Cloud Computing
- First Online: 13 February 2020

Cite this chapter
- Ali Sunyaev 2
8489 Accesses
64 Citations
3 Altmetric
Cloud computing is an evolution of information technology and a dominant business model for delivering IT resources. With cloud computing, individuals and organizations can gain on-demand network access to a shared pool of managed and scalable IT resources, such as servers, storage, and applications. Recently, academics as well as practitioners have paid a great deal of attention to cloud computing. We rely heavily on cloud services in our daily lives, e.g., for storing data, writing documents, managing businesses, and playing games online. Cloud computing also provides the infrastructure that has powered key digital trends such as mobile computing, the Internet of Things, big data, and artificial intelligence, thereby accelerating industry dynamics, disrupting existing business models, and fueling the digital transformation. Still, cloud computing not only provides a vast number of benefits and opportunities; it also comes with several challenges and concerns, e.g., regarding protecting customers’ data.
This is a preview of subscription content, log in via an institution to check access.
Access this chapter
- Available as PDF
- Read on any device
- Instant download
- Own it forever
- Durable hardcover edition
- Dispatched in 3 to 5 business days
- Free shipping worldwide - see info
Tax calculation will be finalised at checkout
Purchases are for personal use only
Institutional subscriptions
Unable to display preview. Download preview PDF.
Ahmed M, Litchfield AT (2018) Taxonomy for identification of security issues in cloud computing environments. J Comput Inf Syst 58(1):79–88
Google Scholar
Anisetti M, Ardagna C, Damiani E, Gaudenzi F (2017) A semi-automatic and trustworthy scheme for continuous cloud service certification. IEEE Trans Serv Comput 10(1):1–14
Annette JR, Banu WA, Chandran PS (2015) Rendering-as-a-service: taxonomy and comparison. Procedia Comput Sci 50:276–281
Article Google Scholar
Apps Run The World (2017) Cloud applications revenue from leading vendors worldwide in 2015 and 2016 (in million U.S. dollars). https://www.statista.com/statistics/475844/cloud-applications-revenues-worldwide-by-vendor/ . Accessed 11 Dec 2018
Arasaratnam O (2011) Introduction to cloud computing. In: Halpert B (ed) Auditing cloud computing, a security and privacy guide. Wiley, Hoboken, NJ, pp 1–13
Bajaj A (2000) A study of senior information systems managers decision models in adopting new computing architectures. J AIS 1(1es):4
Baldwin LP, Irani Z, Love PED (2001) Outsourcing information systems: drawing lessons from a banking case study. Eur J Inf Syst 10(1):15–24
Bayramusta M, Nasir VA (2016) A fad or future of IT?: a comprehensive literature review on the cloud computing research. Int J Inf Manag 36(4):635–644
Benlian A, Hess T (2011) Opportunities and risks of software-as-a-service: findings from a survey of IT executives. Decis Support Syst 52(1):232–246
Benlian A, Kettinger WJ, Sunyaev A, Winkler TJ (2018) Special section: The transformative value of cloud computing: a decoupling, platformization, and recombination theoretical framework. J Manag Inf Syst 35(3):719–739
Bennett C, Timbrell GT (2000) Application service providers: will they succeed? Inf Syst Front 2(2):195–211
Berman F, Hey T (2004) The scientific imperative. In: Foster I, Kesselman C (eds) The grid 2: blueprint for a new computing infrastructure. The Morgan Kaufmann series in computer architecture and design. Morgan Kaufman, San Francisco, CA, pp 13–24
Chapter Google Scholar
Bharadwaj A, El Sawy OA, Pavlou PA, Venkatraman N (2013) Digital business strategy: toward a next generation of insights. MIS Q 37(2):471–482
Bhattacherjee A, Park SC (2014) Why end-users move to the cloud: a migration-theoretic analysis. Eur J Inf Syst 23(3):357–372
Böhm M, Leimeister S, Riedl C, Krcmar H (2011) Cloud computing – outsourcing 2.0 or a new business model for IT provisioning? In: Keuper F, Oecking C, Degenhardt A (eds) Application management: challenges – service creation – strategies. Gabler, Wiesbaden, pp 31–56
Bunker G, Thomson D (2006) Delivering utility computing: business-driver IT optimization. Wiley, Chichester
Burda D, Teuteberg F (2014) The role of trust and risk perceptions in cloud archiving — results from an empirical study. J High Technol Manag Res 25(2):172–187
Burnham TA, Frels JK, Mahajan V (2003) Consumer switching costs: a typology, antecedents, and consequences. J Acad Mark Sci 31(2):109
Buyya R, Yeo CS, Venugopal S, Broberg J, Brandic I (2009) Cloud computing and emerging IT platforms: vision, hype, and reality for delivering computing as the 5th utility. Futur Gener Comput Syst 25(6):599–616
Cafaro M, Aloisio G (2011) Grids, clouds, and virtualization. In: Cafaro M, Aloisio G (eds) Grids, clouds and virtualization. Computer communications and networks. Springer, London, pp 1–21
Carr N (2008) The big switch: rewiring the world, from Edison to Google. W.W. Norton, New York, NY
Cloudscene (2018) Top ten data center operators in North America, EMEA, Oceania and Asia for the January to March 2018 period. https://cloudscene.com/top10 . Accessed 11 Dec 2018
Cusumano M (2010) Cloud computing and SaaS as new computing platforms. Commun ACM 53(4):27–29
Dašić P, Dašić J, Crvenković B (2016) Service models for cloud computing: search as a service (SaaS). Int J Eng Technol (IJET) 8(5):2366–2373
Dillon T, Wu C, Chang E (2010) Cloud computing: issues and challenges. Paper presented at the 24th IEEE international conference on advanced information networking and applications, Perth, WA, 20–23 Apr 2010
Doelitzscher F, Fischer C, Moskal D, Reich C, Knahl M, Clarke N (2012) Validating cloud infrastructure changes by cloud audits. Paper presented at the 8th IEEE world congress on services, Honolulu, HI, 24–29 June 2012
Durkee D (2010) Why cloud computing will never be free. Commun ACM 53(5):62–69
ENISA (2012) Cloud computing – benefits, risks and recommendations for information security. European network and security agency. https://resilience.enisa.europa.eu/cloud-security-and-resilience/publications/cloud-computing-benefits-risks-and-recommendations-for-information-security . Accessed 17 Sept 2019
Fernandes DAB, Soares LFB, Gomes JV, Freire MM, Inácio PRM (2014) Security issues in cloud environments: a survey. Int J Inf Secur 13(2):113–170
Foster I, Kesselman C (2004) The grid 2: blueprint for a new computing infrastructure, 2nd edn. Elsevier, San Francisco, CA
Foster I, Kesselman C, Tuecke S (2001) The anatomy of the grid: enabling scalable virtual organizations. Int J High Perform Comput Appl 15(3):200–222
Foster I, Zhao Y, Raicu I, Lu S (2008) Cloud computing and grid computing 360-degree compared. Paper presented at the grid computing environments workshop, Austin, TX, 12–16 Nov 2008
Gao F, Thiebes S, Sunyaev A (2018) Rethinking the meaning of cloud computing for health care: a taxonomic perspective and future research directions. J Med Internet Res 20(7):e10041
Gartner (2018a) Revenue of cloud computing worldwide. https://de.statista.com/statistik/daten/studie/195760/umfrage/umsatz-mit-cloud-computing-weltweit/ . Accessed 9 Dec 2018
Gartner (2018b) Revenues from public cloud infrastructure as a service (IaaS) market worldwide from 2015 to 2017, by vendor (in million U.S. dollars). https://www.statista.com/statistics/754826/worldwide-public-cloud-infrastructure-services-vendor-revenues/ . Accessed 11 Dec 2018
Gefen D, Karahanna E, Straub DW (2003) Trust and TAM in online shopping: an integrated model. MIS Q 27(1):51–90
Gonzalez R, Gasco J, Llop J (2009) Information systems outsourcing reasons and risks: an empirical study. Int J Soc Sci 4(3):180–191
Grover V, Kohli R (2012) Cocreating IT value: new capabilities and metrics for multifirm environments. MIS Q 36(1):225–232
Grozev N, Buyya R (2014) Inter-cloud architectures and application brokering: taxonomy and survey. Softw Pract Exp 44(3):369–390
Hentschel R, Leyh C, Petznick A (2018) Current cloud challenges in Germany: the perspective of cloud service providers. J Cloud Comput 7(1):5
Hess T, Matt C, Benlian A, Wiesböck F (2016) Options for formulating a digital transformation strategy. MIS Q Exec 15(2):123–139
Höfer CN, Karagiannis G (2011) Cloud computing services: taxonomy and comparison. J Internet Serv Appl 2(2):81–94
Hogendorn C (2011) Excessive(?) entry of national telecom networks, 1990–2001. Telecommun Policy 35(11):920–932
Hussain SA, Fatima M, Saeed A, Raza I, Shahzad RK (2017) Multilevel classification of security concerns in cloud computing. Appl Comput Inform 13(1):57–65
ISO (2004) Conformity assessment – vocabulary and general principles. https://www.iso.org/standard/29316.html . Accessed 17 Sept 2019
ITCandor (2018) Distribution of cloud platform as a service (PaaS) market revenues worldwide from 2015 to June 2018, by vendor. https://www.statista.com/statistics/540521/worldwide-cloud-platform-revenue-share-by-vendor/ . Accessed 11 Dec 2018
Iyer B, Henderson JC (2010) Preparing for the future: understanding the seven capabilities of cloud computing. MIS Q Exec 9(2):117–131
Jones MA, Mothersbaugh DL, Beatty SE (2002) Why customers stay: measuring the underlying dimensions of services switching costs and managing their differential strategic outcomes. J Bus Res 55(6):441–450
Kern T, Lacity MC, Willcocks L (2002) Netsourcing: renting business applications and services over a network. Prentice-Hall, New York, NY
Khan N, Al-Yasiri A (2016) Identifying cloud security threats to strengthen cloud computing adoption framework. Procedia Comput Sci 94:485–490
Killalea T (2008) Meet the virts. ACM Queue 6(1):14–18
Kleinrock L (2005) A vision for the internet. ST J Res 2(1):4–5
Kouatli I (2014) A comparative study of the evolution of vulnerabilities in IT systems and its relation to the new concept of cloud computing. J Manag Hist 20(4):409–433
Kroeker KL (2011) Grid computing’s future. Commun ACM 54(3):15–17
Krotsiani M, Spanoudakis G, Kloukinas C (2015) Monitoring-based certification of cloud service security. Paper presented at the OTM confederated international conferences “On the move to meaningful internet systems”, Rhodes, 26–30 Oct 2015
Kumar N, DuPree L (2011) Protection and privacy of information assets in the cloud. In: Halpert B (ed) Auditing cloud computing, a security and privacy guide. Wiley, Hoboken, NJ, pp 97–128
Kunz I, Stephanow P (2017) A process model to support continuous certification of cloud services. Paper presented at the 31st IEEE international conference on advanced information networking and applications, Taipei, 27–29 Mar 2017
Lansing J, Benlian A, Sunyaev A (2018) ‘Unblackboxing’ decision makers’ interpretations of IS certifications in the context of cloud service certifications. J Assoc Inf Syst 19(11):1064–1096
Lee C, Wan G (2010) Including subjective norm and technology trust in the technology acceptance model: a case of e-ticketing in China. SIGMIS Database 41(4):40–51
Leimeister S, Böhm M, Riedl C, Krcmar H (2010) The business perspective of cloud computing: actors, roles and value networks. Paper presented at the 18th European conference on information systems, Pretoria, 7–9 June 2010
Lins S, Grochol P, Schneider S, Sunyaev A (2016a) Dynamic certification of cloud services: trust, but verify! IEEE Secur Priv 14(2):66–71
Lins S, Teigeler H, Sunyaev A (2016b) Towards a bright future: enhancing diffusion of continuous cloud service auditing by third parties. Paper presented at the 24th European conference on information systems, Istanbul, 12–15 June 2016
Lins S, Schneider S, Sunyaev A (2018) Trust is good, control is better: creating secure clouds by continuous auditing. IEEE Trans Cloud Comput 6(3):890–903
Lins S, Schneider S, Sunyaev A (2019a) Cloud-Service-Zertifizierung: Ein Rahmenwerk und Kriterienkatalog zur Zertifizierung von Cloud-Services, 2nd edn. Springer, Berlin
Book Google Scholar
Lins S, Schneider S, Szefer J, Ibraheem S, Sunyaev A (2019b) Designing monitoring systems for continuous certification of cloud services: deriving meta-requirements and design guidelines. Commun Assoc Inf Syst 44:460–510
Linthicum DS (2009) Cloud computing and SOA convergence in your enterprise: a step-by-step guide: how to use SaaS, SOA, Mashups, and web 2.0 to break down the IT gates. Addison-Wesley, Boston, MA
Liu F, Tong J, Mao J, Bohn R, Messina J, Badger L, Leaf D (2011) NIST cloud computing reference architecture. https://bigdatawg.nist.gov/_uploadfiles/M0008_v1_7256814129.pdf . Accessed 11 Dec 2018
Luftman J, Zadeh HS (2011) Key information technology and management issues 2010–11: an international study. J Inf Technol 26(3):193–204
Malluhi Q, Khan KM (2013) Trust in cloud services: providing more controls to clients. Computer 46(7):94–96
Marston S, Li Z, Bandyopadhyay S, Zhang J, Ghalsasi A (2011) Cloud computing — the business perspective. Decis Support Syst 51(1):176–189
Mell P, Grance T (2011) The NIST definition of cloud computing. https://nvlpubs.nist.gov/nistpubs/Legacy/SP/nistspecialpublication800-145.pdf . Accessed 17 Sept 2019
NIST Cloud Computing Security Working Group (2013) NIST cloud computing security reference architecture. https://csrc.nist.gov/publications/detail/sp/500-299/draft . Accessed 10 Dec 2018
Pahl C (2015) Containerization and the PaaS cloud. IEEE Cloud Comput 2(3):24–31
Park S-T, Park E-M, Seo J-H, Li G (2016) Factors affecting the continuous use of cloud service: focused on security risks. Clust Comput 19(1):485–495
Sarkar P, Young L (2011) Sailing the cloud: a case study of perceptions and changing roles in an Australian University. Paper presented at the 19th European conference on information systems, Helsinki, 9–11 June 2011
Schneider S, Sunyaev A (2016) Determinant factors of cloud-sourcing decisions: reflecting on the IT outsourcing literature in the era of cloud computing. J Inf Technol 31(1):1–31
Schneider S, Lansing J, Gao F, Sunyaev A (2014) A taxonomic perspective on certification schemes: development of a taxonomy for cloud service certification criteria. Paper presented at the 47th Hawaii international conference on system sciences, Waikoloa, HI, 6–9 Jan 2014
Schneider S, Wollersheim J, Krcmar H, Sunyaev A (2018) How do requirements evolve over time? a case study investigating the role of context and experiences in the evolution of enterprise software requirements. J Inf Technol 33(2):151–170
Schwarz A, Jayatilaka B, Hirschheim R, Goles T (2009) A conjoint approach to understanding IT application services outsourcing. J Assoc Inf Syst 10(10):1
Schwiegelshohn U, Badia RM, Bubak M, Danelutto M, Dustdar S, Gagliardi F, Geiger A, Hluchy L, Kranzlmüller D, Laure E, Priol T, Reinefeld A, Resch M, Reuter A, Rienhoff O, Rüter T, Sloot P, Talia D, Ullmann K, Yahyapour R (2010) Perspectives on grid computing. Futur Gener Comput Syst 26(8):1104–1115
Seethamraju R (2013) Determinants of SaaS ERP systems adoption. Paper presented at the 17th Pacific Asia conference on information systems, Jeju Island, 18–22 June 2013
Sehgal NK, Sohoni S, Xiong Y, Fritz D, Mulia W, Acken JM (2011) A cross section of the issues and research activities related to both information security and cloud computing. IETE Tech Rev 28(4):279–291
Sharma D, Dhote C, Potey MM (2016) Identity and access management as security-as-a-service from clouds. Procedia Comput Sci 79:170–174
Singh A, Chatterjee K (2017) Cloud security issues and challenges: a survey. J Netw Comput Appl 79(C):88–115
Singh S, Jeong Y-S, Park JH (2016) A survey on cloud computing security. J Netw Comput Appl 75(C):200–222
Soares J, Carapinha J, Melo M, Monteiro R, Sargento S (2011) Building virtual private clouds with network-aware cloud. Paper presented at the 5th international conference on advanced engineering computing and applications in sciences, Lisbon, 20–25 Nov 2011
Stephanow P, Banse C (2017) Evaluating the performance of continuous test-based cloud service certification. Paper presented at the 17th IEEE/ACM international symposium on cluster, cloud and grid computing, Madrid, 14–17 May 2017
Stephanow P, Khajehmoogahi K (2017) Towards continuous security certification of software-as-a-service applications using web application testing techniques. Paper presented at the 31st IEEE international conference on advanced information networking and applications, Taipei, 27–29 Mar 2017
Stephanow P, Banse C, Schütte J (2016) Generating threat profiles for cloud service certification systems. Paper presented at the 17th IEEE international symposium on high assurance systems engineering, Orlando, FL, 7–9 Jan 2016
Subashini S, Kavitha V (2011) A survey on security issues in service delivery models of cloud computing. J Netw Comput Appl 34(1):1–11
Sunyaev A, Schneider S (2013) Cloud services certification. Commun ACM 56(2):33–36
Susarla A, Barua A, Whinston AB (2003) Understanding the service component of application service provision: an empirical analysis of satisfaction with ASP services. MIS Q 27(1):91–123
Tan W, Fan Y, Ghoneim A, Hossain MA, Dustdar S (2016) From the service-oriented architecture to the web API economy. IEEE Internet Comput 20(4):64–68
Teigeler H, Lins S, Sunyaev A (2018) Drivers vs. inhibitors – what clinches continuous service certification adoption by cloud service providers? Paper presented at the 51th Hawaii international conference on system sciences, Hilton Waikoloa Village, HI, 3–6 Jan 2018
Thiebes S, Kleiber G, Sunyaev A (2017) Cancer genomics research in the cloud: a taxonomy of genome data sets. Paper presented at the 4th international workshop on genome privacy and security, Orlando, FL, 15 Oct 2017
Tiwana A, Konsynski B, Bush AA (2010) Research commentary—platform evolution: coevolution of platform architecture, governance, and environmental dynamics. Inf Syst Res 21(4):675–687
Top Threats Working Group (2016) The treacherous 12. Cloud computing top threats in 2016. https://downloads.cloudsecurityalliance.org/assets/research/top-threats/Treacherous-12_Cloud-Computing_Top-Threats.pdf . Accessed 17 Sept 2019
Trenz M, Huntgeburth JC, Veit DJ (2013) The role of uncertainty in cloud computing continuance: antecedents, mitigators, and consequences. Paper presented at the 21st European conference on information systems, Utrecht, 5–8 June 2013
Trenz M, Huntgeburth J, Veit D (2018) Uncertainty in cloud service relationships: uncovering the differential effect of three social influence processes on potential and current users. Inf Manag 55(8):971–983
Venkatesh V, Morris MG, Davis GB, Davis FD (2003) User acceptance of information technology: toward a unified view. MIS Q 27(3):425–478
Venters W, Whitley EA (2012) A critical review of cloud computing: researching desires and realities. J Inf Technol 27(3):179–197
Voorsluys W, Broberg J, Buyya R (2011) Introduction to cloud computing. In: Buyya R, Broberg J, Goscinski A (eds) Cloud computing. Wiley, Hoboken, NJ, pp 3–42
Wang B, Li B, Li H (2014) Oruta: privacy-preserving public auditing for shared data in the cloud. IEEE Trans Cloud Comput 2(1):43–56
Article MathSciNet Google Scholar
Weinhardt C, Anandasivam A, Blau B, Borissov N, Meinl T, Michalk W, Stößer J (2009) Cloud computing–a classification, business models, and research directions. Bus Inf Syst Eng 1(5):391–399
Whitman M, Mattord H (2011) Cloud-based IT governance. In: Halpert B (ed) Auditing cloud computing: a security and privacy guide. Wiley, Hoboken, NJ, pp 33–55
Windhorst I, Sunyaev A (2013) Dynamic certification of cloud services. Paper presented at the 8th international conference on availability, reliability and security, Regensburg, 2–6 Sept 2013
Yoo Y, Henfridsson O, Lyytinen K (2010) Research commentary—the new organizing logic of digital innovation: an agenda for information systems research. Inf Syst Res 21(4):724–735
Further Reading
Lins S, Schneider S, Sunyaev A (2019) Cloud-Service-Zertifizierung: Ein Rahmenwerk und Kriterienkatalog zur Zertifizierung von Cloud-Services, 2nd edn. Springer, Berlin
Download references
Author information
Authors and affiliations.
Institute of Applied Informatics and Formal Description Methods, Karlsruhe Institute of Technology, Karlsruhe, Germany
Ali Sunyaev
You can also search for this author in PubMed Google Scholar
Rights and permissions
Reprints and permissions
Copyright information
© 2020 Springer Nature Switzerland AG
About this chapter
Sunyaev, A. (2020). Cloud Computing. In: Internet Computing. Springer, Cham. https://doi.org/10.1007/978-3-030-34957-8_7
Download citation
DOI : https://doi.org/10.1007/978-3-030-34957-8_7
Published : 13 February 2020
Publisher Name : Springer, Cham
Print ISBN : 978-3-030-34956-1
Online ISBN : 978-3-030-34957-8
eBook Packages : Computer Science Computer Science (R0)
Share this chapter
Anyone you share the following link with will be able to read this content:
Sorry, a shareable link is not currently available for this article.
Provided by the Springer Nature SharedIt content-sharing initiative
- Publish with us
Policies and ethics
- Find a journal
- Track your research
cloud computing Recently Published Documents
Total documents.
- Latest Documents
- Most Cited Documents
- Contributed Authors
- Related Sources
- Related Keywords
Simulation and performance assessment of a modified throttled load balancing algorithm in cloud computing environment
<span lang="EN-US">Load balancing is crucial to ensure scalability, reliability, minimize response time, and processing time and maximize resource utilization in cloud computing. However, the load fluctuation accompanied with the distribution of a huge number of requests among a set of virtual machines (VMs) is challenging and needs effective and practical load balancers. In this work, a two listed throttled load balancer (TLT-LB) algorithm is proposed and further simulated using the CloudAnalyst simulator. The TLT-LB algorithm is based on the modification of the conventional TLB algorithm to improve the distribution of the tasks between different VMs. The performance of the TLT-LB algorithm compared to the TLB, round robin (RR), and active monitoring load balancer (AMLB) algorithms has been evaluated using two different configurations. Interestingly, the TLT-LB significantly balances the load between the VMs by reducing the loading gap between the heaviest loaded and the lightest loaded VMs to be 6.45% compared to 68.55% for the TLB and AMLB algorithms. Furthermore, the TLT-LB algorithm considerably reduces the average response time and processing time compared to the TLB, RR, and AMLB algorithms.</span>
An improved forensic-by-design framework for cloud computing with systems engineering standard compliance
Reliability of trust management systems in cloud computing.
Cloud computing is an innovation that conveys administrations like programming, stage, and framework over the web. This computing structure is wide spread and dynamic, which chips away at the compensation per-utilize model and supports virtualization. Distributed computing is expanding quickly among purchasers and has many organizations that offer types of assistance through the web. It gives an adaptable and on-request administration yet at the same time has different security dangers. Its dynamic nature makes it tweaked according to client and supplier’s necessities, subsequently making it an outstanding benefit of distributed computing. However, then again, this additionally makes trust issues and or issues like security, protection, personality, and legitimacy. In this way, the huge test in the cloud climate is selecting a perfect organization. For this, the trust component assumes a critical part, in view of the assessment of QoS and Feedback rating. Nonetheless, different difficulties are as yet present in the trust the board framework for observing and assessing the QoS. This paper talks about the current obstructions present in the trust framework. The objective of this paper is to audit the available trust models. The issues like insufficient trust between the supplier and client have made issues in information sharing likewise tended to here. Besides, it lays the limits and their enhancements to help specialists who mean to investigate this point.
Cloud Computing Adoption in the Construction Industry of Singapore: Drivers, Challenges, and Strategies
An extensive review of web-based multi granularity service composition.
The paper reviews the efforts to compose SOAP, non-SOAP and non-web services. Traditionally efforts were made for composite SOAP services, however, these efforts did not include the RESTful and non-web services. A SOAP service uses structured exchange methodology for dealing with web services while a non-SOAP follows different approach. The research paper reviews the invoking and composing a combination of SOAP, non-SOAP, and non-web services into a composite process to execute complex tasks on various devices. It also shows the systematic integration of the SOAP, non-SOAP and non-web services describing the composition of heterogeneous services than the ones conventionally used from the perspective of resource consumption. The paper further compares and reviews different layout model for the discovery of services, selection of services and composition of services in Cloud computing. Recent research trends in service composition are identified and then research about microservices are evaluated and shown in the form of table and graphs.
Integrated Blockchain and Cloud Computing Systems: A Systematic Survey, Solutions, and Challenges
Cloud computing is a network model of on-demand access for sharing configurable computing resource pools. Compared with conventional service architectures, cloud computing introduces new security challenges in secure service management and control, privacy protection, data integrity protection in distributed databases, data backup, and synchronization. Blockchain can be leveraged to address these challenges, partly due to the underlying characteristics such as transparency, traceability, decentralization, security, immutability, and automation. We present a comprehensive survey of how blockchain is applied to provide security services in the cloud computing model and we analyze the research trends of blockchain-related techniques in current cloud computing models. During the reviewing, we also briefly investigate how cloud computing can affect blockchain, especially about the performance improvements that cloud computing can provide for the blockchain. Our contributions include the following: (i) summarizing the possible architectures and models of the integration of blockchain and cloud computing and the roles of cloud computing in blockchain; (ii) classifying and discussing recent, relevant works based on different blockchain-based security services in the cloud computing model; (iii) simply investigating what improvements cloud computing can provide for the blockchain; (iv) introducing the current development status of the industry/major cloud providers in the direction of combining cloud and blockchain; (v) analyzing the main barriers and challenges of integrated blockchain and cloud computing systems; and (vi) providing recommendations for future research and improvement on the integration of blockchain and cloud systems.
Cloud Computing and Undergraduate Researches in Universities in Enugu State: Implication for Skills Demand
Cloud building block chip for creating fpga and asic clouds.
Hardware-accelerated cloud computing systems based on FPGA chips (FPGA cloud) or ASIC chips (ASIC cloud) have emerged as a new technology trend for power-efficient acceleration of various software applications. However, the operating systems and hypervisors currently used in cloud computing will lead to power, performance, and scalability problems in an exascale cloud computing environment. Consequently, the present study proposes a parallel hardware hypervisor system that is implemented entirely in special-purpose hardware, and that virtualizes application-specific multi-chip supercomputers, to enable virtual supercomputers to share available FPGA and ASIC resources in a cloud system. In addition to the virtualization of multi-chip supercomputers, the system’s other unique features include simultaneous migration of multiple communicating hardware tasks, and on-demand increase or decrease of hardware resources allocated to a virtual supercomputer. Partitioning the flat hardware design of the proposed hypervisor system into multiple partitions and applying the chip unioning technique to its partitions, the present study introduces a cloud building block chip that can be used to create FPGA or ASIC clouds as well. Single-chip and multi-chip verification studies have been done to verify the functional correctness of the hypervisor system, which consumes only a fraction of (10%) hardware resources.
Study On Social Network Recommendation Service Method Based On Mobile Cloud Computing
Cloud-based network virtualization in iot with openstack.
In Cloud computing deployments, specifically in the Infrastructure-as-a-Service (IaaS) model, networking is one of the core enabling facilities provided for the users. The IaaS approach ensures significant flexibility and manageability, since the networking resources and topologies are entirely under users’ control. In this context, considerable efforts have been devoted to promoting the Cloud paradigm as a suitable solution for managing IoT environments. Deep and genuine integration between the two ecosystems, Cloud and IoT, may only be attainable at the IaaS level. In light of extending the IoT domain capabilities’ with Cloud-based mechanisms akin to the IaaS Cloud model, network virtualization is a fundamental enabler of infrastructure-oriented IoT deployments. Indeed, an IoT deployment without networking resilience and adaptability makes it unsuitable to meet user-level demands and services’ requirements. Such a limitation makes the IoT-based services adopted in very specific and statically defined scenarios, thus leading to limited plurality and diversity of use cases. This article presents a Cloud-based approach for network virtualization in an IoT context using the de-facto standard IaaS middleware, OpenStack, and its networking subsystem, Neutron. OpenStack is being extended to enable the instantiation of virtual/overlay networks between Cloud-based instances (e.g., virtual machines, containers, and bare metal servers) and/or geographically distributed IoT nodes deployed at the network edge.
Export Citation Format
Share document.
- Reference Manager
- Simple TEXT file
People also looked at
Systematic review article, securing machine learning in the cloud: a systematic review of cloud machine learning security.
- 1 Information Technology University (ITU), Lahore, Pakistan
- 2 AI4Networks Research Center, University of Oklahoma, Norman, OK, United States
- 3 Social Data Science (SDS) Lab, Queen Mary University of London, London, United Kingdom
- 4 School of Computing and Communications, Lancaster University, Lancaster, United Kingdom
- 5 Hamad Bin Khalifa University (HBKU), Doha, Qatar
With the advances in machine learning (ML) and deep learning (DL) techniques, and the potency of cloud computing in offering services efficiently and cost-effectively, Machine Learning as a Service (MLaaS) cloud platforms have become popular. In addition, there is increasing adoption of third-party cloud services for outsourcing training of DL models, which requires substantial costly computational resources (e.g., high-performance graphics processing units (GPUs)). Such widespread usage of cloud-hosted ML/DL services opens a wide range of attack surfaces for adversaries to exploit the ML/DL system to achieve malicious goals. In this article, we conduct a systematic evaluation of literature of cloud-hosted ML/DL models along both the important dimensions— attacks and defenses —related to their security. Our systematic review identified a total of 31 related articles out of which 19 focused on attack, six focused on defense, and six focused on both attack and defense. Our evaluation reveals that there is an increasing interest from the research community on the perspective of attacking and defending different attacks on Machine Learning as a Service platforms. In addition, we identify the limitations and pitfalls of the analyzed articles and highlight open research issues that require further investigation.
1 Introduction
In recent years, machine learning (ML) techniques have been successfully applied to a wide range of applications, significantly outperforming previous state-of-the-art methods in various domains: for example, image classification, face recognition, and object detection. These ML techniques—in particular deep learning (DL)–based ML techniques—are resource intensive and require a large amount of training data to accomplish a specific task with good performance. Training DL models on large-scale datasets is usually performed using high-performance graphics processing units (GPUs) and tensor processing units. However, keeping in mind the cost of GPUs/Tensor Processing Units and the fact that small businesses and individuals cannot afford such computational resources, the training of deep models is typically outsourced to clouds, which is referred to in the literature as “Machine Learning as a Service” (MLaaS).
MLaaS refers to different ML services that are offered as a component of a cloud computing services, for example, predictive analytics, face recognition, natural language services, and data modeling APIs. MLaaS allows users to upload their data and model for training at the cloud. In addition to training, cloud-hosted ML services can also be used for inference purposes, that is, models can be deployed on the cloud environments; the system architecture of a typical MLaaS is shown in Figure 1 .
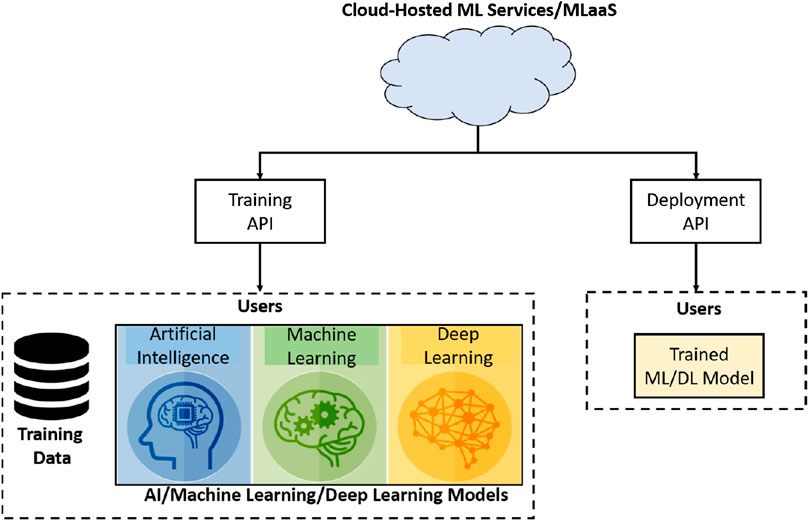
FIGURE 1 . Taxonomy of different defenses proposed for defending attacks on the third-party cloud-hosted machine learning (ML) or deep learning (DL) models.
