
An official website of the United States government
The .gov means it’s official. Federal government websites often end in .gov or .mil. Before sharing sensitive information, make sure you’re on a federal government site.
The site is secure. The https:// ensures that you are connecting to the official website and that any information you provide is encrypted and transmitted securely.
- Publications
- Account settings
Preview improvements coming to the PMC website in October 2024. Learn More or Try it out now .
- Advanced Search
- Journal List
- J Hum Reprod Sci
- v.5(1); Jan-Apr 2012

This article has been retracted.
Sample size estimation and power analysis for clinical research studies.
Department of Biostatistics, National Institute of Animal Nutrition and Physiology, Bangalore, India
S Chandrashekara
1 Department of Immunology and Reumatology, ChanRe Rheumatology and Immunology Center and Research, Bangalore, India
Determining the optimal sample size for a study assures an adequate power to detect statistical significance. Hence, it is a critical step in the design of a planned research protocol. Using too many participants in a study is expensive and exposes more number of subjects to procedure. Similarly, if study is underpowered, it will be statistically inconclusive and may make the whole protocol a failure. This paper covers the essentials in calculating power and sample size for a variety of applied study designs. Sample size computation for single group mean, survey type of studies, 2 group studies based on means and proportions or rates, correlation studies and for case-control for assessing the categorical outcome are presented in detail.
INTRODUCTION
Clinical research studies can be classified into surveys, experiments, observational studies etc. They need to be carefully planned to achieve the objective of the study. The planning of a good research has many aspects. First step is to define the problem and it should be operational. Second step is to define the experimental or observational units and the appropriate subjects and controls. Meticulously, one has to define the inclusion and exclusion criteria, which should take care of all possible variables which could influence the observations and the units which are measured. The study design must be clear and the procedures are defined to the best possible and available methodology. Based on these factors, the study must have an adequate sample size, relative to the goals and the possible variabilities of the study. Sample must be ‘big enough’ such that the effect of expected magnitude of scientific significance, to be also statistically significant. Same time, It is important that the study sample should not be ‘too big’ where an effect of little scientific importance is nevertheless statistically detectable. In addition, sample size is important for economic reasons: An under-sized study can be a waste of resources since it may not produce useful results while an over-sized study uses more resources than necessary. In an experiment involving human or animal subjects, sample size is a critical ethical issue. Since an ill-designed experiment exposes the subjects to potentially harmful treatments without advancing knowledge.[ 1 , 2 ] Thus, a fundamental step in the design of clinical research is the computation of power and sample size. Power is the probability of correctly rejecting the null hypothesis that sample estimates (e.g. Mean, proportion, odds, correlation co-efficient etc.) does not statistically differ between study groups in the underlying population. Large values of power are desirable, at least 80%, is desirable given the available resources and ethical considerations. Power proportionately increases as the sample size for study increases. Accordingly, an investigator can control the study power by adjusting the sample size and vice versa.[ 3 , 4 ]
A clinical study will be expressed in terms of an estimate of effect, appropriate confidence interval, and P value. The confidence interval indicates the likely range of values for the true effect in the population while the P value determines the how likely that the observed effect in the sample is due to chance. A related quantity is the statistical power; this is the probability of identifying an exact difference between 2 groups in the study samples when one genuinely exists in the populations from which the samples were drawn.
Factors that affect the sample size
The calculation of an appropriate sample size relies on choice of certain factors and in some instances on crude estimates. There are 3 factors that should be considered in calculation of appropriate sample size- summarized in Table 1 . The each of these factors influences the sample size independently, but it is important to combine all these factors in order to arrive at an appropriate sample size.
Factors that affect sample size calculations

The Normal deviates for different significance levels (Type I error or Alpha) for one tailed and two tailed alternative hypothesis are shown in Table 2 .
The normal deviates for Type I error (Alpha)

The normal deviates for different power, probability of rejecting null hypothesis when it is not true or one minus probability of type II error are in shown Table 3 .
The normal deviates for statistical power

Study design, outcome variable and sample size
Study design has a major impact on the sample size. Descriptive studies need hundreds of subjects to give acceptable confidence interval for small effects. Experimental studies generally need lesser sample while the cross-over designs needs one-quarter of the number required compared to a control group because every subject gets the experimental treatment in cross-over study. An evaluation studies in single group with pre-post type of design needs half the number for a similar study with a control group. A study design with one-tailed hypothesis requires 20% lesser subjects compared to two-tailed studies. Non-randomized studies needs 20% more subjects compared to randomized studies in order to accommodate confounding factors. Additional 10 - 20% subjects are required to allow adjustment of other factors such as withdrawals, missing data, lost to follow-up etc.
The “outcome” expected under study should be considered. There are 3 possible categories of outcome. The first is a simple case where 2 alternatives exist: Yes/no, death/alive, vaccinated/not vaccinated, etc. The second category covers multiple, mutually exclusive alternatives such as religious beliefs or blood groups. For these 2 categories of outcome, the data are generally expressed as percentages or rates[ 5 – 7 ] The third category covers continuous response variables such as weight, height, blood pressure, VAS score, IL6, TNF-a, homocysteine etc, which are continuous measures and are summarized as means and standard deviations. The statistical methods appropriates the sample size based on which of these outcomes measure is critical for the study, for example, larger sample size is required to assess the categorical variable compared to continuous outcome variable.
Alpha level
The definition of alpha is the probability of detecting a significant difference when the treatments are equally effective or risk of false positive findings. The alpha level used in determining the sample size in most of academic research studies are either 0.05 or 0.01.[ 7 ] Lower the alpha level, larger is the sample size. For example, a study with alpha level of 0.01 requires more subjects when compared to a study with alpha level of 0.05 for similar outcome variable. Lower alpha viz 0.01 or less is used when the decisions based on the research are critical and the errors may cause substantial, financial, or personal harm.
Variance or standard deviation
The variance or standard deviation for sample size calculation is obtained either from previous studies or from pilot study. Larger the standard deviation, larger is the sample size required in a study. For example, in a study, with primary outcome variable is TNF-a, needs more subjects compared to a variable of birth weight, 10-point Vas score etc. as the natural variability of TNF-a is wide compared to others.
Minimum detectable difference
This is the expected difference or relationship between 2 independent samples, also known as the effect size. The obvious question is how to know the difference in a study, which is not conducted. If available, it may be useful to use the effect size found from prior studies. Where no previous study exists, the effect size is determined from literature review, logical assertion, and conjecture.
The difference between 2 groups in a study will be explored in terms of estimate of effect, appropriate confidence interval, and P value. The confidence interval indicates the likely range of values for the true effect in a population while P value determines how likely it is that the observed effect in the sample is due to chance. A related quantity is the statistical power of the study, is the probability of detecting a predefined clinical significance. The ideal study is the one, which has high power. This means that the study has a high chance of detecting a difference between groups if it exists, consequently, if the study demonstrates no difference between the groups, the researcher can reasonably confident in concluding that none exists. The ideal power for any study is considered to be 80%.[ 8 ]
In research, statistical power is generally calculated with 2 objectives. 1) It can be calculated before data collection based on information from previous studies to decide the sample size needed for the current study. 2) It can also be calculated after data analysis. The second situation occurs when the result turns out to be non-significant. In this case, statistical power is calculated to verify whether the non-significance result is due to lack of relationship between the groups or due to lack of statistical power.
Statistical power is positively correlated with the sample size, which means that given the level of the other factors viz. alpha and minimum detectable difference, a larger sample size gives greater power. However, researchers should be clear to find a difference between statistical difference and scientific difference. Although a larger sample size enables researchers to find smaller difference statistically significant, the difference found may not be scientifically meaningful. Therefore, it is recommended that researchers must have prior idea of what they would expect to be a scientifically meaningful difference before doing a power analysis and determine the actual sample size needed. Power analysis is now integral to the health and behavioral sciences, and its use is steadily increasing whenever the empirical studies are performed.
Withdrawals, missing data and losses to follow-up
Sample size calculated is the total number of subjects who are required for the final study analysis. There are few practical issues, which need to be considered while calculating the number of subjects required. It is a fact that all eligible subjects may not be willing to take part and may be necessary screen more subjects than the final number of subjects entering the study. In addition, even in well-designed and conducted studies, it is unusual to finish with a dataset, which is complete for all the subjects recruited, in a usable format. The reason could be subject factor like- subjects may fail or refuse to give valid responses to particular questions, physical measurements may suffer from technical problems, and in studies involving follow-up (eg. Trials or cohort studies), there will be some degree of attrition. The reason could be technical and the procedural problem- like contamination, failure to get the assessment or test performed in time. It may, therefore, necessary to consider these issues before calculating the number of subjects to be recruited in a study in order to achieve the final desired sample size.
Example, say in a study, a total of N number of subjects are required in the end of the study with all the data being complete for analysis, but a proportion (q) are expected to refuse to participate or drop out before the study ends. In this case, the following total number of subjects (N 1 ) would have to be recruited to ensure that the final sample size (N) is achieved:

The proportion of eligible subjects who will refuse to participate or provide the inadequate information will be unknown at the beginning of the study. Approximate estimates is often possible using information from similar studies in comparable populations or from an appropriate pilot study.[ 9 ]
Sample size estimation for proportion in survey type of studies
A common goal of survey research is to collect data representative of population. The researcher uses information gathered from the survey to generalize findings from a drawn sample back to a population, within the limits of random error. The general rule relative to acceptable margins of error in survey research is 5 - 10%. The sample size can be estimated using the following formula

Where P is the prevalence or proportion of event of interest for the study, E is the Precision (or margin of error) with which a researcher want to measure something. Generally, E will be 10% of P and Z α/2 is normal deviate for two-tailed alternative hypothesis at a level of significance; for example, for 5% level of significance, Z α/2 is 1.96 and for 1% level of significance it is 2.58 as shown in Table 2 . D is the design effect reflects the sampling design used in the survey type of study. This is 1 for simple random sampling and higher values (usually 1 to 2) for other designs such as stratified, systematic, cluster random sampling etc, estimated to compensate for deviation from simple random sampling procedure. The design effect for cluster random sampling is taken as 1.5 to 2. For the purposive sampling, convenience or judgment sampling, D will cross 10. Higher the D, the more will be sample size required for a study. Simple random sampling is unlikely to be the sampling method in an actual filed survey. If another sampling method such as systematic, stratified, cluster sampling etc. is used, a larger sample size is likely to be needed because of the “design effect”.[ 10 – 12 ] In case of impact study, P may be estimated at 50% to reflect the assumption that an impact is expected in 50% of the population. A P of 50% is also a conservative estimate; Example: Researcher interested to know the sample size for conducting a survey for measuring the prevalence of obesity in certain community. Previous literature gives the estimate of an obesity at 20% in the population to be surveyed, and assuming 95% confidence interval or 5% level of significance and 10% margin of error, the sample size can be calculated as follow as;
N = (Z α/2 ) 2 P(1-P)*1 / E 2 = (1.96) 2 *0.20*(1-0.20)/(0.1*0.20) 2 = 3.8416*0.16/(0.02) 2 = 1537 for a simple random sampling design. Hence, sample size of 1537 is required to conduct community-based survey to estimate the prevalence of obesity. Note-E is the margin of error, in the present example; it is 10% χ 0.20 = 0.02.
To find the final adjusted sample size, allowing non-response rate of 10% in the above example, the adjusted sample size will be 1537/(1-0.10) = 1537/0.90 = 1708.
Sample size estimation with single group mean
If researcher is conducting a study in single group such as outcome assessment in a group of patients subjected to certain treatment or patients with particular type of illness and the primary outcome is a continuous variable for which the mean and standard deviation are expression of results or estimates of population, the sample size can be estimated using the following formula
N = (Z α/2 ) 2 s 2 / d 2 ,
where s is the standard deviation obtained from previous study or pilot study, and d is the accuracy of estimate or how close to the true mean. Z α/2 is normal deviate for two- tailed alternative hypothesis at a level of significance.
Research studies with one tailed hypothesis, above formula can be rewritten as
N = (Z α ) 2 s 2 / d 2 , the Z α values are 1.64 and 2.33 for 5% and 1% level of significance.
Example: In a study for estimating the weight of population and wants the error of estimation to be less than 2 kg of true mean (that is expected difference of weight to be 2 kg), the sample standard deviation was 5 and with a probability of 95%, and (that is) at an error rate of 5%, the sample size estimated as N = (1.96) 2 (5) 2 / 2 2 gives the sample of 24 subjects, if the allowance of 10% for missing, losses to follow-up, withdrawals is assumed, then the corrected sample will be 27 subjects. Corrected sample size thus obtained is 24/(1.0-0.10) ≅ 24/0.9 = 27 and for 20% allowances, the corrected sample size will be 30.
Sample size estimation with two means
In a study with research hypothesis viz; Null hypothesis H o : m 1 = m 2 vs. alternative hypothesis H a : m 1 = m 2 + d where d is the difference between two means and n1 and n2 are the sample size for Group I and Group II such that N = n1 + n2. The ratio r = n1/n2 is considered whenever the researcher needs unequal sample size due to various reasons, such as ethical, cost, availability etc.
Then, the total sample size for the study is as follows

Sample size estimation with two proportions
In study based on outcome in proportions of event in two populations (groups), such as percentage of complications, mortality improvement, awareness, surgical or medical outcome etc., the sample size estimation is based on proportions of outcome, which is obtained from previous literature review or conducting pilot study on smaller sample size. A study with null hypothesis of H o : π 1 = π 2 vs. H a : π 1 = π 2 + d , where π are population proportion and p1 and p2 are the corresponding sample estimates, the sample size can be estimated using the following formula

If researcher is planning to conduct a study with unequal groups, he or she must calculate N as if we are using equal groups, and then calculate the modified sample size. If r = n1/n2 is the ratio of sample size in 2 groups, then the required sample size is N 1 = N (1+ r ) 2 /4 r , if n1 = 2n2 that is sample size ratio is 2:1 for group 1 and group 2, then N 1 = 9 N /8, a fairly small increase in total sample size.
Example: It is believed that the proportion of patients who develop complications after undergoing one type of surgery is 5% while the proportion of patients who develop complications after a second type of surgery is 15%. How large should the sample be in each of the 2 groups of patients if an investigator wishes to detect, with a power of 90%, whether the second procedure has a complications rate significantly higher than the first at the 5% level of significance?
