Have a language expert improve your writing
Run a free plagiarism check in 10 minutes, automatically generate references for free.
- Knowledge Base
- Methodology
- Correlational Research | Guide, Design & Examples

Correlational Research | Guide, Design & Examples
Published on 5 May 2022 by Pritha Bhandari . Revised on 5 December 2022.
A correlational research design investigates relationships between variables without the researcher controlling or manipulating any of them.
A correlation reflects the strength and/or direction of the relationship between two (or more) variables. The direction of a correlation can be either positive or negative.
Positive correlation | Both variables change in the same direction | As height increases, weight also increases |
---|---|---|
Negative correlation | The variables change in opposite directions | As coffee consumption increases, tiredness decreases |
Zero correlation | There is no relationship between the variables | Coffee consumption is not correlated with height |
Table of contents
Correlational vs experimental research, when to use correlational research, how to collect correlational data, how to analyse correlational data, correlation and causation, frequently asked questions about correlational research.
Correlational and experimental research both use quantitative methods to investigate relationships between variables. But there are important differences in how data is collected and the types of conclusions you can draw.
Correlational research | Experimental research | |
---|---|---|
Purpose | Used to test strength of association between variables | Used to test cause-and-effect relationships between variables |
Variables | Variables are only observed with no manipulation or intervention by researchers | An is manipulated and a dependent variable is observed |
Control | Limited is used, so other variables may play a role in the relationship | are controlled so that they can’t impact your variables of interest |
Validity | High : you can confidently generalise your conclusions to other populations or settings | High : you can confidently draw conclusions about causation |
Prevent plagiarism, run a free check.
Correlational research is ideal for gathering data quickly from natural settings. That helps you generalise your findings to real-life situations in an externally valid way.
There are a few situations where correlational research is an appropriate choice.
To investigate non-causal relationships
You want to find out if there is an association between two variables, but you don’t expect to find a causal relationship between them.
Correlational research can provide insights into complex real-world relationships, helping researchers develop theories and make predictions.
To explore causal relationships between variables
You think there is a causal relationship between two variables, but it is impractical, unethical, or too costly to conduct experimental research that manipulates one of the variables.
Correlational research can provide initial indications or additional support for theories about causal relationships.
To test new measurement tools
You have developed a new instrument for measuring your variable, and you need to test its reliability or validity .
Correlational research can be used to assess whether a tool consistently or accurately captures the concept it aims to measure.
There are many different methods you can use in correlational research. In the social and behavioural sciences, the most common data collection methods for this type of research include surveys, observations, and secondary data.
It’s important to carefully choose and plan your methods to ensure the reliability and validity of your results. You should carefully select a representative sample so that your data reflects the population you’re interested in without bias .
In survey research , you can use questionnaires to measure your variables of interest. You can conduct surveys online, by post, by phone, or in person.
Surveys are a quick, flexible way to collect standardised data from many participants, but it’s important to ensure that your questions are worded in an unbiased way and capture relevant insights.
Naturalistic observation
Naturalistic observation is a type of field research where you gather data about a behaviour or phenomenon in its natural environment.
This method often involves recording, counting, describing, and categorising actions and events. Naturalistic observation can include both qualitative and quantitative elements, but to assess correlation, you collect data that can be analysed quantitatively (e.g., frequencies, durations, scales, and amounts).
Naturalistic observation lets you easily generalise your results to real-world contexts, and you can study experiences that aren’t replicable in lab settings. But data analysis can be time-consuming and unpredictable, and researcher bias may skew the interpretations.
Secondary data
Instead of collecting original data, you can also use data that has already been collected for a different purpose, such as official records, polls, or previous studies.
Using secondary data is inexpensive and fast, because data collection is complete. However, the data may be unreliable, incomplete, or not entirely relevant, and you have no control over the reliability or validity of the data collection procedures.
After collecting data, you can statistically analyse the relationship between variables using correlation or regression analyses, or both. You can also visualise the relationships between variables with a scatterplot.
Different types of correlation coefficients and regression analyses are appropriate for your data based on their levels of measurement and distributions .
Correlation analysis
Using a correlation analysis, you can summarise the relationship between variables into a correlation coefficient : a single number that describes the strength and direction of the relationship between variables. With this number, you’ll quantify the degree of the relationship between variables.
The Pearson product-moment correlation coefficient, also known as Pearson’s r , is commonly used for assessing a linear relationship between two quantitative variables.
Correlation coefficients are usually found for two variables at a time, but you can use a multiple correlation coefficient for three or more variables.
Regression analysis
With a regression analysis , you can predict how much a change in one variable will be associated with a change in the other variable. The result is a regression equation that describes the line on a graph of your variables.
You can use this equation to predict the value of one variable based on the given value(s) of the other variable(s). It’s best to perform a regression analysis after testing for a correlation between your variables.
It’s important to remember that correlation does not imply causation . Just because you find a correlation between two things doesn’t mean you can conclude one of them causes the other, for a few reasons.
Directionality problem
If two variables are correlated, it could be because one of them is a cause and the other is an effect. But the correlational research design doesn’t allow you to infer which is which. To err on the side of caution, researchers don’t conclude causality from correlational studies.
Third variable problem
A confounding variable is a third variable that influences other variables to make them seem causally related even though they are not. Instead, there are separate causal links between the confounder and each variable.
In correlational research, there’s limited or no researcher control over extraneous variables . Even if you statistically control for some potential confounders, there may still be other hidden variables that disguise the relationship between your study variables.
Although a correlational study can’t demonstrate causation on its own, it can help you develop a causal hypothesis that’s tested in controlled experiments.
A correlation reflects the strength and/or direction of the association between two or more variables.
- A positive correlation means that both variables change in the same direction.
- A negative correlation means that the variables change in opposite directions.
- A zero correlation means there’s no relationship between the variables.
A correlational research design investigates relationships between two variables (or more) without the researcher controlling or manipulating any of them. It’s a non-experimental type of quantitative research .
Controlled experiments establish causality, whereas correlational studies only show associations between variables.
- In an experimental design , you manipulate an independent variable and measure its effect on a dependent variable. Other variables are controlled so they can’t impact the results.
- In a correlational design , you measure variables without manipulating any of them. You can test whether your variables change together, but you can’t be sure that one variable caused a change in another.
In general, correlational research is high in external validity while experimental research is high in internal validity .
A correlation is usually tested for two variables at a time, but you can test correlations between three or more variables.
A correlation coefficient is a single number that describes the strength and direction of the relationship between your variables.
Different types of correlation coefficients might be appropriate for your data based on their levels of measurement and distributions . The Pearson product-moment correlation coefficient (Pearson’s r ) is commonly used to assess a linear relationship between two quantitative variables.
Cite this Scribbr article
If you want to cite this source, you can copy and paste the citation or click the ‘Cite this Scribbr article’ button to automatically add the citation to our free Reference Generator.
Bhandari, P. (2022, December 05). Correlational Research | Guide, Design & Examples. Scribbr. Retrieved 2 July 2024, from https://www.scribbr.co.uk/research-methods/correlational-research-design/
Is this article helpful?
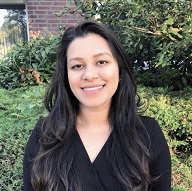
Pritha Bhandari
Other students also liked, a quick guide to experimental design | 5 steps & examples, quasi-experimental design | definition, types & examples, qualitative vs quantitative research | examples & methods.
- Skip to secondary menu
- Skip to main content
- Skip to primary sidebar
Statistics By Jim
Making statistics intuitive
Correlational Study Overview & Examples
By Jim Frost 2 Comments
What is a Correlational Study?
A correlational study is an experimental design that evaluates only the correlation between variables. The researchers record measurements but do not control or manipulate the variables. Correlational research is a form of observational study .
A correlation indicates that as the value of one variable increases, the other tends to change in a specific direction:
- Positive correlation : Two variables increase or decrease together (as height increases, weight tends to increase).
- Negative correlation : As one variable increases, the other tends to decrease (as school absences increase, grades tend to fall).
- No correlation : No relationship exists between the two variables. As one increases, the other does not change in a specific direction (as absences increase, height doesn’t tend to increase or decrease).

For example, researchers conducting correlational research explored the relationship between social media usage and levels of anxiety in young adults. Participants reported their demographic information and daily time on various social media platforms and completed a standardized anxiety assessment tool.
The correlational study looked for relationships between social media usage and anxiety. Is increased social media usage associated with higher anxiety? Is it worse for particular demographics?
Learn more about Interpreting Correlation .
Using Correlational Research
Correlational research design is crucial in various disciplines, notably psychology and medicine. This type of design is generally cheaper, easier, and quicker to conduct than an experiment because the researchers don’t control any variables or conditions. Consequently, these studies often serve as an initial assessment, especially when random assignment and controlling variables for a true experiment are not feasible or unethical.
However, an unfortunate aspect of a correlational study is its limitation in establishing causation. While these studies can reveal connections between variables, they cannot prove that altering one variable will cause changes in another. Hence, correlational research can determine whether relationships exist but cannot confirm causality.
Remember, correlation doesn’t necessarily imply causation !
Correlational Study vs Experiment
The difference between the two designs is simple.
In a correlational study, the researchers don’t systematically control any variables. They’re simply observing events and do not want to influence outcomes.
In an experiment, researchers manipulate variables and explicitly hope to affect the outcomes. For example, they might control the treatment condition by giving a medication or placebo to each subject. They also randomly assign subjects to the control and treatment groups, which helps establish causality.
Learn more about Randomized Controlled Trials (RCTs) , which statisticians consider to be true experiments.
Types of Correlation Studies and Examples
Researchers divide these studies into three broad types.
Secondary Data Sources
One approach to correlational research is to utilize pre-existing data, which may include official records, public polls, or data from earlier studies. This method can be cost-effective and time-efficient because other researchers have already gathered the data. These existing data sources can provide large sample sizes and longitudinal data , thereby showing relationship trends.
However, it also comes with potential drawbacks. The data may be incomplete or irrelevant to the new research question. Additionally, as a researcher, you won’t have control over the original data collection methods, potentially impacting the data’s reliability and validity .
Using existing data makes this approach a retrospective study .
Surveys in Correlation Research
Surveys are a great way to collect data for correlational studies while using a consistent instrument across all respondents. You can use various formats, such as in-person, online, and by phone. And you can ask the questions necessary to obtain the particular variables you need for your project. In short, it’s easy to customize surveys to match your study’s requirements.
However, you’ll need to carefully word all the questions to be clear and not introduce bias in the results. This process can take multiple iterations and pilot studies to produce the finished survey.
For example, you can use a survey to find correlations between various demographic variables and political opinions.
Naturalistic Observation
Naturalistic observation is a method of collecting field data for a correlational study. Researchers observe and measure variables in a natural environment. The process can include counting events, categorizing behavior, and describing outcomes without interfering with the activities.
For example, researchers might observe and record children’s behavior after watching television. Does a relationship exist between the type of television program and behaviors?
Naturalistic observations occur in a prospective study .
Analyzing Data from a Correlational Study
Statistical analysis of correlational research frequently involves correlation and regression analysis .
A correlation coefficient describes the strength and direction of the relationship between two variables with a single number.
Regression analysis can evaluate how multiple variables relate to a single outcome. For example, in the social media correlational study example, how do the demographic variables and daily social media usage collectively correlate with anxiety?
Curtis EA, Comiskey C, Dempsey O. Importance and use of correlational research . Nurse Researcher . 2016;23(6):20-25. doi:10.7748/nr.2016.e1382
Share this:

Reader Interactions
January 14, 2024 at 4:34 pm
Hi Jim. Have you written a blog note dedicated to clinical trials? If not, besides the note on hypothesis testing, are there other blogs ypo have written that touch on clinical trials?
January 14, 2024 at 5:49 pm
Hi Stan, I haven’t written a blog post specifically about clinical trials, but I have the following related posts:
Randomized Controlled Trials Clinical Trial about a COVID vaccine Clinical Trials about flu vaccines
Comments and Questions Cancel reply

Want to create or adapt books like this? Learn more about how Pressbooks supports open publishing practices.
7.2 Correlational Research
Learning objectives.
- Define correlational research and give several examples.
- Explain why a researcher might choose to conduct correlational research rather than experimental research or another type of nonexperimental research.
What Is Correlational Research?
Correlational research is a type of nonexperimental research in which the researcher measures two variables and assesses the statistical relationship (i.e., the correlation) between them with little or no effort to control extraneous variables. There are essentially two reasons that researchers interested in statistical relationships between variables would choose to conduct a correlational study rather than an experiment. The first is that they do not believe that the statistical relationship is a causal one. For example, a researcher might evaluate the validity of a brief extraversion test by administering it to a large group of participants along with a longer extraversion test that has already been shown to be valid. This researcher might then check to see whether participants’ scores on the brief test are strongly correlated with their scores on the longer one. Neither test score is thought to cause the other, so there is no independent variable to manipulate. In fact, the terms independent variable and dependent variable do not apply to this kind of research.
The other reason that researchers would choose to use a correlational study rather than an experiment is that the statistical relationship of interest is thought to be causal, but the researcher cannot manipulate the independent variable because it is impossible, impractical, or unethical. For example, Allen Kanner and his colleagues thought that the number of “daily hassles” (e.g., rude salespeople, heavy traffic) that people experience affects the number of physical and psychological symptoms they have (Kanner, Coyne, Schaefer, & Lazarus, 1981). But because they could not manipulate the number of daily hassles their participants experienced, they had to settle for measuring the number of daily hassles—along with the number of symptoms—using self-report questionnaires. Although the strong positive relationship they found between these two variables is consistent with their idea that hassles cause symptoms, it is also consistent with the idea that symptoms cause hassles or that some third variable (e.g., neuroticism) causes both.
A common misconception among beginning researchers is that correlational research must involve two quantitative variables, such as scores on two extraversion tests or the number of hassles and number of symptoms people have experienced. However, the defining feature of correlational research is that the two variables are measured—neither one is manipulated—and this is true regardless of whether the variables are quantitative or categorical. Imagine, for example, that a researcher administers the Rosenberg Self-Esteem Scale to 50 American college students and 50 Japanese college students. Although this “feels” like a between-subjects experiment, it is a correlational study because the researcher did not manipulate the students’ nationalities. The same is true of the study by Cacioppo and Petty comparing college faculty and factory workers in terms of their need for cognition. It is a correlational study because the researchers did not manipulate the participants’ occupations.
Figure 7.2 “Results of a Hypothetical Study on Whether People Who Make Daily To-Do Lists Experience Less Stress Than People Who Do Not Make Such Lists” shows data from a hypothetical study on the relationship between whether people make a daily list of things to do (a “to-do list”) and stress. Notice that it is unclear whether this is an experiment or a correlational study because it is unclear whether the independent variable was manipulated. If the researcher randomly assigned some participants to make daily to-do lists and others not to, then it is an experiment. If the researcher simply asked participants whether they made daily to-do lists, then it is a correlational study. The distinction is important because if the study was an experiment, then it could be concluded that making the daily to-do lists reduced participants’ stress. But if it was a correlational study, it could only be concluded that these variables are statistically related. Perhaps being stressed has a negative effect on people’s ability to plan ahead (the directionality problem). Or perhaps people who are more conscientious are more likely to make to-do lists and less likely to be stressed (the third-variable problem). The crucial point is that what defines a study as experimental or correlational is not the variables being studied, nor whether the variables are quantitative or categorical, nor the type of graph or statistics used to analyze the data. It is how the study is conducted.
Figure 7.2 Results of a Hypothetical Study on Whether People Who Make Daily To-Do Lists Experience Less Stress Than People Who Do Not Make Such Lists