MLaaS 1 can help reduce the entry barrier to the use of ML and DL through access to managed services of wide hardware heterogeneity and incredible horizontal scale. MLaaS is currently provided by several major organizations such as Google, Microsoft, and Amazon. For example, Google offers Cloud ML Engine 2 that allows developers and data scientists to upload training data and model which is trained on the cloud in the Tensorflow 3 environment. Similarly, Microsoft offers Azure Batch AI 4 —a cloud-based service for training DL models using different frameworks supported by both Linux and Windows operating systems and Amazon offers a cloud service named Deep Learning AMI (DLAMI) 5 that provides several pre-built DL frameworks (e.g., MXNet, Caffe, Theano, and Tensorflow) that are available in Amazon’s EC2 cloud computing infrastructure. Such cloud services are popular among researchers as evidenced by the price lifting of Amazon’s p2.16x large instance to the maximum possible—two days before the deadline of NeurIPS 2017 (the largest research venue on ML)—indicating that a large number of users request to reserve instances.
In addition to MLaaS services that allow users to upload their model and data for training on the cloud, transfer learning is another strategy to reduce computational cost in which a pretrained model is fine-tuned for a new task (using a new dataset). Transfer learning is widely applied for image recognition tasks using a convolutional neural network (CNN). A CNN model learns and encodes features like edges and other patterns. The learned weights and convolutional filters are useful for image recognition tasks in other domains and state-of-the-art results can be obtained with a minimal amount of training even on a single GPU. Moreover, various popular pretrained models such as AlexNet ( Krizhevsky et al., 2012 ), VGG ( Simonyan and Zisserman, 2015 ), and Inception ( Szegedy et al., 2016 ) are available for download and fine-tuning online. Both of the aforementioned outsourcing strategies come with new security concerns. In addition, the literature suggests that different types of attacks can be realized on different components of the communication network as well ( Usama et al., 2020a ), for example, intrusion detection ( Han et al., 2020 ; Usama et al., 2020b ), network traffic classification ( Usama et al., 2019 ), and malware detection systems ( Chen et al., 2018 ). Moreover, adversarial ML attacks have also been devised for client-side ML classifiers, that is, Google’s phishing pages filter ( Liang et al., 2016 ).
Contributions of the article: In this article, we analyze the security of MLaaS and other cloud-hosted ML/DL models and provide a systematic review of associated security challenges and solutions. To the best of our knowledge, this article is the first effort on providing a systematic review of the security of cloud-hosted ML models and services. The following are the major contributions of this article:
(1) We conducted a systematic evaluation of 31 articles related to MLaaS attacks and defenses.
(2) We investigated five themes of approaches aiming to attack MLaaS and cloud-hosted ML services.
(3) We examined five themes of defense methods for securing MLaaS and cloud-hosted ML services.
(4) We identified the pitfalls and limitations of the examined articles. Finally, we have highlighted open research issues that require further investigation.
Organization of the article: The rest of the article is organized as follows. The methodology adopted for the systematic review is presented in Section 2. The results of the systematic review are presented in Section 3. Section 4 presents various security challenges associated with cloud-hosted ML models and potential solutions for securing cloud-hosted ML models are presented in Section 5. The pitfalls and limitations of the reviewed approaches are discussed in Section 6. We briefly reflect on our methodology to identify any threats to the validity in Section 8 and various open research issues that require further investigation are highlighted in Section 7. Finally, we conclude the article in Section 9.
2 Review Methodology
In this section, we present the research objectives and the adopted methodology for the systematic review. The purpose of this article is to identify and systematically review the state-of-the art research related to the security of the cloud-based ML/DL techniques. The methodology followed for this study is depicted in Figure 2 .
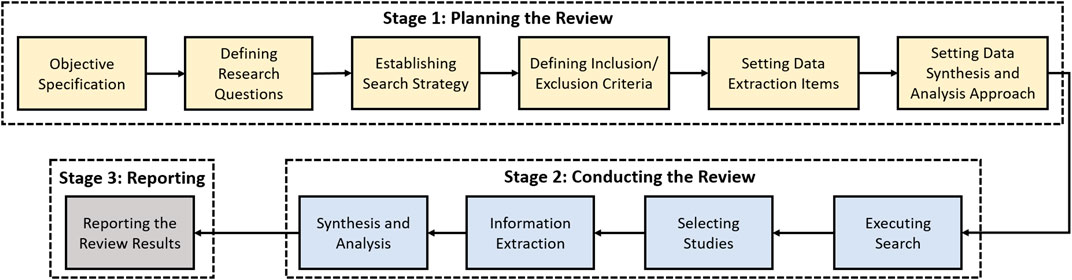
FIGURE 2 . An illustration of a typical cloud-based ML or machine learning as a service (MLaaS) architecture.
2.1 Research Objectives
The following are the key objectives of this article.
O1: To build upon the existing work around the security of cloud-based ML/DL methods and present a broad overview of the existing state-of-the-art literature related to MLaaS and cloud-hosted ML services.
O2: To identify and present a taxonomy of different attack and defense strategies for cloud-hosted ML/DL models.
O3: To identify the pitfalls and limitations of the existing approaches in terms of research challenges and opportunities.
2.2 Research Questions
To achieve our objectives, we consider answering two important questions that are described below and conducted a systematic analysis of 31 articles.
Q1: What are the well-known attacks on cloud-hosted/third-party ML/DL models?
Q2: What are the countermeasures and defenses against such attacks?
2.3 Review Protocol
We developed a review protocol to conduct the systematic review; the details are described below.
2.3.1 Search Strategy and Searching Phase
To build a knowledge base and extract the relevant articles, eight major publishers and online repositories were queried that include ACM Digital Library, IEEE Xplore, ScienceDirect, international conference on machine learning, international conference on learning representations, journal of machine learning research, neural information processing systems, USENIX, and arXiv. As we added non-peer–reviewed articles from electric preprint archive (arXiv), we (AQ and AI) performed the critical appraisal using AACODS checklist; it is designed to enable evaluation and appraisal of gray literature ( Tyndall, 2010 ), which is designed for the critical evaluation of gray literature.
In the initial phase, we queried main libraries using a set of different search terms that evolved using an iterative process to maximize the number of relevant articles. To achieve optimal sensitivity, we used a combination of words: attack, poisoning, Trojan attack, contamination, model inversion, evasion, backdoor, model stealing, black box, ML, neural networks, MLaaS, cloud computing, outsource, third party, secure, robust, and defense. The combinations of search keywords used are depicted in Figure 3 . We then created search strategies with controlled or index terms given in Figure 3 . Please note that no lower limit for the publication date was applied; the last search date was June 2020. The researchers (WI and AI) searched additional articles through citations and by snowballing on Google Scholar. Any disagreement was adjudicated by the third reviewer (AQ). Finally, articles focusing on the attack/defense for cloud-based ML models were retrieved.
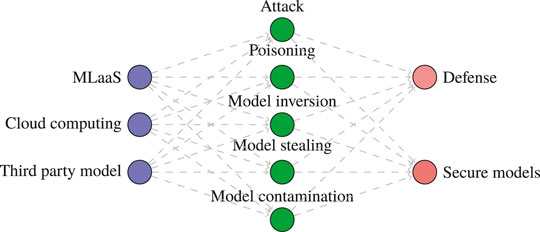
FIGURE 3 . The methodology for systematic review.
2.3.2 Inclusion and Exclusion Criteria
The inclusion and exclusion criteria followed for this systematic review are defined below.
2.3.2.1 Inclusion Criteria
The following are the key points that we considered for screening retrieved articles as relevant for conducting a systematic review.
• We included all articles relevant to the research questions and published in the English language that discusses the attacks on cloud-based ML services, for example, offered by cloud computing service providers.
• We then assessed the eligibility of the relevant articles by identifying whether they discussed either attack or defense for cloud-based ML/DL models.
• Comparative studies that compare the attacks and robustness against different well-known attacks on cloud-hosted ML services (poisoning attacks, black box attacks, Trojan attacks, backdoor attacks, contamination attacks, inversion, stealing, and invasion attacks).
• Finally, we categorized the selected articles into three categories, that is, articles on attacks, articles on defenses, and articles on attacks and defenses.
2.3.2.2 Exclusion Criteria
The exclusion criteria are outlined below.
• Articles that are written in a language other than English.
• Articles not available in full text.
• Secondary studies (e.g., systematic literature reviews, surveys, editorials, and abstracts or short papers) are not included.
• Articles that do not discuss attacks and defenses for cloud-based/third-party ML services, that is, we only consider those articles which have proposed an attack or defense for a cloud-hosted ML or MLaaS service.
2.3.3 Screening Phase
For the screening of articles, we employ two phases based on the content of the retrieved articles: 1) title and abstract screening and 2) full text of the publication. Please note that to avoid bias and to ensure that the judgment about the relevancy of articles is entirely based on the content of the publications, we intentionally do not consider authors, publication type (e.g., conference and journal), and publisher (e.g., IEEE and ACM). Titles and abstracts might not be true reflectors of the articles’ contents; however, we concluded that our review protocol is sufficient to avoid provenance-based bias.
It is very common that the same work got published in multiple venues, for example, conference papers are usually extended to journals. In such cases, we only consider the original article. In the screening phase, every article was screened by at least two authors of this article that were tasked to annotate the articles as either relevant, not relevant, or need further investigation, which was finalized by the discussion between the authors until any such article is either marked relevant or not relevant. Only original technical articles are selected, while survey and review articles are ignored. Finally, all selected publications were thoroughly read by the authors for categorization and thematic analysis.
3 Review Results
3.1 overview of the search and selection process outcome.
The search using the aforementioned strategy identified a total of 4,384 articles. After removing duplicate articles, title, and abstract screening, the overall number of articles reduced to 384. A total of 230 articles did not meet the inclusion criteria and were therefore excluded. From the remaining 154 articles, 123 articles did not discuss attack/defense for third-party cloud-hosted ML models and were excluded as well. Of the remaining articles, a total of 31 articles are identified as relevant. Reasons for excluding articles were documented and reported in a PRISMA flow diagram, depicted in Figure 4 . These articles were categorized into three classes, that is, articles that are specifically focused on attacks, articles that are specifically focused on defenses, and articles that considered both attacks and defenses containing 19, 6, and 6 articles each, respectively.
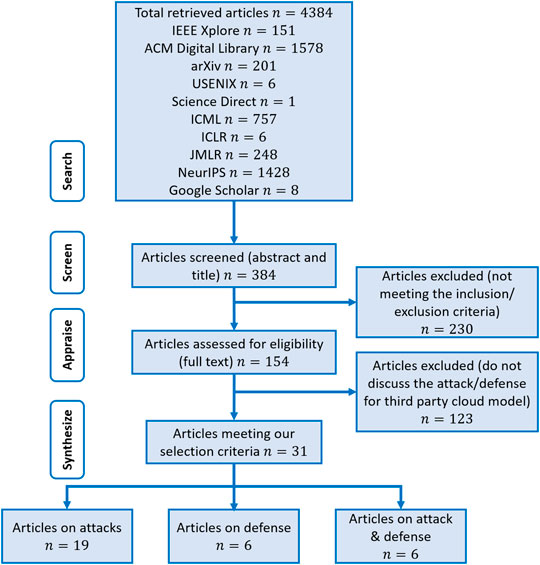
FIGURE 4 . Search queries used to identify publications to include in the systematic review.
3.2 Overview of the Selected Studies
The systematic review eventually identified a set of 31 articles related to cloud-based ML/DL models and MLaaS, which we categorized into three classes as mentioned above and shown in Figure 4 . As shown in Figure 5 , a significant portion of the selected articles were published in conferences (41.94%); comparatively, a very smaller proportion of these articles were published in journals or transactions (19.35%). The percentage of gray literature (i.e., non-peer–reviewed articles) is 25.81%. Yet, a very small proportion of publications are published in symposia (6.45%), and this percentage is the same for workshop papers. The distribution of selected publications by their types over the years is shown in Figure 6 . The figure depicts that the interest in the security of cloud-hosted ML/DL models increased in the year 2017 and was at a peak in the year 2018 and was slightly lower in the year 2019 as compared to 2018. Also, the majority of the articles during these years were published in conferences. The distribution of selected publications by their publishers over the years is depicted in Figure 7 , the figure shows that the majority of the publications have been published at IEEE, ACM, and arXiv. There is a similar trend in the number of articles in the year 2017, 2018, and 2019 as discussed previously.
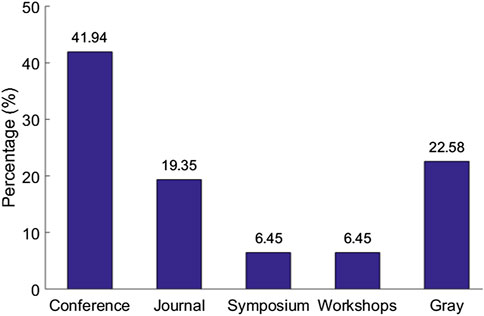
FIGURE 5 . Flowchart of systematic review and categorization.
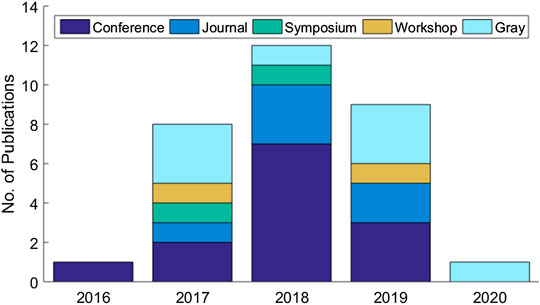
FIGURE 6 . Distribution of selected publications according to their types.
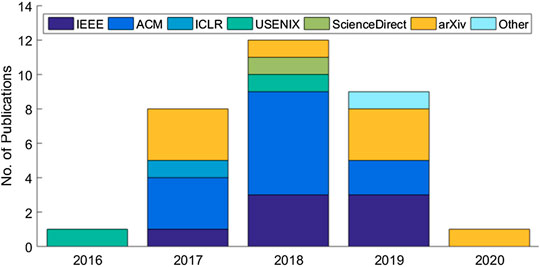
FIGURE 7 . Distribution of selected publications by types over years.
3.3 Some Partially Related Non-Selected Studies: A Discussion
We have described our inclusion and exclusion criteria that help us to identify relevant articles. We note, however, that some seemingly relevant articles failed to meet the inclusion criteria. Here, we briefly describe few such articles for giving a rationale why they were not included.
• Liang et al. (2016) investigated the security challenges for the client-side classifiers via a case study on the Google’s phishing pages filter, a very widely used classifier for automatically detecting unknown phishing pages. They devised an attack that is not relevant to the cloud-based service.
• Demetrio et al. (2020) presented WAF-A-MoLE, a tool that models the presence of an adversary. This tool leverages a set of mutation operators that alter the syntax of a payload without affecting the original semantics. Using the results, the authors demonstrated that ML-based WAFs are exposed to a concrete risk of being bypassed. However, this attack is not associated with any cloud-based services.
• Authors in Apruzzese et al. (2019) discussed adversarial attacks where the machine learning model is compromised to induce an output favorable to the attacker. These attacks are realized in a different setting as compared to the scope of this systematic review, as we only included the articles which discuss the attack or defense when the cloud is outsourcing its services as MLaaS.
• Han et al. (2020) conducted the first systematic study of the practical traffic space evasion attack on learning-based network intrusion detection systems; again it is out of the inclusion criteria of our work.
• Chen et al. (2018) designed and evaluated three types of attackers targeting the training phases to poison our detection. To address this threat, the authors proposed the detection system, KuafuDet, and showed it significantly reduces false negatives and boosts the detection accuracy.
• Song et al. (2020) presented a federated defense approach for mitigating the effect of adversarial perturbations in a federated learning environment. This article can be potentially relevant for our study as they address the problem of defending cloud-hosted ML models; however, instead of using a third-party service, the authors conducted the experiments on a single computer system in a simulated environment; therefore, this study is not included in the analysis of this article.
• In a similar study, Zhang et al. (2019) presented a defense mechanism for defending adversarial attacks on cloud-aided automatic speech recognition (ASR); however, it is not explicitly stated that the cloud is outsourcing ML services and also which ML/DL model or MLaaS was used in experiments.
4 Attacks on Cloud-Hosted Machine Learning Models (Q1)
In this section, we present the findings from the systematically selected articles that aim at attacking cloud-hosted/third-party ML/DL models.
4.1 Attacks on Cloud-Hosted Machine Learning Models: Thematic Analysis
In ML practice, it is very common to outsource the training of ML/DL models to third-party services that provide high computational resources on the cloud. Such services enable ML practitioners to upload their models along with training data which is then trained on the cloud. Although such services have clear benefits for reducing the training and inference time; however, these services can easily be compromised and to this end, different types of attacks against these services have been proposed in the literature. In this section, we present the thematic analysis of 19 articles that are focused on attacking cloud-hosted ML/DL models. These articles are classified into five major themes: 1) attack type, 2) threat model, 3) attack method, 4) target model(s), and 5) dataset.
Attack type: A wide variety of attacks have been proposed in the literature. These are listed below with their descriptions provided in the next section.
• Adversarial attacks ( Brendel et al., 2017 );
• Backdoor attacks 6 ( Chen et al., 2017 ; Gu et al., 2019 );
• Cyber kill chain–based attack ( Nguyen, 2017 );
• Data manipulation attacks ( Liao et al., 2018 );
• Evasion attacks ( Hitaj et al., 2019 );
• Exploration attacks ( Sethi and Kantardzic, 2018 );
• Model extraction attacks ( Correia-Silva et al., 2018 ; Kesarwani et al., 2018 ; Joshi and Tammana, 2019 ; Reith et al., 2019 );
• Model inversion attacks ( Yang et al., 2019 );
• Model-reuse attacks ( Ji et al., 2018 );
• Trojan attacks ( Liu et al., 2018 ).
black box attacks (no knowledge) ( Brendel et al., 2017 ; Chen et al., 2017 ; Hosseini et al., 2017 ; Correia-Silva et al., 2018 ; Sethi and Kantardzic, 2018 ; Hitaj et al., 2019 );
white box attacks (full knowledge) ( Liao et al., 2018 ; Liu et al., 2018 ; Gu et al., 2019 ; Reith et al., 2019 );
gray box attacks (partial knowledge) ( Ji et al., 2018 ; Kesarwani et al., 2018 ).
Attack method: In each article, a different type of method is proposed for attacking cloud-hosted ML/DL models; a brief description of these methods is presented in Table 1 and is discussed in detail in the next section.
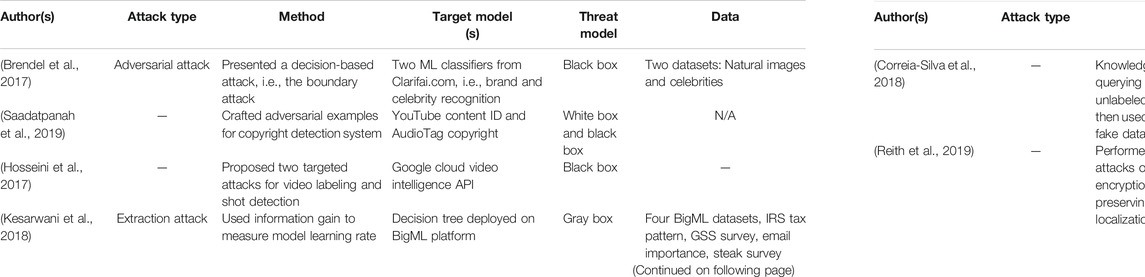
TABLE 1 . Summary of the state-of-the art attack types for cloud-based/third-party ML/DL models.
Target model(s): Considered studies have used different MLaaS services (e.g., Google Cloud ML Services ( Hosseini et al., 2017 ; Salem et al., 2018 ; Sethi and Kantardzic, 2018 ), ML models of BigML Platform ( Kesarwani et al., 2018 ), IBM’s visual recognition ( Nguyen, 2017 ), and Amazon Prediction APIs ( Reith et al., 2019 ; Yang et al., 2019 )).
Dataset: These attacks have been realized using different datasets ranging from small size datasets (e.g., MNIST ( Gu et al., 2019 ) and Fashion-MNIST ( Liu et al., 2018 )) to large size datasets (e.g., YouTube Aligned Face Dataset ( Chen et al., 2017 ), Project Wolf Eye ( Nguyen, 2017 ), and Iris dataset ( Joshi and Tammana, 2019 )). Other datasets include California Housing, Boston House Prices, UJIIndoorLoc, and IPIN 2016 Tutorial ( Reith et al., 2019 ), FaceScrub, CelebA, and CIFAR-10 ( Yang et al., 2019 ). A summary of thematic analyses of these attacks is presented in Table 1 and briefly described in the next section.
4.2 Taxonomy of Attacks on Cloud-Hosted Machine Learning Models
In this section, we present a taxonomy and description of different attacks described above in thematic analysis. A taxonomy of attacks on cloud-hosted ML/DL models is depicted in Figure 8 and is described next.
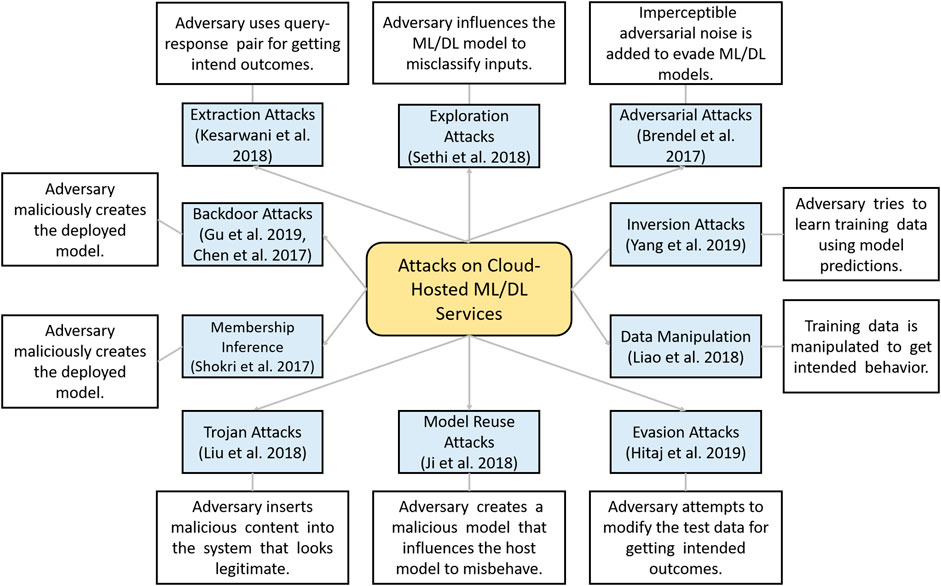
FIGURE 8 . Distribution of selected publications by publishers over years.
4.2.1 Adversarial Attacks
In recent years, DL models have been found vulnerable to carefully crafted imperceptible adversarial examples ( Goodfellow et al., 2014 ). For instance, a decision-based adversarial attack namely the boundary attack against two black box ML models trained for brand and celebrity recognition hosted at Clarifai.com are proposed in ( Brendel et al., 2017 ). The first model identifies brand names from natural images for 500 distinct brands and the second model recognizes over 10,000 celebrities. To date, a variety of adversarial examples generation methods have been proposed in the literature so far, the interesting readers are referred to recent surveys articles for detailed taxonomy of different types of adversarial attacks (i.e., Akhtar and Mian, 2018 ; Yuan et al., 2019 ; Qayyum et al., 2020b ; Demetrio et al., 2020 ).
4.2.2 Exploratory Attacks
These attacks are inference time attacks in which adversary attempts to evade the underlying ML/DL model, for example, by forcing the classifier (i.e., ML/DL model) to misclassify a positive sample as a negative one. Exploratory attacks do not harm the training data and only affects the model at test time. A data-driven exploratory attack using the Seed – Explore – Exploit strategy for evading Google’s cloud prediction API considering black box settings is presented in ( Sethi and Kantardzic, 2018 ). The performance evaluation of the proposed framework was performed using 10 real-world datasets.
4.2.3 Model Extraction Attacks
In model extraction attacks, adversaries can query the deployed ML model and can use query–response pair for compromising future predictions and also, they can potentially realize privacy breaches of the training data and can steal the model by learning extraction queries. In Kesarwani et al. (2018) , the authors presented a novel method for quantifying the extraction status of models for users with an increasing number of queries, which aims to measure model learning rate using information gain observed by query and response streams of users. The key objective of the authors was to design a cloud-based system for monitoring model extraction status and warnings. The performance evaluation of the proposed method was performed using a decision tree model deployed on the BigML MLaaS platform for different adversarial attack scenarios. Similarly, a model extraction/stealing strategy is presented by Correia-Silva et al. (2018) . The authors queried the cloud-hosted DL model with random unlabeled samples and used their predictions for creating a fake dataset. Then they used the fake dataset for building a fake model by training an oracle (copycat) model in an attempt to achieve similar performance as of the target model.
4.2.4 Backdooring Attacks
In backdooring attacks, an adversary maliciously creates the trained model which performs as good as expected on the users’ training and validation data, but it performs badly on attacker input samples. The backdooring attacks on deep neural networks (DNNs) are explored and evaluated in ( Gu et al., 2019 ). The authors first explored the properties of backdooring for a toy example and created a backdoor model for handwritten digit classifier and then demonstrated that backdoors are powerful for DNN by creating a backdoor model for a United States street sign classifier. Where, two scenarios were considered, that is, outsourced training of the model and transfer learning where an attacker can acquire a backdoor pretrained model online. In another similar study ( Chen et al., 2017 ), a targeted backdoor attack for two state-of-the art face recognition models, that is, DeepID ( Sun et al., 2014 ) and VGG-Face ( Parkhi et al., 2015 ) is presented. The authors proposed two categories of backdooring poisoning attacks, that is, input–instance–key attacks and pattern–key attacks using two different data poising strategies, that is, input–instance–key strategies and pattern–key strategies, respectively.
4.2.5 Trojan Attacks
In Trojan attacks, the attacker inserts malicious content into the system that looks legitimate but can take over the control of the system. However, the purpose of Trojan insertion can be varied, for example, stealing, disruption, misbehaving, or getting intended behavior. In Liu et al. (2018) , the authors proposed a stealth infection on neural networks, namely, SIN2 to realize a practical supply chain triggered neural Trojan attacks. Also, they proposed a variety of Trojan insertion strategies for agile and practical Trojan attacks. The proof of the concept is demonstrated by developing a prototype of the proposed neural Trojan attack (i.e., SIN2) in Linux sandbox and used Torch ( Collobert et al., 2011 ) ML/DL framework for building visual recognition models using the Fashion-MNIST dataset.
4.2.6 Model-Reuse Attacks
In model-reuse attacks, an adversary creates a malicious model (i.e., adversarial model) that influences the host model to misbehave on targeted inputs (i.e., triggers) in extremely predictable fashion, that is, getting a sample classified into specific (intended class). For instance, experimental evaluation of model-reuse attacks for four pretrained primitive DL models (i.e., speech recognition, autonomous steering, face verification, and skin cancer screening) is evaluated by Ji et al. (2018) .
4.2.7 Data Manipulation Attacks
Those attacks in which training data are manipulated to get intended behavior by the ML/DL model are known as data manipulation attacks. Data manipulation attacks for stealthily manipulating traditional supervised ML techniques and logistic regression (LR) and CNN models are studied by Liao et al. (2018) . In the attack strategy, the authors added a new constraint on fully connected layers of the models and used gradient descent for retraining them, and other layers were frozen (i.e., were made non-trainable).
4.2.8 Cyber Kill Chain–Based Attacks
Kill chain is a term used to define steps for attacking a target usually used in the military. In cyber kill chain–based attacks, the cloud-hosted ML/DL models are attacked, for example, a high-level threat model targeting ML cyber kill chain is presented by Nguyen (2017) . Also, the authors provided proof of concept by providing a case study using IBM visual recognition MLaaS (i.e., cognitive classifier for classification cats and female lions) and provided recommendations for ensuring secure and robust ML.
4.2.9 Membership Inference Attacks
In a typical membership inference attack, for given input data and black box access to the ML model, an attacker attempts to figure out if the given input sample was the part of the training set or not. To realize a membership inference attack against a target model, a classification model is trained for distinguishing between the predictions of the target model against the inputs on which it was trained and that those on which it was not trained ( Shokri et al., 2017 ).
4.2.10 Evasion Attacks
Evasion attacks are inference time attacks in which an adversary attempts to modify the test data for getting the intended outcome from the ML/DL model. Two evasion attacks against watermarking techniques for DL models hosted as MLaaS have been presented by Hitaj et al. (2019) . The authors used five publicly available models and trained them for distinguishing between watermarked and clean (non-watermarked) images, that is, binary image classification tasks.
4.2.11 Model Inversion Attacks
In model inversion attacks, an attacker tries to learn about training data using the model’s outcomes. Two model inversion techniques have been proposed by Yang et al. (2019) , that is, training an inversion model using auxiliary set composed by utilizing adversary’s background knowledge and truncation-based method for aligning the inversion model. The authors evaluated their proposed methods on a commercial prediction MLaaS named Amazon Rekognition.
5 Toward Securing Cloud-Hosted Machine Learning Models (Q2)
In this section, we present the insights from the systematically selected articles that provide tailored defense against specific attacks and report the articles that along with creating attacks propose countermeasure for the attacks for cloud-hosted/third-party ML/DL models.
5.1 Defenses for Attacks on Cloud-Hosted Machine Learning Models: Thematic Analysis
Leveraging cloud-based ML services for computational offloading and minimizing the communication overhead is accepted as a promising trend. While cloud-based prediction services have significant benefits, however, by sharing the model and the training data raises many privacy and security challenges. Several attacks that can compromise the model and data integrity, as described in the previous section. To avoid such issues, users can download the model and make inferences locally. However, this approach has certain drawbacks, including, confidentiality issues, service providers cannot update the models, adversaries can use the model to develop evading strategies, and privacy of the user data is compromised. To outline the countermeasures against these attacks, we present the thematic analysis of six articles that are focused on defense against the tailored attacks for cloud-hosted ML/DL models or data. In addition, we also provide the thematic analysis of those six articles that propose defense against specific attacks. These articles are classified into five major themes: 1) attack type, 2) defense, 3) target model(s), 4) dataset, and 5) measured outcomes. The thematic analysis of these systematically reviewed articles that are focused on developing defense strategies against attacks is given below.
Considered attacks for developing defenses: The defenses proposed in the reviewed articles are developed against the following specific attacks.
• Extraction attacks ( Tramèr et al., 2016 ; Liu et al., 2017 );
• Inversion attacks ( Liu et al., 2017 ; Sharma and Chen, 2018 );
• Adversarial attacks ( Hosseini et al., 2017 ; Wang et al., 2018b ; Rouhani et al., 2018 );
• Evasion attacks ( Lei et al., 2020 );
• GAN attacks ( Sharma and Chen, 2018 );
• Privacy threat attacks ( Hesamifard et al., 2017 );
• ide channel and cache-timing attacks ( Jiang et al., 2018 );
• Membership inference attacks ( Shokri et al., 2017 ; Salem et al., 2018 ).
Most of the aforementioned attacks are elaborated in previous sections. However, in the selected articles that are identified as either defense or attack and defense articles, some attacks are specifically created, for instance, GAN attacks, side channel, cache-timing attack, privacy threats, etc. Therefore, the attacks are worth mentioning in this section to explain the specific countermeasures proposed against them in the defense articles.
Defenses against different attacks: To provide resilience against these attacks, the authors of selected articles proposed different defense algorithms, which are listed below against each type of attack.
• Extraction attacks: MiniONN ( Liu et al., 2017 ), rounding confidence, differential, and ensemble methods ( Tramèr et al., 2016 );
• Adversarial attacks: ReDCrypt ( Rouhani et al., 2018 ) and Arden ( Wang et al., 2018b );
• Inversion attacks: MiniONN ( Liu et al., 2017 ) and image disguising techniques ( Sharma and Chen, 2018 );
• Privacy attacks: encryption-based defense ( Hesamifard et al., 2017 ; Jiang et al., 2018 );
• Side channel and cache-timing attacks: encryption-based defense ( Hesamifard et al., 2017 ; Jiang et al., 2018 );
• Membership inference attack: dropout and model stacking ( Salem et al., 2018 ).