In the example,
- a) Test value of difference in complication rate 0%
- b) Anticipated complication rate 5%, 15% in 2 groups
- c) Level of significance 5%
- d) Power of the test 90%
- e) Alternative hypothesis(one tailed) (p 1 -p 2 ) < 0%
The total sample size required is 74 for equal size distribution, for unequal distribution of sample size with 1.5:1 that is r = 1.5, the total sample size will be 77 with 46 for group I and 31 for group II.
Sample size estimation with correlation co-efficient
In an observational studies, which involves to estimate a correlation (r) between 2 variables of interest say, X and Y, a typical hypothesis of form H 0 : r = 0 against H a :r ≠ 0, the sample size for correlation study can be obtained by computing

Example: According to the literature, the correlation between salt intake and systolic blood pressure is around 0.30. A study is conducted to attests this correlation in a population, with the significance level of 1% and power of 90%. The sample size for such a study can be estimated as follows:

Sample size estimation with odds ratio
In case-control study, data are usually summarized in odds ratio, rather than difference between two proportions when the outcome variables of interest were categorical in nature. If P1 and P2 are proportion of cases and controls, respectively, exposed to a risk factor, then:

Example: The prevalence of vertebral fracture in a population is 25%. When the study is interested to estimate the effect of smoking on the fracture, with an odds ratio of 2, at the significance level of 5% (one-sided test) and power of 80%, the total sample size for the study of equal sample size can be estimated by:

The equations in this paper assume that the selection of individual is random and unbiased. The decisions to include a subject in the study depend on whether or not that subject has the characteristic or the outcome studied. Second, in studies in which the mean is calculated, the measurements are assumed to have normal distributions.[ 13 , 14 ]
The concept of statistical power is more associated with sample size, the power of the study increases with an increase in sample size. Ideally, minimum power of a study required is 80%. Hence, the sample size calculation is critical and fundamental for designing a study protocol. Even after completion of study, a retrospective power analysis will be useful, especially when a statistically not a significant results are obtained.[ 15 ] Here, actual sample size and alpha-level are known, and the variance observed in the sample provides an estimate of variance of population. The analysis of power retrospectively re-emphasizes the fact negative finding is a true negative finding.
The ideal study for the researcher is one in which the power is high. This means that the study has a high chance of detecting a difference between groups if one exists; consequently, if the study demonstrates no difference between groups, the researcher can be reasonably confident in concluding that none exists. The Power of the study depends on several factors, but as a general rule, higher power is achieved by increasing the sample size.[ 16 ] Many apparently null studies may be under-powered rather than genuinely demonstrating no difference between groups, absence of evidence is not evidence of absence.[ 9 ]
A Sample size calculation is an essential step in research protocols and is a must to justify the size of clinical studies in papers, reports etc. Nevertheless, one of the most common error in papers reporting clinical trials is a lack of justification of the sample size, and it is a major concern that important therapeutic effects are being missed because of inadequately sized studies.[ 17 , 18 ] The purpose of this review is to make available a collection of formulas for sample size calculations and examples for variety of situations likely to be encountered.
Often, the research is faced with various constraints that may force them to use an inadequate sample size because of both practical and statistical reasons. These constraints may include budget, time, personnel, and other resource limitations. In these cases, the researchers should report both the appropriate sample size along with sample size actually used in the study; the reasons for using inadequate sample sizes and a discussion of the effect of inadequate sample size may have on the results of the study. The researcher should exercise caution when making pragmatic recommendations based on the research with an inadequate sample size.
Sample size determination is an important major step in the design of a research study. Appropriately-sized samples are essential to infer with confidence that sample estimated are reflective of underlying population parameters. The sample size required to reject or accept a study hypothesis is determined by the power of an a-test. A study that is sufficiently powered has a statistical rescannable chance of answering the questions put forth at the beginning of research study. Inadequately sized studies often results in investigator's unrealistic assumptions about the effectiveness of study treatment. Misjudgment of the underlying variability for parameter estimates wrong estimate of follow-up period to observe the intended effects of the treatment and inability to predict the lack of compliance of the study regimen, and a high drop-rate rates and/or the failure to account for the multiplicity of study endpoints are the common error in a clinical research. Conducting a study that has little chance of answering the hypothesis at hand is a misuse of time and valuable resources and may unnecessarily expose participants to potential harm or unwarranted expectations of therapeutic benefits. As scientific and ethical issue go hand-in-hand, the awareness of determination of minimum required sample size and application of appropriate sampling methods are extremely important in achieving scientifically and statistically sound results. Using an adequate sample size along with high quality data collection efforts will result in more reliable, valid and generalizable results, it could also result in saving resources. This paper was designed as a tool that a researcher could use in planning and conducting quality research.
Source of Support: Nil
Conflict of Interest: None declared.
- Skip to primary navigation
- Skip to main content
- Skip to primary sidebar
Institute for Digital Research and Education
Introduction to Power Analysis
This seminar treats power and the various factors that affect power on both a conceptual and a mechanical level. While we will not cover the formulas needed to actually run a power analysis, later on we will discuss some of the software packages that can be used to conduct power analyses.
OK, let’s start off with a basic definition of what a power is. Power is the probability of detecting an effect, given that the effect is really there. In other words, it is the probability of rejecting the null hypothesis when it is in fact false. For example, let’s say that we have a simple study with drug A and a placebo group, and that the drug truly is effective; the power is the probability of finding a difference between the two groups. So, imagine that we had a power of .8 and that this simple study was conducted many times. Having power of .8 means that 80% of the time, we would get a statistically significant difference between the drug A and placebo groups. This also means that 20% of the times that we run this experiment, we will not obtain a statistically significant effect between the two groups, even though there really is an effect in reality.
There are several of reasons why one might do a power analysis. Perhaps the most common use is to determine the necessary number of subjects needed to detect an effect of a given size. Note that trying to find the absolute, bare minimum number of subjects needed in the study is often not a good idea. Additionally, power analysis can be used to determine power, given an effect size and the number of subjects available. You might do this when you know, for example, that only 75 subjects are available (or that you only have the budget for 75 subjects), and you want to know if you will have enough power to justify actually doing the study. In most cases, there is really no point to conducting a study that is seriously underpowered. Besides the issue of the number of necessary subjects, there are other good reasons for doing a power analysis. For example, a power analysis is often required as part of a grant proposal. And finally, doing a power analysis is often just part of doing good research. A power analysis is a good way of making sure that you have thought through every aspect of the study and the statistical analysis before you start collecting data.
Despite these advantages of power analyses, there are some limitations. One limitation is that power analyses do not typically generalize very well. If you change the methodology used to collect the data or change the statistical procedure used to analyze the data, you will most likely have to redo the power analysis. In some cases, a power analysis might suggest a number of subjects that is inadequate for the statistical procedure. For example, a power analysis might suggest that you need 30 subjects for your logistic regression, but logistic regression, like all maximum likelihood procedures, require much larger sample sizes. Perhaps the most important limitation is that a standard power analysis gives you a “best case scenario” estimate of the necessary number of subjects needed to detect the effect. In most cases, this “best case scenario” is based on assumptions and educated guesses. If any of these assumptions or guesses are incorrect, you may have less power than you need to detect the effect. Finally, because power analyses are based on assumptions and educated guesses, you often get a range of the number of subjects needed, not a precise number. For example, if you do not know what the standard deviation of your outcome measure will be, you guess at this value, run the power analysis and get X number of subjects. Then you guess a slightly larger value, rerun the power analysis and get a slightly larger number of necessary subjects. You repeat this process over the plausible range of values of the standard deviation, which gives you a range of the number of subjects that you will need.
After all of this discussion of power analyses and the necessary number of subjects, we need to stress that power is not the only consideration when determining the necessary sample size. For example, different researchers might have different reasons for conducting a regression analysis. One might want to see if the regression coefficient is different from zero, while the other wants to get a very precise estimate of the regression coefficient with a very small confidence interval around it. This second purpose requires a larger sample size than does merely seeing if the regression coefficient is different from zero. Another consideration when determining the necessary sample size is the assumptions of the statistical procedure that is going to be used. The number of statistical tests that you intend to conduct will also influence your necessary sample size: the more tests that you want to run, the more subjects that you will need. You will also want to consider the representativeness of the sample, which, of course, influences the generalizability of the results. Unless you have a really sophisticated sampling plan, the greater the desired generalizability, the larger the necessary sample size. Finally, please note that most of what is in this presentation does not readily apply to people who are developing a sampling plan for a survey or psychometric analyses.
Definitions
Before we move on, let’s make sure we are all using the same definitions. We have already defined power as the probability of detecting a “true” effect, when the effect exists. Most recommendations for power fall between .8 and .9. We have also been using the term “effect size”, and while intuitively it is an easy concept, there are lots of definitions and lots of formulas for calculating effect sizes. For example, the current APA manual has a list of more than 15 effect sizes, and there are more than a few books mostly dedicated to the calculation of effect sizes in various situations. For now, let’s stick with one of the simplest definitions, which is that an effect size is the difference of two group means divided by the pooled standard deviation. Going back to our previous example, suppose the mean of the outcome variable for the drug A group was 10 and it was 5 for the placebo group. If the pooled standard deviation was 2.5, we would have and effect size which is equal to (10-5)/2.5 = 2 (which is a large effect size).
We also need to think about “statistically significance” versus “clinically relevant”. This issue comes up often when considering effect sizes. For example, for a given number of subjects, you might only need a small effect size to have a power of .9. But that effect size might correspond to a difference between the drug and placebo groups that isn’t clinically meaningful, say reducing blood pressure by two points. So even though you would have enough power, it still might not be worth doing the study, because the results would not be useful for clinicians.
There are a few other definitions that we will need later in this seminar. A Type I error occurs when the null hypothesis is true (in other words, there really is no effect), but you reject the null hypothesis. A Type II error occurs when the alternative hypothesis is correct, but you fail to reject the null hypothesis (in other words, there really is an effect, but you failed to detect it). Alpha inflation refers to the increase in the nominal alpha level when the number of statistical tests conducted on a given data set is increased.
When discussing statistical power, we have four inter-related concepts: power, effect size, sample size and alpha. These four things are related such that each is a function of the other three. In other words, if three of these values are fixed, the fourth is completely determined (Cohen, 1988, page 14). We mention this because, by increasing one, you can decrease (or increase) another. For example, if you can increase your effect size, you will need fewer subjects, given the same power and alpha level. Specifically, increasing the effect size, the sample size and/or alpha will increase your power.
While we are thinking about these related concepts and the effect of increasing things, let’s take a quick look at a standard power graph. (This graph was made in SPSS Sample Power, and for this example, we’ve used .61 and 4 for our two proportion positive values.)
We like these kinds of graphs because they make clear the diminishing returns you get for adding more and more subjects. For example, let’s say that we have only 10 subjects per group. We can see that we have a power of about .15, which is really, really low. We add 50 subjects per group, now we have a power of about .6, an increase of .45. However, if we started with 100 subjects per group (power of about .8) and added 50 per group, we would have a power of .95, an increase of only .15. So each additional subject gives you less additional power. This curve also illustrates the “cost” of increasing your desired power from .8 to .9.
Knowing your research project
As we mentioned before, one of the big benefits of doing a power analysis is making sure that you have thought through every detail of your research project.
Now most researchers have thought through most, if not all, of the substantive issues involved in their research. While this is absolutely necessary, it often is not sufficient. Researchers also need to carefully consider all aspects of the experimental design, the variables involved, and the statistical analysis technique that will be used. As you will see in the next sections of this presentation, a power analysis is the union of substantive knowledge (i.e., knowledge about the subject matter), experimental or quasi-experimental design issues, and statistical analysis. Almost every aspect of the experimental design can affect power. For example, the type of control group that is used or the number of time points that are collected will affect how much power you have. So knowing about these issues and carefully considering your options is important. There are plenty of excellent books that cover these issues in detail, including Shadish, Cook and Campbell (2002); Cook and Campbell (1979); Campbell and Stanley (1963); Brickman (2000a, 2000b); Campbell and Russo (2001); Webb, Campbell, Schwartz and Sechrest (2000); and Anderson (2001).
Also, you want to know as much as possible about the statistical technique that you are going to use. If you learn that you need to use a binary logistic regression because your outcome variable is 0/1, don’t stop there; rather, get a sample data set (there are plenty of sample data sets on our web site) and try it out. You may discover that the statistical package that you use doesn’t do the type of analysis that need to do. For example, if you are an SPSS user and you need to do a weighted multilevel logistic regression, you will quickly discover that SPSS doesn’t do that (as of version 25), and you will have to find (and probably learn) another statistical package that will do that analysis. Maybe you want to learn another statistical package, or maybe that is beyond what you want to do for this project. If you are writing a grant proposal, maybe you will want to include funds for purchasing the new software. You will also want to learn what the assumptions are and what the “quirks” are with this particular type of analysis. Remember that the number of necessary subjects given to you by a power analysis assumes that all of the assumptions of the analysis have been met, so knowing what those assumptions are is important deciding if they are likely to be met or not.
The point of this section is to make clear that knowing your research project involves many things, and you may find that you need to do some research about experimental design or statistical techniques before you do your power analysis.
We want to emphasize that this is time and effort well spent. We also want to remind you that for almost all researchers, this is a normal part of doing good research. UCLA researchers are welcome and encouraged to come by walk-in consulting at this stage of the research process to discuss issues and ideas, check out books and try out software.
What you need to know to do a power analysis
In the previous section, we discussed in general terms what you need to know to do a power analysis. In this section we will discuss some of the actual quantities that you need to know to do a power analysis for some simple statistics. Although we understand very few researchers test their main hypothesis with a t-test or a chi-square test, our point here is only to give you a flavor of the types of things that you will need to know (or guess at) in order to be ready for a power analysis.
– For an independent samples t-test, you will need to know the population means of the two groups (or the difference between the means), and the population standard deviations of the two groups. So, using our example of drug A and placebo, we would need to know the difference in the means of the two groups, as well as the standard deviation for each group (because the group means and standard deviations are the best estimate that we have of those population values). Clearly, if we knew all of this, we wouldn’t need to conduct the study. In reality, researchers make educated guesses at these values. We always recommend that you use several different values, such as decreasing the difference in the means and increasing the standard deviations, so that you get a range of values for the number of necessary subjects.