Data Collection in Correlational Research
Again, the defining feature of correlational research is that neither variable is manipulated. It does not matter how or where the variables are measured. A researcher could have participants come to a laboratory to complete a computerized backward digit span task and a computerized risky decision-making task and then assess the relationship between participants’ scores on the two tasks. Or a researcher could go to a shopping mall to ask people about their attitudes toward the environment and their shopping habits and then assess the relationship between these two variables. Both of these studies would be correlational because no independent variable is manipulated. However, because some approaches to data collection are strongly associated with correlational research, it makes sense to discuss them here. The two we will focus on are naturalistic observation and archival data. A third, survey research, is discussed in its own chapter.
Naturalistic Observation
Naturalistic observation is an approach to data collection that involves observing people’s behavior in the environment in which it typically occurs. Thus naturalistic observation is a type of field research (as opposed to a type of laboratory research). It could involve observing shoppers in a grocery store, children on a school playground, or psychiatric inpatients in their wards. Researchers engaged in naturalistic observation usually make their observations as unobtrusively as possible so that participants are often not aware that they are being studied. Ethically, this is considered to be acceptable if the participants remain anonymous and the behavior occurs in a public setting where people would not normally have an expectation of privacy. Grocery shoppers putting items into their shopping carts, for example, are engaged in public behavior that is easily observable by store employees and other shoppers. For this reason, most researchers would consider it ethically acceptable to observe them for a study. On the other hand, one of the arguments against the ethicality of the naturalistic observation of “bathroom behavior” discussed earlier in the book is that people have a reasonable expectation of privacy even in a public restroom and that this expectation was violated.
Researchers Robert Levine and Ara Norenzayan used naturalistic observation to study differences in the “pace of life” across countries (Levine & Norenzayan, 1999). One of their measures involved observing pedestrians in a large city to see how long it took them to walk 60 feet. They found that people in some countries walked reliably faster than people in other countries. For example, people in the United States and Japan covered 60 feet in about 12 seconds on average, while people in Brazil and Romania took close to 17 seconds.
Because naturalistic observation takes place in the complex and even chaotic “real world,” there are two closely related issues that researchers must deal with before collecting data. The first is sampling. When, where, and under what conditions will the observations be made, and who exactly will be observed? Levine and Norenzayan described their sampling process as follows:
Male and female walking speed over a distance of 60 feet was measured in at least two locations in main downtown areas in each city. Measurements were taken during main business hours on clear summer days. All locations were flat, unobstructed, had broad sidewalks, and were sufficiently uncrowded to allow pedestrians to move at potentially maximum speeds. To control for the effects of socializing, only pedestrians walking alone were used. Children, individuals with obvious physical handicaps, and window-shoppers were not timed. Thirty-five men and 35 women were timed in most cities. (p. 186)
Precise specification of the sampling process in this way makes data collection manageable for the observers, and it also provides some control over important extraneous variables. For example, by making their observations on clear summer days in all countries, Levine and Norenzayan controlled for effects of the weather on people’s walking speeds.
The second issue is measurement. What specific behaviors will be observed? In Levine and Norenzayan’s study, measurement was relatively straightforward. They simply measured out a 60-foot distance along a city sidewalk and then used a stopwatch to time participants as they walked over that distance. Often, however, the behaviors of interest are not so obvious or objective. For example, researchers Robert Kraut and Robert Johnston wanted to study bowlers’ reactions to their shots, both when they were facing the pins and then when they turned toward their companions (Kraut & Johnston, 1979). But what “reactions” should they observe? Based on previous research and their own pilot testing, Kraut and Johnston created a list of reactions that included “closed smile,” “open smile,” “laugh,” “neutral face,” “look down,” “look away,” and “face cover” (covering one’s face with one’s hands). The observers committed this list to memory and then practiced by coding the reactions of bowlers who had been videotaped. During the actual study, the observers spoke into an audio recorder, describing the reactions they observed. Among the most interesting results of this study was that bowlers rarely smiled while they still faced the pins. They were much more likely to smile after they turned toward their companions, suggesting that smiling is not purely an expression of happiness but also a form of social communication.
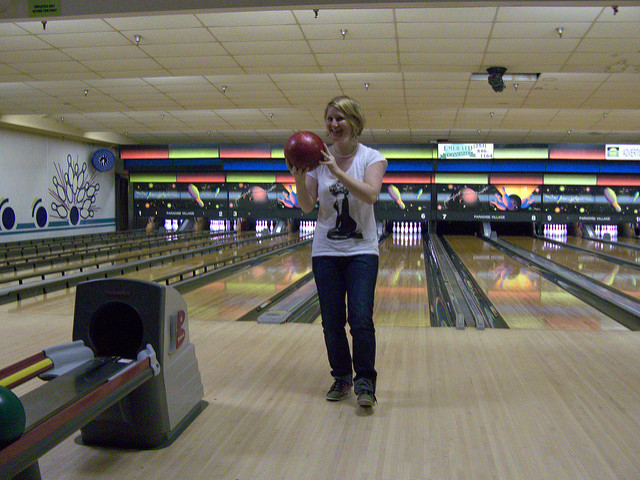
Naturalistic observation has revealed that bowlers tend to smile when they turn away from the pins and toward their companions, suggesting that smiling is not purely an expression of happiness but also a form of social communication.
sieneke toering – bowling big lebowski style – CC BY-NC-ND 2.0.
When the observations require a judgment on the part of the observers—as in Kraut and Johnston’s study—this process is often described as coding . Coding generally requires clearly defining a set of target behaviors. The observers then categorize participants individually in terms of which behavior they have engaged in and the number of times they engaged in each behavior. The observers might even record the duration of each behavior. The target behaviors must be defined in such a way that different observers code them in the same way. This is the issue of interrater reliability. Researchers are expected to demonstrate the interrater reliability of their coding procedure by having multiple raters code the same behaviors independently and then showing that the different observers are in close agreement. Kraut and Johnston, for example, video recorded a subset of their participants’ reactions and had two observers independently code them. The two observers showed that they agreed on the reactions that were exhibited 97% of the time, indicating good interrater reliability.
Archival Data
Another approach to correlational research is the use of archival data , which are data that have already been collected for some other purpose. An example is a study by Brett Pelham and his colleagues on “implicit egotism”—the tendency for people to prefer people, places, and things that are similar to themselves (Pelham, Carvallo, & Jones, 2005). In one study, they examined Social Security records to show that women with the names Virginia, Georgia, Louise, and Florence were especially likely to have moved to the states of Virginia, Georgia, Louisiana, and Florida, respectively.
As with naturalistic observation, measurement can be more or less straightforward when working with archival data. For example, counting the number of people named Virginia who live in various states based on Social Security records is relatively straightforward. But consider a study by Christopher Peterson and his colleagues on the relationship between optimism and health using data that had been collected many years before for a study on adult development (Peterson, Seligman, & Vaillant, 1988). In the 1940s, healthy male college students had completed an open-ended questionnaire about difficult wartime experiences. In the late 1980s, Peterson and his colleagues reviewed the men’s questionnaire responses to obtain a measure of explanatory style—their habitual ways of explaining bad events that happen to them. More pessimistic people tend to blame themselves and expect long-term negative consequences that affect many aspects of their lives, while more optimistic people tend to blame outside forces and expect limited negative consequences. To obtain a measure of explanatory style for each participant, the researchers used a procedure in which all negative events mentioned in the questionnaire responses, and any causal explanations for them, were identified and written on index cards. These were given to a separate group of raters who rated each explanation in terms of three separate dimensions of optimism-pessimism. These ratings were then averaged to produce an explanatory style score for each participant. The researchers then assessed the statistical relationship between the men’s explanatory style as college students and archival measures of their health at approximately 60 years of age. The primary result was that the more optimistic the men were as college students, the healthier they were as older men. Pearson’s r was +.25.
This is an example of content analysis —a family of systematic approaches to measurement using complex archival data. Just as naturalistic observation requires specifying the behaviors of interest and then noting them as they occur, content analysis requires specifying keywords, phrases, or ideas and then finding all occurrences of them in the data. These occurrences can then be counted, timed (e.g., the amount of time devoted to entertainment topics on the nightly news show), or analyzed in a variety of other ways.
Key Takeaways
- Correlational research involves measuring two variables and assessing the relationship between them, with no manipulation of an independent variable.
- Correlational research is not defined by where or how the data are collected. However, some approaches to data collection are strongly associated with correlational research. These include naturalistic observation (in which researchers observe people’s behavior in the context in which it normally occurs) and the use of archival data that were already collected for some other purpose.
Discussion: For each of the following, decide whether it is most likely that the study described is experimental or correlational and explain why.
- An educational researcher compares the academic performance of students from the “rich” side of town with that of students from the “poor” side of town.
- A cognitive psychologist compares the ability of people to recall words that they were instructed to “read” with their ability to recall words that they were instructed to “imagine.”
- A manager studies the correlation between new employees’ college grade point averages and their first-year performance reports.
- An automotive engineer installs different stick shifts in a new car prototype, each time asking several people to rate how comfortable the stick shift feels.
- A food scientist studies the relationship between the temperature inside people’s refrigerators and the amount of bacteria on their food.
- A social psychologist tells some research participants that they need to hurry over to the next building to complete a study. She tells others that they can take their time. Then she observes whether they stop to help a research assistant who is pretending to be hurt.
Kanner, A. D., Coyne, J. C., Schaefer, C., & Lazarus, R. S. (1981). Comparison of two modes of stress measurement: Daily hassles and uplifts versus major life events. Journal of Behavioral Medicine, 4 , 1–39.
Kraut, R. E., & Johnston, R. E. (1979). Social and emotional messages of smiling: An ethological approach. Journal of Personality and Social Psychology, 37 , 1539–1553.
Levine, R. V., & Norenzayan, A. (1999). The pace of life in 31 countries. Journal of Cross-Cultural Psychology, 30 , 178–205.
Pelham, B. W., Carvallo, M., & Jones, J. T. (2005). Implicit egotism. Current Directions in Psychological Science, 14 , 106–110.
Peterson, C., Seligman, M. E. P., & Vaillant, G. E. (1988). Pessimistic explanatory style is a risk factor for physical illness: A thirty-five year longitudinal study. Journal of Personality and Social Psychology, 55 , 23–27.
Research Methods in Psychology Copyright © 2016 by University of Minnesota is licensed under a Creative Commons Attribution-NonCommercial-ShareAlike 4.0 International License , except where otherwise noted.
What is Correlational Research? (+ Design, Examples)
Appinio Research · 04.03.2024 · 30min read
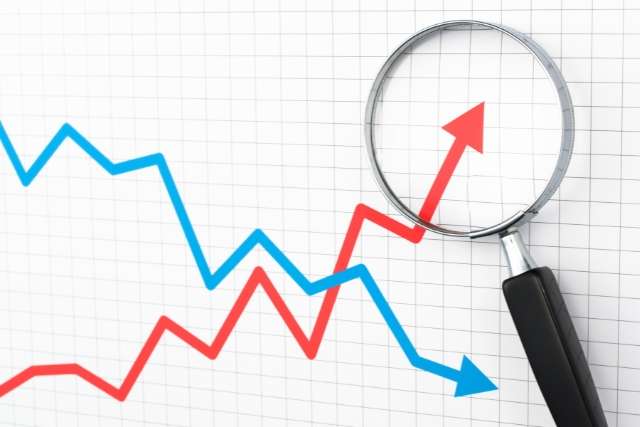
Ever wondered how researchers explore connections between different factors without manipulating them? Correlational research offers a window into understanding the relationships between variables in the world around us. From examining the link between exercise habits and mental well-being to exploring patterns in consumer behavior, correlational studies help us uncover insights that shape our understanding of human behavior, inform decision-making, and drive innovation. In this guide, we'll dive into the fundamentals of correlational research, exploring its definition, importance, ethical considerations, and practical applications across various fields. Whether you're a student delving into research methods or a seasoned researcher seeking to expand your methodological toolkit, this guide will equip you with the knowledge and skills to conduct and interpret correlational studies effectively.
What is Correlational Research?
Correlational research is a methodological approach used in scientific inquiry to examine the relationship between two or more variables. Unlike experimental research , which seeks to establish cause-and-effect relationships through manipulation and control of variables, correlational research focuses on identifying and quantifying the degree to which variables are related to one another. This method allows researchers to investigate associations, patterns, and trends in naturalistic settings without imposing experimental manipulations.
Importance of Correlational Research
Correlational research plays a crucial role in advancing scientific knowledge across various disciplines. Its importance stems from several key factors:
- Exploratory Analysis : Correlational studies provide a starting point for exploring potential relationships between variables. By identifying correlations, researchers can generate hypotheses and guide further investigation into causal mechanisms and underlying processes.
- Predictive Modeling : Correlation coefficients can be used to predict the behavior or outcomes of one variable based on the values of another variable. This predictive ability has practical applications in fields such as economics, psychology, and epidemiology, where forecasting future trends or outcomes is essential.
- Diagnostic Purposes: Correlational analyses can help identify patterns or associations that may indicate the presence of underlying conditions or risk factors. For example, correlations between certain biomarkers and disease outcomes can inform diagnostic criteria and screening protocols in healthcare.
- Theory Development: Correlational research contributes to theory development by providing empirical evidence for proposed relationships between variables. Researchers can refine and validate theoretical models in their respective fields by systematically examining correlations across different contexts and populations.
- Ethical Considerations: In situations where experimental manipulation is not feasible or ethical, correlational research offers an alternative approach to studying naturally occurring phenomena. This allows researchers to address research questions that may otherwise be inaccessible or impractical to investigate.
Correlational vs. Causation in Research
It's important to distinguish between correlation and causation in research. While correlational studies can identify relationships between variables, they cannot establish causal relationships on their own. Several factors contribute to this distinction:
- Directionality: Correlation does not imply the direction of causation. A correlation between two variables does not indicate which variable is causing the other; it merely suggests that they are related in some way. Additional evidence, such as experimental manipulation or longitudinal studies , is needed to establish causality.
- Third Variables: Correlations may be influenced by third variables, also known as confounding variables, that are not directly measured or controlled in the study. These third variables can create spurious correlations or obscure true causal relationships between the variables of interest.
- Temporal Sequence: Causation requires a temporal sequence, with the cause preceding the effect in time. Correlational studies alone cannot establish the temporal order of events, making it difficult to determine whether one variable causes changes in another or vice versa.
Understanding the distinction between correlation and causation is critical for interpreting research findings accurately and drawing valid conclusions about the relationships between variables. While correlational research provides valuable insights into associations and patterns, establishing causation typically requires additional evidence from experimental studies or other research designs.
Key Concepts in Correlation
Understanding key concepts in correlation is essential for conducting meaningful research and interpreting results accurately.
Correlation Coefficient
The correlation coefficient is a statistical measure that quantifies the strength and direction of the relationship between two variables. It's denoted by the symbol r and ranges from -1 to +1.
- A correlation coefficient of -1 indicates a perfect negative correlation, meaning that as one variable increases, the other decreases in a perfectly predictable manner.
- A coefficient of +1 signifies a perfect positive correlation, where both variables increase or decrease together in perfect sync.
- A coefficient of 0 implies no correlation, indicating no systematic relationship between the variables.
Strength and Direction of Correlation
The strength of correlation refers to how closely the data points cluster around a straight line on the scatterplot. A correlation coefficient close to -1 or +1 indicates a strong relationship between the variables, while a coefficient close to 0 suggests a weak relationship.
- Strong correlation: When the correlation coefficient approaches -1 or +1, it indicates a strong relationship between the variables. For example, a correlation coefficient of -0.9 suggests a strong negative relationship, while a coefficient of +0.8 indicates a strong positive relationship.
- Weak correlation: A correlation coefficient close to 0 indicates a weak or negligible relationship between the variables. For instance, a coefficient of -0.1 or +0.1 suggests a weak correlation where the variables are minimally related.
The direction of correlation determines how the variables change relative to each other.
- Positive correlation: When one variable increases, the other variable also tends to increase. Conversely, when one variable decreases, the other variable tends to decrease. This is represented by a positive correlation coefficient.
- Negative correlation: In a negative correlation, as one variable increases, the other variable tends to decrease. Similarly, when one variable decreases, the other variable tends to increase. This relationship is indicated by a negative correlation coefficient.
Scatterplots
A scatterplot is a graphical representation of the relationship between two variables. Each data point on the plot represents the values of both variables for a single observation. By plotting the data points on a Cartesian plane, you can visualize patterns and trends in the relationship between the variables.
- Interpretation: When examining a scatterplot, observe the pattern of data points. If the points cluster around a straight line, it indicates a strong correlation. However, if the points are scattered randomly, it suggests a weak or no correlation.
- Outliers: Identify any outliers or data points that deviate significantly from the overall pattern. Outliers can influence the correlation coefficient and may warrant further investigation to determine their impact on the relationship between variables.
- Line of Best Fit: In some cases, you may draw a line of best fit through the data points to visually represent the overall trend in the relationship. This line can help illustrate the direction and strength of the correlation between the variables.
Understanding these key concepts will enable you to interpret correlation coefficients accurately and draw meaningful conclusions from your data.
How to Design a Correlational Study?
When embarking on a correlational study, careful planning and consideration are crucial to ensure the validity and reliability of your research findings.
Research Question Formulation
Formulating clear and focused research questions is the cornerstone of any successful correlational study. Your research questions should articulate the variables you intend to investigate and the nature of the relationship you seek to explore. When formulating your research questions:
- Be Specific: Clearly define the variables you are interested in studying and the population to which your findings will apply.
- Be Testable: Ensure that your research questions are empirically testable using correlational methods. Avoid vague or overly broad questions that are difficult to operationalize.
- Consider Prior Research: Review existing literature to identify gaps or unanswered questions in your area of interest. Your research questions should build upon prior knowledge and contribute to advancing the field.
For example, if you're interested in examining the relationship between sleep duration and academic performance among college students, your research question might be: "Is there a significant correlation between the number of hours of sleep per night and GPA among undergraduate students?"
Participant Selection
Selecting an appropriate sample of participants is critical to ensuring the generalizability and validity of your findings. Consider the following factors when selecting participants for your correlational study:
- Population Characteristics: Identify the population of interest for your study and ensure that your sample reflects the demographics and characteristics of this population.
- Sampling Method: Choose a sampling method that is appropriate for your research question and accessible, given your resources and constraints. Standard sampling methods include random sampling, stratified sampling, and convenience sampling.
- Sample Size: Determine the appropriate sample size based on factors such as the effect size you expect to detect, the desired level of statistical power, and practical considerations such as time and budget constraints.
For example, suppose you're studying the relationship between exercise habits and mental health outcomes in adults aged 18-65. In that case, you might use stratified random sampling to ensure representation from different age groups within the population.
Variables Identification
Identifying and operationalizing the variables of interest is essential for conducting a rigorous correlational study. When identifying variables for your research:
- Independent and Dependent Variables: Clearly distinguish between independent variables (factors that are hypothesized to influence the outcome) and dependent variables (the outcomes or behaviors of interest).
- Control Variables: Identify any potential confounding variables or extraneous factors that may influence the relationship between your independent and dependent variables. These variables should be controlled for in your analysis.
- Measurement Scales: Determine the appropriate measurement scales for your variables (e.g., nominal, ordinal, interval, or ratio) and select valid and reliable measures for assessing each construct.
For instance, if you're investigating the relationship between socioeconomic status (SES) and academic achievement, SES would be your independent variable, while academic achievement would be your dependent variable. You might measure SES using a composite index based on factors such as income, education level, and occupation.
Data Collection Methods
Selecting appropriate data collection methods is essential for obtaining reliable and valid data for your correlational study. When choosing data collection methods:
- Quantitative vs. Qualitative : Determine whether quantitative or qualitative methods are best suited to your research question and objectives. Correlational studies typically involve quantitative data collection methods like surveys, questionnaires, or archival data analysis.
- Instrument Selection: Choose measurement instruments that are valid, reliable, and appropriate for your variables of interest. Pilot test your instruments to ensure clarity and comprehension among your target population.
- Data Collection Procedures : Develop clear and standardized procedures for data collection to minimize bias and ensure consistency across participants and time points.
For example, if you're examining the relationship between smartphone use and sleep quality among adolescents, you might administer a self-report questionnaire assessing smartphone usage patterns and sleep quality indicators such as sleep duration and sleep disturbances.
Crafting a well-designed correlational study is essential for yielding meaningful insights into the relationships between variables. By meticulously formulating research questions , selecting appropriate participants, identifying relevant variables, and employing effective data collection methods, researchers can ensure the validity and reliability of their findings.
With Appinio , conducting correlational research becomes even more seamless and efficient. Our intuitive platform empowers researchers to gather real-time consumer insights in minutes, enabling them to make informed decisions with confidence.
Experience the power of Appinio and unlock valuable insights for your research endeavors. Schedule a demo today and revolutionize the way you conduct correlational studies!
Book a Demo
How to Analyze Correlational Data?
Once you have collected your data in a correlational study, the next crucial step is to analyze it effectively to draw meaningful conclusions about the relationship between variables.
How to Calculate Correlation Coefficients?
The correlation coefficient is a numerical measure that quantifies the strength and direction of the relationship between two variables. There are different types of correlation coefficients, including Pearson's correlation coefficient (for linear relationships), Spearman's rank correlation coefficient (for ordinal data ), and Kendall's tau (for non-parametric data). Here, we'll focus on calculating Pearson's correlation coefficient (r), which is commonly used for interval or ratio-level data.
To calculate Pearson's correlation coefficient (r), you can use statistical software such as SPSS, R, or Excel. However, if you prefer to calculate it manually, you can use the following formula:
r = Σ((X - X̄)(Y - Ȳ)) / ((n - 1) * (s_X * s_Y))
- X and Y are the scores of the two variables,
- X̄ and Ȳ are the means of X and Y, respectively,
- n is the number of data points,
- s_X and s_Y are the standard deviations of X and Y, respectively.
Interpreting Correlation Results
Once you have calculated the correlation coefficient (r), it's essential to interpret the results correctly. When interpreting correlation results:
- Magnitude: The absolute value of the correlation coefficient (r) indicates the strength of the relationship between the variables. A coefficient close to 1 or -1 suggests a strong correlation, while a coefficient close to 0 indicates a weak or no correlation.
- Direction: The sign of the correlation coefficient (positive or negative) indicates the direction of the relationship between the variables. A positive correlation coefficient indicates a positive relationship (as one variable increases, the other tends to increase), while a negative correlation coefficient indicates a negative relationship (as one variable increases, the other tends to decrease).
- Statistical Significance : Assess the statistical significance of the correlation coefficient to determine whether the observed relationship is likely to be due to chance. This is typically done using hypothesis testing, where you compare the calculated correlation coefficient to a critical value based on the sample size and desired level of significance (e.g., α =0.05).
Statistical Significance
Determining the statistical significance of the correlation coefficient involves conducting hypothesis testing to assess whether the observed correlation is likely to occur by chance. The most common approach is to use a significance level (alpha, α ) of 0.05, which corresponds to a 5% chance of obtaining the observed correlation coefficient if there is no true relationship between the variables.
To test the null hypothesis that the correlation coefficient is zero (i.e., no correlation), you can use inferential statistics such as the t-test or z-test. If the calculated p-value is less than the chosen significance level (e.g., p <0.05), you can reject the null hypothesis and conclude that the correlation coefficient is statistically significant.
Remember that statistical significance does not necessarily imply practical significance or the strength of the relationship. Even a statistically significant correlation with a small effect size may not be meaningful in practical terms.
By understanding how to calculate correlation coefficients, interpret correlation results, and assess statistical significance, you can effectively analyze correlational data and draw accurate conclusions about the relationships between variables in your study.
Correlational Research Limitations
As with any research methodology, correlational studies have inherent considerations and limitations that researchers must acknowledge and address to ensure the validity and reliability of their findings.
Third Variables
One of the primary considerations in correlational research is the presence of third variables, also known as confounding variables. These are extraneous factors that may influence or confound the observed relationship between the variables under study. Failing to account for third variables can lead to spurious correlations or erroneous conclusions about causality.
For example, consider a correlational study examining the relationship between ice cream consumption and drowning incidents. While these variables may exhibit a positive correlation during the summer months, the true causal factor is likely to be a third variable—such as hot weather—that influences both ice cream consumption and swimming activities, thereby increasing the risk of drowning.
To address the influence of third variables, researchers can employ various strategies, such as statistical control techniques, experimental designs (when feasible), and careful operationalization of variables.
Causal Inferences
Correlation does not imply causation—a fundamental principle in correlational research. While correlational studies can identify relationships between variables, they cannot determine causality. This is because correlation merely describes the degree to which two variables co-vary; it does not establish a cause-and-effect relationship between them.
For example, consider a correlational study that finds a positive relationship between the frequency of exercise and self-reported happiness. While it may be tempting to conclude that exercise causes happiness, it's equally plausible that happier individuals are more likely to exercise regularly. Without experimental manipulation and control over potential confounding variables, causal inferences cannot be made.
To strengthen causal inferences in correlational research, researchers can employ longitudinal designs, experimental methods (when ethical and feasible), and theoretical frameworks to guide their interpretations.
Sample Size and Representativeness
The size and representativeness of the sample are critical considerations in correlational research. A small or non-representative sample may limit the generalizability of findings and increase the risk of sampling bias .
For example, if a correlational study examines the relationship between socioeconomic status (SES) and educational attainment using a sample composed primarily of high-income individuals, the findings may not accurately reflect the broader population's experiences. Similarly, an undersized sample may lack the statistical power to detect meaningful correlations or relationships.
To mitigate these issues, researchers should aim for adequate sample sizes based on power analyses, employ random or stratified sampling techniques to enhance representativeness and consider the demographic characteristics of the target population when interpreting findings.
Ensure your survey delivers accurate insights by using our Sample Size Calculator . With customizable options for margin of error, confidence level, and standard deviation, you can determine the optimal sample size to ensure representative results. Make confident decisions backed by robust data.
Reliability and Validity
Ensuring the reliability and validity of measures is paramount in correlational research. Reliability refers to the consistency and stability of measurement over time, whereas validity pertains to the accuracy and appropriateness of measurement in capturing the intended constructs.
For example, suppose a correlational study utilizes self-report measures of depression and anxiety. In that case, it's essential to assess the measures' reliability (e.g., internal consistency, test-retest reliability) and validity (e.g., content validity, criterion validity) to ensure that they accurately reflect participants' mental health status.
To enhance reliability and validity in correlational research, researchers can employ established measurement scales, pilot-test instruments, use multiple measures of the same construct, and assess convergent and discriminant validity.
By addressing these considerations and limitations, researchers can enhance the robustness and credibility of their correlational studies and make more informed interpretations of their findings.
Correlational Research Examples and Applications
Correlational research is widely used across various disciplines to explore relationships between variables and gain insights into complex phenomena. We'll examine examples and applications of correlational studies, highlighting their practical significance and impact on understanding human behavior and societal trends across various industries and use cases.
Psychological Correlational Studies
In psychology, correlational studies play a crucial role in understanding various aspects of human behavior, cognition, and mental health. Researchers use correlational methods to investigate relationships between psychological variables and identify factors that may contribute to or predict specific outcomes.
For example, a psychological correlational study might examine the relationship between self-esteem and depression symptoms among adolescents. By administering self-report measures of self-esteem and depression to a sample of teenagers and calculating the correlation coefficient between the two variables, researchers can assess whether lower self-esteem is associated with higher levels of depression symptoms.
Other examples of psychological correlational studies include investigating the relationship between:
- Parenting styles and academic achievement in children
- Personality traits and job performance in the workplace
- Stress levels and coping strategies among college students
These studies provide valuable insights into the factors influencing human behavior and mental well-being, informing interventions and treatment approaches in clinical and counseling settings.
Business Correlational Studies
Correlational research is also widely utilized in the business and management fields to explore relationships between organizational variables and outcomes. By examining correlations between different factors within an organization, researchers can identify patterns and trends that may impact performance, productivity, and profitability.
For example, a business correlational study might investigate the relationship between employee satisfaction and customer loyalty in a retail setting. By surveying employees to assess their job satisfaction levels and analyzing customer feedback and purchase behavior, researchers can determine whether higher employee satisfaction is correlated with increased customer loyalty and retention.
Other examples of business correlational studies include examining the relationship between:
- Leadership styles and employee motivation
- Organizational culture and innovation
- Marketing strategies and brand perception
These studies provide valuable insights for organizations seeking to optimize their operations, improve employee engagement, and enhance customer satisfaction.
Marketing Correlational Studies
In marketing, correlational studies are instrumental in understanding consumer behavior, identifying market trends, and optimizing marketing strategies. By examining correlations between various marketing variables, researchers can uncover insights that drive effective advertising campaigns, product development, and brand management.
For example, a marketing correlational study might explore the relationship between social media engagement and brand loyalty among millennials. By collecting data on millennials' social media usage, brand interactions, and purchase behaviors, researchers can analyze whether higher levels of social media engagement correlate with increased brand loyalty and advocacy.
Another example of a marketing correlational study could focus on investigating the relationship between pricing strategies and customer satisfaction in the retail sector. By analyzing data on pricing fluctuations, customer feedback , and sales performance, researchers can assess whether pricing strategies such as discounts or promotions impact customer satisfaction and repeat purchase behavior.
Other potential areas of inquiry in marketing correlational studies include examining the relationship between:
- Product features and consumer preferences
- Advertising expenditures and brand awareness
- Online reviews and purchase intent
These studies provide valuable insights for marketers seeking to optimize their strategies, allocate resources effectively, and build strong relationships with consumers in an increasingly competitive marketplace. By leveraging correlational methods, marketers can make data-driven decisions that drive business growth and enhance customer satisfaction.
Correlational Research Ethical Considerations
Ethical considerations are paramount in all stages of the research process, including correlational studies. Researchers must adhere to ethical guidelines to ensure the rights, well-being, and privacy of participants are protected. Key ethical considerations to keep in mind include:
- Informed Consent: Obtain informed consent from participants before collecting any data. Clearly explain the purpose of the study, the procedures involved, and any potential risks or benefits. Participants should have the right to withdraw from the study at any time without consequence.
- Confidentiality: Safeguard the confidentiality of participants' data. Ensure that any personal or sensitive information collected during the study is kept confidential and is only accessible to authorized individuals. Use anonymization techniques when reporting findings to protect participants' privacy.
- Voluntary Participation: Ensure that participation in the study is voluntary and not coerced. Participants should not feel pressured to take part in the study or feel that they will suffer negative consequences for declining to participate.
- Avoiding Harm: Take measures to minimize any potential physical, psychological, or emotional harm to participants. This includes avoiding deceptive practices, providing appropriate debriefing procedures (if necessary), and offering access to support services if participants experience distress.
- Deception: If deception is necessary for the study, it must be justified and minimized. Deception should be disclosed to participants as soon as possible after data collection, and any potential risks associated with the deception should be mitigated.
- Researcher Integrity: Maintain integrity and honesty throughout the research process. Avoid falsifying data, manipulating results, or engaging in any other unethical practices that could compromise the integrity of the study.
- Respect for Diversity: Respect participants' cultural, social, and individual differences. Ensure that research protocols are culturally sensitive and inclusive, and that participants from diverse backgrounds are represented and treated with respect.
- Institutional Review: Obtain ethical approval from institutional review boards or ethics committees before commencing the study. Adhere to the guidelines and regulations set forth by the relevant governing bodies and professional organizations.
Adhering to these ethical considerations ensures that correlational research is conducted responsibly and ethically, promoting trust and integrity in the scientific community.
Correlational Research Best Practices and Tips
Conducting a successful correlational study requires careful planning, attention to detail, and adherence to best practices in research methodology. Here are some tips and best practices to help you conduct your correlational research effectively:
- Clearly Define Variables: Clearly define the variables you are studying and operationalize them into measurable constructs. Ensure that your variables are accurately and consistently measured to avoid ambiguity and ensure reliability.
- Use Valid and Reliable Measures: Select measurement instruments that are valid and reliable for assessing your variables of interest. Pilot test your measures to ensure clarity, comprehension, and appropriateness for your target population.
- Consider Potential Confounding Variables: Identify and control for potential confounding variables that could influence the relationship between your variables of interest. Consider including control variables in your analysis to isolate the effects of interest.
- Ensure Adequate Sample Size: Determine the appropriate sample size based on power analyses and considerations of statistical power. Larger sample sizes increase the reliability and generalizability of your findings.
- Random Sampling: Whenever possible, use random sampling techniques to ensure that your sample is representative of the population you are studying. If random sampling is not feasible, carefully consider the characteristics of your sample and the extent to which findings can be generalized.
- Statistical Analysis : Choose appropriate statistical techniques for analyzing your data, taking into account the nature of your variables and research questions. Consult with a statistician if necessary to ensure the validity and accuracy of your analyses.
- Transparent Reporting: Transparently report your methods, procedures, and findings in accordance with best practices in research reporting. Clearly articulate your research questions, methods, results, and interpretations to facilitate reproducibility and transparency.
- Peer Review: Seek feedback from colleagues, mentors, or peer reviewers throughout the research process. Peer review helps identify potential flaws or biases in your study design, analysis, and interpretation, improving your research's overall quality and credibility.
By following these best practices and tips, you can conduct your correlational research with rigor, integrity, and confidence, leading to valuable insights and contributions to your field.
Conclusion for Correlational Research
Correlational research serves as a powerful tool for uncovering connections between variables in the world around us. By examining the relationships between different factors, researchers can gain valuable insights into human behavior, health outcomes, market trends, and more. While correlational studies cannot establish causation on their own, they provide a crucial foundation for generating hypotheses, predicting outcomes, and informing decision-making in various fields. Understanding the principles and practices of correlational research empowers researchers to explore complex phenomena, advance scientific knowledge, and address real-world challenges. Moreover, embracing ethical considerations and best practices in correlational research ensures the integrity, validity, and reliability of study findings. By prioritizing informed consent, confidentiality, and participant well-being, researchers can conduct studies that uphold ethical standards and contribute meaningfully to the body of knowledge. Incorporating transparent reporting, peer review, and continuous learning further enhances the quality and credibility of correlational research. Ultimately, by leveraging correlational methods responsibly and ethically, researchers can unlock new insights, drive innovation, and make a positive impact on society.
How to Collect Data for Correlational Research in Minutes?
Discover the revolutionary power of Appinio , the real-time market research platform. With Appinio, conducting your own correlational research has never been easier or more exciting. Gain access to real-time consumer insights, empowering you to make data-driven decisions in minutes. Here's why Appinio stands out:
- From questions to insights in minutes: Say goodbye to lengthy research processes. With Appinio, you can gather valuable insights swiftly, allowing you to act on them immediately.
- Intuitive platform for everyone: No need for a PhD in research. Appinio's user-friendly interface makes it accessible to anyone, empowering you to conduct professional-grade research effortlessly.
- Extensive reach, global impact: Define your target group from over 1200 characteristics and survey consumers in over 90 countries. With Appinio, the world is your research playground.
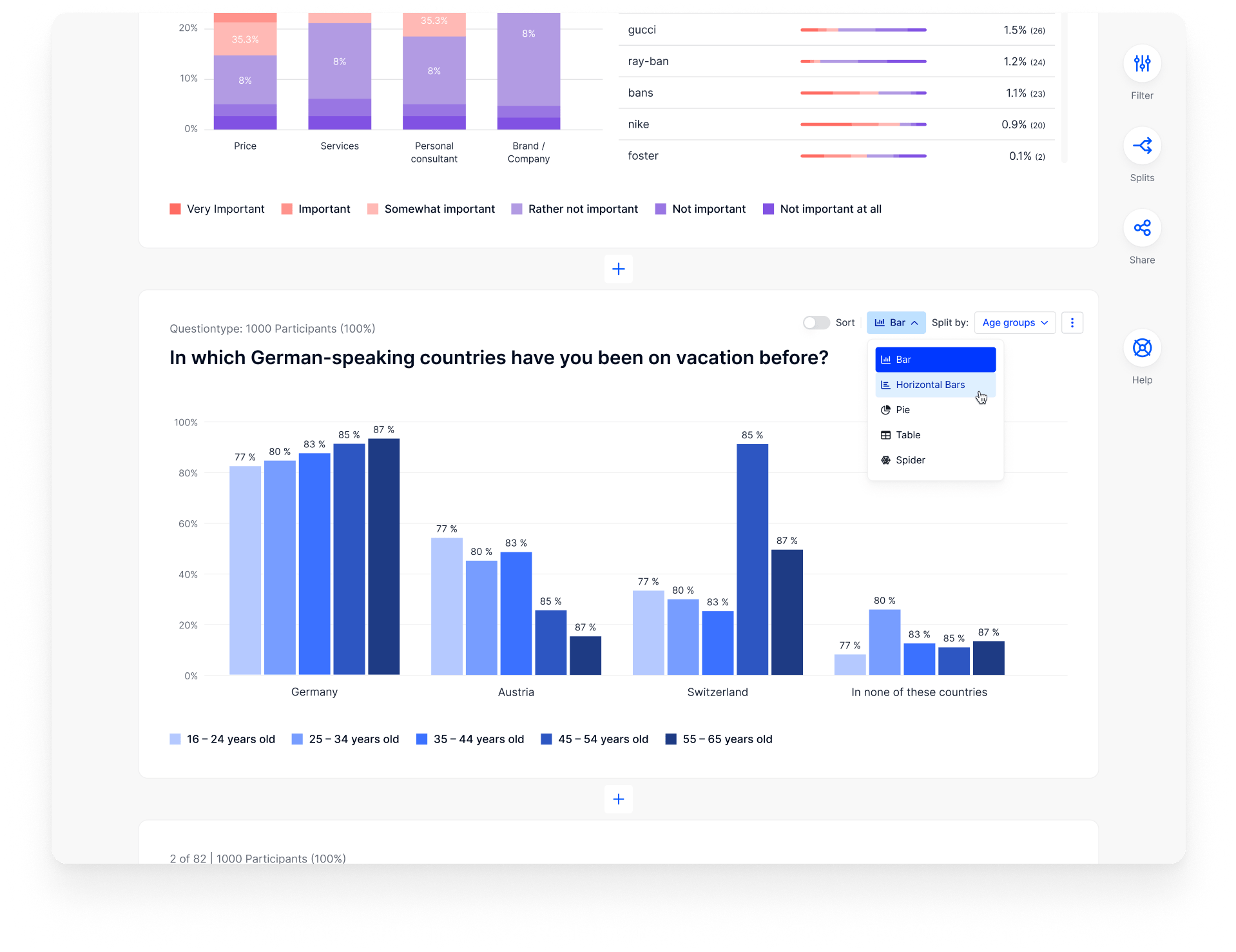
Get free access to the platform!
Join the loop 💌
Be the first to hear about new updates, product news, and data insights. We'll send it all straight to your inbox.
Get the latest market research news straight to your inbox! 💌
Wait, there's more
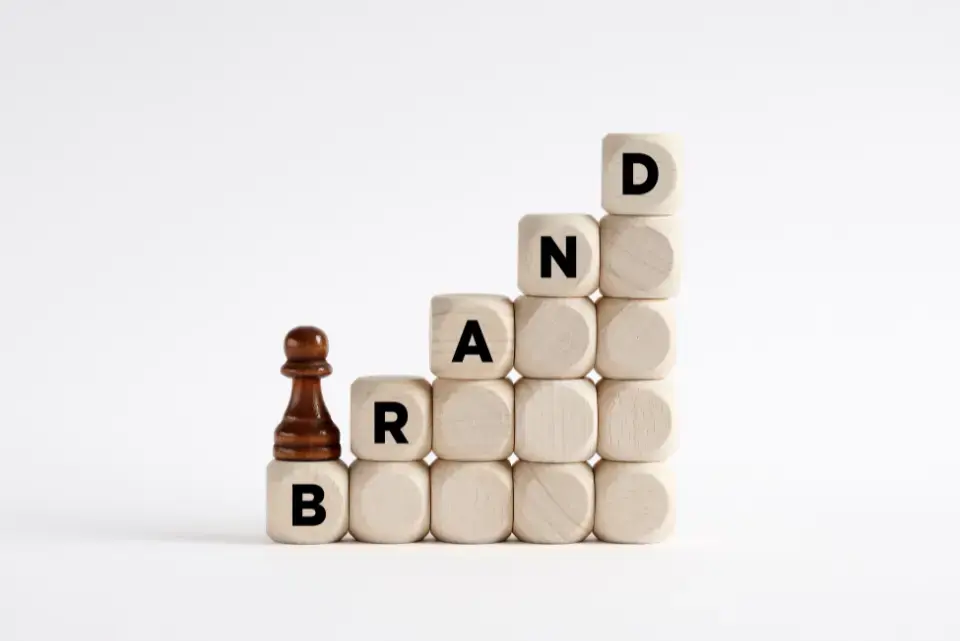
26.06.2024 | 35min read
Brand Development: Definition, Process, Strategies, Examples
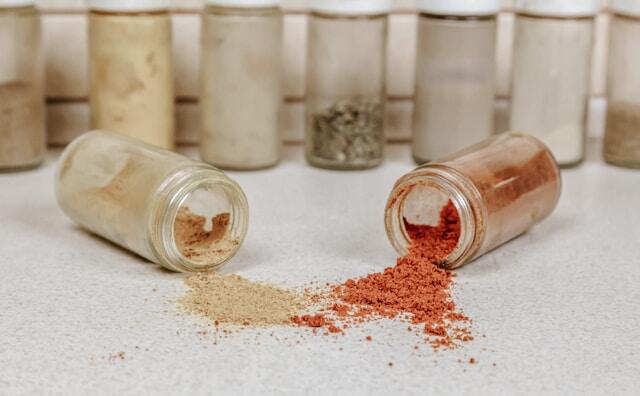
18.06.2024 | 7min read
Future Flavors: How Burger King nailed Concept Testing with Appinio's Predictive Insights
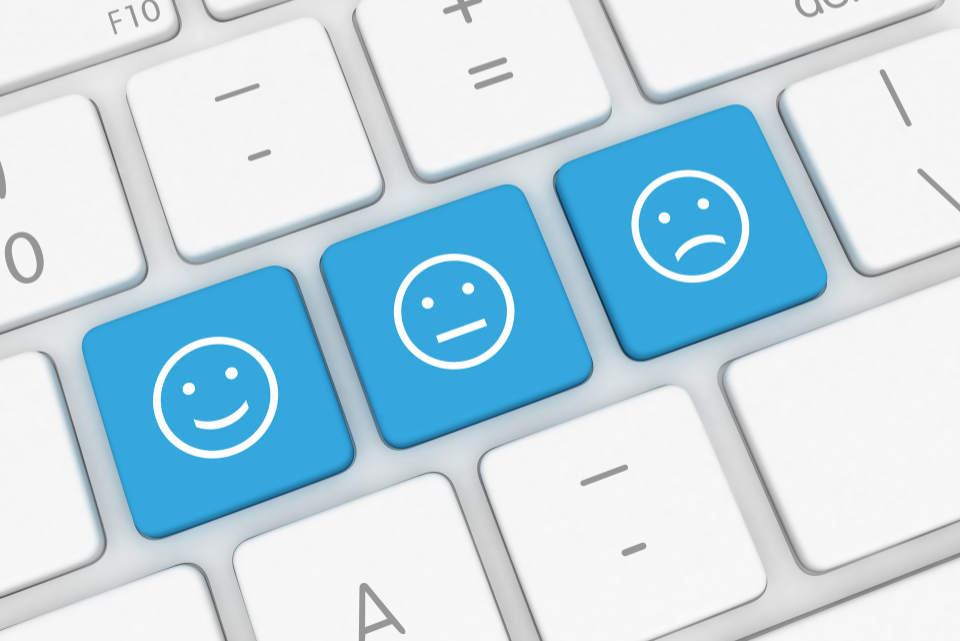
18.06.2024 | 32min read
What is a Pulse Survey? Definition, Types, Questions
- How it works
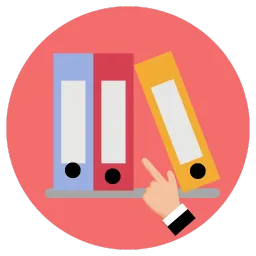
Correlational Research – Steps & Examples
Published by Carmen Troy at August 14th, 2021 , Revised On August 29, 2023
In correlational research design , a researcher measures the association between two or more variables or sets of scores. A researcher doesn’t have control over the variables .
Example: Relationship between income and age.
Types of Correlations
Based on the number of variables
Type of correlation | Definition | Example |
---|---|---|
Simple correlation | A simple correlation aims at studying the relationship between only two variables. | Correlation between height and weight. |
Partial correlation | In partial correlation, you consider multiple variables but focus on the relationship between them and assume other variables as constant. | Correlation between investment and profit when the influence of production cost and advertisement cost remains constant. |
Multiple correlations | Multiple correlations aim at studying the association between three or more variables. | Capital, production, Cost, Advertisement cost, and profit. |
Based on the direction of change of variables
Type of correlation | Definition | Example |
---|---|---|
Positive correlation | The two variables change in a similar direction. | If fat increases, the weight also increases. |
Negative correlation | The two variables change in the opposite direction. | Drinking warm water decreases body fat. |
Zero correlation | The two variables are not interrelated. | There is no relationship between drinking water and increasing height. |
When to Use Correlation Design?
Correlation research design is used when experimental studies are difficult to design.
Example: You want to know the impact of tobacco on people’s health and the extent of their addiction. You can’t distribute tobacco among your participants to understand its effect and addiction level. Instead of it, you can collect information from the people who are already addicted to tobacco and affected by it.
It is used to identify the association between two or more variables.
Example: You want to find out whether there is a correlation between the increasing population and poverty among the people. You don’t think that an increasing population leads to unemployment, but identifying a relationship can help you find a better answer to your study.
Example: You want to find out whether high income causes obesity. However, you don’t see any relationship. However, you can still find out the association between the lifestyle, age, and eating patterns of the people to make predictions of your research question.
Does your Research Methodology Have the Following?
- Great Research/Sources
- Perfect Language
- Accurate Sources
If not, we can help. Our panel of experts makes sure to keep the 3 pillars of Research Methodology strong.
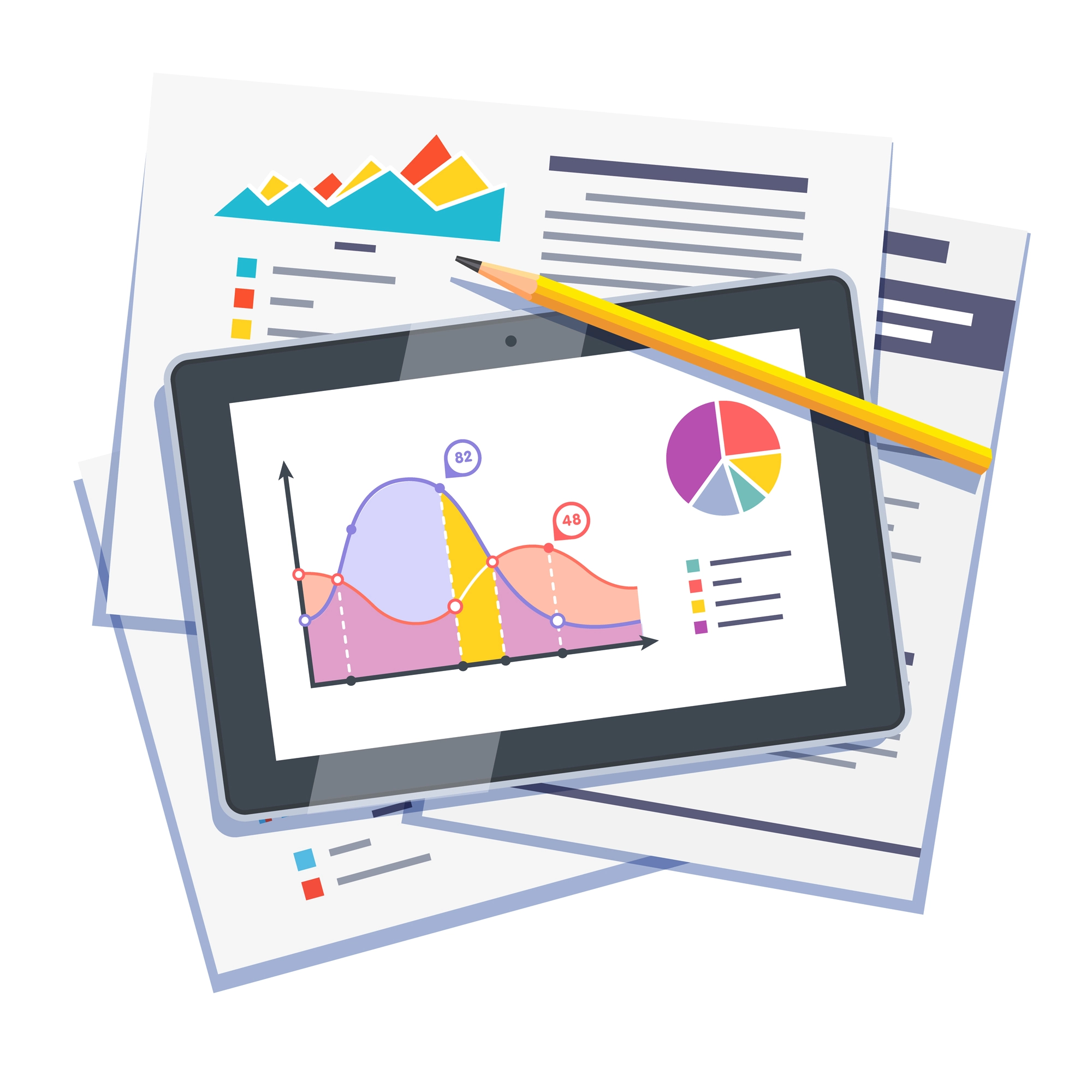
How to Conduct Correlation Research?
Step 1: select the problem.
You can select the issues according to the requirement of your research. There are three common types of problems as follows;
- Is there any relationship between the two variables?
- How well does a variable predict another variable?
- What could be the association between a large number of variables and what predictions you can make?
Step 2: Select the Sample
You need to select the sample carefully and randomly if necessary. Your sample size should not be more than 30.
Step 3: Collect the Data
There are various types of data collection methods used in correlational research. The most common methods used for data collection are as follows:
Surveys are the most frequently used method for collecting data. It helps find the association between variables based on the participants’ responses selected for the study. You can carry out the surveys online, face-to-face, and on the phone.
Example: You want to find out the association between poverty and unemployment. You need to distribute a questionnaire about the sources of income and expenses among the participants. You can analyse the information obtained to identify whether unemployment leads to poverty.
Pros | Cons |
---|---|
Easy to conduct. You get quick responses. | Responses may not be reliable or dishonest. Some questions may not be easier to analyse |
Naturalistic Observation
In the naturalistic observation method, you need to collect the participants’ data by observing them in their natural surroundings. You can consider it as a type of field research. You can observe people and gather information from them in various public places such as stores, malls, parks, playgrounds, etc. The participants are not informed about the research. However, you need to ensure the anonymity of the participants. It includes both qualitative and quantitative data.
Example: You want to find out the correlation between the price hike of vegetables and whether changes. You need to visit the market and talk to vegetable vendors to collect the required information. You can categorise the information according to the price, whether change effects and challenges the vendors/farmers face during such periods.
Pros | Cons |
---|---|
It can be conducted in a natural environment. The observation is natural without any manipulation. It provides better qualitative data. | A researcher cannot control the variables. Lack of rigidity and standardisation. |
Archival Data
Archival data is a type of data or information that already exists. Instead of collecting new data, you can use the existing data in your research if it fulfills your research requirements. Generally, previous studies or theories, records, documents, and transcripts are used as the primary source of information. This type of research is also called retrospective research.
Example: Suppose you want to find out the relation between exercise and weight loss. You can use various scholarly journals, health records, and scientific studies and discoveries based on people’s age and gender. You can identify whether exercise leads to significant weight loss among people of various ages and gender.
Pros | Cons |
---|---|
The researcher has control over variables. Easy to establish the relationship between cause and effect. Inexpensive and convenient. | The artificial environment may impact the behaviour of the participants. Inaccurate results |
Pros | Cons |
---|---|
Cost-effective Suitable for trend analysis and identification. An ample amount of existing data is available. | You need to manipulate data to make it relevant. Information may be incomplete or inaccurate. |
What is Causation?
The association between cause and effect is called causation . You can identify the correlation between the two variables, but they may not influence each other. It can be considered as the limitation of correlation research.
Example: You’ve found that people who exercise regularly lost maximum weight. However, it doesn’t prove that people who don’t use will gain weight. There could be many other possible variables, such as a healthy diet, age, stress, gender, and health condition, impacting people’s weight. You can’t find out the causation of your research problem. Still, you can collect and analyse data to support the theory. You can only predict the possibilities of the method, phenomena, or problem you are studying.
Frequently Asked Questions
How to describe correlational research.
Correlational research examines the relationship between two or more variables. It doesn’t imply causation but measures the strength and direction of association. Statistical analysis determines if changes in one variable correspond to changes in another, helping understand patterns and predict outcomes.
You May Also Like
Textual analysis is the method of analysing and understanding the text. We need to look carefully at the text to identify the writer’s context and message.
Ethnography is a type of research where a researcher observes the people in their natural environment. Here is all you need to know about ethnography.
In historical research, a researcher collects and analyse the data, and explain the events that occurred in the past to test the truthfulness of observations.
USEFUL LINKS
LEARNING RESOURCES
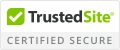
COMPANY DETAILS
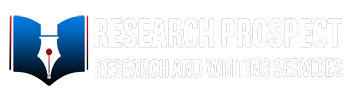
- How It Works
- Bipolar Disorder
- Therapy Center
- When To See a Therapist
- Types of Therapy
- Best Online Therapy
- Best Couples Therapy
- Best Family Therapy
- Managing Stress
- Sleep and Dreaming
- Understanding Emotions
- Self-Improvement
- Healthy Relationships
- Student Resources
- Personality Types
- Guided Meditations
- Verywell Mind Insights
- 2024 Verywell Mind 25
- Mental Health in the Classroom
- Editorial Process
- Meet Our Review Board
- Crisis Support
Correlation Studies in Psychology Research
Determining the relationship between two or more variables.
Kendra Cherry, MS, is a psychosocial rehabilitation specialist, psychology educator, and author of the "Everything Psychology Book."
:max_bytes(150000):strip_icc():format(webp)/IMG_9791-89504ab694d54b66bbd72cb84ffb860e.jpg)
Emily is a board-certified science editor who has worked with top digital publishing brands like Voices for Biodiversity, Study.com, GoodTherapy, Vox, and Verywell.
:max_bytes(150000):strip_icc():format(webp)/Emily-Swaim-1000-0f3197de18f74329aeffb690a177160c.jpg)
Verywell / Brianna Gilmartin
- Characteristics
Potential Pitfalls
Frequently asked questions.
A correlational study is a type of research design that looks at the relationships between two or more variables. Correlational studies are non-experimental, which means that the experimenter does not manipulate or control any of the variables.
A correlation refers to a relationship between two variables. Correlations can be strong or weak and positive or negative. Sometimes, there is no correlation.
There are three possible outcomes of a correlation study: a positive correlation, a negative correlation, or no correlation. Researchers can present the results using a numerical value called the correlation coefficient, a measure of the correlation strength. It can range from –1.00 (negative) to +1.00 (positive). A correlation coefficient of 0 indicates no correlation.
- Positive correlations : Both variables increase or decrease at the same time. A correlation coefficient close to +1.00 indicates a strong positive correlation.
- Negative correlations : As the amount of one variable increases, the other decreases (and vice versa). A correlation coefficient close to -1.00 indicates a strong negative correlation.
- No correlation : There is no relationship between the two variables. A correlation coefficient of 0 indicates no correlation.
Characteristics of a Correlational Study
Correlational studies are often used in psychology, as well as other fields like medicine. Correlational research is a preliminary way to gather information about a topic. The method is also useful if researchers are unable to perform an experiment.
Researchers use correlations to see if a relationship between two or more variables exists, but the variables themselves are not under the control of the researchers.
While correlational research can demonstrate a relationship between variables, it cannot prove that changing one variable will change another. In other words, correlational studies cannot prove cause-and-effect relationships.
When you encounter research that refers to a "link" or an "association" between two things, they are most likely talking about a correlational study.
Types of Correlational Research
There are three types of correlational research: naturalistic observation, the survey method, and archival research. Each type has its own purpose, as well as its pros and cons.
Naturalistic Observation
The naturalistic observation method involves observing and recording variables of interest in a natural setting without interference or manipulation.
Can inspire ideas for further research
Option if lab experiment not available
Variables are viewed in natural setting
Can be time-consuming and expensive
Extraneous variables can't be controlled
No scientific control of variables
Subjects might behave differently if aware of being observed
This method is well-suited to studies where researchers want to see how variables behave in their natural setting or state. Inspiration can then be drawn from the observations to inform future avenues of research.
In some cases, it might be the only method available to researchers; for example, if lab experimentation would be precluded by access, resources, or ethics. It might be preferable to not being able to conduct research at all, but the method can be costly and usually takes a lot of time.
Naturalistic observation presents several challenges for researchers. For one, it does not allow them to control or influence the variables in any way nor can they change any possible external variables.
However, this does not mean that researchers will get reliable data from watching the variables, or that the information they gather will be free from bias.
For example, study subjects might act differently if they know that they are being watched. The researchers might not be aware that the behavior that they are observing is not necessarily the subject's natural state (i.e., how they would act if they did not know they were being watched).
Researchers also need to be aware of their biases, which can affect the observation and interpretation of a subject's behavior.
Surveys and questionnaires are some of the most common methods used for psychological research. The survey method involves having a random sample of participants complete a survey, test, or questionnaire related to the variables of interest. Random sampling is vital to the generalizability of a survey's results.
Cheap, easy, and fast
Can collect large amounts of data in a short amount of time
Results can be affected by poor survey questions
Results can be affected by unrepresentative sample
Outcomes can be affected by participants
If researchers need to gather a large amount of data in a short period of time, a survey is likely to be the fastest, easiest, and cheapest option.
It's also a flexible method because it lets researchers create data-gathering tools that will help ensure they get the information they need (survey responses) from all the sources they want to use (a random sample of participants taking the survey).
Survey data might be cost-efficient and easy to get, but it has its downsides. For one, the data is not always reliable—particularly if the survey questions are poorly written or the overall design or delivery is weak. Data is also affected by specific faults, such as unrepresented or underrepresented samples .
The use of surveys relies on participants to provide useful data. Researchers need to be aware of the specific factors related to the people taking the survey that will affect its outcome.
For example, some people might struggle to understand the questions. A person might answer a particular way to try to please the researchers or to try to control how the researchers perceive them (such as trying to make themselves "look better").
Sometimes, respondents might not even realize that their answers are incorrect or misleading because of mistaken memories .
Archival Research
Many areas of psychological research benefit from analyzing studies that were conducted long ago by other researchers, as well as reviewing historical records and case studies.
For example, in an experiment known as "The Irritable Heart ," researchers used digitalized records containing information on American Civil War veterans to learn more about post-traumatic stress disorder (PTSD).
Large amount of data
Can be less expensive
Researchers cannot change participant behavior
Can be unreliable
Information might be missing
No control over data collection methods
Using records, databases, and libraries that are publicly accessible or accessible through their institution can help researchers who might not have a lot of money to support their research efforts.
Free and low-cost resources are available to researchers at all levels through academic institutions, museums, and data repositories around the world.
Another potential benefit is that these sources often provide an enormous amount of data that was collected over a very long period of time, which can give researchers a way to view trends, relationships, and outcomes related to their research.
While the inability to change variables can be a disadvantage of some methods, it can be a benefit of archival research. That said, using historical records or information that was collected a long time ago also presents challenges. For one, important information might be missing or incomplete and some aspects of older studies might not be useful to researchers in a modern context.
A primary issue with archival research is reliability. When reviewing old research, little information might be available about who conducted the research, how a study was designed, who participated in the research, as well as how data was collected and interpreted.
Researchers can also be presented with ethical quandaries—for example, should modern researchers use data from studies that were conducted unethically or with questionable ethics?
You've probably heard the phrase, "correlation does not equal causation." This means that while correlational research can suggest that there is a relationship between two variables, it cannot prove that one variable will change another.
For example, researchers might perform a correlational study that suggests there is a relationship between academic success and a person's self-esteem. However, the study cannot show that academic success changes a person's self-esteem.
To determine why the relationship exists, researchers would need to consider and experiment with other variables, such as the subject's social relationships, cognitive abilities, personality, and socioeconomic status.
The difference between a correlational study and an experimental study involves the manipulation of variables. Researchers do not manipulate variables in a correlational study, but they do control and systematically vary the independent variables in an experimental study. Correlational studies allow researchers to detect the presence and strength of a relationship between variables, while experimental studies allow researchers to look for cause and effect relationships.
If the study involves the systematic manipulation of the levels of a variable, it is an experimental study. If researchers are measuring what is already present without actually changing the variables, then is a correlational study.
The variables in a correlational study are what the researcher measures. Once measured, researchers can then use statistical analysis to determine the existence, strength, and direction of the relationship. However, while correlational studies can say that variable X and variable Y have a relationship, it does not mean that X causes Y.
The goal of correlational research is often to look for relationships, describe these relationships, and then make predictions. Such research can also often serve as a jumping off point for future experimental research.
Heath W. Psychology Research Methods . Cambridge University Press; 2018:134-156.
Schneider FW. Applied Social Psychology . 2nd ed. SAGE; 2012:50-53.
Curtis EA, Comiskey C, Dempsey O. Importance and use of correlational research . Nurse Researcher . 2016;23(6):20-25. doi:10.7748/nr.2016.e1382
Carpenter S. Visualizing Psychology . 3rd ed. John Wiley & Sons; 2012:14-30.
Pizarro J, Silver RC, Prause J. Physical and mental health costs of traumatic war experiences among civil war veterans . Arch Gen Psychiatry . 2006;63(2):193. doi:10.1001/archpsyc.63.2.193
Post SG. The echo of Nuremberg: Nazi data and ethics . J Med Ethics . 1991;17(1):42-44. doi:10.1136/jme.17.1.42
Lau F. Chapter 12 Methods for Correlational Studies . In: Lau F, Kuziemsky C, eds. Handbook of eHealth Evaluation: An Evidence-based Approach . University of Victoria.
Akoglu H. User's guide to correlation coefficients . Turk J Emerg Med . 2018;18(3):91-93. doi:10.1016/j.tjem.2018.08.001
Price PC. Research Methods in Psychology . California State University.
By Kendra Cherry, MSEd Kendra Cherry, MS, is a psychosocial rehabilitation specialist, psychology educator, and author of the "Everything Psychology Book."
6.2 Correlational Research
Learning objectives.
- Define correlational research and give several examples.
- Explain why a researcher might choose to conduct correlational research rather than experimental research or another type of non-experimental research.
- Interpret the strength and direction of different correlation coefficients.
- Explain why correlation does not imply causation.
What Is Correlational Research?
Correlational research is a type of non-experimental research in which the researcher measures two variables and assesses the statistical relationship (i.e., the correlation) between them with little or no effort to control extraneous variables. There are many reasons that researchers interested in statistical relationships between variables would choose to conduct a correlational study rather than an experiment. The first is that they do not believe that the statistical relationship is a causal one or are not interested in causal relationships. Recall two goals of science are to describe and to predict and the correlational research strategy allows researchers to achieve both of these goals. Specifically, this strategy can be used to describe the strength and direction of the relationship between two variables and if there is a relationship between the variables then the researchers can use scores on one variable to predict scores on the other (using a statistical technique called regression).
Another reason that researchers would choose to use a correlational study rather than an experiment is that the statistical relationship of interest is thought to be causal, but the researcher cannot manipulate the independent variable because it is impossible, impractical, or unethical. For example, while I might be interested in the relationship between the frequency people use cannabis and their memory abilities I cannot ethically manipulate the frequency that people use cannabis. As such, I must rely on the correlational research strategy; I must simply measure the frequency that people use cannabis and measure their memory abilities using a standardized test of memory and then determine whether the frequency people use cannabis use is statistically related to memory test performance.
Correlation is also used to establish the reliability and validity of measurements. For example, a researcher might evaluate the validity of a brief extraversion test by administering it to a large group of participants along with a longer extraversion test that has already been shown to be valid. This researcher might then check to see whether participants’ scores on the brief test are strongly correlated with their scores on the longer one. Neither test score is thought to cause the other, so there is no independent variable to manipulate. In fact, the terms independent variable and dependent variabl e do not apply to this kind of research.
Another strength of correlational research is that it is often higher in external validity than experimental research. Recall there is typically a trade-off between internal validity and external validity. As greater controls are added to experiments, internal validity is increased but often at the expense of external validity. In contrast, correlational studies typically have low internal validity because nothing is manipulated or control but they often have high external validity. Since nothing is manipulated or controlled by the experimenter the results are more likely to reflect relationships that exist in the real world.
Finally, extending upon this trade-off between internal and external validity, correlational research can help to provide converging evidence for a theory. If a theory is supported by a true experiment that is high in internal validity as well as by a correlational study that is high in external validity then the researchers can have more confidence in the validity of their theory. As a concrete example, correlational studies establishing that there is a relationship between watching violent television and aggressive behavior have been complemented by experimental studies confirming that the relationship is a causal one (Bushman & Huesmann, 2001) [1] . These converging results provide strong evidence that there is a real relationship (indeed a causal relationship) between watching violent television and aggressive behavior.
Data Collection in Correlational Research
Again, the defining feature of correlational research is that neither variable is manipulated. It does not matter how or where the variables are measured. A researcher could have participants come to a laboratory to complete a computerized backward digit span task and a computerized risky decision-making task and then assess the relationship between participants’ scores on the two tasks. Or a researcher could go to a shopping mall to ask people about their attitudes toward the environment and their shopping habits and then assess the relationship between these two variables. Both of these studies would be correlational because no independent variable is manipulated.
Correlations Between Quantitative Variables
Correlations between quantitative variables are often presented using scatterplots . Figure 6.3 shows some hypothetical data on the relationship between the amount of stress people are under and the number of physical symptoms they have. Each point in the scatterplot represents one person’s score on both variables. For example, the circled point in Figure 6.3 represents a person whose stress score was 10 and who had three physical symptoms. Taking all the points into account, one can see that people under more stress tend to have more physical symptoms. This is a good example of a positive relationship , in which higher scores on one variable tend to be associated with higher scores on the other. A negative relationship is one in which higher scores on one variable tend to be associated with lower scores on the other. There is a negative relationship between stress and immune system functioning, for example, because higher stress is associated with lower immune system functioning.

Figure 6.3 Scatterplot Showing a Hypothetical Positive Relationship Between Stress and Number of Physical Symptoms. The circled point represents a person whose stress score was 10 and who had three physical symptoms. Pearson’s r for these data is +.51.
The strength of a correlation between quantitative variables is typically measured using a statistic called Pearson’s Correlation Coefficient (or Pearson’s r ) . As Figure 6.4 shows, Pearson’s r ranges from −1.00 (the strongest possible negative relationship) to +1.00 (the strongest possible positive relationship). A value of 0 means there is no relationship between the two variables. When Pearson’s r is 0, the points on a scatterplot form a shapeless “cloud.” As its value moves toward −1.00 or +1.00, the points come closer and closer to falling on a single straight line. Correlation coefficients near ±.10 are considered small, values near ± .30 are considered medium, and values near ±.50 are considered large. Notice that the sign of Pearson’s r is unrelated to its strength. Pearson’s r values of +.30 and −.30, for example, are equally strong; it is just that one represents a moderate positive relationship and the other a moderate negative relationship. With the exception of reliability coefficients, most correlations that we find in Psychology are small or moderate in size. The website http://rpsychologist.com/d3/correlation/ , created by Kristoffer Magnusson, provides an excellent interactive visualization of correlations that permits you to adjust the strength and direction of a correlation while witnessing the corresponding changes to the scatterplot.

Figure 6.4 Range of Pearson’s r, From −1.00 (Strongest Possible Negative Relationship), Through 0 (No Relationship), to +1.00 (Strongest Possible Positive Relationship)
There are two common situations in which the value of Pearson’s r can be misleading. Pearson’s r is a good measure only for linear relationships, in which the points are best approximated by a straight line. It is not a good measure for nonlinear relationships, in which the points are better approximated by a curved line. Figure 6.5, for example, shows a hypothetical relationship between the amount of sleep people get per night and their level of depression. In this example, the line that best approximates the points is a curve—a kind of upside-down “U”—because people who get about eight hours of sleep tend to be the least depressed. Those who get too little sleep and those who get too much sleep tend to be more depressed. Even though Figure 6.5 shows a fairly strong relationship between depression and sleep, Pearson’s r would be close to zero because the points in the scatterplot are not well fit by a single straight line. This means that it is important to make a scatterplot and confirm that a relationship is approximately linear before using Pearson’s r . Nonlinear relationships are fairly common in psychology, but measuring their strength is beyond the scope of this book.

Figure 6.5 Hypothetical Nonlinear Relationship Between Sleep and Depression
The other common situations in which the value of Pearson’s r can be misleading is when one or both of the variables have a limited range in the sample relative to the population. This problem is referred to as restriction of range . Assume, for example, that there is a strong negative correlation between people’s age and their enjoyment of hip hop music as shown by the scatterplot in Figure 6.6. Pearson’s r here is −.77. However, if we were to collect data only from 18- to 24-year-olds—represented by the shaded area of Figure 6.6—then the relationship would seem to be quite weak. In fact, Pearson’s r for this restricted range of ages is 0. It is a good idea, therefore, to design studies to avoid restriction of range. For example, if age is one of your primary variables, then you can plan to collect data from people of a wide range of ages. Because restriction of range is not always anticipated or easily avoidable, however, it is good practice to examine your data for possible restriction of range and to interpret Pearson’s r in light of it. (There are also statistical methods to correct Pearson’s r for restriction of range, but they are beyond the scope of this book).