Target model(s): Different cloud-hosted ML/DL models have been used for the evaluation of the proposed defenses, as shown in Table 2 .
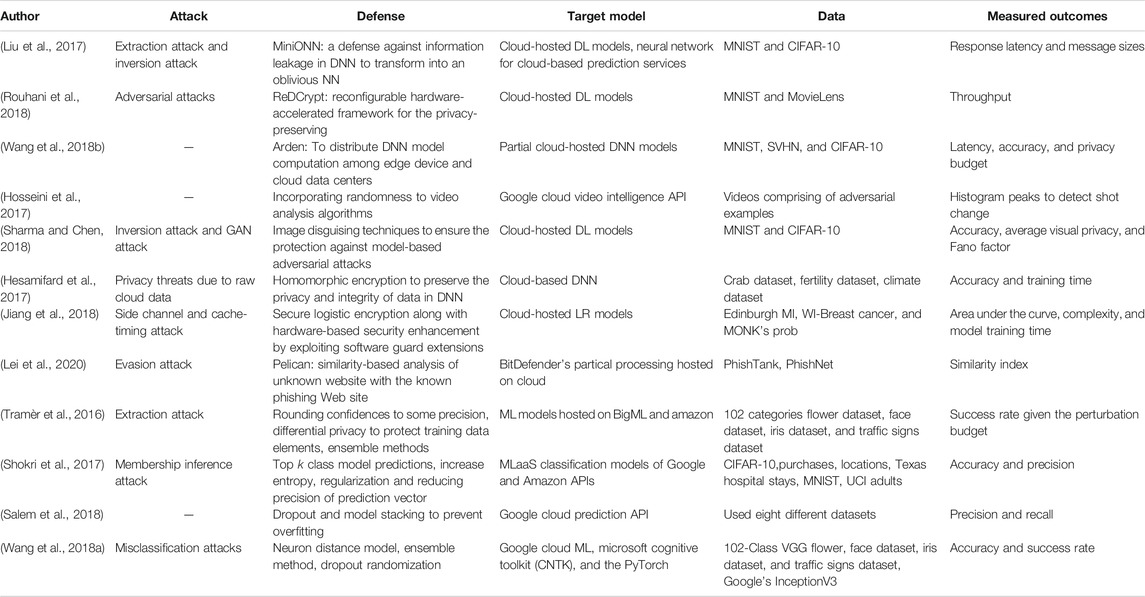
TABLE 2 . Summary of attack types and corresponding defenses for cloud-based/third-party ML/DL models.
Dataset(s) used: The robustness of these defenses have been evaluated using various datasets ranging from small size datasets (e.g., MNIST ( Liu et al., 2017 ; Wang et al., 2018b ; Rouhani et al., 2018 ; Sharma and Chen, 2018 )) and CIFAR-10 ( Liu et al., 2017 ; Wang et al., 2018b ; Sharma and Chen, 2018 )), to large size datasets (e.g., Iris dataset ( Tramèr et al., 2016 ), fertility and climate dataset ( Hesamifard et al., 2017 ), and breast cancer ( Jiang et al., 2018 )). Other datasets include Crab dataset ( Hesamifard et al., 2017 ), Face dataset, Traffic signs dataset, Traffic signs dataset ( Tramèr et al., 2016 ), SVHN ( Wang et al., 2018b ), Edinburgh MI, Edinburgh MI, WI-Breast Cancerband MONKs Prob ( Jiang et al., 2018 ), crab dataset, fertility dataset, and climate dataset ( Hesamifard et al., 2017 ). Each of the defense techniques discussed above is mapped in Table 2 to the specific attack for which it was developed.
Measured outcomes: The measured outcomes based on which the defenses are evaluated are response latency and message sizes ( Liu et al., 2017 ; Wang et al., 2018b ), throughput comparison ( Rouhani et al., 2018 ), average on the cache miss rates per second ( Sharma and Chen, 2018 ), AUC, space complexity to demonstrate approximated storage costs ( Jiang et al., 2018 ), classification accuracy of the model as well as running time ( Hesamifard et al., 2017 ; Sharma and Chen, 2018 ), similarity index ( Lei et al., 2020 ), and training time ( Hesamifard et al., 2017 ; Jiang et al., 2018 ).
5.2 Taxonomy of Defenses on Cloud-Hosted Machine Learning Model Attacks
In this section, we present a taxonomy and summary of different defensive strategies against attacks on cloud-hosted ML/DL models as described above in thematic analysis. A taxonomy of these defenses strategies is presented in Figure 9 and is described next.
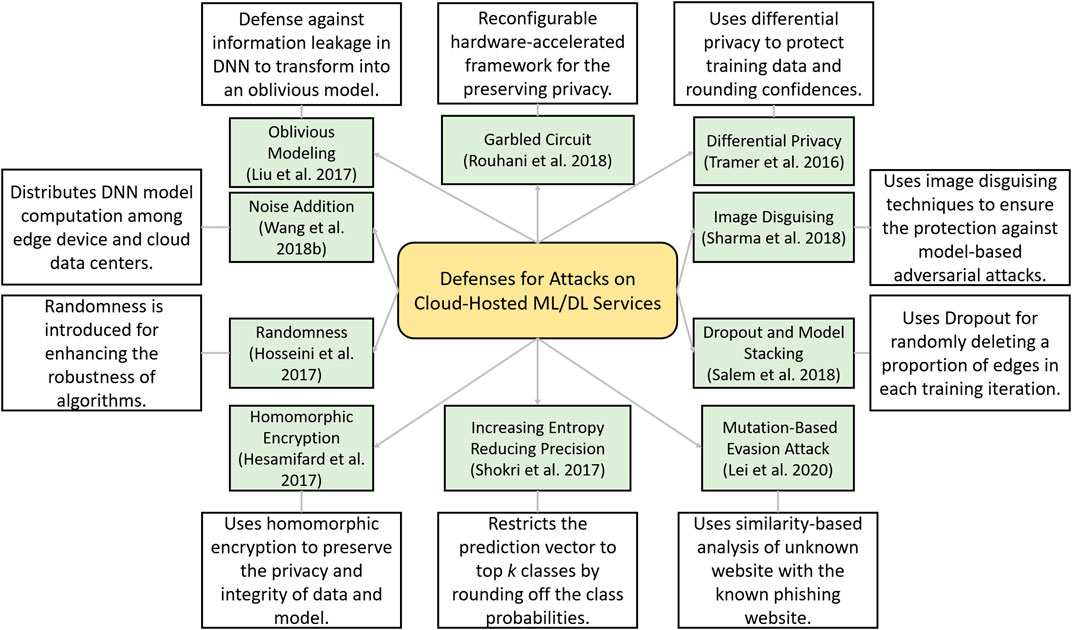
FIGURE 9 . Taxonomy of different attacks realized on the third-party cloud-hosted machine learning (ML) or deep learning (DL) models.
5.2.1 MiniONN
DNNs are vulnerable to model inversion and extraction attacks. Liu et al. (2017) proposed that without making any changes to the training phase of the model it is possible to change the model into an oblivious neural network. They make the nonlinear function such as tanh and sigmoid function more flexible, and by training the models on several datasets, the authors demonstrated significant results with minimal loss in the accuracy. In addition, they also implemented the offline precomputation phase to perform encryption incremental operations along with the SIMD batch processing technique.
5.2.2 ReDCrypt
A reconfigurable hardware-accelerated framework is proposed by Rouhani et al. (2018) , for protecting the privacy of deep neural models in cloud networks. The authors perform an innovative and power-efficient implementation of Yao’s Garbled Circuit (GC) protocol on FPGAs for preserving privacy. The proposed framework is evaluated for different DL applications, and it has achieved up to 57-fold throughput gain per core.
5.2.3 Arden
To offload the large portion of DNNs from the mobile devices to the clouds and to make the framework secure, a privacy-preserving mechanism Arden is proposed by Wang et al. (2018b) . While uploading the data to the mobile-cloud perturbation, noisy samples are included to make the data secure. To verify the robustness, the authors perform rigorous analysis based on three image datasets and demonstrated that this defense is capable to preserve the user privacy along with inference performance.
5.2.4 Image Disguising Techniques
While leveraging services from the cloud GPU server, the adversary can realize an attack by introducing malicious created training data, perform model inversion, and use the model for getting desirable incentives and outcomes. To protect from such attacks and to preserve the data as well as the model, Sharma and Chen (2018) proposed an image disguising mechanism. They developed a toolkit that can be leveraged to calibrate certain parameter settings. They claim that the disguised images with block-wise permutation and transformations are resilient to GAN-based attack and model inversion attacks.
5.2.5 Homomorphic Encryption
For making the cloud services of outsourced MLaaS secure, Hesamifard et al. (2017) proposed a privacy-preserving framework using homomorphic encryption. They trained the neural network using the encrypted data and then performed the encrypted predictions. The authors demonstrated that by carefully choosing the polynomials of the activation functions to adopt neural networks, it is possible to achieve the desired accuracy along with privacy-preserving training and classification.
In a similar study, to preserve the privacy of outsourced biomedical data and computation on public cloud servers, Jiang et al. (2018) built a homomorphically encrypted model that reinforces the hardware security through Software Guard Extensions. They combined homomorphic encryption and Software Guard Extensions to devise a hybrid model for the security of the most commonly used model for biomedical applications, that is, LR. The robustness of the Secure LR framework is evaluated on various datasets, and the authors also compared its performance with state-of-the-art secure LR solutions and demonstrated its superior efficiency.
5.2.6 Pelican
Lei et al. (2020) proposed three mutation-based evasion attacks and a sample-based collision attack in white-, gray-, and black box scenarios. They evaluated the attacks and demonstrated a 100% success rate of attack on Google’s phishing page filter classifier, while a success rate of up to 81% for the transferability on Bitdefender TrafficLight. To deal with such attacks and to increase the robustness of classifiers, they proposed a defense method known as Pelican.
5.2.7 Rounding Confidences and Differential Privacy
Tramèr et al. (2016) presented the model extraction attacks against the online services of BigML and Amazon ML. The attacks are capable of model evasion, monetization, and can compromise the privacy of training data. The authors also proposed and evaluated countermeasures such as rounding confidences against equation-solving and decision tree pathfinding attacks; however, this defense has no impact on the regression tree model attack. For the preservation of training data, differential privacy is proposed; this defense reduces the ability of an attacker to learn insights about the training dataset. The impact of both defenses is evaluated on the attacks for different models, while the authors also proposed ensemble models to mitigate the impact of attacks; however, their resilience is not evaluated.
5.2.8 Increasing Entropy and Reducing Precision
The training of attack using shadow training techniques against black box models in the cloud-based Google Prediction API and Amazon ML models are studied by Shokri et al. (2017) . The attack does not require prior knowledge of training data distribution. The authors emphasize that in order to protect the privacy of medical-related datasets or other public-related data, countermeasures should be designed. For instance, restriction of prediction vector to top k classes, which will prevent the leakage of important information or rounding down or up the classification probabilities in the prediction. They show that regularization can be effective to cope with overfitting and increasing the randomness of the prediction vector.
5.2.9 Dropout and Model Stacking
In the study by Salem et al. (2018) , the authors created three diverse attacks and tested the applicability of these attacks on eight datasets from which six are similar as used by Shokri et al. (2017) , whereas in this work, news dataset and face dataset is included. In the threat model, the authors considered black box access to the target model which is a supervised ML classifier with binary classes that was trained for binary classification. To mitigate the privacy threats, the authors proposed a dropout-based method which reduces the impact of an attack by randomly deleting a proportion of edges in each training iteration in a fully connected neural network. The second defense strategy is model stacking, which hierarchically organizes multiple ML models to avoid overfitting. After extensive evaluation, these defense techniques showed the potential to mitigate the performance of the membership inference attack.
5.2.10 Randomness to Video Analysis Algorithms
Hosseini et al. designed two attacks specifically to analyze the robustness of video classification and shot detection ( Hosseini et al., 2017 ). The attack can subtly manipulate the content of the video in such a way that it is undetected by humans, while the output from the automatic video analysis method is altered. Depending on the fact that the video and shot labels are generated by API by processing only the first video frame of every second, the attack can successfully deceive API. To deal with the shot removal and generation attacks, the authors proposed the inclusion of randomness for enhancing the robustness of algorithms. However, in this article, the authors thoroughly evaluated the applicability of these attacks in different video setting, but the purposed defense is not rigorously evaluated.
5.2.11 Neuron Distance Threshold and Obfuscation
Transfer learning is an effective technique for quickly building DL student models in which knowledge from a Teacher model is transferred to a Student model. However, Wang et al. (2018a) discussed that due to the centralization of model training, the vulnerability against misclassification attacks for image recognition on black box Student models increases. The authors proposed several defenses to mitigate the impact of such an attack, such as changing the internal representation of the Student model from the Teacher model. Other defense methods include increasing dropout randomization which alters the student model training process, modification in input data before classification, adding redundancy, and using orthogonal model against transfer learning attack. The authors analyzed the robustness of these attacks and demonstrated that the neuron distance threshold is the most effective in obfuscating the identity of the Teacher model.
6 Pitfalls and Limitations
6.1 lack of attack diversity.
The attacks presented in the selected articles have limited scope and lack diversity, that is, they are limited to a specific setting, and the variability of attacks is limited as well. However, the diversity of attacks is an important consideration for developing robust attacks from the perspective of adversaries, and it ensures the detection and prevention of the attacks to be difficult. The diversity of attacks ultimately helps in the development of robust defense strategies. Moreover, the empirical evaluation of attack variabilities can identify the potential vulnerabilities of cybersecurity systems. Therefore, to make a more robust defense solution, it is important to test the model robustness under a diverse set of attacks.
6.2 Lack of Consideration for Adaptable Adversaries
Most of the defenses in the systematically reviewed articles are proposed for a specific attack and did not consider the adaptable adversaries. On the other hand, in practice, the adversarial attacks are an arms race between attackers and defenders. That is, the attackers continuously evolve and enhance their knowledge and attacking strategies to evade the underlying defensive system. Therefore, the consideration of adaptable adversaries is crucial for developing a robust and long-lasting defense mechanism. If we do not consider this, the adversary will adapt to our defensive system over time and will bypass it to get the intended behavior or outcomes.
6.3 Limited Progress in Developing Defenses
From the systematically selected articles that are collected from different databases, only 12 articles have presented defense methods for the proposed attack as compared to the articles that are focused on attacks, that is, 19. In these 12 articles, six have only discussed/presented a defense strategy and six have developed a defense against a particular attack. This indicates that there is limited activity from the research community in developing defense strategies for already proposed attacks in the literature. In addition, the proposed defenses only mitigate or detect those attacks for which they have been developed, and therefore, they are not generalizable. On the contrary, the increasing interest in developing different attacks and the popularity of cloud-hosted/third-party services demand a proportionate amount of interest in developing defense systems as well.
7 Open Research Issues
7.1 adversarially robust machine learning models.
In recent years, adversarial ML attacks have emerged as a major panacea for ML/DL models and the systematically selected articles have highlighted the threat of these attacks for cloud-hosted Ml/DL models as well. Moreover, the diversity of these attacks is drastically increasing as compared with the defensive strategies that can pose serious challenges and consequences for the security of cloud-hosted ML/DL models. Each defense method presented in the literature so far has been shown resilient to a particular attack which is realized in specific, settings and it fails to withstand for yet stronger and unseen attacks. Therefore, the development of adversarially robust ML/DL models remains an open research problem, while the literature suggests that worst-case robustness analysis should be performed while considering adversarial ML settings ( Qayyum et al., 2020a ; Qayyum et al., 2020b ; Ilahi et al., 2020 ). In addition, it has been argued in the literature that most of ML developers and security incident responders are unequipped with the required tools for securing industry-grade ML systems against adversarial ML attacks Kumar et al. (2020) . This indicates the increasing need for the development of defense strategies for securing ML/DL models against adversarial ML attacks.
7.2 Privacy-Preserving Machine Learning Models
In cloud-hosted ML services, preserving user privacy is fundamentally important and is a matter of high concern. Also, it is desirable that ML models built using users’ data should not learn information that can compromise the privacy of the individuals. However, the literature on developing privacy-preserving ML/DL models or MLaaS is limited. On the other hand, one of the privacy-preserving techniques that have been used for privacy protection for building a defense system for cloud-hosted ML/DL models, that is, the homomorphic encryption-based protocol ( Jiang et al., 2018 ), has been shown vulnerable to model extraction attack ( Reith et al., 2019 ). Therefore, the development of privacy-preserving ML models for cloud computing platforms is another open research problem.
7.3 Proxy Metrics for Evaluating Security and Robustness
From systematically reviewed literature on the security of cloud-hosted ML/DL models, we orchestrate that the interest from the research community in the development of novel security-centric proxy metrics for the evaluation of security threats and model robustness of cloud-hosted models is very limited. However, with the increasing proliferation of cloud-hosted ML services (i.e., MLaaS) and with the development/advancements of different attacks (e.g., adversarial ML attacks), the development of effective and scalable metrics for evaluating the robustness ML/DL models toward different attacks and defense strategies is required.
8 Threats to Validity
We now briefly reflect on our methodology in order to identify any threats to the validity of our findings. First, internal validity is maintained as the research questions we pose in Section 2.2 capture the objectives of the study. Construct validity relies on a sound understanding of the literature and how it represents the state of the field. A detailed study of the reviewed articles along with deep discussions between the members of the research team helped ensure the quality of this understanding. Note that the research team is of diverse skills and expertise in ML, DL, cloud computing, ML/DL security, and analytics. Also, the inclusion and exclusion criteria (Section 2.3) help define the remit of our survey. Data extraction is prone to human error as is always the case. This was mitigated by having different members of the research team review each reviewed article. However, we did not attempt to evaluate the quality of the reviewed studies or validate their content due to time constraints. In order to minimize selection bias, we cast a wide net in order to capture articles from different communities publishing in the area of MLaaS via a comprehensive set of bibliographical databases without discriminating based on the venue/source.
9 Conclusion
In this article, we presented a systematic review of literature that is focused on the security of cloud-hosted ML/DL models, also named as MLaaS. The relevant articles were collected from eight major publishers that include ACM Digital Library, IEEE Xplore, ScienceDirect, international conference on machine learning, international conference on learning representations, journal of machine learning research, USENIX, neural information processing systems, and arXiv. For the selection of articles, we developed a review protocol that includes inclusion and exclusion formulas and analyzed the selected articles that fulfill these criteria across two dimensions (i.e., attacks and defenses) on MLaaS and provide a thematic analysis of these articles across five attack and five defense themes, respectively. We also identified the limitations and pitfalls from the reviewed literature, and finally, we have highlighted various open research issues that require further investigation.
Data Availability Statement
The original contributions presented in the study are included in the article/supplementary material, further inquiries can be directed to the corresponding author/s.
Author Contributions
AQ led the work in writing the manuscript and performed the annotation of the data and analysis as well. AI performed data acquisition, annotation, and analysis from four venues, and contributed to the paper write-up. MU contributed to writing a few sections, did annotations of papers, and helped in analysis. WI performed data scrapping, annotation, and analysis from four venues, and helped in developing graphics. All the first four authors validated the data, analysis, and contributed to the interpretation of the results. AQ and AI helped in developing and refining the methodology for this systematic review. JQ conceived the idea and supervises the overall work. JQ, YEK, and AF provided critical feedback and helped shape the research, analysis, and manuscript. All authors contributed to the final version of the manuscript.
Conflict of Interest
The authors declare that the research was conducted in the absence of any commercial or financial relationships that could be construed as a potential conflict of interest.
1 We use MLaaS to cover both ML and DL as a Service cloud provisions.
2 https://cloud.google.com/ml-engine/ .
3 A popular Python library for DL.
4 https://azure.microsoft.com/en-us/services/machine-learning-service/ .
5 https://docs.aws.amazon.com/dlami/latest/devguide/AML2_0.html .
6 Backdoor attacks on cloud-hosted models can be further categorized into three categories ( Chen et al., 2020 ): 1) complete model–based attacks, 2) partial model–based attacks, and 3) model-free attacks).
Akhtar, N., and Mian, A. (2018). Threat of adversarial attacks on deep learning in computer vision: a survey. IEEE Access 6, 14410–14430. doi:10.1109/access.2018.2807385
CrossRef Full Text | Google Scholar
Apruzzese, G., Colajanni, M., Ferretti, L., and Marchetti, M. (2019). “Addressing adversarial attacks against security systems based on machine learning,” in 2019 11th International conference on cyber conflict (CyCon) , Tallinn, Estonia , May 28–31, 2019 ( IEEE ), 900, 1–18
Google Scholar
Brendel, W., Rauber, J., and Bethge, M. (2017). “Decision-based adversarial attacks: reliable attacks against black-box machine learning models,” in International Conference on Learning Representations (ICLR)
Chen, S., Xue, M., Fan, L., Hao, S., Xu, L., Zhu, H., et al. (2018). Automated poisoning attacks and defenses in malware detection systems: an adversarial machine learning approach. Comput. Secur. 73, 326–344. doi:10.1016/j.cose.2017.11.007
Chen, X., Liu, C., Li, B., Lu, K., and Song, D. (2017). Targeted backdoor attacks on deep learning systems using data poisoning. arXiv
Chen, Y., Gong, X., Wang, Q., Di, X., and Huang, H. (2020). Backdoor attacks and defenses for deep neural networks in outsourced cloud environments. IEEE Network 34 (5), 141–147. doi:10.1109/MNET.011.1900577
Collobert, R., Kavukcuoglu, K., and Farabet, C. (2011). “Torch7: a Matlab-like environment for machine learning,” in BigLearn, NIPS workshop .
Correia-Silva, J. R., Berriel, R. F., Badue, C., de Souza, A. F., and Oliveira-Santos, T. (2018). “Copycat CNN: stealing knowledge by persuading confession with random non-labeled data,” in 2018 International joint conference on neural networks (IJCNN) , Rio de Janeiro, Brazil , July 8–13, 2018 ( IEEE ), 1–8
Demetrio, L., Valenza, A., Costa, G., and Lagorio, G. (2020). “Waf-a-mole: evading web application firewalls through adversarial machine learning,” in Proceedings of the 35th annual ACM symposium on applied computing , Brno, Czech Republic , March 2020 , 1745–1752
Gong, Y., Li, B., Poellabauer, C., and Shi, Y. (2019). “Real-time adversarial attacks,” in Proceedings of the 28th International Joint Conference on Artificial Intelligence (IJCAI) , Macao, China , August 2019
Goodfellow, I. J., Shlens, J., and Szegedy, C. (2014). Explaining and harnessing adversarial examples. arXiv
Gu, T., Liu, K., Dolan-Gavitt, B., and Garg, S. (2019). BadNets: evaluating backdooring attacks on deep neural networks. IEEE Access 7, 47230–47244. doi:10.1109/access.2019.2909068
Han, D., Wang, Z., Zhong, Y., Chen, W., Yang, J., Lu, S., et al. (2020). Practical traffic-space adversarial attacks on learning-based nidss. arXiv
Hesamifard, E., Takabi, H., Ghasemi, M., and Jones, C. (2017). “Privacy-preserving machine learning in cloud,” in Proceedings of the 2017 on cloud computing security workshop , 39–43
Hilprecht, B., Härterich, M., and Bernau, D. (2019). “Monte Carlo and reconstruction membership inference attacks against generative models,” in Proceedings on Privacy Enhancing Technologies , Stockholm, Sweden , July 2019 , 2019, 232–249
Hitaj, D., Hitaj, B., and Mancini, L. V. (2019). “Evasion attacks against watermarking techniques found in MLaaS systems,” in 2019 sixth international conference on software defined systems (SDS) , Rome, Italy , June 10–13, 2019 ( IEEE )
Hosseini, H., Xiao, B., Clark, A., and Poovendran, R. (2017). “Attacking automatic video analysis algorithms: a case study of google cloud video intelligence API,” in Proceedings of the 2017 conference on multimedia Privacy and security (ACM) , 21–32
Ilahi, I., Usama, M., Qadir, J., Janjua, M. U., Al-Fuqaha, A., Hoang, D. T., et al. (2020). Challenges and countermeasures for adversarial attacks on deep reinforcement learning. arXiv
Ji, Y., Zhang, X., Ji, S., Luo, X., and Wang, T. (2018). “Model-reuse attacks on deep learning systems, “in Proceedings of the 2018 ACM SIGSAC Conference on Computer and Communications Security (New York, NY: ACM) , December 2018 , 349–363
Jiang, Y., Hamer, J., Wang, C., Jiang, X., Kim, M., Song, Y., et al. (2018). Securelr: secure logistic regression model via a hybrid cryptographic protocol. IEEE ACM Trans. Comput. Biol. Bioinf 16, 113–123. doi:10.1109/TCBB.2018.2833463
Joshi, N., and Tammana, R. (2019). “GDALR: an efficient model duplication attack on black box machine learning models,” in 2019 IEEE international Conference on system, computation, Automation and networking (ICSCAN) , Pondicherry, India , March 29–30, 2019 ( IEEE ), 1–6
Kesarwani, M., Mukhoty, B., Arya, V., and Mehta, S. (2018). Model extraction warning in MLaaS paradigm. In Proceedings of the 34th Annual Computer Security Applications Conference (ACM) , 371–380
Krizhevsky, A., Sutskever, I., and Hinton, G. E. (2012). “Imagenet classification with deep convolutional neural networks,” in Advances in neural information processing systems , 1097–1105 Available at: http://papers.nips.cc/paper/4824-imagenet-classification-with-deep-convolutional-neural-networks.pdf
Kumar, R. S. S., Nyström, M., Lambert, J., Marshall, A., Goertzel, M., Comissoneru, A., et al. (2020). Adversarial machine learning–industry perspectives. arXiv . Available at: https://papers.ssrn.com/sol3/papers.cfm?abstract_id=3532474
Lei, Y., Chen, S., Fan, L., Song, F., and Liu, Y. (2020). Advanced evasion attacks and mitigations on practical ml-based phishing website classifiers. arXiv
Liang, B., Su, M., You, W., Shi, W., and Yang, G. (2016). “Cracking classifiers for evasion: a case study on the google’s phishing pages filter,” in Proceedings of the 25th international conference on world wide web Montréal, Québec, Canada , 345–356
Liao, C., Zhong, H., Zhu, S., and Squicciarini, A. (2018). “Server-based manipulation attacks against machine learning models,” in Proceedings of the eighth ACM conference on data and application security and privacy (ACM) , New York, NY , March 2018 , 24–34
Liu, J., Juuti, M., Lu, Y., and Asokan, N.. (2017). “Oblivious neural network predictions via minionn transformations,” in Proceedings of the 2017 ACM SIGSAC Conference on Computer and Communications Security , October 2017 , 619–631
Liu, T., Wen, W., and Jin, Y. (2018). “SIN 2: stealth infection on neural network—a low-cost agile neural Trojan attack methodology,” in 2018 IEEE international symposium on hardware oriented security and trust (HOST) , Washington, DC , April 30–4 May, 2018 ( IEEE ), 227–230
Nguyen, T. N. (2017). Attacking machine learning models as part of a cyber kill chain. arXiv
Parkhi, O. M., Vedaldi, A., Zisserman, A., et al. (2015). Deep face recognition. Bmvc 1, 6. doi:10.5244/C.29.41
Qayyum, A., Qadir, J., Bilal, M., and Al-Fuqaha, A. (2020a). Secure and robust machine learning for healthcare: a survey. IEEE Rev. Biomed. Eng. , 1. doi:10.1109/RBME.2020.3013489
Qayyum, A., Usama, M., Qadir, J., and Al-Fuqaha, A. (2020b). Securing connected & autonomous vehicles: challenges posed by adversarial machine learning and the way forward. IEEE Commun. Surv. Tutorials 22, 998–1026. doi:10.1109/comst.2020.2975048
Reith, R. N., Schneider, T., and Tkachenko, O. (2019). “Efficiently stealing your machine learning models,” in Proceedings of the 18th ACM workshop on privacy in the electronic society , November 2019 , 198–210
Rouhani, B. D., Hussain, S. U., Lauter, K., and Koushanfar, F. (2018). Redcrypt: real-time privacy-preserving deep learning inference in clouds using fpgas. ACM Trans. Reconfigurable Technol. Syst. 11, 1–21. doi:10.1145/3242899
Saadatpanah, P., Shafahi, A., and Goldstein, T. (2019). Adversarial attacks on copyright detection systems. arXiv .
Salem, A., Zhang, Y., Humbert, M., Berrang, P., Fritz, M., and Backes, M. (2018). ML-leaks: model and data independent membership inference attacks and defenses on machine learning models. arXiv .
Sehwag, V., Bhagoji, A. N., Song, L., Sitawarin, C., Cullina, D., Chiang, M., et al. (2019). Better the devil you know: an analysis of evasion attacks using out-of-distribution adversarial examples. arXiv .
Sethi, T. S., and Kantardzic, M. (2018). Data driven exploratory attacks on black box classifiers in adversarial domains. Neurocomputing 289, 129–143. doi:10.1016/j.neucom.2018.02.007
Sharma, S., and Chen, K.. (2018). “Image disguising for privacy-preserving deep learning,” in Proceedings of the 2018 ACM SIGSAC Conference on Computer and Communications Security , ( ACM, Toronto, Canada ), 2291–2293
Shokri, R., Stronati, M., Song, C., and Shmatikov, V. (2017). “Membership inference attacks against machine learning models,” in 2017 IEEE Symposium on Security and privacy (SP) , San Jose, CA , May 22–26, 2017 ( IEEE ), 3–18
Simonyan, K., and Zisserman, A. (2015). “Very deep convolutional networks for large-scale image recognition,”in International Conference on Learning Representations (ICLR)
Song, Y., Liu, T., Wei, T., Wang, X., Tao, Z., and Chen, M. (2020). Fda3: federated defense against adversarial attacks for cloud-based iiot applications. IEEE Trans. Industr. Inform. , 1. doi:10.1109/TII.2020.3005969
Sun, Y., Wang, X., and Tang, X. (2014). “Deep learning face representation from predicting 10,000 classes,” in Proceedings of the IEEE conference on computer vision and pattern recognition , Columbus, OH , June 23–28, 2014 , ( IEEE ).
Szegedy, C., Vanhoucke, V., Ioffe, S., Shlens, J., and Wojna, Z. “(2016). Rethinking the inception architecture for computer vision,” in Proceedings of the IEEE conference on computer vision and pattern recognition (CVPR) , Las Vegas, NV , June 27–30, 2016 ( IEEE ), 2818–2826
Tramèr, F., Zhang, F., Juels, A., Reiter, M. K., and Ristenpart, T. (2016). “Stealing machine learning models via prediction APIs,” in 25th USENIX security symposium (USENIX Security 16) , 601–618
Tyndall, J. (2010). AACODS checklist . Adelaide, Australia: Adelaide Flinders University
Usama, M., Mitra, R. N., Ilahi, I., Qadir, J., and Marina, M. K. (2020a). Examining machine learning for 5g and beyond through an adversarial lens. arXiv . Available at: https://arxiv.org/abs/2009.02473 .
Usama, M., Qadir, J., Al-Fuqaha, A., and Hamdi, M. (2020b). The adversarial machine learning conundrum: can the insecurity of ML become the achilles' heel of cognitive networks? IEEE Network 34, 196–203. doi:10.1109/mnet.001.1900197
Usama, M., Qayyum, A., Qadir, J., and Al-Fuqaha, A. (2019). “Black-box adversarial machine learning attack on network traffic classification, “in 2019 15th international wireless communications and mobile computing conference (IWCMC) , Tangier, Morocco , June 24–28, 2019
Wang, B., Yao, Y., Viswanath, B., Zheng, H., and Zhao, B. Y. (2018a). “With great training comes great vulnerability: practical attacks against transfer learning,” in 27th USENIX security symposium (USENIX Security 18) , Baltimore, MD , August 2018 , 1281–1297
Wang, J., Zhang, J., Bao, W., Zhu, X., Cao, B., and Yu, P. S. (2018b). “Not just privacy: improving performance of private deep learning in mobile cloud,” in Proceedings of the 24th ACM SIGKDD International Conference on Knowledge Discovery & Data Mining London, United Kingdom , January 2018 , 2407–2416
Yang, Z., Zhang, J., Chang, E.-C., and Liang, Z. (2019). “Neural network inversion in adversarial setting via background knowledge alignment,” in Proceedings of the 2019 ACM SIGSAC conference on computer and communications security , London, UK , November 2019 , 225–240
Yuan, X., He, P., Zhu, Q., and Li, X. (2019). Adversarial examples: attacks and defenses for deep learning. IEEE Trans. Neural. Netw. Learn. Syst. 30 (9), 2805–2824. doi:10.1109/TNNLS.2018.2886017
Zhang, J., Zhang, B., and Zhang, B. (2019). “Defending adversarial attacks on cloud-aided automatic speech recognition systems, “in Proceedings of the seventh international workshop on security in cloud computing , New York , 23–31. Available at: https://dl.acm.org/doi/proceedings/10.1145/3327962
Keywords: Machine Learning as a Service, cloud-hosted machine learning models, machine learning security, cloud machine learning security, systematic review, attacks, defenses
Citation: Qayyum A, Ijaz A, Usama M, Iqbal W, Qadir J, Elkhatib Y and Al-Fuqaha A (2020) Securing Machine Learning in the Cloud: A Systematic Review of Cloud Machine Learning Security. Front. Big Data 3:587139. doi: 10.3389/fdata.2020.587139
Received: 24 July 2020; Accepted: 08 October 2020; Published: 12 November 2020.