In SPSS Sample Power, we would have a screen that looks like the one below, and we would fill in the necessary values. As we can see, we would need a total of 70 subjects (35 per group) to have a power of .91 if we had a mean of 5 and a standard deviation of 2.5 in the drug A group, and a mean of 3 and a standard deviation of 2.5 in the placebo group. If we decreased the difference in the means and increased the standard deviations such that for the drug A group, we had a mean of 4.5 and a standard deviation of 3, and for the placebo group a mean of 3.5 and a standard deviation of 3, we would need 190 subjects per group, or a total of 380 subjects, to have a power of .90. In other words, seemingly small differences in means and standard deviations can have a huge effect on the number of subjects required.
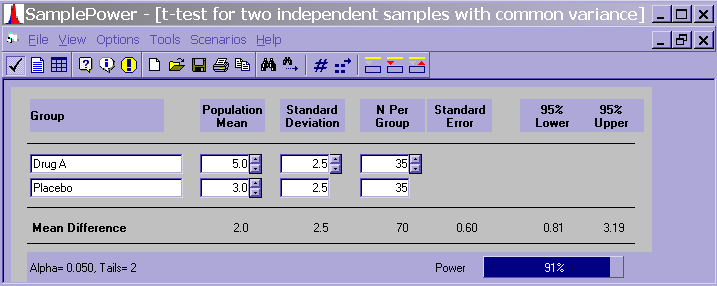
– For a correlation, you need to know/guess at the correlation in the population. This is a good time to remember back to an early stats class where they emphasized that correlation is a large N procedure (Chen and Popovich, 2002). If you guess that the population correlation is .6, a power analysis would suggest (with an alpha of .05 and for a power of .8) that you would need only 16 subjects. There are several points to be made here. First, common sense suggests that N = 16 is pretty low. Second, a population correlation of .6 is pretty high, especially in the social sciences. Third, the power analysis assumes that all of the assumptions of the correlation have been met. For example, we are assuming that there is no restriction of range issue, which is common with Likert scales; the sample data for both variables are normally distributed; the relationship between the two variables is linear; and there are no serious outliers. Also, whereas you might be able to say that the sample correlation does not equal zero, you likely will not have a very precise estimate of the population correlation coefficient.
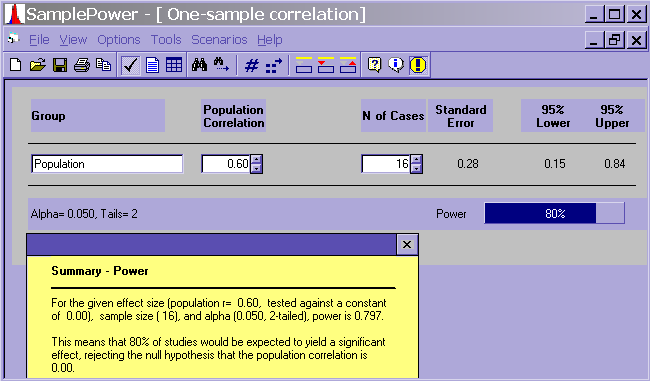
– For a chi-square test, you will need to know the proportion positive for both populations (i.e., rows and columns). Let’s assume that we will have a 2 x 2 chi-square, and let’s think of both variables as 0/1. Let’s say that we wanted to know if there was a relationship between drug group (drug A/placebo) and improved health. In SPSS Sample Power, you would see a screen like this.
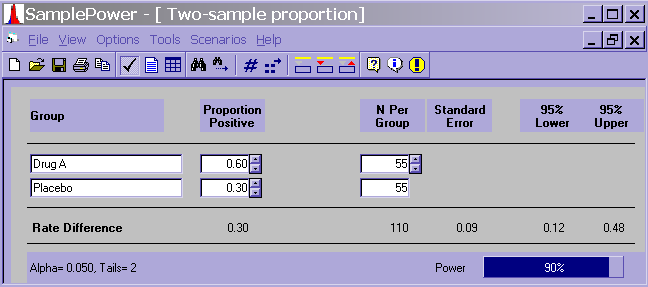
In order to get the .60 and the .30, we would need to know (or guess at) the number of people whose health improved in both the drug A and placebo groups.
We would also need to know (or guess at) either the number of people whose health did not improve in those two groups, or the total number of people in each group.
– For an ordinary least squares regression, you would need to know things like the R 2 for the full and reduced model. For a simple logistic regression analysis with only one continuous predictor variable, you would need to know the probability of a positive outcome (i.e., the probability that the outcome equals 1) at the mean of the predictor variable and the probability of a positive outcome at one standard deviation above the mean of the predictor variable. Especially for the various types of logistic models (e.g., binary, ordinal and multinomial), you will need to think very carefully about your sample size, and information from a power analysis will only be part of your considerations. For example, according to Long (1997, pages 53-54), 100 is a minimum sample size for logistic regression, and you want *at least* 10 observations per predictor. This does not mean that if you have only one predictor you need only 10 observations.
Also, if you have categorical predictors, you may need to have more observations to avoid computational difficulties caused by empty cells or cells with few observations. More observations are needed when the outcome variable is very lopsided; in other words, when there are very few 1s and lots of 0s, or vice versa. These cautions emphasize the need to know your data set well, so that you know if your outcome variable is lopsided or if you are likely to have a problem with empty cells.
The point of this section is to give you a sense of the level of detail about your variables that you need to be able to estimate in order to do a power analysis. Also, when doing power analyses for regression models, power programs will start to ask for values that most researchers are not accustomed to providing. Guessing at the mean and standard deviation of your response variable is one thing, but increments to R 2 is a metric in which few researchers are used to thinking. In our next section we will discuss how you can guestimate these numbers.
Obtaining the necessary numbers to do a power analysis
There are at least three ways to guestimate the values that are needed to do a power analysis: a literature review, a pilot study and using Cohen’s recommendations. We will review the pros and cons of each of these methods. For this discussion, we will focus on finding the effect size, as that is often the most difficult number to obtain and often has the strongest impact on power.
Literature review: Sometimes you can find one or more published studies that are similar enough to yours that you can get a idea of the effect size. If you can find several such studies, you might be able to use meta-analysis techniques to get a robust estimate of the effect size. However, oftentimes there are no studies similar enough to your study to get a good estimate of the effect size. Even if you can find such an study, the necessary effect sizes or other values are often not clearly stated in the article and need to be calculated (if they can) based on the information provided.
Pilot studies: There are lots of good reasons to do a pilot study prior to conducting the actual study. From a power analysis prospective, a pilot study can give you a rough estimate of the effect size, as well as a rough estimate of the variability in your measures. You can also get some idea about where missing data might occur, and as we will discuss later, how you handle missing data can greatly affect your power. Other benefits of a pilot study include allowing you to identify coding problems, setting up the data base, and inputting the data for a practice analysis. This will allow you to determine if the data are input in the correct shape, etc.
Of course, there are some limitations to the information that you can get from a pilot study. (Many of these limitations apply to small samples in general.) First of all, when estimating effect sizes based on nonsignificant results, the effect size estimate will necessarily have an increased error; in other words, the standard error of the effect size estimate will be larger than when the result is significant. The effect size estimate that you obtain may be unduly influenced by some peculiarity of the small sample. Also, you often cannot get a good idea of the degree of missingness and attrition that will be seen in the real study. Despite these limitations, we strongly encourage researchers to conduct a pilot study. The opportunity to identify and correct “bugs” before collecting the real data is often invaluable. Also, because of the number of values that need to be guestimated in a power analysis, the precision of any one of these values is not that important. If you can estimate the effect size to within 10% or 20% of the true value, that is probably sufficient for you to conduct a meaningful power analysis, and such fluctuations can be taken into account during the power analysis.
Cohen’s recommendations: Jacob Cohen has many well-known publications regarding issues of power and power analyses, including some recommendations about effect sizes that you can use when doing your power analysis. Many researchers (including Cohen) consider the use of such recommendations as a last resort, when a thorough literature review has failed to reveal any useful numbers and a pilot study is either not possible or not feasible. From Cohen (1988, pages 24-27):
– Small effect: 1% of the variance; d = 0.25 (too small to detect other than statistically; lower limit of what is clinically relevant)
– Medium effect: 6% of the variance; d = 0.5 (apparent with careful observation)
– Large effect: at least 15% of the variance; d = 0.8 (apparent with a superficial glance; unlikely to be the focus of research because it is too obvious)
Lipsey and Wilson (1993) did a meta analysis of 302 meta analyses of over 10,000 studies and found that the average effect size was .5, adding support to Cohen’s recommendation that, as a last resort, guess that the effect size is .5 (cited in Bausell and Li, 2002). Sedlmeier and Gigerenzer (1989) found that the average effect size for articles in The Journal of Abnormal Psychology was a medium effect. According to Keppel and Wickens (2004), when you really have no idea what the effect size is, go with the smallest effect size of practical value. In other words, you need to know how small of a difference is meaningful to you. Keep in mind that research suggests that most researchers are overly optimistic about the effect sizes in their research, and that most research studies are under powered (Keppel and Wickens, 2004; Tversky and Kahneman, 1971). This is part of the reason why we stress that a power analysis gives you a lower limit to the number of necessary subjects.
Factors that affect power
From the preceding discussion, you might be starting to think that the number of subjects and the effect size are the most important factors, or even the only factors, that affect power. Although effect size is often the largest contributor to power, saying it is the only important issue is far from the truth. There are at least a dozen other factors that can influence the power of a study, and many of these factors should be considered not only from the perspective of doing a power analysis, but also as part of doing good research. The first couple of factors that we will discuss are more “mechanical” ways of increasing power (e.g., alpha level, sample size and effect size). After that, the discussion will turn to more methodological issues that affect power.
1. Alpha level: One obvious way to increase your power is to increase your alpha (from .05 to say, .1). Whereas this might be an advisable strategy when doing a pilot study, increasing your alpha usually is not a viable option. We should point out here that many researchers are starting to prefer to use .01 as an alpha level instead of .05 as a crude attempt to assure results are clinically relevant; this alpha reduction reduces power.
1a. One- versus two-tailed tests: In some cases, you can test your hypothesis with a one-tailed test. For example, if your hypothesis was that drug A is better than the placebo, then you could use a one-tailed test. However, you would fail to detect a difference, even if it was a large difference, if the placebo was better than drug A. The advantage of one-tailed tests is that they put all of your power “on one side” to test your hypothesis. The disadvantage is that you cannot detect differences that are in the opposite direction of your hypothesis. Moreover, many grant and journal reviewers frown on the use of one-tailed tests, believing it is a way to feign significance (Stratton and Neil, 2004).
2. Sample size: A second obvious way to increase power is simply collect data on more subjects. In some situations, though, the subjects are difficult to get or extremely costly to run. For example, you may have access to only 20 autistic children or only have enough funding to interview 30 cancer survivors. If possible, you might try increasing the number of subjects in groups that do not have these restrictions, for example, if you are comparing to a group of normal controls. While it is true that, in general, it is often desirable to have roughly the same number of subjects in each group, this is not absolutely necessary. However, you get diminishing returns for additional subjects in the control group: adding an extra 100 subjects to the control group might not be much more helpful than adding 10 extra subjects to the control group.
3. Effect size: Another obvious way to increase your power is to increase the effect size. Of course, this is often easier said than done. A common way of increasing the effect size is to increase the experimental manipulation. Going back to our example of drug A and placebo, increasing the experimental manipulation might mean increasing the dose of the drug. While this might be a realistic option more often than increasing your alpha level, there are still plenty of times when you cannot do this. Perhaps the human subjects committee will not allow it, it does not make sense clinically, or it doesn’t allow you to generalize your results the way you want to. Many of the other issues discussed below indirectly increase effect size by providing a stronger research design or a more powerful statistical analysis.
4. Experimental task: Well, maybe you can not increase the experimental manipulation, but perhaps you can change the experimental task, if there is one. If a variety of tasks have been used in your research area, consider which of these tasks provides the most power (compared to other important issues, such as relevancy, participant discomfort, and the like). However, if various tasks have not been reviewed in your field, designing a more sensitive task might be beyond the scope of your research project.
5. Response variable: How you measure your response variable(s) is just as important as what task you have the subject perform. When thinking about power, you want to use a measure that is as high in sensitivity and low in measurement error as is possible. Researchers in the social sciences often have a variety of measures from which they can choose, while researchers in other fields may not. For example, there are numerous established measures of anxiety, IQ, attitudes, etc. Even if there are not established measures, you still have some choice. Do you want to use a Likert scale, and if so, how many points should it have? Modifications to procedures can also help reduce measurement error. For example, you want to make sure that each subject knows exactly what he or she is supposed to be rating. Oral instructions need to be clear, and items on questionnaires need to be unambiguous to all respondents. When possible, use direct instead of indirect measures. For example, asking people what tax bracket they are in is a more direct way of determining their annual income than asking them about the square footage of their house. Again, this point may be more applicable to those in the social sciences than those in other areas of research. We should also note that minimizing the measurement error in your predictor variables will also help increase your power.
Just as an aside, most texts on experimental design strongly suggest collecting more than one measure of the response in which you are interested. While this is very good methodologically and provides marked benefits for certain analyses and missing data, it does complicate the power analysis.
6. Experimental design: Another thing to consider is that some types of experimental designs are more powerful than others. For example, repeated measures designs are virtually always more powerful than designs in which you only get measurements at one time. If you are already using a repeated measures design, increasing the number of time points a response variable is collected to at least four or five will also provide increased power over fewer data collections. There is a point of diminishing return when a researcher collects too many time points, though this depends on many factors such as the response variable, statistical design, age of participants, etc.
7. Groups: Another point to consider is the number and types of groups that you are using. Reducing the number of experimental conditions will reduce the number of subjects that is needed, or you can keep the same number of subjects and just have more per group. When thinking about which groups to exclude from the design, you might want to leave out those in the middle and keep the groups with the more extreme manipulations. Going back to our drug A example, let’s say that we were originally thinking about having a total of four groups: the first group will be our placebo group, the second group would get a small dose of drug A, the third group a medium dose, and the fourth group a large dose. Clearly, much more power is needed to detect an effect between the medium and large dose groups than to detect an effect between the large dose group and the placebo group. If we found that we were unable to increase the power enough such that we were likely to find an effect between small and medium dose groups or between the medium and the large dose groups, then it would probably make more sense to run the study without these groups. In some cases, you may even be able to change your comparison group to something more extreme. For example, we once had a client who was designing a study to compare people with clinical levels of anxiety to a group that had subclinical levels of anxiety. However, while doing the power analysis and realizing how many subjects she would need to detect the effect, she found that she needed far fewer subjects if she compared the group with the clinical levels of anxiety to a group of “normal” people (a number of subjects she could reasonably obtain).