Figure 6.6 Hypothetical Data Showing How a Strong Overall Correlation Can Appear to Be Weak When One Variable Has a Restricted Range.The overall correlation here is −.77, but the correlation for the 18- to 24-year-olds (in the blue box) is 0.
Correlation Does Not Imply Causation
You have probably heard repeatedly that “Correlation does not imply causation.” An amusing example of this comes from a 2012 study that showed a positive correlation (Pearson’s r = 0.79) between the per capita chocolate consumption of a nation and the number of Nobel prizes awarded to citizens of that nation [2] . It seems clear, however, that this does not mean that eating chocolate causes people to win Nobel prizes, and it would not make sense to try to increase the number of Nobel prizes won by recommending that parents feed their children more chocolate.
There are two reasons that correlation does not imply causation. The first is called the directionality problem . Two variables, X and Y , can be statistically related because X causes Y or because Y causes X . Consider, for example, a study showing that whether or not people exercise is statistically related to how happy they are—such that people who exercise are happier on average than people who do not. This statistical relationship is consistent with the idea that exercising causes happiness, but it is also consistent with the idea that happiness causes exercise. Perhaps being happy gives people more energy or leads them to seek opportunities to socialize with others by going to the gym. The second reason that correlation does not imply causation is called the third-variable problem . Two variables, X and Y , can be statistically related not because X causes Y , or because Y causes X , but because some third variable, Z , causes both X and Y . For example, the fact that nations that have won more Nobel prizes tend to have higher chocolate consumption probably reflects geography in that European countries tend to have higher rates of per capita chocolate consumption and invest more in education and technology (once again, per capita) than many other countries in the world. Similarly, the statistical relationship between exercise and happiness could mean that some third variable, such as physical health, causes both of the others. Being physically healthy could cause people to exercise and cause them to be happier. Correlations that are a result of a third-variable are often referred to as spurious correlations.
Some excellent and funny examples of spurious correlations can be found at http://www.tylervigen.com (Figure 6.7 provides one such example).

“Lots of Candy Could Lead to Violence”
Although researchers in psychology know that correlation does not imply causation, many journalists do not. One website about correlation and causation, http://jonathan.mueller.faculty.noctrl.edu/100/correlation_or_causation.htm , links to dozens of media reports about real biomedical and psychological research. Many of the headlines suggest that a causal relationship has been demonstrated when a careful reading of the articles shows that it has not because of the directionality and third-variable problems.
One such article is about a study showing that children who ate candy every day were more likely than other children to be arrested for a violent offense later in life. But could candy really “lead to” violence, as the headline suggests? What alternative explanations can you think of for this statistical relationship? How could the headline be rewritten so that it is not misleading?
As you have learned by reading this book, there are various ways that researchers address the directionality and third-variable problems. The most effective is to conduct an experiment. For example, instead of simply measuring how much people exercise, a researcher could bring people into a laboratory and randomly assign half of them to run on a treadmill for 15 minutes and the rest to sit on a couch for 15 minutes. Although this seems like a minor change to the research design, it is extremely important. Now if the exercisers end up in more positive moods than those who did not exercise, it cannot be because their moods affected how much they exercised (because it was the researcher who determined how much they exercised). Likewise, it cannot be because some third variable (e.g., physical health) affected both how much they exercised and what mood they were in (because, again, it was the researcher who determined how much they exercised). Thus experiments eliminate the directionality and third-variable problems and allow researchers to draw firm conclusions about causal relationships.
Key Takeaways
- Correlational research involves measuring two variables and assessing the relationship between them, with no manipulation of an independent variable.
- Correlation does not imply causation. A statistical relationship between two variables, X and Y , does not necessarily mean that X causes Y . It is also possible that Y causes X , or that a third variable, Z , causes both X and Y .
- While correlational research cannot be used to establish causal relationships between variables, correlational research does allow researchers to achieve many other important objectives (establishing reliability and validity, providing converging evidence, describing relationships and making predictions)
- Correlation coefficients can range from -1 to +1. The sign indicates the direction of the relationship between the variables and the numerical value indicates the strength of the relationship.
- A cognitive psychologist compares the ability of people to recall words that they were instructed to “read” with their ability to recall words that they were instructed to “imagine.”
- A manager studies the correlation between new employees’ college grade point averages and their first-year performance reports.
- An automotive engineer installs different stick shifts in a new car prototype, each time asking several people to rate how comfortable the stick shift feels.
- A food scientist studies the relationship between the temperature inside people’s refrigerators and the amount of bacteria on their food.
- A social psychologist tells some research participants that they need to hurry over to the next building to complete a study. She tells others that they can take their time. Then she observes whether they stop to help a research assistant who is pretending to be hurt.
2. Practice: For each of the following statistical relationships, decide whether the directionality problem is present and think of at least one plausible third variable.
- People who eat more lobster tend to live longer.
- People who exercise more tend to weigh less.
- College students who drink more alcohol tend to have poorer grades.
- Bushman, B. J., & Huesmann, L. R. (2001). Effects of televised violence on aggression. In D. Singer & J. Singer (Eds.), Handbook of children and the media (pp. 223–254). Thousand Oaks, CA: Sage. ↵
- Messerli, F. H. (2012). Chocolate consumption, cognitive function, and Nobel laureates. New England Journal of Medicine, 367 , 1562-1564. ↵

Share This Book
- Increase Font Size
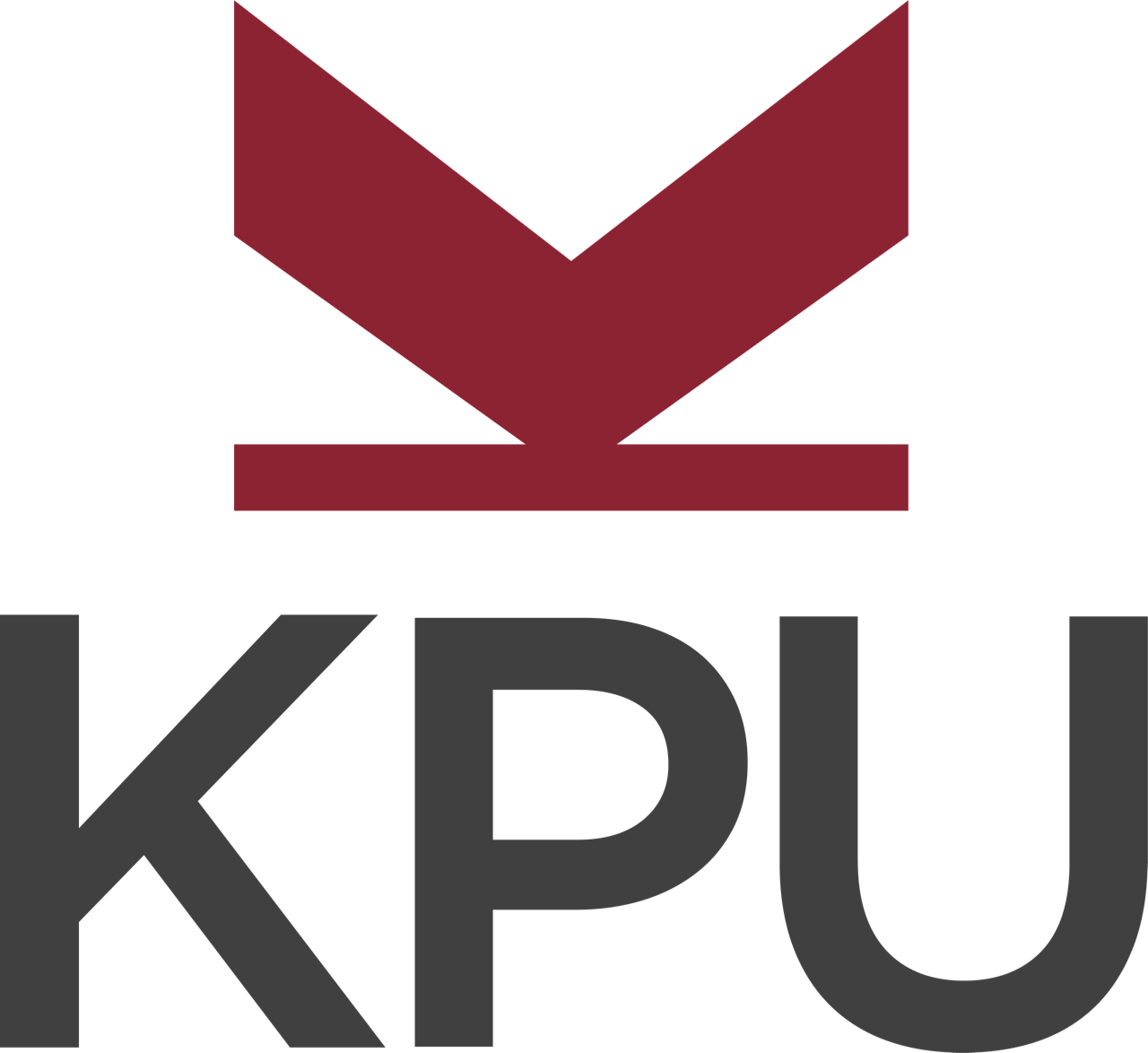
Want to create or adapt books like this? Learn more about how Pressbooks supports open publishing practices.
Non-Experimental Research
29 Correlational Research
Learning objectives.
- Define correlational research and give several examples.
- Explain why a researcher might choose to conduct correlational research rather than experimental research or another type of non-experimental research.
- Interpret the strength and direction of different correlation coefficients.
- Explain why correlation does not imply causation.
What Is Correlational Research?
Correlational research is a type of non-experimental research in which the researcher measures two variables (binary or continuous) and assesses the statistical relationship (i.e., the correlation) between them with little or no effort to control extraneous variables. There are many reasons that researchers interested in statistical relationships between variables would choose to conduct a correlational study rather than an experiment. The first is that they do not believe that the statistical relationship is a causal one or are not interested in causal relationships. Recall two goals of science are to describe and to predict and the correlational research strategy allows researchers to achieve both of these goals. Specifically, this strategy can be used to describe the strength and direction of the relationship between two variables and if there is a relationship between the variables then the researchers can use scores on one variable to predict scores on the other (using a statistical technique called regression, which is discussed further in the section on Complex Correlation in this chapter).
Another reason that researchers would choose to use a correlational study rather than an experiment is that the statistical relationship of interest is thought to be causal, but the researcher cannot manipulate the independent variable because it is impossible, impractical, or unethical. For example, while a researcher might be interested in the relationship between the frequency people use cannabis and their memory abilities they cannot ethically manipulate the frequency that people use cannabis. As such, they must rely on the correlational research strategy; they must simply measure the frequency that people use cannabis and measure their memory abilities using a standardized test of memory and then determine whether the frequency people use cannabis is statistically related to memory test performance.
Correlation is also used to establish the reliability and validity of measurements. For example, a researcher might evaluate the validity of a brief extraversion test by administering it to a large group of participants along with a longer extraversion test that has already been shown to be valid. This researcher might then check to see whether participants’ scores on the brief test are strongly correlated with their scores on the longer one. Neither test score is thought to cause the other, so there is no independent variable to manipulate. In fact, the terms independent variable and dependent variabl e do not apply to this kind of research.
Another strength of correlational research is that it is often higher in external validity than experimental research. Recall there is typically a trade-off between internal validity and external validity. As greater controls are added to experiments, internal validity is increased but often at the expense of external validity as artificial conditions are introduced that do not exist in reality. In contrast, correlational studies typically have low internal validity because nothing is manipulated or controlled but they often have high external validity. Since nothing is manipulated or controlled by the experimenter the results are more likely to reflect relationships that exist in the real world.
Finally, extending upon this trade-off between internal and external validity, correlational research can help to provide converging evidence for a theory. If a theory is supported by a true experiment that is high in internal validity as well as by a correlational study that is high in external validity then the researchers can have more confidence in the validity of their theory. As a concrete example, correlational studies establishing that there is a relationship between watching violent television and aggressive behavior have been complemented by experimental studies confirming that the relationship is a causal one (Bushman & Huesmann, 2001) [1] .
Does Correlational Research Always Involve Quantitative Variables?
A common misconception among beginning researchers is that correlational research must involve two quantitative variables, such as scores on two extraversion tests or the number of daily hassles and number of symptoms people have experienced. However, the defining feature of correlational research is that the two variables are measured—neither one is manipulated—and this is true regardless of whether the variables are quantitative or categorical. Imagine, for example, that a researcher administers the Rosenberg Self-Esteem Scale to 50 American college students and 50 Japanese college students. Although this “feels” like a between-subjects experiment, it is a correlational study because the researcher did not manipulate the students’ nationalities. The same is true of the study by Cacioppo and Petty comparing college faculty and factory workers in terms of their need for cognition. It is a correlational study because the researchers did not manipulate the participants’ occupations.
Figure 6.2 shows data from a hypothetical study on the relationship between whether people make a daily list of things to do (a “to-do list”) and stress. Notice that it is unclear whether this is an experiment or a correlational study because it is unclear whether the independent variable was manipulated. If the researcher randomly assigned some participants to make daily to-do lists and others not to, then it is an experiment. If the researcher simply asked participants whether they made daily to-do lists, then it is a correlational study. The distinction is important because if the study was an experiment, then it could be concluded that making the daily to-do lists reduced participants’ stress. But if it was a correlational study, it could only be concluded that these variables are statistically related. Perhaps being stressed has a negative effect on people’s ability to plan ahead (the directionality problem). Or perhaps people who are more conscientious are more likely to make to-do lists and less likely to be stressed (the third-variable problem). The crucial point is that what defines a study as experimental or correlational is not the variables being studied, nor whether the variables are quantitative or categorical, nor the type of graph or statistics used to analyze the data. What defines a study is how the study is conducted.
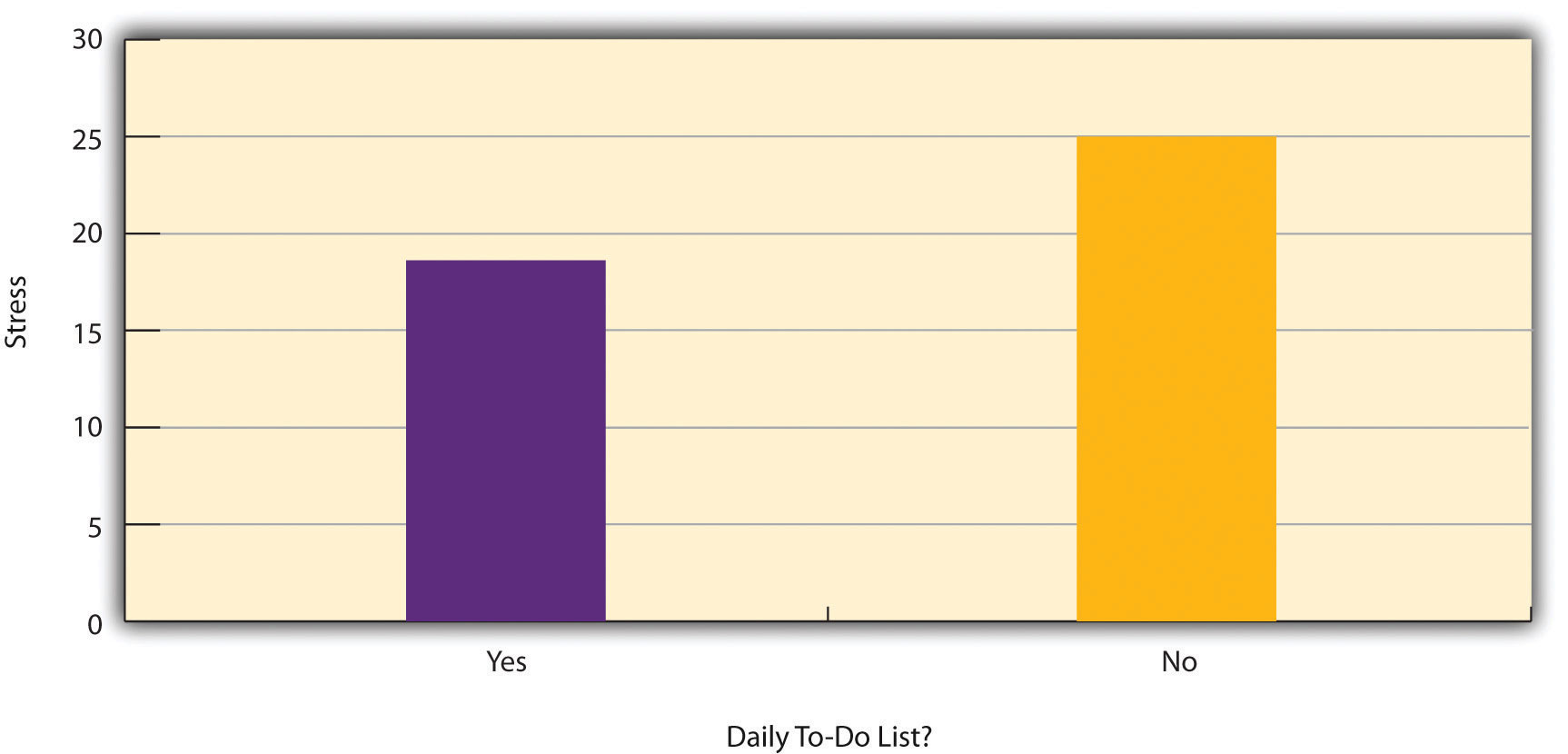
Data Collection in Correlational Research
Again, the defining feature of correlational research is that neither variable is manipulated. It does not matter how or where the variables are measured. A researcher could have participants come to a laboratory to complete a computerized backward digit span task and a computerized risky decision-making task and then assess the relationship between participants’ scores on the two tasks. Or a researcher could go to a shopping mall to ask people about their attitudes toward the environment and their shopping habits and then assess the relationship between these two variables. Both of these studies would be correlational because no independent variable is manipulated.
Correlations Between Quantitative Variables
Correlations between quantitative variables are often presented using scatterplots . Figure 6.3 shows some hypothetical data on the relationship between the amount of stress people are under and the number of physical symptoms they have. Each point in the scatterplot represents one person’s score on both variables. For example, the circled point in Figure 6.3 represents a person whose stress score was 10 and who had three physical symptoms. Taking all the points into account, one can see that people under more stress tend to have more physical symptoms. This is a good example of a positive relationship , in which higher scores on one variable tend to be associated with higher scores on the other. In other words, they move in the same direction, either both up or both down. A negative relationship is one in which higher scores on one variable tend to be associated with lower scores on the other. In other words, they move in opposite directions. There is a negative relationship between stress and immune system functioning, for example, because higher stress is associated with lower immune system functioning.
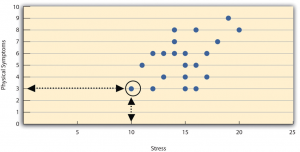
The strength of a correlation between quantitative variables is typically measured using a statistic called Pearson’s Correlation Coefficient (or Pearson's r ) . As Figure 6.4 shows, Pearson’s r ranges from −1.00 (the strongest possible negative relationship) to +1.00 (the strongest possible positive relationship). A value of 0 means there is no relationship between the two variables. When Pearson’s r is 0, the points on a scatterplot form a shapeless “cloud.” As its value moves toward −1.00 or +1.00, the points come closer and closer to falling on a single straight line. Correlation coefficients near ±.10 are considered small, values near ± .30 are considered medium, and values near ±.50 are considered large. Notice that the sign of Pearson’s r is unrelated to its strength. Pearson’s r values of +.30 and −.30, for example, are equally strong; it is just that one represents a moderate positive relationship and the other a moderate negative relationship. With the exception of reliability coefficients, most correlations that we find in Psychology are small or moderate in size. The website http://rpsychologist.com/d3/correlation/ , created by Kristoffer Magnusson, provides an excellent interactive visualization of correlations that permits you to adjust the strength and direction of a correlation while witnessing the corresponding changes to the scatterplot.
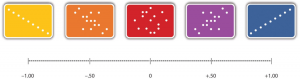
There are two common situations in which the value of Pearson’s r can be misleading. Pearson’s r is a good measure only for linear relationships, in which the points are best approximated by a straight line. It is not a good measure for nonlinear relationships, in which the points are better approximated by a curved line. Figure 6.5, for example, shows a hypothetical relationship between the amount of sleep people get per night and their level of depression. In this example, the line that best approximates the points is a curve—a kind of upside-down “U”—because people who get about eight hours of sleep tend to be the least depressed. Those who get too little sleep and those who get too much sleep tend to be more depressed. Even though Figure 6.5 shows a fairly strong relationship between depression and sleep, Pearson’s r would be close to zero because the points in the scatterplot are not well fit by a single straight line. This means that it is important to make a scatterplot and confirm that a relationship is approximately linear before using Pearson’s r . Nonlinear relationships are fairly common in psychology, but measuring their strength is beyond the scope of this book.
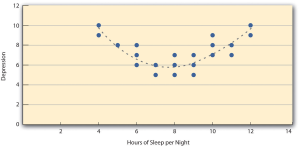
The other common situations in which the value of Pearson’s r can be misleading is when one or both of the variables have a limited range in the sample relative to the population. This problem is referred to as restriction of range . Assume, for example, that there is a strong negative correlation between people’s age and their enjoyment of hip hop music as shown by the scatterplot in Figure 6.6. Pearson’s r here is −.77. However, if we were to collect data only from 18- to 24-year-olds—represented by the shaded area of Figure 6.6—then the relationship would seem to be quite weak. In fact, Pearson’s r for this restricted range of ages is 0. It is a good idea, therefore, to design studies to avoid restriction of range. For example, if age is one of your primary variables, then you can plan to collect data from people of a wide range of ages. Because restriction of range is not always anticipated or easily avoidable, however, it is good practice to examine your data for possible restriction of range and to interpret Pearson’s r in light of it. (There are also statistical methods to correct Pearson’s r for restriction of range, but they are beyond the scope of this book).
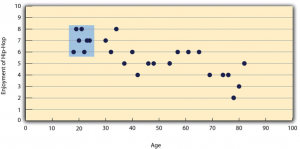
Correlation Does Not Imply Causation
You have probably heard repeatedly that “Correlation does not imply causation.” An amusing example of this comes from a 2012 study that showed a positive correlation (Pearson’s r = 0.79) between the per capita chocolate consumption of a nation and the number of Nobel prizes awarded to citizens of that nation [2] . It seems clear, however, that this does not mean that eating chocolate causes people to win Nobel prizes, and it would not make sense to try to increase the number of Nobel prizes won by recommending that parents feed their children more chocolate.
There are two reasons that correlation does not imply causation. The first is called the directionality problem . Two variables, X and Y , can be statistically related because X causes Y or because Y causes X . Consider, for example, a study showing that whether or not people exercise is statistically related to how happy they are—such that people who exercise are happier on average than people who do not. This statistical relationship is consistent with the idea that exercising causes happiness, but it is also consistent with the idea that happiness causes exercise. Perhaps being happy gives people more energy or leads them to seek opportunities to socialize with others by going to the gym. The second reason that correlation does not imply causation is called the third-variable problem . Two variables, X and Y , can be statistically related not because X causes Y , or because Y causes X , but because some third variable, Z , causes both X and Y . For example, the fact that nations that have won more Nobel prizes tend to have higher chocolate consumption probably reflects geography in that European countries tend to have higher rates of per capita chocolate consumption and invest more in education and technology (once again, per capita) than many other countries in the world. Similarly, the statistical relationship between exercise and happiness could mean that some third variable, such as physical health, causes both of the others. Being physically healthy could cause people to exercise and cause them to be happier. Correlations that are a result of a third-variable are often referred to as spurious correlations .
Some excellent and amusing examples of spurious correlations can be found at http://www.tylervigen.com (Figure 6.7 provides one such example).
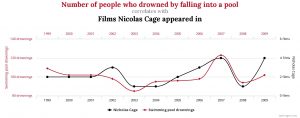
“Lots of Candy Could Lead to Violence”
Although researchers in psychology know that correlation does not imply causation, many journalists do not. One website about correlation and causation, http://jonathan.mueller.faculty.noctrl.edu/100/correlation_or_causation.htm , links to dozens of media reports about real biomedical and psychological research. Many of the headlines suggest that a causal relationship has been demonstrated when a careful reading of the articles shows that it has not because of the directionality and third-variable problems.
One such article is about a study showing that children who ate candy every day were more likely than other children to be arrested for a violent offense later in life. But could candy really “lead to” violence, as the headline suggests? What alternative explanations can you think of for this statistical relationship? How could the headline be rewritten so that it is not misleading?
As you have learned by reading this book, there are various ways that researchers address the directionality and third-variable problems. The most effective is to conduct an experiment. For example, instead of simply measuring how much people exercise, a researcher could bring people into a laboratory and randomly assign half of them to run on a treadmill for 15 minutes and the rest to sit on a couch for 15 minutes. Although this seems like a minor change to the research design, it is extremely important. Now if the exercisers end up in more positive moods than those who did not exercise, it cannot be because their moods affected how much they exercised (because it was the researcher who used random assignment to determine how much they exercised). Likewise, it cannot be because some third variable (e.g., physical health) affected both how much they exercised and what mood they were in. Thus experiments eliminate the directionality and third-variable problems and allow researchers to draw firm conclusions about causal relationships.
Media Attributions
- Nicholas Cage and Pool Drownings © Tyler Viegen is licensed under a CC BY (Attribution) license
- Bushman, B. J., & Huesmann, L. R. (2001). Effects of televised violence on aggression. In D. Singer & J. Singer (Eds.), Handbook of children and the media (pp. 223–254). Thousand Oaks, CA: Sage. ↵
- Messerli, F. H. (2012). Chocolate consumption, cognitive function, and Nobel laureates. New England Journal of Medicine, 367 , 1562-1564. ↵
A graph that presents correlations between two quantitative variables, one on the x-axis and one on the y-axis. Scores are plotted at the intersection of the values on each axis.
A relationship in which higher scores on one variable tend to be associated with higher scores on the other.
A relationship in which higher scores on one variable tend to be associated with lower scores on the other.
A statistic that measures the strength of a correlation between quantitative variables.
When one or both variables have a limited range in the sample relative to the population, making the value of the correlation coefficient misleading.
The problem where two variables, X and Y , are statistically related either because X causes Y, or because Y causes X , and thus the causal direction of the effect cannot be known.
Two variables, X and Y, can be statistically related not because X causes Y, or because Y causes X, but because some third variable, Z, causes both X and Y.
Correlations that are a result not of the two variables being measured, but rather because of a third, unmeasured, variable that affects both of the measured variables.
Research Methods in Psychology Copyright © 2019 by Rajiv S. Jhangiani, I-Chant A. Chiang, Carrie Cuttler, & Dana C. Leighton is licensed under a Creative Commons Attribution-NonCommercial-ShareAlike 4.0 International License , except where otherwise noted.
Share This Book
- Correlational Research Designs: Types, Examples & Methods

A human mind is a powerful tool that allows you to sift through seemingly unrelated variables and establish a connection with regards to a specific subject at hand. This skill is what comes to play when we talk about correlational research.
Correlational research is something that we do every day; think about how you establish a connection between the doorbell ringing at a particular time and the milkman’s arrival. As such, it is expedient to understand the different types of correlational research that are available and more importantly, how to go about it.
What is Correlational Research?
Correlational research is a type of research method that involves observing two variables in order to establish a statistically corresponding relationship between them. The aim of correlational research is to identify variables that have some sort of relationship do the extent that a change in one creates some change in the other.
This type of research is descriptive, unlike experimental research that relies entirely on scientific methodology and hypothesis. For example, correlational research may reveal the statistical relationship between high-income earners and relocation; that is, the more people earn, the more likely they are to relocate or not.
What are the Types of Correlational Research?
Essentially, there are 3 types of correlational research which are positive correlational research, negative correlational research, and no correlational research. Each of these types is defined by peculiar characteristics.
- Positive Correlational Research
Positive correlational research is a research method involving 2 variables that are statistically corresponding where an increase or decrease in 1 variable creates a like change in the other. An example is when an increase in workers’ remuneration results in an increase in the prices of goods and services and vice versa.
- Negative Correlational Research
Negative correlational research is a research method involving 2 variables that are statistically opposite where an increase in one of the variables creates an alternate effect or decrease in the other variable. An example of a negative correlation is if the rise in goods and services causes a decrease in demand and vice versa.
- Zero Correlational Research
Zero correlational research is a type of correlational research that involves 2 variables that are not necessarily statistically connected. In this case, a change in one of the variables may not trigger a corresponding or alternate change in the other variable.
Zero correlational research caters for variables with vague statistical relationships. For example, wealth and patience can be variables under zero correlational research because they are statistically independent.
Sporadic change patterns that occur in variables with zero correlational are usually by chance and not as a result of corresponding or alternate mutual inclusiveness.
Correlational research can also be classified based on data collection methods. Based on these, there are 3 types of correlational research: Naturalistic observation research, survey research and archival research.
What are the Data Collection Methods in Correlational research?
Data collection methods in correlational research are the research methodologies adopted by persons carrying out correlational research in order to determine the linear statistical relationship between 2 variables. These data collection methods are used to gather information in correlational research.
The 3 methods of data collection in correlational research are naturalistic observation method, archival data method, and the survey method. All of these would be clearly explained in the subsequent paragraphs.
- Naturalistic Observation
Naturalistic observation is a correlational research methodology that involves observing people’s behaviors as shown in the natural environment where they exist, over a period of time. It is a type of research-field method that involves the researcher paying closing attention to natural behavior patterns of the subjects under consideration.
This method is extremely demanding as the researcher must take extra care to ensure that the subjects do not suspect that they are being observed else they deviate from their natural behavior patterns. It is best for all subjects under observation to remain anonymous in order to avoid a breach of privacy.
The major advantages of the naturalistic observation method are that it allows the researcher to fully observe the subjects (variables) in their natural state. However, it is a very expensive and time-consuming process plus the subjects can become aware of this act at any time and may act contrary.
- Archival Data
Archival data is a type of correlational research method that involves making use of already gathered information about the variables in correlational research. Since this method involves using data that is already gathered and analyzed, it is usually straight to the point.
For this method of correlational research, the research makes use of earlier studies conducted by other researchers or the historical records of the variables being analyzed. This method helps a researcher to track already determined statistical patterns of the variables or subjects.
This method is less expensive, saves time and provides the researcher with more disposable data to work with. However, it has the problem of data accuracy as important information may be missing from previous research since the researcher has no control over the data collection process.
- Survey Method
The survey method is the most common method of correlational research; especially in fields like psychology. It involves random sampling of the variables or the subjects in the research in which the participants fill a questionnaire centered on the subjects of interest.
This method is very flexible as researchers can gather large amounts of data in very little time. However, it is subject to survey response bias and can also be affected by biased survey questions or under-representation of survey respondents or participants.
These would be properly explained under data collection methods in correlational research.
Examples of Correlational Research
Correlational research examples are numerous and highlight several instances where a correlational study may be carried out in order to determine the statistical behavioral trend with regards to the variables under consideration. Here are 3 case examples of correlational research.
- You want to know if wealthy people are less likely to be patient. From your experience, you believe that wealthy people are impatient. However, you want to establish a statistical pattern that proves or disproves your belief. In this case, you can carry out correlational research to identify a trend that links both variables.
- You want to know if there’s a correlation between how much people earn and the number of children that they have. You do not believe that people with more spending power have more children than people with less spending power.
You think that how much people earn hardly determines the number of children that they have. Yet, carrying out correlational research on both variables could reveal any correlational relationship that exists between them.
- You believe that domestic violence causes a brain hemorrhage. You cannot carry out an experiment as it would be unethical to deliberately subject people to domestic violence.
However, you can carry out correlational research to find out if victims of domestic violence suffer brain hemorrhage more than non-victims.
What are the Characteristics of Correlational Research?
- Correlational Research is non-experimental
Correlational research is non-experimental as it does not involve manipulating variables using a scientific methodology in order to agree or disagree with a hypothesis. In correlational research, the researcher simply observes and measures the natural relationship between 2 variables; without subjecting either of the variables to external conditioning.
- Correlational Research is Backward-looking
Correlational research doesn’t take the future into consideration as it only observes and measures the recent historical relationship that exists between 2 variables. In this sense, the statistical pattern resulting from correlational research is backward-looking and can seize to exist at any point, going forward.
Correlational research observes and measures historical patterns between 2 variables such as the relationship between high-income earners and tax payment. Correlational research may reveal a positive relationship between the aforementioned variables but this may change at any point in the future.
- Correlational Research is Dynamic
Statistical patterns between 2 variables that result from correlational research are ever-changing. The correlation between 2 variables changes on a daily basis and such, it cannot be used as a fixed data for further research.
For example, the 2 variables can have a negative correlational relationship for a period of time, maybe 5 years. After this time, the correlational relationship between them can become positive; as observed in the relationship between bonds and stocks.
- Data resulting from correlational research are not constant and cannot be used as a standard variable for further research.
What is the Correlation Coefficient?
A correlation coefficient is an important value in correlational research that indicates whether the inter-relationship between 2 variables is positive, negative or non-existent. It is usually represented with the sign [r] and is part of a range of possible correlation coefficients from -1.0 to +1.0.
The strength of a correlation between quantitative variables is typically measured using a statistic called Pearson’s Correlation Coefficient (or Pearson’s r) . A positive correlation is indicated by a value of 1.0, a perfect negative correlation is indicated by a value of -1.0 while zero correlation is indicated by a value of 0.0.
It is important to note that a correlation coefficient only reflects the linear relationship between 2 variables; it does not capture non-linear relationships and cannot separate dependent and independent variables. The correlation coefficient helps you to determine the degree of statistical relationship that exists between variables.
What are the Advantages of Correlational Research?
- In cases where carrying out experimental research is unethical, correlational research can be used to determine the relationship between 2 variables. For example, when studying humans, carrying out an experiment can be seen as unsafe or unethical; hence, choosing correlational research would be the best option.
- Through correlational research, you can easily determine the statistical relationship between 2 variables.
- Carrying out correlational research is less time-consuming and less expensive than experimental research. This becomes a strong advantage when working with a minimum of researchers and funding or when keeping the number of variables in a study very low.
- Correlational research allows the researcher to carry out shallow data gathering using different methods such as a short survey. A short survey does not require the researcher to personally administer it so this allows the researcher to work with a few people.
What are the Disadvantages of Correlational Research?
- Correlational research is limiting in nature as it can only be used to determine the statistical relationship between 2 variables. It cannot be used to establish a relationship between more than 2 variables.
- It does not account for cause and effect between 2 variables as it doesn’t highlight which of the 2 variables is responsible for the statistical pattern that is observed. For example, finding that education correlates positively with vegetarianism doesn’t explain whether being educated leads to becoming a vegetarian or whether vegetarianism leads to more education.
- Reasons for either can be assumed, but until more research is done, causation can’t be determined. Also, a third, unknown variable might be causing both. For instance, living in the state of Detroit can lead to both education and vegetarianism.
- Correlational research depends on past statistical patterns to determine the relationship between variables. As such, its data cannot be fully depended on for further research.
- In correlational research, the researcher has no control over the variables. Unlike experimental research, correlational research only allows the researcher to observe the variables for connecting statistical patterns without introducing a catalyst.
- The information received from correlational research is limited. Correlational research only shows the relationship between variables and does not equate to causation.
What are the Differences between Correlational and Experimental Research?
- Methodology
The major difference between correlational research and experimental research is methodology. In correlational research, the researcher looks for a statistical pattern linking 2 naturally-occurring variables while in experimental research, the researcher introduces a catalyst and monitors its effects on the variables.
- Observation
In correlational research, the researcher passively observes the phenomena and measures whatever relationship that occurs between them. However, in experimental research, the researcher actively observes phenomena after triggering a change in the behavior of the variables.
In experimental research, the researcher introduces a catalyst and monitors its effects on the variables, that is, cause and effect. In correlational research, the researcher is not interested in cause and effect as it applies; rather, he or she identifies recurring statistical patterns connecting the variables in research.
- Number of Variables
research caters to an unlimited number of variables. Correlational research, on the other hand, caters to only 2 variables.
- Experimental research is causative while correlational research is relational.
- Correlational research is preliminary and almost always precedes experimental research.
- Unlike correlational research, experimental research allows the researcher to control the variables.
How to Use Online Forms for Correlational Research
One of the most popular methods of conducting correlational research is by carrying out a survey which can be made easier with the use of an online form. Surveys for correlational research involve generating different questions that revolve around the variables under observation and, allowing respondents to provide answers to these questions.
Using an online form for your correlational research survey would help the researcher to gather more data in minimum time. In addition, the researcher would be able to reach out to more survey respondents than is plausible with printed correlational research survey forms .
In addition, the researcher would be able to swiftly process and analyze all responses in order to objectively establish the statistical pattern that links the variables in the research. Using an online form for correlational research also helps the researcher to minimize the cost incurred during the research period.
To use an online form for a correlational research survey, you would need to sign up on a data-gathering platform like Formplus . Formplus allows you to create custom forms for correlational research surveys using the Formplus builder.
You can customize your correlational research survey form by adding background images, new color themes or your company logo to make it appear even more professional. In addition, Formplus also has a survey form template that you can edit for a correlational research study.
You can create different types of survey questions including open-ended questions , rating questions, close-ended questions and multiple answers questions in your survey in the Formplus builder. After creating your correlational research survey, you can share the personalized link with respondents via email or social media.
Formplus also enables you to collect offline responses in your form.
Conclusion
Correlational research enables researchers to establish the statistical pattern between 2 seemingly interconnected variables; as such, it is the starting point of any type of research. It allows you to link 2 variables by observing their behaviors in the most natural state.
Unlike experimental research, correlational research does not emphasize the causative factor affecting 2 variables and this makes the data that results from correlational research subject to constant change. However, it is quicker, easier, less expensive and more convenient than experimental research.
It is important to always keep the aim of your research at the back of your mind when choosing the best type of research to adopt. If you simply need to observe how the variables react to change then, experimental research is the best type to subscribe for.
It is best to conduct correlational research using an online correlational research survey form as this makes the data-gathering process, more convenient. Formplus is a great online data-gathering platform that you can use to create custom survey forms for correlational research.
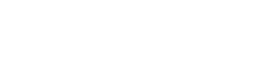
Connect to Formplus, Get Started Now - It's Free!
- characteristics of correlational research
- types of correlational research
- what is correlational research
- busayo.longe
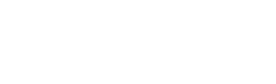
You may also like:
What is Pure or Basic Research? + [Examples & Method]
Simple guide on pure or basic research, its methods, characteristics, advantages, and examples in science, medicine, education and psychology

Recall Bias: Definition, Types, Examples & Mitigation
This article will discuss the impact of recall bias in studies and the best ways to avoid them during research.
Extrapolation in Statistical Research: Definition, Examples, Types, Applications
In this article we’ll look at the different types and characteristics of extrapolation, plus how it contrasts to interpolation.
Exploratory Research: What are its Method & Examples?
Overview on exploratory research, examples and methodology. Shows guides on how to conduct exploratory research with online surveys
Formplus - For Seamless Data Collection
Collect data the right way with a versatile data collection tool. try formplus and transform your work productivity today..