Reviewed by:
Copyright © 2020 Qayyum, Ijaz, Usama, Iqbal, Qadir, Elkhatib and Al-Fuqaha. This is an open-access article distributed under the terms of the Creative Commons Attribution License (CC BY). The use, distribution or reproduction in other forums is permitted, provided the original author(s) and the copyright owner(s) are credited and that the original publication in this journal is cited, in accordance with accepted academic practice. No use, distribution or reproduction is permitted which does not comply with these terms.
*Correspondence: Adnan Qayyum, [email protected]

This article is part of the Research Topic
Safe and Trustworthy Machine Learning
- Original Papers
- Open access
- Published: 20 April 2010
Cloud computing: state-of-the-art and research challenges
- Qi Zhang 1 ,
- Lu Cheng 1 &
- Raouf Boutaba 1
Journal of Internet Services and Applications volume 1 , pages 7–18 ( 2010 ) Cite this article
90k Accesses
2082 Citations
28 Altmetric
Metrics details
Cloud computing has recently emerged as a new paradigm for hosting and delivering services over the Internet. Cloud computing is attractive to business owners as it eliminates the requirement for users to plan ahead for provisioning, and allows enterprises to start from the small and increase resources only when there is a rise in service demand. However, despite the fact that cloud computing offers huge opportunities to the IT industry, the development of cloud computing technology is currently at its infancy, with many issues still to be addressed. In this paper, we present a survey of cloud computing, highlighting its key concepts, architectural principles, state-of-the-art implementation as well as research challenges. The aim of this paper is to provide a better understanding of the design challenges of cloud computing and identify important research directions in this increasingly important area.
Al-Fares M et al (2008) A scalable, commodity data center network architecture. In: Proc SIGCOMM
Amazon Elastic Computing Cloud, aws.amazon.com/ec2
Amazon Web Services, aws.amazon.com
Ananthanarayanan R, Gupta K et al (2009) Cloud analytics: do we really need to reinvent the storage stack? In: Proc of HotCloud
Armbrust M et al (2009) Above the clouds: a Berkeley view of cloud computing. UC Berkeley Technical Report
Berners-Lee T, Fielding R, Masinter L (2005) RFC 3986: uniform resource identifier (URI): generic syntax, January 2005
Bodik P et al (2009) Statistical machine learning makes automatic control practical for Internet datacenters. In: Proc HotCloud
Brooks D et al (2000) Power-aware microarchitecture: design and modeling challenges for the next-generation microprocessors, IEEE Micro
Chandra A et al (2009) Nebulas: using distributed voluntary resources to build clouds. In: Proc of HotCloud
Chang F, Dean J et al (2006) Bigtable: a distributed storage system for structured data. In: Proc of OSDI
Chekuri C, Khanna S (2004) On multi-dimensional packing problems. SIAM J Comput 33(4):837–851
Article MATH MathSciNet Google Scholar
Church K et al (2008) On delivering embarrassingly distributed cloud services. In: Proc of HotNets
Clark C, Fraser K, Hand S, Hansen JG, Jul E, Limpach C, Pratt I, Warfield A (2005) Live migration of virtual machines. In: Proc of NSDI
Cloud Computing on Wikipedia, en.wikipedia.org/wiki/Cloudcomputing , 20 Dec 2009
Cloud Hosting, CLoud Computing and Hybrid Infrastructure from GoGrid, http://www.gogrid.com
Dean J, Ghemawat S (2004) MapReduce: simplified data processing on large clusters. In: Proc of OSDI
Dedicated Server, Managed Hosting, Web Hosting by Rackspace Hosting, http://www.rackspace.com
FlexiScale Cloud Comp and Hosting, www.flexiscale.com
Ghemawat S, Gobioff H, Leung S-T (2003) The Google file system. In: Proc of SOSP, October 2003
Google App Engine, URL http://code.google.com/appengine
Greenberg A, Jain N et al (2009) VL2: a scalable and flexible data center network. In: Proc SIGCOMM
Guo C et al (2008) DCell: a scalable and fault-tolerant network structure for data centers. In: Proc SIGCOMM
Guo C, Lu G, Li D et al (2009) BCube: a high performance, server-centric network architecture for modular data centers. In: Proc SIGCOMM
Hadoop Distributed File System, hadoop.apache.org/hdfs
Hadoop MapReduce, hadoop.apache.org/mapreduce
Hamilton J (2009) Cooperative expendable micro-slice servers (CEMS): low cost, low power servers for Internet-scale services In: Proc of CIDR
IEEE P802.3az Energy Efficient Ethernet Task Force, www.ieee802.org/3/az
Kalyvianaki E et al (2009) Self-adaptive and self-configured CPU resource provisioning for virtualized servers using Kalman filters. In: Proc of international conference on autonomic computing
Kambatla K et al (2009) Towards optimizing Hadoop provisioning in the cloud. In: Proc of HotCloud
Kernal Based Virtual Machine, www.linux-kvm.org/page/MainPage
Krautheim FJ (2009) Private virtual infrastructure for cloud computing. In: Proc of HotCloud
Kumar S et al (2009) vManage: loosely coupled platform and virtualization management in data centers. In: Proc of international conference on cloud computing
Li B et al (2009) EnaCloud: an energy-saving application live placement approach for cloud computing environments. In: Proc of international conf on cloud computing
Meng X et al (2010) Improving the scalability of data center networks with traffic-aware virtual machine placement. In: Proc INFOCOM
Mysore R et al (2009) PortLand: a scalable fault-tolerant layer 2 data center network fabric. In: Proc SIGCOMM
NIST Definition of Cloud Computing v15, csrc.nist.gov/groups/SNS/cloud-computing/cloud-def-v15.doc
Osman S, Subhraveti D et al (2002) The design and implementation of zap: a system for migrating computing environments. In: Proc of OSDI
Padala P, Hou K-Y et al (2009) Automated control of multiple virtualized resources. In: Proc of EuroSys
Parkhill D (1966) The challenge of the computer utility. Addison-Wesley, Reading
Google Scholar
Patil S et al (2009) In search of an API for scalable file systems: under the table or above it? HotCloud
Salesforce CRM, http://www.salesforce.com/platform
Sandholm T, Lai K (2009) MapReduce optimization using regulated dynamic prioritization. In: Proc of SIGMETRICS/Performance
Santos N, Gummadi K, Rodrigues R (2009) Towards trusted cloud computing. In: Proc of HotCloud
SAP Business ByDesign, www.sap.com/sme/solutions/businessmanagement/businessbydesign/index.epx
Sonnek J et al (2009) Virtual putty: reshaping the physical footprint of virtual machines. In: Proc of HotCloud
Srikantaiah S et al (2008) Energy aware consolidation for cloud computing. In: Proc of HotPower
Urgaonkar B et al (2005) Dynamic provisioning of multi-tier Internet applications. In: Proc of ICAC
Valancius V, Laoutaris N et al (2009) Greening the Internet with nano data centers. In: Proc of CoNext
Vaquero L, Rodero-Merino L, Caceres J, Lindner M (2009) A break in the clouds: towards a cloud definition. ACM SIGCOMM computer communications review
Vasic N et al (2009) Making cluster applications energy-aware. In: Proc of automated ctrl for datacenters and clouds
Virtualization Resource Chargeback, www.vkernel.com/products/EnterpriseChargebackVirtualAppliance
VMWare ESX Server, www.vmware.com/products/esx
Windows Azure, www.microsoft.com/azure
Wood T et al (2007) Black-box and gray-box strategies for virtual machine migration. In: Proc of NSDI
XenSource Inc, Xen, www.xensource.com
Zaharia M et al (2009) Improving MapReduce performance in heterogeneous environments. In: Proc of HotCloud
Zhang Q et al (2007) A regression-based analytic model for dynamic resource provisioning of multi-tier applications. In: Proc ICAC
Download references
Author information
Authors and affiliations.
University of Waterloo, Waterloo, Ontario, Canada, N2L 3G1
Qi Zhang, Lu Cheng & Raouf Boutaba
You can also search for this author in PubMed Google Scholar
Corresponding author
Correspondence to Raouf Boutaba .
Rights and permissions
Open Access This article is distributed under the terms of the Creative Commons Attribution 2.0 International License ( https://creativecommons.org/licenses/by/2.0 ), which permits unrestricted use, distribution, and reproduction in any medium, provided the original work is properly cited.
Reprints and permissions
About this article
Cite this article.
Zhang, Q., Cheng, L. & Boutaba, R. Cloud computing: state-of-the-art and research challenges. J Internet Serv Appl 1 , 7–18 (2010). https://doi.org/10.1007/s13174-010-0007-6
Download citation
Received : 08 January 2010
Accepted : 25 February 2010
Published : 20 April 2010
Issue Date : May 2010
DOI : https://doi.org/10.1007/s13174-010-0007-6
Share this article
Anyone you share the following link with will be able to read this content:
Sorry, a shareable link is not currently available for this article.
Provided by the Springer Nature SharedIt content-sharing initiative
- Cloud computing
- Data centers
- Virtualization
Analysis of Big Data in Cloud Computing Technologies
Ieee account.
- Change Username/Password
- Update Address
Purchase Details
- Payment Options
- Order History
- View Purchased Documents
Profile Information
- Communications Preferences
- Profession and Education
- Technical Interests
- US & Canada: +1 800 678 4333
- Worldwide: +1 732 981 0060
- Contact & Support
- About IEEE Xplore
- Accessibility
- Terms of Use
- Nondiscrimination Policy
- Privacy & Opting Out of Cookies
A not-for-profit organization, IEEE is the world's largest technical professional organization dedicated to advancing technology for the benefit of humanity. © Copyright 2024 IEEE - All rights reserved. Use of this web site signifies your agreement to the terms and conditions.
Advances, Systems and Applications
- Open access
- Published: 12 July 2021
Survey on serverless computing
- Hassan B. Hassan 1 ,
- Saman A. Barakat 2 &
- Qusay I. Sarhan 2
Journal of Cloud Computing volume 10 , Article number: 39 ( 2021 ) Cite this article
30k Accesses
46 Citations
1 Altmetric
Metrics details
Serverless computing has gained importance over the last decade as an exciting new field, owing to its large influence in reducing costs, decreasing latency, improving scalability, and eliminating server-side management, to name a few. However, to date there is a lack of in-depth survey that would help developers and researchers better understand the significance of serverless computing in different contexts. Thus, it is essential to present research evidence that has been published in this area. In this systematic survey, 275 research papers that examined serverless computing from well-known literature databases were extensively reviewed to extract useful data. Then, the obtained data were analyzed to answer several research questions regarding state-of-the-art contributions of serverless computing, its concepts, its platforms, its usage, etc. We moreover discuss the challenges that serverless computing faces nowadays and how future research could enable its implementation and usage.
Introduction
Cloud computing emerged after the appearance of virtualization in software and hardware infrastructures; hence cloud providers increasingly adopted it to offer their services to customers [ 1 , 2 ]. Customers can access these cloud services via the Internet. Software developers have been using cloud technologies in their software solutions owing to their benefits including scalability, availability, and flexibility [ 3 ].
In general, cloud computing is divided into three main categories based on the provision of services, which are software as a service (SaaS), platform as a service (PaaS), and infrastructure as a service (IaaS). In the SaaS category, cloud providers offer different types of software as services to the users. For example, Google provides many applications as a service (e.g., Gmail, Google docs, Google sheets, and Google forms). In this type of cloud, the user is not responsible for the services development, deployment, and management. The user here only uses them without worrying about their settings, configurations, etc. Meanwhile, in the PaaS, cloud companies provide services such as network access, storage, servers, and operating systems to be purchased by developers. The developers access these services to deploy, run, and manage their applications. In this kind of cloud, the developer is responsible for the deployment and management (settings and configurations) of their software to ensure that the application is running, while they do not control the services. Finally, in the IaaS category, the cloud consumers control and manage services such as network access, servers, operating systems, and storage.
Managing cloud services is not an easy task at all. The authors in [ 4 ] have addressed several challenges while managing a cloud environment by a user such as availability, load balancing, auto-scaling, security, monitoring, etc. For example, the cloud user has to ensure the availability of the services in which if a single machine failure occurs, it does not affect the whole services. Also, he/she has to consider distributing copies of the services geographically to protect them when disasters happen. Another challenge is load balancing. In this case, the cloud user has to ensure that requests to the services are balanced to utilize all resources efficiently.
These challenges have led to introduce another cloud computing model, which is called serverless cloud computing [ 4 ]. Serverless cloud computing offers backend as a service (BaaS) and function as a service (FaaS), as shown in Fig. 1 . The BaaS includes services like storage, messaging, user management, etc. While, the FaaS enables developers to deploy and execute their code on computing platforms. The FaaS relies on the services provided by the BaaS such as a database, messaging, user authentications, etc. The FaaS is considered as the most dominant model of serverless, and it is also known as “event-driven functions” [ 5 , 6 ].
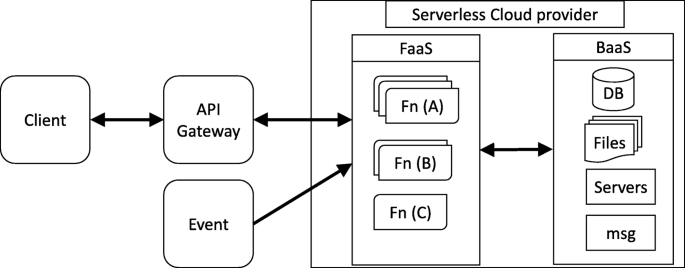
Serverless architecture
Serverless cloud model was for the first time introduced by Amazon Lambda in 2014, after which cloud companies like Google and Microsoft adopted it in 2016. Serverless cloud computing adds an additional abstraction layer to the existing cloud computing paradigms, while it abstracts away the server-side management from the developers [ 7 ]. Serverless model lets the developers focus on the application logic rather than the server-side management and configurations. For example, the developers deploy their applications to the serverless cloud as functions see Fig. 1 . Then, the cloud provider takes responsibility for managing, scaling, and providing different resources to ensure the smooth running of these functions [ 8 , 9 ].
However, FaaS and the term “serverless” could be used interchangeably, as the FaaS platform automatically configures and maintains the execution context of functions and connects them to cloud services without requiring server provision by developers [ 10 , 11 ]. We refer to the FaaS when we use the term serverless computing.
Serverless cloud computing has many good characteristics [ 12 , 13 ], one of which is scalability. Scaling could be vertical or horizontal; vertical scaling adds or removes cores from the running container, while horizontal scaling creates new containers or eliminates running ones without affecting the current resource allocations [ 14 ]. In serverless computing, the applications automatically scale up and down on demand, and the developer does not have to concern themselves about the scaling issues. For example, when an application runs on a serverless cloud, it will scale up automatically when the application requests increase. Another characteristic of serverless computing is the payment per resource usage. This paradigm of cloud computing charges developers based on the actual resource usage. For example, deploying an application will not cost the developer in the case where the application is idle, and the serverless provider will only charge whenever the application has started using resources.
However, any new technology will face numerous technical and operational issues and obstacles at the beginning. Since the recent introduction of serverless cloud computing, several drawbacks have been identified [ 7 ]. Serverless cloud computing lacks tools that help managing and monitoring serverless applications. Moreover, it might comprise security concerns. Further, the serverless providers have a vendor lock-in problem. Nevertheless, serverless cloud computing has gained positive attention in the industry, despite that it has not been studied extensively in academic research [ 7 ].
Therefore, the aim of this research is to answer some crucial research questions related to serverless cloud computing and thereby help researchers as well as developers to better understand serverless cloud computing and contribute to its development.
The rest of this paper is structured as follows: “ Related works ” section presents the related works for this study. “ Research methodology ” section describes in detail the research methodology used to conduct this survey study. “ Results ” section presents the results and outcomes of the study. “ Threats to validity ” section presents the threats to validity of this study. Finally, the conclusions of the study are provided in “ Conclusions ” section.
Related works
The most relevant studies published on the topic are briefly presented here. The authors in [ 15 ] and [ 16 ] discussed some important background to the origin and evolution of serverless computing and the long road that serverless computing has taken over the years. The authors in [ 9 ] thoroughly discussed the true meaning of serverless architectures and how they are changing the way in which applications are built, deployed, and distributed.
Numerous studies focused on technical interpretations of serverless computing, while other more recent research suggested various benefits that it brings to developers. Nowadays, this type of computing is being used in several ways. In an empirical study, the authors in [ 17 ] aimed to investigate the development practices of serverless computing in the industry. They concluded that for developers, it remains a barrier to adopt the right mindset to best utilize the tools inherent to serverless architecture. More documentation and easier access to such resources would help developers to embrace the possibilities that serverless computing has to offer.
The concept of serverless computing within the scope of the IT industry has the great potential of progressively increasing its capabilities to involve a wider set of domains. Thus, the implementation of serverless computing is not restricted only to the enhancement of infrastructure, and it can be employed for many different purposes, e.g., serverless messaging, neural network training [ 18 ], video processing [ 19 ], and big data [ 20 ]. Undeniably, their contributions are valuable to the general public and researchers in the field, as it is of primarily importance to comprehend how this technology works.
However, it is presently crucial to provide more than only theories and concepts: it is time to weigh the benefits and drawbacks of serverless computing and to analyze how far the field has progressed, to assess what remains to be done and improved. As an example, the authors in [ 21 ] discussed some possible new abstraction levels to reduce processing limitations. The authors in [ 22 ] discussed the results from an open-source framework to achieve on-premises serverless computing that can process big workloads with a scalable and sensible usage of resources. We can infer from these related publications that researchers everywhere are working to determine how to best exploit the potential that serverless computing frameworks could introduce to software development.
In [ 23 ], the authors described how serverless computing is becoming the next step in the evolution of cloud computing and its platforms. In our paper, we focus on the ongoing challenges, benefits, and drawbacks of using it.
The authors in [ 24 ] have conducted a systematic exploration of serverless computing-related research papers. As they mentioned, their work is not a survey, but it is a supporting source for future research papers. They proposed an open dataset for serverless computing papers. The dataset includes 60 papers for the period (2016-July 2018). Also, they have analyzed the dataset according to bibliometric, content, technology, and produced statistics about each section. In contrast, our paper aims to conduct a systematic survey. In this survey, we try to find answers to several critical questions related to serverless computing. In addition to that, our study covered the duration (2016–2020) and thus 275 papers have been considered.
The authors in [ 25 ] mainly focused on scheduling tasks in the cloud. They described the various techniques in scheduling workflows to reduce the execution time, cost, or both. Moreover, they proposed a hybrid method by both FaaS and IaaS. The small tasks could be executed remotely using the FaaS, which reduces the execution cost; hence, the number of virtual machines will be decreased as well. Therefore, the whole focus would be on the long-running tasks on IaaS.
The authors in [ 26 ] covered only 24 research papers during 2017–2019. In their paper, they considered both the white and grey literatures. Besides, they identified 32 characteristics of serverless and the possible issues related to them, only eight of them were stated by both white and grey literatures while the remaining are from grey literature only. All the characteristics are explained and presented briefly. In our paper, 275 research papers from 2016–2020 have been covered and more research questions have been answered. Besides, a well-defined systematic literature study process has been employed. Thus, the grey literature has been excluded in our paper and, our results are reproducible compared to their results.
The authors in [ 27 ] mainly concentrated on difficulties and gaps in data-centric and distributed computing using FaaS. Additionally, they evaluated the severity of these challenges via taking three case studies from big data and distributed computing settings: model training, low-latency prediction serving using the batch and, distributed computing. While our paper is a broad and comprehensive study on FaaS, 275 research papers are taken from the white literature during 2016–2020.
The paper [ 28 ] presented only four use cases of FaaS: event-triggered computing, video broadcasting, Internet of Things (IoT) data processing, and shared delivery system. Additionally, the paper only compared three platforms namely, Amazon web services (AWS) Lambda, Google Cloud Function, and Microsoft Azure Function. On the other hand, our paper presents a comprehensive study about FaaS. We identified in detail eight use cases: chatbot, information retrieval, file processing, smart grid, security, networks and, mobile and IoT. Moreover, our paper compared ten FaaS platforms namely, AWS Lambda, Apache OpenWhisk, Microsoft Azure functions, Google Cloud functions, OpenLambda, IBM Cloud functions, OpenFaaS, Knative, FunctionStage, Huawei Cloud, and Nuclio.
The authors in [ 29 ] covered only 15 papers during 2016–2018. They took both the white and grey literatures into account. On the other hand, our paper includes 275 research papers published in the period 2016–2020; they are taken from the white literature only. Moreover, our paper has formulated and answered eight clear and well-defined research questions.
The authors in [ 30 ] focused on the FaaS performance evaluation and their publication trends during 2016–2019. They identified the most commonly evaluated FaaS platforms. Additionally, they evaluated the performance features for benchmark types, micro-benchmarks, and common features across FaaS platforms. Moreover, they presented the most common platform configurations in FaaS, namely language runtimes, function triggers, and external services. This paper presents a survey of the most important and state of the art aspects of FaaS. Besides, comprehensive theoretical aspects of FaaS are covered taking from the white literature during 2016–2020.
The authors in [ 11 ] have conducted a systematic mapping study on serverless cloud computing. The main aim of their study is to concentrate on FaaS engineering. They raised two main concerns: (a) identifying publication research that considers developing or modifying serverless platforms and tools. (b) identifying the challenges and drivers related to these publications. On the other hand, our study extends the challenges and issues related to serverless computing. Moreover, we provide more details about serverless computing platforms and the use of these platforms in the literature. Also, it provides a detailed comparison among the most widely used serverless platforms. Besides, it addresses more aspects of serverless cloud computing such as application areas of serverless computing, future directions of serverless computing, etc.
The authors in [ 7 ] provided useful observations about serverless computing, its architecture, and use cases. Also, they discussed the challenges and benefits of moving forward from monolithic applications and the differences between traditional cloud services and serverless computing. Our work has extended the details of their work regarding the benefits and drawbacks of using serverless computing. It has also included more use cases and workloads to deepen the findings of previous studies.
The authors in [ 4 ] presented a technical report on serverless computing. They covered the serverless emergence with its limitations, including limited storage for fine-grained tasks, lack of coordination among functions, inadequate performance for standard communication patterns, and functions’ performance. Also, they compared AWS serverful with AWS serverless. Moreover, they also explained the challenges of architecture, networking, security, and abstractions of serverless computing. They identified five application areas including, video encoding in real-time, MapReduce, linear algebra, machine learning training, and databases. While our paper has covered 275 research papers from 2016–2020 forming a well-defined systematic literature study. We also identified 21 serverless challenges and issues. Besides, we compared serverless with the traditional cloud computing paradigm. We identified more application areas including, chatbot, information retrieval, file processing, smart grid, security, networks, IoT, and edge computing.
The authors in [ 31 ] presented a white paper based on published research papers during 2015–2017. They outlined the serverless definition alongside its advantages and disadvantages. Also, they classified serverless use-cases into six categories, namely, backends, web applications, chatbots, big data, IT automation, and Amazon Alexa. Moreover, they addressed a few research questions including, the need for the stateless feature in serverless, whether serverless could execute long-running tasks, programming models, serverless standards, and the importance of serverless for scientific research. While our paper is a comprehensive study on FaaS; we covered 275 research papers which are taken from the grey literature during 2016–2020. In our paper, eight application areas have been identified as mentioned earlier. We have identified and answered ten research questions that cover many aspects of the topic in detail compared to the aforementioned study.
We are in fact addressing with this paper ten important research questions about the topic, potentially making it a more complete guide to the development and use of serverless computing. Our work contributes to the analysis of the serverless paradigm in the context of similar applications and how could they better fit specific computing needs. Moreover, information about the current state of serverless platforms, tools, and frameworks has been updated for this survey. This due to the importance of the topic and its potential to change how both the industry and academia have managed the deployment of cloud applications until now. Updated information about the area could benefit future studies focused on the serverless computing paradigm as they make researchers aware of the latest resources and opportunities in the area.
Research methodology
Research questions.
In this study, a number of research questions (RQs) have been identified and answered. Each RQ addresses a particular aspect of serverless computing as follows.
RQ1. What is the number and distribution of studies published on serverless computing in the period (2016–2020)?
RQ2. Which researchers, organizations, and countries are active in serverless computing research?
RQ3. What are the differences between serverless computing and traditional cloud computing?
RQ4. What are the benefits of using serverless computing?
RQ5. What are the most used software platforms that enable serverless computing in the literature?
RQ6. What are the application areas of serverless computing in the literature?
RQ7. What are the challenges and issues of using serverless computing?
RQ8. What tools are available for serverless computing? (serverless tools)
RQ9. What are the available research approaches to analyze the migration of monolithic applications to serverless computing?
RQ10. What are the potential future directions of research on serverless computing?
Search strategy
Literature sources.
In this study, five standard online databases have been selected as sources that index the literature of software engineering and computer science. These sources are presented in Table 1 .
Search string
To find the publications relevant to this study, the following extensive search string has been applied on the database sources of literature:
(serverless OR FaaS OR “function as a service” OR “function-as-a-service”) AND (computing OR paradigm OR architecture OR model OR application OR function OR service OR platform OR programming)
To obtain the best publication list, a generic search string is created. It contains serverless cloud computing-related keywords. The string with duration (2016 - 2020) have been applied to all libraries. Because the Springer Link library covers many fields, the result of search was greater than other libraries. This because the keyword FaaS is used in many research areas for different purposes. For instance, fish as a service (FaaS) and FPGA as a fervice (FaaS). Therefore, we used Computer Science subject filter with Springer Link, ScienceDirect, and Scopus to reduce the number of incorrect papers. The results of the initial search are shown in Fig. 2 . Additionally, some inaccurate results have been obtained due to the partial similarity to FaaS, such as the federal aviation administration (FAA). The results of the initial search were 5,021 papers in total.
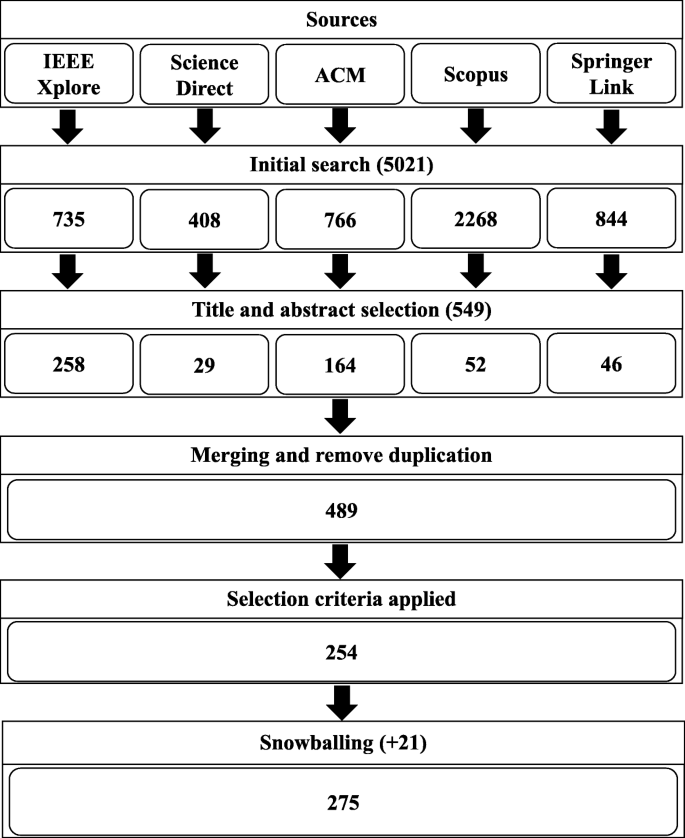
Results of papers selection process
After obtaining the initial list of publications, some filters have been applied to reduce the number of incorrect results based on their relation to the serverless computing and FaaS topics. Most of the papers have been analyzed based on the title and abstract. However, when we were unable to make a decision based on the title and the abstract, we read the content of the paper to ascertain whether to include or exclude. As a result, the list of papers which are related to serverless computing has been decreased to 549 papers.
After filtering the papers based on the title and abstract, we merged all the papers that were relevant to serverless cloud computing, which was 549 papers into a single dataset. Then we removed the duplicated papers based on the combination of a title, author names, publication year, and venue. Thus, the number of publications has been reduced to 489 papers.
Then, the publications have been selected based on the content of the paper and based on a set of inclusion/exclusion criteria (see the following section) that have been selected carefully. Eventually, we could obtain 254 papers that are related to serverless cloud computing. In the next step, we applied backward snowballing to increase the set of relevant papers to serverless cloud computing. In this phase, we could add 21 more papers to our list of papers. As a result, the total numbers of relevant papers become 275 papers. The list of these papers and its meta-data have been published in Zenodo website as a dataset [ 32 ].
Paper inclusion/exclusion criteria
To decide whether a publication is relevant to the scope of this research, a set of inclusion and exclusion criteria have been established and employed as follows:
Inclusion criteria:
Publications in the field of software engineering and computer science.
Publications published online from 2016 – 2020.
Publications related directly to serverless computing.
Exclusion criteria:
Publications not published in English.
Publications without accessible full text.
Publications not formally peer reviewed (e.g., gray literature).
Publications not published electronically.
Publications that are duplicates of other previous publications.
The selected publications were carefully read to answer the raised RQs. Here, a short title is used to represent each RQ. The following subsections present and discuss the results based on each RQ.
Distribution of publications (RQ1)
Publication frequency.
All the selected papers of this study were analyzed to determine their frequency and evolution. Figure 3 shows the results of this analysis. The results show that the average number of publications per year is approximately 55 papers.
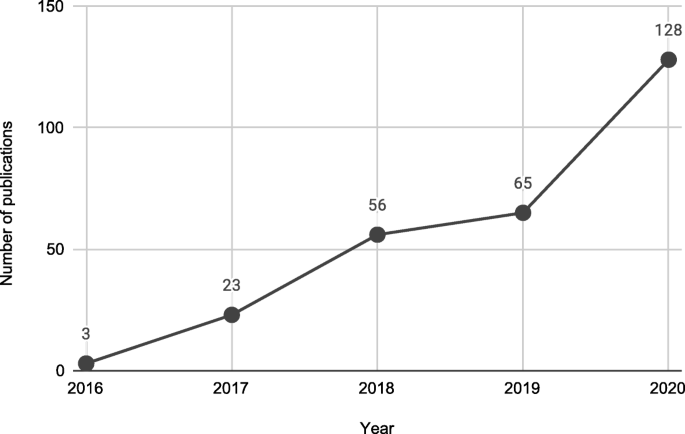
Published papers per year
Serverless computing has trended a significant engagement over the past two years. This boost has been caused by industry, academia, and developers for several reasons. The first important reason is the attractive engagement opportunities that serverless offers cloud providers. Serverless nature equipped cloud providers with more convenient and efficient methods to manage and utilize idle computing resources. Another reason is that the billing is only on the basis of function execution time and resource allocation. Also, the developers are not required to be aware of the underlying infrastructure and workflows. Hence, this attracts cloud providers and businesses to migrate and support serverless alongside many directions. At the same time, researchers are paying more attention to serverless as it is becoming the future paradigm for cloud computing. Moreover, current challenges and limits in serverless computing draw attention to more academics to address the issues and enhance the currently available features. For the aforementioned reasons, developers and customers are well encouraged and satisfied to select serverless computing for developing applications and services.