8. Statistical procedure: Changing the type of statistical analysis may also help increase power, especially when some of the assumptions of the test are violated. For example, as Maxwell and Delaney (2004) noted, “Even when ANOVA is robust, it may not provide the most powerful test available when its assumptions have been violated.” In particular, violations of assumptions regarding independence, normality and heterogeneity can reduce power. In such cases, nonparametric alternatives may be more powerful.
9. Statistical model: You can also modify the statistical model. For example, interactions often require more power than main effects. Hence, you might find that you have reasonable power for a main effects model, but not enough power when the model includes interactions. Many (perhaps most?) power analysis programs do not have an option to include interaction terms when describing the proposed analysis, so you need to keep this in mind when using these programs to help you determine how many subjects will be needed. When thinking about the statistical model, you might want to consider using covariates or blocking variables. Ideally, both covariates and blocking variables reduce the variability in the response variable. However, it can be challenging to find such variables. Moreover, your statistical model should use as many of the response variable time points as possible when examining longitudinal data. Using a change-score analysis when one has collected five time points makes little sense and ignores the added power from these additional time points. The more the statistical model “knows” about how a person changes over time, the more variance that can be pulled out of the error term and ascribed to an effect.
9a. Correlation between time points: Understanding the expected correlation between a response variable measured at one time in your study with the same response variable measured at another time can provide important and power-saving information. As noted previously, when the statistical model has a certain amount of information regarding the manner by which people change over time, it can enhance the effect size estimate. This is largely dependent on the correlation of the response measure over time. For example, in a before-after data collection scenario, response variables with a .00 correlation from before the treatment to after the treatment would provide no extra benefit to the statistical model, as we can’t better understand a subject’s score by knowing how he or she changes over time. Rarely, however, do variables have a .00 correlation on the same outcomes measured at different times. It is important to know that outcome variables with larger correlations over time provide enhanced power when used in a complimentary statistical model.
10. Modify response variable: Besides modifying your statistical model, you might also try modifying your response variable. Possible benefits of this strategy include reducing extreme scores and/or meeting the assumptions of the statistical procedure. For example, some response variables might need to be log transformed. However, you need to be careful here. Transforming variables often makes the results more difficult to interpret, because now you are working in, say, a logarithm metric instead of the metric in which the variable was originally measured. Moreover, if you use a transformation that adjusts the model too much, you can loose more power than is necessary. Categorizing continuous response variables (sometimes used as a way of handling extreme scores) can also be problematic, because logistic or ordinal logistic regression often requires many more subjects than does OLS regression. It makes sense that categorizing a response variable will lead to a loss of power, as information is being “thrown away.”
11. Purpose of the study: Different researchers have different reasons for conducting research. Some are trying to determine if a coefficient (such as a regression coefficient) is different from zero. Others are trying to get a precise estimate of a coefficient. Still others are replicating research that has already been done. The purpose of the research can affect the necessary sample size. Going back to our drug A and placebo study, let’s suppose our purpose is to test the difference in means to see if it equals zero. In this case, we need a relatively small sample size. If our purpose is to get a precise estimate of the means (i.e., minimizing the standard errors), then we will need a larger sample size. If our purpose is to replicate previous research, then again we will need a relatively large sample size. Tversky and Kahneman (1971) pointed out that we often need more subjects in a replication study than were in the original study. They also noted that researchers are often too optimistic about how much power they really have. They claim that researchers too readily assign “causal” reasons to explain differences between studies, instead of sampling error. They also mentioned that researchers tend to underestimate the impact of sampling and think that results will replicate more often than is the case.
12. Missing data: A final point that we would like to make here regards missing data. Almost all researchers have issues with missing data. When designing your study and selecting your measures, you want to do everything possible to minimize missing data. Handling missing data via imputation methods can be very tricky and very time-consuming. If the data set is small, the situation can be even more difficult. In general, missing data reduces power; poor imputation methods can greatly reduce power. If you have to impute, you want to have as few missing data points on as few variables as possible. When designing the study, you might want to collect data specifically for use in an imputation model (which usually involves a different set of variables than the model used to test your hypothesis). It is also important to note that the default technique for handling missing data by virtually every statistical program is to remove the entire case from an analysis (i.e., listwise deletion). This process is undertaken even if the analysis involves 20 variables and a subject is missing only one datum of the 20. Listwise deletion is one of the biggest contributors to loss of power, both because of the omnipresence of missing data and because of the omnipresence of this default setting in statistical programs (Graham et al., 2003).
This ends the section on the various factors that can influence power. We know that was a lot, and we understand that much of this can be frustrating because there is very little that is “black and white”. We hope that this section made clear the close relationship between the experimental design, the statistical analysis and power.
Cautions about small sample sizes and sampling variation
We want to take a moment here to mention some issues that frequently arise when using small samples. (We aren’t going to put a lower limit on what we mean be “small sample size.”) While there are situations in which a researcher can either only get or afford a small number of subjects, in most cases, the researcher has some choice in how many subjects to include. Considerations of time and effort argue for running as few subjects as possible, but there are some difficulties associated with small sample sizes, and these may outweigh any gains from the saving of time, effort or both. One obvious problem with small sample sizes is that they have low power. This means that you need to have a large effect size to detect anything. You will also have fewer options with respect to appropriate statistical procedures, as many common procedures, such as correlations, logistic regression and multilevel modeling, are not appropriate with small sample sizes. It may also be more difficult to evaluate the assumptions of the statistical procedure that is used (especially assumptions like normality). In most cases, the statistical model must be smaller when the data set is small. Interaction terms, which often test interesting hypotheses, are frequently the first casualties. Generalizability of the results may also be comprised, and it can be difficult to argue that a small sample is representative of a large and varied population. Missing data are also more problematic; there are a reduced number of imputations methods available to you, and these are not considered to be desirable imputation methods (such as mean imputation). Finally, with a small sample size, alpha inflation issues can be more difficult to address, and you are more likely to run as many tests as you have subjects.
While the issue of sampling variability is relevant to all research, it is especially relevant to studies with small sample sizes. To quote Murphy and Myors (2004, page 59), “The lack of attention to power analysis (and the deplorable habit of placing too much weight on the results of small sample studies) are well documented in the literature, and there is no good excuse to ignore power in designing studies.” In an early article entitled The Law of Small Numbers , Tversky and Kahneman (1971) stated that many researchers act like the Law of Large Numbers applies to small numbers. People often believe that small samples are more representative of the population than they really are.
The last two points to be made here is that there is usually no point to conducting an underpowered study, and that underpowered studies can cause chaos in the literature because studies that are similar methodologically may report conflicting results.
We will briefly discuss some of the programs that you can use to assist you with your power analysis. Most programs are fairly easy to use, but you still need to know effect sizes, means, standard deviations, etc.
Among the programs specifically designed for power analysis, we use SPSS Sample Power, PASS and GPower. These programs have a friendly point-and-click interface and will do power analyses for things like correlations, OLS regression and logistic regression. We have also started using Optimal Design for repeated measures, longitudinal and multilevel designs. We should note that Sample Power is a stand-alone program that is sold by SPSS; it is not part of SPSS Base or an add-on module. PASS can be purchased directly from NCSS at http://www.ncss.com/index.htm . GPower (please see GPower for details) and Optimal Design (please see http://sitemaker.umich.edu/group-based/home for details) are free.
Several general use stat packages also have procedures for calculating power. SAS has proc power , which has a lot of features and is pretty nice. Stata has the sampsi command, as well as many user-written commands, including fpower , powerreg and aipe (written by our IDRE statistical consultants). Statistica has an add-on module for power analysis. There are also many programs online that are free.
For more advanced/complicated analyses, Mplus is a good choice. It will allow you to do Monte Carlo simulations, and there are some examples at http://www.statmodel.com/power.shtml and http://www.statmodel.com/ugexcerpts.shtml .
Most of the programs that we have mentioned do roughly the same things, so when selecting a power analysis program, the real issue is your comfort; all of the programs require you to provide the same kind of information.
Multiplicity
This issue of multiplicity arises when a researcher has more than one outcome of interest in a given study. While it is often good methodological practice to have more than one measure of the response variable of interest, additional response variables mean more statistical tests need to be conducted on the data set, and this leads to question of experimentwise alpha control. Returning to our example of drug A and placebo, if we have only one response variable, then only one t test is needed to test our hypothesis. However, if we have three measures of our response variable, we would want to do three t tests, hoping that each would show results in the same direction. The question is how to control the Type I error (AKA false alarm) rate. Most researchers are familiar with Bonferroni correction, which calls for dividing the prespecified alpha level (usually .05) by the number of tests to be conducted. In our example, we would have .05/3 = .0167. Hence, .0167 would be our new critical alpha level, and statistics with a p-value greater than .0167 would be classified as not statistically significant. It is well-known that the Bonferroni correction is very conservative; there are other ways of adjusting the alpha level.
Afterthoughts: A post-hoc power analysis
In general, just say “No!” to post-hoc analyses. There are many reasons, both mechanical and theoretical, why most researchers should not do post-hoc power analyses. Excellent summaries can be found in Hoenig and Heisey (2001) The Abuse of Power: The Pervasive Fallacy of Power Calculations for Data Analysis and Levine and Ensom (2001) Post Hoc Power Analysis: An Idea Whose Time Has Passed? . As Hoenig and Heisey show, power is mathematically directly related to the p-value; hence, calculating power once you know the p-value associated with a statistic adds no new information. Furthermore, as Levine and Ensom clearly explain, the logic underlying post-hoc power analysis is fundamentally flawed.
However, there are some things that you should look at after your study is completed. Have a look at the means and standard deviations of your variables and see how close they are (or are not) from the values that you used in the power analysis. Many researchers do a series of related studies, and this information can aid in making decisions in future research. For example, if you find that your outcome variable had a standard deviation of 7, and in your power analysis you were guessing it would have a standard deviation of 2, you may want to consider using a different measure that has less variance in your next study.
The point here is that in addition to answering your research question(s), your current research project can also assist with your next power analysis.
Conclusions
Conducting research is kind of like buying a car. While buying a car isn’t the biggest purchase that you will make in your life, few of us enter into the process lightly. Rather, we consider a variety of things, such as need and cost, before making a purchase. You would do your research before you went and bought a car, because once you drove the car off the dealer’s lot, there is nothing you can do about it if you realize this isn’t the car that you need. Choosing the type of analysis is like choosing which kind of car to buy. The number of subjects is like your budget, and the model is like your expenses. You would never go buy a car without first having some idea about what the payments will be. This is like doing a power analysis to determine approximately how many subjects will be needed. Imagine signing the papers for your new Maserati only to find that the payments will be twice your monthly take-home pay. This is like wanting to do a multilevel model with a binary outcome, 10 predictors and lots of cross-level interactions and realizing that you can’t do this with only 50 subjects. You don’t have enough “currency” to run that kind of model. You need to find a model that is “more in your price range.” If you had $530 a month budgeted for your new car, you probably wouldn’t want exactly $530 in monthly payments. Rather you would want some “wiggle-room” in case something cost a little more than anticipated or you were running a little short on money that month. Likewise, if your power analysis says you need about 300 subjects, you wouldn’t want to collect data on exactly 300 subjects. You would want to collect data on 300 subjects plus a few, just to give yourself some “wiggle-room” just in case.
Don’t be afraid of what you don’t know. Get in there and try it BEFORE you collect your data. Correcting things is easy at this stage; after you collect your data, all you can do is damage control. If you are in a hurry to get a project done, perhaps the worst thing that you can do is start collecting data now and worry about the rest later. The project will take much longer if you do this than if you do what we are suggesting and do the power analysis and other planning steps. If you have everything all planned out, things will go much smoother and you will have fewer and/or less intense panic attacks. Of course, some thing unexpected will always happen, but it is unlikely to be as big of a problem. UCLA researchers are always welcome and strongly encouraged to come into our walk-in consulting and discuss their research before they begin the project.
Power analysis = planning. You will want to plan not only for the test of your main hypothesis, but also for follow-up tests and tests of secondary hypotheses. You will want to make sure that “confirmation” checks will run as planned (for example, checking to see that interrater reliability was acceptable). If you intend to use imputation methods to address missing data issues, you will need to become familiar with the issues surrounding the particular procedure as well as including any additional variables in your data collection procedures. Part of your planning should also include a list of the statistical tests that you intend to run and consideration of any procedure to address alpha inflation issues that might be necessary.
The number output by any power analysis program is often just a starting point of thought more than a final answer to the question of how many subjects will be needed. As we have seen, you also need to consider the purpose of the study (coefficient different from 0, precise point estimate, replication), the type of statistical test that will be used (t-test versus maximum likelihood technique), the total number of statistical tests that will be performed on the data set, genearlizability from the sample to the population, and probably several other things as well.
The take-home message from this seminar is “do your research before you do your research.”
Anderson, N. H. (2001). Empirical Direction in Design and Analysis. Mahwah, New Jersey: Lawrence Erlbaum Associates.
Bausell, R. B. and Li, Y. (2002). Power Analysis for Experimental Research: A Practical Guide for the Biological, Medical and Social Sciences. Cambridge University Press, New York, New York.
Bickman, L., Editor. (2000). Research Design: Donald Campbell’s Legacy, Volume 2. Thousand Oaks, CA: Sage Publications.
Bickman, L., Editor. (2000). Validity and Social Experimentation. Thousand Oaks, CA: Sage Publications.
Campbell, D. T. and Russo, M. J. (2001). Social Measurement. Thousand Oaks, CA: Sage Publications.
Campbell, D. T. and Stanley, J. C. (1963). Experimental and Quasi-experimental Designs for Research. Reprinted from Handbook of Research on Teaching . Palo Alto, CA: Houghton Mifflin Co.