How to Report Pearson’s r in APA Format (With Examples)
A Pearson Correlation Coefficient , often denoted r , measures the linear association between two variables.
It always takes on a value between -1 and 1 where:
- -1 indicates a perfectly negative linear correlation between two variables
- 0 indicates no linear correlation between two variables
- 1 indicates a perfectly positive linear correlation between two variables
We use the following general structure to report a Pearson’s r in APA format:
A Pearson correlation coefficient was computed to assess the linear relationship between [variable 1] and [variable 2] . There was a [negative or positive] correlation between the two variables, r( df ) = [r value] , p = [p-value] .
Keep in mind the following when reporting Pearson’s r in APA format:
- Round the p-value to three decimal places.
- Round the value for r to two decimal places.
- Drop the leading 0 for the p-value and r (e.g. use .77, not 0.77)
- The degrees of freedom (df) is calculated as N – 2.
The following examples show how to report Pearson’s r in APA format in various scenarios.
Example 1: Hours Studied vs. Exam Score Received
A professor collected data for the number of hours studied and the exam score received for 40 students in his class. He found the Pearson correlation coefficient between the two variables to be 0.48 with a corresponding p-value of 0.002.
Here is how to report Pearson’s r in APA format:
A Pearson correlation coefficient was computed to assess the linear relationship between hours studied and exam score. There was a positive correlation between the two variables, r(38) = .48, p = .002.
Example 2: Time Spent Running vs. Body Fat
A doctor collected data for the number of hours spent running per week and body fat percentage for 35 patients. He found the Pearson correlation coefficient between the two variables to be -0.37 with a corresponding p-value of 0.029.
A Pearson correlation coefficient was computed to assess the linear relationship between hours spent running and body fat percentage. There was a negative correlation between the two variables, r(33) = -.37, p = .029.
Example 3: Ad Spend vs. Revenue Generated
A company collected data for the amount of money spent on advertising and the total revenue generated during 15 consecutive sales periods. They found the Pearson correlation coefficient between the two variables to be 0.71 with a corresponding p-value of 0.003.
A Pearson correlation coefficient was computed to assess the linear relationship between advertising spend and total revenue. There was a positive correlation between the two variables, r(13) = .71, p = .003.
Additional Resources
The following tutorials explain how to report other statistical tests and procedures in APA format:
How to Report Cronbach’s Alpha (With Examples) How to Report t-Test Results (With Examples) How to Report Regression Results (With Examples) How to Report ANOVA Results (With Examples)
Featured Posts

Hey there. My name is Zach Bobbitt. I have a Masters of Science degree in Applied Statistics and I’ve worked on machine learning algorithms for professional businesses in both healthcare and retail. I’m passionate about statistics, machine learning, and data visualization and I created Statology to be a resource for both students and teachers alike. My goal with this site is to help you learn statistics through using simple terms, plenty of real-world examples, and helpful illustrations.
3 Replies to “How to Report Pearson’s r in APA Format (With Examples)”
Your effort here helped remove statistical anxiety for me and my students. Thanks a lot.
what about a p-value that is not significant?
Thank you for writing an interpretation with examples.
Leave a Reply Cancel reply
Your email address will not be published. Required fields are marked *
Join the Statology Community
Sign up to receive Statology's exclusive study resource: 100 practice problems with step-by-step solutions. Plus, get our latest insights, tutorials, and data analysis tips straight to your inbox!
By subscribing you accept Statology's Privacy Policy.
Our websites may use cookies to personalize and enhance your experience. By continuing without changing your cookie settings, you agree to this collection. For more information, please see our University Websites Privacy Notice .
Neag School of Education
Educational Research Basics by Del Siegle
Introduction to correlation research.
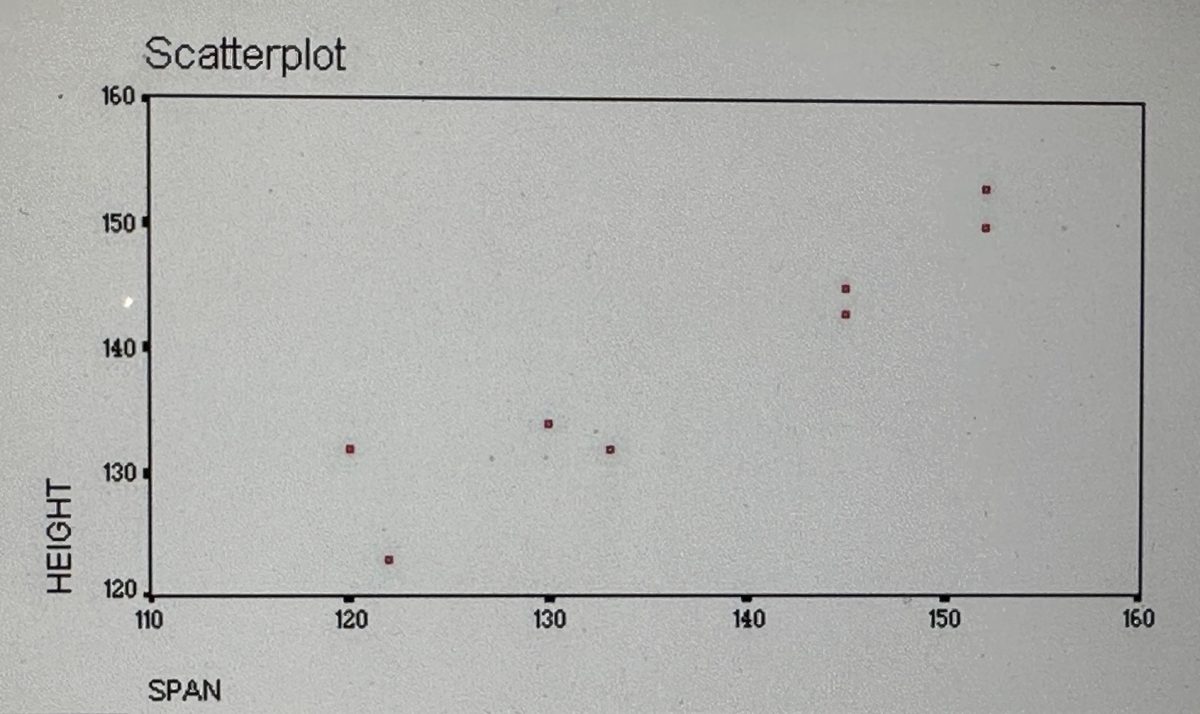
The PowerPoint presentation contains important information for this unit on correlations. Contact the instructor, [email protected] …if you have trouble viewing it.
Some content on this website may require the use of a plug-in, such as Microsoft PowerPoint .
When are correlation methods used?
- They are used to determine the extent to which two or more variables are related among a single group of people (although sometimes each pair of score does not come from one person…the correlation between father’s and son’s height would not).
- There is no attempt to manipulate the variables (random variables)
How is correlational research different from experimental research? In correlational research we do not (or at least try not to) influence any variables but only measure them and look for relations (correlations) between some set of variables, such as blood pressure and cholesterol level. In experimental research, we manipulate some variables and then measure the effects of this manipulation on other variables; for example, a researcher might artificially increase blood pressure and then record cholesterol level. Data analysis in experimental research also comes down to calculating “correlations” between variables, specifically, those manipulated and those affected by the manipulation. However, experimental data may potentially provide qualitatively better information: Only experimental data can conclusively demonstrate causal relations between variables. For example, if we found that whenever we change variable A then variable B changes, then we can conclude that “A influences B.” Data from correlational research can only be “interpreted” in causal terms based on some theories that we have, but correlational data cannot conclusively prove causality. Source: http://www.statsoft.com/textbook/stathome.html
Although a relationship between two variables does not prove that one caused the other, if there is no relationship between two variables then one cannot have caused the other.
Correlation research asks the question: What relationship exists?
- A correlation has direction and can be either positive or negative (note exceptions listed later). With a positive correlation, individuals who score above (or below) the average (mean) on one measure tend to score similarly above (or below) the average on the other measure. The scatterplot of a positive correlation rises (from left to right). With negative relationships, an individual who scores above average on one measure tends to score below average on the other (or vise verse). The scatterplot of a negative correlation falls (from left to right).
- A correlation can differ in the degree or strength of the relationship (with the Pearson product-moment correlation coefficient that relationship is linear). Zero indicates no relationship between the two measures and r = 1.00 or r = -1.00 indicates a perfect relationship. The strength can be anywhere between 0 and + 1.00. Note: The symbol r is used to represent the Pearson product-moment correlation coefficient for a sample. The Greek letter rho ( r ) is used for a population. The stronger the correlation–the closer the value of r (correlation coefficient) comes to + 1.00–the more the scatterplot will plot along a line.
When there is no relationship between the measures (variables), we say they are unrelated, uncorrelated, orthogonal, or independent .
Some Math for Bivariate Product Moment Correlation (not required for EPSY 5601): Multiple the z scores of each pair and add all of those products. Divide that by one less than the number of pairs of scores. (pretty easy)
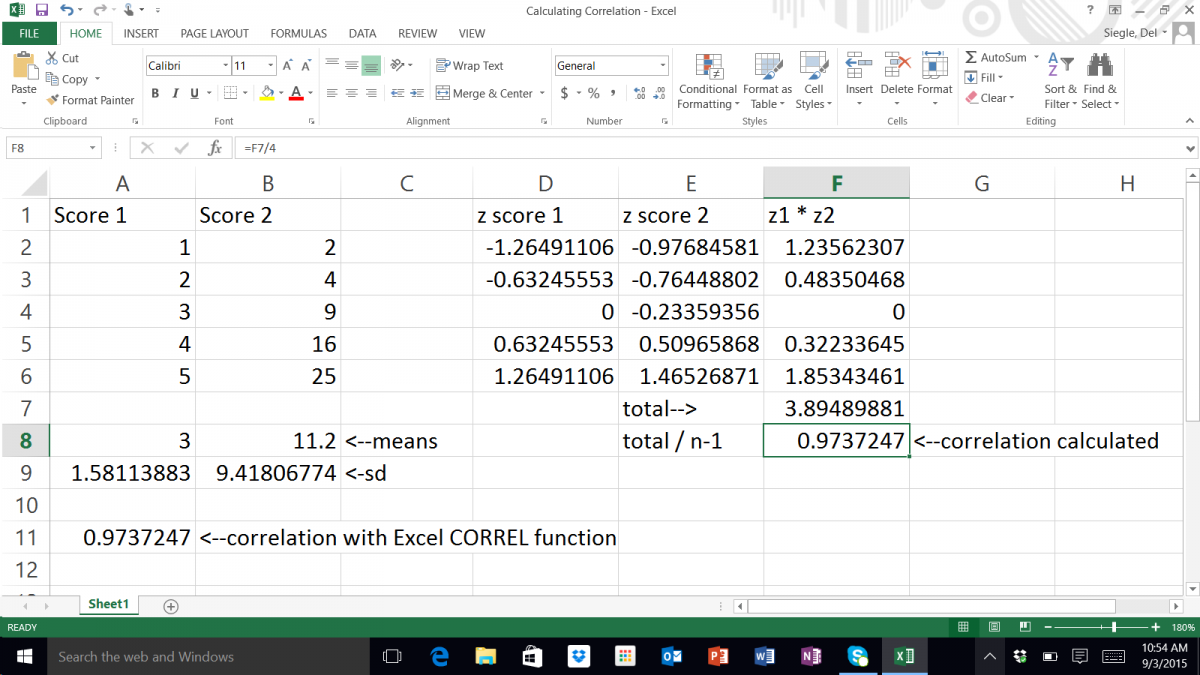
Rather than calculating the correlation coefficient with either of the formulas shown above, you can simply follow these linked directions for using the function built into Microsoft’s Excel .
Some correlation questions elementary students can investigate are What is the relationship between…
- school attendance and grades in school?
- hours spend each week doing homework and school grades?
- length of arm span and height?
- number of children in a family and the number of bedrooms in the house?
Correlations only describe the relationship, they do not prove cause and effect. Correlation is a necessary, but not a sufficient condition for determining causality.
There are Three Requirements to Infer a Causal Relationship
- A statistically significant relationship between the variables
- The causal variable occurred prior to the other variable
- There are no other factors that could account for the cause
(Correlation studies do not meet the last requirement and may not meet the second requirement. However, not having a relationship does mean that one variable did not cause the other.)
There is a strong relationship between the number of ice cream cones sold and the number of people who drown each month. Just because there is a relationship (strong correlation) does not mean that one caused the other.
If there is a relationship between A (ice cream cone sales) and B (drowning) it could be because
- A->B (Eating ice cream causes drowning)
- A<-B (Drowning cause people to eat ice cream– perhaps the mourners are so upset that they buy ice cream cones to cheer themselves)
- A<-C->B (Something else is related to both ice cream sales and the number of drowning– warm weather would be a good guess)
The points is…just because there is a correlation, you CANNOT say that the one variable causes the other. On the other hand, if there is NO correlations, you can say that one DID NOT cause the other (assuming the measures are valid and reliable).
Format for correlations research questions and hypotheses:
Question: Is there a (statistically significant) relationship between height and arm span? H O : There is no (statistically significant) relationship between height and arm span (H 0 : r =0). H A : There is a (statistically significant) relationship between height and arm span (H A : r <>0).
Coefficient of Determination (Shared Variation)
One way researchers often express the strength of the relationship between two variables is by squaring their correlation coefficient. This squared correlation coefficient is called a COEFFICIENT OF DETERMINATION. The coefficient of determination is useful because it gives the proportion of the variance of one variable that is predictable from the other variable.
Factors which could limit a product-moment correlation coefficient ( PowerPoint demonstrating these factors )
- Homogenous group (the subjects are very similar on the variables)
- Unreliable measurement instrument (your measurements can’t be trusted and bounce all over the place)
- Nonlinear relationship (Pearson’s r is based on linear relationships…other formulas can be used in this case)
- Ceiling or Floor with measurement (lots of scores clumped at the top or bottom…therefore no spread which creates a problem similar to the homogeneous group)
Assumptions one must meet in order to use the Pearson product-moment correlation
- The measures are approximately normally distributed
- The variance of the two measures is similar ( homoscedasticity ) — check with scatterplot
- The relationship is linear — check with scatterplot
- The sample represents the population
- The variables are measured on a interval or ratio scale
There are different types of relationships: Linear – Nonlinear or Curvilinear – Non-monotonic (concave or cyclical). Different procedures are used to measure different types of relationships using different types of scales . The issue of measurement scales is very important for this class. Be sure that you understand them.
Predictor and Criterion Variables (NOT NEEDED FOR EPSY 5601)
- Multiple Correlation- lots of predictors and one criterion ( R )
- Partial Correlation- correlation of two variables after their correlation with other variables is removed
- Serial or Autocorrelation- correlation of a set of number with itself (only staggered one)
- Canonical Correlation- lots of predictors and lots of criterion R c
When using a critical value table for Pearson’s product-moment correlation , the value found through the intersection of degree of freedom ( n – 2) and the alpha level you are testing ( p = .05) is the minimum r value needed in order for the relationship to be above chance alone.
The statistics package SPSS as well as Microsoft’s Excel can be used to calculate the correlation.
We will use Microsoft’s Excel .
Reading a Correlations Table in a Journal Article
Most research studies report the correlations among a set of variables. The results are presented in a table such as the one shown below.
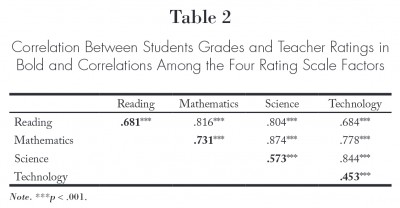
The intersection of a row and column shows the correlation between the variable listed for the row and the variable listed for the column. For example, the intersection of the row mathematics and the column science shows that the correlation between mathematics and science was .874. The footnote states that the three *** after .874 indicate the relationship was statistically significant at p <.001.
Most tables do not report the perfect correlation along the diagonal that occurs when a variable is correlated with itself. In the example above, the diagonal was used to report the correlation of the four factors with a different variable. Because the correlation between reading and mathematics can be determined in the top section of the table, the correlations between those two variables is not repeated in the bottom half of the table. This is true for all of the relationships reported in the table. .
Del Siegle, Ph.D. Neag School of Education – University of Connecticut [email protected] www.delsiegle.com
Last updated 10/11/2015

- Basics of Research Process
- Methodology
Correlational Study: Design, Methods and Examples
- Speech Topics
- Basics of Essay Writing
- Essay Topics
- Other Essays
- Main Academic Essays
- Research Paper Topics
- Basics of Research Paper Writing
- Miscellaneous
- Chicago/ Turabian
- Data & Statistics
- Admission Writing Tips
- Admission Advice
- Other Guides
- Student Life
- Studying Tips
- Understanding Plagiarism
- Academic Writing Tips
- Basics of Dissertation & Thesis Writing

- Essay Guides
- Research Paper Guides
- Formatting Guides
- Admission Guides
- Dissertation & Thesis Guides

Table of contents

Use our free Readability checker
Correlational research is a type of research design used to examine the relationship between two or more variables. In correlational research, researchers measure the extent to which two or more variables are related, without manipulating or controlling any of the variables.
Whether you are a beginner or an experienced researcher, chances are you’ve heard something about correlational research. It’s time that you learn more about this type of study more in-depth, since you will be using it a lot.
- What is correlation?
- When to use it?
- How is it different from experimental studies?
- What data collection method will work?
Grab your pen and get ready to jot down some notes as our paper writing service is going to cover all questions you may have about this type of study. Let’s get down to business!
What Is Correlational Research: Definition
A correlational research is a preliminary type of study used to explore the connection between two variables. In this type of research, you won’t interfere with the variables. Instead of manipulating or adjusting them, researchers focus more on observation. Correlational study is a perfect option if you want to figure out if there is any link between variables. You will conduct it in 2 cases:
- When you want to test a theory about non-causal connection. For example, you may want to know whether drinking hot water boosts the immune system. In this case, you expect that vitamins, healthy lifestyle and regular exercise are those factors that have a real positive impact. However, this doesn’t mean that drinking hot water isn’t associated with the immune system. So measuring this relationship will be really useful.
- When you want to investigate a causal link. You want to study whether using aerosol products leads to ozone depletion. You don’t have enough expenses for conducting complex research. Besides, you can’t control how often people use aerosols. In this case, you will opt for a correlational study.
Correlational Study: Purpose
Correlational research is most useful for purposes of observation and prediction. Researcher's goal is to observe and measure variables to determine if any relationship exists. In case there is some association, researchers assess how strong it is. As an initial type of research, this method allows you to test and write the hypotheses. Correlational study doesn’t require much time and is rather cheap.
Correlational Research Design
Correlational research designs are often used in psychology, epidemiology , medicine and nursing. They show the strength of correlation that exists between the variables within a population. For this reason, these studies are also known as ecological studies. Correlational research design methods are characterized by such traits:
- Non-experimental method. No manipulation or exposure to extra conditions takes place. Researchers only examine how variables act in their natural environment without any interference.
- Fluctuating patterns. Association is never the same and can change due to various factors.
- Quantitative research. These studies require quantitative research methods . Researchers mostly run a statistical analysis and work with numbers to get results.
- Association-oriented study. Correlational study is aimed at finding an association between 2 or more phenomena or events. This has nothing to do with causal relationships between dependent and independent variables .
Correlational Research Questions
Correlational research questions usually focus on how one variable related to another one. If there is some connection, you will observe how strong it is. Let’s look at several examples.
|
|
|
Is there any relationship between the regular use of social media and eating habits? | There is a positive relationship between the frequent use of social media and excessive eating. | There is no relationship between the time spent on social media and eating habits. |
What effect does social distancing have on depression? | There is a strong association between the time people are isolated and the level of depression. | There is no association between isolation and depression. |
Correlational Research Types
Depending on the direction and strength of association, there are 3 types of correlational research:
- Positive correlation If one variable increases, the other one will grow accordingly. If there is any reduction, both variables will decrease.

- Negative correlation All changes happen in the reverse direction. If one variable increases, the other one should decrease and vice versa.

- Zero correlation No association between 2 factors or events can be found.

Correlational Research: Data Collection Methods
There are 3 main methods applied to collect data in correlational research:
- Surveys and polls
- Naturalistic observation
- Secondary or archival data.
It’s essential that you select the right study method. Otherwise, it won’t be possible to achieve accurate results and answer the research question correctly. Let’s have a closer look at each of these methods to make sure that you make the right choice.
Surveys in Correlational Study
Survey is an easy way to collect data about a population in a correlational study. Depending on the nature of the question, you can choose different survey variations. Questionnaires, polls and interviews are the three most popular formats used in a survey research study. To conduct an effective study, you should first identify the population and choose whether you want to run a survey online, via email or in person.
Naturalistic Observation: Correlational Research
Naturalistic observation is another data collection approach in correlational research methodology. This method allows us to observe behavioral patterns in a natural setting. Scientists often document, describe or categorize data to get a clear picture about a group of people. During naturalistic observations, you may work with both qualitative and quantitative research information. Nevertheless, to measure the strength of association, you should analyze numeric data. Members of a population shouldn’t know that they are being studied. Thus, you should blend in a target group as naturally as possible. Otherwise, participants may behave in a different way which may cause a statistical error.
Correlational Study: Archival Data
Sometimes, you may access ready-made data that suits your study. Archival data is a quick correlational research method that allows to obtain necessary details from the similar studies that have already been conducted. You won’t deal with data collection techniques , since most of numbers will be served on a silver platter. All you will be left to do is analyze them and draw a conclusion. Unfortunately, not all records are accurate, so you should rely only on credible sources.
Pros and Cons of Correlational Research
Choosing what study to run can be difficult. But in this article, we are going to take an in-depth look at advantages and disadvantages of correlational research. This should help you decide whether this type of study is the best fit for you. Without any ado, let’s dive deep right in.
Advantages of Correlational Research
Obviously, one of the many advantages of correlational research is that it can be conducted when an experiment can’t be the case. Sometimes, it may be unethical to run an experimental study or you may have limited resources. This is exactly when ecological study can come in handy. This type of study also has several benefits that have an irreplaceable value:
- Works well as a preliminary study
- Allows examining complex connection between multiple variables
- Helps you study natural behavior
- Can be generalized to other settings.
If you decide to run an archival study or conduct a survey, you will be able to save much time and expenses.
Disadvantages of Correlational Research
There are several limitations of correlational research you should keep in mind while deciding on the main methodology. Here are the advantages one should consider:
- No causal relationships can be identified
- No chance to manipulate extraneous variables
- Biased results caused by unnatural behavior
- Naturalistic studies require quite a lot of time.
As you can see, these types of studies aren’t end-all, be-all. They may indicate a direction for further research. Still, correlational studies don’t show a cause-and-effect relationship which is probably the biggest disadvantage.
Difference Between Correlational and Experimental Research
Now that you’ve come this far, let’s discuss correlational vs experimental research design . Both studies involve quantitative data. But the main difference lies in the aim of research. Correlational studies are used to identify an association which is measured with a coefficient, while an experiment is aimed at determining a causal relationship. Due to a different purpose, the studies also have different approaches to control over variables. In the first case, scientists can’t control or otherwise manipulate the variables in question. Meanwhile, experiments allow you to control variables without limit. There is a causation vs correlation blog on our website. Find out their differences as it will be useful for your research.
Example of Correlational Research
Above, we have offered several correlational research examples. Let’s have a closer look at how things work using a more detailed example.
Example You want to determine if there is any connection between the time employees work in one company and their performance. An experiment will be rather time-consuming. For this reason, you can offer a questionnaire to collect data and assess an association. After running a survey, you will be able to confirm or disprove your hypothesis.
Correlational Study: Final Thoughts
That’s pretty much everything you should know about correlational study. The key takeaway is that this type of study is used to measure the connection between 2 or more variables. It’s a good choice if you have no chance to run an experiment. However, in this case you won’t be able to control for extraneous variables . So you should consider your options carefully before conducting your own research.

We’ve got your back! Entrust your assignment to our skilled paper writers and they will complete a custom research paper with top quality in mind!
Frequently Asked Questions About Correlational Study
1. what is a correlation.
Correlation is a connection that shows to which extent two or more variables are associated. It doesn’t show a causal link and only helps to identify a direction (positive, negative or zero) or the strength of association.
2. How many variables are in a correlation?
There can be many different variables in a correlation which makes this type of study very useful for exploring complex relationships. However, most scientists use this research to measure the association between only 2 variables.
3. What is a correlation coefficient?
Correlation coefficient (ρ) is a statistical measure that indicates the extent to which two variables are related. Association can be strong, moderate or weak. There are different types of p coefficients: positive, negative and zero.
4. What is a correlational study?
Correlational study is a type of statistical research that involves examining two variables in order to determine association between them. It’s a non-experimental type of study, meaning that researchers can’t change independent variables or control extraneous variables.

Joe Eckel is an expert on Dissertations writing. He makes sure that each student gets precious insights on composing A-grade academic writing.
You may also like

- Privacy Policy

Home » Correlation Analysis – Types, Methods and Examples
Correlation Analysis – Types, Methods and Examples
Table of Contents

Correlation Analysis
Correlation analysis is a statistical method used to evaluate the strength and direction of the relationship between two or more variables . The correlation coefficient ranges from -1 to 1.
- A correlation coefficient of 1 indicates a perfect positive correlation. This means that as one variable increases, the other variable also increases.
- A correlation coefficient of -1 indicates a perfect negative correlation. This means that as one variable increases, the other variable decreases.
- A correlation coefficient of 0 means that there’s no linear relationship between the two variables.
Correlation Analysis Methodology
Conducting a correlation analysis involves a series of steps, as described below:
- Define the Problem : Identify the variables that you think might be related. The variables must be measurable on an interval or ratio scale. For example, if you’re interested in studying the relationship between the amount of time spent studying and exam scores, these would be your two variables.
- Data Collection : Collect data on the variables of interest. The data could be collected through various means such as surveys , observations , or experiments. It’s crucial to ensure that the data collected is accurate and reliable.
- Data Inspection : Check the data for any errors or anomalies such as outliers or missing values. Outliers can greatly affect the correlation coefficient, so it’s crucial to handle them appropriately.
- Choose the Appropriate Correlation Method : Select the correlation method that’s most appropriate for your data. If your data meets the assumptions for Pearson’s correlation (interval or ratio level, linear relationship, variables are normally distributed), use that. If your data is ordinal or doesn’t meet the assumptions for Pearson’s correlation, consider using Spearman’s rank correlation or Kendall’s Tau.
- Compute the Correlation Coefficient : Once you’ve selected the appropriate method, compute the correlation coefficient. This can be done using statistical software such as R, Python, or SPSS, or manually using the formulas.
- Interpret the Results : Interpret the correlation coefficient you obtained. If the correlation is close to 1 or -1, the variables are strongly correlated. If the correlation is close to 0, the variables have little to no linear relationship. Also consider the sign of the correlation coefficient: a positive sign indicates a positive relationship (as one variable increases, so does the other), while a negative sign indicates a negative relationship (as one variable increases, the other decreases).
- Check the Significance : It’s also important to test the statistical significance of the correlation. This typically involves performing a t-test. A small p-value (commonly less than 0.05) suggests that the observed correlation is statistically significant and not due to random chance.
- Report the Results : The final step is to report your findings. This should include the correlation coefficient, the significance level, and a discussion of what these findings mean in the context of your research question.
Types of Correlation Analysis
Types of Correlation Analysis are as follows:
Pearson Correlation
This is the most common type of correlation analysis. Pearson correlation measures the linear relationship between two continuous variables. It assumes that the variables are normally distributed and have equal variances. The correlation coefficient (r) ranges from -1 to +1, with -1 indicating a perfect negative linear relationship, +1 indicating a perfect positive linear relationship, and 0 indicating no linear relationship.
Spearman Rank Correlation
Spearman’s rank correlation is a non-parametric measure that assesses how well the relationship between two variables can be described using a monotonic function. In other words, it evaluates the degree to which, as one variable increases, the other variable tends to increase, without requiring that increase to be consistent.
Kendall’s Tau
Kendall’s Tau is another non-parametric correlation measure used to detect the strength of dependence between two variables. Kendall’s Tau is often used for variables measured on an ordinal scale (i.e., where values can be ranked).
Point-Biserial Correlation
This is used when you have one dichotomous and one continuous variable, and you want to test for correlations. It’s a special case of the Pearson correlation.
Phi Coefficient
This is used when both variables are dichotomous or binary (having two categories). It’s a measure of association for two binary variables.
Canonical Correlation
This measures the correlation between two multi-dimensional variables. Each variable is a combination of data sets, and the method finds the linear combination that maximizes the correlation between them.

Partial and Semi-Partial (Part) Correlations
These are used when the researcher wants to understand the relationship between two variables while controlling for the effect of one or more additional variables.
Cross-Correlation
Used mostly in time series data to measure the similarity of two series as a function of the displacement of one relative to the other.
Autocorrelation
This is the correlation of a signal with a delayed copy of itself as a function of delay. This is often used in time series analysis to help understand the trend in the data over time.
Correlation Analysis Formulas
There are several formulas for correlation analysis, each corresponding to a different type of correlation. Here are some of the most commonly used ones:
Pearson’s Correlation Coefficient (r)
Pearson’s correlation coefficient measures the linear relationship between two variables. The formula is:
r = Σ[(xi – Xmean)(yi – Ymean)] / sqrt[(Σ(xi – Xmean)²)(Σ(yi – Ymean)²)]
- xi and yi are the values of X and Y variables.
- Xmean and Ymean are the mean values of X and Y.
- Σ denotes the sum of the values.
Spearman’s Rank Correlation Coefficient (rs)
Spearman’s correlation coefficient measures the monotonic relationship between two variables. The formula is:
rs = 1 – (6Σd² / n(n² – 1))
- d is the difference between the ranks of corresponding variables.
- n is the number of observations.
Kendall’s Tau (τ)
Kendall’s Tau is a measure of rank correlation. The formula is:
τ = (nc – nd) / 0.5n(n-1)
- nc is the number of concordant pairs.
- nd is the number of discordant pairs.
This correlation is a special case of Pearson’s correlation, and so, it uses the same formula as Pearson’s correlation.
Phi coefficient is a measure of association for two binary variables. It’s equivalent to Pearson’s correlation in this specific case.
Partial Correlation
The formula for partial correlation is more complex and depends on the Pearson’s correlation coefficients between the variables.
For partial correlation between X and Y given Z:
rp(xy.z) = (rxy – rxz * ryz) / sqrt[(1 – rxz^2)(1 – ryz^2)]
- rxy, rxz, ryz are the Pearson’s correlation coefficients.
Correlation Analysis Examples
Here are a few examples of how correlation analysis could be applied in different contexts:
- Education : A researcher might want to determine if there’s a relationship between the amount of time students spend studying each week and their exam scores. The two variables would be “study time” and “exam scores”. If a positive correlation is found, it means that students who study more tend to score higher on exams.
- Healthcare : A healthcare researcher might be interested in understanding the relationship between age and cholesterol levels. If a positive correlation is found, it could mean that as people age, their cholesterol levels tend to increase.
- Economics : An economist may want to investigate if there’s a correlation between the unemployment rate and the rate of crime in a given city. If a positive correlation is found, it could suggest that as the unemployment rate increases, the crime rate also tends to increase.
- Marketing : A marketing analyst might want to analyze the correlation between advertising expenditure and sales revenue. A positive correlation would suggest that higher advertising spending is associated with higher sales revenue.
- Environmental Science : A scientist might be interested in whether there’s a relationship between the amount of CO2 emissions and average temperature increase. A positive correlation would indicate that higher CO2 emissions are associated with higher average temperatures.
Importance of Correlation Analysis
Correlation analysis plays a crucial role in many fields of study for several reasons:
- Understanding Relationships : Correlation analysis provides a statistical measure of the relationship between two or more variables. It helps in understanding how one variable may change in relation to another.
- Predicting Trends : When variables are correlated, changes in one can predict changes in another. This is particularly useful in fields like finance, weather forecasting, and technology, where forecasting trends is vital.
- Data Reduction : If two variables are highly correlated, they are conveying similar information, and you may decide to use only one of them in your analysis, reducing the dimensionality of your data.
- Testing Hypotheses : Correlation analysis can be used to test hypotheses about relationships between variables. For example, a researcher might want to test whether there’s a significant positive correlation between physical exercise and mental health.
- Determining Factors : It can help identify factors that are associated with certain behaviors or outcomes. For example, public health researchers might analyze correlations to identify risk factors for diseases.
- Model Building : Correlation is a fundamental concept in building multivariate statistical models, including regression models and structural equation models. These models often require an understanding of the inter-relationships (correlations) among multiple variables.
- Validity and Reliability Analysis : In psychometrics, correlation analysis is used to assess the validity and reliability of measurement instruments such as tests or surveys.
Applications of Correlation Analysis
Correlation analysis is used in many fields to understand and quantify the relationship between variables. Here are some of its key applications:
- Finance : In finance, correlation analysis is used to understand the relationship between different investment types or the risk and return of a portfolio. For example, if two stocks are positively correlated, they tend to move together; if they’re negatively correlated, they move in opposite directions.
- Economics : Economists use correlation analysis to understand the relationship between various economic indicators, such as GDP and unemployment rate, inflation rate and interest rates, or income and consumption patterns.
- Marketing : Correlation analysis can help marketers understand the relationship between advertising spend and sales, or the relationship between price changes and demand.
- Psychology : In psychology, correlation analysis can be used to understand the relationship between different psychological variables, such as the correlation between stress levels and sleep quality, or between self-esteem and academic performance.
- Medicine : In healthcare, correlation analysis can be used to understand the relationships between various health outcomes and potential predictors. For example, researchers might investigate the correlation between physical activity levels and heart disease, or between smoking and lung cancer.
- Environmental Science : Correlation analysis can be used to investigate the relationships between different environmental factors, such as the correlation between CO2 levels and average global temperature, or between pesticide use and biodiversity.
- Social Sciences : In fields like sociology and political science, correlation analysis can be used to investigate relationships between different social and political phenomena, such as the correlation between education levels and political participation, or between income inequality and social unrest.
Advantages and Disadvantages of Correlation Analysis
Advantages | Disadvantages |
---|---|
Provides statistical measure of the relationship between variables. | Cannot establish causality, only association. |
Useful for prediction if variables are known to have a correlation. | Can be misleading if important variables are left out (omitted variable bias). |
Can help in hypothesis testing about the relationships between variables. | Outliers can greatly affect the correlation coefficient. |
Can help in data reduction by identifying closely related variables. | Assumes a linear relationship in Pearson correlation, which may not always hold. |
Fundamental concept in building multivariate statistical models. | May not capture complex relationships (e.g., quadratic or cyclical relationships). |
Helps in validity and reliability analysis in psychometrics. | Correlation can be affected by the range of observed values (restriction of range). |
About the author
Muhammad Hassan
Researcher, Academic Writer, Web developer
You may also like

Textual Analysis – Types, Examples and Guide

Critical Analysis – Types, Examples and Writing...

Documentary Analysis – Methods, Applications and...

