamics, which is an approach to model systems by emphasizing their feedback loops.
The systems approach is a problem solving paradigm. That is to say, the systems approach. considers the attributes of an e ntire system to achieve the object ive of a system, which is to solve. a ...
Systems thinking is a holistic approach to problem-solving. It's a way of looking at how systems work, what that system's perspective is, and how to better improve system behaviors. ... Once you've mapped out the systems to help solve your problem, you can do some systems modeling to help understand how they're connected. Which leads us ...
Systems thinking is also a diagnostic tool. As in the medical field, effective treatment follows thorough diagnosis. In this sense, systems thinking is a disciplined approach for examining problems more completely and accurately before acting. It allows us to ask better questions before jumping to conclusions.
A systemic approach, however, provides a structured problem-solving process for digging deeper into our most vexing problems. To get a sense for how systems thinking can be used for problem identification, problem solving, and solution testing, we have outlined a six-step process. To use this process on a problem in your workplace, try the ...
New approaches are needed to help to manage that conflict. In this chapter, which continues to develop Theme C, we introduce a number of these approaches to "problem solving" each able to contribute in particular ways to deal with the complexities of modern society. Let us first set the scene. Download to read the full chapter text.
When we do problem definition well in classic problem solving, we are demonstrating the kind of empathy, at the very beginning of our problem, that design thinking asks us to approach. When we ideate—and that's very similar to the disaggregation, prioritization, and work-planning steps—we do precisely the same thing, and often we use ...
Systems thinking is problem-solving approach that examines the relationships between functions in an organization. Systems thinking is powerful because it enables you to predict the consequences of a potential change. This problem-solving method can also help you eliminate silos, see different viewpoints, and remain focused on the big picture. ...
Brainstorm options to solve the problem. Select an option. Create an implementation plan. Execute the plan and monitor the results. Evaluate the solution. Read more: Effective Problem Solving Steps in the Workplace. 2. Collaborative. This approach involves including multiple people in the problem-solving process.
By endorsing a definition of data as a sociotechnical process, of ethics as a collective practice, and of the city as a complex system, the course enacts a transdisciplinary approach and problem-opening method that compel students to recognize and tackle the unavoidable multifacetedness of all ethical stances, as well as the intrinsic open ...
We presented a scalable approach for OIMs capable of solving optimization problems in Ising as well as QUBO formulation. The critical characteristic of such OIM systems is the network connectivity.
Contradiction Matrix: A tool for identifying conflicts between different system components or requirements, helping to pinpoint areas where traditional solutions fail due to contradictory constraints. 40 Principles of Invention: A comprehensive set of principles used to resolve contradictions and enhance problem-solving creativity.