Chen, P. and Popovich, P. M. (2002). Correlation: Parametric and Nonparametric Measures. Thousand Oaks, CA: Sage Publications.
Cohen, J. (1988). Statistical Power Analysis for the Behavioral Sciences, Second Edition. Hillsdale, New Jersey: Lawrence Erlbaum Associates.
Cook, T. D. and Campbell, D. T. Quasi-experimentation: Design and Analysis Issues for Field Settings. (1979). Palo Alto, CA: Houghton Mifflin Co.
Graham, J. W., Cumsille, P. E., and Elek-Fisk, E. (2003). Methods for handling missing data. In J. A. Schinka and W. F. Velicer (Eds.), Handbook of psychology (Vol. 2, pp. 87-114). New York: Wiley.
Green, S. B. (1991). How many subjects does it take to do a regression analysis? Multivariate Behavioral Research, 26(3) , 499-510.
Hoenig, J. M. and Heisey, D. M. (2001). The Abuse of Power: The Pervasive Fallacy of Power Calculations for Data Analysis. The American Statistician, 55(1) , 19-24.
Kelley, K and Maxwell, S. E. (2003). Sample size for multiple regression: Obtaining regression coefficients that are accurate, not simply significant. Psychological Methods, 8(3) , 305-321.
Keppel, G. and Wickens, T. D. (2004). Design and Analysis: A Researcher’s Handbook, Fourth Edition. Pearson Prentice Hall: Upper Saddle River, New Jersey.
Kline, R. B. Beyond Significance (2004). Beyond Significance Testing: Reforming Data Analysis Methods in Behavioral Research. American Psychological Association: Washington, D.C.
Levine, M., and Ensom M. H. H. (2001). Post Hoc Power Analysis: An Idea Whose Time Has Passed? Pharmacotherapy, 21(4) , 405-409.
Lipsey, M. W. and Wilson, D. B. (1993). The Efficacy of Psychological, Educational, and Behavioral Treatment: Confirmation from Meta-analysis. American Psychologist, 48(12) , 1181-1209.
Long, J. S. (1997). Regression Models for Categorical and Limited Dependent Variables. Thousand Oaks, CA: Sage Publications.
Maxwell, S. E. (2000). Sample size and multiple regression analysis. Psychological Methods, 5(4) , 434-458.
Maxwell, S. E. and Delany, H. D. (2004). Designing Experiments and Analyzing Data: A Model Comparison Perspective, Second Edition. Lawrence Erlbaum Associates, Mahwah, New Jersey.
Murphy, K. R. and Myors, B. (2004). Statistical Power Analysis: A Simple and General Model for Traditional and Modern Hypothesis Tests. Mahwah, New Jersey: Lawrence Erlbaum Associates.
Publication Manual of the American Psychological Association, Fifth Edition. (2001). Washington, D.C.: American Psychological Association.
Sedlmeier, P. and Gigerenzer, G. (1989). Do Studies of Statistical Power Have an Effect on the Power of Studies? Psychological Bulletin, 105(2) , 309-316.
Shadish, W. R., Cook, T. D. and Campbell, D. T. (2002). Experimental and Quasi-experimental Designs for Generalized Causal Inference. Boston: Houghton Mifflin Co.
Stratton, I. M. and Neil, A. (2004). How to ensure your paper is rejected by the statistical reviewer. Diabetic Medicine , 22, 371-373.
Tversky, A. and Kahneman, D. (1971). Belief in the Law of Small Numbers. Psychological Bulletin, 76(23) , 105-110.
Webb, E., Campbell, D. T., Schwartz, R. D., and Sechrest, L. (2000). Unobtrusive Measures, Revised Edition. Thousand Oaks, CA: Sage Publications.
Your Name (required)
Your Email (must be a valid email for us to receive the report!)
Comment/Error Report (required)
How to cite this page
- © 2021 UC REGENTS
Harvey Cushing/John Hay Whitney Medical Library
- Collections
- Research Help
YSN Doctoral Programs: Steps in Conducting a Literature Review
- Biomedical Databases
- Global (Public Health) Databases
- Soc. Sci., History, and Law Databases
- Grey Literature
- Trials Registers
- Data and Statistics
- Public Policy
- Google Tips
- Recommended Books
- Steps in Conducting a Literature Review
What is a literature review?
A literature review is an integrated analysis -- not just a summary-- of scholarly writings and other relevant evidence related directly to your research question. That is, it represents a synthesis of the evidence that provides background information on your topic and shows a association between the evidence and your research question.
A literature review may be a stand alone work or the introduction to a larger research paper, depending on the assignment. Rely heavily on the guidelines your instructor has given you.
Why is it important?
A literature review is important because it:
- Explains the background of research on a topic.
- Demonstrates why a topic is significant to a subject area.
- Discovers relationships between research studies/ideas.
- Identifies major themes, concepts, and researchers on a topic.
- Identifies critical gaps and points of disagreement.
- Discusses further research questions that logically come out of the previous studies.
APA7 Style resources
APA Style Blog - for those harder to find answers
1. Choose a topic. Define your research question.
Your literature review should be guided by your central research question. The literature represents background and research developments related to a specific research question, interpreted and analyzed by you in a synthesized way.
- Make sure your research question is not too broad or too narrow. Is it manageable?
- Begin writing down terms that are related to your question. These will be useful for searches later.
- If you have the opportunity, discuss your topic with your professor and your class mates.
2. Decide on the scope of your review
How many studies do you need to look at? How comprehensive should it be? How many years should it cover?
- This may depend on your assignment. How many sources does the assignment require?
3. Select the databases you will use to conduct your searches.
Make a list of the databases you will search.
Where to find databases:
- use the tabs on this guide
- Find other databases in the Nursing Information Resources web page
- More on the Medical Library web page
- ... and more on the Yale University Library web page
4. Conduct your searches to find the evidence. Keep track of your searches.
- Use the key words in your question, as well as synonyms for those words, as terms in your search. Use the database tutorials for help.
- Save the searches in the databases. This saves time when you want to redo, or modify, the searches. It is also helpful to use as a guide is the searches are not finding any useful results.
- Review the abstracts of research studies carefully. This will save you time.
- Use the bibliographies and references of research studies you find to locate others.
- Check with your professor, or a subject expert in the field, if you are missing any key works in the field.
- Ask your librarian for help at any time.
- Use a citation manager, such as EndNote as the repository for your citations. See the EndNote tutorials for help.
Review the literature
Some questions to help you analyze the research:
- What was the research question of the study you are reviewing? What were the authors trying to discover?
- Was the research funded by a source that could influence the findings?
- What were the research methodologies? Analyze its literature review, the samples and variables used, the results, and the conclusions.
- Does the research seem to be complete? Could it have been conducted more soundly? What further questions does it raise?
- If there are conflicting studies, why do you think that is?
- How are the authors viewed in the field? Has this study been cited? If so, how has it been analyzed?
Tips:
- Review the abstracts carefully.
- Keep careful notes so that you may track your thought processes during the research process.
- Create a matrix of the studies for easy analysis, and synthesis, across all of the studies.
- << Previous: Recommended Books
- Last Updated: Jan 4, 2024 10:52 AM
- URL: https://guides.library.yale.edu/YSNDoctoral
4.0 Inputs for Power Analysis: Literature Review
In this lesson, we will talk about what inputs are needed for power and sample size analysis and discuss how to find these inputs through a literature review process. Some questions to consider while completing this lesson include:
- How many key inputs are there for power or sample size analysis?
- Do you need to specify standard deviations and correlations between measures to compute power or sample size analysis?
Learning Objectives
- Identify the inputs for power or sample size analysis.
- Describe how to search the literature for inputs.
Video Tutorial
Lecture Notes: 4.0 Inputs for Power Analysis: Literature Review
Purdue Online Writing Lab Purdue OWL® College of Liberal Arts
Writing a Literature Review
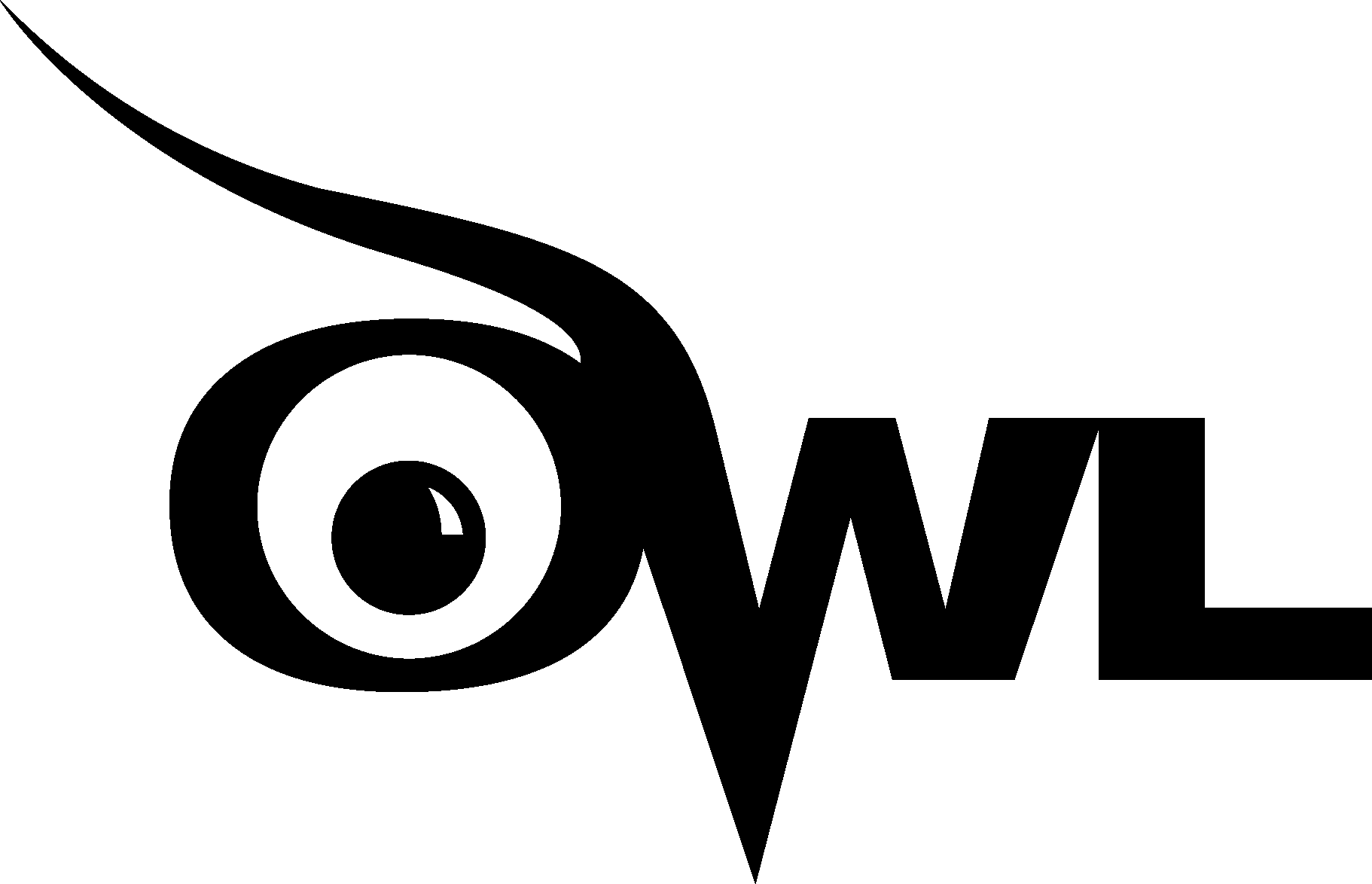
Welcome to the Purdue OWL
This page is brought to you by the OWL at Purdue University. When printing this page, you must include the entire legal notice.
Copyright ©1995-2018 by The Writing Lab & The OWL at Purdue and Purdue University. All rights reserved. This material may not be published, reproduced, broadcast, rewritten, or redistributed without permission. Use of this site constitutes acceptance of our terms and conditions of fair use.
A literature review is a document or section of a document that collects key sources on a topic and discusses those sources in conversation with each other (also called synthesis ). The lit review is an important genre in many disciplines, not just literature (i.e., the study of works of literature such as novels and plays). When we say “literature review” or refer to “the literature,” we are talking about the research ( scholarship ) in a given field. You will often see the terms “the research,” “the scholarship,” and “the literature” used mostly interchangeably.
Where, when, and why would I write a lit review?
There are a number of different situations where you might write a literature review, each with slightly different expectations; different disciplines, too, have field-specific expectations for what a literature review is and does. For instance, in the humanities, authors might include more overt argumentation and interpretation of source material in their literature reviews, whereas in the sciences, authors are more likely to report study designs and results in their literature reviews; these differences reflect these disciplines’ purposes and conventions in scholarship. You should always look at examples from your own discipline and talk to professors or mentors in your field to be sure you understand your discipline’s conventions, for literature reviews as well as for any other genre.
A literature review can be a part of a research paper or scholarly article, usually falling after the introduction and before the research methods sections. In these cases, the lit review just needs to cover scholarship that is important to the issue you are writing about; sometimes it will also cover key sources that informed your research methodology.
Lit reviews can also be standalone pieces, either as assignments in a class or as publications. In a class, a lit review may be assigned to help students familiarize themselves with a topic and with scholarship in their field, get an idea of the other researchers working on the topic they’re interested in, find gaps in existing research in order to propose new projects, and/or develop a theoretical framework and methodology for later research. As a publication, a lit review usually is meant to help make other scholars’ lives easier by collecting and summarizing, synthesizing, and analyzing existing research on a topic. This can be especially helpful for students or scholars getting into a new research area, or for directing an entire community of scholars toward questions that have not yet been answered.
What are the parts of a lit review?
Most lit reviews use a basic introduction-body-conclusion structure; if your lit review is part of a larger paper, the introduction and conclusion pieces may be just a few sentences while you focus most of your attention on the body. If your lit review is a standalone piece, the introduction and conclusion take up more space and give you a place to discuss your goals, research methods, and conclusions separately from where you discuss the literature itself.