Publication venue
The distribution of the selected papers in various publication venues is shown in Fig. 4 . The percentages of publications in conference papers, workshop papers, symposium papers, and journal papers are approximately 62%, 11%, 14%, and 13%, respectively. However, almost 13% of the studies have been published in journals, which indicates the immaturity of research in serverless computing [ 33 , 34 ]. It is worth mentioning that some conference papers were published as book chapters. Thus, the original venues, which are conferences, of such papers were considered.
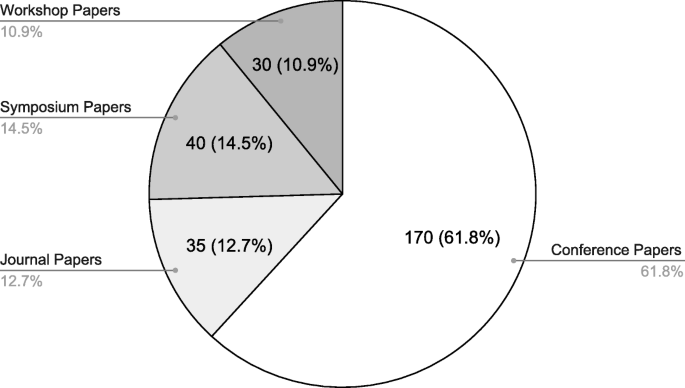
Published papers ratio per each venue
Following the interpretation of publications, the most productive and primary journals, symposiums, conferences, and workshops venues related to serverless computing can be clarified. Due to their long names, abbreviations are used in this paper. The active journals are shown in Fig. 5 and their full names can be found in Table 2 . It can be observed from the figure that the top and vital three journals are “FGCS”, “IoT”, and “JSS”. Also, it can be noticed that the top three journals contain almost 34% of the published journal papers, while the others own approximately 66%.
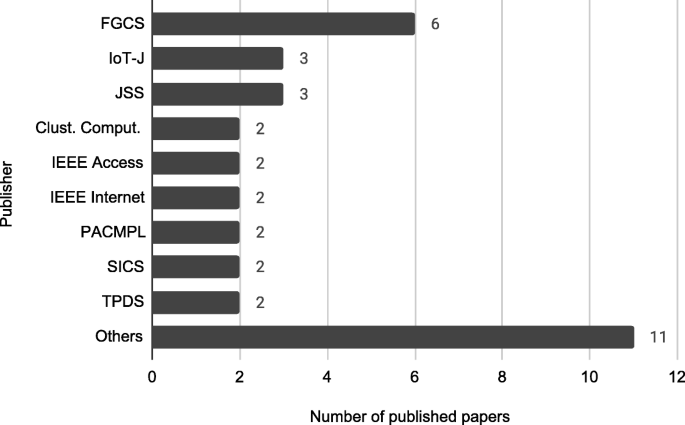
Published papers vs. journal name
The active conferences are shown in Fig. 6 and their full names are presented in Table 3 . The “WOSC”, “Cloud”, “UCC”, “SoCC”, and “Middleware” are considered the most active conferences that hold approximately 28% of the published conference papers. By including other conferences with three published papers or more, then approximately 23% of the conference papers are published in annual conferences. The majority (almost 49%) of the conference papers were published at individual conferences, which are denoted as “Others” in Fig. 6 .
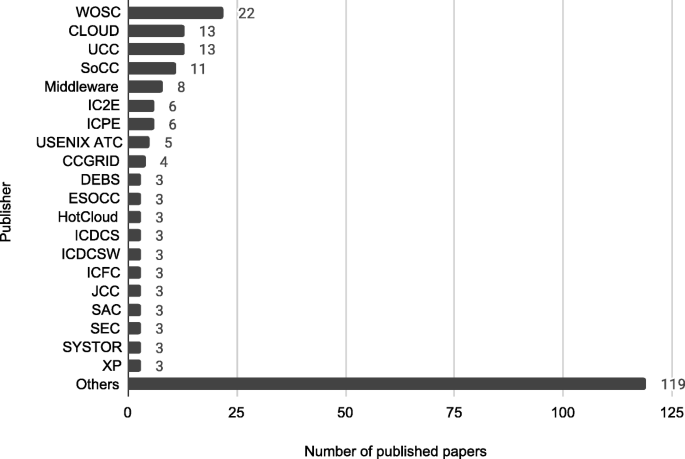
Published papers vs. conference name
Active researchers (RQ2)
Serverless computing is a vital research area through the contribution of several scholars. Yet, the researchers are counted active if they contributed to more than two research studies, as presented in Fig. 7 . The figure shows that the top six active researchers are “Pedro Garcáa López”, “Erwin Van Eyk”, “Alexandru Iosup”, “Marc Sánchez-Artigas”, “Sebastian Werner”, and “Wes Lloyd”. Table 4 presents the active nations, research institutions, researchers, references to the published papers, and the total number of publications.
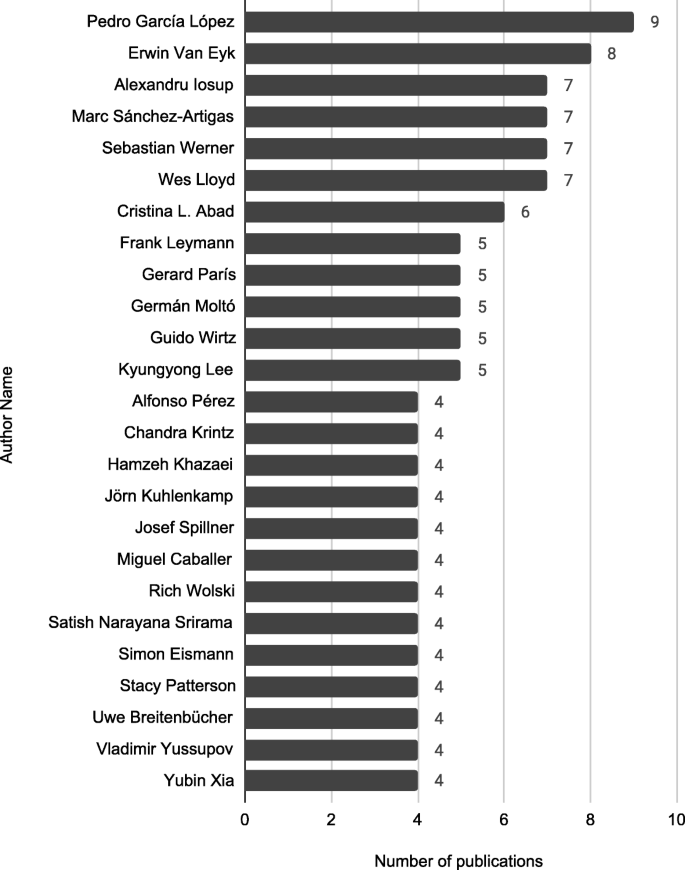
Active researchers based on the published papers
The active nations in the number of papers are obtained from the information presented in Table 4 by extracting the institutional affiliation of the authors and co-authors. An overview of the most active nations and the total number of publications is shown in Fig. 8 . It is observable that the United States and Germany are the largest contributors to papers published on serverless computing with 104 and 39 published papers, respectively.

Active countries
Serverless computing vs. traditional cloud
computing (RQ3) There are several differences between serverless and traditional cloud computing. In the traditional cloud architecture, the server acts as a monolithic system containing all business logic. Meanwhile, the serverless architecture is modeled into smaller, event-driven, and stateless ‘triggers’ (events) and ‘actions’ (functions) [ 175 ]. Each component handles different pieces of data and runs independently [ 176 ]. Spreading business logic into smaller functions increase the development efficiency [ 77 , 177 ] and also decreases the chance of a single point of failure [ 77 ]. On the other hand, the component dependency within monolithic applications affects the availability of other services adversely.
In a serverless architecture, the developers are unable to take control of listening to the TCP socket, managing load balancers, maintenance or configuration of the server, as well as provisioning and resource allocation. Therefore, there is no need for system administrators; the developers only focus on handling client requests and paying attention to deliver valuable services [ 8 ].
Serverless computing also differs from monolithic computing as the functions have shorter life cycles.
The traditional monitoring and debugging tools that are used in monolithic applications are not included in the serverless architecture; the developers are compelled to use built-in tools for debugging and monitoring. The computing power is no longer a concern for the developers in the serverless paradigm, as it could scale horizontally almost indefinitely [ 178 , 179 ]. Meanwhile, in the client-server architecture, it usually requires dedicating two server instances; the primary instance and a second in case if the former fails. This leads to higher costs in the monolith paradigm. Serverless architecture could be more economical for unsteady load conditions while the server-based is more suitable for steady loads [ 152 ]. As serverless applications scale up and down according to the requests, thus, unlike the traditional systems, it is unnecessary to keep the sessions in the memory [ 8 ]. Hence, it is difficult to keep track across requests.
FaaS boosts the security level as cloud providers continuously update their infrastructure with the latest security patches; this also removes the security burden on developers [ 17 ]. Directly accessing the backend resources in the traditional model is considered a critical security issue. Thus, any requests from the clients and internal functions in the serverless environment must go through a distributed request-level authorization mechanism that strengthens the security level [ 8 ]. Additionally, denial of service (DoS) attacks are controlled, as it is more difficult to attack distributed servers than a single server [ 175 ]. However, some security concerns remain due to the third-party API usage [ 9 ]. Besides, there is a lack of tools to identify vulnerabilities and access control risks. Table 5 summarizes the aforementioned differences.
Benefits of serverless computing (RQ4)
Serverless computing offers numerous benefits to its users, and Table 6 presents papers that states these benefits. This section summarizes those benefits as follows:
Cost effective
Serverless applications are abstracted from server infrastructure, which results in cost-based services depending on usage [ 180 ]. For example, applications run whenever a user makes a request to a service within the application. The cloud vendors charge only for the used space, and there is no cost while their applications are in an idle state.
Scalability
Serverless reasonably solved the resource allocation problem [ 191 ]. Therefore, developers do not have to concern themselves with the application scalability, because the application will scale automatically whenever user application requests are increased. If there are numerous requests to a function within the application, the serverless providers will start servers to handle these requests.
Server-side management
In serverless computing, developers do not need to concern themselves with the server-side and its management. Serverless cloud providers take care of managing and maintaining the hardware and software required to deploy applications. In addition to that, they handle all administration operations to let developers focus on different kinds of resources such as central processing unit (CPU), memory, and storage.
Easy to deploy
Serverless applications are easy to deploy. For example, to deploy an application, developers only need to upload some functions and release a new product. The serveless will take care of deployment management and infrastructure related concerns such as server provisioning and scaling.
Decrease latency
Serverless applications are not hosted on a specific server; the code can run from any server in any location. Therefore, cloud vendors can run the application on servers near the end user’ location. This reduces latency, because end user requests do not have to travel across the Internet to access the original server.
Serverless platforms in the literature (RQ5)
The software platforms are generally implemented to deal with resources from several clouds and ensure proper running of client applications. The heterogeneous nature of the cloud providers’ infrastructure (hardware and operating systems) led to the necessity to direct the developers’ focus to the functional part, rather than the underlying infrastructure [ 199 ].
With the emergence of the first serverless platform, AWS Lambda by Amazon in 2014 [ 8 ], cloud computing has evolved to a new generation referred to as serverless computing. However, serverless was not a brand-new paradigm; it emerged after the advancements in adopting virtual machines and container technologies [ 120 ]. By 2016, other competitors, namely Google, Microsoft, and IBM followed the trend. Several commercial and open-source platforms offer serverless computing. The well-known commercial systems are AWS Lambda, Google Cloud Functions, and Azure functions. Alternately, there are several open source platforms available including IBM Cloud Functions, and Apache OpenWhisk.
There are several criteria to help developers in selecting a serverless platform: cost, performance, supported programming languages and model, deployment easiness, easiness in composing functions from different providers, security, and monitoring and debugging tools [ 184 ].
Table 7 presents the serverless platforms used in the considered papers of this study. It can be noted that “AWS Lambda”, “Apache OpenWhisk”, and “Azure Functions” are the most used platforms with 78, 23, and 11 published papers, respectively. However, it is worth mentioning that each platform has its own set of features and differs from others.
The application areas of serverless computing in the literature (RQ6)
Serverless computing can be utilized in a number of application areas as follows:
A chatbot application is developed using serverless computing, which enables interaction with users via voice or text commands. Within this application, six functionalities have been implemented, namely the Date, News, Jokes, Weather, Music Tutor, and Alarm Service. For example, a user can ask for the current date using a voice or text command. The request is routed to a required serverless action on OpenWhisk for further processing. The Date action is activated via the issued command and retrieves the current date to the user in the form of text or voice [ 44 ].
Another example is the ticketing chatbot service developed using serverless computing and natural language processing (NLP). The architecture of the system consists of three parts: (1) the node.js Webhook, which works based on hypertext transfer protocol (HTTP) POST or GET requests (2) Wit.AI, which is a NLP service (3) Ticket.com, which is a ticketing order API. For example, when a user books a flight ticket; a specific function on Webhook will be activated, which routes the user query to the Wit.AI service. Wit.AI will process the query and extract useful parameters from the request such as destination, date, and time, then send it back to Webhook. After receiving the processed query from Wit.Ai; another action will be triggered and pass the processed query to Tickt.com API to retrieve flight information such as the flight number, airline name, departure time, and ticket price from several airline companies. Finally, Webhook will provide flight information to the user [ 44 , 179 , 248 ].
Information retrieval
A search engine web-based application is developed based on serverless architecture. Search engine functionalities are implemented as Amazon lambda functions. The search engine executes all the details of retrieval processing after receiving the user query (e.g., tokenization, stop-word removal, term weighting, similarity calculation, and ranking). Then, it sends back the results to the user as documents stored in the DynamoDB database to be accessed using the web application interface [ 173 ].
File processing
Serverless computing can been utilized in file processing applications [ 119 , 249 ]. For instance, in [ 119 ] a model for highly parallel file processing applications based on serverless architecture is proposed. This model provides users with different ways to process their files.
The first method is by using the API gateway. In this method, users submit files using the HTTP request employing the API gateway to a lambda function to process the file (e.g., medical images and video files).
The second method is by uploading/reading files to the Amazon simple storage service (Amazon S3) bucket. This method provides the user with three different ways to execute a lambda function using S3 buckets: (a) by uploading a file to S3 buckets. When the file is uploaded, S3 creates an event to invoke a lambda function; (b) by copying a file from another bucket to the bucket linked with the lambda function. This will cause the trigger of an event from S3 to invoke a lambda function as in the previous manner; (c) by specifying a bucket where the files to be processed are stored. Then, for each file found, the lambda function is invoked in parallel using an S3 bucket.
The third method is by specifying the output file. By this method, the user can set a chain of lambda functions to be invoked by S3 buckets. In this case, the user defines the input/output buckets for each of the lambda functions. Thus, the output bucket can be used as an input to another lambda function [ 119 ]. Here, serverless functions can handle different types of data (stored in files) such as sensory, textual, and biological data [ 200 ]. Also, many preprocessing operations using NLP may be applied to data files before processing, such as stemming and noise removal [ 78 ].
A MATLAB simulation scenario is created to illustrate the use of the smart grid with serverless cloud computing to control and manage electrical loads (devices). In this scenario, the Simulink tool is employed for simulation. A MATLAB program is developed to indicate the start and end of the simulated grid model via a batch file. The batch file is used to upload grid model data generated by the program to Amazon S3. Afterwards, a lambda function in the serverless side will be activated to process the uploaded data, and subsequently the result will be sent back to the batch file as a response. In return, the program will read continuously the response from the batch file and interpret its content to manage the electrical switch (loads) [ 201 ].
Also, An electrical overload warning system is implemented in the smart grid, based on serverless architecture. The system uses some Amazon web services, including S3, lambda functions, simple notification service (SNS), and CloudWatch. S3 is used as a storage service in the system. Lambda functions constitute a computing service that executes the code of the application. CloudWatch is a monitoring tool that monitors AWS resources and applications. The SNS is a notification service that sends and receives notifications.
The main sections of this warning system consist of data collection, data acquisition, data analysis, data mining, conclusion verification, and conclusion publishing. In this architecture, the AWS Lambda is used in data analysis and data mining. AWS CloudWatch is used for data conclusion verification. The SNS is used to generate alarms. For instance, the data is uploaded to S3, and subsequently, a lambda function is activated for data analysis and data mining. After the lambda function execution, its log data is stored in CloudWatch logs. CloudWatch is used for conclusion verification. CloudWatch defines an alarm size to a specific value, upon which it compares the value of log data with a predefined alarm size to check the current state. Then, the CloudWatch uses SNS for publishing conclusions. If the receiving data is greater than the alarm size, an alarm signal will be triggered and send an email via SNS [ 5 ].
An automated threat detection system is introduced using serverless cloud computing and Kubernetes. Kubernetes is an open source system to automatically deploy and manage application containers [ 243 , 250 ]. The system deals with threats (e.g., software vulnerabilities and insecure configurations) automatically based on user-defined policies. The system includes a vulnerability scanner (VS), which is a thread detection component. Whenever users deploy new application containers, the containers are registered with the VS, and a scanner agent is installed. When a thread is detected by the scanner, a notification is sent to the OpenWhisk component, which activates a serverless function that takes actions to reduce the threat. OpenWhisk will invoke a Kubernetes API extension and let the security enforcement operator (SEO) handle the operation [ 35 ].
Serverless cloud computing has been employed in different networking domains[ 175 , 188 , 251 , 252 ]. In [ 188 ], a variety of networking fields including software-defined networking (SDN) which can utilize advantages of serverless computing architecture have been discussed. The SDN is a network architecture approach that enables the network to be manageable and adaptive. This architecture separates the network control plane from the forwarding functions (the data plane). This decoupling enables network switches to become a simple forwarding device, and the network control is implemented as a network application that executed on a logically centralized controller. Serverless computing can be used in the SDN controllers. These controllers can be implemented as independent functions deployed on serverless platforms. For example, when a packet arrives to the SDN forwarding device, the device will parse the packet header and forward it to the SDN controller. The functions within the SDN controller will be activated then it will determine what action to be taken with the packet. After that, it will send the information to the forwarding device. The action might be modifying the header, dropping the packet, etc.
Serverless computing has been utilized in many IoT applications, as shown in Table 8 . For example, a camera can be installed to monitor a house, after which processing images captured by the camera can be performed by some serverless functions provided by the OpenWhisk platform. When a camera detects an interesting object such as a car or a human, the camera sends its pictures to the serverless platform for further processing. To extract features, a serverless function is called to perform feature extraction and then reports its status to the users [ 232 ].
Edge computing
Serverless cloud computing and edge computing have been used to build different kinds of applications, as presented in Table 8 . For instance, the authors of [ 217 ] have implemented an autonomous mobile robot (AMR) system based on serverless computing and edge computing. The system consists of three main components: an AMR with NVIDIA Jetson TX2 module for edge computing, a serverless architecture based on AWS, and a cross-platform mobile application developed using React Native. The main idea of the system is to deliver a package to a user. For example, the user will interact with the mobile application to send a package. Once the delivery request has been received from the user, the AWS IoT can activate related lambda functions, such as position coordinate. Then, the AMR would start its mission, sending the package to the receiver’s location. Also, facial images were regularly retrieved by AWS lambda to identify the receiver’s face. Finally, the task is completed when the receiver’s identification is confirmed [ 217 ].
Serverless computing challenges and issues (RQ7)
Studying the literature reveals a number of challenges and issues posed by employing serverless computing. These challenges cover the functional and non-functional aspects of serverless computing as follows:
Cost and pricing model
Cost is a fundamental challenge; therefore, serverless computing providers should reduce the usage of resources to the minimum, while functioning in both execution and idle states. Further, the pricing model is another challenge in serverless computing compared to other cloud computing approaches. For example, the CPU bound is cheap, whereas the input/output (I/O) bound functions may be more expensive from dedicated servers. Table 9 presents papers that investigate issues on cost and pricing models in serverless cloud computing.
Serverless computing can scale to zero while there is no request for functions and services. Scaling to zero leads to a problem called cold start. A cold start occurs when serverless functions remain idle for some time, and the next time these functions are invoked, a longer start time is required. Methods and techniques to reduce the cold start problem are crucial as a result, many papers have been studied this problem, as shown in Table 9 .
Resource limits
In serverless computing, resources are required to ensure that the platform can deal with load increasing. This includes CPU usage, memory, execution time, and bandwidth [ 94 , 202 , 210 , 235 , 280 ].
Security is the most challenging issue in serverless cloud computing. One of the security issues is isolation, because functions are running on a shared platform by many users. Therefore, strong isolation is required. Another security issue is trust when it comes to process-sensitive data. The serverless applications work with many system components, which must function correctly to maintain security properties. Table 9 presents several papers associated with serverless security.
Serverless computing must ensure function scalability and elasticity. For example, when many requests are issued to a serverless application, these requests should all be served and the used serverless cloud provider should provide the required resources to process all these requests and should scale up with the number of requests [ 210 , 280 , 281 ].
Long-running
Serverless computing runs function in a limited and short execution time, while there are some tasks might require long execution time. This does not support long execution running, since these functions are stateless, which means that if the function is paused it cannot be resumed again [ 11 , 202 , 234 , 280 ].
Programming & debugging
There is currently a lack of debugging tools. Further, monitoring tools are required, since developers need to monitor the application and observe how functions are working. More advanced integrated development environments (IDEs) are needed, so developers can perform refactoring functions, such as merging or splitting functions, and reverting functions to the previous version. Moreover, logs from serverless function invocations need to be sent to the developer and provide detailed stack traces. When an error occurs, a good method is required to report details on the occurrence to the developer. The equivalent of a stack trace for serverless computing is currently not available. Table 9 shows many papers that consider programming and debugging challenges and issues.
Vendor lock-in
The FaaS paradigm separates the code from the data, which leads the functions to depend strongly on the could provider’s ecosystem for storing, obtaining, and transferring data [ 282 ]. This issue makes the customers dependent on the serverless provider for products and services, and the customers cannot easily use different vendors in the future without substantial cost. Thus, customers have to wait on the serverless provider for additional services [ 9 , 130 , 202 ].
Performance
Serverless computing has many performance challenges and issues such as scheduling and service calling overhead. For instance, scheduling means when a serverless function is activated in response to an event this function should be mapped to a specific resource (e.g., container or VM) to be run. The resource can have a significant effect on performance based on available resources, location of input data and code, load balancing, etc. Table 9 shows papers related to serverless performance.
Fault tolerance
It refers to a system that continues working and provides its services despite the failure in some components. It mostly occurs when some containers fail. To overcome this challenge, a basic retry mechanism is used [ 11 , 210 , 235 ].
Function composition
Serverless cloud vendors provide users the ability to deploy small stateless functions to the cloud to handle a specific task. However, some complex tasks require multiple functions to work with each other collaboratively to be performed. Therefore, more research needs to be done on how function composition can be used effectively and efficiently in serverless cloud computing [ 11 , 38 , 235 ].
Resource sharing
Functions in serverless cloud computing share resources to achieve inexpensive cloud computing. Sharing resources among functions and other serverless components is a challenging task. Therefore, good techniques are required to be investigated to achieve this goal [ 98 , 210 , 283 ].
A serverless application consists of many small functions. These functions work together to accomplish the application’s functionality. Therefore, integration testing for these functions is a crucial issue to make sure that the application works properly [ 9 , 84 , 284 ].
Naming and addressing system
Users deploy functions to serverless cloud computing to solve problems. These functions cannot listen to network communications. The existing serverless cloud computing frameworks do not support this feature. Instead, they use third party services such as Amazon S3 to communicate with other functions. Therefore, finding the internet protocol (IP) address of a function by other functions and services is a challenging issue in serverless cloud computing [ 98 ].
Legacy systems
Legacy systems refer to old technologies, techniques, hardware, and software systems that are still in use. It should be possible to reach these systems from serverless cloud computing. Also, these systems might be required to be transferred to cloud computing. Therefore, more work needs to be done on the migration process and how the functions can be extracted from legacy systems to be deployed as serverless cloud functions [ 84 , 119 , 120 , 210 , 280 ].
Managing hybrid cloud
In a hybrid cloud, a developer may deploy an application to different clouds (hybrid cloud). For example, if some functions of an application are on a specific serverless cloud vendor and others are hosted on other public clouds; then, managing these functions and their interactions is a challenging issue [ 84 , 210 , 280 ].
Lack of quality of service (QoS) support
Existing serverless platforms and frameworks do not provide users the control over the QoS of serverless functions [ 235 ]. Cold starts, queuing, and orchestration are the main reasons affecting the QoS in serverless computing [ 8 ].
Architecture complexity
A serverless application may consist of several functions; the number of functions increases the complexity of the architecture. Managing these functions and services related to the application also leads to a complex architecture [ 9 ].
Interactions tracking
Stateful requests are usually used by real-life applications. It means deployed systems keep track of the state of users’ interactions and store them on the server-side for further uses. However, in stateless serverless functions, it is not obvious how these functions will handle and manage the states of each user [ 210 , 280 ].
Concurrency management
Concurrency means a function can handle any number of requests whenever a function is invoked. For example, if a request has been made to a serverless function, the function will process that request. However, if another request has been made to that function and the function is still processing the previous request, then the serverless should provide another instance of that function to serve the new request [ 210 , 280 ].
Support for heterogeneous hardware
Existing serverless platforms may not support some specialized hardware such as graphics processing unit (GPU) and field programmable gate arrays (FPGAs). This is a challenging issue for vendors to provide support for heterogeneous hardware [ 210 , 280 ].
Tools available for serverless computing (RQ8)
Nowadays, various providers strive to facilitate the adjustable use and allocation of machine resources on the cloud [ 9 ]. Likewise, plenty of supportive tools and services are aiding developers to more efficiently manage and deploy applications using serverless computing. Serverless computing is auto-scalable, reliable, and easily accessible [ 203 ]; for these reasons, big cloud providers such as Amazon, Microsoft, Google, IBM have realized the importance of offering frameworks, IDEs, software development kits (SDKs), function development kits (FDK), migrating mechanisms, logs, and monitoring tools to enhance and simplify the development, testing, deployment, and monitoring of serverless applications [ 17 ]. For instance, Amazon offers Cloud9 IDE for local deploying and testing [ 205 ].
Apart from the cloud providers’ specific tools, plenty of third-party tools exist for the developers. With the concept of these tools, developers can build and deploy applications on multi-cloud providers. Developers are also able to control platforms and resources by programming. The advantages of this are linking the applications with auto-scaling controllers and including advanced self-mechanisms into the code to automatically configure, secure, optimize, and recover the cloud applications. The core advantage of this feature is the acceleration in applying changes to the application environment [ 272 ].
There are several tools available to model serverless applications, which are based on deployment models as either imperative or declarative. The imperative model defines the execution steps to obtain a specific deployment task. While the declarative model describes the structure of a desired application deployment. However, to fully benefit from employing a serverless architecture, cloud providers should address issues that have arisen with the use of a serverless paradigm. For instance, debugging tools are unable to track and identify the exact reason behind errors [ 44 ], as most of them are limited to what cloud providers offer [ 179 ]. Although many powerful tools have been mentioned in this study and can be used in serverless computing in real scenarios, there is still a great opportunity to develop further tools and services.
Migration of monolithic applications to serverless computing (RQ9)
The nature of most existing applications is monolithic. Monolithic applications have several drawbacks; they are characterized by continuous growth in complexity and size over time.
The bigger size of the monolithic applications leads to slower startup time. Moreover, novice developers face difficulties in digesting the traditional programming paradigm. Economically, monolithic systems take more effort to be developed and debugged. Furthermore, integrating the latest technological development into monolithic systems is a tough and expensive process. Generally, monolithic applications are designed to be tightly coupled – the entire application will be unable to run or compile if one component is missing or fails [ 128 ]. It is also difficult to scale the application when multiple components have limited resources.
Another drawback is that updating any component will require redeployment of the entire project. The migration process to serverless computing involves transferring the legacy application code to serverless functions. This process could be more efficient and functional in applications with less size [ 76 ].
The key challenging aspect of migration is about extracting the serverless computing from the monolithic systems. There are several approaches to accomplish this task, one of them is Lift and shift [ 205 ]. This technique transfers the whole infrastructure to the cloud, however, this method also brings the already existing problems within the source to the destination. In [ 205 ] the authors proposed toLambda to automatically refactor, test, and deploy the monolithic applications (Java) into microservices (AWS Lambda Node.js). While rebuilding the legacy application from scratch is recommended for applications that no longer depend on the existing cloud services [ 130 ].
However, not all applications are suitable for migration to serverless computing [ 76 , 128 ]; therefore, the first important aspect to be considered before rebuilding the applications is whether it would save money [ 188 ]. For such cases, newly desired features could be implemented and added via serverless computing as an extension to the current systems [ 128 ].
The other approach is to refactor the entire legacy code into FaaS services. During the migration phase, it is crucial to address the coupling of the systems not only in the application logic but also in the databases, as more functions will call the same database. However, migrating the server-side while keeping the user interface could lead to problems. Moreover, the client cannot obtain integrated data by a single request. As the functions are decoupled into smaller entities, the server is unable to aggregate data from different entities. Thus, it is the client’s responsibility to call the necessary entities to achieve this task [ 76 ].
Future directions of research (RQ10)
As the evolution of serverless computing is relatively new, there are several research paths available to be focused on as follows:
Function startup
One of the major research opportunities is overcoming the cold start problem without affecting the primary feature of serverless which is scaling to zero [ 160 , 188 ]. The first call of functions needs initializing the required libraries, which will cause a cold start. To bypass this, the computing resources will be warm for a certain time. Hence, upcoming requests will be handled faster. This could be performed via enhancing scheduling policies and developing more accurate function performance measurements [ 86 ]. Serverless providers follow their approaches to keep the functions in the warm pool. However, most of them are based on the number of requests for a certain time. Thus, if a function is not called frequently, it will suffer again from the cold start.
Very few studies such as [ 272 ] suggested a periodic event scheduler for Kotless (a serverless framework for Kotlin) which will trigger a list of warm functions every few minutes. The authors of the study claimed that this will reduce the cold start without bringing extra costs. While in [ 233 ] argues that pre-warming methods are unnecessarily using resources with idle containers. The researchers are still working to avoid cold start by reducing high delayed function startups via optimizing compute resources [ 11 ].
Recycling and rebalancing minutes and hours of idle runtime is an expensive process for cloud providers. Therefore, reducing the cold start penalties will help cloud providers in the first place and hence customers. The authors in [ 202 ] proposed FaaStest an autonomous approach based on machine learning to capture the function call behavior and then dynamically select the optimal ones. This technique could reduce the cold start by 90%. They proposed a strategy to predict functions invoking time and warming the function using fine-grained regression method [ 285 ]. However, overcoming the issue of function startups is still considered as a research direction to be more investigated.
Keeping a guaranteed QoS level in the software level agreement (SLA) that describes the lower service level offered by the service providers [ 166 ] is a major obstacle for cloud providers to offer optimal performance metrics [ 167 , 207 ]. However, serverless frameworks should consider the objectives of both providers and users [ 242 ]; customers and developers have none or little QoS support over the functions [ 236 ]. In addition, the auto-scaling feature lacks QoS guarantees. This lack of QoS affects the performance of serverless applications. Increasing response time leads to decreasing the QoS level [ 207 ]. It also raises the cost of the service [ 236 ]. Therefore, achieving an ideal resource allocation management is a complicated and challenging task as several objectives should be fulfilled together [ 209 ]. Hence, providing more efficient QoS management of functions by the auto-scaling is essential to be considered without degrading the fault-tolerance features and increasing the cost.
Pricing is crucial for both customers and cloud providers. However, there is a shortage in pricing models, as there is an imbalance in needs between serverless providers, developers, and service end-users [ 236 ]. The pricing scheme for most cloud providers is based on the number of functions’ requests and execution time-the quantity of consumed resources [ 123 , 200 ]. Currently, FaaS is less expensive when functions are bound to I/O than CPU. Moreover, services that dynamically adjust resource consumption are unable to predict the optical computing technology. It is crucial to implement solutions that offer cost-effective computing resources. FaStest reduced the cost by 50% via learning the behavioral pattern of functions using machine learning [ 202 ]. Price estimation has a great impact on selecting the most optimal provider. Therefore there should be more researches on developing tools to predict the pricing in advance.