Histogram – Types, Examples and Making Guide

Content Analysis – Methods, Types and Examples

Factor Analysis – Steps, Methods and Examples
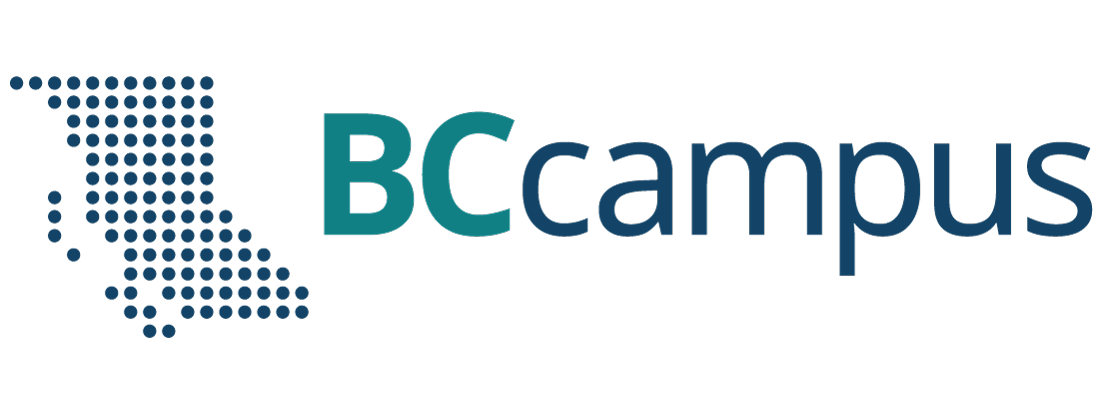
Want to create or adapt books like this? Learn more about how Pressbooks supports open publishing practices.
Chapter 7: Nonexperimental Research
Correlational Research
Learning Objectives
- Define correlational research and give several examples.
- Explain why a researcher might choose to conduct correlational research rather than experimental research or another type of nonexperimental research.
What Is Correlational Research?
Correlational research is a type of nonexperimental research in which the researcher measures two variables and assesses the statistical relationship (i.e., the correlation) between them with little or no effort to control extraneous variables. There are essentially two reasons that researchers interested in statistical relationships between variables would choose to conduct a correlational study rather than an experiment. The first is that they do not believe that the statistical relationship is a causal one. For example, a researcher might evaluate the validity of a brief extraversion test by administering it to a large group of participants along with a longer extraversion test that has already been shown to be valid. This researcher might then check to see whether participants’ scores on the brief test are strongly correlated with their scores on the longer one. Neither test score is thought to cause the other, so there is no independent variable to manipulate. In fact, the terms independent variable and dependent variabl e do not apply to this kind of research.
The other reason that researchers would choose to use a correlational study rather than an experiment is that the statistical relationship of interest is thought to be causal, but the researcher cannot manipulate the independent variable because it is impossible, impractical, or unethical. For example, Allen Kanner and his colleagues thought that the number of “daily hassles” (e.g., rude salespeople, heavy traffic) that people experience affects the number of physical and psychological symptoms they have (Kanner, Coyne, Schaefer, & Lazarus, 1981). [1] But because they could not manipulate the number of daily hassles their participants experienced, they had to settle for measuring the number of daily hassles—along with the number of symptoms—using self-report questionnaires. Although the strong positive relationship they found between these two variables is consistent with their idea that hassles cause symptoms, it is also consistent with the idea that symptoms cause hassles or that some third variable (e.g., neuroticism) causes both.
A common misconception among beginning researchers is that correlational research must involve two quantitative variables, such as scores on two extroversion tests or the number of hassles and number of symptoms people have experienced. However, the defining feature of correlational research is that the two variables are measured—neither one is manipulated—and this is true regardless of whether the variables are quantitative or categorical. Imagine, for example, that a researcher administers the Rosenberg Self-Esteem Scale to 50 American university students and 50 Japanese university students. Although this “feels” like a between-subjects experiment, it is a correlational study because the researcher did not manipulate the students’ nationalities. The same is true of the study by Cacioppo and Petty comparing professors and factory workers in terms of their need for cognition. It is a correlational study because the researchers did not manipulate the participants’ occupations.
Figure 7.2 shows data from a hypothetical study on the relationship between whether people make a daily list of things to do (a “to-do list”) and stress. Notice that it is unclear whether this design is an experiment or a correlational study because it is unclear whether the independent variable was manipulated. If the researcher randomly assigned some participants to make daily to-do lists and others not to, then it is an experiment. If the researcher simply asked participants whether they made daily to-do lists, then it is a correlational study. The distinction is important because if the study was an experiment, then it could be concluded that making the daily to-do lists reduced participants’ stress. But if it was a correlational study, it could only be concluded that these variables are related. Perhaps being stressed has a negative effect on people’s ability to plan ahead (the directionality problem). Or perhaps people who are more conscientious are more likely to make to-do lists and less likely to be stressed (the third-variable problem). The crucial point is that what defines a study as experimental or correlational is not the variables being studied, nor whether the variables are quantitative or categorical, nor the type of graph or statistics used to analyze the data. It is how the study is conducted.
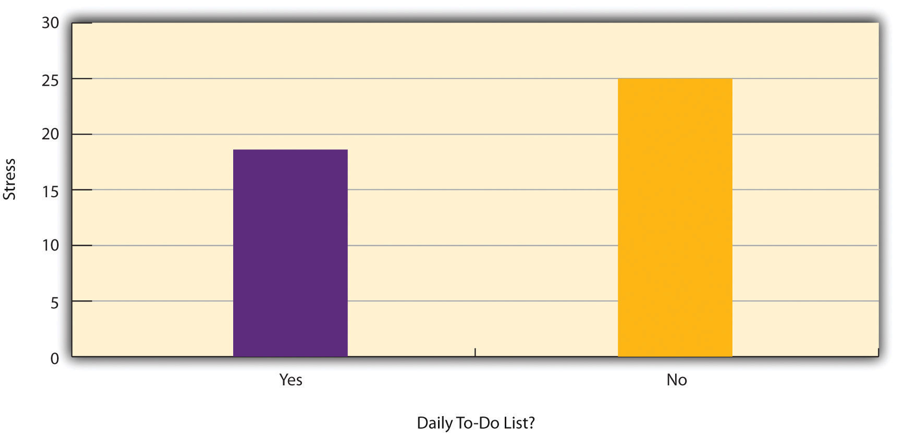
Data Collection in Correlational Research
Again, the defining feature of correlational research is that neither variable is manipulated. It does not matter how or where the variables are measured. A researcher could have participants come to a laboratory to complete a computerized backward digit span task and a computerized risky decision-making task and then assess the relationship between participants’ scores on the two tasks. Or a researcher could go to a shopping mall to ask people about their attitudes toward the environment and their shopping habits and then assess the relationship between these two variables. Both of these studies would be correlational because no independent variable is manipulated. However, because some approaches to data collection are strongly associated with correlational research, it makes sense to discuss them here. The two we will focus on are naturalistic observation and archival data. A third, survey research, is discussed in its own chapter, Chapter 9.
Naturalistic Observation
Naturalistic observation is an approach to data collection that involves observing people’s behaviour in the environment in which it typically occurs. Thus naturalistic observation is a type of field research (as opposed to a type of laboratory research). It could involve observing shoppers in a grocery store, children on a school playground, or psychiatric inpatients in their wards. Researchers engaged in naturalistic observation usually make their observations as unobtrusively as possible so that participants are often not aware that they are being studied. Ethically, this method is considered to be acceptable if the participants remain anonymous and the behaviour occurs in a public setting where people would not normally have an expectation of privacy. Grocery shoppers putting items into their shopping carts, for example, are engaged in public behaviour that is easily observable by store employees and other shoppers. For this reason, most researchers would consider it ethically acceptable to observe them for a study. On the other hand, one of the arguments against the ethicality of the naturalistic observation of “bathroom behaviour” discussed earlier in the book is that people have a reasonable expectation of privacy even in a public restroom and that this expectation was violated.
Researchers Robert Levine and Ara Norenzayan used naturalistic observation to study differences in the “pace of life” across countries (Levine & Norenzayan, 1999). [2] One of their measures involved observing pedestrians in a large city to see how long it took them to walk 60 feet. They found that people in some countries walked reliably faster than people in other countries. For example, people in Canada and Sweden covered 60 feet in just under 13 seconds on average, while people in Brazil and Romania took close to 17 seconds.
Because naturalistic observation takes place in the complex and even chaotic “real world,” there are two closely related issues that researchers must deal with before collecting data. The first is sampling. When, where, and under what conditions will the observations be made, and who exactly will be observed? Levine and Norenzayan described their sampling process as follows:
“Male and female walking speed over a distance of 60 feet was measured in at least two locations in main downtown areas in each city. Measurements were taken during main business hours on clear summer days. All locations were flat, unobstructed, had broad sidewalks, and were sufficiently uncrowded to allow pedestrians to move at potentially maximum speeds. To control for the effects of socializing, only pedestrians walking alone were used. Children, individuals with obvious physical handicaps, and window-shoppers were not timed. Thirty-five men and 35 women were timed in most cities.” (p. 186)
Precise specification of the sampling process in this way makes data collection manageable for the observers, and it also provides some control over important extraneous variables. For example, by making their observations on clear summer days in all countries, Levine and Norenzayan controlled for effects of the weather on people’s walking speeds.
The second issue is measurement. What specific behaviours will be observed? In Levine and Norenzayan’s study, measurement was relatively straightforward. They simply measured out a 60-foot distance along a city sidewalk and then used a stopwatch to time participants as they walked over that distance. Often, however, the behaviours of interest are not so obvious or objective. For example, researchers Robert Kraut and Robert Johnston wanted to study bowlers’ reactions to their shots, both when they were facing the pins and then when they turned toward their companions (Kraut & Johnston, 1979). [3] But what “reactions” should they observe? Based on previous research and their own pilot testing, Kraut and Johnston created a list of reactions that included “closed smile,” “open smile,” “laugh,” “neutral face,” “look down,” “look away,” and “face cover” (covering one’s face with one’s hands). The observers committed this list to memory and then practised by coding the reactions of bowlers who had been videotaped. During the actual study, the observers spoke into an audio recorder, describing the reactions they observed. Among the most interesting results of this study was that bowlers rarely smiled while they still faced the pins. They were much more likely to smile after they turned toward their companions, suggesting that smiling is not purely an expression of happiness but also a form of social communication.
When the observations require a judgment on the part of the observers—as in Kraut and Johnston’s study—this process is often described as coding . Coding generally requires clearly defining a set of target behaviours. The observers then categorize participants individually in terms of which behaviour they have engaged in and the number of times they engaged in each behaviour. The observers might even record the duration of each behaviour. The target behaviours must be defined in such a way that different observers code them in the same way. This difficulty with coding is the issue of interrater reliability, as mentioned in Chapter 5. Researchers are expected to demonstrate the interrater reliability of their coding procedure by having multiple raters code the same behaviours independently and then showing that the different observers are in close agreement. Kraut and Johnston, for example, video recorded a subset of their participants’ reactions and had two observers independently code them. The two observers showed that they agreed on the reactions that were exhibited 97% of the time, indicating good interrater reliability.
Archival Data
Another approach to correlational research is the use of archival data , which are data that have already been collected for some other purpose. An example is a study by Brett Pelham and his colleagues on “implicit egotism”—the tendency for people to prefer people, places, and things that are similar to themselves (Pelham, Carvallo, & Jones, 2005). [4] In one study, they examined Social Security records to show that women with the names Virginia, Georgia, Louise, and Florence were especially likely to have moved to the states of Virginia, Georgia, Louisiana, and Florida, respectively.
As with naturalistic observation, measurement can be more or less straightforward when working with archival data. For example, counting the number of people named Virginia who live in various states based on Social Security records is relatively straightforward. But consider a study by Christopher Peterson and his colleagues on the relationship between optimism and health using data that had been collected many years before for a study on adult development (Peterson, Seligman, & Vaillant, 1988). [5] In the 1940s, healthy male college students had completed an open-ended questionnaire about difficult wartime experiences. In the late 1980s, Peterson and his colleagues reviewed the men’s questionnaire responses to obtain a measure of explanatory style—their habitual ways of explaining bad events that happen to them. More pessimistic people tend to blame themselves and expect long-term negative consequences that affect many aspects of their lives, while more optimistic people tend to blame outside forces and expect limited negative consequences. To obtain a measure of explanatory style for each participant, the researchers used a procedure in which all negative events mentioned in the questionnaire responses, and any causal explanations for them, were identified and written on index cards. These were given to a separate group of raters who rated each explanation in terms of three separate dimensions of optimism-pessimism. These ratings were then averaged to produce an explanatory style score for each participant. The researchers then assessed the statistical relationship between the men’s explanatory style as undergraduate students and archival measures of their health at approximately 60 years of age. The primary result was that the more optimistic the men were as undergraduate students, the healthier they were as older men. Pearson’s r was +.25.
This method is an example of content analysis —a family of systematic approaches to measurement using complex archival data. Just as naturalistic observation requires specifying the behaviours of interest and then noting them as they occur, content analysis requires specifying keywords, phrases, or ideas and then finding all occurrences of them in the data. These occurrences can then be counted, timed (e.g., the amount of time devoted to entertainment topics on the nightly news show), or analyzed in a variety of other ways.
Key Takeaways
- Correlational research involves measuring two variables and assessing the relationship between them, with no manipulation of an independent variable.
- Correlational research is not defined by where or how the data are collected. However, some approaches to data collection are strongly associated with correlational research. These include naturalistic observation (in which researchers observe people’s behaviour in the context in which it normally occurs) and the use of archival data that were already collected for some other purpose.
Discussion: For each of the following, decide whether it is most likely that the study described is experimental or correlational and explain why.
- An educational researcher compares the academic performance of students from the “rich” side of town with that of students from the “poor” side of town.
- A cognitive psychologist compares the ability of people to recall words that they were instructed to “read” with their ability to recall words that they were instructed to “imagine.”
- A manager studies the correlation between new employees’ college grade point averages and their first-year performance reports.
- An automotive engineer installs different stick shifts in a new car prototype, each time asking several people to rate how comfortable the stick shift feels.
- A food scientist studies the relationship between the temperature inside people’s refrigerators and the amount of bacteria on their food.
- A social psychologist tells some research participants that they need to hurry over to the next building to complete a study. She tells others that they can take their time. Then she observes whether they stop to help a research assistant who is pretending to be hurt.
- Kanner, A. D., Coyne, J. C., Schaefer, C., & Lazarus, R. S. (1981). Comparison of two modes of stress measurement: Daily hassles and uplifts versus major life events. Journal of Behavioural Medicine, 4 , 1–39. ↵
- Levine, R. V., & Norenzayan, A. (1999). The pace of life in 31 countries. Journal of Cross-Cultural Psychology, 30 , 178–205. ↵
- Kraut, R. E., & Johnston, R. E. (1979). Social and emotional messages of smiling: An ethological approach. Journal of Personality and Social Psychology, 37 , 1539–1553. ↵
- Pelham, B. W., Carvallo, M., & Jones, J. T. (2005). Implicit egotism. Current Directions in Psychological Science, 14 , 106–110. ↵
- Peterson, C., Seligman, M. E. P., & Vaillant, G. E. (1988). Pessimistic explanatory style is a risk factor for physical illness: A thirty-five year longitudinal study. Journal of Personality and Social Psychology, 55 , 23–27. ↵
An approach to data collection that involves observing people’s behaviour in the environment in which it typically occurs.
A judgment on part of the observers by clearly defining a set of target behaviours.
Data that have already been collected for some other purpose.
A family of systematic approaches to measurement using complex archival data.
Research Methods in Psychology - 2nd Canadian Edition Copyright © 2015 by Paul C. Price, Rajiv Jhangiani, & I-Chant A. Chiang is licensed under a Creative Commons Attribution-NonCommercial-ShareAlike 4.0 International License , except where otherwise noted.
Share This Book
Correlation in Psychology: Meaning, Types, Examples & coefficient
Saul Mcleod, PhD
Editor-in-Chief for Simply Psychology
BSc (Hons) Psychology, MRes, PhD, University of Manchester
Saul Mcleod, PhD., is a qualified psychology teacher with over 18 years of experience in further and higher education. He has been published in peer-reviewed journals, including the Journal of Clinical Psychology.
Learn about our Editorial Process
Olivia Guy-Evans, MSc
Associate Editor for Simply Psychology
BSc (Hons) Psychology, MSc Psychology of Education
Olivia Guy-Evans is a writer and associate editor for Simply Psychology. She has previously worked in healthcare and educational sectors.
On This Page:
Correlation means association – more precisely, it measures the extent to which two variables are related. There are three possible results of a correlational study: a positive correlation, a negative correlation, and no correlation.
- A positive correlation is a relationship between two variables in which both variables move in the same direction. Therefore, one variable increases as the other variable increases, or one variable decreases while the other decreases. An example of a positive correlation would be height and weight. Taller people tend to be heavier.
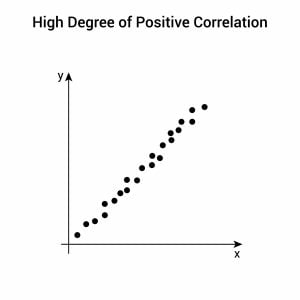
- A negative correlation is a relationship between two variables in which an increase in one variable is associated with a decrease in the other. An example of a negative correlation would be the height above sea level and temperature. As you climb the mountain (increase in height), it gets colder (decrease in temperature).
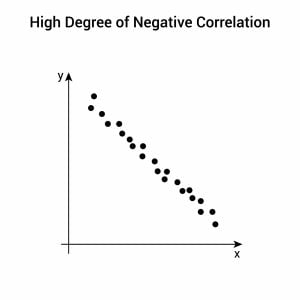
- A zero correlation exists when there is no relationship between two variables. For example, there is no relationship between the amount of tea drunk and the level of intelligence.
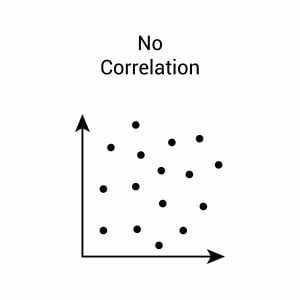
Scatter Plots
A correlation can be expressed visually. This is done by drawing a scatter plot (also known as a scattergram, scatter graph, scatter chart, or scatter diagram).
A scatter plot is a graphical display that shows the relationships or associations between two numerical variables (or co-variables), which are represented as points (or dots) for each pair of scores.
A scatter plot indicates the strength and direction of the correlation between the co-variables.
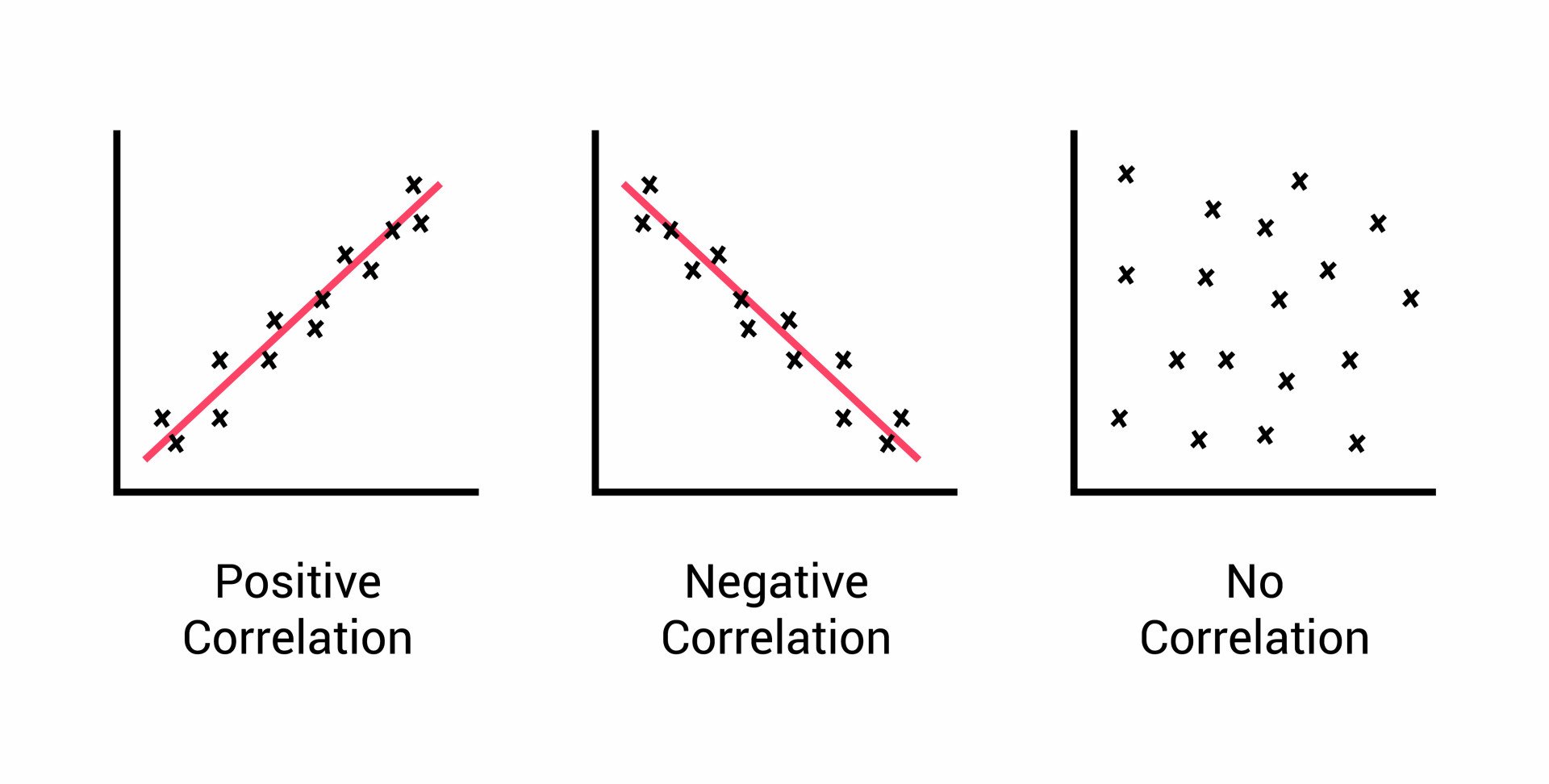
When you draw a scatter plot, it doesn’t matter which variable goes on the x-axis and which goes on the y-axis.
Remember, in correlations, we always deal with paired scores, so the values of the two variables taken together will be used to make the diagram.
Decide which variable goes on each axis and then simply put a cross at the point where the two values coincide.
Uses of Correlations
- If there is a relationship between two variables, we can make predictions about one from another.
- Concurrent validity (correlation between a new measure and an established measure).
Reliability
- Test-retest reliability (are measures consistent?).
- Inter-rater reliability (are observers consistent?).
Theory verification
- Predictive validity.
Correlation Coefficients
Instead of drawing a scatter plot, a correlation can be expressed numerically as a coefficient, ranging from -1 to +1. When working with continuous variables, the correlation coefficient to use is Pearson’s r.
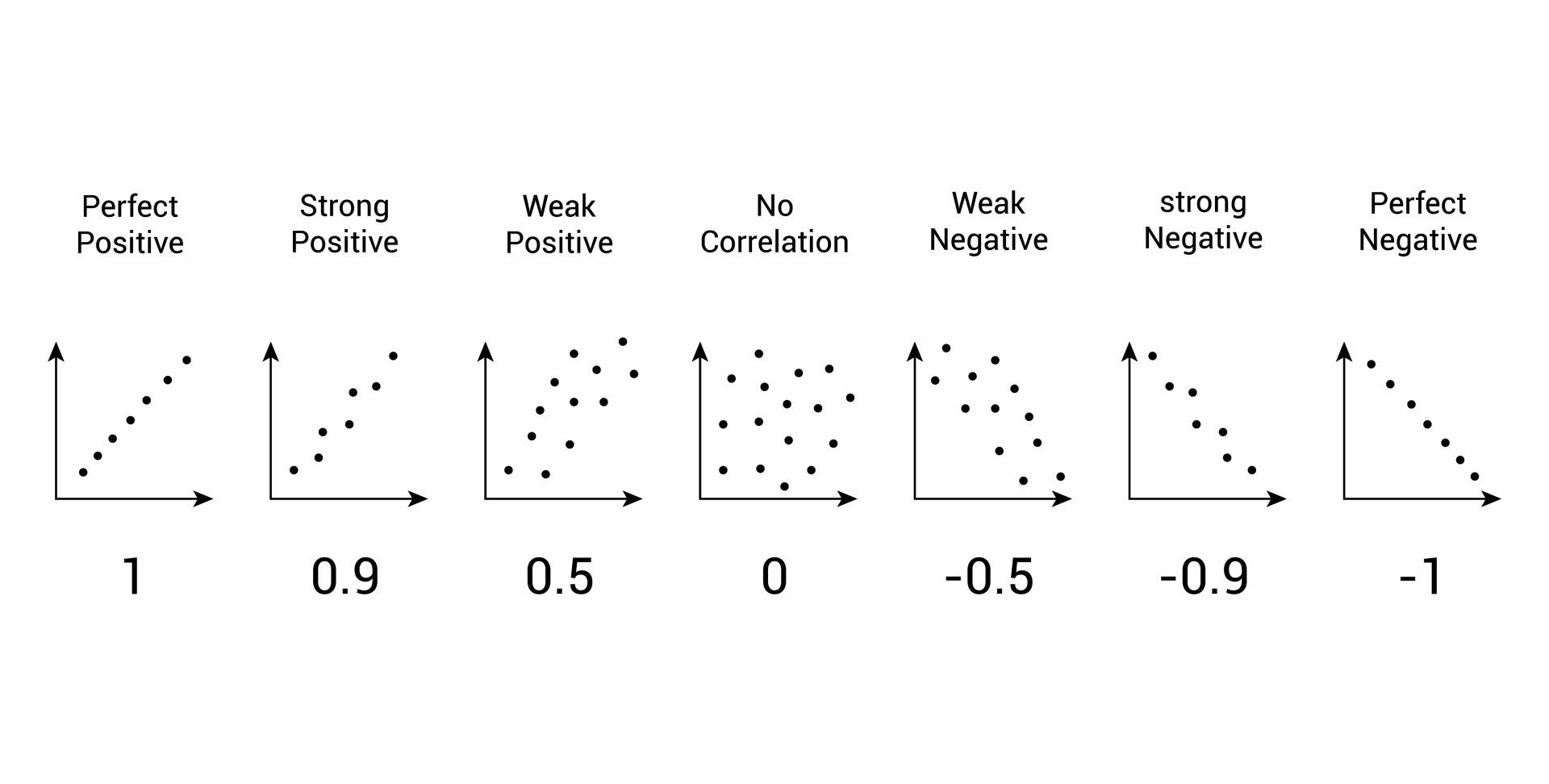
The correlation coefficient ( r ) indicates the extent to which the pairs of numbers for these two variables lie on a straight line. Values over zero indicate a positive correlation, while values under zero indicate a negative correlation.
A correlation of –1 indicates a perfect negative correlation, meaning that as one variable goes up, the other goes down. A correlation of +1 indicates a perfect positive correlation, meaning that as one variable goes up, the other goes up.
There is no rule for determining what correlation size is considered strong, moderate, or weak. The interpretation of the coefficient depends on the topic of study.
When studying things that are difficult to measure, we should expect the correlation coefficients to be lower (e.g., above 0.4 to be relatively strong). When we are studying things that are easier to measure, such as socioeconomic status, we expect higher correlations (e.g., above 0.75 to be relatively strong).)
In these kinds of studies, we rarely see correlations above 0.6. For this kind of data, we generally consider correlations above 0.4 to be relatively strong; correlations between 0.2 and 0.4 are moderate, and those below 0.2 are considered weak.
When we are studying things that are more easily countable, we expect higher correlations. For example, with demographic data, we generally consider correlations above 0.75 to be relatively strong; correlations between 0.45 and 0.75 are moderate, and those below 0.45 are considered weak.
Correlation vs. Causation
Causation means that one variable (often called the predictor variable or independent variable) causes the other (often called the outcome variable or dependent variable).
Experiments can be conducted to establish causation. An experiment isolates and manipulates the independent variable to observe its effect on the dependent variable and controls the environment in order that extraneous variables may be eliminated.
A correlation between variables, however, does not automatically mean that the change in one variable is the cause of the change in the values of the other variable. A correlation only shows if there is a relationship between variables.
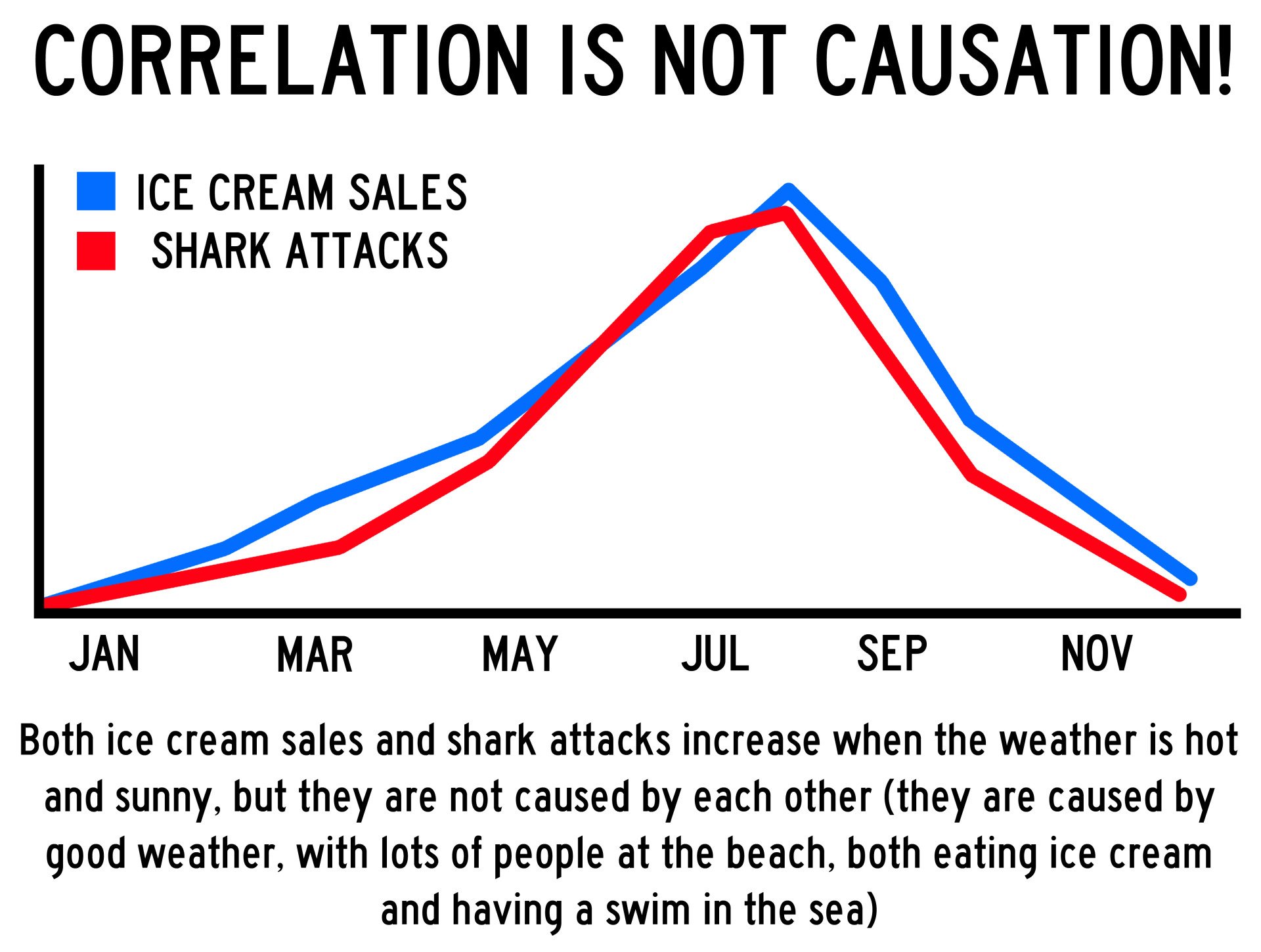
While variables are sometimes correlated because one does cause the other, it could also be that some other factor, a confounding variable , is actually causing the systematic movement in our variables of interest.
Correlation does not always prove causation, as a third variable may be involved. For example, being a patient in a hospital is correlated with dying, but this does not mean that one event causes the other, as another third variable might be involved (such as diet and level of exercise).
“Correlation is not causation” means that just because two variables are related it does not necessarily mean that one causes the other.
A correlation identifies variables and looks for a relationship between them. An experiment tests the effect that an independent variable has upon a dependent variable but a correlation looks for a relationship between two variables.
This means that the experiment can predict cause and effect (causation) but a correlation can only predict a relationship, as another extraneous variable may be involved that it not known about.
1. Correlation allows the researcher to investigate naturally occurring variables that may be unethical or impractical to test experimentally. For example, it would be unethical to conduct an experiment on whether smoking causes lung cancer.
2 . Correlation allows the researcher to clearly and easily see if there is a relationship between variables. This can then be displayed in a graphical form.
Limitations
1 . Correlation is not and cannot be taken to imply causation. Even if there is a very strong association between two variables, we cannot assume that one causes the other.
For example, suppose we found a positive correlation between watching violence on T.V. and violent behavior in adolescence.
It could be that the cause of both these is a third (extraneous) variable – for example, growing up in a violent home – and that both the watching of T.V. and the violent behavior is the outcome of this.
2 . Correlation does not allow us to go beyond the given data. For example, suppose it was found that there was an association between time spent on homework (1/2 hour to 3 hours) and the number of G.C.S.E. passes (1 to 6).
It would not be legitimate to infer from this that spending 6 hours on homework would likely generate 12 G.C.S.E. passes.
How do you know if a study is correlational?
A study is considered correlational if it examines the relationship between two or more variables without manipulating them. In other words, the study does not involve the manipulation of an independent variable to see how it affects a dependent variable.
One way to identify a correlational study is to look for language that suggests a relationship between variables rather than cause and effect.
For example, the study may use phrases like “associated with,” “related to,” or “predicts” when describing the variables being studied.
Another way to identify a correlational study is to look for information about how the variables were measured. Correlational studies typically involve measuring variables using self-report surveys, questionnaires, or other measures of naturally occurring behavior.
Finally, a correlational study may include statistical analyses such as correlation coefficients or regression analyses to examine the strength and direction of the relationship between variables.
Why is a correlational study used?
Correlational studies are particularly useful when it is not possible or ethical to manipulate one of the variables.
For example, it would not be ethical to manipulate someone’s age or gender. However, researchers may still want to understand how these variables relate to outcomes such as health or behavior.
Additionally, correlational studies can be used to generate hypotheses and guide further research.
If a correlational study finds a significant relationship between two variables, this can suggest a possible causal relationship that can be further explored in future research.
What is the goal of correlational research?
The ultimate goal of correlational research is to increase our understanding of how different variables are related and to identify patterns in those relationships.
This information can then be used to generate hypotheses and guide further research aimed at establishing causality.

Have a language expert improve your writing
Run a free plagiarism check in 10 minutes, generate accurate citations for free.
- Knowledge Base
Methodology
- Correlation vs. Causation | Difference, Designs & Examples
Correlation vs. Causation | Difference, Designs & Examples
Published on July 12, 2021 by Pritha Bhandari . Revised on June 22, 2023.
Correlation means there is a statistical association between variables. Causation means that a change in one variable causes a change in another variable.
In research, you might have come across the phrase “correlation doesn’t imply causation.” Correlation and causation are two related ideas, but understanding their differences will help you critically evaluate sources and interpret scientific research.
Table of contents
What’s the difference, why doesn’t correlation mean causation, correlational research, third variable problem, regression to the mean, spurious correlations, directionality problem, causal research, other interesting articles, frequently asked questions about correlation and causation.
Correlation describes an association between types of variables : when one variable changes, so does the other. A correlation is a statistical indicator of the relationship between variables. These variables change together: they covary. But this covariation isn’t necessarily due to a direct or indirect causal link.
Causation means that changes in one variable brings about changes in the other; there is a cause-and-effect relationship between variables. The two variables are correlated with each other and there is also a causal link between them.
Receive feedback on language, structure, and formatting
Professional editors proofread and edit your paper by focusing on:
- Academic style
- Vague sentences
- Style consistency
See an example
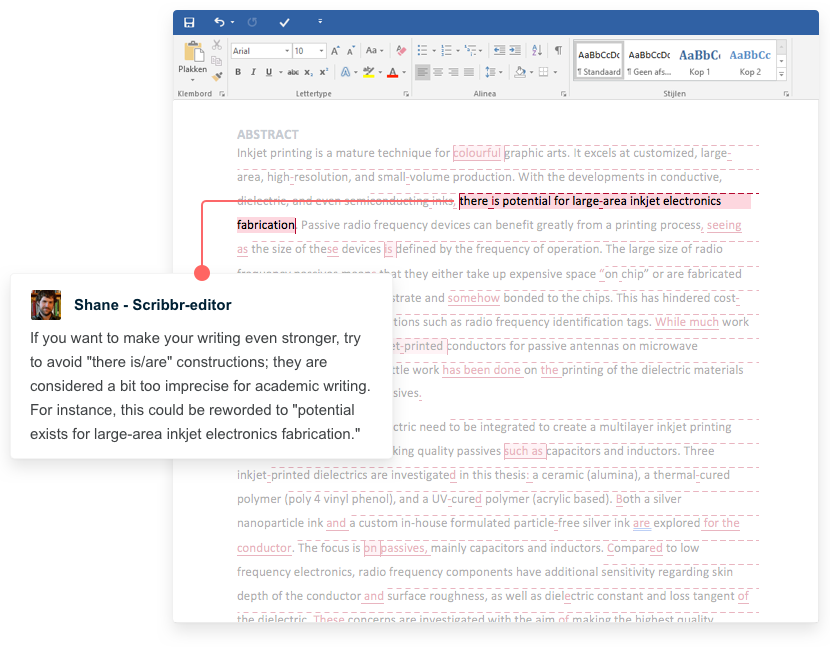
There are two main reasons why correlation isn’t causation. These problems are important to identify for drawing sound scientific conclusions from research.
The third variable problem means that a confounding variable affects both variables to make them seem causally related when they are not. For example, ice cream sales and violent crime rates are closely correlated, but they are not causally linked with each other. Instead, hot temperatures, a third variable, affects both variables separately. Failing to account for third variables can lead research biases to creep into your work.
The directionality problem occurs when two variables correlate and might actually have a causal relationship, but it’s impossible to conclude which variable causes changes in the other. For example, vitamin D levels are correlated with depression, but it’s not clear whether low vitamin D causes depression, or whether depression causes reduced vitamin D intake.
You’ll need to use an appropriate research design to distinguish between correlational and causal relationships:
- Correlational research designs can only demonstrate correlational links between variables.
- Experimental designs can test causation.
In a correlational research design, you collect data on your variables without manipulating them.
Correlational research is usually high in external validity , so you can generalize your findings to real life settings. But these studies are low in internal validity , which makes it difficult to causally connect changes in one variable to changes in the other.
These research designs are commonly used when it’s unethical, too costly, or too difficult to perform controlled experiments. They are also used to study relationships that aren’t expected to be causal.
Without controlled experiments, it’s hard to say whether it was the variable you’re interested in that caused changes in another variable. Extraneous variables are any third variable or omitted variable other than your variables of interest that could affect your results.
Limited control in correlational research means that extraneous or confounding variables serve as alternative explanations for the results. Confounding variables can make it seem as though a correlational relationship is causal when it isn’t.
When two variables are correlated, all you can say is that changes in one variable occur alongside changes in the other.
Here's why students love Scribbr's proofreading services
Discover proofreading & editing
Regression to the mean is observed when variables that are extremely higher or extremely lower than average on the first measurement move closer to the average on the second measurement. Particularly in research that intentionally focuses on the most extreme cases or events, RTM should always be considered as a possible cause of an observed change.
Players or teams featured on the cover of SI have earned their place by performing exceptionally well. But athletic success is a mix of skill and luck, and even the best players don’t always win.
Chances are that good luck will not continue indefinitely, and neither can exceptional success.
A spurious correlation is when two variables appear to be related through hidden third variables or simply by coincidence.
The Theory of the Stork draws a simple causal link between the variables to argue that storks physically deliver babies. This satirical study shows why you can’t conclude causation from correlational research alone.
When you analyze correlations in a large dataset with many variables, the chances of finding at least one statistically significant result are high. In this case, you’re more likely to make a type I error . This means erroneously concluding there is a true correlation between variables in the population based on skewed sample data.
To demonstrate causation, you need to show a directional relationship with no alternative explanations. This relationship can be unidirectional, with one variable impacting the other, or bidirectional, where both variables impact each other.
A correlational design won’t be able to distinguish between any of these possibilities, but an experimental design can test each possible direction, one at a time.
- Physical activity may affect self esteem
- Self esteem may affect physical activity
- Physical activity and self esteem may both affect each other
In correlational research, the directionality of a relationship is unclear because there is limited researcher control. You might risk concluding reverse causality, the wrong direction of the relationship.
Causal links between variables can only be truly demonstrated with controlled experiments . Experiments test formal predictions, called hypotheses , to establish causality in one direction at a time.
Experiments are high in internal validity , so cause-and-effect relationships can be demonstrated with reasonable confidence.
You can establish directionality in one direction because you manipulate an independent variable before measuring the change in a dependent variable.
In a controlled experiment, you can also eliminate the influence of third variables by using random assignment and control groups.
Random assignment helps distribute participant characteristics evenly between groups so that they’re similar and comparable. A control group lets you compare the experimental manipulation to a similar treatment or no treatment (or a placebo, to control for the placebo effect ).
If you want to know more about statistics , methodology , or research bias , make sure to check out some of our other articles with explanations and examples.
- Chi square test of independence
- Statistical power
- Descriptive statistics
- Degrees of freedom
- Pearson correlation
- Null hypothesis
- Double-blind study
- Case-control study
- Research ethics
- Data collection
- Hypothesis testing
- Structured interviews
Research bias
- Hawthorne effect
- Unconscious bias
- Recall bias
- Halo effect
- Self-serving bias
- Information bias
A correlation reflects the strength and/or direction of the association between two or more variables.
- A positive correlation means that both variables change in the same direction.
- A negative correlation means that the variables change in opposite directions.
- A zero correlation means there’s no relationship between the variables.
Correlation describes an association between variables : when one variable changes, so does the other. A correlation is a statistical indicator of the relationship between variables.
Causation means that changes in one variable brings about changes in the other (i.e., there is a cause-and-effect relationship between variables). The two variables are correlated with each other, and there’s also a causal link between them.
While causation and correlation can exist simultaneously, correlation does not imply causation. In other words, correlation is simply a relationship where A relates to B—but A doesn’t necessarily cause B to happen (or vice versa). Mistaking correlation for causation is a common error and can lead to false cause fallacy .
The third variable and directionality problems are two main reasons why correlation isn’t causation .
The third variable problem means that a confounding variable affects both variables to make them seem causally related when they are not.
The directionality problem is when two variables correlate and might actually have a causal relationship, but it’s impossible to conclude which variable causes changes in the other.
Controlled experiments establish causality, whereas correlational studies only show associations between variables.
- In an experimental design , you manipulate an independent variable and measure its effect on a dependent variable. Other variables are controlled so they can’t impact the results.
- In a correlational design , you measure variables without manipulating any of them. You can test whether your variables change together, but you can’t be sure that one variable caused a change in another.
In general, correlational research is high in external validity while experimental research is high in internal validity .
Cite this Scribbr article
If you want to cite this source, you can copy and paste the citation or click the “Cite this Scribbr article” button to automatically add the citation to our free Citation Generator.
Bhandari, P. (2023, June 22). Correlation vs. Causation | Difference, Designs & Examples. Scribbr. Retrieved July 2, 2024, from https://www.scribbr.com/methodology/correlation-vs-causation/
Is this article helpful?
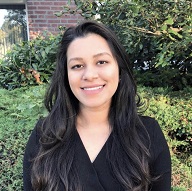
Pritha Bhandari
Other students also liked, correlational research | when & how to use, guide to experimental design | overview, steps, & examples, confounding variables | definition, examples & controls, what is your plagiarism score.
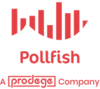
- Pollfish School
- Market Research
- Survey Guides
- Get started
- What is Correlational Research and How to Conduct it for Business Success
What is Correlational Research and How Do You Conduct it for Business Success
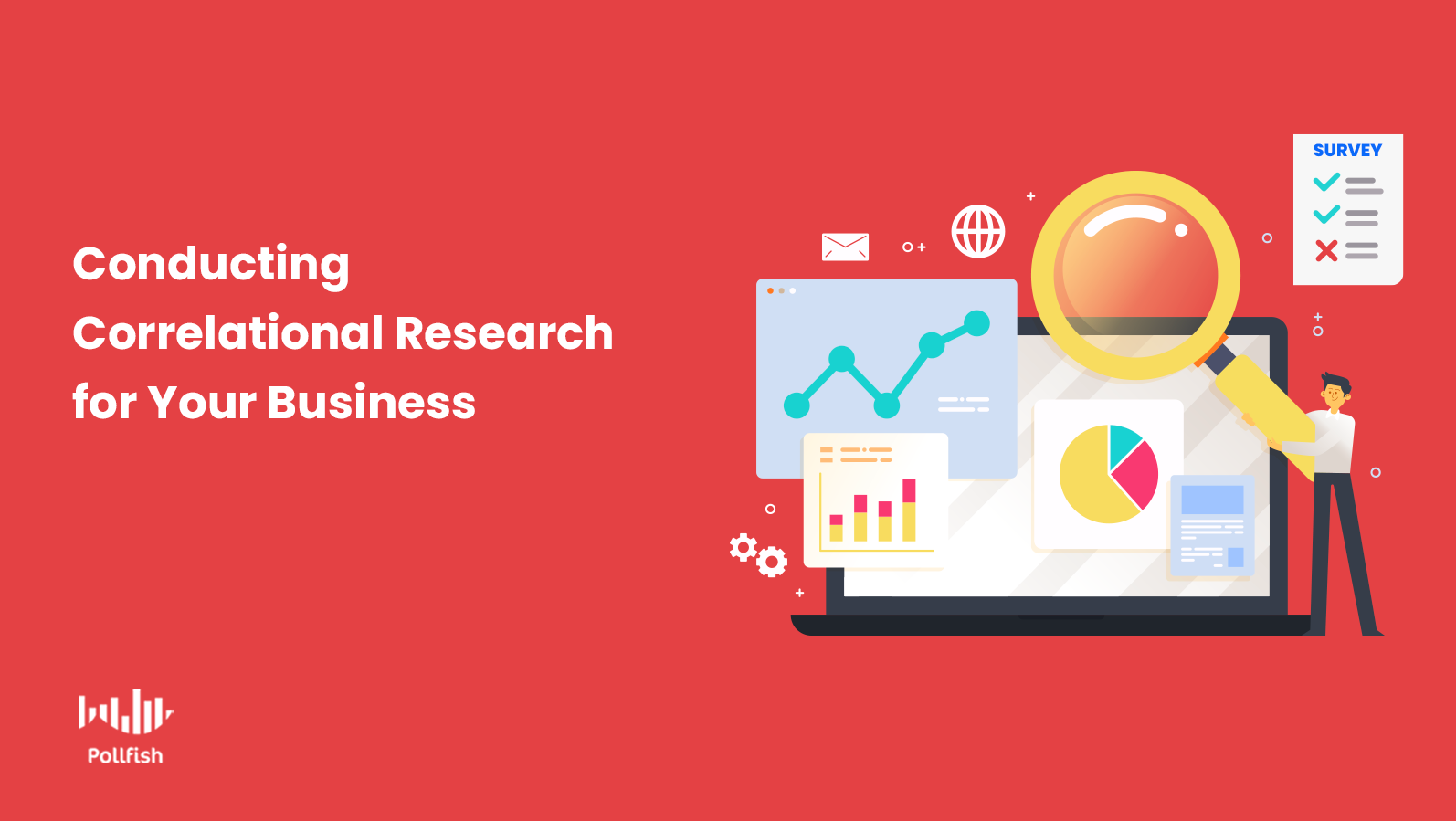
Correlational research is a critical form of research that researchers ought to deploy in the mid-late stages of the entire research process. It is especially necessary if it concerns a new topic.
Sequentially following descriptive research , which follows exploratory research , correlational research studies the relationship between two variables. This is important when attempting to make sense out of the variables discovered from exploratory and descriptive research.
Although correlation does not equal causation, understanding the correlation between two variables is crucial to see how similar such relationships may occur and how they behave within an existing occurrence, problem or phenomenon.
This article explains correlational research in-depth, including why it is important to conduct for business research, how to conduct it and more.
Defining Correlational Research
One of the chief forms of research, correlational research depicts and explains the relationship between two variables. This form of research is non-experimental, thus the researcher exerts little to no efforts to control or manipulate the variables .
The variables studied in this kind of research can be either quantitative or categorical.
Correlational research aims to find unknown or suspected relationships between variables, as this can point to similar behaviors or occurrences in other variables. If not, this kind of research is still powerful, as it paints a clearer picture of an issue or phenomenon that had been discovered in exploratory or descriptive research.
In correlational research, researchers measure the relationship between two variables, without controlling either one. The point of this kind of study is to discover:
- Positive correlation : Occurs when variables exhibit changes in the same way. Ex: As purchases increase, store traffic increases.
- Negative correlation : Describes variables that change in contrasting ways. Ex: Increase in employee burnout and a decrease in production.
- Zero correlation: When there is no relationship between the variables. Ex: Seeing employees in the office does not increase signed contracts with B2B partners.
The Key Aspects of Correlational Research
This form of research has several defining characteristics that researchers ought to consider. These aspects help identify this form of research, helping them use it for the future or to contrast it with causal or experimental research.
The following enumerates the key features of this research:
- As nonexperimental research , researchers do not need to manipulate variables to either agree or disagree with a hypothesis.
- It involves measuring and observing the relationship between the variables, with no external conditioning or alterations of any kind.
- It incorporates retrospective elements, as it looks back at past events and data of the variables, as a means to find and calculate historical patterns between them.
- It is conducted when there is some prior knowledge present from either exploratory or descriptive research so that at least one of the factors will relate to the predicted outcome.
- Although it may show a certain kind of correlation between variables, e.g. a positive one, it may not remain as such, as it can change in the future .
- Variables can be observed in a closed environment or via field research, i.e., a public space.
- It is used when the variables are too complex to be studied via the experimental method and controlled manipulation.
- It simultaneously measures variables and their relationships in realistic settings.
- It is executed when a relationship is suspected to be causal , but the researcher can’t manipulate the independent variable due to difficulty, impossibility or legal constraints.
- It is applied when one or a set of objectives requires gathering some degree of prediction.
Why Your Business Needs Correlational Research
Businesses benefit from conducting correlational research in a number of ways. Firstly, this research zeros in on previously murky or uncovered aspects from the entire research process, that of relationships between variables .
This research can c onfirm or refute these relationships, the hypothesis of their existence , along with the hypothesis of how they relate to the subject matter at large .
Secondly, when a business forms a clear understanding of the relationships between certain variables — whether they are good, bad or nonexistent, it is effectively equipped with the knowledge on how to move forward. For example, a correlational study may require further correlational research, causal research or experimental research.
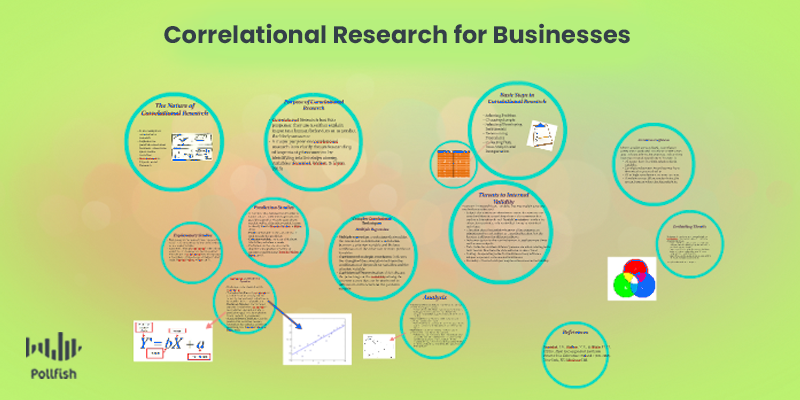
Or, it may require fewer studies while presenting actionable insights on how to proceed with the studied variables and their environments.
Moreover, it helps researchers understand their studied variables in relation to the past, as it compares present behaviors and other attributes of the variables with retrospective ones. Piggybacking off of this concept, correlational research also helps businesses form predictions .
These predictions pertain to other similar variables, or the same variables and can have a bearing on the near and later futures. Correlational predictions are particularly useful for detecting patterns.
Example of Correlational Research for Business
While there are many different verticals in the sciences and scientific offshoots (ex: psychology, medicine) that appear to require this research, it may not often be so clear as to how it can help businesses.
However, as expounded on above, correlational research is crucial for business and market research. Here is an example of how a business used correlational research in their overall research campaign:
A business noticed the reduction of sales when conducting exploratory research. They noticed that in correlational research, there were particular items that were especially plunging in sales.
Thus, they performed correlational research and discovered that there are two variables that may have a correlation: the rise in kitchen appliances and the decrease in sales of those appliances.
Accordingly, the business deployed surveys to their target market and loyal customers to understand whether in fact, there was a negative correlation present. The surveys proved that this was indeed the case. With this knowledge in tow, the business offered a discount on the appliances, also having considered lowering the prices after some time.
Correlational Research Survey Examples
- Helps understand variables in greater depth.
- Can explain variable behaviors in relation to their environment/context.
- Delves into past events, occurrences and attitudes in regards to the variables.
- Shows whether the variables changed and how so.
- Can find associations between variables over a period of time.
- Useful for studying variables to form predictions and understand outcomes.
- Great for comparing loyal customers against those who don’t buy from you but are in your target market.
- Measures the variable of continuous purchases.
- Measures relationships between employee satisfaction and workplace or company policy occurrences.
- Helps compare happy and dissatisfied employees.
How Correlational Research Differs from Causal, Exploratory and Experimental Research
Correlational research differs significantly from the other main types of research methods such as exploratory, descriptive and experimental research.
However, causal research is often conflated with causal research . While both kinds of research concern studying the relationships between variables and are conducted in the mid-late stages of research, they study very different aspects. As such, they follow different approaches and measure different things.
Whereas correlational research simply aims to understand how two variables relate to one another, causal research attempts to find a cause and effect relationship between two variables. This research type is more involved in making comparisons.
Exploratory research stands in complete contrast with correlational research, as it provides the very basis of a research problem and forms a hypothesis for later research, without involving variables.
Descriptive research delves into describing something already established, discovered or suspected in exploratory research . Therefore, it is composed after exploratory research in the overall process. It is still an early part of the entire research process.
Unlike correlational research, it attempts to describe what exploratory research had already established in greater detail, with new aspects emerging. It is not concerned with examining variables.
Experimental research contrasts with correlational research, in that it i nvolves manipulating and controlling variables , whereas correlational research exerts absolutely no control or alterations to the studied variables.
As its name suggests, experimental research forms experiments on the variables as a means to discover cause-and-effect relationships. Using a scientific research design, this research involves a controlled environment, one in which the variables can be measured, calculated and then compared.
The Advantages and Disadvantages of Correlational Research
Correlational research offers several benefits for researchers and businesses. However, as with all other research methods, there are a few setbacks to this type of research.
The Advantages
- Allows researchers to determine the statistical relationship between two variables.
- An inexpensive and effective (not time-consuming) research method.
- Grants the research to understand a variable unaltered, existing in its natural state to fully understand it.
- Variables in their natural state are more applicable to everyday situations.
- Although it can’t prove causation, a large amount of collected and analyzed correlational data can support a causal hypothesis .
- Enables researchers to understand the duration and strength of the relationship.
- Using the correlation coefficient to measure a relationship’s strength (ranging from 1.00 to -1.00), the results are easy to classify.
- Provides the most ideal transition to conducting causal or experimental research.
- Provides insight into the way variables operate and exist with each other that other methods cannot find.
- Can occur through quick research such as via surveys or observations.
The Disadvantages
- It is limited, as it can only glean the statistical information from no more than two variables.
- Does not take cause and effect relationships into account, as it doesn’t find which of the 2 variables creates the statistical pattern.
- Does not show which variable wields the most influence.
- Cannot be fully depended on for further research, as it employs past statistical patterns to determine the relationship.
How to Conduct Correlational Research
A comparatively simple and quick way to conduct research, correlational research can be conducted using field research, i.e., via natural observations, through surveys and via secondary research. Here is how to execute this research using all of these techniques.
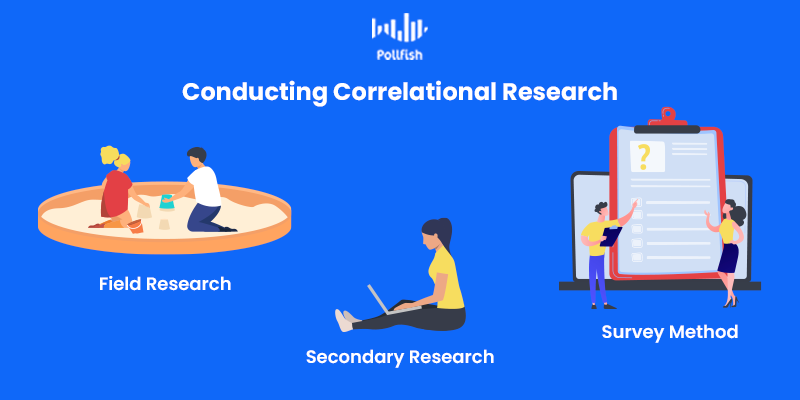
- Ask questions about the variables. Use both open-ended and close-ended questions. The former is better for understanding the variables at a greater depth.
- Then, statistically analyze the answers.
- Study customers and passersby in physical shops, use a session replay tool in online shops.
- Record behaviors as they relate to the variables.
- These depend on your vertical and subject of study.
- Put them together and compare them to your own findings.
Mastering Correlational Research
Correlational research, although in the mid-stages of research, forms the very onset of relationship research into variables. Like descriptive research, correlational research describes variables, going a step further, as it tracks how one relates with the other.
It is essential to conduct this research to understand the kinds of relationships that exist between two variables before performing any testing on them, whether it’s for comparisons in causal research, or for experimentation in experimental research.
A strong online survey tool makes this possible and practical to carry out. As aforementioned, there are various surveys relevant to use for correlational research. An adept online survey provider will make it feasible to implement all forms in your research, along with sending them to their intended sampling pool .
Do you want to distribute your survey? Pollfish offers you access to millions of targeted consumers to get survey responses from $0.95 per complete. Launch your survey today.
Privacy Preference Center
Privacy preferences.
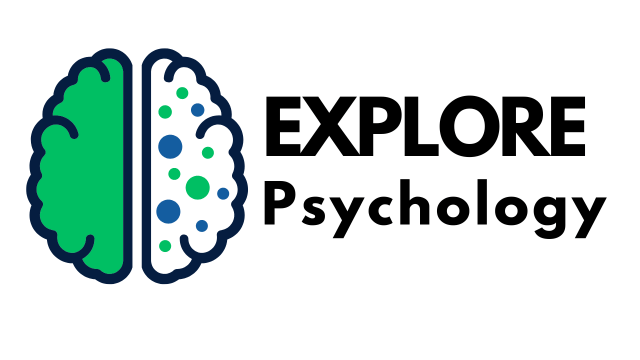
Correlational Research in Psychology: Definition and How It Works
Categories Research Methods
Correlational research is a type of scientific investigation in which a researcher looks at the relationships between variables but does not vary, manipulate, or control them. It can be a useful research method for evaluating the direction and strength of the relationship between two or more different variables.
When examining how variables are related to one another, researchers may find that the relationship is positive or negative. Or they may also find that there is no relationship at all.
Table of Contents
How Does Correlational Research Work?
In correlational research, the researcher measures the values of the variables of interest and calculates a correlation coefficient, which quantifies the strength and direction of the relationship between the variables.
The correlation coefficient ranges from -1.0 to +1.0, where -1.0 represents a perfect negative correlation, 0 represents no correlation, and +1.0 represents a perfect positive correlation.
A negative correlation indicates that as the value of one variable increases, the value of the other variable decreases, while a positive correlation indicates that as the value of one variable increases, the value of the other variable also increases. A zero correlation indicates that there is no relationship between the variables.
The variables both increase together | The more you walk on a treadmill, the more calories you burn. | |
The variables decrease together | The less you study, the lower your grades will be. | |
No relationship exists between the variables | How much you walk on a treadmill is not associated with grades on exams. |
Correlational Research vs. Experimental Research
Correlational research differs from experimental research in that it does not involve manipulating variables. Instead, it focuses on analyzing the relationship between two or more variables.
In other words, correlational research seeks to determine whether there is a relationship between two variables and, if so, the nature of that relationship.
Experimental research, on the other hand, involves manipulating one or more variables to determine the effect on another variable. Because of this manipulation and control of variables, experimental research allows for causal conclusions to be drawn, while correlational research does not.
Both types of research are important in understanding the world around us, but they serve different purposes and are used in different situations.
Utilized to assess the strength and direction of the relationship between variables | Utilized to look for cause-and-effect relationships between variables |
Involves measuring but not manipulating variables | Involves manipulating an independent variable and measuring the effect on the dependent variable |
Results may be influenced by other variables that the researcher cannot control | Researchers are better able to control extraneous variables that might impact results |
Types of Correlational Research
There are three main types of correlational studies:
Cohort Correlational Study
This type of study involves following a cohort of participants over a period of time. This type of research can be useful for understanding how certain events might influence outcomes.
For example, researchers might study how exposure to a traumatic natural disaster influences the mental health of a group of people over time.
By examining the data collected from these individuals, researchers can determine whether there is a correlation between the two variables under investigation. This information can be used to develop strategies for preventing or treating certain conditions or illnesses.
Cross-Sectional Correlational Study
A cross-sectional design is a research method that examines a group of individuals at a single time. This type of study collects information from a diverse group of people, usually from different backgrounds and age groups, to gain insight into a particular phenomenon or issue.
The data collected from this type of study is used to analyze relationships between variables and identify patterns and trends within the group.
Cross-sectional studies can help identify potential risk factors for certain conditions or illnesses, and can also be used to evaluate the prevalence of certain behaviors, attitudes, or beliefs within a population.
Case-Control Correlational Study
A case-control correlational study is a type of research design that investigates the relationship between exposure and health outcomes. In this study, researchers identify a group of individuals with the health outcome of interest (cases) and another group of individuals without the health outcome (controls).
The researchers then compare the exposure history of the cases and controls to determine whether the exposure and health outcome correlate.
This type of study design is often used in epidemiology and can provide valuable information about potential risk factors for a particular disease or condition.
When to Use Correlational Research
There are a number of situations where researchers might opt to use a correlational study instead of some other research design.
Correlational research can be used to investigate a wide range of psychological phenomena, including the relationship between personality traits and academic performance, the association between sleep duration and mental health, and the correlation between parental involvement and child outcomes.
To Generate Hypotheses
Correlational research can also be used to generate hypotheses for further research by identifying variables that are associated with each other.
To Investigate Variables Without Manipulating Them
Researchers should use correlational research when they want to investigate the relationship between two variables without manipulating them. This type of research is useful when the researcher cannot or should not manipulate one of the variables or when it is impossible to conduct an experiment due to ethical or practical concerns.
To Identify Patterns
Correlational research allows researchers to identify patterns and relationships between variables, which can inform future research and help to develop theories. However, it is important to note that correlational research does not prove that one variable causes changes in the other.
While correlational research has its limitations, it is still a valuable tool for researchers in many fields, including psychology, sociology, and education.
How to Collect Data in Correlational Research
Researchers can collect data for correlational research in a few different ways. To conduct correlational research, data can be collected using the following:
- Surveys : One method is through surveys, where participants are asked to self-report their behaviors or attitudes. This approach allows researchers to gather large amounts of data quickly and affordably.
- Naturalistic observation : Another method is through observation, where researchers observe and record behaviors in a natural or controlled setting. This method allows researchers to learn more about the behavior in question and better generalize the results to real-world settings.
- Archival, retrospective data : Additionally, researchers can collect data from archival sources, such as medical, school records, official records, or past polls.
The key is to collect data from a large and representative sample to measure the relationship between two variables accurately.
Pros and Cons of Correlational Research
There are some advantages of using correlational research, but there are also some downsides to consider.
- One of the strengths of correlational research is its ability to identify patterns and relationships between variables that may be difficult or unethical to manipulate in an experimental study.
- Correlational research can also be used to examine variables that are not under the control of the researcher , such as age, gender, or socioeconomic status.
- Correlational research can be used to make predictions about future behavior or outcomes, which can be valuable in a variety of fields.
- Correlational research can be conducted quickly and inexpensively , making it a practical option for researchers with limited resources.
- Correlational research is limited by its inability to establish causality between variables. Correlation does not imply causation, and it is possible that a third variable may be influencing both variables of interest, creating a spurious correlation. Therefore, it is important for researchers to use multiple methods of data collection and to be cautious when interpreting correlational findings.
- Correlational research relies heavily on self-reported data , which can be biased or inaccurate.
- Correlational research is limited in its ability to generalize findings to larger populations, as it only measures the relationship between two variables in a specific sample.
Frequently Asked Questions About Correlational Research
What are the main problems with correlational research.
Some of the main problems that can occur in correlational research include selection bias, confounding variables. and misclassification.
- Selecting participants based on their exposure to an event means that the sample might be biased since the selection was not randomized.
- Correlational studies may also be impacted by extraneous factors that researchers cannot control.
- Finally, there may be problems with how accurately data is recorded and classified, which can be particularly problematic in retrospective studies.
What are the variables in a correlational study?
In a correlational study, variables refer to any measurable factors being examined for their potential relationship or association with each other. These variables can be continuous (meaning they can take on a range of values) or categorical (meaning they fall into distinct categories or groups).
For example, in a study examining the correlation between exercise and mental health, the independent variable would be exercise frequency (measured in times per week), while the dependent variable would be mental health (measured using a standardized questionnaire).
What is the goal of correlational research?
The goal of correlational research is to examine the relationship between two or more variables. It involves analyzing data to determine if there is a statistically significant connection between the variables being studied.
Correlational research is useful for identifying patterns and making predictions but cannot establish causation. Instead, it helps researchers to better understand the nature of the relationship between variables and to generate hypotheses for further investigation.
How do you identify correlational research?
To identify correlational research, look for studies that measure two or more variables and analyze their relationship using statistical techniques. The results of correlational studies are typically presented in the form of correlation coefficients or scatterplots, which visually represent the degree of association between the variables being studied.
Correlational research can be useful for identifying potential causal relationships between variables but cannot establish causation on its own.
Curtis EA, Comiskey C, Dempsey O. Importance and use of correlational research . Nurse Researcher . 2016;23(6):20-25. doi10.7748/nr.2016.e1382
Lau F. Chapter 12 Methods for Correlational Studies . University of Victoria; 2017.
Mitchell TR. An evaluation of the validity of correlational research conducted in organizations . The Academy of Management Review . 1985;10(2):192. doi:10.5465/amr.1985.4277939
Seeram E. An overview of correlational research . Radiol Technol . 2019;91(2):176-179.
- Skip to main content
- Skip to primary sidebar
- Skip to footer
- QuestionPro