Introduction:
- An introductory paragraph that explains what your working topic and thesis is
- A forecast of key topics or texts that will appear in the review
- Potentially, a description of how you found sources and how you analyzed them for inclusion and discussion in the review (more often found in published, standalone literature reviews than in lit review sections in an article or research paper)
- Summarize and synthesize: Give an overview of the main points of each source and combine them into a coherent whole
- Analyze and interpret: Don’t just paraphrase other researchers – add your own interpretations where possible, discussing the significance of findings in relation to the literature as a whole
- Critically Evaluate: Mention the strengths and weaknesses of your sources
- Write in well-structured paragraphs: Use transition words and topic sentence to draw connections, comparisons, and contrasts.
Conclusion:
- Summarize the key findings you have taken from the literature and emphasize their significance
- Connect it back to your primary research question
How should I organize my lit review?
Lit reviews can take many different organizational patterns depending on what you are trying to accomplish with the review. Here are some examples:
- Chronological : The simplest approach is to trace the development of the topic over time, which helps familiarize the audience with the topic (for instance if you are introducing something that is not commonly known in your field). If you choose this strategy, be careful to avoid simply listing and summarizing sources in order. Try to analyze the patterns, turning points, and key debates that have shaped the direction of the field. Give your interpretation of how and why certain developments occurred (as mentioned previously, this may not be appropriate in your discipline — check with a teacher or mentor if you’re unsure).
- Thematic : If you have found some recurring central themes that you will continue working with throughout your piece, you can organize your literature review into subsections that address different aspects of the topic. For example, if you are reviewing literature about women and religion, key themes can include the role of women in churches and the religious attitude towards women.
- Qualitative versus quantitative research
- Empirical versus theoretical scholarship
- Divide the research by sociological, historical, or cultural sources
- Theoretical : In many humanities articles, the literature review is the foundation for the theoretical framework. You can use it to discuss various theories, models, and definitions of key concepts. You can argue for the relevance of a specific theoretical approach or combine various theorical concepts to create a framework for your research.
What are some strategies or tips I can use while writing my lit review?
Any lit review is only as good as the research it discusses; make sure your sources are well-chosen and your research is thorough. Don’t be afraid to do more research if you discover a new thread as you’re writing. More info on the research process is available in our "Conducting Research" resources .
As you’re doing your research, create an annotated bibliography ( see our page on the this type of document ). Much of the information used in an annotated bibliography can be used also in a literature review, so you’ll be not only partially drafting your lit review as you research, but also developing your sense of the larger conversation going on among scholars, professionals, and any other stakeholders in your topic.
Usually you will need to synthesize research rather than just summarizing it. This means drawing connections between sources to create a picture of the scholarly conversation on a topic over time. Many student writers struggle to synthesize because they feel they don’t have anything to add to the scholars they are citing; here are some strategies to help you:
- It often helps to remember that the point of these kinds of syntheses is to show your readers how you understand your research, to help them read the rest of your paper.
- Writing teachers often say synthesis is like hosting a dinner party: imagine all your sources are together in a room, discussing your topic. What are they saying to each other?
- Look at the in-text citations in each paragraph. Are you citing just one source for each paragraph? This usually indicates summary only. When you have multiple sources cited in a paragraph, you are more likely to be synthesizing them (not always, but often
- Read more about synthesis here.
The most interesting literature reviews are often written as arguments (again, as mentioned at the beginning of the page, this is discipline-specific and doesn’t work for all situations). Often, the literature review is where you can establish your research as filling a particular gap or as relevant in a particular way. You have some chance to do this in your introduction in an article, but the literature review section gives a more extended opportunity to establish the conversation in the way you would like your readers to see it. You can choose the intellectual lineage you would like to be part of and whose definitions matter most to your thinking (mostly humanities-specific, but this goes for sciences as well). In addressing these points, you argue for your place in the conversation, which tends to make the lit review more compelling than a simple reporting of other sources.
Get science-backed answers as you write with Paperpal's Research feature
What is a Literature Review? How to Write It (with Examples)
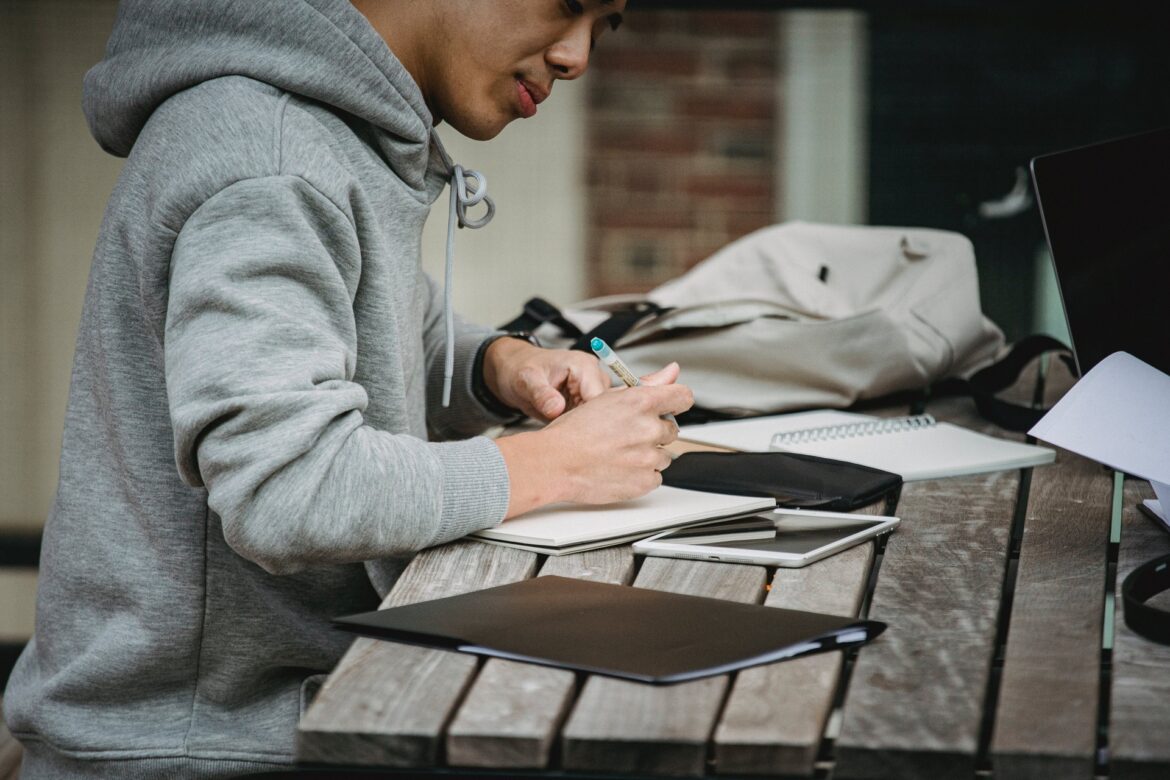
A literature review is a critical analysis and synthesis of existing research on a particular topic. It provides an overview of the current state of knowledge, identifies gaps, and highlights key findings in the literature. 1 The purpose of a literature review is to situate your own research within the context of existing scholarship, demonstrating your understanding of the topic and showing how your work contributes to the ongoing conversation in the field. Learning how to write a literature review is a critical tool for successful research. Your ability to summarize and synthesize prior research pertaining to a certain topic demonstrates your grasp on the topic of study, and assists in the learning process.
Table of Contents
- What is the purpose of literature review?
- a. Habitat Loss and Species Extinction:
- b. Range Shifts and Phenological Changes:
- c. Ocean Acidification and Coral Reefs:
- d. Adaptive Strategies and Conservation Efforts:
- How to write a good literature review
- Choose a Topic and Define the Research Question:
- Decide on the Scope of Your Review:
- Select Databases for Searches:
- Conduct Searches and Keep Track:
- Review the Literature:
- Organize and Write Your Literature Review:
- Frequently asked questions
What is a literature review?
A well-conducted literature review demonstrates the researcher’s familiarity with the existing literature, establishes the context for their own research, and contributes to scholarly conversations on the topic. One of the purposes of a literature review is also to help researchers avoid duplicating previous work and ensure that their research is informed by and builds upon the existing body of knowledge.
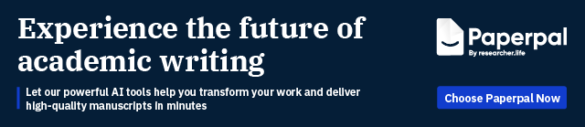
What is the purpose of literature review?
A literature review serves several important purposes within academic and research contexts. Here are some key objectives and functions of a literature review: 2
- Contextualizing the Research Problem: The literature review provides a background and context for the research problem under investigation. It helps to situate the study within the existing body of knowledge.
- Identifying Gaps in Knowledge: By identifying gaps, contradictions, or areas requiring further research, the researcher can shape the research question and justify the significance of the study. This is crucial for ensuring that the new research contributes something novel to the field.
- Understanding Theoretical and Conceptual Frameworks: Literature reviews help researchers gain an understanding of the theoretical and conceptual frameworks used in previous studies. This aids in the development of a theoretical framework for the current research.
- Providing Methodological Insights: Another purpose of literature reviews is that it allows researchers to learn about the methodologies employed in previous studies. This can help in choosing appropriate research methods for the current study and avoiding pitfalls that others may have encountered.
- Establishing Credibility: A well-conducted literature review demonstrates the researcher’s familiarity with existing scholarship, establishing their credibility and expertise in the field. It also helps in building a solid foundation for the new research.
- Informing Hypotheses or Research Questions: The literature review guides the formulation of hypotheses or research questions by highlighting relevant findings and areas of uncertainty in existing literature.
Literature review example
Let’s delve deeper with a literature review example: Let’s say your literature review is about the impact of climate change on biodiversity. You might format your literature review into sections such as the effects of climate change on habitat loss and species extinction, phenological changes, and marine biodiversity. Each section would then summarize and analyze relevant studies in those areas, highlighting key findings and identifying gaps in the research. The review would conclude by emphasizing the need for further research on specific aspects of the relationship between climate change and biodiversity. The following literature review template provides a glimpse into the recommended literature review structure and content, demonstrating how research findings are organized around specific themes within a broader topic.
Literature Review on Climate Change Impacts on Biodiversity:
Climate change is a global phenomenon with far-reaching consequences, including significant impacts on biodiversity. This literature review synthesizes key findings from various studies:
a. Habitat Loss and Species Extinction:
Climate change-induced alterations in temperature and precipitation patterns contribute to habitat loss, affecting numerous species (Thomas et al., 2004). The review discusses how these changes increase the risk of extinction, particularly for species with specific habitat requirements.
b. Range Shifts and Phenological Changes:
Observations of range shifts and changes in the timing of biological events (phenology) are documented in response to changing climatic conditions (Parmesan & Yohe, 2003). These shifts affect ecosystems and may lead to mismatches between species and their resources.
c. Ocean Acidification and Coral Reefs:
The review explores the impact of climate change on marine biodiversity, emphasizing ocean acidification’s threat to coral reefs (Hoegh-Guldberg et al., 2007). Changes in pH levels negatively affect coral calcification, disrupting the delicate balance of marine ecosystems.

d. Adaptive Strategies and Conservation Efforts:
Recognizing the urgency of the situation, the literature review discusses various adaptive strategies adopted by species and conservation efforts aimed at mitigating the impacts of climate change on biodiversity (Hannah et al., 2007). It emphasizes the importance of interdisciplinary approaches for effective conservation planning.
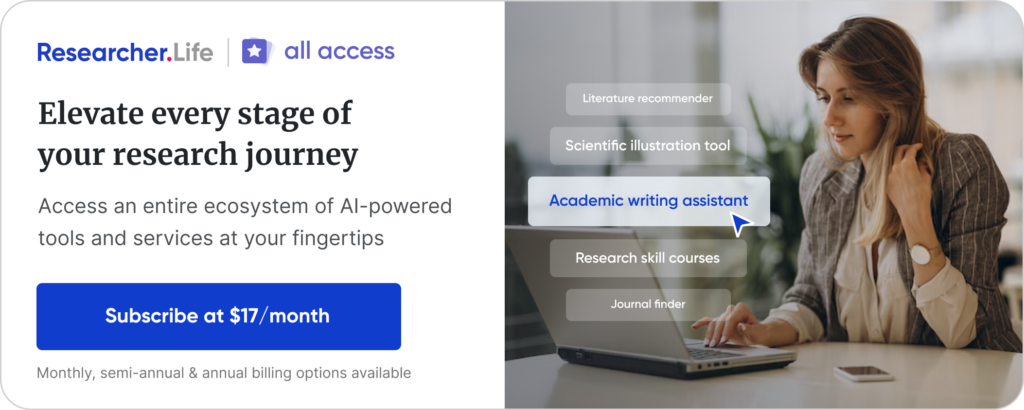
How to write a good literature review
Writing a literature review involves summarizing and synthesizing existing research on a particular topic. A good literature review format should include the following elements.
Introduction: The introduction sets the stage for your literature review, providing context and introducing the main focus of your review.
- Opening Statement: Begin with a general statement about the broader topic and its significance in the field.
- Scope and Purpose: Clearly define the scope of your literature review. Explain the specific research question or objective you aim to address.
- Organizational Framework: Briefly outline the structure of your literature review, indicating how you will categorize and discuss the existing research.
- Significance of the Study: Highlight why your literature review is important and how it contributes to the understanding of the chosen topic.
- Thesis Statement: Conclude the introduction with a concise thesis statement that outlines the main argument or perspective you will develop in the body of the literature review.
Body: The body of the literature review is where you provide a comprehensive analysis of existing literature, grouping studies based on themes, methodologies, or other relevant criteria.
- Organize by Theme or Concept: Group studies that share common themes, concepts, or methodologies. Discuss each theme or concept in detail, summarizing key findings and identifying gaps or areas of disagreement.
- Critical Analysis: Evaluate the strengths and weaknesses of each study. Discuss the methodologies used, the quality of evidence, and the overall contribution of each work to the understanding of the topic.
- Synthesis of Findings: Synthesize the information from different studies to highlight trends, patterns, or areas of consensus in the literature.
- Identification of Gaps: Discuss any gaps or limitations in the existing research and explain how your review contributes to filling these gaps.
- Transition between Sections: Provide smooth transitions between different themes or concepts to maintain the flow of your literature review.
Conclusion: The conclusion of your literature review should summarize the main findings, highlight the contributions of the review, and suggest avenues for future research.