Since the serverless emergence, researchers are working on the open question of how to decompose legacy systems into FaaS without degrading performance [ 208 ]. Several works have been done on migrating to FaaS [ 76 , 130 , 286 ]. The currently available automated tools for migrating legacy code into FaaS are not fully practical due to the remaining manual work that needs to be done [ 17 ]. Therefore, finding optimal automatic migration solutions for existing legacy systems is an interesting research direction [ 130 ]. Moreover, research on tools for checking whether a legacy system will fit the serverless paradigm is a crucial line. Also, developing and enhancing automatic and semi-automatic analysis strategies based on artificial intelligence could be another future research field.
Debugging, testing, and benchmarking
The available tools for testing, debugging, and deployment are immature, this prevents some developers from entering the serverless environment. The shortage of tools in FaaS is a core problem, particularly the testing tools [ 17 ]. Moreover, most FaaS environments lack powerful local emulation platforms for testing. Therefore, developers are mostly depending on the server-side, which is expensive. Developers need to be ensured about the adequate testing tools before diving into the serverless world. A challenging aspect in benchmarking is the lack of information due to the heterogeneity of the cloud provider data center: hardware, software, and configurations [ 287 ]. Additionally, benchmarking FaaS platforms should take advantage of analyzing the cloud services, which lacks limited accessible measurements and hidden modification of services over time [ 55 ]. Thus, it is essential to have transparent, fair, and standardized benchmarking tools available for developers.
Threats to validity
Several threats might impact the validity of the literature mapping studies. In this paper, popular instructions and guidelines were taken into account to avoid threats to validity as follows:
Coverage of research questions: All up-to-date research aspects of serverless computing might not be included in this study. To overcome this threat, the brainstorming was conducted by all the authors in determining the most current research questions in the area.
Coverage of related papers: The process of obtaining all the related studies in serverless computing cannot be secured. In this study, various literature databases were employed; moreover, the method based on different terms and synonymous is followed by all the authors in determining the related questions.
Paper inclusion/exclusion criteria: The individual bias and interpretation could affect the implementation of the criteria. Therefore, to solve this problem, the agreements of all authors were considered in excluding or including a paper.
Accuracy of data extraction: The individual experience effects extracting the data, therefore online meetings were conducted after the data extraction process by each author. During the meetings, the outcomes from each author were compared with other findings to determine the differences and reach a final consensus.
Reproducibility of the study: Whether other researchers could obtain similar outcomes of this study is another threat. Thus, to address this, the research methodology contains the well-explained steps and actions conducted in this paper (as shown in “ Research methodology ” section).
Conclusions
The contributions of the work presented in this paper are threefold: (a) a methodical review of related literature on the topic of serverless computing, to address the issue of the lack of compiling information on the state-of-the-art of the field; (b) a comparison of the platforms and tools used in serverless computing; (c) an extensive analysis of the differences, benefits, and issues related to serverless computing, to provide a more complete understanding of the topic. Given the fast evolution and growing interest in the field, this survey focused on gathering the most outstanding trends and outcomes of serverless computing, as described by recent researchers. This survey could significantly reduce ambiguity and the entry barrier for novice developers to adapt to the serverless environment. Furthermore, the findings presented in this study could be of great value for future researchers interested in further investigating serverless computing. Finally, it is worth mentioning that the interest that both commercial and academic efforts fueled into studying, developing, and implementing serverless tools in forthcoming years could help maximize the potential that serverless computing could bring to the IT community.
Availability of data and materials
Not applicable.
Großmann M, Ioannidis C, Le DT (2019) Applicability of serverless computing in fog computing environments for iot scenarios In: Proceedings of the 12th IEEE/ACM International Conference on Utility and Cloud Computing Companion (UCC ‘19 Companion), 29–34.. Association for Computing Machinery, New York. https://doi.org/10.1145/3368235.3368834 .
Google Scholar
Boza EF, Abad CL, Villavicencio M, Quimba S, Plaza JA (2017) Reserved, on demand or serverless: Model-based simulations for cloud budget planning In: 2017 IEEE Second Ecuador Technical Chapters Meeting (ETCM), 1–6. https://doi.org/10.1109/ETCM.2017.8247460 .
Villamizar M, Garcés O, Ochoa L, Castro H, Salamanca L, Verano M, Casallas R, Gil S, Valencia C, Zambrano A, Lang M (2017) Cost comparison of running web applications in the cloud using monolithic, microservice, and aws lambda architectures. SOCA 11(2):233–247. https://doi.org/10.1007/s11761-017-0208-y .
Article Google Scholar
Jonas E, Schleier-Smith J, Sreekanti V, Tsai C-C, Khandelwal A, Pu Q, Shankar V, Carreira J, Krauth K, Yadwadkar N, Gonzalez JE, Popa RA, Stoica I, Patterson DA (2019) Cloud Programming Simplified: A Berkeley View on Serverless Computing. http://arxiv.org/abs/1902.03383. Accessed 6 Jan 2021.
Geng X, Ma Q, Pei Y, Xu Z, Zeng W, Zou J (2018) Research on early warning system of power network overloading under serverless architecture In: 2018 2nd IEEE Conference on Energy Internet and Energy System Integration (EI2), 1–6. https://doi.org/10.1109/EI2.2018.8582355 .
Kulkarni SG, Liu G, Ramakrishnan KK, Wood T (2019) Living on the edge: Serverless computing and the cost of failure resiliency In: 2019 IEEE International Symposium on Local and Metropolitan Area Networks (LANMAN), 1–6. https://doi.org/10.1109/LANMAN.2019.8846970 .
Baldini I, Castro P, Chang K, Cheng P, Fink S, Ishakian V, Mitchell N, Muthusamy V, Rabbah R, Slominski A, Suter P (2017) Serverless Computing: Current Trends and Open Problems In: Research Advances in Cloud Computing, 1–20.. Springer, Singapore. https://doi.org/10.1007/978-981-10-5026-8_1 .
Adzic G, Chatley R (2017) Serverless computing: Economic and architectural impact In: Proceedings of the 2017 11th Joint Meeting on Foundations of Software Engineering (ESEC/FSE 2017), 884–889.. Association for Computing Machinery, New York. https://doi.org/10.1145/3106237.3117767 .
Chapter Google Scholar
Jambunathan B, Yoganathan K (2018) Architecture decision on using microservices or serverless functions with containers In: 2018 International Conference on Current Trends Towards Converging Technologies (ICCTCT), 1–7. https://doi.org/10.1109/ICCTCT.2018.8551035 .
Wolski R, Krintz C, Bakir F, George G, Lin W-T (2019) Cspot: Portable, multi-scale functions-as-a-service for iot In: Proceedings of the 4th ACM/IEEE Symposium on Edge Computing (SEC ‘19), 236–249.. Association for Computing Machinery, New York. https://doi.org/10.1145/3318216.3363314 .
Yussupov V, Breitenbücher U, Leymann F, Wurster M (2019) A systematic mapping study on engineering function-as-a-service platforms and tools In: Proceedings of the 12th IEEE/ACM International Conference on Utility and Cloud Computing (UCC’19), 229–240.. Association for Computing Machinery, New York. https://doi.org/10.1145/3344341.3368803 .
Brenner S, Kapitza R (2019) Trust more, serverless In: Proceedings of the 12th ACM International Conference on Systems and Storage (SYSTOR ‘19), 33–43.. Association for Computing Machinery, New York. https://doi.org/10.1145/3319647.3325825 .
Kuhlenkamp J, Werner S (2018) Benchmarking faas platforms: Call for community participation In: 2018 IEEE/ACM International Conference on Utility and Cloud Computing Companion (UCC Companion), 189–194. https://doi.org/10.1109/UCC-Companion.2018.00055 .
Somma G, Ayimba C, Casari P, Romano SP, Mancuso V (2020) When less is more: Core-restricted container provisioning for serverless computing In: IEEE INFOCOM 2020 - IEEE Conference on Computer Communications Workshops (INFOCOM WKSHPS), 1153–1159. https://doi.org/10.1109/INFOCOMWKSHPS50562.2020.9162876 .
Sewak M, Singh S (2018) Winning in the era of serverless computing and function as a service In: 2018 3rd International Conference for Convergence in Technology (I2CT), 1–5. https://doi.org/10.1109/I2CT.2018.8529465 .
van Eyk E, Toader L, Talluri S, Versluis L, Ută̧ A, Iosup A (2018) Serverless is more: From paas to present cloud computing. IEEE Internet Comput 22(5):8–17. https://doi.org/10.1109/MIC.2018.053681358 .
Leitner P, Wittern E, Spillner J, Hummer W (2019) A mixed-method empirical study of function-as-a-service software development in industrial practice. J Syst Softw 149:340–359. https://doi.org/10.1016/j.jss.2018.12.013 .
Feng L, Kudva P, Da Silva D, Hu J (2018) Exploring serverless computing for neural network training In: 2018 IEEE 11th International Conference on Cloud Computing (CLOUD), 334–341. https://doi.org/10.1109/CLOUD.2018.00049 .
Ao L, Izhikevich L, Voelker GM, Porter G (2018) Sprocket: A serverless video processing framework In: Proceedings of the ACM Symposium on Cloud Computing (SoCC ‘18), 263–274.. Association for Computing Machinery, New York. https://doi.org/10.1145/3267809.3267815 .
Werner S, Kuhlenkamp J, Klems M, Müller J, Tai S (2018) Serverless big data processing using matrix multiplication as example In: 2018 IEEE International Conference on Big Data (Big Data), 358–365. https://doi.org/10.1109/BigData.2018.8622362 .
Al-Ali Z, Goodarzy S, Hunter E, Ha S, Han R, Keller E, Rozner E (2018) Making serverless computing more serverless In: 2018 IEEE 11th International Conference on Cloud Computing (CLOUD), 456–459. https://doi.org/10.1109/CLOUD.2018.00064 .
Pérez A, Risco S, Naranjo DM, Caballer M, Moltó G (2019) On-premises serverless computing for event-driven data processing applications In: 2019 IEEE 12th International Conference on Cloud Computing (CLOUD), 414–421. https://doi.org/10.1109/CLOUD.2019.00073 .
Glikson A, Nastic S, Dustdar S (2017) Deviceless edge computing: Extending serverless computing to the edge of the network In: Proceedings of the 10th ACM International Systems and Storage Conference (SYSTOR ‘17).. Association for Computing Machinery, New York. https://doi.org/10.1145/3078468.3078497 .
Al-Ameen M, Spillner J (2019) A systematic and open exploration of faas research In: Proceedings of the European Symposium on Serverless Computing and Applications (CEUR Workshop Proceedings ; 2330), 30–35.. CEUR-WS, Zurich. https://doi.org/10.21256/zhaw-3271 .
Alqaryouti O, Siyam N (2018) Serverless computing and scheduling tasks on cloud: A review. Am Sci Res J Eng Technol Sci (ASRJETS) 40(1):235–247.
Taibi D, El Ioini N, Pahl C, Niederkofler J (2020) Patterns for Serverless Functions (Function-as-a-Service): A Multivocal Literature Review In: Proceedings of the 10th International Conference on Cloud Computing and Services Science - Volume 1: CLOSER, 181–192. https://doi.org/10.5220/0009578501810192 .
Hellerstein JM, Faleiro J, Gonzalez JE, Schleier-Smith J, Sreekanti V, Tumanov A, Wu C (2018) Serverless Computing: One Step Forward, Two Steps Back. http://arxiv.org/abs/1812.03651. Accessed 4 Oct 2021.
Rajan AP (2020) A review on serverless architectures - function as a service (faas) in cloud computing. Telecommun Comput Electron Control 18(1):530–537. https://doi.org/10.12928/telkomnika.v18i1.12169 .
Sadaqat M, Colomo-Palacios R, Knudsen LES (2018) Serverless Computing: A Multivocal Literature Review. NOKOBIT - Norsk Konferanse for Organisasjoners Bruk Av Informasjonsteknologi 26(1):1–13.
Scheuner J, Leitner P (2020) Function-as-a-service performance evaluation: A multivocal literature review. J Syst Softw 170:110708. https://doi.org/10.1016/j.jss.2020.110708 .
Fox GC, Ishakian V, Muthusamy V, Slominski A (2017) Status of Serverless Computing and Function-as-a-Service(FaaS) in Industry and Research. arXiv e-prints:1708–08028. http://arxiv.org/abs/1708.08028. Accessed 6 Jan 2021.
Hassan HB, Barakat SA, Sarhan QI (2021) Serverless Literature Dataset. Zenodo. https://doi.org/10.5281/zenodo.4660553 .
Pedreira O, García F, Brisaboa N, Piattini M (2015) Gamification in software engineering - a systematic mapping. Inf Softw Technol 57:157–168.
Lopez-herrejon RE, Linsbauer L, Egyed A (2015) A systematic mapping study of search-based software engineering for software product lines. Inf Softw Technol 61:33–51.
Bila N, Dettori P, Kanso A, Watanabe Y, Youssef A (2017) Leveraging the serverless architecture for securing linux containers In: 2017 IEEE 37th International Conference on Distributed Computing Systems Workshops (ICDCSW), 401–404. https://doi.org/10.1109/ICDCSW.2017.66 .
Chang KS, Fink SJ (2017) Visualizing serverless cloud application logs for program understanding In: 2017 IEEE Symposium on Visual Languages and Human-Centric Computing (VL/HCC), 261–265. https://doi.org/10.1109/VLHCC.2017.8103476 .
Ishakian V, Muthusamy V, Slominski A (2018) Serving deep learning models in a serverless platform In: 2018 IEEE International Conference on Cloud Engineering (IC2E), 257–262. https://doi.org/10.1109/IC2E.2018.00052 .
Baldini I, Cheng P, Fink SJ, Mitchell N, Muthusamy V, Rabbah R, Suter P, Tardieu O (2017) The serverless trilemma: Function composition for serverless computing In: Proceedings of the 2017 ACM SIGPLAN International Symposium on New Ideas, New Paradigms, and Reflections on Programming and Software (Onward! 2017), 89–103.. Association for Computing Machinery, New York. https://doi.org/10.1145/3133850.3133855 .
Kanso A, Youssef A (2017) Serverless: Beyond the cloud In: Proceedings of the 2nd International Workshop on Serverless Computing (WoSC ‘17), 6–10.. Association for Computing Machinery, New York. https://doi.org/10.1145/3154847.3154854 .
Koller R, Williams D (2017) Will serverless end the dominance of linux in the cloud? In: Proceedings of the 16th Workshop on Hot Topics in Operating Systems (HotOS ‘17), 169–173.. Association for Computing Machinery, New York. https://doi.org/10.1145/3102980.3103008 .
Mukhi NK, Prabhu S, Slawson B (2017) Using a serverless framework for implementing a cognitive tutor: Experiences and issues In: Proceedings of the 2nd International Workshop on Serverless Computing (WoSC ‘17), 11–15.. Association for Computing Machinery, New York. https://doi.org/10.1145/3154847.3154852 .
Nadgowda S, Bila N, Isci C (2017) The less server architecture for cloud functions In: Proceedings of the 2nd International Workshop on Serverless Computing (WoSC ‘17), 22–27.. Association for Computing Machinery, New York. https://doi.org/10.1145/3154847.3154850 .
Klimovic A, Wang Y, Stuedi P, Trivedi A, Pfefferle J, Kozyrakis C (2018) Pocket: Elastic ephemeral storage for serverless analytics In: Proceedings of the 12th USENIX Conference on Operating Systems Design and Implementation (OSDI’18), 427–444.. USENIX Association, USA.
Yan M, Castro P, Cheng P, Ishakian V (2016) Building a chatbot with serverless computing In: Proceedings of the 1st International Workshop on Mashups of Things and APIs (MOTA ‘16), 1–4.. Association for Computing Machinery, New York. https://doi.org/10.1145/3007203.3007217 .
Barcelona-Pons D, García-López P, Ruiz A, Gómez-Gómez A, París G, Sánchez-Artigas M (2019) Faas orchestration of parallel workloads In: Proceedings of the 5th International Workshop on Serverless Computing (WOSC ‘19), 25–30.. Association for Computing Machinery, New York. https://doi.org/10.1145/3366623.3368137 .
Barcelona-Pons D, Sánchez-Artigas M, París G, Sutra P, García-López P (2019) On the faas track: Building stateful distributed applications with serverless architectures In: Proceedings of the 20th International Middleware Conference (Middleware ‘19), 41–54.. Association for Computing Machinery, New York. https://doi.org/10.1145/3361525.3361535 .
Kaviani N, Kalinin D, Maximilien M (2019) Towards serverless as commodity: A case of knative In: Proceedings of the 5th International Workshop on Serverless Computing (WOSC ‘19), 13–18.. Association for Computing Machinery, New York. https://doi.org/10.1145/3366623.3368135 .
Byrne A, Nadgowda S, Coskun AK (2020) Ace: Just-in-time serverless software component discovery through approximate concrete execution In: Proceedings of the 2020 Sixth International Workshop on Serverless Computing (WoSC’20), 37–42.. Association for Computing Machinery, New York. https://doi.org/10.1145/3429880.3430098 .
Sánchez-Artigas M, Eizaguirre GT, Vernik G, Stuart L, García-López P (2020) Primula: A Practical Shuffle/Sort Operator for Serverless Computing. Association for Computing Machinery, New York.
Book Google Scholar
Parás G, Garcáa-López P, Sánchez-Artigas M (2020) Serverless elastic exploration of unbalanced algorithms In: 2020 IEEE 13th International Conference on Cloud Computing (CLOUD), 149–157. https://doi.org/10.1109/CLOUD49709.2020.00033 .
López PG, Arjona A, Sampé J, Slominski A, Villard L (2020) Triggerflow: Trigger-based orchestration of serverless workflows In: Proceedings of the 14th ACM International Conference on Distributed and Event-Based Systems (DEBS ’20), 3–14.. Association for Computing Machinery, New York. https://doi.org/10.1145/3401025.3401731 .
Carver B, Zhang J, Wang A, Anwar A, Wu P, Cheng Y (2020) Wukong: A scalable and locality-enhanced framework for serverless parallel computing In: Proceedings of the 11th ACM Symposium on Cloud Computing (SoCC ’20), 1–15.. Association for Computing Machinery, New York. https://doi.org/10.1145/3419111.3421286 .
Klimovic A, Wang Y, Kozyrakis C, Stuedi P, Pfefferle J, Trivedi A (2018) Understanding ephemeral storage for serverless analytics In: Proceedings of the 2018 USENIX Conference on Usenix Annual Technical Conference (USENIX ATC ’18), 789–794.. USENIX Association, USA.
Wang A, Zhang J, Ma X, Anwar A, Rupprecht L, Skourtis D, Tarasov V, Yan F, Cheng Y (2020) Infinicache: Exploiting ephemeral serverless functions to build a cost-effective memory cache In: 18th USENIX Conference on File and Storage Technologies (FAST 20), 267–281.. USENIX Association, Santa Clara.
Kuhlenkamp J, Werner S, Borges MC, El Tal K, Tai S (2019) An evaluation of faas platforms as a foundation for serverless big data processing In: Proceedings of the 12th IEEE/ACM International Conference on Utility and Cloud Computing (UCC’19), 1–9.. Association for Computing Machinery, New York. https://doi.org/10.1145/3344341.3368796 .
Werner S, Girke R, Kuhlenkamp J (2020) An evaluation of serverless data processing frameworks In: Proceedings of the 2020 Sixth International Workshop on Serverless Computing (WoSC’20), 19–24.. Association for Computing Machinery, New York. https://doi.org/10.1145/3429880.3430095 .
Kuhlenkamp J, Werner S, Borges MC, Ernst D, Wenzel D (2020) Benchmarking elasticity of faas platforms as a foundation for objective-driven design of serverless applications In: Proceedings of the 35th Annual ACM Symposium on Applied Computing (SAC ’20), 1576–1585.. Association for Computing Machinery, New York. https://doi.org/10.1145/3341105.3373948 .
Werner S, Kuhlenkamp J, Pallas F, Anders N, Mucaj N, Tsaplina O, Schmidt C, Yildirim K (2020) Diminuendo! tactics in support of faas migrations. In: Paasivaara M Kruchten P (eds)Agile Processes in Software Engineering and Extreme Programming – Workshops, 125–132.. Springer, Cham.
Kuhlenkamp J, Werner S, Tai S (2020) The ifs and buts of less is more: A serverless computing reality check In: 2020 IEEE International Conference on Cloud Engineering (IC2E), 154–161. https://doi.org/10.1109/IC2E48712.2020.00023 .
Pfandzelter T, Bermbach D (2020) tinyfaas: A lightweight faas platform for edge environments In: 2020 IEEE International Conference on Fog Computing (ICFC), 17–24. https://doi.org/10.1109/ICFC49376.2020.00011 .
Bermbach D, Karakaya A-S, Buchholz S (2020) Using application knowledge to reduce cold starts in faas services In: Proceedings of the 35th Annual ACM Symposium on Applied Computing (SAC ’20), 134–143.. Association for Computing Machinery, New York. https://doi.org/10.1145/3341105.3373909 .
Bermbach D, Maghsudi S, Hasenburg J, Pfandzelter T (2020) Towards auction-based function placement in serverless fog platforms In: 2020 IEEE International Conference on Fog Computing (ICFC), 25–31. https://doi.org/10.1109/ICFC49376.2020.00012 .
Garcia Lopez P, Sanchez-Artigas M, Paris G, Barcelona Pons D, Ruiz Ollobarren A, Arroyo Pinto D2018. Comparison of faas orchestration systems. https://doi.org/10.1109/ucc-companion.2018.00049 .
Sampé J, Sánchez-Artigas M, García-López P, París G (2017) Data-driven serverless functions for object storage In: Proceedings of the 18th ACM/IFIP/USENIX Middleware Conference (Middleware ‘17), 121–133.. Association for Computing Machinery, New York. https://doi.org/10.1145/3135974.3135980 .
Sampé J, Vernik G, Sánchez-Artigas M, García-López P (2018) Serverless data analytics in the ibm cloud In: Proceedings of the 19th International Middleware Conference Industry (Middleware ‘18), 1–8.. Association for Computing Machinery, New York. https://doi.org/10.1145/3284028.3284029 .
Mirabelli ME, García-López P, Vernik G (2020) Bringing scaling transparency to proteomics applications with serverless computing In: Proceedings of the 2020 Sixth International Workshop on Serverless Computing (WoSC’20), 55–60.. Association for Computing Machinery, New York. https://doi.org/10.1145/3429880.3430101 .
Carreira J, Fonseca P, Tumanov A, Zhang A, Katz R (2019) Cirrus: A serverless framework for end-to-end ml workflows In: Proceedings of the ACM Symposium on Cloud Computing (SoCC ‘19), 13–24.. Association for Computing Machinery, New York. https://doi.org/10.1145/3357223.3362711 .
Sreekanti V, Wu C, Chhatrapati S, Gonzalez JE, Hellerstein JM, Faleiro JM (2020) A fault-tolerance shim for serverless computing In: Proceedings of the Fifteenth European Conference on Computer Systems (EuroSys ’20).. Association for Computing Machinery, New York. https://doi.org/10.1145/3342195.3387535 .
Ichnowski J, Lee W, Murta V, Paradis S, Alterovitz R, Gonzalez JE, Stoica I, Goldberg K (2020) Fog robotics algorithms for distributed motion planning using lambda serverless computing In: 2020 IEEE International Conference on Robotics and Automation (ICRA), 4232–4238. https://doi.org/10.1109/ICRA40945.2020.9196651 .
Zhang W, Fang V, Panda A, Shenker S (2020) Kappa: A programming framework for serverless computing In: Proceedings of the 11th ACM Symposium on Cloud Computing (SoCC ’20), 328–343.. Association for Computing Machinery, New York. https://doi.org/10.1145/3419111.3421277 .
Shankar V, Krauth K, Vodrahalli K, Pu Q, Recht B, Stoica I, Ragan-Kelley J, Jonas E, Venkataraman S (2020) Serverless linear algebra In: Proceedings of the 11th ACM Symposium on Cloud Computing (SoCC ’20), 281–295.. Association for Computing Machinery, New York. https://doi.org/10.1145/3419111.3421287 .
Gupta V, Carrano D, Yang Y, Shankar V, Courtade T, Ramchandran K (2020) Serverless straggler mitigation using error-correcting codes In: 2020 IEEE 40th International Conference on Distributed Computing Systems (ICDCS), 135–145. https://doi.org/10.1109/ICDCS47774.2020.00019 .
Wu C, Sreekanti V, Hellerstein JM (2020) Transactional causal consistency for serverless computing In: Proceedings of the 2020 ACM SIGMOD International Conference on Management of Data (SIGMOD ’20), 83–97.. Association for Computing Machinery, New York. https://doi.org/10.1145/3318464.3389710 .
Pu Q, Venkataraman S, Stoica I (2019) Shuffling, fast and slow: Scalable analytics on serverless infrastructure In: Proceedings of the 16th USENIX Conference on Networked Systems Design and Implementation (NSDI’19), 193–206.. USENIX Association, USA.
Lloyd W, Ramesh S, Chinthalapati S, Ly L, Pallickara S (2018) Serverless computing: An investigation of factors influencing microservice performance In: 2018 IEEE International Conference on Cloud Engineering (IC2E), 159–169. https://doi.org/10.1109/IC2E.2018.00039 .
Lloyd W, Vu M, Zhang B, David O, Leavesley G (2018) Improving application migration to serverless computing platforms: Latency mitigation with keep-alive workloads In: 2018 IEEE/ACM International Conference on Utility and Cloud Computing Companion (UCC Companion), 195–200. https://doi.org/10.1109/UCC-Companion.2018.00056 .
Al-Masri E, Diabate I, Jain R, Lam MHL, Nathala SR (2018) A serverless iot architecture for smart waste management systems In: 2018 IEEE International Conference on Industrial Internet (ICII), 179–180. https://doi.org/10.1109/ICII.2018.00034 .
Fotouhi M, Chen D, Lloyd WJ (2019) Function-as-a-service application service composition: Implications for a natural language processing application In: Proceedings of the 5th International Workshop on Serverless Computing (WOSC ‘19), 49–54.. Association for Computing Machinery, New York. https://doi.org/10.1145/3366623.3368141 .
Niu X, Kumanov D, Hung L-H, Lloyd W, Yeung KY (2019) Leveraging serverless computing to improve performance for sequence comparison In: Proceedings of the 10th ACM International Conference on Bioinformatics, Computational Biology and Health Informatics (BCB ‘19), 683–687.. Association for Computing Machinery, New York. https://doi.org/10.1145/3307339.3343465 .
Cordingly R, Yu H, Hoang V, Perez D, Foster D, Sadeghi Z, Hatchett R, Lloyd WJ (2020) Implications of programming language selection for serverless data processing pipelines In: 2020 IEEE Intl Conf on Dependable, Autonomic and Secure Computing, Intl Conf on Pervasive Intelligence and Computing, Intl Conf on Cloud and Big Data Computing, Intl Conf on Cyber Science and Technology Congress (DASC/PiCom/CBDCom/CyberSciTech), 704–711. https://doi.org/10.1109/DASC-PICom-CBDCom-CyberSciTech49142.2020.00120 .
Cordingly R, Shu W, Lloyd WJ (2020) Predicting performance and cost of serverless computing functions with saaf In: 2020 IEEE Intl Conf on Dependable, Autonomic and Secure Computing, Intl Conf on Pervasive Intelligence and Computing, Intl Conf on Cloud and Big Data Computing, Intl Conf on Cyber Science and Technology Congress (DASC/PiCom/CBDCom/CyberSciTech), 640–649. https://doi.org/10.1109/DASC-PICom-CBDCom-CyberSciTech49142.2020.00111 .
Cordingly R, Yu H, Hoang V, Sadeghi Z, Foster D, Perez D, Hatchett R, Lloyd W (2020) The serverless application analytics framework: Enabling design trade-off evaluation for serverless software In: Proceedings of the 2020 Sixth International Workshop on Serverless Computing (WoSC’20), 67–72.. Association for Computing Machinery, New York. https://doi.org/10.1145/3429880.3430103 .
Toader L, Uta A, Musaafir A, Iosup A (2019) Graphless: Toward serverless graph processing In: 2019 18th International Symposium on Parallel and Distributed Computing (ISPDC), 66–73. https://doi.org/10.1109/ISPDC.2019.00012 .
van Eyk E, Iosup A, Seif S, Thömmes M (2017) The spec cloud group’s research vision on faas and serverless architectures In: Proceedings of the 2nd International Workshop on Serverless Computing (WoSC ‘17), 1–4.. Association for Computing Machinery, New York. https://doi.org/10.1145/3154847.3154848 .
van Eyk E, Iosup A, Abad CL, Grohmann J, Eismann S (2018) A spec rg cloud group’s vision on the performance challenges of faas cloud architectures In: Companion of the 2018 ACM/SPEC International Conference on Performance Engineering (ICPE ‘18), 21–24.. Association for Computing Machinery, New York. https://doi.org/10.1145/3185768.3186308 .
van Eyk E, Grohmann J, Eismann S, Bauer A, Versluis L, Toader L, Schmitt N, Herbst N, Abad CL, Iosup A (2019) The spec-rg reference architecture for faas: From microservices and containers to serverless platforms. IEEE Internet Comput 23(6):7–18. https://doi.org/10.1109/MIC.2019.2952061 .
van Eyk E, Scheuner J, Eismann S, Abad CL, Iosup A (2020) Beyond microbenchmarks: The spec-rg vision for a comprehensive serverless benchmark In: Companion of the ACM/SPEC International Conference on Performance Engineering (ICPE ’20), 26–31.. Association for Computing Machinery, New York. https://doi.org/10.1145/3375555.3384381 .
Eismann S, Grohmann J, van Eyk E, Herbst N, Kounev S (2020) Predicting the costs of serverless workflows In: Proceedings of the ACM/SPEC International Conference on Performance Engineering (ICPE ’20), 265–276.. Association for Computing Machinery, New York. https://doi.org/10.1145/3358960.3379133 .
van Eyk E, Iosup A (2018) Addressing performance challenges in serverless computing In: ICT. OPEN.
Hendrickson S, Sturdevant S, Harter T, Venkataramani V, Arpaci-Dusseau AC, Arpaci-Dusseau RH (2016) Serverless computation with openlambda In: 8th USENIX Workshop on Hot Topics in Cloud Computing (HotCloud 16), 1–7.. USENIX Association, Denver.
Oakes E, Yang L, Zhou D, Houck K, Harter T, Arpaci-Dusseau AC, Arpaci-Dusseau RH (2018) Sock: Rapid task provisioning with serverless-optimized containers In: Proceedings of the 2018 USENIX Conference on Usenix Annual Technical Conference (USENIX ATC ‘18), 57–69.. USENIX Association, USA.
Oakes E, Yang L, Houck K, Harter T, Arpaci-Dusseau AC, Arpaci-Dusseau RH (2017) Pipsqueak: Lean lambdas with large libraries In: 2017 IEEE 37th International Conference on Distributed Computing Systems Workshops (ICDCSW), 395–400. https://doi.org/10.1109/ICDCSW.2017.32 .
Singhvi A, Khalid J, Akella A, Banerjee S (2020) Snf: Serverless network functions In: Proceedings of the 11th ACM Symposium on Cloud Computing (SoCC ’20), 296–310.. Association for Computing Machinery, New York. https://doi.org/10.1145/3419111.3421295 .
Wang L, Li M, Zhang Y, Ristenpart T, Swift M (2018) Peeking behind the curtains of serverless platforms In: Proceedings of the 2018 USENIX Conference on Usenix Annual Technical Conference (USENIX ATC ’18), 133–145.. USENIX Association, USA.
Abad CL, Boza EF, van Eyk E (2018) Package-aware scheduling of faas functions In: Companion of the 2018 ACM/SPEC International Conference on Performance Engineering (ICPE ‘18), 101–106.. Association for Computing Machinery, New York. https://doi.org/10.1145/3185768.3186294 .
Aumala G, Boza E, Ortiz-Avilés L, Totoy G, Abad C (2019) Beyond load balancing: Package-aware scheduling for serverless platforms In: 2019 19th IEEE/ACM International Symposium on Cluster, Cloud and Grid Computing (CCGRID), 282–291. https://doi.org/10.1109/CCGRID.2019.00042 .
Alpernas K, Flanagan C, Fouladi S, Ryzhyk L, Sagiv M, Schmitz T, Winstein K (2018) Secure serverless computing using dynamic information flow control. Proc ACM Program Lang 2(OOPSLA):1–26. https://doi.org/10.1145/3276488 .
Kaffes K, Yadwadkar NJ, Kozyrakis C (2019) Centralized core-granular scheduling for serverless functions In: Proceedings of the ACM Symposium on Cloud Computing (SoCC ‘19), 158–164.. Association for Computing Machinery, New York. https://doi.org/10.1145/3357223.3362709 .