- Solutions Industries Gaming Automotive Sports and events Education Government Travel & Hospitality Financial Services Healthcare Cannabis Technology Use Case NPS+ Communities Audience Contactless surveys Mobile LivePolls Member Experience GDPR Positive People Science 360 Feedback Surveys
- Resources Blog eBooks Survey Templates Case Studies Training Help center

Home Market Research
Zero Correlation: Definition, Examples + How to Determine It
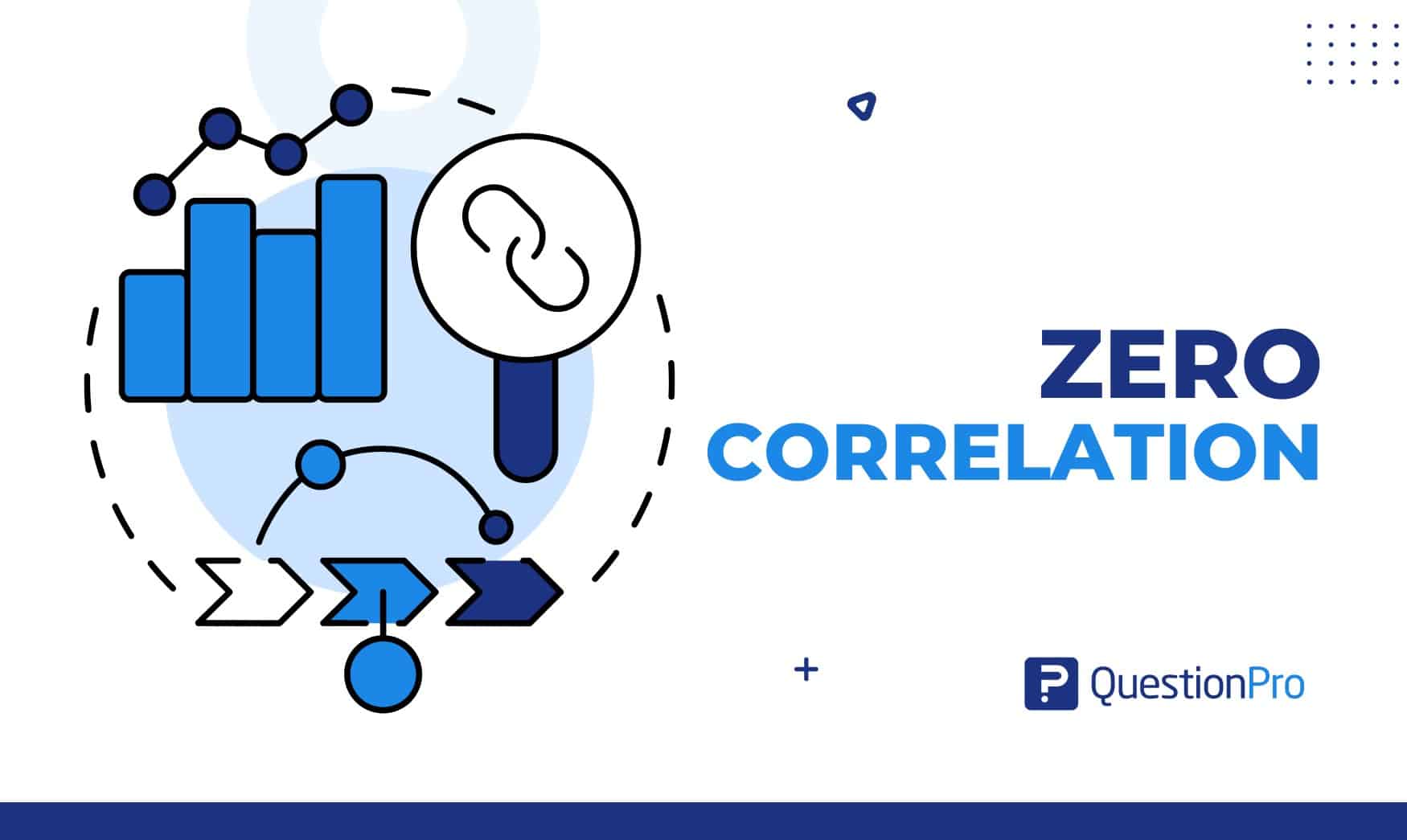
Correlation is a fundamental concept in statistics and data analysis, helping to understand the relationship between two variables. While strong positive or negative correlations are often highlighted, zero correlation is equally important.
It means there is no linear relationship between the variables. In other words, changes in one variable do not predict changes in the other.
In this blog, we will explore the concept of zero correlation, providing a clear definition, illustrative examples, and methods to determine it.
What is a Zero Correlation?
Zero correlation is a statistical term that describes a situation where there is no linear relationship between two variables. When two variables have zero correlation, changes in one variable do not predict changes in the other. The correlation coefficient, which measures the degree and direction of the relationship between variables, is exactly zero in this case.
Understanding this correlation is important in statistical analysis because it helps identify variables that do not have a predictive relationship with each other, which is crucial when building statistical models or interpreting data patterns.
Why is Zero Correlation important?
Zero correlation is an important concept in statistics and data analysis for several reasons such as:
It Identifies Independence
It helps identify variables that are linearly independent of each other. If two variables have zero correlation, changes in one variable do not provide any information about changes in the other. This is crucial for understanding the structure of the data and the relationships (or lack thereof) between variables.
It Improves Statistical Models
In regression analysis and other statistical models, including variables with this correlation to the dependent variable can add noise and reduce the model’s predictive power. By identifying and excluding such variables, models can be simplified and made more efficient, leading to better performance and interpretability.
This Correlation Helps Avoiding Misinterpretation
Understanding this correlation prevents misinterpretation of data.
- For example, a researcher might mistakenly infer a relationship between two variables based on intuition or initial observations.
Calculating the correlation coefficient and finding it to be zero clarifies that no linear relationship exists, avoiding false conclusions.
It Highlights Non-linear Relationships
It highlights the possibility of non-linear relationships. If two variables have zero correlation, it doesn’t necessarily mean they are unrelated; they might have a complex, non-linear relationship. Recognizing this can prompt further investigation using other methods, such as non-linear regression or data transformations.
Correlation Helps in Guiding Experimental Design
In experimental design, knowing which variables have zero correlation can guide the selection of variables to include or control for. This helps in designing more robust experiments where the influence of irrelevant variables is minimized, leading to clearer, more reliable results.
It Understands Variable Behavior
It provides insights into the behavior of variables in a dataset. In financial analysis, understanding which assets have zero correlation with each other can help in portfolio diversification, as combining such assets can reduce overall risk.
It Supports Hypothesis Testing
In hypothesis testing, this correlation is often a null hypothesis.
- For example, in testing whether two variables are related, the null hypothesis might state that the correlation between them is zero.
Establishing whether this is true or false helps in validating or refuting hypotheses.
What are the Examples of Zero Correlation?
Examples of this correlation, where changes in one variable do not correspond with changes in another variable, can be found across various fields such as:
Field of Research
Example: Number of Scientific Publications and Favorite Ice Cream Flavor
A study investigates the relationship between the number of scientific publications a researcher has and their favorite ice cream flavor.
There is no logical connection between the number of scientific papers a researcher publishes and their preference for a particular ice cream flavor. As a result, these two variables are expected to exhibit this correlation.
Field of Education
Example: Students’ Shoe Size and Their Grades in Mathematics
An educational study examines whether there is any relationship between students’ shoe sizes and their grades in mathematics.
Shoe size is a physical characteristic that has no bearing on a student’s academic performance in mathematics. Therefore, the correlation between shoe size and math grades is likely to be zero.
Field of Healthcare
Example: Blood Type and Incidence of the Common Cold
A healthcare study looks into whether there is a relationship between a person’s blood type and the number of times they catch the common cold in a year.
Blood type is not associated with the frequency of contracting the common cold, which is influenced by various other factors such as exposure to viruses and immune system strength. Hence, the correlation between blood type and the incidence of the common cold is expected to be zero.
How to Identify Zero Correlation?
Here, we’ll explore how to identify this correlation through visual inspection, statistical calculation, hypothesis testing, and contextual analysis.
1. Visual The Inspection Using Scatter Plots
Scatter plots are an effective tool for visually assessing the relationship between two variables.
Create a Scatter Plot:
- Place one variable on the x-axis and the other on the y-axis.
- Look for any discernible trend or pattern in the data points.
Identifying Correlation:
- If the points are scattered randomly with no clear trend (neither upward nor downward), it suggests correlation.
- A random scatter implies that no line (whether straight or curved) can fit the data points well.
- Students’ Shoe Sizes vs. Math Grades: If you plot shoe sizes against math grades and see a random scatter of points with no trend, this indicates zero correlation.
2. Calculate the Correlation Coefficient
The Pearson correlation coefficient (r) is the most common measure of linear correlation.
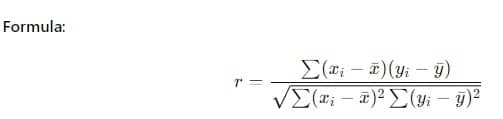
- Gather paired data points for the two variables.
- Find the mean (average) of each variable.
- Calculate how far each data point is from the mean.
- Multiply the deviations for each pair and sum the products.
- Use the formula to find the correlation coefficient.
Interpreting Correlation:
Value Close to 0: If 𝑟 r is close to 0, it indicates little to no linear relationship between the variables.
- Shoe Sizes and Math Grades: If the calculated 𝑟 is approximately 0, it confirms zero correlation.
3. Do The Hypothesis Testing
Statistical hypothesis testing can determine whether an observed correlation coefficient is significantly different from zero.
- Null Hypothesis: Assume that the correlation coefficient is zero.
- Alternative Hypothesis: Assume that the correlation coefficient is not zero.
- Compute Test Statistic: Use a t-test for the correlation coefficient.
- Determine p-value: Compare the p-value to a significance level (e.g., 0.05).
Zero Correlation:
- If the p-value is greater than the significance level, do not reject the null hypothesis, suggesting that the correlation is not significantly different from zero.
- Blood Type and Common Cold Incidence: Testing the correlation between blood type and the incidence of the common cold, if the p-value is high, it indicates that any observed correlation is not statistically significant, supporting zero correlation.
4. Understanding Contextual Analysis
Understanding the context and theoretical background of the variables is essential for interpreting correlation results.
- Examine Variables: Consider the nature and expected relationships between the variables.
- Apply Domain Knowledge: Use knowledge from the field to hypothesize whether a relationship is expected.
Zero Correlation:
- If theory and prior research suggest no logical relationship, this supports the finding of this correlation.
- Blood Type and Common Cold Incidence: Knowing that blood type does not affect susceptibility to the common cold supports the interpretation of zero correlation if found.
Negative vs Positive Correlation vs Zero Correlation
Correlation is a statistical measure that describes the strength and direction of the relationship between two variables. Here’s a detailed explanation of negative, positive, and zero correlation:
Positive Correlation
- Definition: A positive correlation occurs when two variables move in the same direction. As one variable increases, the other variable also increases, and as one decreases, the other also decreases.
- Example: The relationship between height and weight. Generally, as a person’s height increases, their weight also tends to increase.
- Graphical Representation: In a scatter plot, points tend to cluster around a line that slopes upwards from left to right.
Negative Correlation
- Definition: A negative correlation occurs when two variables move in opposite directions. As one variable increases, the other variable decreases, and vice versa.
- Example: The relationship between the amount of time spent studying and the number of errors made on a test. Generally, as the time spent studying increases, the number of errors decreases.
- Graphical Representation: In a scatter plot, points tend to cluster around a line that slopes downwards from left to right.
Zero Correlation
- Definition: It indicates that there is no relationship between the two variables. Changes in one variable do not predict changes in the other variable.
- Example: The relationship between a person’s shoe size and their intelligence quotient (IQ). There is no logical connection between these two variables.
- Graphical Representation: In a scatter plot, points are distributed randomly with no discernible pattern or slope.
How QuestionPro Can Help in Correlation Analysis?
QuestionPro, a robust survey platform, offers comprehensive tools to facilitate correlation analysis effectively. Here’s how QuestionPro can help you in conducting correlation analysis:
Effortless Data Collection
QuestionPro simplifies the data collection process through its user-friendly survey creation tools. You can design and distribute surveys to gather quantitative data on various variables of interest. The platform supports various question types, allowing you to capture detailed and relevant data efficiently.
Automated Data Analysis
Once the data is collected, QuestionPro provides built-in analytics tools for correlation analysis. You can easily calculate correlations, which measure the strength and direction of the linear relationship between two variables. The linear correlation coefficient ranges from -1 to 1, where:
- 1 indicates a perfect positive correlation.
- -1 indicates a perfect negative correlation.
- 0 indicates no correlation.
Visual Representation
QuestionPro offers visualization tools to help you interpret the results of your correlation analysis. Scatter plots and correlation matrices can be generated to provide a clear graphical representation of the relationships between variables. This visual aid is crucial for quickly identifying trends and patterns.
Identifying Patterns and Trends
Using QuestionPro’s correlation analysis, researchers observed correlation ( positive, negative, or zero) between variables:
- Positive Correlation: Both variables move in the same direction. For example, increased advertising spending may correlate with increased sales.
- Negative Correlation: The variables tend to move in opposite directions. For example, increased screen time might correlate with decreased academic performance.
- Zero Correlation: No relationship exists between the variables. For example, the number of years in school might not correlate with the number of letters in a person’s name.
Practical Applications
Correlation analysis in QuestionPro can be used for various practical applications, such as:
- Market Research: Measure the effectiveness of marketing campaigns by correlating advertising spending with sales performance.
- Healthcare: Assess the relationship between medication usage and patient outcomes, such as blood pressure levels.
- Education: Determine the impact of study habits on academic performance by correlating hours studied with grades.
Zero correlation between two variables signifies the absence of a linear relationship, indicating that changes in one variable do not correspond with changes in another. By computing correlation coefficients and visualizing data through scatter plots, researchers can accurately determine whether variables are correlated, positively correlated, negatively correlated, or show this correlation.
Using QuestionPro for correlation analysis in your surveys provides a powerful way to uncover meaningful relationships between variables. By exploring QuestionPro’s intuitive interface, advanced analytical tools, and comprehensive reporting features, you can efficiently conduct correlation analysis and derive valuable insights from your data. Contact QuestionPro today for further information!
FREE TRIAL RICHIEDI DEMO
MORE LIKE THIS
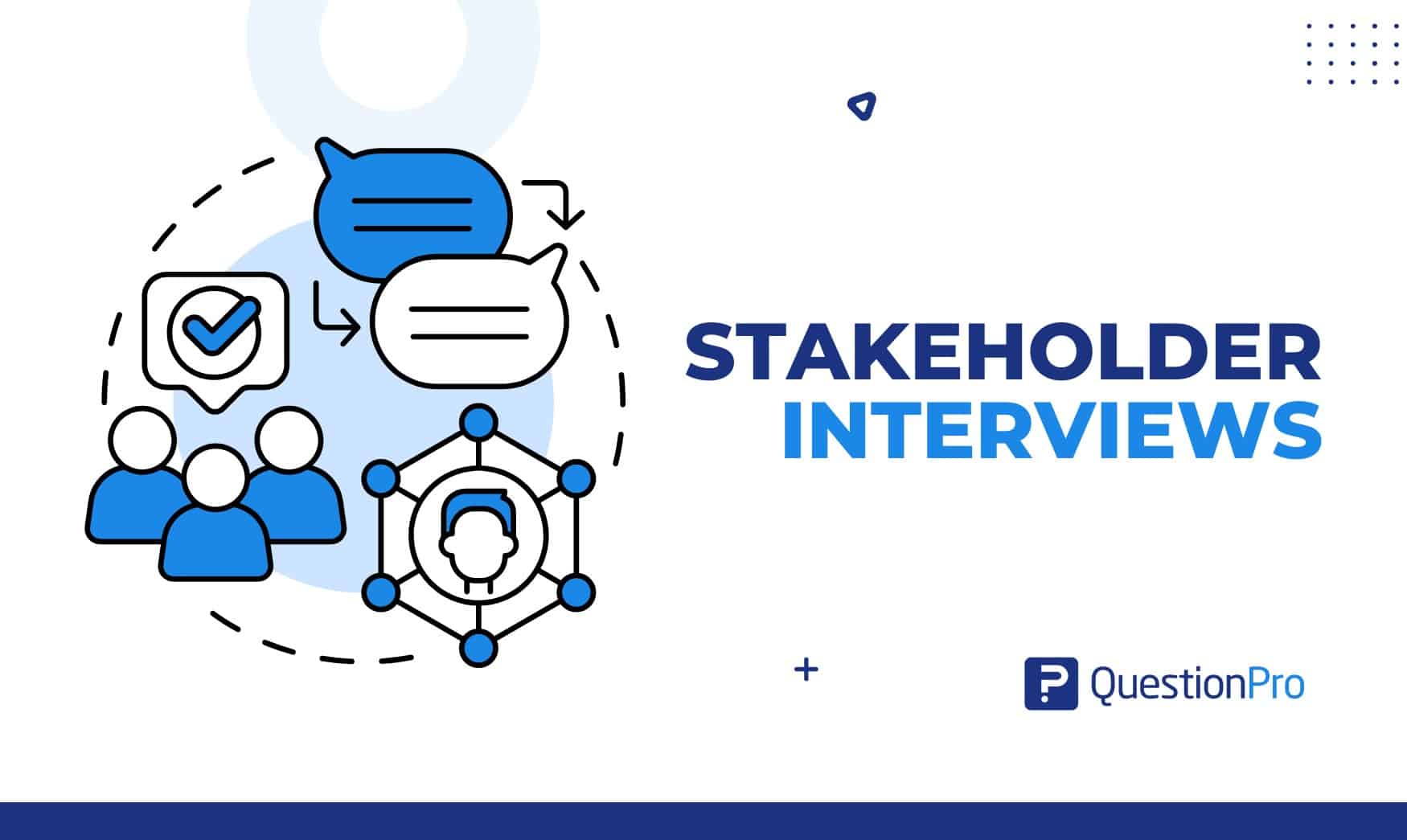
Stakeholder Interviews: A Guide to Effective Engagement
Jul 2, 2024
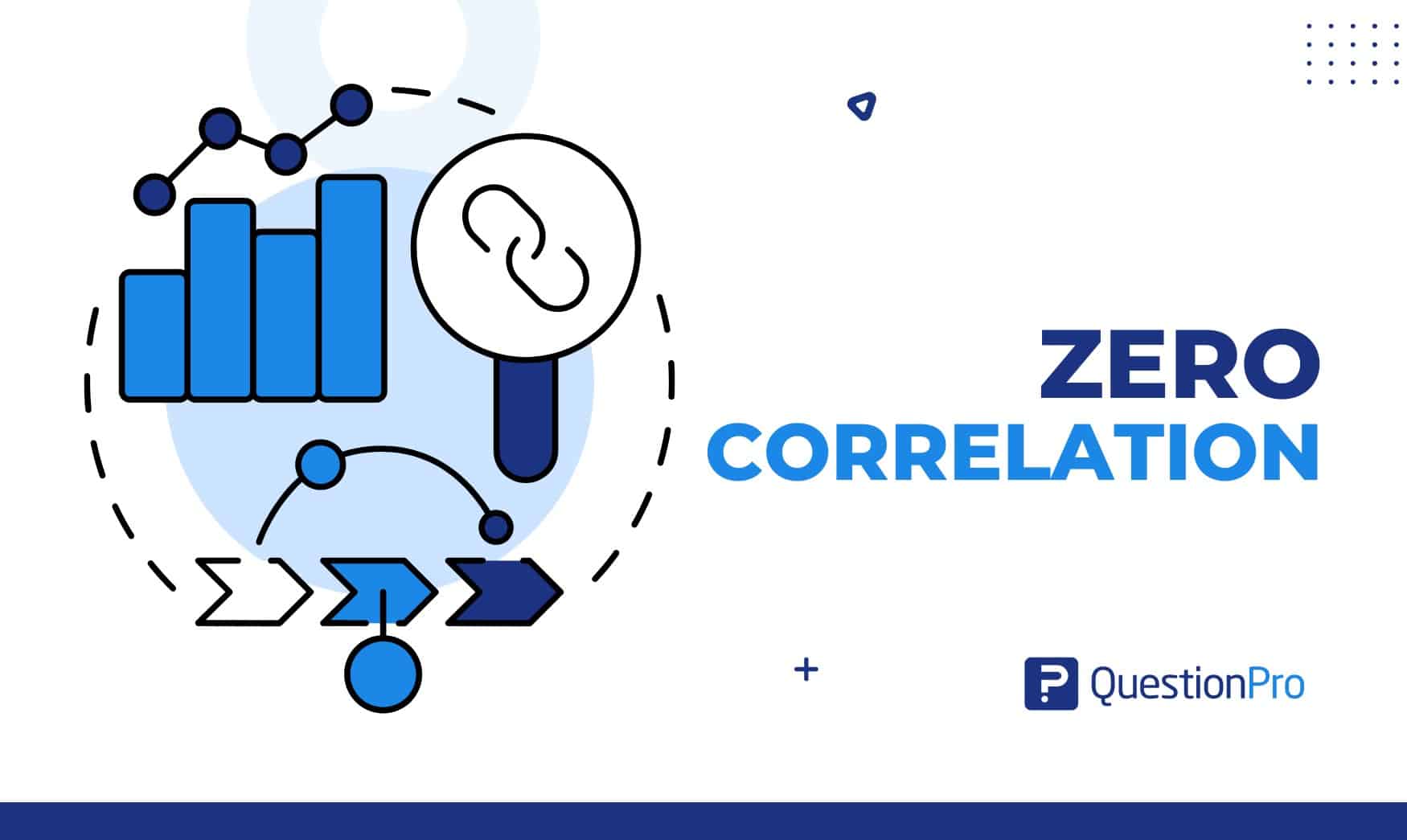
Jul 1, 2024
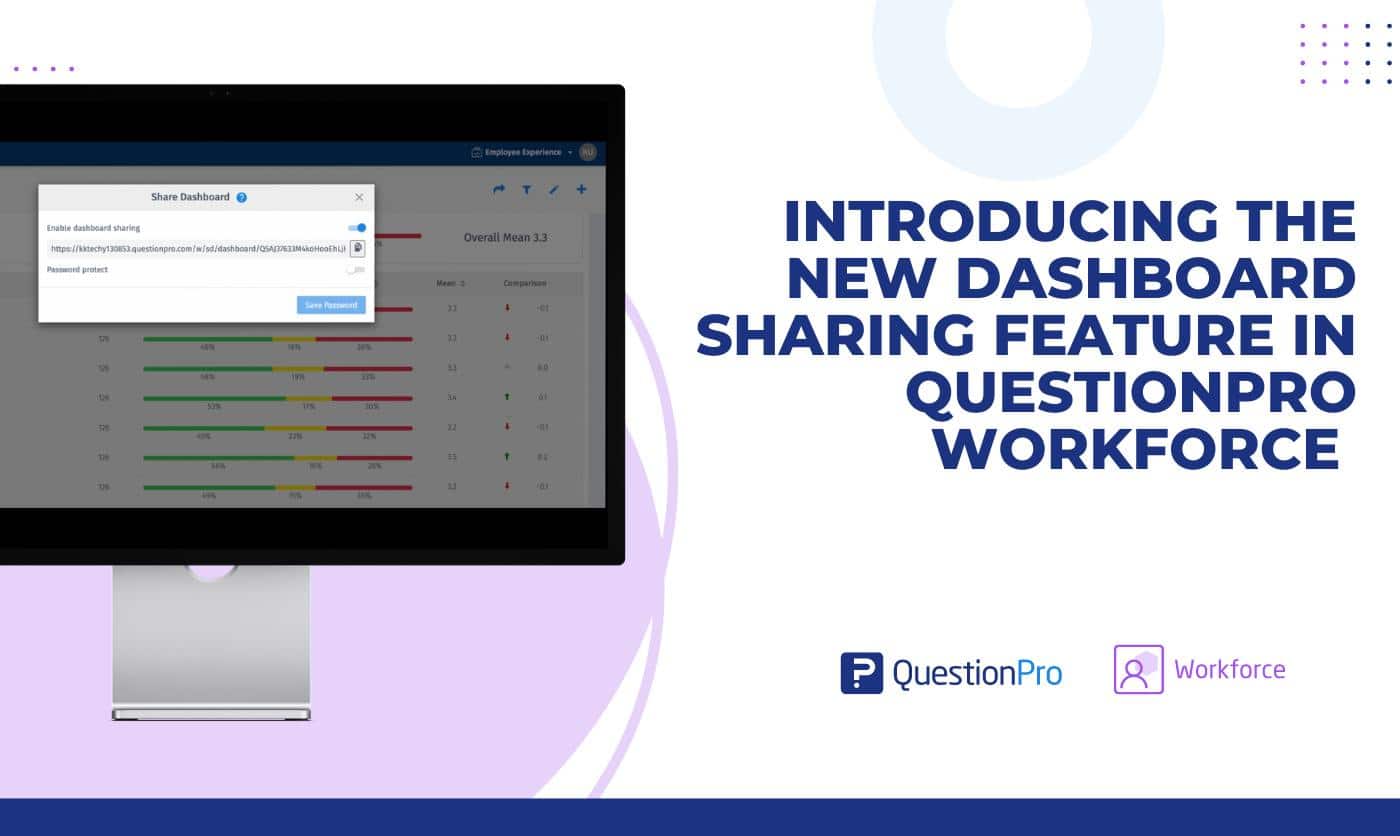
When You Have Something Important to Say, You want to Shout it From the Rooftops
Jun 28, 2024
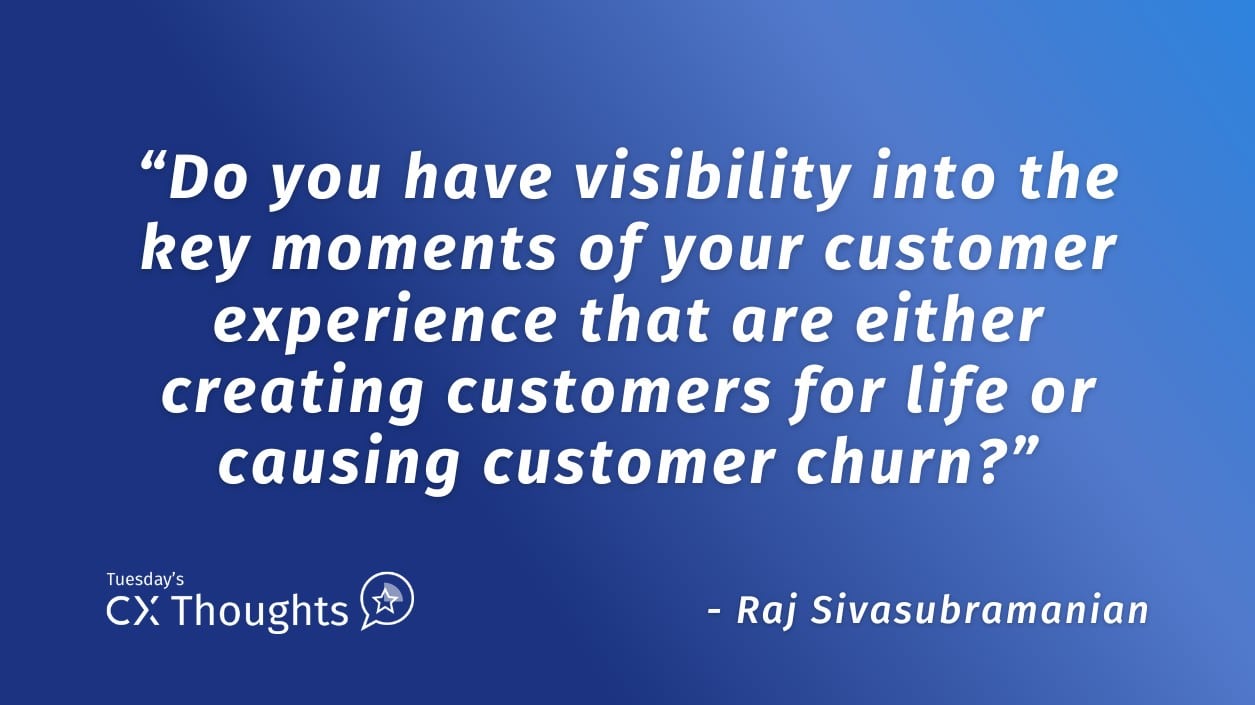
The Item I Failed to Leave Behind — Tuesday CX Thoughts
Jun 25, 2024
Other categories
- Academic Research
- Artificial Intelligence
- Assessments
- Brand Awareness
- Case Studies
- Communities
- Consumer Insights
- Customer effort score
- Customer Engagement
- Customer Experience
- Customer Loyalty
- Customer Research
- Customer Satisfaction
- Employee Benefits
- Employee Engagement
- Employee Retention
- Friday Five
- General Data Protection Regulation
- Insights Hub
- Life@QuestionPro
- Market Research
- Mobile diaries
- Mobile Surveys
- New Features
- Online Communities
- Question Types
- Questionnaire
- QuestionPro Products
- Release Notes
- Research Tools and Apps
- Revenue at Risk
- Survey Templates
- Training Tips
- Tuesday CX Thoughts (TCXT)
- Uncategorized
- Video Learning Series
- What’s Coming Up
- Workforce Intelligence
- Essay Editor
How to Write Recommendations in Research Paper