- Summary of Key Findings: Recap the main findings from the literature and restate how they contribute to your research question or objective.
- Contributions to the Field: Discuss the overall contribution of your literature review to the existing knowledge in the field.
- Implications and Applications: Explore the practical implications of the findings and suggest how they might impact future research or practice.
- Recommendations for Future Research: Identify areas that require further investigation and propose potential directions for future research in the field.
- Final Thoughts: Conclude with a final reflection on the importance of your literature review and its relevance to the broader academic community.
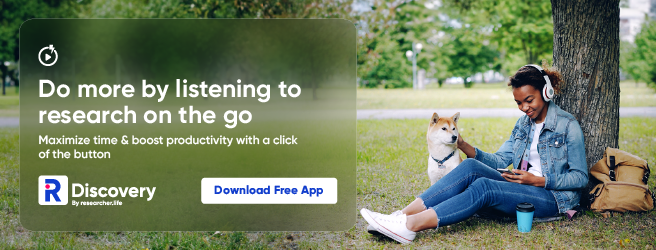
Conducting a literature review
Conducting a literature review is an essential step in research that involves reviewing and analyzing existing literature on a specific topic. It’s important to know how to do a literature review effectively, so here are the steps to follow: 1
Choose a Topic and Define the Research Question:
- Select a topic that is relevant to your field of study.
- Clearly define your research question or objective. Determine what specific aspect of the topic do you want to explore?
Decide on the Scope of Your Review:
- Determine the timeframe for your literature review. Are you focusing on recent developments, or do you want a historical overview?
- Consider the geographical scope. Is your review global, or are you focusing on a specific region?
- Define the inclusion and exclusion criteria. What types of sources will you include? Are there specific types of studies or publications you will exclude?
Select Databases for Searches:
- Identify relevant databases for your field. Examples include PubMed, IEEE Xplore, Scopus, Web of Science, and Google Scholar.
- Consider searching in library catalogs, institutional repositories, and specialized databases related to your topic.
Conduct Searches and Keep Track:
- Develop a systematic search strategy using keywords, Boolean operators (AND, OR, NOT), and other search techniques.
- Record and document your search strategy for transparency and replicability.
- Keep track of the articles, including publication details, abstracts, and links. Use citation management tools like EndNote, Zotero, or Mendeley to organize your references.
Review the Literature:
- Evaluate the relevance and quality of each source. Consider the methodology, sample size, and results of studies.
- Organize the literature by themes or key concepts. Identify patterns, trends, and gaps in the existing research.
- Summarize key findings and arguments from each source. Compare and contrast different perspectives.
- Identify areas where there is a consensus in the literature and where there are conflicting opinions.
- Provide critical analysis and synthesis of the literature. What are the strengths and weaknesses of existing research?
Organize and Write Your Literature Review:
- Literature review outline should be based on themes, chronological order, or methodological approaches.
- Write a clear and coherent narrative that synthesizes the information gathered.
- Use proper citations for each source and ensure consistency in your citation style (APA, MLA, Chicago, etc.).
- Conclude your literature review by summarizing key findings, identifying gaps, and suggesting areas for future research.
The literature review sample and detailed advice on writing and conducting a review will help you produce a well-structured report. But remember that a literature review is an ongoing process, and it may be necessary to revisit and update it as your research progresses.
Frequently asked questions
A literature review is a critical and comprehensive analysis of existing literature (published and unpublished works) on a specific topic or research question and provides a synthesis of the current state of knowledge in a particular field. A well-conducted literature review is crucial for researchers to build upon existing knowledge, avoid duplication of efforts, and contribute to the advancement of their field. It also helps researchers situate their work within a broader context and facilitates the development of a sound theoretical and conceptual framework for their studies.
Literature review is a crucial component of research writing, providing a solid background for a research paper’s investigation. The aim is to keep professionals up to date by providing an understanding of ongoing developments within a specific field, including research methods, and experimental techniques used in that field, and present that knowledge in the form of a written report. Also, the depth and breadth of the literature review emphasizes the credibility of the scholar in his or her field.
Before writing a literature review, it’s essential to undertake several preparatory steps to ensure that your review is well-researched, organized, and focused. This includes choosing a topic of general interest to you and doing exploratory research on that topic, writing an annotated bibliography, and noting major points, especially those that relate to the position you have taken on the topic.
Literature reviews and academic research papers are essential components of scholarly work but serve different purposes within the academic realm. 3 A literature review aims to provide a foundation for understanding the current state of research on a particular topic, identify gaps or controversies, and lay the groundwork for future research. Therefore, it draws heavily from existing academic sources, including books, journal articles, and other scholarly publications. In contrast, an academic research paper aims to present new knowledge, contribute to the academic discourse, and advance the understanding of a specific research question. Therefore, it involves a mix of existing literature (in the introduction and literature review sections) and original data or findings obtained through research methods.
Literature reviews are essential components of academic and research papers, and various strategies can be employed to conduct them effectively. If you want to know how to write a literature review for a research paper, here are four common approaches that are often used by researchers. Chronological Review: This strategy involves organizing the literature based on the chronological order of publication. It helps to trace the development of a topic over time, showing how ideas, theories, and research have evolved. Thematic Review: Thematic reviews focus on identifying and analyzing themes or topics that cut across different studies. Instead of organizing the literature chronologically, it is grouped by key themes or concepts, allowing for a comprehensive exploration of various aspects of the topic. Methodological Review: This strategy involves organizing the literature based on the research methods employed in different studies. It helps to highlight the strengths and weaknesses of various methodologies and allows the reader to evaluate the reliability and validity of the research findings. Theoretical Review: A theoretical review examines the literature based on the theoretical frameworks used in different studies. This approach helps to identify the key theories that have been applied to the topic and assess their contributions to the understanding of the subject. It’s important to note that these strategies are not mutually exclusive, and a literature review may combine elements of more than one approach. The choice of strategy depends on the research question, the nature of the literature available, and the goals of the review. Additionally, other strategies, such as integrative reviews or systematic reviews, may be employed depending on the specific requirements of the research.
The literature review format can vary depending on the specific publication guidelines. However, there are some common elements and structures that are often followed. Here is a general guideline for the format of a literature review: Introduction: Provide an overview of the topic. Define the scope and purpose of the literature review. State the research question or objective. Body: Organize the literature by themes, concepts, or chronology. Critically analyze and evaluate each source. Discuss the strengths and weaknesses of the studies. Highlight any methodological limitations or biases. Identify patterns, connections, or contradictions in the existing research. Conclusion: Summarize the key points discussed in the literature review. Highlight the research gap. Address the research question or objective stated in the introduction. Highlight the contributions of the review and suggest directions for future research.
Both annotated bibliographies and literature reviews involve the examination of scholarly sources. While annotated bibliographies focus on individual sources with brief annotations, literature reviews provide a more in-depth, integrated, and comprehensive analysis of existing literature on a specific topic. The key differences are as follows:
References
- Denney, A. S., & Tewksbury, R. (2013). How to write a literature review. Journal of criminal justice education , 24 (2), 218-234.
- Pan, M. L. (2016). Preparing literature reviews: Qualitative and quantitative approaches . Taylor & Francis.
- Cantero, C. (2019). How to write a literature review. San José State University Writing Center .
Paperpal is an AI writing assistant that help academics write better, faster with real-time suggestions for in-depth language and grammar correction. Trained on millions of research manuscripts enhanced by professional academic editors, Paperpal delivers human precision at machine speed.
Try it for free or upgrade to Paperpal Prime , which unlocks unlimited access to premium features like academic translation, paraphrasing, contextual synonyms, consistency checks and more. It’s like always having a professional academic editor by your side! Go beyond limitations and experience the future of academic writing. Get Paperpal Prime now at just US$19 a month!
Related Reads:
- Empirical Research: A Comprehensive Guide for Academics
- How to Write a Scientific Paper in 10 Steps
- Life Sciences Papers: 9 Tips for Authors Writing in Biological Sciences
- What is an Argumentative Essay? How to Write It (With Examples)
6 Tips for Post-Doc Researchers to Take Their Career to the Next Level
Self-plagiarism in research: what it is and how to avoid it, you may also like, what is academic writing: tips for students, why traditional editorial process needs an upgrade, paperpal’s new ai research finder empowers authors to..., what is hedging in academic writing , how to use ai to enhance your college..., ai + human expertise – a paradigm shift..., how to use paperpal to generate emails &..., ai in education: it’s time to change the..., is it ethical to use ai-generated abstracts without..., do plagiarism checkers detect ai content.
Introduction and Literature Review of Power System Challenges and Issues
- First Online: 21 October 2021
Cite this chapter
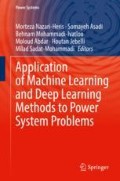
- Ali Ardeshiri 7 ,
- Amir Lotfi 7 ,
- Reza Behkam 8 ,
- Arash Moradzadeh 9 &
- Ashkan Barzkar 7
Part of the book series: Power Systems ((POWSYS))
1434 Accesses
17 Citations
Over many decades, the electric power industry has evolved from a single low-power generator serving a small area to highly interconnected networks serving a large number of countries, or even continents. Nowadays, an electric power system is one of the largest man-made systems ever created, consisting of an enormous number of components ranging from small electric appliances to very large turbo-generators. Running such a large system is a significant challenge. It has necessitated the resolution of numerous issues by educational and industrial institutions. The main issues of the power system can be categorized into planning, operation, and control issues which are analyzed in this chapter, separately. Machine learning, deep learning, and a variety of regression, classification, and clustering algorithms are all extremely effective tools for addressing these issues. These procedures can be used to resolve a variety of power system issues and concerns, including planning, operation, fault detection and protection, power system analysis and control, and cyber security.
This is a preview of subscription content, log in via an institution to check access.
Access this chapter
- Available as PDF
- Read on any device
- Instant download
- Own it forever
- Available as EPUB and PDF
- Compact, lightweight edition
- Dispatched in 3 to 5 business days
- Free shipping worldwide - see info
- Durable hardcover edition
Tax calculation will be finalised at checkout
Purchases are for personal use only
Institutional subscriptions
P. Kundur, Power System Stability and Control (McGraw-Hill, New York, 1993)
Google Scholar
M. Fotuhi-Firuzabad, A. Safdarian, M. Moeini-Aghtaie, R. Ghorani, M. Rastegar, H. Farzin, Upcoming challenges of future electric power systems: sustainability and resiliency. Sci. Iranica 23 , 1565 (2016)
Article Google Scholar
IEA, World Energy Outlook (2019)
A. Moradzadeh, O. Sadeghian, K. Pourhossein, B. Mohammadi-Ivatloo, A. Anvari-Moghaddam, Improving residential load disaggregation for sustainable development of energy via principal component analysis. Sustainability (Switzerland) 12 (8), 3158 (2020). https://doi.org/10.3390/SU12083158
C. Canizares, J. Nathwani, D. Kammen, Electricity for all: issues, challenges, and solutions for energy-disadvantaged communities, in Proceedings of the IEEE , vol. 107 (2019)
A. Moradzadeh, A. Mansour-Saatloo, B. Mohammadi-Ivatloo, A. Anvari-Moghaddam, Performance evaluation of two machine learning techniques in heating and cooling loads forecasting of residential buildings. Appl. Sci. (Switzerland) 10 (11), 3829 (2020). https://doi.org/10.3390/app10113829
A. Lotfi, B. Mohammadi-Ivatloo, S. Asadi, Introduction to FEW Nexus, in Food-Energy-Water Nexus Resilience and Sustainable Development , ed. by S. Asadi, B. Mohammadi-Ivatloo, (Springer, Switzerland, 2020)
O. Sadeghian, A. Moradzadeh, B. Mohammadi-Ivatloo, M. Abapour, F.P.G. Marquez, Generation units maintenance in combined heat and power integrated systems using the mixed integer quadratic programming approach. Energies 13 (11), 2840 (2020). https://doi.org/10.3390/en13112840
A. Demir, N. Hadžijahić, Power system planning: part I—basic principles, in Advanced Technologies, Systems, and Applications II , ed. by M. Hadžikadić, S. Avdaković, vol. 28, (Springer, New York, 2018)