Choi S, Shahbaz M, Prabhakar B, Rosenblum M (2019) λ -nic: Interactive serverless compute on smartnics In: Proceedings of the ACM SIGCOMM 2019 Conference Posters and Demos (SIGCOMM Posters and Demos ’19), 151–152.. Association for Computing Machinery, New York. https://doi.org/10.1145/3342280.3342341 .
Manner J, Endreß M, Heckel T, Wirtz G (2018) Cold start influencing factors in function as a service In: 2018 IEEE/ACM International Conference on Utility and Cloud Computing Companion (UCC Companion), 181–188. https://doi.org/10.1109/UCC-Companion.2018.00054 .
Manner J, Kolb S, Wirtz G (2019) Troubleshooting serverless functions: a combined monitoring and debugging approach. SICS Softw-Intensiv Cyber-Physical Syst 34(2):99–104. https://doi.org/10.1007/s00450-019-00398-6 .
Winzinger S, Wirtz G (2019) Model-based analysis of serverless applications In: 2019 IEEE/ACM 11th International Workshop on Modelling in Software Engineering (MiSE), 82–88. https://doi.org/10.1109/MiSE.2019.00020 .
Winzinger S, Wirtz G (2020) Applicability of coverage criteria for serverless applications In: 2020 IEEE International Conference on Service Oriented Systems Engineering (SOSE), 49–56. https://doi.org/10.1109/SOSE49046.2020.00013 .
Prechtl M, Lichtenthäler R, Wirtz G (2020) Investigating possibilites for protecting and hardening installable faas platforms. In: Dustdar S (ed)Service-Oriented Computing, 107–126.. Springer, Cham.
Gias AU, Casale G (2020) Cocoa: Cold start aware capacity planning for function-as-a-service platforms In: 2020 28th International Symposium on Modeling, Analysis, and Simulation of Computer and Telecommunication Systems (MASCOTS), 1–8. https://doi.org/10.1109/MASCOTS50786.2020.9285966 .
Chatley R, Allerton T (2020) Nimbus: Improving the developer experience for serverless applications In: 2020 IEEE/ACM 42nd International Conference on Software Engineering: Companion Proceedings (ICSE-Companion), 85–88.
Casale G, Artač M, van den Heuvel W-J, van Hoorn A, Jakovits P, Leymann F, Long M, Papanikolaou V, Presenza D, Russo A, Srirama SN, Tamburri DA, Wurster M, Zhu L (2020) Radon: rational decomposition and orchestration for serverless computing. SICS Softw-Intens Cyber-Phys Syst 35(1):77–87. https://doi.org/10.1007/s00450-019-00413-w .
Vandebon J, Coutinho JGF, Luk W, Nurvitadhi E, Naik M (2020) Slate: Managing heterogeneous cloud functions In: 2020 IEEE 31st International Conference on Application-specific Systems, Architectures and Processors (ASAP), 141–148. https://doi.org/10.1109/ASAP49362.2020.00032 .
Kim J, Lee K (2019) Functionbench: A suite of workloads for serverless cloud function service In: 2019 IEEE 12th International Conference on Cloud Computing (CLOUD), 502–504. https://doi.org/10.1109/CLOUD.2019.00091 .
Kim J, Park J, Lee K (2019) Network resource isolation in serverless cloud function service In: 2019 IEEE 4th International Workshops on Foundations and Applications of Self* Systems (FAS*W), 182–187. https://doi.org/10.1109/FAS-W.2019.00051 .
Park J, Kim H, Lee K (2020) Evaluating concurrent executions of multiple function-as-a-service runtimes with microvm In: 2020 IEEE 13th International Conference on Cloud Computing (CLOUD), 532–536. https://doi.org/10.1109/CLOUD49709.2020.00080 .
Choi J, Lee K (2020) Evaluation of network file system as a shared data storage in serverless computing In: Proceedings of the 2020 Sixth International Workshop on Serverless Computing (WoSC’20), 25–30.. Association for Computing Machinery, New York. https://doi.org/10.1145/3429880.3430096 .
Kim J, Lee K (2020) I/o resource isolation of public cloud serverless function runtimes for data-intensive applications. Clust Comput 23(3):2249–2259. https://doi.org/10.1007/s10586-020-03103-4 .
Wu M, Mi Z, Xia Y (2020) A survey on serverless computing and its implications for jointcloud computing In: 2020 IEEE International Conference on Joint Cloud Computing, 94–101. https://doi.org/10.1109/JCC49151.2020.00023 .
Li Z, Chen Q, Xue S, Ma T, Yang Y, Song Z, Guo M (2020) Amoeba: Qos-awareness and reduced resource usage of microservices with serverless computing In: 2020 IEEE International Parallel and Distributed Processing Symposium (IPDPS), 399–408. https://doi.org/10.1109/IPDPS47924.2020.00049 .
Du D, Yu T, Xia Y, Zang B, Yan G, Qin C, Wu Q, Chen H (2020) Catalyzer: Sub-millisecond startup for serverless computing with initialization-less booting In: Proceedings of the Twenty-Fifth International Conference on Architectural Support for Programming Languages and Operating Systems (ASPLOS ’20), 467–481.. Association for Computing Machinery, New York. https://doi.org/10.1145/3373376.3378512 .
Yu T, Liu Q, Du D, Xia Y, Zang B, Lu Z, Yang P, Qin C, Chen H (2020) Characterizing serverless platforms with serverlessbench In: Proceedings of the 11th ACM Symposium on Cloud Computing (SoCC ’20), 30–44.. Association for Computing Machinery, New York. https://doi.org/10.1145/3419111.3421280 .
Liu J, Mi Z, Huang Z, Hua Z, Xia Y (2020) Hcloud: A serverless platform for jointcloud computing In: 2020 IEEE International Conference on Joint Cloud Computing, 86–93. https://doi.org/10.1109/JCC49151.2020.00022 .
Pérez A, Moltó G, Caballer M, Calatrava A (2019) A programming model and middleware for high throughput serverless computing applications In: Proceedings of the 34th ACM/SIGAPP Symposium on Applied Computing (SAC ‘19), 106–113.. Association for Computing Machinery, New York. https://doi.org/10.1145/3297280.3297292 .
Pérez A, Moltó G, Caballer M, Calatrava A (2018) Serverless computing for container-based architectures. Future Generation Computer Systems 83:50–59. https://doi.org/10.1016/j.future.2018.01.022 .
Giménez-Alventosa V, Moltó G, Caballer M (2019) A framework and a performance assessment for serverless mapreduce on aws lambda. Future Generation Computer Systems 97:259–274. https://doi.org/10.1016/j.future.2019.02.057 .
Naranjo DM, Risco S, de Alfonso C, Pérez A, Blanquer I, Moltó G (2020) Accelerated serverless computing based on gpu virtualization. J Parallel Distrib Comput 139:32–42. https://doi.org/10.1016/j.jpdc.2020.01.004 .
Wang H, Niu D, Li B (2019) Distributed machine learning with a serverless architecture In: IEEE INFOCOM 2019 - IEEE Conference on Computer Communications, 1288–1296. https://doi.org/10.1109/INFOCOM.2019.8737391 .
Ghaemi S, Khazaei H, Musilek P (2020) Chainfaas: An open blockchain-based serverless platform. IEEE Access 8:131760–131778. https://doi.org/10.1109/ACCESS.2020.3010119 .
Goli A, Hajihassani O, Khazaei H, Ardakanian O, Rashidi M, Dauphinee T (2020) Migrating from monolithic to serverless: A fintech case study In: Companion of the ACM/SPEC International Conference on Performance Engineering (ICPE ’20), 20–25.. Association for Computing Machinery, New York. https://doi.org/10.1145/3375555.3384380 .
Mahmoudi N, Khazaei H (2020) Performance modeling of serverless computing platforms. IEEE Trans Cloud Comput:1–1. https://doi.org/10.1109/TCC.2020.3033373 .
Mahmoudi N, Khazaei H (2020) Temporal performance modelling of serverless computing platforms In: Proceedings of the 2020 Sixth International Workshop on Serverless Computing (WoSC’20), 1–6.. Association for Computing Machinery, New York. https://doi.org/10.1145/3429880.3430092 .
Wurster M, Breitenbücher U, Képes K, Leymann F, Yussupov V (2018) Modeling and automated deployment of serverless applications using tosca In: 2018 IEEE 11th Conference on Service-Oriented Computing and Applications (SOCA), 73–80. https://doi.org/10.1109/SOCA.2018.00017 .
Yussupov V, Breitenbücher U, Hahn M, Leymann F (2019) Serverless parachutes: Preparing chosen functionalities for exceptional workloads In: 2019 IEEE 23rd International Enterprise Distributed Object Computing Conference (EDOC), 226–235. https://doi.org/10.1109/EDOC.2019.00035 .
Yussupov V, Breitenbücher U, Leymann F, Müller C (2019) Facing the unplanned migration of serverless applications: A study on portability problems, solutions, and dead ends In: Proceedings of the 12th IEEE/ACM International Conference on Utility and Cloud Computing (UCC’19), 273–283.. Association for Computing Machinery, New York. https://doi.org/10.1145/3344341.3368813 .
Spillner J (2019) Serverless computing and cloud function-based applications In: Proceedings of the 12th IEEE/ACM International Conference on Utility and Cloud Computing Companion (UCC ‘19 Companion), 177–178.. Association for Computing Machinery, New York. https://doi.org/10.1145/3368235.3370269 .
Murphy S, Persaud L, Martini W, Bosshard B (2020) On the use of web assembly in a serverless context. In: Paasivaara M Kruchten P (eds)Agile Processes in Software Engineering and Extreme Programming – Workshops, 141–145.. Springer, Cham.
Spillner J (2020) Resource management for cloud functions with memory tracing, profiling and autotuning In: Proceedings of the 2020 Sixth International Workshop on Serverless Computing (WoSC’20), 13–18.. Association for Computing Machinery, New York. https://doi.org/10.1145/3429880.3430094 .
Spillner J, Mateos C, Monge DA (2018) Faaster, better, cheaper: The prospect of serverless scientific computing and hpc. In: Mocskos E Nesmachnow S (eds)High Performance Computing, 154–168.. Springer, Cham.
Alder F, Asokan N, Kurnikov A, Paverd A, Steiner M (2019) S-faas: Trustworthy and accountable function-as-a-service using intel sgx In: Proceedings of the 2019 ACM SIGSAC Conference on Cloud Computing Security Workshop (CCSW’19), 185–199.. Association for Computing Machinery, New York. https://doi.org/10.1145/3338466.3358916 .
Kuriata A, Illikkal RG (2020) Predictable performance for qos-sensitive, scalable, multi-tenant function-as-a-service deployments. In: Paasivaara M Kruchten P (eds)Agile Processes in Software Engineering and Extreme Programming – Workshops, 133–140.. Springer, Cham.
Mohan A, Sane H, Doshi K, Edupuganti S, Nayak N, Sukhomlinov V (2019) Agile cold starts for scalable serverless In: Proceedings of the 11th USENIX Conference on Hot Topics in Cloud Computing (HotCloud’19), 21.. USENIX Association, USA.
Shahrad M, Fonseca R, Goiri I, Chaudhry G, Batum P, Cooke J, Laureano E, Tresness C, Russinovich M, Bianchini R (2020) Serverless in the wild: Characterizing and optimizing the serverless workload at a large cloud provider In: 2020 USENIX Annual Technical Conference (USENIX ATC 20), 205–218.
Obetz M, Das A, Castiglia T, Patterson S, Milanova A (2020) Formalizing event-driven behavior of serverless applications. In: Brogi A, Zimmermann W, Kritikos K (eds)Service-Oriented and Cloud Computing, 19–29.. Springer, Cham.
Das A, Imai S, Patterson S, Wittie MP (2020) Performance optimization for edge-cloud serverless platforms via dynamic task placement In: 2020 20th IEEE/ACM International Symposium on Cluster, Cloud and Internet Computing (CCGRID), 41–50. https://doi.org/10.1109/CCGrid49817.2020.00-89 .
Das A, Leaf A, Varela CA, Patterson S (2020) Skedulix: Hybrid cloud scheduling for cost-efficient execution of serverless applications In: 2020 IEEE 13th International Conference on Cloud Computing (CLOUD), 609–618. https://doi.org/10.1109/CLOUD49709.2020.00090 .
Obetz M, Patterson S, Milanova A (2019) Static call graph construction in aws lambda serverless applications In: Proceedings of the 11th USENIX Conference on Hot Topics in Cloud Computing (HotCloud’19), 20.. USENIX Association, USA.
Lin W, Krintz C, Wolski R, Zhang M, Cai X, Li T, Xu W (2018) Tracking causal order in aws lambda applications In: 2018 IEEE International Conference on Cloud Engineering (IC2E), 50–60. https://doi.org/10.1109/IC2E.2018.00027 .
George G, Bakir F, Wolski R, Krintz C (2020) Nanolambda: Implementing functions as a service at all resource scales for the internet of things In: 2020 IEEE/ACM Symposium on Edge Computing (SEC), 220–231. https://doi.org/10.1109/SEC50012.2020.00035 .
Zhang M, Krintz C, Wolski R (2020) Stoic: Serverless teleoperable hybrid cloud for machine learning applications on edge device In: 2020 IEEE International Conference on Pervasive Computing and Communications Workshops (PerCom Workshops), 1–6. https://doi.org/10.1109/PerComWorkshops48775.2020.9156239 .
Datta P, Kumar P, Morris T, Grace M, Rahmati A, Bates A (2020) Valve: Securing function workflows on serverless computing platforms In: Proceedings of The Web Conference 2020 (WWW ’20), 939–950.. Association for Computing Machinery, New York. https://doi.org/10.1145/3366423.3380173 .
Sankaran A, Datta P, Bates A (2020) Workflow integration alleviates identity and access management in serverless computing In: Annual Computer Security Applications Conference (ACSAC ’20), 496–509.. Association for Computing Machinery, New York. https://doi.org/10.1145/3427228.3427665 .
Elgamal T (2018) Costless: Optimizing cost of serverless computing through function fusion and placement In: 2018 IEEE/ACM Symposium on Edge Computing (SEC), 300–312. https://doi.org/10.1109/SEC.2018.00029 .
Dehury CK, Srirama SN, Chhetri TR (2020) Ccodamic: A framework for coherent coordination of data migration and computation platforms. Futur Gener Comput Syst 109:1–16. https://doi.org/10.1016/j.future.2020.03.029 .
Dehury C, Jakovits P, Srirama SN, Tountopoulos V, Giotis G (2020) Data pipeline architecture for serverless platform. In: Muccini H, Avgeriou P, Buhnova B, Camara J, Caporuscio M, Franzago M, Koziolek A, Scandurra P, Trubiani C, Weyns D, Zdun U (eds)Software Architecture, 241–246.. Springer, Cham.
Sarkar S, Wankar R, Srirama SN, Suryadevara NK (2020) Serverless management of sensing systems for fog computing framework. IEEE Sensors J 20(3):1564–72. https://doi.org/10.1109/JSEN.2019.2939182 .
Malawski M, Gajek A, Zima A, Balis B, Figiela K (2017) Serverless execution of scientific workflows: Experiments with hyperflow, aws lambda and google cloud functions. Futur Gener Comput Syst:1–13. https://doi.org/10.1016/j.future.2017.10.029 .
Moczurad P, Malawski M (2018) Visual-textual framework for serverless computation: A luna language approach In: 2018 IEEE/ACM International Conference on Utility and Cloud Computing Companion (UCC Companion), 169–74. https://doi.org/10.1109/UCC-Companion.2018.00052 .
Pawlik M, Banach P, Malawski M (2020) Adaptation of workflow application scheduling algorithm to serverless infrastructure. In: Schwardmann U, Boehme C, B. Heras D, Cardellini V, Jeannot E, Salis A, Schifanella C, Manumachu RR, Schwamborn D, Ricci L, Sangyoon O, Gruber T, Antonelli L, Scott SL (eds)Euro-Par 2019: Parallel Processing Workshops, 345–356.. Springer, Cham.
Akhtar N, Raza A, Ishakian V, Matta I (2020) Cose: Configuring serverless functions using statistical learning In: IEEE INFOCOM 2020 - IEEE Conference on Computer Communications, 129–38. https://doi.org/10.1109/INFOCOM41043.2020.9155363 .
Cadden J, Unger T, Awad Y, Dong H, Krieger O, Appavoo J (2020) Seuss: Skip redundant paths to make serverless fast In: Proceedings of the Fifteenth European Conference on Computer Systems (EuroSys ’20).. Association for Computing Machinery, New York. https://doi.org/10.1145/3342195.3392698 .
Balla D, Maliosz M, Simon C (2020) Open source faas performance aspects In: 2020 43rd International Conference on Telecommunications and Signal Processing (TSP), 358–364. https://doi.org/10.1109/TSP49548.2020.9163456 .
Pelle I, Czentye J, Dóka J, Kern A, Gerő BP, Sonkoly B (2020) Operating latency sensitive applications on public serverless edge cloud platforms. IEEE Internet Things J:1–1. https://doi.org/10.1109/JIOT.2020.3042428 .
Balla D, Maliosz M, Simon C, Gehberger D (2020) Tuning runtimes in open source faas. In: Hsu C-H, Kallel S, Lan K-C, Zheng Z (eds)Internet of Vehicles. Technologies and Services Toward Smart Cities, 250–266.. Springer, Cham.
Carver B, Zhang J, Wang A, Cheng Y (2019) In search of a fast and efficient serverless dag engine In: 2019 IEEE/ACM Fourth International Parallel Data Systems Workshop (PDSW), 1–10. https://doi.org/10.1109/PDSW49588.2019.00005 .
Gadepalli PK, McBride S, Peach G, Cherkasova L, Parmer G (2020) Sledge: A Serverless-First, Light-Weight Wasm Runtime for the Edge. Association for Computing Machinery, New York.
Gadepalli PK, Peach G, Cherkasova L, Aitken R, Parmer G (2019) Challenges and opportunities for efficient serverless computing at the edge In: 2019 38th Symposium on Reliable Distributed Systems (SRDS), 261–2615. https://doi.org/10.1109/SRDS47363.2019.00036 .
Somu N, Daw N, Bellur U, Kulkarni P (2020) Panopticon: A comprehensive benchmarking tool for serverless applications In: 2020 International Conference on COMmunication Systems NETworkS (COMSNETS), 144–151. https://doi.org/10.1109/COMSNETS48256.2020.9027346 .
Bajaj D, Bharti U, Goel A, Gupta SC (2020) Partial migration for re-architecting a cloud native monolithic application into microservices and faas. In: Badica C, Liatsis P, Kharb L, Chahal D (eds)Information, Communication and Computing Technology, 111–124.. Springer, Singapore.
Daw N, Bellur U, Kulkarni P (2020) Xanadu: Mitigating Cascading Cold Starts in Serverless Function Chain Deployments. Association for Computing Machinery, New York.
HoseinyFarahabady M, Lee YC, Zomaya AY, Tari Z (2017) A qos-aware resource allocation controller for function as a service (faas) platform. In: Maximilien M, Vallecillo A, Wang J, Oriol M (eds)Service-Oriented Computing, 241–255.. Springer, Cham. https://doi.org/10.1007/978-3-319-69035-3_17 .
Kim YK, HoseinyFarahabady MR, Lee YC, Zomaya AY, Jurdak R (2018) Dynamic control of cpu usage in a lambda platform In: 2018 IEEE International Conference on Cluster Computing (CLUSTER), 234–244. https://doi.org/10.1109/CLUSTER.2018.00041 .
Kim YK, HoseinyFarahabady MR, Lee YC, Zomaya AY (2020) Automated fine-grained cpu cap control in serverless computing platform. IEEE Transactions on Parallel and Distributed Systems 31(10):2289–2301. https://doi.org/10.1109/TPDS.2020.2989771 .
Suresh A, Somashekar G, Varadarajan A, Kakarla VR, Upadhyay H, Gandhi A (2020) Ensure: Efficient scheduling and autonomous resource management in serverless environments In: 2020 IEEE International Conference on Autonomic Computing and Self-Organizing Systems (ACSOS), 1–10. https://doi.org/10.1109/ACSOS49614.2020.00020 .
Suresh A, Gandhi A (2019) Fnsched: An efficient scheduler for serverless functions In: Proceedings of the 5th International Workshop on Serverless Computing (WOSC ’19), 19–24.. Association for Computing Machinery, New York. https://doi.org/10.1145/3366623.3368136 .
Hunhoff E, Irshad S, Thurimella V, Tariq A, Rozner E (2020) Proactive serverless function resource management In: Proceedings of the 2020 Sixth International Workshop on Serverless Computing (WoSC’20), 61–66.. Association for Computing Machinery, New York. https://doi.org/10.1145/3429880.3430102 .
Tariq A, Pahl A, Nimmagadda S, Rozner E, Lanka S (2020) Sequoia: Enabling quality-of-service in serverless computing In: Proceedings of the 11th ACM Symposium on Cloud Computing (SoCC ’20), 311–327.. Association for Computing Machinery, New York. https://doi.org/10.1145/3419111.3421306 .
Crane M, Lin J (2017) An exploration of serverless architectures for information retrieval In: Proceedings of the ACM SIGIR International Conference on Theory of Information Retrieval (ICTIR ‘17), 241–244.. Association for Computing Machinery, New York. https://doi.org/10.1145/3121050.3121086 .
Kim Y, Lin J (2018) Serverless data analytics with flint In: 2018 IEEE 11th International Conference on Cloud Computing (CLOUD), 451–455. https://doi.org/10.1109/CLOUD.2018.00063 .
Król M, Psaras I (2017) Nfaas: Named function as a service In: Proceedings of the 4th ACM Conference on Information-Centric Networking (ICN ‘17), 134–144.. Association for Computing Machinery, New York. https://doi.org/10.1145/3125719.3125727 .
Parres-Peredo A, Piza-Davila I, Cervantes F (2019) Building and evaluating user network profiles for cybersecurity using serverless architecture In: 2019 42nd International Conference on Telecommunications and Signal Processing (TSP), 164–167. https://doi.org/10.1109/TSP.2019.8768825 .
Ivanov V, Smolander K (2018) Implementation of a devops pipeline for serverless applications. In: Kuhrmann M, Schneider K, Pfahl D, Amasaki S, Ciolkowski M, Hebig R, Tell P, Klünder J, Küpper S (eds)Product-Focused Software Process Improvement, 48–64.. Springer, Cham. https://doi.org/10.1007/978-3-030-03673-7_4 .
Chen H, Zhang L-J (2018) Fbaas: Functional blockchain as a service. In: Chen S, Wang H, Zhang L-J (eds)Blockchain – ICBC 2018, 243–250.. Springer, Cham. https://doi.org/10.1007/978-3-319-94478-4_17 .
Lehvä J, Mäkitalo N, Mikkonen T (2018) Case study: Building a serverless messenger chatbot. In: Garrigós I Wimmer M (eds)Current Trends in Web Engineering, 75–86.. Springer, Cham. https://doi.org/10.1007/978-3-319-74433-9_6 .
Poth A, Schubert N, Riel A (2020) Sustainability efficiency challenges of modern it architectures – a quality model for serverless energy footprint. In: Yilmaz M, Niemann J, Clarke P, Messnarz R (eds)Systems, Software and Services Process Improvement, 289–301.. Springer, Cham.
Deese A (2018) Implementation of unsupervised k-means clustering algorithm within amazon web services lambda In: 2018 18th IEEE/ACM International Symposium on Cluster, Cloud and Grid Computing (CCGRID), 626–632. https://doi.org/10.1109/CCGRID.2018.00093 .
Jangda A, Pinckney D, Brun Y, Guha A (2019) Formal foundations of serverless computing. Proc ACM Program Lang 3(OOPSLA):1–26. https://doi.org/10.1145/3360575 .
Back T, Andrikopoulos V (2018) Using a microbenchmark to compare function as a service solutions. In: Kritikos K, Plebani P, de Paoli F (eds)Service-Oriented and Cloud Computing, 146–160.. Springer, Cham. https://doi.org/10.1007/978-3-319-99819-0\_11 .
Kritikos K, Skrzypek P (2018) A review of serverless frameworks In: 2018 IEEE/ACM International Conference on Utility and Cloud Computing Companion (UCC Companion), 161–168. https://doi.org/10.1109/UCC-Companion.2018.00051 .
Kritikos K, Skrzypek P (2019) Simulation-as-a-service with serverless computing In: 2019 IEEE World Congress on Services (SERVICES), vol. 2642-939X, 200–205. https://doi.org/10.1109/SERVICES.2019.00056 .
Ast M, Gaedke M (2017) Self-contained web components through serverless computing In: Proceedings of the 2nd International Workshop on Serverless Computing (WoSC ‘17), 28–33.. Association for Computing Machinery, New York. https://doi.org/10.1145/3154847.3154849 .
Trach B, Oleksenko O, Gregor F, Bhatotia P, Fetzer C (2019) Clemmys: Towards secure remote execution in faas In: Proceedings of the 12th ACM International Conference on Systems and Storage (SYSTOR ‘19), 44–54.. Association for Computing Machinery, New York. https://doi.org/10.1145/3319647.3325835 .
Aditya P, Akkus IE, Beck A, Chen R, Hilt V, Rimac I, Satzke K, Stein M (2019) Will serverless computing revolutionize nfv?Proc IEEE 107(4):667–678. https://doi.org/10.1109/JPROC.2019.2898101 .
Baresi L, Filgueira Mendonça D, Garriga M (2017) Empowering low-latency applications through a serverless edge computing architecture. In: De Paoli F, Schulte S, Broch Johnsen E (eds)Service-Oriented and Cloud Computing, 196–210.. Springer, Cham.
Tang Y, Yang J (2020) Lambdata: Optimizing serverless computing by making data intents explicit In: 2020 IEEE 13th International Conference on Cloud Computing (CLOUD), 294–303. https://doi.org/10.1109/CLOUD49709.2020.00049 .
Ali A, Pinciroli R, Yan F, Smirni E (2020) Batch: Machine learning inference serving on serverless platforms with adaptive batching In: Proceedings of the International Conference for High Performance Computing, Networking, Storage and Analysis (SC ’20), 1–15.
Christidis A, Moschoyiannis S, Hsu C-H, Davies R (2020) Enabling serverless deployment of large-scale ai workloads. IEEE Access 8:70150–70161. https://doi.org/10.1109/ACCESS.2020.2985282 .
Aske A, Zhao X (2018) Supporting multi-provider serverless computing on the edge In: Proceedings of the 47th International Conference on Parallel Processing Companion (ICPP ‘18).. Association for Computing Machinery, New York. https://doi.org/10.1145/3229710.3229742 .
Gunasekaran JR, Thinakaran P, Nachiappan NC, Srivatsa Kannan R, Kandemir MT, Das CR (2020) Characterizing bottlenecks in scheduling microservices on serverless platforms In: 2020 IEEE 40th International Conference on Distributed Computing Systems (ICDCS), 1197–1198. https://doi.org/10.1109/ICDCS47774.2020.00195 .
Dziurzanski P, Swan J, Indrusiak LS (2018) Value-based manufacturing optimisation in serverless clouds for industry 4.0 In: Proceedings of the Genetic and Evolutionary Computation Conference (GECCO ‘18), 1222–1229.. Association for Computing Machinery, New York. https://doi.org/10.1145/3205455.3205501 .
Mujezinović A, Ljubović V (2019) Serverless architecture for workflow scheduling with unconstrained execution environment In: 2019 42nd International Convention on Information and Communication Technology, Electronics and Microelectronics (MIPRO), 242–246. https://doi.org/10.23919/MIPRO.2019.8756833 .
Tricomi G, Giosa D, Merlino G, Romeo O, Longo F (2020) Toward a function-as-a-service framework for genomic analysis In: 2020 IEEE International Conference on Smart Computing (SMARTCOMP), 314–319. https://doi.org/10.1109/SMARTCOMP50058.2020.00070 .
Fingler H, Akshintala A, Rossbach CJ (2019) Usetl: Unikernels for serverless extract transform and load why should you settle for less? In: Proceedings of the 10th ACM SIGOPS Asia-Pacific Workshop on Systems (APSys ’19), 23–30.. Association for Computing Machinery, New York. https://doi.org/10.1145/3343737.3343750 .
Soltani B, Ghenai A, Zeghib N (2018) Towards distributed containerized serverless architecture in multi cloud environment. Proc Comput Sci 134:121–128. https://doi.org/10.1016/j.procs.2018.07.152. The 15th International Conference on Mobile Systems and Pervasive Computing (MobiSPC 2018) / The 13th International Conference on Future Networks and Communications (FNC-2018) / Affiliated Workshops.
Crespo-Cepeda R, Agapito G, Vazquez-Poletti JL, Cannataro M (2019) Challenges and opportunities of amazon serverless lambda services in bioinformatics In: Proceedings of the 10th ACM International Conference on Bioinformatics, Computational Biology and Health Informatics (BCB ‘19), 663–668.. Association for Computing Machinery, New York. https://doi.org/10.1145/3307339.3343462 .
Dash S, Dash DK (2016) Serverless cloud computing framework for smart grid architecture In: 2016 IEEE 7th Power India International Conference (PIICON), 1–6. https://doi.org/10.1109/POWERI.2016.8077240 .
Horovitz S, Amos R, Baruch O, Cohen T, Oyar T, Deri A (2019) Faastest - machine learning based cost and performance faas optimization. In: Coppola M, Carlini E, D’Agostino D, Altmann J, Bañares JÁ (eds)Economics of Grids, Clouds, Systems, and Services, 171–186.. Springer, Cham. https://doi.org/10.1007/978-3-030-13342-9\_15 .
Bardsley D, Ryan L, Howard J (2018) Serverless performance and optimization strategies In: 2018 IEEE International Conference on Smart Cloud (SmartCloud), 19–26. https://doi.org/10.1109/SmartCloud.2018.00012 .
Jackson D, Clynch G (2018) An investigation of the impact of language runtime on the performance and cost of serverless functions In: 2018 IEEE/ACM International Conference on Utility and Cloud Computing Companion (UCC Companion), 154–160. https://doi.org/10.1109/UCC-Companion.2018.00050 .
Kaplunovich A (2019) Tolambda–automatic path to serverless architectures In: 2019 IEEE/ACM 3rd International Workshop on Refactoring (IWoR), 1–8. https://doi.org/10.1109/IWoR.2019.00008 .
Lee H, Satyam K, Fox G (2018) Evaluation of production serverless computing environments In: 2018 IEEE 11th International Conference on Cloud Computing (CLOUD), 442–450. https://doi.org/10.1109/CLOUD.2018.00062 .
Asghar T, Rasool S, Iqbal MU, Qayyum Z, Mian AN, Ubakanma G (2018) Feasibility of serverless cloud services for disaster management information systems In: 2018 IEEE 20th International Conference on High Performance Computing and Communications; IEEE 16th International Conference on Smart City; IEEE 4th International Conference on Data Science and Systems (HPCC/SmartCity/DSS), 1054–1057. https://doi.org/10.1109/HPCC/SmartCity/DSS.2018.00175 .
McGrath G, Brenner PR (2017) Serverless computing: Design, implementation, and performance In: 2017 IEEE 37th International Conference on Distributed Computing Systems Workshops (ICDCSW), 405–410. https://doi.org/10.1109/ICDCSW.2017.36 .
Christoforou A, Andreou AS (2018) An effective resource management approach in a faas environment In: ESSCA@UCC, 2–8.
Rajan RAP (2018) Serverless architecture - a revolution in cloud computing In: 2018 Tenth International Conference on Advanced Computing (ICoAC), 88–93. https://doi.org/10.1109/ICoAC44903.2018.8939081 .
Rahman MM, Hasibul Hasan M (2019) Serverless architecture for big data analytics In: 2019 Global Conference for Advancement in Technology (GCAT), 1–5. https://doi.org/10.1109/GCAT47503.2019.8978443 .
Huber F, Körber N, Mock M (2019) Selena: A serverless energy management system In: Proceedings of the 5th International Workshop on Serverless Computing (WOSC ‘19), 7–12.. Association for Computing Machinery, New York. https://doi.org/10.1145/3366623.3368134 .
Aytekin A, Johansson M (2019) Exploiting serverless runtimes for large-scale optimization In: 2019 IEEE 12th International Conference on Cloud Computing (CLOUD), 499–501. https://doi.org/10.1109/CLOUD.2019.00090 .