Every research paper should end with a conclusion and recommendations concerning the main topic. How to write a recommendation report? What should be added in this part, and what – shouldn't be? What is the structure of this section? Having answered these questions, a person may write this fragment of the project perfectly. Let's get into this issue together.
Recommendations in a research paper: meaning and purpose
What is the purpose of recommendations? Why are they so important? Generally, it is the essential element of the research paper because it shows how deeply the material was investigated and analyzed. They are usually given in the form of suggestions based on the research findings and implications.
So, this part of the project deals with areas that occurred underexplored. The author may point out the direction of further possible research or the ways of improving the situation available.
Where to put recommendations?
As a rule, the recommendation section of a research paper is placed in the conclusion or discussion. However, it may depend on the sphere investigated. For example, in business papers, it is possible to write suggestions in an advisory report or set them into a separate segment.
Wherever being included, it should be complete, supporting implications and findings of the project. Clearness, possible practical application, and relevance are the characteristics of this block.
What should recommendations look like?
Your ideas and suggestions are to be well-organized and reasonable. Moreover, value for other researchers in this field should be presented. The approach to the section being solution-oriented, similar investigators will be able to achieve the same results or continue developing the brunch.
There may be three variants of recommendations:
- directives,
- obligations.
The choice depends on the problems and sphere discussed.
When writing your thoughts, try to use a bullet system, not a long straight text. Actionable words are also welcome.
Structure of recommendations
A recommendation section should contain such points as:
- research questions to be discussed;
- a summary of research results;
- their significance for practical application;
- strengths and weaknesses of the work;
- correlation to similar investigations in the sphere mentioned;
- suggestions for future research.
If speaking about a short version of the structure, it may consist of 3 parts:
- A research question.
- A conclusion.
- Recommendations.
As an example
What brand of salty nuts is of low sale in Region A? | research question |
According to the investigation, consumers didn't buy brand T in this region. | conclusion |
It is recommended that Brand T should run the promotion (TV, billboards) in region A. | recommendation |
Recommendations in research papers: key features
The whole investigation process should be systematic and logical; the results – objective, unprejudiced, and replicable. A researcher usually prepares a solid basis of evidence, which serves as a background for further recommendations.
They should be:
- achievable,
- meaningful,
If your ideas meet these requirements, be sure of their success.
How to write recommendations in research papers: a working guideline
Here we'd like to give some definite tips. Just follow the matter to make recommendations as smart and useful as possible.
- Use a clear and neutral language. Try to avoid both professional terms and jargon. Let your recommendation consist of one precise sentence.
- Organize all your thoughts in a coherent system. Define bullet points; think over relevant headings and subheadings.
- Make up measurable and specific recommendations. There should be a clear connection between the investigated issue and the solutions you point out.
- Be sure that all your suggestions are in line with conclusions and research results. It is much better to recommend one efficient thing for all conclusions than to give a bunch of vague ones.
- Formulate only achievable solutions. All of them should be practical and easy-to-render.
- Analyze everything in complex. Give a recommendation, covering all important spheres, explored in your project.
- Avoid presenting new data in this segment. It should be based only on the researched phenomenon.
- Prepare the content, suitable for your audience (colleagues, teammates, companions in the researching field, etc.). Your ideas should make further investigation possible.
- Give an expanded explanation of your recommendations. Do not only list them but provide the readers with evidence, underlining their effectiveness.
- Do not minimize your achievements and merits in the investigated field. Just accentuate that your work is like a takeoff runway for further work.
- Define and analyze the lack of information or possibilities, concerning your research, if there are any. It will testify to your critical thinking skills.
- Finish this part of the research paper with a summary, highlighting the significance and practical value of your suggestions.
Except for the paper segment filling, be sure that you know the standards of recommendation report format. You may find them in local institution guidelines.
Finally, you have enough information to create effective and well-structured recommendations for your research paper. And if you still need any inspiration, visit the essay generator Aithor . It is free and easy to use.
Related articles
How to write a business report with example.
One of the most effective ways to convey essential information is through a business report. This article will guide you through the purpose of a business report, provide valuable writing tips, outline how to format your business report correctly and offer an example for better understanding. What is the Purpose of a Business Report? A business report serves as a critical tool for decision-making within an organization. Its primary purpose is to analyze a particular situation or issue, evalua ...
How to Write a Good Conclusion For a Lab Report
Writing a good conclusion for your science lab report can be the difference between a good grade and a great one. It's your last chance to show you understand the experiment and why it matters. This article will help you learn how to write a lab conclusion that sums up your work and shows your teacher that you understood what you did. What Should Be in Your Lab Report Conclusion? A good lab report conclusion wraps up your lab work in a neat package. When you're thinking about how to write a c ...
Diagnostic Essay Writing Guide and Outline Sample
Imagine stepping into a classroom on the first day and being asked to write an essay. This exercise, commonly referred to as a diagnostic essay, is a common tool used by instructors to gauge their students' writing proficiency. Interestingly, in a study exploring the effectiveness of evaluation papers, over 70% of participants reported that these tasks significantly improved their understanding of their writing strengths and challenges. This finding underscores the assessment assignment's role i ...
How to Write Essay Introductions
When you acquaint two strangers, you introduce one person to another, right? The same thing concerns the text and any material you wish to present to your reader or listener. At that moment an introduction composed of letters comes onto the stage and plays its leading part. Being the first thing the audience is faced with, an essay introduction should catch a person's attention, give information about the topic and idea the author discusses, and prepare him for the following comprehension. How ...
Classification Essay Guide
A classification essay is a powerful tool in academic writing, enabling writers to break down broad topics into organized categories for better understanding. This guide will show you how to write a classification essay, from designing a perfect outline to selecting compelling topics. Continue reading to learn how to create a clear, insightful, and engaging classification essay. What is a Classification Essay? A Brief Overview A classification essay is a type of academic writing that involves ...
How to Write a Successful Letter of Motivation
Every person wishing to get to College or University faces a real challenge – writing a motivation letter. Through lack of knowledge, the process may seem quite stressful and backbreaking. But the devil is not so black as he is painted. Just calm down and let's start. Today we will: 1. discuss the structure of a motivation letter and its peculiarities in terms of sense and format; 2. accentuate the moments, colleges pay special attention to; and 3. give some tips on how to create a real suc ...
Essay Format Tips from an English Teacher
Writing a solid and well-crafted essay is crucial for students and researchers, as it involves presenting arguments clearly and succinctly. Whether you are writing a paper for an assignment, a scientific journal, or a personal statement, understanding the correct essay format is pivotal. This meticulously collated guide covers key features of essay formatting and provides tips to refine your writing. What is an Essay Format? An essay format is a blueprint for shaping your written assignment, ...
How to Write a Reaction Paper Nice and Easy
In the world of student home assignments there lives an interesting and creative project, a reaction essay, by name. It deals with the person's feedback on a movie, book, article, a piece of work, evoking thoughts and emotions. What is the essence of this paper? How to write a perfect one? Let's get acquainted with this issue. At first, imagine the situation when you've just watched a deep philosophical movie or read an article that cut you to the very heart. You feel like thunderstruck. You ne ...
Thank you for visiting nature.com. You are using a browser version with limited support for CSS. To obtain the best experience, we recommend you use a more up to date browser (or turn off compatibility mode in Internet Explorer). In the meantime, to ensure continued support, we are displaying the site without styles and JavaScript.
- View all journals
- Explore content
- About the journal
- Publish with us
- Sign up for alerts
- Open access
- Published: 01 July 2024
Complex artificial intelligence models for energy sustainability in educational buildings
- Rasikh Tariq ORCID: orcid.org/0000-0002-3310-432X 1 ,
- Awsan Mohammed ORCID: orcid.org/0000-0001-6500-5994 2 , 3 ,
- Adel Alshibani ORCID: orcid.org/0000-0001-7809-106X 2 , 4 &
- Maria Soledad Ramírez-Montoya ORCID: orcid.org/0000-0002-1274-706X 1 , 5
Scientific Reports volume 14 , Article number: 15020 ( 2024 ) Cite this article
57 Accesses
Metrics details
- Energy science and technology
- Mathematics and computing
Energy consumption of constructed educational facilities significantly impacts economic, social and environment sustainable development. It contributes to approximately 37% of the carbon dioxide emissions associated with energy use and procedures. This paper aims to introduce a study that investigates several artificial intelligence-based models to predict the energy consumption of the most important educational buildings; schools. These models include decision trees, K-nearest neighbors, gradient boosting, and long-term memory networks. The research also investigates the relationship between the input parameters and the yearly energy usage of educational buildings. It has been discovered that the school sizes and AC capacities are the most impact variable associated with higher energy consumption. While 'Type of School' is less direct or weaker correlation with 'Annual Consumption'. The four developed models were evaluated and compared in training and testing stages. The Decision Tree model demonstrates strong performance on the training data with an average prediction error of about 3.58%. The K-Nearest Neighbors model has significantly higher errors, with RMSE on training data as high as 38,429.4, which may be indicative of overfitting. In contrast, Gradient Boosting can almost perfectly predict the variations within the training dataset. The performance metrics suggest that some models manage this variability better than others, with Gradient Boosting and LSTM standing out in terms of their ability to handle diverse data ranges, from the minimum consumption of approximately 99,274.95 to the maximum of 683,191.8. This research underscores the importance of sustainable educational buildings not only as physical learning spaces but also as dynamic environments that contribute to informal educational processes. Sustainable buildings serve as real-world examples of environmental stewardship, teaching students about energy efficiency and sustainability through their design and operation. By incorporating advanced AI-driven tools to optimize energy consumption, educational facilities can become interactive learning hubs that encourage students to engage with concepts of sustainability in their everyday surroundings.
Similar content being viewed by others
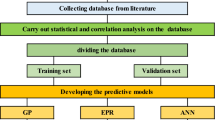
Estimating the energy consumption for residential buildings in semiarid and arid desert climate using artificial intelligence
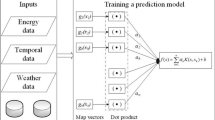
Proposing a hybrid metaheuristic optimization algorithm and machine learning model for energy use forecast in non-residential buildings
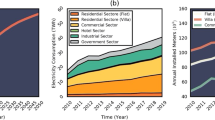
Reshaping energy policy based on social and human dimensions: an analysis of human-building interactions among societies in transition in GCC countries
Introduction.
Energy consumption of constructed facility has a significant impact on the environment. The world is currently experiencing significant fluctuations in the energy landscape, which has far-reaching implications for many aspects of life on a daily basis. Furthermore, global energy demand has risen by 0.9% to 120 millions tons of oil equivalent (Mtoe) 1 . The International Energy Agency anticipates that by 2021, the buildings and construction industry will account for more than one-third of global energy demand. Furthermore, this sector is projected to contribute to approximately 37% of the carbon dioxide emissions associated with energy use and procedures 2 . The impact of this dioxide emissions on the environments, social, and have recently increased, emphasizing the growing importance of sustainable development. Meanwhile, there is a growing demand for building energy services. Therefore, there is a critical need to develop effective strategies for energy planning and prediction. This not only ensures the future progress of the built environment, but also enhances energy efficiency, conserves resources, and promotes sustainable development 3 , 4 . Efficient strategies involve accurately forecasting building energy usage to effectively oversee and conserve energy within buildings.
Educational institutions, including schools, represent a major part of built environment facility that play a crucial role in shaping the future of society through knowledge dissemination and fostering intellectual growth. However, schools represent significant energy consumers, with diverse facilities requiring heating, cooling, lighting, and other services. The efficient management of energy resources in educational buildings is not only essential for cost savings but also aligns with broader sustainability goals aimed at reducing carbon emissions and mitigating climate change impacts 5 . The energy usage in school buildings is influenced by various factors, including their location, size, number of occupants, age, and extent of air conditioning. Accurately predicting energy usage is essential for the effective functioning of contemporary electrical grids 6 , 7 . Numerous research studies have emphasized the importance of precise electricity forecasting. Accurate and reliable estimates of electricity consumption are critical for planning future electricity generation systems to meet the growing demand for electrical energy 8 , 9 . Understanding the variations in building energy usage allows to development of focused and efficient energy conservation strategies 10 . Comprehensive knowledge of building energy consumption prediction is essential for developing innovative approaches such as demand-side management plans 11 , intelligent control systems 12 , and fault detection and diagnosis methods 13 . These methods use predictive analysis to improve energy efficiency, reduce waste, and ensure that building systems run smoothly. These systems encourage both energy conservation and building infrastructure efficiency by determining potential energy-saving possibilities and resolving inefficiencies in operation 14 . Studies have shown that even slight improvements in estimating building energy usage can result in significant energy savings 15 . Managers of buildings and users may adopt better decisions and take proactive steps to increase energy efficiency by precisely predicting energy use patterns. This could involve changing HVAC configurations, improving the schedule of lighting, installing energy-efficient devices, and applying behavioral adjustments to help achieve energy-saving targets 16 .
However, accurate prediction of energy consumption is difficult due to a variety of unpredictable situations or noisy data disorders, and the methods used frequently produce inaccurate projections. Thus, this field requires additional work and attention, particularly from governmental agencies. Consequently, this paper investigates number of artificial intelligence-based models to estimate the energy consumption of school buildings. These models include decision trees, K-nearest neighbors, gradient boosting, and long-term memory networks. The investigated algorithms are developed and validated using real data collected for educational buildings; school. In addition, different statistical tests are conducted to ensure the quality of data. The models are expected to contribute to effective resource management, environmental sustainability, educational opportunities, operational efficiency, and regulatory compliance. Educational institutions can create greener schools while saving money by accurately estimating energy needs and implementing consumption-reduction strategies.
Literature review
Sustainable school buildings contributing towards educational innovation.
In the context of complexity, it is important to look for innovative options that support institutions to become more sustainable in terms of energy. Purnell et al. 17 , implemented plans involving the academic and social community, where they learned about innovation, leadership, coal mining, greenhouse problems, energy audits, alternative energy and promotion of energy efficiency practices in the school and community. Designing the construction or restructuring of school buildings is vital to improve energy consumption 18 . New solutions are needed, hence Zeiler and De Waard 19 presented alternatives for sustainable schools through ‘Plus Energy Schools’, the authors based on a study comparing passive house schools, near-zero energy schools and additional energy schools, where they evaluated the indoor air quality and comfort of some of the schools measured, with a view to contribute to the challenging design towards more sustainable schools. Improving school infrastructure requires attention to the principles of sustainable development.
The designs of school institutions require new ecological, sustainable and healthy practices that accompany educational innovation practices. Educational innovation integrates inputs of processes, products, services or knowledge with a view to improving complex environments 20 and in institutions, the use of new technologies, evolving sustainable design practices and innovative ‘green’ building materials should be promoted for each new project to be reflected in constantly improving energy efficiency results 21 . In parallel to sustainable care, it is relevant that institutions are energy neutral, energy positive and provide comfort to stakeholders (students, teachers, managers, parents) in learning environments 22 . Zhang et al. 23 provided an energy prototype with sustainable strategies and energy-saving technologies, which can reduce energy consumption in school facilities as well as improve the indoor environment. Sustainable design and construction strategies that combine high levels of energy efficiency, performance standards and indoor environmental quality should integrate experimentation and contribute to formulating innovative sustainable building strategies 24 . Innovation and sustainability are substantial elements for educational institutions in the context of constant change.
Complex artificial intelligence modelling through machine learning methods in educational buildings
In Artificial intelligence has been of value in modelling solutions in various sectors. For example, in river studies, Msaddek et al. 25 employed artificial intelligence to reduce potential uncertainties using the Unsupervised Multi-Frameworks Technique and Fuzzy Membership Framework and supervised learning based on the Multi-Model Approach and Gene Expression Programming. Similarly, Tao et al. 26 applied artificial intelligence models for suspended river sediment prediction to provide an updated description of the most recent and relevant AI-based applications for modelling sediment transport in watershed systems. A combination of water management combined with socio-technical systems is presented by Baki et al. 27 , assessing the dynamic nature of socio-economic variables in order to test the effectiveness of different policies, such as awareness campaigns, and dynamically simulate the subsequent response of the urban water system over time. In the financial sector, Méndez-Suárez et al. 28 integrated artificial intelligence for automated financial advice in the copper market with promising results, both in terms of statistics and trading metrics. Modelling through machine learning provides opportunities for various sectors, including the education sector.
Educational buildings also benefit from complex artificial intelligence modelling. Reddy et al. 29 conducted a plug load study in educational buildings using machine learning algorithms to monitor their energy consumption where they applied machine learning techniques. López-Pérez & Flores-Prieto 30 studied energy savings in an air-conditioned educational building in the tropical climate of Aw in relation to annual cooling load and degree-days, with adequate comfort levels following the adaptive thermal comfort approach. Comfort temperature modelling was performed using fuzzy logic, artificial neural networks, adaptive neuro-fuzzy inference system and a local linear model. Hosseini et al. 31 modelled, analysed and optimised an integrated energy system for the provision of triple loads of an educational building using artificial intelligence, showing that the dynamic load pattern provides the highest energy efficiency and the lowest total cost rate. Lee & Zhang 32 provide a new artificial intelligence of things (AIoT)-based framework for predicting multidimensional indoor environment quality (IEQ) conditions that provides information on IEQ conditions and their potential impacts on student well-being, thus facilitating the future development of climate-adaptive, data-driven, human-centric educational facilities. Artificial intelligence modelling through machine learning methods in educational buildings has a high potential to improve educational environments, their sustainability and energy efficiency.
Summary of literature review and research contribution
Since 2002, government agencies, including the European Union, have assessed building energy efficiency to forecast energy consumption 33 . The fundamental purpose of energy prediction is identifying and forecasting potential enhancements to optimize energy consumption within a building. There are several energy prediction methodologies available, each with its own set of benefits and drawbacks 34 . Previous studies provided a review of energy prediction methodologies used to forecast building energy performance 35 , 36 , 37 . Chae et al. 38 used an Artificial Neural Network (ANN) model with a Bayesian regularization technique to predict the short term energy usage of building. The authors analyzed several design criteria to determine the most effective structure for the prediction model. Biswas et al. 39 built and verified an ANN model to meet the non linear difficulty of acquiring data on energy use while providing reliable computation of large dynamical datasets. Deb et al. 40 also introduced an ANN for estimating the consumption of energy from both night and day cooling demands in educational facilities. The authors separated the energy usage data into various kinds of inputs.
Yan et al. 41 proposed a hybrid model based on a neural network to forecaste energy consumption in individual households. This model was created primarily to address issues related to irregular human behavior and univariate datasets found in single household energy consumption prediction. Zhong et al. 42 developed a support vector machine to address issues with the accuracy of forecasting related to the significant non-linearity between both outcomes and inputs in energy usage prediction models. Wang 43 and Tabrizchi 44 also used a self-adaptive multiverse optimization to enhance the accuracy of prediction and tune the features of the support vector machine. Meanwhile, Iwafune et al. 45 used regression models to forecast the electricity usage in 50 households. Albuquerque et al. 46 employed standardized machine-learning algorithms to forecast Brazil's consumption of electricity. The findings showed that random forests provided forecasts. In addition, Dong et al. 47 introduced a technique for forecasting energy consumption specific to building operations across multiple timeframes, employing pattern classification and integrated learning. They utilized random forests to assess the significance of input features in the model and conducted correlation analysis to explore the connection between the input parameters and output. The results showed that the integrated consumption of energy forecasting approach with pattern categorization outperformed the unclassified integrated energy consumption prediction model.
Cao et al. 48 presented an integrated model for predicting energy consumption that uses spatial features taken from time-series information to estimate short-term energy usage in higher education organizations. They used the collaborative game theory SHAP approach to evaluate the influence of features on model performance, using ablation analysis to identify the optimum amount of features needed. Faiq et al. 49 utilized LSTM to estimate energy usage in institutional buildings. The authors evaluated the model's performance against SVM and Gaussian process models. Álvarez et al. 50 studied 453 homes in Spain to estimate their U-opaque rating. Several ANN structures were developed and evaluated on actual recorded data, with a Pearson correlation factor of 0.967. Beccali et al. 51 proposed using artificial neural networks to anticipate building energy efficiency in buildings in Italy. Ahmad et al. 52 investigated the performance of random forest and ANN models for predicting HVAC power usage in hostels. The findings indicated that ANN outperformed random forest slightly. Martellotta et al. 53 employed to estimate heat energy usage, training the network on EnergyPlus-generated simulated data. Williams and Gomez 54 studied 426,305 single-family dwellings to anticipate monthly energy usage using building features and monthly weather data. Sun and Han 55 provided an ANN model that took into account a variety of input factors such as orientation, window size, windows per bay, number of floors, and floor height. Similarly, Wong et al. 56 utilized ANN to forecast the overall energy usage, taking into account nine weather-related input factors, four-building envelope variables, and one-day category.
Moreover, Catalina et al. 57 constructed regression models to forecast the monthly heat demand of homes in France. The study incorporated factors such as the building's structural U-value, window-to-wall ratios, and the construction shape factor. Al-Rashed and Asif 58 investigated a variety of variables that impact the use of energy in residential buildings, including building envelopes, weather patterns, cooking appliances, dwelling types, and air conditioning systems. the findings indicated the use of double-glazing installations and air conditioning with mini-split systems. However, the authors point out a limitation: the inability to evaluate architectural design in order to reduce energy costs during the early design phase. Abdel-Aal et al. 59 developed a model to predict electric power energy consumption in Saudi Arabia's Eastern Province that takes into account conditions as well as economic and population aspects. Nasr et al. 60 proposed an ANN forecasting approach for estimating electric energy consumption in Lebanon using weather variables and time series data. Meng et al. 61 used a hybrid approach to forecast the increasing trend for electrical consumption of energy. However, one disadvantage of this methodology is that it fails to include architectural aspects and design integration when estimating monthly electric energy demand. Karatasou et al. 62 used statistical analysis tools to investigate potential improvements in ANNs accuracy for forecasting the consumption of building energy. Mena-Yedra et al. 63 developed a short term ANN approach for estimating energy load at an industrial buildings, resulting in timely solutions.
In addition to the studies mentioned above, Somu et al. 64 presented an integrated approach for forecasting residential energy consumption that combines long short-term memory and neural network. The findings demonstrated promising results in predicting previously difficult energy consumption patterns. Fath U Min Ullah et al. 65 employed an ANN algorithm to anticipate household electricity demand in Korea, demonstrating the efficacy of the prediction technique. Meanwhile, Liu et al. 66 also used an artificial neural network to predict electricity consumption in China, demonstrating the model's outstanding precision. Khalil et al. 67 developed an ANN approach to anticipate a building's cooling and heating requirements. The model addressed several variables including wall thickness, and orientation, glazing density distribution, glazing, roofing surface, surface area, relative compactness, and overall height. The dataset used for model construction was obtained from existing publications. Furthermore, Rahman et al. 68 developed a recurrent neural network model to forecast medium- to long-term power demand patterns in both residential and commercial buildings, recognizing their significant effect on total electrical usage in the United States. Tartibu and Kabengele 69 proposed an innovative way to estimate South Africa's prospective consumption of energy using an ANN. The proposed technique for estimating power consumption has been verified and evaluated using data from the Council for Scientific and Industrial Research spanning 2014 to 2050. The findings demonstrated that the ANN was capable of accurately predicting energy demand. Fayaz et al. 70 presented a technique for evaluating short-term home energy use. This system had four separate layers: data gathering, preparation, forecasting, and validation. The research employed real data from four multi-story buildings in Seoul, South Korea, to demonstrate the efficiency of the proposed approach. Alshibani 7 identified the factors impacting the schools’ energy consumption in hot and humid climate weather conditions. Also investigated the influence of the identified factors on the energy consumption of school facilities. Mohammed et al. 71 proposed a regression model for predicting the energy consumption of schools in Saudi Arabia. The results revealed the regression models are proposing tools for estimating energy consumption.
The literature review indicated that there is a need to develop efficient models for predicting the energy consumption of educational buildings. Therefore, this paper proposes effective artificial intelligence approaches to estimate the energy consumption of educational buildings. Specifically, this paper proposes machine learning algorithms based on decision trees, K-nearest neighbors, gradient boosting, and long-term memory networks for estimating the energy consumption of school buildings. Real data are collected to develop and evaluate the proposed model. In addition, the variables that influence the energy consumption of educational buildings are determined based on the literature and experts. The relationship between the input factors and the energy consumption is investigated using the Pearson correlation test. The collected data are filtered and prepared. Moreover, the hyperparameters of the proposed models are tuned optimally.
Material and method
This paper proposes machine learning algorithms for estimating the energy consumption of educational buildings. These models include decision trees, K-Nearest neighbor, gradient boosting, and long-term memory networks. The development process begins with identifying the factors influencing energy consumption, which are identified based on both literature reviews and experts. The actual data on energy consumption and relevant input parameters are then gathered and separated into distinct subsets for training, validation, and testing. The collected data are filtered and analyzed to develop robust machine-learning models.
In this paper, descriptive statistics are conducted to provide concise summaries that facilitate understanding and interpretation of the collected data. A Scatter matrix also is constructed to investigate relationships among multiple variables within a dataset and to offer a comprehensive overview of how variables interact. The correlation between the input variables and the target, which is the annual energy consumption of educational buildings, is investigated. Correlation statistics are thoroughly applied to all input variables to identify redundant variables and explore the relationship between the factors and energy consumption. Moreover, parallel coordinate plots are developed to provide a comprehensive visual representation of data while also providing insights into the dataset's underlying structure. The second phase of the research methodology is to select and train the machine learning algorithm that will estimate the energy usage of educational buildings on a yearly bases. The optimal machine learning structure is selected so that the Coefficient of Determination (COD), Mean Absolute Percentage Error (MAPE), Mean Absolute Error (MAE), and Root Mean Square Error (RMSE) are minimized. The model's performance is then evaluated using the testing dataset. Figure 1 depicts the research methodology for the proposed model.
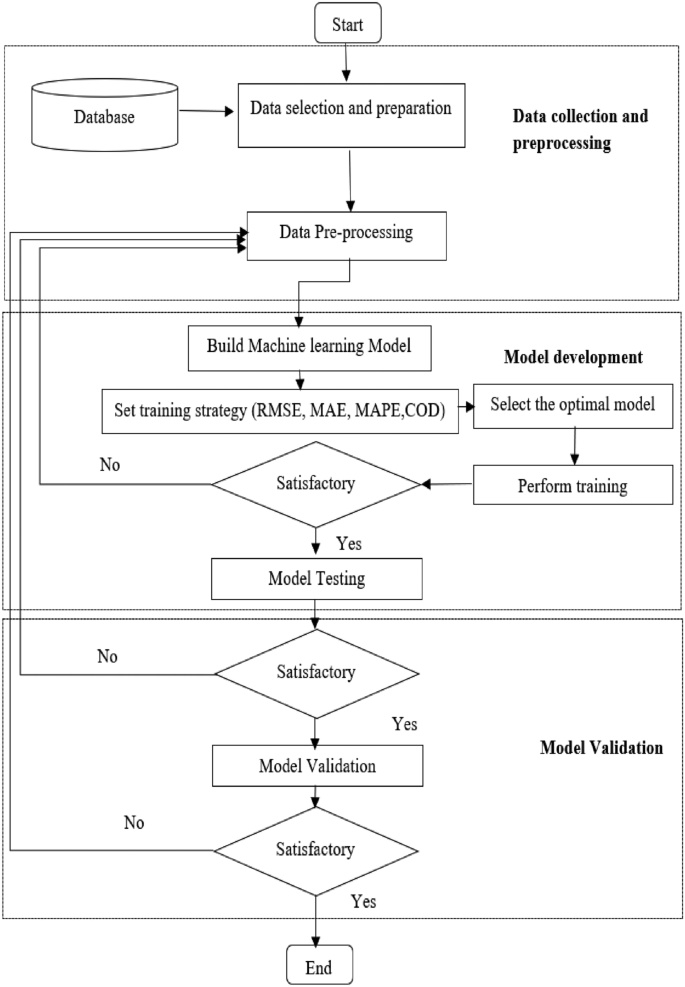
Overall flow diagram of the research method.
Data collection
Direct data collection from educational buildings has replaced paper records as the most efficient way to monitor energy usage. This method seeks to shorten the amount of time needed to collect data. The data gathered covered a wide range of factors that affect energy use and was used as input for a machine-learning model. one parameter was the energy consumption, which was expressed in kWh annually and designated as the output. The dataset was established by filtering real energy consumption data from 352 educational facilities in order to eliminate any outliers. Eleven input variables and one output variable were selected from this refined dataset. In particular, some variables—like different kinds of lamps—were left out of the inputs since they had less of an effect on consumption than other variables. Table 1 illustrates the primary variables impacting the consumption of energy of educational buildings, which were employed as inputs for the machine learning models.
Data pre-processing
Data pre-processing plays a crucial role in the development of machine learning models. It involves a set of steps designed to prepare raw data for analysis. In this paper, descriptive statistics are used to better understand the interpretation of data science algorithms. The average, standard deviation, maximum, minimum, and quartile values for each parameter are calculated. In addition, different visualization tools to understand the behavior of the data are used. Data visualization is an effective tool for interpreting and communicating insights derived from complex datasets. By converting raw data into graphical representations, we can reveal the patterns, trends, and relationships that would otherwise be hidden. Through visualization, we can overcome the limitations of tabular data and gain a thorough understanding of its inherent structure and characteristics. This deeper understanding enables us to draw meaningful conclusions and make informed decisions. In this research, we also use the scatter matrix to perform a detailed pairwise comparison of variables, revealing their interrelationships and individual distributions. Moreover, we use a parallel coordinate plot to visualize multivariate data, allowing us to discern intricate patterns and correlations among the dataset's various dimensions, with a particular focus on annual energy consumption in schools. These visualization techniques not only illuminate complex data landscapes but also help to identify key insights that are critical for informed decision-making and strategic planning. Furthermore, the relationship between the input factors and between the inputs and output is investigated. Pearson's coefficient of correlation is used to improve understanding of the relationship between the input variables and the target, which is the annual energy consumption of educational buildings.
Complex artificial intelligence models through machine and deep learning
In this paper, various machine-learning models are proposed to estimate the energy consumption of educational buildings. These techniques include decision trees, K-nearest neighbors, gradient boosting, and long short-term memory. A brief description of each algorithm is provided in the subsequent subsections:
Decision Tree: A decision tree algorithm is a machine learning technique that partitions input data recursively based on feature values, resulting in the prediction of a target variable. Starting with the entire dataset at the root node, the algorithm selects the best attribute to split the data into subsets, to maximize information gain, minimize impurity, or reduce variance, depending on the task. This process continues iteratively, resulting in a tree-like structure with internal nodes representing decision points based on feature values and leaf nodes representing predicted outcomes. Overfitting can be prevented by using a variety of stopping criteria, such as maximum depth or minimum samples per leaf. Pruning techniques can help refine the tree by removing unnecessary branches. Prediction involves traversing the tree from the root to a leaf node based on the attribute values of a new instance, and the majority class or average value in the leaf node is assigned as the prediction 72 .
K-Nearest Neighbor: The k-nearest neighbors is a useful and simple technique for prediction and classification. It works by identifying the k closest data points (neighbors) in the feature space to a given query instance and then making predictions based on their labels or values. In classification, the predicted class is usually determined by a majority vote of the k nearest neighbors, whereas in regression, the predicted value is frequently the average of the k nearest neighbors' values. The number of neighbors to consider (k) is an important hyperparameter that can have a significant impact on the algorithm's performance. A lower value of k results in more flexible decision boundaries but may increase sensitivity to noise, whereas a higher value of k smooths out the decision boundaries but may oversimplify the model. The k-NN algorithm is a popular choice for a variety of machine learning tasks due to its simplicity and effectiveness, particularly when the underlying data distribution is unknown or interpretability is required 73 .
Gradient Boosting: Gradient boosting is an effective machine-learning technique for both regression and classification tasks. It constructs a predictive model sequentially using an ensemble of weak learners, typically decision trees. The algorithm works by fitting a series of trees to the previous trees' residuals (or gradients), with each new tree attempting to correct the errors made by the predecessors. Gradient boosting, an iterative process, builds a strong learner by combining the predictions of multiple weak learners, frequently achieving high predictive accuracy. Gradient boosting's key components include the selection of a loss function, the learning rate, which controls the contribution of each tree to the ensemble, and the maximum depth or complexity of the individual trees 74 .
Long Short-Term Memory: Long Short-Term Memory (LSTM) is a recurrent neural network structure that aims to overcome the limitations of traditional RNNs in recording extensive dependents within data that is ordered. LSTMs use a gating mechanism consisting of input, forget, and output gates to control information flow throughout the network, in contrast to traditional RNNs, which have difficulties with the vanishing gradient problem when learning from remote dependencies. With the use of this gating mechanism, long sequences of data can be retained with greater relevance while lowering the likelihood of signal degradation. This allows LSTMs to selectively update and discard information over time. Furthermore, LSTMs include a cell state that acts as a conveyor belt, carrying information across time steps and facilitating the flow of gradients during training. As a result, LSTMs have become a key component in sequence modeling tasks, outperforming traditional RNN architectures 75 .
Performance evaluation of machine learning models
Different assessment measures are utilized to assess the accuracy of the proposed machine learning algorithms. These measures include Root Mean Square Error (RMSE), Mean Absolute Error (MAE), Mean Absolute Percentage Error (MAPE), and Coefficient of Determination (COD).
1. Mean Absolute Error 76 : It measures the mean absolute difference between the values that a model predicts and the values that are actually in a dataset. It is given by the following formula:
where the yi is the actual value of the energy consumption at point , n represents the number of educational buildings, and \(\widehat{{y}_{i}}\) is predicted value of the energy consumption at point.
2. Root Mean Square Error 77 : It calculates the square root of the average squared variances between the actual and anticipated values in a dataset. Mathematically, RMSE is calculated as:
3. Mean Absolute Percentage Error 78 : It calculates the mean absolute percentage difference between a dataset's actual values and its predicted values. Mathematically, MAPE is calculated as:
4. Coefficient of Determination 79 : It demonstrates the percentage of a machine learning model's dependent variable's variance that can be predicted based on the independent variables. In other words, COD quantifies the goodness of fit of the model to the actual data. It is given by the following formula:
Results and discussion
Descriptive statistics of the data variables.
The descriptive statistics is fundamental to understand the interpretation of data science algorithms, which is depicted in Table 2 . The study encompassed data collection from 352 schools, presenting a comprehensive analysis of various structural and operational characteristics. The mean number of floors was approximately 3.26, with the built area averaging at 2,596.59 square meters and the roof area at 4,165.63 square meters. School types varied with a mean value suggesting a mix of primary and secondary education facilities. On average, schools had 381.10 students and 28.18 staff members. The average age of the school buildings was noted to be approximately 32.82 years, reflecting a moderate level of infrastructural maturity. Classrooms averaged at 14.26 per school, indicating moderate to large school sizes. Air-conditioned areas averaged 817.71 square meters, with the AC capacity averaging at 160.12 tons of refrigeration. The mean annual energy consumption of the schools was a substantial 410,968 kWh/year. The data displayed a standard deviation in total built area and roof area of 1902.62 and 649.17 square meters, respectively, indicating significant variability among the sizes of the schools surveyed. The standard deviation in the number of students and staff was 217.57 and 9.35, respectively, which illustrates a diverse range of school populations and staffing levels. The variability in air-conditioned area and AC capacity (std of 354.60 m 2 and 55.82 tons of refrigeration) reflects different approaches to climate control.
Data visualization and interpretation
The data visualization is supported through the usage of a scatter matrix which is displayed in Fig. 2 . The scatter matrix provided allows for a comprehensive pairwise comparison of the variables in relation to one another, including their individual distributions. This form of analysis is instrumental in discerning the nature of relationships across multiple dimensions of the dataset, especially focusing on how they may correlate with annual energy consumption in schools. Analyzing the histograms on the diagonal, a distribution of each variable can be noted. For instance, 'City' appears to have a uniform distribution, whereas 'Annual Consumption' seems to be skewed, with a high occurrence of lower values and fewer instances of high energy consumption, suggesting a concentration of schools with lower energy use. The scatter plots off the diagonal offer insights into bivariate relationships. For example, 'Total Built Area' vs. 'Total Roof Area' displays a linear trend, indicating a strong positive correlation, as expected since larger built areas typically result in larger roof areas. Similarly, 'Number of Students' vs. 'Number of Classrooms' shows a clear positive trend, highlighting that schools with more students tend to have more classrooms. Considering 'Annual Consumption', the plots against variables like 'Total Built Area', 'Total Roof Area', and 'AC Capacity' reveal positive correlations, as indicated by the concentration of points along an upward trajectory. This supports the hypothesis that larger school sizes and greater AC capacities are associated with higher energy consumption. Conversely, variables such as 'Type of School' show a more dispersed scatter with 'Annual Consumption', suggesting a less direct or weaker correlation. This implies that the type of school may not be a strong determinant of its energy consumption levels. The 'Number of Floors' does not show a distinct trend in relation to 'Annual Consumption', which might indicate that the number of floors in a school is not a straightforward predictor of its energy use, possibly due to variations in building design and usage patterns. It is also interesting to note the patterns in 'Number of Students', 'Number of Staff', and 'Number of Classrooms', which when plotted against 'Annual Consumption', show a more cloud-like distribution with a slight positive correlation. This suggests that while larger numbers in these categories can lead to increased energy use, the relationship is not as pronounced or linear as with physical building characteristics. Finally, the relationship between 'Age of Building' and 'Annual Consumption' does not present a clear pattern, indicating that the age may not have a straightforward impact on energy usage. This could be due to a variety of factors, such as renovations, maintenance practices, or the installation of energy-efficient systems in older buildings. In summary, the scatter matrix provides a rich visual framework for understanding the complex interrelationships between school characteristics and energy consumption. It highlights significant variables that warrant closer examination and could inform strategies for energy efficiency, facility design, and management within the educational sector.
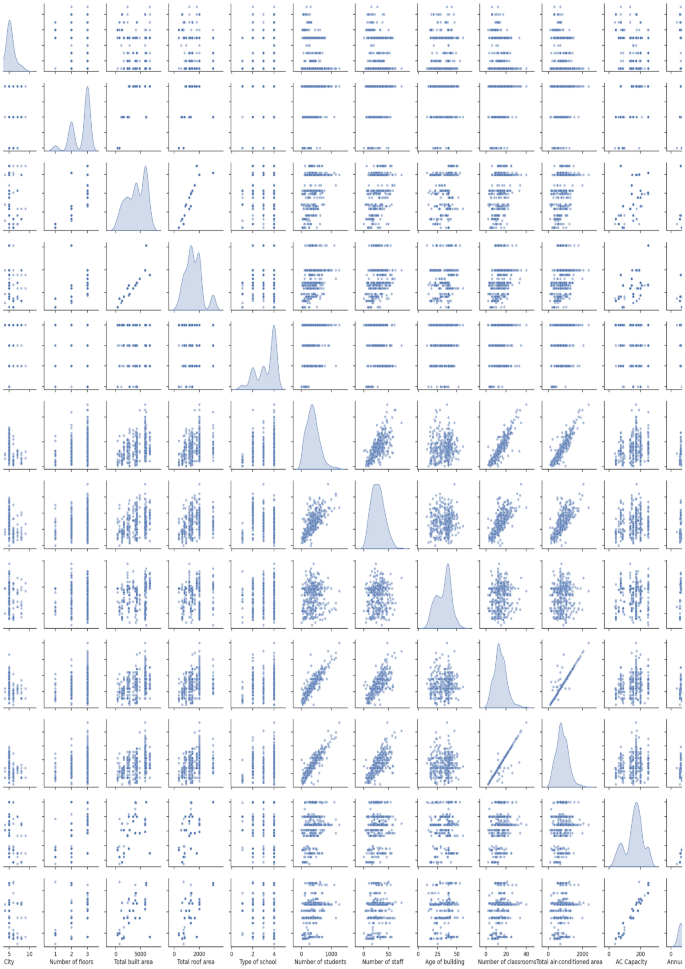
Scatter matrix of the variables. A full resolution figure can be reviewed on the link: https://doi.org/10.6084/m9.figshare.26024836 .
The visualization of data is higher order dimensions is quite tricky, therefore a parallel coordinate plot of all the variables is displayed in Fig. 3 , whereas, the line colors on it are only tracing the variability in the annual energy consumption. Therefore, the parallel coordinate plot provides a visual representation of multivariate data, allowing us to discern patterns and relationships between the various dimensions of the dataset, particularly in relation to annual energy consumption in schools. From the visualization, it is evident that certain variables exhibit a greater range of values and are more densely interwoven with annual consumption, which is color-coded from purple to yellow, indicating low to high energy usage, respectively. Notably, the lines connecting 'Total Roof Area', 'Number of Students', 'Number of Classrooms', and 'AC Capacity' to 'Annual Consumption' display a gradient trend, transitioning from cooler to warmer colors as the values increase. This suggests a positive correlation where higher values in these variables tend to be associated with greater energy consumption. The variables 'City' and 'Number of Floors', while also connected to varying degrees of energy consumption, show less of a clear gradient, indicating that the relationship with energy use may be influenced by other factors or is less directly correlated. Furthermore, the 'Type of School' and 'Age of Building' appear to have a more diffuse distribution of colors across their range, implying a weaker or less direct relationship with energy consumption. These lines do not follow a clear pattern, suggesting that the type of school and the age of the building are not as strongly predictive of energy usage as the size or occupancy-related variables. It is also noteworthy that 'Total Built Area', much like 'Total Roof Area', displays a concentration of warmer colors at higher values, supporting the idea that larger physical dimensions are indicative of increased energy needs. The densely packed lines connecting 'Number of Classrooms' and 'Total Air-Conditioned Area' with higher annual consumption rates further underscore the significant impact of space usage on energy consumption. The correlation appears stronger as the air-conditioned space is a direct consumer of energy, primarily through cooling systems. In contrast, the visual representation does not suggest a strong direct relationship between 'Number of Staff' and energy consumption, as evidenced by the more uniformly distributed color range across its values. Lastly, 'AC Capacity' shows a very strong association with energy consumption, indicated by a predominance of yellow lines as AC capacity increases, reinforcing the assumption that air conditioning is a substantial component of a school's energy profile. Overall, the parallel coordinate plot underlines the multidimensional nature of energy consumption in educational facilities. It highlights the significance of spatial parameters and student density as strong indicators of energy use, while also illustrating the intricate relationships and potential confounding factors that must be considered in the pursuit of energy efficiency and sustainability in school design and operation.
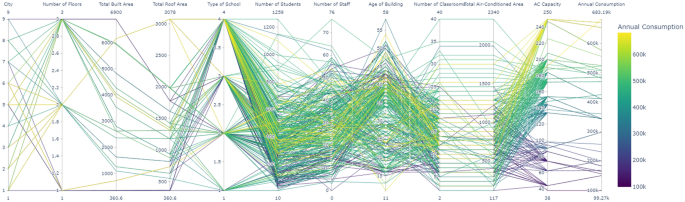
Parallel coordinate plot of the variables. A full resolution figure can be reviewed on the link: https://doi.org/10.6084/m9.figshare.26025634 .
The coefficient of correlation based upon the mathematics of Pearson can improve the understanding between the correlation of the input variables and the target which is annual energy consumption of the educational buildings, which is depicted in Fig. 4 . Upon analyzing the Pearson Coefficient of Correlation between various school characteristics and their annual energy consumption, several interesting relationships emerge. The city shows a moderate negative correlation (− 0.3256) with energy consumption, indicating that certain urban factors might be leading to more efficient energy use or that different locales may have varying energy requirements. In contrast, the number of floors within a school displays a positive correlation (0.3218) with energy consumption. This could be due to the additional energy required for heating or cooling larger vertical spaces and the use of elevators. The total built area and roof area of schools show positive correlations of 0.4678 and 0.6511 with annual energy consumption, respectively, which are among the strongest observed in the dataset. These correlations suggest that as the physical footprint of a school increases, so does its energy demand, likely due to the larger volumes of space requiring climate control. Interestingly, the type of school has an almost negligible positive correlation (0.0119) with energy consumption. The student and staff numbers show positive correlations with energy consumption, 0.3088 and 0.1589 respectively, though the strength of these relationships is varied. The number of students has a stronger correlation, which could be due to the fact that more students likely require more resources, including lighting, heating, cooling, and technology, all of which contribute to energy use. The number of staff has a weaker positive correlation, indicating that staff numbers alone are not as significant a determinant of energy consumption as student numbers. Age of the building shows a very weak positive correlation (0.0217) with energy consumption, suggesting that older buildings may not necessarily consume more energy than newer ones, possibly due to factors such as construction materials, design, or retrofits. The number of classrooms and the total air-conditioned area both show weak to moderate positive correlations (0.2495 and 0.2301, respectively) with energy consumption. More classrooms could imply more space to heat, cool, and light, whereas more air-conditioned space directly relates to higher energy use due to the demands of cooling systems. Finally, the AC capacity shows a very strong positive correlation (0.9703) with annual energy consumption. This is to be expected, as air conditioning is a major energy consumer within buildings, especially in climates that require it year-round or for large parts of the year. In summary, the size of the school (in terms of both physical dimensions and occupancy) and the capacity of air conditioning systems are prominent factors in energy consumption. The relationship between these variables and energy use is multifaceted, with implications for energy management, sustainability initiatives, and the design of educational facilities. While certain correlations are stronger than others, each variable contributes to the overall energy footprint of a school, and understanding these relationships is key for developing strategies to reduce energy consumption and promote efficiency.
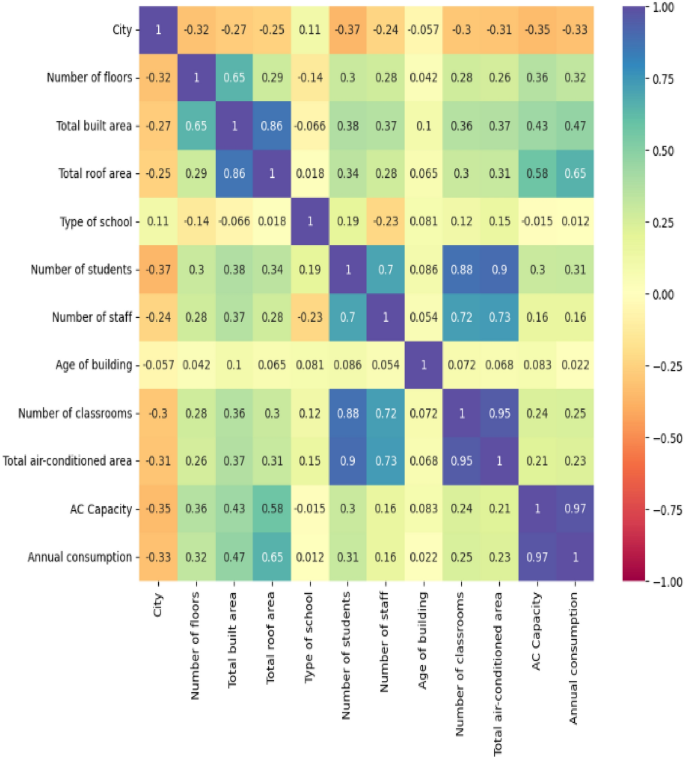
Heat map based upon Pearson coefficient of correlation.
Artificial intelligence models to predict the energy consumption in educational buildings
Various artificial intelligence methods from the machine learning block like decision tree, K-Nearest Neighbors, Gradient Boosting, and one deep learning method which is Long-Short-Term Memory was implemented, and the construction of the hyper-parameters are developed as follows:
Decision tree: In this study, a Decision Tree Regressor algorithm was developed to model and predict annual energy consumption in schools, considering various structural and operational characteristics as predictors. The model was constructed using a robust machine learning pipeline employing Scikit-learn, a popular Python library for data science and machine learning. The predictive features (X) incorporated in the model were: 'Number of floors', 'Total built area', 'Total roof area', 'Number of students', 'Number of staff', 'Age of building', 'Number of classrooms', 'Total air-conditioned area', and 'AC Capacity', with the target variable (y) being 'Annual consumption'. The dataset was divided into a training set (80%) and a testing set (20%), ensuring the model's ability to generalize to new, unseen data. To optimize the model's performance, a grid search with five-fold cross-validation was executed over a predefined range of hyperparameters. The parameters included various depths, minimum samples for splitting and at leaf nodes, maximum features at each split, and the maximum number of leaf nodes. The grid search aimed to find the optimal combination of parameters that minimized the negative mean squared error (MSE). The best hyperparameters for the Decision Tree were determined to be a maximum depth of 5, utilizing all features at each split ('auto'), a limit of 10 maximum leaf nodes, a minimum of 10 samples at each leaf node, and a minimum of 20 samples required to split a node. This configuration indicates a model complex enough to capture underlying patterns, yet restrained to prevent overfitting.
K-Nearest Neighbor: A K-Nearest Neighbors (KNN) Regressor algorithm was implemented to forecast the annual energy consumption in educational institutions, leveraging a variety of structural and operational features as predictive variables. The model was constructed using Scikit-learn, an esteemed Python library renowned for its proficiency in machine learning and data science endeavors. The feature set incorporated into the model constituted all available variables except for the 'Annual consumption', which served as the target variable (y). The dataset was prudently partitioned into training and testing subsets, with an 80–20 split, reinforcing the model's robustness in generalizing to unseen data points. To ascertain the most efficacious configuration of hyperparameters, an exhaustive grid search, coupled with five-fold cross-validation, was executed. This entailed a methodical exploration of different numbers of neighbors, weight schemes, algorithms, and distance metrics. The grid search's chief objective was to pinpoint the hyperparameter combination that would minimize the negative mean squared error, thereby enhancing the model's predictive accuracy. The implementation of the K-Nearest Neighbors (KNN) algorithm involved an extensive hyperparameter tuning process using GridSearchCV, a method that systematically works through multiple combinations of parameter tunes, cross-validating as it goes to determine which tune gives the best performance. The algorithm evaluated a total of 64 candidate models across 5 different folds of the dataset, resulting in 320 individual fits. Subsequent predictions on both the training and testing data were then generated using this refined model.
Gradient Boosting: Gradient Boosting Regressor model was implemented to forecast annual energy consumption in schools based on a set of selected features. Employing Scikit-learn's comprehensive toolkit, the integrated features that represent both physical characteristics and demographic factors of schools, such as 'Number of floors', 'Total built area', 'Total roof area', 'Number of students', 'Number of staff', 'Age of building', 'Number of classrooms', 'Total air-conditioned area', and 'AC Capacity', with the target variable being the 'Annual consumption'. A systematic division of the dataset facilitated the creation of distinct training and testing cohorts, comprising 80% and 20% of the data, respectively. This was followed by standardization of the features to normalize the data, enabling more effective learning by the model. The model's calibration was meticulously conducted through a GridSearchCV process that spanned a diverse array of hyperparameters, including the number of estimators, learning rate, subsample rate, and tree depth, across fivefold cross-validation to enhance the reliability of the results. The objective was to discover the optimal settings that minimize the negative mean squared error, a metric that captures the average squared difference between the estimated values and the actual value. The search across the hyperparameter space evaluated 54 unique candidates in 270 fitting iterations, rigorously assessing each combination to ensure the selection of the most predictive model configuration.
Long-Short Term Memory (LSTM) : LSTM neural network model was developed to predict the annual energy consumption of schools. This model represents a sophisticated form of recurrent neural network capable of learning order dependence in sequence prediction problems. Normalization of the data was paramount, achieved through the application of MinMaxScaler , which scaled the feature set and target variables to a bounded interval of [0, 1]. This scaling facilitated the neural network's convergence during training by providing numerical stability and improved efficiency. The dataset was then divided into a training subset accounting for 80% of the data and a testing subset forming the remaining 20%, with the intention of validating the model's predictive performance on unseen data. The LSTM model's architecture was constructed with an input layer designed to accept the reshaped feature set, followed by an LSTM layer with 50 units and 'relu' activation, and culminating in a Dense output layer for prediction. The model's learning process involved an 'adam' optimizer and mean squared error loss function across 50 epochs, with a batch size of 32, underscoring the iterative refinement of the model's weights.
Once the computer program for the corresponding machine learning algorithm is developed, afterwards, it is evaluated graphically and quantitatively. The graphical evaluation can be noted in Fig. 5 which shows the regression for all of the techniques. Figure 5 comprises four panels labeled (a), (b), (c), and (d), each representing a scatter plot comparing actual versus predicted annual energy consumption using different predictive models: Decision Tree, K-Nearest Neighbors (KNN), Gradient Boosting, and Long Short-Term Memory (LSTM) neural network, respectively. The actual annual consumption is plotted along the x-axis, while the predicted values are on the y-axis for each model. The presence of a trend line in each graph provides a reference for perfect prediction, where the predicted values would ideally match the actual consumption exactly. The points are color-coded, with blue dots representing training data and red crosses denoting testing data. This color scheme allows for immediate visual discrimination between the model's performance on the data it was trained on and its predictive power on new, unseen data. In the similar context, Fig. 5 e explains another way of presenting LSTM data points.
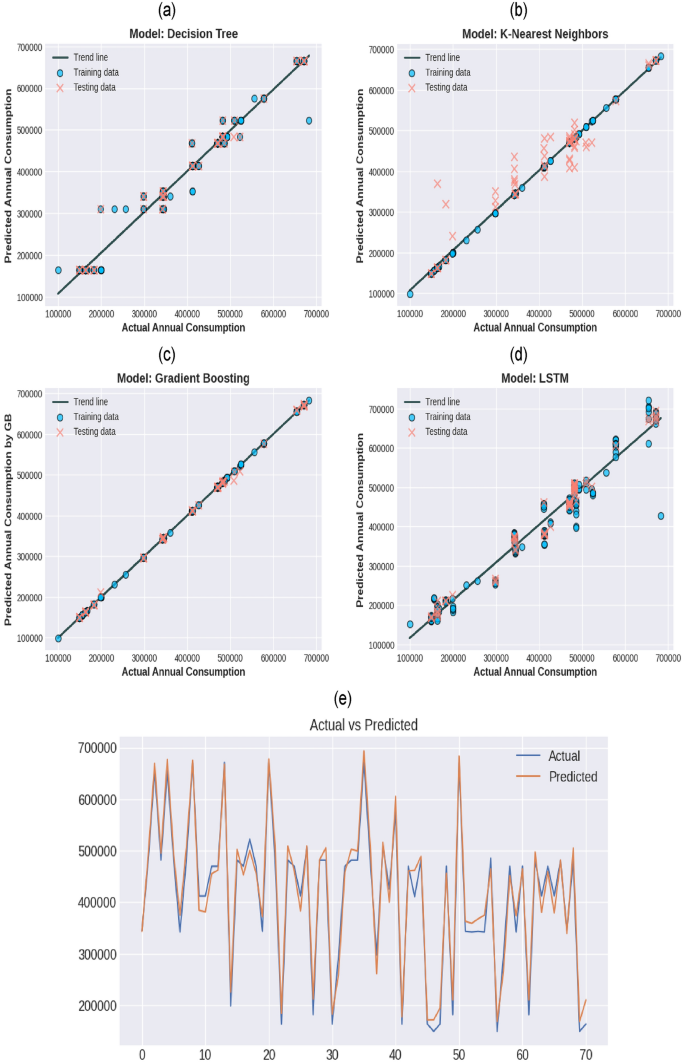
Regression fit of ( a ) Decision trees, ( b ) K-nearest neighbor, ( c ) gradient boosting, ( d ) LSTM. ( e ) LSTM representation in time series.
The Decision Tree model displays a reasonable spread of training data around the trend line but shows some variance in the predictions of testing data, as seen by the red crosses that stray further from the trend line. This might suggest a degree of overfitting to the training data, or it could simply reflect the model's inherent variance when dealing with more complex patterns not captured during training. In contrast, the KNN model exhibits a more consistent pattern of prediction across both training and testing datasets, with the majority of points closely aligned with the trend line. However, there are noticeable outliers in the testing data, which may imply that while the model generalizes well in most cases, it may be sensitive to certain data points or to noise within the dataset. The Gradient Boosting scatter plot demonstrates an impressive adherence of the data points to the trend line, with both training and testing data showing tight clustering around this line. This indicates that the model not only learned the training data effectively but also generalized well to the testing data, showcasing the strength of Gradient Boosting in capturing complex relationships within the data. Lastly, the LSTM neural network, known for its ability to capture sequential and temporal relationships, shows a distinctive pattern. Training data is well-fitted, and testing data, while slightly more scattered, still follows the trend line reasonably well. Notably, the LSTM shows more variation in the higher range of actual consumption values, which could indicate the model's different handling of more extreme values or its response to the nuances of the sequence-based data. When comparing the four models, Gradient Boosting (Panel c) seems to outperform the others in terms of the tightness of data points around the trend line, suggesting a high accuracy and good generalization. The KNN and LSTM model also generalizes well but with some noted exceptions.
Each model's unique characteristics influence its performance, with tree-based models like Decision Trees and Gradient Boosting often handling non-linear relationships effectively, while KNN relies on localized data patterns, and LSTM models excel in capturing temporal dynamics. The selection of the best model would therefore depend on the specific characteristics of the dataset and the performance metric of interest, whether it be accuracy, robustness, or the ability to generalize from limited data.
Our analysis compares four machine learning techniques across two phases: training and testing. Table 3 provides performance metrics such as Root Mean Square Error (RMSE), Mean Absolute Error (MAE), Mean Absolute Percentage Error (MAPE), and Coefficient of Determination (COD). The Decision Tree model exhibits strong performance on the training data, with a relatively low RMSE of 20,716.25 and MAE of 10,764.99, indicating good fit. The MAPE value of 3.580435 shows that, on average, the prediction error is about 3.58% of the actual value, which is respectable in practical applications. The high COD of 0.978928 for training and 0.980852 for testing indicates that the model explains a large portion of the variance in the data, confirming its effectiveness. The K-Nearest Neighbors model has significantly higher errors, with RMSE on training data as high as 38,429.4, which may be indicative of overfitting, considering the perfect COD of 0.934134. The testing phase does not fare much better, with a further increased RMSE of 40,603.04. This could be due to the model's sensitivity to the local structure of the training data, which doesn't generalize well. In contrast, Gradient Boosting outperforms other models during the training phase with a remarkably low RMSE of 374.7943 and an almost perfect COD, signifying that the model can almost perfectly predict the variations within the training dataset. However, the model's ability to generalize is not as clear without the testing phase COD, even though the testing RMSE increases to 3559.443. The LSTM network, designed to capture long-term dependencies and temporal dynamics in sequential data, shows good performance on both training and testing data. The RMSE and MAE values are moderate, with the model achieving a higher COD value on the testing set (0.975606) than on the training set (0.956432), which suggests it generalizes well and confirms its suitability for time-series forecasting like annual consumption. Our dataset's characteristics, with a substantial standard deviation, imply significant variability in annual consumption, which is a common challenge in time-series prediction. The performance metrics suggest that some models manage this variability better than others, with Gradient Boosting and LSTM standing out in terms of their ability to handle diverse data ranges, from the minimum consumption of approximately 99,274.95 to the maximum of 683,191.8. This evaluation demonstrates the importance of choosing the right model for time-series forecasting. While Decision Trees and KNN provided baseline performances, the Gradient Boosting and LSTM models showed advanced capabilities, which could be attributed to their sophisticated handling of non-linear relationships and temporal dependencies in the data.
Educational facilities, including schools, represent a major piece of infrastructure that significantly impacts economic, social and environment sustainable development. They contribute to approximately 37% of the carbon dioxide emissions associated with energy use and procedures. This paper presents an investigation study for prediction energy consumption of school buildings utilizing machine learning technologies. The methodology followed to meet the objectives of the study consists of four main steps. The first step begins with identifying the variable impacting consumption of energy in school buildings based on both review of the literature and meeting the experts. In the second step, the actual data on energy consumption was collected, filtered, and analyzed to develop robust machine-learning models. The third step comprises of separating the collected data into distinct subsets for training, validation, and testing. The fourth step involves the development and the investigation of several artificial intelligence-based models for energy consumption of educational buildings including decision trees, K-nearest neighbors, gradient boosting, and long-term memory networks.
The study revealed that the decision tree-based prediction model illustrates strong performance with an average prediction error of about 3.58%. The K-Nearest Neighbors model has significantly higher errors, with RMSE on training data as high as 38,429.4. Conversely, Gradient Boosting perfectly predicts energy consumption of school buildings. The performance metrics suggest that some models manage this variability better than others. Gradient boosting and LSTM stand out in terms of their ability to handle diverse data ranges, from the minimum consumption of approximately 99,274.95 to the maximum of 683,191.8. Furthermore, the relationship between the input factors and the annual consumption of energy of educational buildings illustrates that school sizes and AC capacities are the most impacted variable associated with higher energy consumption. While 'Type of School' is less direct or weaker correlation with 'Annual Consumption'.
It is essential to note that this study was carried out based on typical school facilities in hot climate. Thus, the results of this research may not be suitable to other school types and/or climates. Moreover, extended study can be conducted in the future to include different school types, structure system, and different climate.
Future research should focus on enhancing the role of educational buildings as interactive learning environments that promote sustainability principles. By using advanced AI-driven tools to optimize energy consumption, future studies could develop and implement smart, adaptive systems that not only manage energy use more efficiently but also serve as educational tools themselves. These systems could provide real-time data and simulations that allow students to observe, interact with, and learn from the building’s energy dynamics. This approach would not only improve the sustainability of educational facilities but also actively engage students with practical lessons on energy efficiency and environmental concepts, thereby embedding these critical concepts into their daily experiences and learning environments, as a part of lifelong learning.
Data availability
Data will be made available on request. Please request Professor Rasikh Tariq for the data simulation and Professor Awsan Mohammed for the experimentation.
Alfaoyzan, F. A. & Almasri, R. A. Benchmarking of energy consumption in higher education buildings in Saudi Arabia to be sustainable: Sulaiman Al-Rajhi University case. Energies 16 (3), 1204 (2023).
Article CAS Google Scholar
IEA. Buildings. Paris. License: IEA. https://www.iea.org/reports/buildings . CC BY 4.0 (2022).
Zhao, H. X. & Magoules, F. A review on the prediction of building energy consumption. Renew. Sustain. Energy Rev. 16 (6), 3586–3592 (2012).
Article Google Scholar
Gassar, A. A. A. & Cha, S. H. Energy prediction techniques for large-scale buildings towards a sustainable built environment: A review. Energy Build. 224 , 110238 (2020).
Asimakopoulos, D. N. & Doulamis, A. D. Predictive analytics for energy consumption in educational buildings: A review of modeling techniques. Sustain. Cities Soc. 50 , 101656 (2019).
Google Scholar
Debnath, K. B. & Mourshed, M. Forecasting methods in energy planning models. Renew. Sustain. Energy Rev. 88 , 297–325 (2018).
Alshibani, A. Prediction of the energy consumption of school buildings. Appl. Sci. 10 , 5885 (2020).
Kim, S.-G., Jung, J.-Y. & Sim, M. K. A two-step approach to solar power generation prediction based on weather data using machine learning. Sustainability 11 , 1501 (2019).
Blumsack, S. & Fernandez, A. Ready or not, here comes the smart grid!. Energy 37 , 61–68 (2012).
Zhong, H., Wang, J., Jia, H., Mu, Y. & Lv, S. Vector field-based support vector regression for building energy consumption prediction. Appl. Energy 242 , 403–414 (2019).
Article ADS Google Scholar
Zhao, Y., Zhang, C., Zhang, Y., Wang, Z. & Li, J. A review of data mining technologies in building energy systems: Load prediction, pattern identification, fault detection and diagnosis. Energy Built. Environ. 1 , 149–164 (2020).
Wang, J., Hou, J., Chen, J., Fu, Q. & Huang, G. Data mining approach for improving the optimal control of HVAC systems: An event-driven strategy. J. Build Eng. 39 , 102246 (2021).
Darwazeh, D., Duquette, J., Gunay, B., Wilton, I. & Shillinglaw, S. Review of peak load management strategies in commercial buildings. Sustain. Cities Soc. 77 , 103493 (2022).
Jin, W. et al. A novel building energy consumption prediction method using deep reinforcement learning with consideration of fluctuation points. J. Build. Eng. 63 , 105458. https://doi.org/10.1016/j.jobe.2022.105458 (2023).
Gellert, A., Fiore, U., Florea, A., Chis, R. & Palmieri, F. Forecasting electricity consumption and production in smart homes through statistical methods. Sustain. Cities Soc. 76 , 103426 (2022).
Zhang, W., Chen, Q., Yan, J., Zhang, S. & Xu, J. A novel asynchronous deep reinforcement learning model with adaptive early forecasting method and reward incentive mechanism for short-term load forecasting. Energy 236 , 121492. https://doi.org/10.1016/j.energy.2021.121492 (2021).
Purnell, K., Sinclair, M. & Gralton, A. Sustainable schools: Making energy efficiency a lifestyle priority. Aust. J. Environ. Educ. 20 (2), 81–91. https://doi.org/10.1017/S0814062600002226 (2004).
Rogora, A., & Dessì, V. Recent Examples of Low Energy and Sustainable Schools in Italy. In 22nd International Conference, PLEA 2005: Passive and Low Energy Architecture - Environmental Sustainability: The Challenge of Awareness in Developing Societies, Proceedings , 1, 275–280. https://re.public.polimi.it/bitstream/11311/693887/1/plea%202005-%20esempi%20di%20scuole.pdf (2005).
Zeiler W. & De Waard M. Some dutch examples of sustainable school concepts towards plus energy schools. In 28th Conference PLEA, Opportunities, Limits & Needs Towards an environmentally responsible architecture . Lima: Pontificia Universidad Católica del Perú (2012).
Ramírez-Montoya, M.S., Basabe, E., Carlos Arroyo, M., Patiño Zúñiga, I.A., & Portuguez Castro, M. Modelo abierto de pensamiento complejo para el futuro de la educación. Octaedro. https://hdl.handle.net/11285/652033 (2024).
Passa, J. & Rompf, D. Energy efficient sustainable schools in Canada South. J. Green Build. 2 (2), 14–30. https://doi.org/10.3992/jgb.2.2.14 (2007).
Golshan, M., Thoen, H. & Zeiler, W. Dutch sustainable schools towards energy positive. J. Build. Eng. 19 , 161–171. https://doi.org/10.1016/j.jobe.2018.05.002 (2018).
Zhang, Q., Koh, B. B., & Ahn, Y. H. Energy saving technologies and sustainable strategies of sustainable school buildings: A case study of isaac dickson elementary school. Int. J. Sustain. Build. Technol. Urban Dev. 11 (2), 94–111. https://doi.org/10.22712/susb.20200008 (2020).
Boeri, A. & Longo, D. Environmental quality and energy efficiency: sustainable school buildings design strategies. Int. J. Sustain. Dev. Plan. 8 (2), 140–157. https://doi.org/10.2495/SDP-V8-N2-140-157 (2013).
Msaddek, M. H., Moumni, Y., Ayari, A., El May, M. & Chenini, I. Artificial intelligence modelling framework for mapping groundwater vulnerability of fractured aquifer. Geocarto Int. 37 (25), 10480–10510. https://doi.org/10.1080/10106049.2022.2037729 (2022).
Tao, H. et al. Artificial intelligence models for suspended river sediment prediction: state-of-the art, modeling framework appraisal, and proposed future research directions. Eng. Appl. Comput. Fluid Mech. 15 (1), 1585–1612. https://doi.org/10.1080/19942060.2021.1984992 (2021).
Baki, S., Koutiva, I. & Makropoulos, C. A hybrid artificial intelligence modelling framework for the simulation of the complete, socio-technical, urban water system. Eng. Appl. Comput. Fluid Mech. 15 (1), 1585–1612. https://doi.org/10.1080/19942060.2021.1984992 (2012).
Méndez-Suárez, M., García-Fernández, F. & Gallardo, F. Artificial intelligence modelling framework for financial automated advising in the copper market. J. Open Innov. Technol. Mark. Complex. 5 (4), 81. https://doi.org/10.3390/joitmc5040081 (2019).
Reddy, R. S., Keesara, N., Pudi, V., & Garg, V. Plug load identification in educational buildings using machine learning algorithms. In Proceedings of BS2015: 14th conference of international building performance simulation association, Hyderabad, India , pp. 1940–1946 (2015).
López-Pérez, L. A. & Flores-Prieto, J. J. Adaptive thermal comfort approach to save energy in tropical climate educational building by artificial intelligence. Energy 263 , 125706. https://doi.org/10.1016/j.energy.2022.125706 (2023).
Hosseini, P., Nikbakht Naserabad, S., Keshavarzzadeh, A. H. & Ansari, N. Artificial intelligence-based tri-objective optimization of different demand load patterns on the optimal sizing of a smart educational buildings. Int. J. Energy Res. 46 (15), 21373–21396. https://doi.org/10.1002/er.8095 (2022).
Lee, M. J. & Zhang, R. Human-centric artificial intelligence of things-based indoor environment quality modeling framework for supporting student well-being in educational facilities. J. Comput. Civ. Eng. 38 (2), 04024002. https://doi.org/10.1061/JCCEE5.CPENG-5632 (2024).
Directive 2002/91/EC of the European parliament and of the council of 16 December 2002 on the energy performance of buildings. Off J Eur Union 65e71. https://doi.org/10.1039/ap9842100196 (2002).
Foucquier, A., Robert, S., Suard, F., Stephan, L. & Jay, A. State of the art in building modelling and energy performances prediction: A review. Renew. Sustain. Energy Rev. 23 , 272–288 (2013).
Foucquier, S., Robert, F., Suard, L. & Stephan, A. Jay, State of the art in building modelling and energy performances prediction: A review. Renew. Sustain. Energy Rev. 23 , 272–288 (2013).
Runge, J. & Zmeureanu, R. A review of deep learning techniques for forecasting energy use in buildings. Energies 14 , 1 (2021).
Fathi, S., Srinivasan, R., Fenner, A. & Fathi, S. Machine learning applications in urban building energy performance forecasting: A systematic review. Renew. Sustain. Energy Rev. 133 , 110287 (2020).
Chae, Y. T. et al. An artificial neural network model for forecasting sub-hourly electricity usage in commercial buildings[J]. Energy Build 111 , 184–194 (2016).
Biswas, M., Robinson, M. D. & Fumo, N. Prediction of residential building energy consumption: A neural network approach [J]. Energy 117 , 84–92 (2016).
Deb, C. et al. Forecasting diurnal cooling energy load for institutional buildings using artificial neural networks [J]. Energy Build 121 , 284–297 (2016).
Yan, K. et al. A hybrid LSTM neural network for energy consumption forecasting of individual households[J]. IEEE Access 7 , 157633–157642 (2019).
Zhong, H. et al. Vector field-based support vector regression for building energy consumption prediction[J]. Appl. Energy 242 , 403–414 (2019).
Wang, X. et al. Estimates of energy consumption in China using a self-adaptive multi-verse optimizer-based support vector machine with rolling cross-validation[J]. Energy 152 , 539–548 (2018).
Tabrizchi, H., Javidi, M. M. & Amirzadeh, V. Estimates of residential building energy consumption using a multi-verse optimizer-based support vector machine with k-fold cross-validation[J]. Evol. Syst. 12 , 755–767 (2019).
Iwafune, Y., Yagita, Y., & Ikegami, T., et al. Short-term forecasting of residential building load for distributed energy management[C]. In 2014 IEEE international energy conference (ENERGYCON). IEEE ; pp. 1197–204 (2014).
Albuquerque, P. C., Cajueiro, D. O. & Rossi, M. D. C. Machine learning models for forecasting power electricity consumption using a high dimensional dataset[J]. Expert. Syst. Appl. 187 , 115917 (2022).
Dong, Z. et al. Hourly energy consumption prediction of an office building based on ensemble learning and energy consumption pattern classification [J]. Energy Build 241 , 110929 (2021).
Cao, W. et al. Short-term energy consumption prediction method for educational buildings based on model integration. Energy 283 , 128580 (2023).
Faiq, M. et al. Prediction of energy consumption in campus buildings using long short-term memory. Alex. Eng. J. 67 , 65–76 (2023).
Álvarez, J.A., et al. Modeling of energy efficiency for residential buildings using artificial neuronal networks. Adv. Civ. Eng. (2018).
Beccali, M. et al. Artificial neural network decision support tool for assessment of the energy performance and the refurbishment actions for the non-residential building stock in southern Italy. Energy. 137 , 1201–1218 (2017).
Ahmad, M. W., Mourshed, M. & Rezgui, Y. Trees vs neurons: Comparison between random forest and ANN forhigh-resolution prediction of building energy consumption. Energy Build. 147 , 77–89 (2017).
Martellotta, F. et al. On the use of artificial neural networks to model household energy consumptions. Energy Proc. 126 , 250–257 (2017).
Williams, K. T. & Gomez, J. D. Predicting future monthly residential energy consumption using building characteristics and climate data: A statistical learning approach. Energy Build. 128 , 1–11 (2016).
Sun, C. & Han, Y. Constructing heating energy consumption forecast ANN model for office building in severe cold zone. Architectural 538 (10), 154–158 (2013).
Wong, S., Wan, K. K. & Lam, T. N. Artificial neural networks for energy analysis of office buildings with daylighting. Appl. Energy 87 (2), 551–557 (2010).
Catalina, T., Virgone, J. & Blanco, E. Development and validation of regression models to predict monthly heating demand for residential buildings. Energy Build. 40 (10), 1825–1832 (2008).
Alrashed, F. & Asif, M. Trends in residential energy consumption in Saudi Arabia with particular reference to the Eastern Province. J. Sustain. Dev. Energy Water Environ. Syst. 2 (4), 376–387 (2014).
Abdel-Aal, R. E., Al-Garni, A. Z. & Al-Nassar, Y. N. Modelling and forecasting monthly electric energy consumption in eastern Saudi Arabia using abductive networks. Energy 22 (9), 911–921 (1997).
Nasr, G. E., Badr, E. A. & Younes, M. R. Neural networks in forecasting electrical energy consumption: Univariate and multivariate approaches. Int. J. Energy Res. 26 (1), 67–78 (2002).
Meng, M., Shang, W. & Niu, D. Monthly electric energy consumption forecasting using multiwindow moving average and hybrid growth models. J. Appl. Math. 2014 (1), 243171 (2014).
Karatasou, S., Santamouris, M. & Geros, V. Modeling and predicting building’s energy use with artificial neural networks: Methods and results. Energy Build. 38 (8), 949–958 (2006).
Mena-Yedra, R., Rodriguez, F., Castilla, M. M., & Arahal, M. R. A Neural Network Model for Energy Consumption Prediction of CIESOL Bioclimatic Building. In International Joint Conference SOCO (2013).
Somu, N., Gauthama Raman, M. R. & Ramamritham, K. A hybrid model for building energy consumption forecasting using long short term memory networks. Appl. Energy 261 , 114131 (2020).
Ullah, F., & Min, U. Short-term prediction of residential power energy consumption via CNN and multilayer bi-directional LSTM networks. IEEE Access (2019).
Liu, B. & Chuanchuan, Fu. Arlene Bielefield and Yan Quan Liu “Forecasting of Chinese primary energy consumption in 2021 with GRU artificial neural network”. Energies 10 (10), 1453 (2017).
Khalil, A. J., Barhoom, A. M., Abu-Nasser, B. S., Musleh, M. M., & Abu-Naser, S. S. Energy efficiency predicting using artificial neural network. 3 (9), 1–1 (2019).
Rahman, A., Srikumar, V. & Smith, A. D. Predicting electricity consumption for commercial and residential buildings using deep recurrent neural networks. Appl. Energy 212 , 372–385 (2018).
Tartibu, L. K., & Kabengele, K. T. Forecasting net energy consumption of South Africa using artificial neural network. In: 2018 International Conference on the Industrial and Commercial Use of Energy (ICUE). IEEE, pp. 1–7 (2018).
Fayaz, M., Shah, H., Aseere, A. M., Mashwani, W. K. & Shah, A. S. A framework for prediction of household energy consumption using feed forward back propagation neural network. Technologies 7 (2), 30 (2019).
Mohammed, A., Alshibani, A., Alshamrani, O. & Hassanain, M. A regression-based model for estimating the energy consumption of school facilities in Saudi Arabia. Energy Build. 237 , 110809 (2021).
Breiman, L. Classification and regression trees (Routledge, 2017).
Book Google Scholar
Peterson, L. E. K-nearest neighbor. Scholarpedia 4 (2), 1883 (2009).
Chen, T., & Guestrin, C. Xgboost: A scalable tree boosting system. In Proceedings of the 22nd acm sigkdd international conference on knowledge discovery and data mining (pp. 785–794) (2016).
Graves, A., & Graves, A. Long short-term memory. Supervised sequence labelling with recurrent neural networks, 37–45 (2012).
Uzuner, S., & Çekmecelioğlu, D. Comparison of artificial neural networks (ANN) and adaptive neuro-fuzzy inference system (ANFIS) models in simulating polygalacturonase production (2016).
Sada, S. O., & Ikpeseni, S. C. Evaluation of ANN and ANFIS modeling ability in the prediction of AISI 1050 steel machining performance. Heliyon 7 (2) (2021).
Zhang, G., Patuwo, B. E. & Hu, M. Y. Forecasting with artificial neural networks: The state of the art. Int. J. Forecast. 14 , 35–62 (1998).
Chong, D. J. S., Chan, Y. J., Arumugasamy, S. K., Yazdi, S. K. & Lim, J. W. Optimisation and performance evaluation of response surface methodology (RSM), artificial neural network (ANN) and adaptive neuro-fuzzy inference system (ANFIS) in the prediction of biogas production from palm oil mill effluent (POME). Energy 266 , 126449 (2023).
Download references
Acknowledgements
The authors would like to thank Tecnológico de Monterrey for the financial support provided through the ‘Challenge-Based Research Funding Program 2023’, Project ID #IJXT070-23EG99001, titled ‘Complex Thinking Education for All (CTE4A): A Digital Hub and School for Lifelong Learners’. The authors would like to acknowledge the financial and the technical support of Writing Lab, Institute for the Future of Education, Tecnologico de Monterrey, Mexico, in the production of this work.
Author information
Authors and affiliations.
Institute for the Future of Education, Tecnologico de Monterrey, Ave. Eugenio Garza Sada 2501, 64849, Monterrey, NL, Mexico
Rasikh Tariq & Maria Soledad Ramírez-Montoya
Architectural Engineering and Construction Management Department, King Fahd University of Petroleum and Minerals, 31261, Dhahran, Saudi Arabia
Awsan Mohammed & Adel Alshibani
Interdisciplinary Research Center for Smart Mobility and Logistics, King Fahd University of Petroleum and Minerals, 31261, Dhahran, Saudi Arabia
Awsan Mohammed
Interdisciplinary Research Center of Construction and Building Materials, King Fahd University of Petroleum and Minerals, 34463, Dhahran, Saudi Arabia
Adel Alshibani
EGADE Business School, Tecnologico de Monterrey, 64849, Monterrey, NL, Mexico
Maria Soledad Ramírez-Montoya
You can also search for this author in PubMed Google Scholar
Contributions
Awsan Mohammed and Adel Alshibani gathered the experimental data, wrote the method, abstract, Introduction, and Current-State-of-the-Art. Rasikh Tariq analyzed the experimental data, applied machine learning algorithms and wrote the results section. Maria Soledad Ramírez-Montoya developed the complex modeling framework through AI, wrote the theoretical framework, and reviewed the manuscript.
Corresponding authors
Correspondence to Rasikh Tariq or Awsan Mohammed .
Ethics declarations
Competing interests.
The authors declare no competing interests.
Additional information
Publisher's note.
Springer Nature remains neutral with regard to jurisdictional claims in published maps and institutional affiliations.
Rights and permissions
Open Access This article is licensed under a Creative Commons Attribution 4.0 International License, which permits use, sharing, adaptation, distribution and reproduction in any medium or format, as long as you give appropriate credit to the original author(s) and the source, provide a link to the Creative Commons licence, and indicate if changes were made. The images or other third party material in this article are included in the article's Creative Commons licence, unless indicated otherwise in a credit line to the material. If material is not included in the article's Creative Commons licence and your intended use is not permitted by statutory regulation or exceeds the permitted use, you will need to obtain permission directly from the copyright holder. To view a copy of this licence, visit http://creativecommons.org/licenses/by/4.0/ .
Reprints and permissions
About this article
Cite this article.
Tariq, R., Mohammed, A., Alshibani, A. et al. Complex artificial intelligence models for energy sustainability in educational buildings. Sci Rep 14 , 15020 (2024). https://doi.org/10.1038/s41598-024-65727-5
Download citation
Received : 07 May 2024
Accepted : 24 June 2024
Published : 01 July 2024
DOI : https://doi.org/10.1038/s41598-024-65727-5
Share this article
Anyone you share the following link with will be able to read this content:
Sorry, a shareable link is not currently available for this article.
Provided by the Springer Nature SharedIt content-sharing initiative
- Higher education
- Educational innovation
- Educational buildings
- Energy consumption
- Machine learning
By submitting a comment you agree to abide by our Terms and Community Guidelines . If you find something abusive or that does not comply with our terms or guidelines please flag it as inappropriate.
Quick links
- Explore articles by subject
- Guide to authors
- Editorial policies
Sign up for the Nature Briefing: AI and Robotics newsletter — what matters in AI and robotics research, free to your inbox weekly.


IMAGES
VIDEO
COMMENTS
Correlational research is a type of study that explores how variables are related to each other. It can help you identify patterns, trends, and predictions in your data. In this guide, you will learn when and how to use correlational research, and what its advantages and limitations are. You will also find examples of correlational research questions and designs. If you want to know the ...
Revised on 5 December 2022. A correlational research design investigates relationships between variables without the researcher controlling or manipulating any of them. A correlation reflects the strength and/or direction of the relationship between two (or more) variables. The direction of a correlation can be either positive or negative.
A correlational study is an experimental design that evaluates only the correlation between variables. The researchers record measurements but do not control or manipulate the variables. Correlational research is a form of observational study. A correlation indicates that as the value of one variable increases, the other tends to change in a ...
Correlational research is a type of nonexperimental research in which the researcher measures two variables and assesses the statistical relationship (i.e., the correlation) between them with little or no effort to control extraneous variables. There are essentially two reasons that researchers interested in statistical relationships between ...
Mainly three types of correlational research have been identified: 1. Positive correlation:A positive relationship between two variables is when an increase in one variable leads to a rise in the other variable. A decrease in one variable will see a reduction in the other variable. For example, the amount of money a person has might positively ...
Correlational research design is a type of nonexperimental research that is used to examine the relationship between two or more variables. ... Examples of Correlational Research. ... Report the findings of your study in a research report or manuscript. Be sure to include the research question, methods, results, and conclusions. ...
Conclusion for Correlational Research. Correlational research serves as a powerful tool for uncovering connections between variables in the world around us. By examining the relationships between different factors, researchers can gain valuable insights into human behavior, health outcomes, market trends, and more.
Correlational Research - Steps & Examples. Published by Carmen Troy at August 14th, 2021 , Revised On August 29, 2023. In correlational research design, a researcher measures the association between two or more variables or sets of scores. A researcher doesn't have control over the variables. Example: Relationship between income and age.
In correlational research, you simply observe the two variables, their natural relationship, and their effects on each other. Observation takes place in the natural environment of the variables, and neither variable is manipulated. Data collection. Correlational research generally involves two or more sets of data.
A correlational study is a type of research design that looks at the relationships between two or more variables. Correlational studies are non-experimental, which means that the experimenter does not manipulate or control any of the variables. A correlation refers to a relationship between two variables. Correlations can be strong or weak and ...
Correlational research is a type of non-experimental research in which the researcher measures two variables and assesses the statistical relationship (i.e., the correlation) between them with little or no effort to control extraneous variables. There are many reasons that researchers interested in statistical relationships between variables ...
Correlational research is a type of non-experimental research in which the researcher measures two variables (binary or continuous) and assesses the statistical relationship (i.e., the correlation) between them with little or no effort to control extraneous variables. There are many reasons that researchers interested in statistical ...
Positive correlational research is a research method involving 2 variables that are statistically corresponding where an increase or decrease in 1 variable creates a like change in the other. An example is when an increase in workers' remuneration results in an increase in the prices of goods and services and vice versa.
There was a [negative or positive] correlation between the two variables, r (df) = [r value], p = [p-value]. Keep in mind the following when reporting Pearson's r in APA format: Round the p-value to three decimal places. Round the value for r to two decimal places. Drop the leading 0 for the p-value and r (e.g. use .77, not 0.77)
A correlation has direction and can be either positive or negative (note exceptions listed later). With a positive correlation, individuals who score above (or below) the average (mean) on one measure tend to score similarly above (or below) the average on the other measure. The scatterplot of a positive correlation rises (from left to right).
Using a correlation coefficient. In correlational research, you investigate whether changes in one variable are associated with changes in other variables.. Correlational research example You investigate whether standardized scores from high school are related to academic grades in college. You predict that there's a positive correlation: higher SAT scores are associated with higher college ...
Correlational research designs are often used in psychology, epidemiology, medicine and nursing. They show the strength of correlation that exists between the variables within a population. For this reason, these studies are also known as ecological studies. Correlational research design methods are characterized by such traits:
Here are a few examples of how correlation analysis could be applied in different contexts: Education: A researcher might want to determine if there's a relationship between the amount of time students spend studying each week and their exam scores. The two variables would be "study time" and "exam scores".
Correlational research is a type of nonexperimental research in which the researcher measures two variables and assesses the statistical relationship (i.e., the correlation) between them with little or no effort to control extraneous variables. There are essentially two reasons that researchers interested in statistical relationships between ...
Types. A positive correlation is a relationship between two variables in which both variables move in the same direction. Therefore, one variable increases as the other variable increases, or one variable decreases while the other decreases. An example of a positive correlation would be height and weight. Taller people tend to be heavier.
Example: Correlational research To study whether consuming violent media is related to aggression, you collect data on children's video game use and their behavioral tendencies. You ask parents to report the number of weekly hours their child spent playing violent video games, and you survey parents and teachers on the children's behaviors.
Correlational research is a critical form of research that researchers ought to deploy in the mid-late stages of the entire research process. It is especially necessary if it concerns a new topic. Sequentially following descriptive research, which follows exploratory research, correlational research studies the relationship between two variables.
Research Methods. Correlational research is a type of scientific investigation in which a researcher looks at the relationships between variables but does not vary, manipulate, or control them. It can be a useful research method for evaluating the direction and strength of the relationship between two or more different variables.
For example, the number of years in school might not correlate with the number of letters in a person's name. Practical Applications. Correlation analysis in QuestionPro can be used for various practical applications, such as: Market Research: Measure the effectiveness of marketing campaigns by correlating advertising spending with sales ...
Previously observed negative correlations between sample size and effect size (n-ES correlation) in psychological research have been interpreted as evidence for publication bias and related undesirable biases. Here, we present two studies aimed at better understanding to what extent negative n-ES correlations reflect such biases or might be explained by unproblematic adjustments of sample size ...
a summary of research results; their significance for practical application; strengths and weaknesses of the work; correlation to similar investigations in the sphere mentioned; suggestions for future research. If speaking about a short version of the structure, it may consist of 3 parts: A research question. A conclusion. Recommendations. As ...
To fill the gap, this study examined a small-scale blasting test by investigating the velocity of the cracks implementing the digital image correlation (DIC) technique and avoiding contact methods such as strain gauges. An ultra-high-speed camera (UHSC) was used to record the blasting test in a single blasthole rock-like sample with a PETN cord.
For example, 'Total Built Area' vs. 'Total Roof Area' displays a linear trend, indicating a strong positive correlation, as expected since larger built areas typically result in larger roof areas.
Save & Close Corporate Research & Development Report 2022. Deloitte's ongoing focus on research and development (R&D) is what has inspired us to carry out this survey - our first research project of this kind since the outbreak of COVID-19 in 2020. Dealmaking in Central Europe. What is the most frequently used purchase price mechanism for M ...
The World Economic Forum's Top 10 Emerging Technologies of 2024 report lists this year's most impactful emerging technologies. The list includes ways artificial intelligence is accelerating scientific research with a focus on applications in health, communication, infrastructure and sustainability. ... Digital twins, for example, can be used to ...