Gabbrielli M, Giallorenzo S, Lanese I, Montesi F, Peressotti M, Zingaro SP (2019) No more, no less: A formal model for serverless computing. In: Riis Nielson H Tuosto E (eds)Coordination Models and Languages, 148–157.. Springer, Cham. https://doi.org/10.1007/978-3-030-22397-7_9 .
Fasogbon P, You Y, Aksu E (2020) 3d human model creation on a serverless environment In: 2020 IEEE International Symposium on Mixed and Augmented Reality Adjunct (ISMAR-Adjunct), 118–122. https://doi.org/10.1109/ISMAR-Adjunct51615.2020.00044 .
Dash S, Sodhi R, Sodhi B (2020) A serverless cloud computing framework for real-time appliance-usage recommendation In: 2020 21st National Power Systems Conference (NPSC), 1–6. https://doi.org/10.1109/NPSC49263.2020.9331847 .
Thong Tran T, Zhang Y-C, Liao W-T, Lin Y-J, Li M-C, Huang H-S (2020) An autonomous mobile robot system based on serverless computing and edge computing In: 2020 21st Asia-Pacific Network Operations and Management Symposium (APNOMS), 334–337. https://doi.org/10.23919/APNOMS50412.2020.9236976 .
Witte PA, Louboutin M, Modzelewski H, Jones C, Selvage J, Herrmann FJ (2020) An event-driven approach to serverless seismic imaging in the cloud. IEEE Trans Parallel Distributed Syst 31(9):2032–2049. https://doi.org/10.1109/TPDS.2020.2982626 .
Ghosh BC, Addya SK, Somy NB, Nath SB, Chakraborty S, Ghosh SK (2020) Caching techniques to improve latency in serverless architectures In: 2020 International Conference on COMmunication Systems NETworkS (COMSNETS), 666–669. https://doi.org/10.1109/COMSNETS48256.2020.9027427 .
Quaresma D, Fireman D, Pereira TE (2020) Controlling garbage collection and request admission to improve performance of faas applications In: 2020 IEEE 32nd International Symposium on Computer Architecture and High Performance Computing (SBAC-PAD), 175–182. https://doi.org/10.1109/SBAC-PAD49847.2020.00033 .
Birman Y, Hindi S, Katz G, Shabtai A (2020) Cost-effective malware detection as a service over serverless cloud using deep reinforcement learning In: 2020 20th IEEE/ACM International Symposium on Cluster, Cloud and Internet Computing (CCGRID), 420–429. https://doi.org/10.1109/CCGrid49817.2020.00-51 .
Quang T, Peng Y (2020) Device-driven on-demand deployment of serverless computing functions In: 2020 IEEE International Conference on Pervasive Computing and Communications Workshops (PerCom Workshops), 1–6. https://doi.org/10.1109/PerComWorkshops48775.2020.9156140 .
Gunasekaran JR, Thinakaran P, Nachiappan NC, Kandemir MT, Das CR (2020) Fifer: Tackling Resource Underutilization in the Serverless Era. Association for Computing Machinery, New York.
Müller I, Marroquín R, Alonso G (2020) Lambada: Interactive data analytics on cold data using serverless cloud infrastructure In: Proceedings of the 2020 ACM SIGMOD International Conference on Management of Data (SIGMOD ’20), 115–130.. Association for Computing Machinery, New York. https://doi.org/10.1145/3318464.3389758 .
Chahal D, Ojha R, Ramesh M, Singhal R (2020) Migrating large deep learning models to serverless architecture In: 2020 IEEE International Symposium on Software Reliability Engineering Workshops (ISSREW), 111–116. https://doi.org/10.1109/ISSREW51248.2020.00047 .
Jain P, Munjal Y, Gera J, Gupta P (2020) Performance analysis of various server hosting techniques. Proc Comput Sci 173:70–77. https://doi.org/10.1016/j.procs.2020.06.010. International Conference on Smart Sustainable Intelligent Computing and Applications under ICITETM2020.
Khatri D, Khatri SK, Mishra D (2020) Potential bottleneck and measuring performance of serverless computing: A literature study In: 2020 8th International Conference on Reliability, Infocom Technologies and Optimization (Trends and Future Directions) (ICRITO), 161–164. https://doi.org/10.1109/ICRITO48877.2020.9197837 .
Kehrer S, Zietlow D, Scheffold J, Blochinger W (2020) Self-tuning serverless task farming using proactive elasticity control. Clust Comput. https://doi.org/10.1007/s10586-020-03158-3 .
Kelly D, Glavin F, Barrett E (2020) Serverless computing: Behind the scenes of major platforms In: 2020 IEEE 13th International Conference on Cloud Computing (CLOUD), 304–312. https://doi.org/10.1109/CLOUD49709.2020.00050 .
Ivan C, Vasile R, Dadarlat V (2019) Serverless computing: An investigation of deployment environments for web apis. Computers 8(2). https://doi.org/10.3390/computers8020050 .
Baresi L, Filgueira Mendonça D (2019) Towards a serverless platform for edge computing In: 2019 IEEE International Conference on Fog Computing (ICFC), 1–10. https://doi.org/10.1109/ICFC.2019.00008 .
Hall A, Ramachandran U (2019) An execution model for serverless functions at the edge In: Proceedings of the International Conference on Internet of Things Design and Implementation (IoTDI ‘19), 225–236.. Association for Computing Machinery, New York. https://doi.org/10.1145/3302505.3310084 .
Akkus IE, Chen R, Rimac I, Stein M, Satzke K, Beck A, Aditya P, Hilt V (2018) Sand: Towards high-performance serverless computing In: Proceedings of the 2018 USENIX Conference on Usenix Annual Technical Conference (USENIX ATC ‘18), 923–935.. USENIX Association, USA.
Keshavarzian A, Sharifian S, Seyedin S (2019) Modified deep residual network architecture deployed on serverless framework of iot platform based on human activity recognition application. Futur Gener Comput Syst 101:14–28. https://doi.org/10.1016/j.future.2019.06.009 .
Palade A, Kazmi A, Clarke S (2019) An evaluation of open source serverless computing frameworks support at the edge In: 2019 IEEE World Congress on Services (SERVICES), vol. 2642-939X, 206–211. https://doi.org/10.1109/SERVICES.2019.00057 .
Shahrad M, Balkind J, Wentzlaff D (2019) Architectural implications of function-as-a-service computing In: Proceedings of the 52nd Annual IEEE/ACM International Symposium on Microarchitecture (MICRO ‘52), 1063–1075.. Association for Computing Machinery, New York. https://doi.org/10.1145/3352460.3358296 .
Cicconetti C, Conti M, Passarella A (2020) A decentralized framework for serverless edge computing in the internet of things. IEEE Trans Netw Serv Manag:1–1. https://doi.org/10.1109/TNSM.2020.3023305 .
De Palma G, Giallorenzo S, Mauro J, Zavattaro G (2020) Allocation priority policies for serverless function-execution scheduling optimisation. In: Kafeza E, Benatallah B, Martinelli F, Hacid H, Bouguettaya A, Motahari H (eds)Service-Oriented Computing, 416–430.. Springer, Cham.
Djemame K, Parker M, Datsev D (2020) Open-source serverless architectures: an evaluation of apache openwhisk In: 2020 IEEE/ACM 13th International Conference on Utility and Cloud Computing (UCC), 329–335. https://doi.org/10.1109/UCC48980.2020.00052 .
Chadha M, Jindal A, Gerndt M (2020) Towards federated learning using faas fabric In: Proceedings of the 2020 Sixth International Workshop on Serverless Computing (WoSC’20), 49–54.. Association for Computing Machinery, New York. https://doi.org/10.1145/3429880.3430100 .
Cheng B, Fuerst J, Solmaz G, Sanada T (2019) Fog function: Serverless fog computing for data intensive iot services In: 2019 IEEE International Conference on Services Computing (SCC), 28–35. https://doi.org/10.1109/SCC.2019.00018 .
Mohanty SK, Premsankar G, di Francesco M (2018) An evaluation of open source serverless computing frameworks In: 2018 IEEE International Conference on Cloud Computing Technology and Science (CloudCom), 115–120. https://doi.org/10.1109/CloudCom2018.2018.00033 .
Li J, Kulkarni SG, Ramakrishnan KK, Li D (2019) Understanding open source serverless platforms: Design considerations and performance In: Proceedings of the 5th International Workshop on Serverless Computing (WOSC ‘19), 37–42.. Association for Computing Machinery, New York. https://doi.org/10.1145/3366623.3368139 .
Nguyen HD, Zhang C, Xiao Z, Chien AA (2019) Real-time serverless: Enabling application performance guarantees In: Proceedings of the 5th International Workshop on Serverless Computing (WOSC ’19), 1–6.. Association for Computing Machinery, New York. https://doi.org/10.1145/3366623.3368133 .
Saha A, Jindal S (2018) Emars: Efficient management and allocation of resources in serverless In: 2018 IEEE 11th International Conference on Cloud Computing (CLOUD), 827–830. https://doi.org/10.1109/CLOUD.2018.00113 .
Solaiman K, Adnan MA (2020) Wlec: A not so cold architecture to mitigate cold start problem in serverless computing In: 2020 IEEE International Conference on Cloud Engineering (IC2E), 144–153. https://doi.org/10.1109/IC2E48712.2020.00022 .
Chan A, Wang K-TA, Kumar V (2019) BalloonJVM : Dynamically Resizable Heap for FaaS In: CLOUD COMPUTING 2019 : The Tenth International Conference on Cloud Computing, GRIDs, and Virtualization, 99–104.
Handoyo E, Arfan M, Soetrisno YAA, Somantri M, Sofwan A, Sinuraya EW (2018) Ticketing chatbot service using serverless nlp technology In: 2018 5th International Conference on Information Technology, Computer, and Electrical Engineering (ICITACEE), 325–330. https://doi.org/10.1109/ICITACEE.2018.8576921 .
Zhang M, Zhu Y, Zhang C, Liu J (2019) Video processing with serverless computing: A measurement study In: Proceedings of the 29th ACM Workshop on Network and Operating Systems Support for Digital Audio and Video (NOSSDAV ’19), 61–66.. Association for Computing Machinery, New York. https://doi.org/10.1145/3304112.3325608 .
Fan D, He D (2020) A scheduler for serverless framework base on kubernetes In: Proceedings of the 2020 4th High Performance Computing and Cluster Technologies Conference; 2020 3rd International Conference on Big Data and Artificial Intelligence (HPCCT; BDAI 2020), 229–232.. Association for Computing Machinery, New York. https://doi.org/10.1145/3409501.3409503 .
Thomas S, Ao L, Voelker GM, Porter G (2020) Particle: Ephemeral endpoints for serverless networking In: Proceedings of the 11th ACM Symposium on Cloud Computing (SoCC ’20), 16–29.. Association for Computing Machinery, New York. https://doi.org/10.1145/3419111.3421275 .
Gramaglia M, Serrano P, Banchs A, Garcia-Aviles G, Garcia-Saavedra A, Perez R (2020) The case for serverless mobile networking In: 2020 IFIP Networking Conference (Networking), 779–784.
Danayi A, Sharifian S (2018) Pess-mina: A proactive stochastic task allocation algorithm for faas edge-cloud environments In: 2018 4th Iranian Conference on Signal Processing and Intelligent Systems (ICSPIS), 27–31. https://doi.org/10.1109/ICSPIS.2018.8700543 .
Mendki P (2020) Evaluating webassembly enabled serverless approach for edge computing In: 2020 IEEE Cloud Summit, 161–166. https://doi.org/10.1109/IEEECloudSummit48914.2020.00031 .
Chaudhry SR, Palade A, Kazmi A, Clarke S (2020) Improved qos at the edge using serverless computing to deploy virtual network functions. IEEE Internet Things J 7(10):10673–10683. https://doi.org/10.1109/JIOT.2020.3011057 .
Benedict S (2020) Serverless blockchain-enabled architecture for iot societal applications. IEEE Trans Comput Soc Syst 7(5):1146–1158. https://doi.org/10.1109/TCSS.2020.3008995 .
Cicconetti C, Conti M, Passarella A (2020) Uncoordinated access to serverless computing in mec systems for iot. Comput Netw 172:107184. https://doi.org/10.1016/j.comnet.2020.107184 .
Qiang W, Dong Z, Jin H (2018) Se-lambda: Securing privacy-sensitive serverless applications using sgx enclave. In: Beyah R, Chang B, Li Y, Zhu S (eds)Security and Privacy in Communication Networks, 451–470.. Springer, Cham. https://doi.org/10.1007/978-3-030-01701-9\_25 .
Prasetyadi G, Hantoro UT, Mutiara AB, Muslim A, Refianti R (2019) Heresy: A serverless web application to store compressed and encrypted document in the form of url In: 2019 Fourth International Conference on Informatics and Computing (ICIC), 1–5. https://doi.org/10.1109/ICIC47613.2019.8985735 .
Kim B, Heo S, Lee J, Jeong S, Lee Y, Kim H (2020) Compiler-assisted semantic-aware encryption for efficient and secure serverless computing. IEEE Internet Things J:1–1. https://doi.org/10.1109/JIOT.2020.3031550 .
O’Meara W, Lennon RG (2020) Serverless computing security: Protecting application logic In: 2020 31st Irish Signals and Systems Conference (ISSC), 1–5. https://doi.org/10.1109/ISSC49989.2020.9180214 .
Agache A, Brooker M, Iordache A, Liguori A, Neugebauer R, Piwonka P, Popa D-M (2020) Firecracker: Lightweight virtualization for serverless applications In: 17th USENIX Symposium on Networked Systems Design and Implementation (NSDI 20), 419–434.. USENIX Association, Santa Clara.
Pinto D, Dias JP, Sereno Ferreira H2018. Dynamic allocation of serverless functions in iot environments. https://doi.org/10.1109/euc.2018.00008 .
Mejáa A, Marcillo D, Guaño M, Gualotuña T (2020) Serverless based control and monitoring for search and rescue robots In: 2020 15th Iberian Conference on Information Systems and Technologies (CISTI), 1–6. https://doi.org/10.23919/CISTI49556.2020.9140444 .
Meißner D, Erb B, Kargl F, Tichy M (2018) Retro- λ : An event-sourced platform for serverless applications with retroactive computing support In: Proceedings of the 12th ACM International Conference on Distributed and Event-Based Systems (DEBS ‘18), 76–87.. Association for Computing Machinery, New York. https://doi.org/10.1145/3210284.3210285 .
Zhang T, Xie D, Li F, Stutsman R (2019) Narrowing the gap between serverless and its state with storage functions In: Proceedings of the ACM Symposium on Cloud Computing (SoCC ‘19), 1–12.. Association for Computing Machinery, New York. https://doi.org/10.1145/3357223.3362723 .
Grogan J, Mulready C, McDermott J, Urbanavicius M, Yilmaz M, Abgaz Y, McCarren A, MacMahon ST, Garousi V, Elger P, Clarke P (2020) A multivocal literature review of function-as-a-service (faas) infrastructures and implications for software developers. In: Yilmaz M, Niemann J, Clarke P, Messnarz R (eds)Systems, Software and Services Process Improvement, 58–75.. Springer, Cham.
Qin S, Wu H, Wu Y, Yan B, Xu Y, Zhang W (2020) Nuka: A generic engine with millisecond initialization for serverless computing In: 2020 IEEE International Conference on Joint Cloud Computing, 78–85. https://doi.org/10.1109/JCC49151.2020.00021 .
Zuk P, Rzadca K (2020) Scheduling methods to reduce response latency of function as a service In: 2020 IEEE 32nd International Symposium on Computer Architecture and High Performance Computing (SBAC-PAD), 132–140. https://doi.org/10.1109/SBAC-PAD49847.2020.00028 .
Shen J, Yu H, Zheng Z, Sun C, Xu M, Wang J (2020) Serpens: A high-performance serverless platform for nfv In: 2020 IEEE/ACM 28th International Symposium on Quality of Service (IWQoS), 1–10. https://doi.org/10.1109/IWQoS49365.2020.9213030 .
Ginzburg S, Freedman MJ (2020) Serverless isn’t server-less: Measuring and exploiting resource variability on cloud faas platforms In: Proceedings of the 2020 Sixth International Workshop on Serverless Computing (WoSC’20), 43–48.. Association for Computing Machinery, New York. https://doi.org/10.1145/3429880.3430099 .
Tankov V, Golubev Y, Bryksin T (2019) Kotless: A serverless framework for kotlin In: 2019 34th IEEE/ACM International Conference on Automated Software Engineering (ASE), 1110–1113. https://doi.org/10.1109/ASE.2019.00114 .
Vahidinia P, Farahani B, Aliee FS (2020) Cold start in serverless computing: Current trends and mitigation strategies In: 2020 International Conference on Omni-layer Intelligent Systems (COINS), 1–7. https://doi.org/10.1109/COINS49042.2020.9191377 .
Silva P, Fireman D, Pereira TE (2020) Prebaking Functions to Warm the Serverless Cold Start. Association for Computing Machinery, New York.
Tan B, Liu H, Rao J, Liao X, Jin H, Zhang Y (2020) Towards lightweight serverless computing via unikernel as a function In: 2020 IEEE/ACM 28th International Symposium on Quality of Service (IWQoS), 1–10. https://doi.org/10.1109/IWQoS49365.2020.9213020 .
Cordasco G, D’Auria M, Negro A, Scarano V, Spagnuolo C (2020) Fly: A domain-specific language for scientific computing on faas. In: Schwardmann U, Boehme C, B. Heras D, Cardellini V, Jeannot E, Salis A, Schifanella C, Manumachu RR, Schwamborn D, Ricci L, Sangyoon O, Gruber T, Antonelli L, Scott SL (eds)Euro-Par 2019: Parallel Processing Workshops, 531–544.. Springer, Cham.
Nupponen J, Taibi D (2020) Serverless: What it is, what to do and what not to do In: 2020 IEEE International Conference on Software Architecture Companion (ICSA-C), 49–50. https://doi.org/10.1109/ICSA-C50368.2020.00016 .
Reuter A, Back T, Andrikopoulos V (2020) Cost efficiency under mixed serverless and serverful deployments In: 2020 46th Euromicro Conference on Software Engineering and Advanced Applications (SEAA), 242–245. https://doi.org/10.1109/SEAA51224.2020.00049 .
Mahajan K, Figueiredo D, Misra V, Rubenstein D (2019) Optimal pricing for serverless computing In: 2019 IEEE Global Communications Conference (GLOBECOM), 1–6. https://doi.org/10.1109/GLOBECOM38437.2019.9013156 .
Lynn T, Rosati P, Lejeune A, Emeakaroha V (2017) A preliminary review of enterprise serverless cloud computing (function-as-a-service) platforms In: 2017 IEEE International Conference on Cloud Computing Technology and Science (CloudCom), 162–169. https://doi.org/10.1109/CloudCom.2017.15 .
Enes J, Expósito RR, Touriño J (2020) Real-time resource scaling platform for big data workloads on serverless environments. Futur Gener Comput Syst 105:361–379. https://doi.org/10.1016/j.future.2019.11.037 .
Elsakhawy M, Bauer M (2020) Faas2f: A framework for defining execution-sla in serverless computing In: 2020 IEEE Cloud Summit, 58–65. https://doi.org/10.1109/IEEECloudSummit48914.2020.00015 .
Bhattacharjee A, Chhokra AD, Kang Z, Sun H, Gokhale A, Karsai G (2019) Barista: Efficient and scalable serverless serving system for deep learning prediction services In: 2019 IEEE International Conference on Cloud Engineering (IC2E), 23–33. https://doi.org/10.1109/IC2E.2019.00-10 .
Maissen P, Felber P, Kropf P, Schiavoni V (2020) Faasdom: A benchmark suite for serverless computing In: Proceedings of the 14th ACM International Conference on Distributed and Event-Based Systems (DEBS ’20), 73–84.. Association for Computing Machinery, New York. https://doi.org/10.1145/3401025.3401738 .
Xu Z, Zhang H, Geng X, Wu Q, Ma H (2019) Adaptive function launching acceleration in serverless computing platforms In: 2019 IEEE 25th International Conference on Parallel and Distributed Systems (ICPADS), 9–16. https://doi.org/10.1109/ICPADS47876.2019.00011 .
Soltani B, Ghenai A, Zeghib N (2018) A migration-based approach to execute long-duration multi-cloud serverless functions. In: Maamri R Belala F (eds)Proceedings of the 3rd International Conference on Advanced Aspects of Software Engineering, ICAASE 2018, Constantine, Algeria, December 1-2, 2018 (CEUR Workshop Proceedings), vol. 2326, 42–50.
Martins H, Araujo F, da Cunha PR (2020) Benchmarking serverless computing platforms. J Grid Comput 18(4):691–709. https://doi.org/10.1007/s10723-020-09523-1 .
Download references
Acknowledgements
Author information, authors and affiliations.
Software Engineering and Embedded Systems (SEES) Research Group, College of Medicine, University of Duhok, Duhok, Kurdistan Region, Iraq
Hassan B. Hassan
Software Engineering and Embedded Systems (SEES) Research Group, Department of Computer Science, College of Science, University of Duhok, Duhok, Kurdistan Region, Iraq
Saman A. Barakat & Qusay I. Sarhan
You can also search for this author in PubMed Google Scholar
Contributions
Authors’ contributions.
Conceptualization: HBH, SAB, and QIS; methodology: HBH, SAB, and QIS; validation: HBH, SAB, and QIS; formal analysis: HBH, SAB, and QIS; investigation: HBH, SAB, and QIS; resources: HBH; data curation, HBH and SAB; writing—original draft preparation: HBH, SAB, and QIS; writing—review and editing: HBH, SAB, and QIS; visualization: SAB; supervision: QIS; It is noted that all authors cooperated with each other to achieve suitable information flow across the entire paper. The authors read and approved the final manuscript.
Authors’ information
Hassan B. Hassan received the B.Sc. degree in Computer Science from University of Duhok, Iraq, in 2010. He completed the M.Sc. degree in Web Applications and Services, from Leicester University, UK, in 2015. He is currently working as an assistant lecturer at the college of medicine, University of Duhok, Iraq. His main areas of research interest are cloud computing, web programming, big data, and human computer interaction.
Saman A. Barakat received the B.Sc. degree in Computer Science from University of Duhok, Iraq, in 2008. He completed the M.Sc. degree in Advanced Computer Science, from Newcastle University, UK, in 2012. He is currently working as a lecturer at the college of science, University of Duhok, Iraq. His main areas of research interest are cloud computing, and software engineering.
Qusay I. Sarhan received the B.Sc. degree in Software Engineering from University of Mosul, Iraq, in 2007 and the M.Tech. degree in Software Engineering from Jawaharlal Nehru Technological University, India, in 2011. Currently, he is a lecturer and the leader of Software Engineering and Embedded Systems (SEES) research group at University of Duhok, Iraq. He has a couple of national and international publications and his research interests include software engineering, internet of things, and embedded systems.
Corresponding author
Correspondence to Qusay I. Sarhan .
Ethics declarations
Competing interests.
The authors declare that they have no competing interests.
Additional information
Publisher’s note.
Springer Nature remains neutral with regard to jurisdictional claims in published maps and institutional affiliations.
Rights and permissions
Open Access This article is licensed under a Creative Commons Attribution 4.0 International License, which permits use, sharing, adaptation, distribution and reproduction in any medium or format, as long as you give appropriate credit to the original author(s) and the source, provide a link to the Creative Commons licence, and indicate if changes were made. The images or other third party material in this article are included in the article’s Creative Commons licence, unless indicated otherwise in a credit line to the material. If material is not included in the article’s Creative Commons licence and your intended use is not permitted by statutory regulation or exceeds the permitted use, you will need to obtain permission directly from the copyright holder. To view a copy of this licence, visit http://creativecommons.org/licenses/by/4.0/ .
Reprints and permissions
About this article
Cite this article.
Hassan, H.B., Barakat, S.A. & Sarhan, Q.I. Survey on serverless computing. J Cloud Comp 10 , 39 (2021). https://doi.org/10.1186/s13677-021-00253-7
Download citation
Received : 01 July 2020
Accepted : 21 June 2021
Published : 12 July 2021
DOI : https://doi.org/10.1186/s13677-021-00253-7
Share this article
Anyone you share the following link with will be able to read this content:
Sorry, a shareable link is not currently available for this article.
Provided by the Springer Nature SharedIt content-sharing initiative
- Cloud computing
- Serverless computing
- Serverless platforms
- Serverless benefits
- Serverless challenges
Managing Cloud Computing Across the Product Lifecycle
Managing Cloud Computing Across the Product Lifecycle
MANAGING CLOUD COMPUTING ACROSS THE PRODUCT LIFECYCLE: DEVELOPMENT OF A
Outline 1. motivation 2. theoretical foundation 3. results 4. conclusion, 1. motivation focus of research: provide a conceptual model, 2. theoretical foundation cloud computing a way for organizations, 3. result – mapping cloud benefits to the product lifecycle, 4. result – cloud-lifecycle-benefit-matrix applicability of benefit stage cloud computing, 4. result – most beneficial cloud deployment model per lifecycle, 5. conclusion the contribution of this paper is twofold:.

IMAGES
VIDEO
COMMENTS
This paper presents a meta-analysis of cloud computing research in information systems with the aim of taking stock of literature and their associated research frameworks, research methodology, geographical distribution, level of analysis as well as trends of these studies over the period of 7 years. ... Cloud computing research started to gain ...
Due to growing network data dissemination in cloud, the elasticity, pay as you go options, globally accessible facilities, and security of networks have become increasingly important in today's world. Cloud se... R. Julian Menezes, P. Jesu Jayarin and A. Chandra Sekar. Journal of Cloud Computing 2024 13 :101.
The Journal of Cloud Computing: Advances, Systems and Applications (JoCCASA) will publish research articles on all aspects of Cloud Computing. Principally, articles will address topics that are core to Cloud Computing, focusing on the Cloud applications, the Cloud systems, and the advances that will lead to the Clouds of the future.
Additionally, assist cloud computing researchers in making intelligent decisions and identifying research gaps that require further investigation. The remaining structure of the paper is as follows: Section 2 begins with a brief introduction to review methodology, followed by the research questions, article selection procedure, and ...
Metrics. Since 2012, the Journal of Cloud Computing has been promoting research and technology development related to Cloud Computing, as an elastic framework for provisioning complex infrastructure services on-demand, including service models such as Infrastructure as a Service (IaaS), Platform as a Service (PaaS), and Software as a Service ...
We present a meta-analysis of cloud computing research in information systems. The study includes 152 referenced journal articles published between January 2010 to June 2023. We take stock of the literature and the associated research themes, research frameworks, the employed research methodology, and the geographical distribution of the articles.
Next generation cloud computing systems are aimed at becoming more ambient, pervasive and ubiquitous given the emerging trends of distributed, heterogeneous and ad hoc cloud infrastructure and associated computing architectures. This will impact at least the following four areas considered in this paper.
review is thought to inspire enterprises and managers that would like to use cloud computing in. terms of the scope, solution methods, factors, dimensions, and the results achieved in a holistic ...
Cloud computing is an evolution of information technology and a dominant business model for delivering IT resources. With cloud computing, individuals and organizations can gain on-demand network access to a shared pool of managed and scalable IT resources, such as servers, storage, and applications. Recently, academics as well as practitioners ...
The paper also gives a concise description of Cloud Computing concepts and technologies. It starts with a brief history about cloud computing, tracing its roots. Then, architectural models of cloud services are described, and the most relevant products for Cloud Computing are briefly discussed along with a comprehensive literature review.
Browse the latest documents on cloud computing from various journals and sources. Find topics such as load balancing, forensics, trust management, service composition, and more.
Student. , M.Sc. I.T., I.C.S. College, Khed, Ratnagri. Abstract: Cloud Computing has come of age later Amazons introduce the first of its kind of cloud services in2006. It is. particularly ...
Assistant Professor, Ajman University, UAE. Doi: 10.36941/ajis-2020-0008. Abstract. This study is a literature review on cloud computing cloud computing trends as on e the fastest growing ...
With the advances in machine learning (ML) and deep learning (DL) techniques, and the potency of cloud computing in offering services efficiently and cost-effectively, Machine Learning as a Service (MLaaS) cloud platforms have become popular. In addition, there is increasing adoption of third-party cloud services for outsourcing training of DL models, which requires substantial costly ...
This paper investigates the organizational adaptation for cloud computing technology - reviewing case studies from various institutions and companies worldwide to provide a detailed analysis of innovative techniques with cloud computing. ... Finally, we investigate the future research directions for cloud computing and expand this paper into ...
Cloud computing has become a widely exploited research area in academia and industry. Cloud computing benefits both cloud services providers (CSPs) and consumers. The security challenges associated with cloud computing have been widely studied in the literature. This systematic literature review (SLR) is aimed to review the existing research studies on cloud computing security, threats, and ...
In this paper, we present a survey of cloud computing, highlighting its key concepts, architectural principles, state-of-the-art implementation as well as research challenges. The aim of this paper is to provide a better understanding of the design challenges of cloud computing and identify important research directions in this increasingly ...
This paper surveys the two frontiers - Big Data and cloud computing - and reviews the advantages and consequences of utilizing cloud computing to tackling Big Data in the digital earth and relevant science domains. ... This review introduces future innovations and a research agenda for cloud computing supporting the transformation of the ...
In a complex situation, this paper proposes a comprehensive cloud computing privacy protection security system based on a variety of technologies, such as access control, trust, ... and conforms to the real model of cloud computing. (4) Research on access control model of space-time awareness. When the cloud computing environment is expanded to ...
2017b) whose prices should be indicative of cloud services prices.2 This paper builds on. their work by developing measures to quantify the service prices and quantities and the. capital investment relevant for understanding the U.S. cloud services industry —the Ps, Qs, and K's of the title.
The abstract provides a brief summary of the research paper, highlighting the key topics covered. It explains that the paper will give a brief description of the various technologies and tools used for Big Data in cloud computing environments, as well as the advantages and difficulties posed by this technology. This paper reviews the research challenges and issues associated with big data ...
A COMPREHENSIVE STUDY ON CLOUD. COMPUTING PARADIGM. Ab Rashid Dar 1, Dr. D. Ravindran 2. 1,2 Department of Computer Science, St. Joseph's College. (Autonomous), Tiruchirappalli Tamil Nadu, (Indi ...
This paper proposes cloud-based service architecture for managing ML models that best fit different IoT device operational configurations for security, and suggests an IoT device may benefit from offloading to the cloud heavy-weight activities such as feature selection, model building, training, and validation, thus reducing its IDS maintenance workload at the IoT device and get the security ...
The authors in have conducted a systematic exploration of serverless computing-related research papers. As they mentioned, their work is not a survey, but it is a supporting source for future research papers. ... Suter P (2017) Serverless Computing: Current Trends and Open Problems In: Research Advances in Cloud Computing, 1-20.. Springer ...
From 2010 to 2021, we found 52 papers that were of top ranked journals and conferences. From 2010 to 2017, there is linear work in cloud computing, however, after 2017, a lot of work is done in cloud computing data security. From 2018 to 2021, 37 papers are published. After 2018, the trend about data security in cloud computing increased very ...
Virtual private network (VPN) gateways are widely applied to provide secure end-to-end remote access and to relay reliable interconnected communication in cloud computing. As network convergence nodes, the performance of VPN gateways is limited by traditional methods of packet receiving and sending, the kernel protocol stack and the virtual network interface card. This paper proposes a generic ...
Gartner (20 19) forecasted that there will b e a 17 percent growth of cloud computing in 2020, which totals to 266.4. billion USD in m arket value from 227.8 billion USD in 2019. Sub sequently ...
1. MOTIVATION Focus of research: provide a conceptual model for the use of cloud computing in digital product development Cloud computing is considered a - and possibly the - strategic driver of digital product development (Bharadwaj et al. 2013; Hanelt et al. 2015), with the main reasons being low entry barriers and high scalability In a recent review, only about 9% of the 285 articles ...
In order to solve the shortcomings of ant colony algorithm in solving large-scale task scheduling problems in cloud computing, the convergence speed is slow and easy to fall into local optimal solutions. This paper designs an adaptive task scheduling algorithm for cloud computing based on ant colony algorithm. On the basis of the polymorphic ant colony algorithm, a pheromone adaptive update ...
Dr Dmitrii Ustiugov (left) from College of Computing and Data Science, NTU and Dr Marios Kogias (right) from Department of Computing, Imperial College London have secured an NTU-Imperial collaboration grant, aiming to foster joint research and innovation in future cloud systems and technologies. To initiate this venture, the College of Computing and Data Science (CCDS) will host the inaugural ...