A.M. Al-Shaalan, Essential aspects of power system planning in developing countries. J. King Saud Univ. Eng. Sci. 23 , 27–32 (2011)
A. Lotfi, S.H. Hosseini, Composite distributed generation and transmission expansion planning considering security. World Acad. Sci. Eng. Technol. Int. J. Energy Power Eng. 11 (2017)
A.J. Conejo, L. Baringo, Power Systems. In Power System Operations, pp. 1–15, (Springer, Cham, 2018)
X. Wang, J.R. McDonald, Modern Power System Planning (McGraw-Hill, New York, 1994)
A. Moradzadeh, K. Khaffafi, Comparison and evaluation of the performance of various types of neural networks for planning issues related to optimal management of charging and discharging electric cars in intelligent power grids. Emerg. Sci. J. 1 (4), 201–207 (2017). https://doi.org/10.28991/ijse-01123
A. Moradzadeh, B. Mohammadi-Ivatloo, M. Abapour, A. Anvari-Moghaddam, S. Gholami Farkoush, S.B. Rhee, A practical solution based on convolutional neural network for non-intrusive load monitoring. J. Ambient Intell. Humaniz. Comput. (2021). https://doi.org/10.1007/s12652-020-02720-6
H. Seifi, M.S. Sepasian, Electric Power System Planning: Issues, Algorithms and Solutions (Springer, New York, 2011)
Book Google Scholar
R. Hemmati, R.A. Hooshmand, A. Khodabakhshian, Comprehensive review of generation and transmission expansion planning. IET Gener. Transm. Distrib. 7 (9), 955–964 (2013)
G. Latorre, R.D. Crus, J.M. Areiza, A. Villegas, Classification of publications and models on transmission expansion planning. IEEE Trans. Power Syst. 18 (2), 938–946 (2003)
R.S. Najafi, H. Khatami, Optimal and robust distribution system planning to forecasting uncertainty. Tabriz J. Electr. Eng. 46 (2), 323–332 (2016)
A. Moradzadeh, K. Pourhossein, Early detection of turn-to-turn faults in power transformer winding: an experimental study, in Proceedings 2019 International Aegean Conference on Electrical Machines and Power Electronics, ACEMP 2019 and 2019 International Conference on Optimization of Electrical and Electronic Equipment, OPTIM 2019 (2019), pp. 199–204, https://doi.org/10.1109/ACEMP-OPTIM44294.2019.9007169
A. Mansour-Saatloo, A. Moradzadeh, B. Mohammadi-Ivatloo, A. Ahmadian, A. Elkamel, Machine learning based PEVs load extraction and analysis. Electronics (Switzerland) 9 (7), 1–15 (2020). https://doi.org/10.3390/electronics9071150
S.N. Ravandanegh, N. Jahanyari, A. Amini, N. Taghizadeghan, Smart distribution grid multistage expansion planning under load forecasting uncertainty. IET Gener. Transm. Distrib. 10 (5), 1136–1144 (2016)
P. Prakash, D.K. Khatod, Optimal sizing and siting techniques for distributed generation in distribution systems: A review. Renew. Sust. Energ. Rev. 57 , 111–130 (2016)
A.R. Jordehi, Allocation of distributed generation units in electric power systems: A review. Renew. Sust. Energ. Rev. 56 , 893–905 (2016)
J.P. Lopes, N. Hatziargyriou, J. Mutale, P. Djapic, N. Jenkins, Integrating distributed generation into electric power systems: A review of drivers, challenges and opportunities. Electr. Power Syst. Res. 77 (9), 1189–1203 (2007)
A. Patt, S. Pfenninger, J. Lilliestam, Vulnerability of solar energy infrastructure and output to climate change. Clim. Change 121 , 93–102 (2013). https://doi.org/10.1007/s10584-013-0887-0
J.A. Crook, L.A. Jones, M. Forster, R. Crook, Climate change impacts on future photovoltaic and concentrated solar power energy output. Energy Environ. Sci. 4 , 3101–3109 (2011). https://doi.org/10.1039/c1ee01495a
M. Gaetani, T. Huld, E. Vignati, F. Monforti-ferrario, A. Dosio, F. Raes, The near future availability of photovoltaic energy in Europe and Africa in climate-aerosol modeling experiments. Renew. Sust. Energ. Rev. 38 , 706–716 (2014). https://doi.org/10.1016/j.rser.2014.07.041
I.S. Panagea, I.K. Tsanis, A.G. Koutroulis, M.G. Grillakis, Climate change impact on photovoltaic energy output : the case of Greece. Adv Meteorol 2014 , 63–86 (2014)
S.C. Pryor, R.J. Barthelmie, Climate change impacts on wind energy: a review. Renewable and sustainable energy reviews 14 , 430–437 (2010). https://doi.org/10.1016/j.rser.2009.07.028
I. Tobin et al., Assessing climate change impacts on European wind energy from ENSEMBLES high-resolution climate projections. Clim. Change 128 , 99–112 (2015). https://doi.org/10.1007/s10584-014-1291-0
R. Davy, N. Gnatiuk, L. Pettersson, L. Bobylev, Climate change impacts on wind energy potential in the European domain with a focus on the Black Sea. Renew. Sustain. Energy Rev. 2016 , 1–8 (2017). https://doi.org/10.1016/j.rser.2017.05.253
C.S. Santos, D. Carvalho, A. Rocha, M. Gómez-Gesteira, Potential impacts of climate change on European wind energy resource under the CMIP5 future climate projections. Renew. Energy 101 (2017), 29–40 (2020). https://doi.org/10.1016/j.renene.2016.08.036
L. Chen, S.C. Pryor, D. Li, Assessing the performance of Intergovernmental Panel on Climate Change AR5 climate models in simulating and projecting wind speeds over China. Journal of Geophysical Research: Atmospheres 117 , 1–15 (2012). https://doi.org/10.1029/2012JD017533
C. Fant, C.A. Schlosser, K. Strzepek, The impact of climate change on wind and solar resources in southern Africa. Appl. Energy (2015). https://doi.org/10.1016/j.apenergy.2015.03.042
B. Kamranzad, A. Etemad-shahidi, V. Chegini, Climate change impact on wave energy in the Persian Gulf. (2015). https://doi.org/10.1007/s10236-015-0833-y
J.P. Sierra, M. Casas-prat, E. Campins, Impact of climate change on wave energy resource : the case of Menorca (Spain). Renew. Energy 101 , 275–285 (2017). https://doi.org/10.1016/j.renene.2016.08.060
D.E. Reeve, Y. Chen, S. Pan, V. Magar, D.J. Simmonds, A. Zacharioudaki, An investigation of the impacts of climate change on wave energy generation : The Wave Hub, Cornwall, UK. Renew. Energy 36 (9), 2404–2413 (2011). https://doi.org/10.1016/j.renene.2011.02.020
B. Hamududu, A. Killingtveit, E. Engineering, Assessing climate change impacts on global hydropower. Energies 5 (2), 305–322 (2012). https://doi.org/10.3390/en5020305
S.W.D. Turner, J. Yi, S. Galelli, Science of the total environment examining global electricity supply vulnerability to climate change using a high-fidelity hydropower dam model. Sci. Total Environ. (2017). https://doi.org/10.1016/j.scitotenv.2017.03.022
M.T.H. Van Vliet, D. Wiberg, S. Leduc, K. Riahi, Power-generation system vulnerability and adaptation to changes in climate and water resources. Nature Climate Change 6 (4), 375–380 (2016). https://doi.org/10.1038/NCLIMATE2903
H. Haberl et al., Global bioenergy potentials from agricultural land in 2050: sensitivity to climate change, diets and yields. Biomass and bioenergy 35 (12), 4753–4769 (2011). https://doi.org/10.1016/j.biombioe.2011.04.035
G. Tuck, M.J. Glendining, P. Smith, J.I. House, M. Wattenbach, The potential distribution of bioenergy crops in Europe under present and future climate. Biomass Bioenergy 30 , 183–197 (2006). https://doi.org/10.1016/j.biombioe.2005.11.019
J.N. Barney, J.M. Ditomaso, Bioclimatic predictions of habitat suitability for the biofuel switchgrass in North America under current and future climate scenarios. Biomass Bioenergy 34 (1), 124–133 (2010). https://doi.org/10.1016/j.biombioe.2009.10.009
H.A. Hager, S.E. Sinasac, Z. Gedalof, J.A. Newman, Predicting potential global distributions of two miscanthus grasses : implications for horticulture, biofuel production, and biological invasions. PLoS One 9 (6), e100032 (2014). https://doi.org/10.1371/journal.pone.0100032
C. Chuang, D. Sue, Performance effects of combined cycle power plant with variable condenser pressure and loading. Energy 30 , 1793–1801 (2005). https://doi.org/10.1016/j.energy.2004.10.003
A. Durmayaz, O.S. Sogut, Influence of cooling water temperature on the efficiency of a pressurized-water reactor nuclear-power plant. International Journal of Energy Research, 2005 , 799–810 (2006). https://doi.org/10.1002/er.1186
K. Linnerud, T.K. Mideksa, G.S. Eskeland, The impact of climate change on nuclear power supply. Energy J. 32 , 149–168 (2011)
M. Bartos et al., Environ. Res. Lett. 11 (2016)
R. Contreras-Lisperguer, K. De-Cuba, The potential impact of climate change on the energy sector in the Caribbean region. Organization of American States, Washington DC (2008)
W. Li, E. Vaahedi, P. Choudhury, Power system equipment aging. IEEE Power Energy Mag 4 (3), 52–58 (2006). https://doi.org/10.1109/MPAE.2006.1632454
A. Moradnouri, A. Ardeshiri, M. Vakilian, A. Hekmati, M. Fardmanesh, Survey on high-temperature superconducting transformer windings design. J. Superconductivity Novel Magnet. 33 , 2581–2599 (2020). https://doi.org/10.1007/s10948-020-05539-6
S.S. Kalsi, Application of High-Temperature Superconductors to Electric Power Equipment (IEEE Press, Wiley, 2011)
We Energies, Disturbance types and solutions [Online], https://www.we-energies.com/safety/power-quality/disturbance-types
R. Godse, S. Bhat, Mathematical morphology-based feature-extraction technique for detection and classification of faults on power transmission line. IEEE Access 8 , 38459–38471 (2020). https://doi.org/10.1109/ACCESS.2020.2975431
M.M. Taheri, H. Seyedi, B. Mohammadi-ivatloo, DT-based relaying scheme for fault classification in transmission lines using MODP. IET Generation Transm. Distrib. 11 (11), 2796–2804 (2017). https://doi.org/10.1049/iet-gtd.2016.1821
M. Mohammad Taheri, H. Seyedi, M. Nojavan, M. Khoshbouy, B. Mohammadi Ivatloo, High-speed decision tree based series-compensated transmission lines protection using differential phase angle of superimposed current. IEEE Trans. Power Deliv. 33 (6), 3130–3138 (2018). https://doi.org/10.1109/TPWRD.2018.2861841
H. Teimourzadeh, A. Moradzadeh, M. Shoaran, B. Mohammadi-Ivatloo, R. Razzaghi, High impedance single-phase faults diagnosis in transmission lines via deep reinforcement learning of transfer functions. IEEE Access (2021). https://doi.org/10.1109/ACCESS.2021.3051411
K. Chen, J. Hu, Y. Zhang, Z. Yu, J. He, Fault location in power distribution systems via deep graph convolutional networks. IEEE J. Sel. Areas Commun. 38 (1), 119–131 (2020). https://doi.org/10.1109/JSAC.2019.2951964
S. Zhang, Y. Wang, M. Liu, Z. Bao, Data-based line trip fault prediction in power systems using LSTM networks and SVM. IEEE Access 6 , 7675–7686 (2018). https://doi.org/10.1109/ACCESS.2017.2785763
G. Luo, Y. Tan, M. Li, M. Cheng, Y. Liu, J. He, Stacked auto-encoder-based fault location in distribution network. IEEE Access 8 , 28043–28053 (2020). https://doi.org/10.1109/ACCESS.2020.2971582
B. Li, J. Wu, L. Hao, M. Shao, R. Zhang, W. Zhao, Anti-jitter and refined power system transient stability assessment based on long-short term memory network. IEEE Access 8 , 35231–35244 (2020). https://doi.org/10.1109/ACCESS.2020.2974915
J. Liu, Z. Zhao, C. Tang, C. Yao, C. Li, S. Islam, Classifying transformer winding deformation fault types and degrees using FRA based on support vector machine. IEEE Access 7 , 112494–112504 (2019). https://doi.org/10.1109/access.2019.2932497
A. Moradzadeh, K. Pourhossein, Short circuit location in transformer winding using deep learning of its frequency responses, in Proceedings 2019 International Aegean Conference on Electrical Machines and Power Electronics, ACEMP 2019 and 2019 International Conference on Optimization of Electrical and Electronic Equipment, OPTIM 2019 (2019), pp. 268–273, https://doi.org/10.1109/ACEMP-OPTIM44294.2019.9007176
A. Moradzadeh, K. Pourhossein, Application of support vector machines to locate minor short circuits in transformer windings, in 2019 54th International Universities Power Engineering Conference (UPEC) , (2019), pp. 1–6
S. Lan, M.-J. Chen, D.-Y. Chen, A novel HVDC double-terminal non-synchronous fault location method based on convolutional neural network. IEEE Trans. Power Deliv. 34 (3), 848–857 (2019). https://doi.org/10.1109/TPWRD.2019.2901594
R. Rohani, A. Koochaki, A hybrid method based on optimized neuro-fuzzy system and effective features for fault location in VSC-HVDC systems. IEEE Access 8 , 70861–70869 (2020). https://doi.org/10.1109/ACCESS.2020.2986919
G. Luo, J. Hei, C. Yao, J. He, M. Li, An end-to-end transient recognition method for VSC-HVDC based on deep belief network. J. Mod. Power Syst. Clean Energy 8 (6), 1070–1079 (2020). https://doi.org/10.35833/MPCE.2020.000190
SGTF_EG2, 2nd Interim Report Recommendations for the European Commission on Implementation of a Network Code on Cybersecurity (2018)
The European Economic and Social Committee and the Committee of the Regions Cybersecurity strategy of the E. U. European Commission. Joint communication to the European parliament, the council, An open, safe and secure cyberspace (2013)
ANL_GSS_15/4, Analysis of critical infrastructure dependencies and interdependencies, Argonne-risk and infrastructure science center , (2015)
A. Dagoumas, Assessing the impact of cybersecurity attacks on power systems. Energies (2019). https://doi.org/10.3390/en12040725
A. Humayed, J. Lin, F. Li, B. Luo, Cyber-physical systems security - a survey. IEEE Internet Things J. 4 (6), 1802–1831 (2017). https://doi.org/10.1109/JIOT.2017.2703172
B. Jimada-Ojuolape, J. Teh, Surveys on the reliability impacts of power system cyber–physical layers. Sustain. Cities Soc. 62 , 102384 (2020). https://doi.org/10.1016/j.scs.2020.102384
Y. Ashibani, Q.H. Mahmoud, Cyber physical systems security: Analysis, challenges and solutions. Comput. Secur. 68 , 81–97 (2017). https://doi.org/10.1016/j.cose.2017.04.005
H. He, J. Yan, Cyber-physical attacks and defences in the smart grid: A survey. IET Cyber-Phys. Syst. Theory Appl. 1 (1), 13–27 (2016). https://doi.org/10.1049/iet-cps.2016.0019
J.P.A. Yaacoub, O. Salman, H.N. Noura, N. Kaaniche, A. Chehab, M. Malli, Cyber-physical systems security: Limitations, issues and future trends. Microprocess. Microsyst. 77 , 103201 (2020). https://doi.org/10.1016/j.micpro.2020.103201
M. Husak, J. Komarkova, E. Bou-Harb, P. Celeda, Survey of attack projection, prediction, and forecasting in cyber security. IEEE Commun. Surv. Tutorials 21 (1), 640–660 (2019). https://doi.org/10.1109/COMST.2018.2871866
Y. Wang, M.M. Amin, J. Fu, H.B. Moussa, A novel data analytical approach for false data injection cyber-physical attack mitigation in smart grids. IEEE Access 5 , 26022–26033 (2017). https://doi.org/10.1109/ACCESS.2017.2769099
H. Karimipour, A. Dehghantanha, R.M. Parizi, K.-K.R. Choo, H. Leung, A deep and scalable unsupervised machine learning system for cyber-attack detection in large-scale smart grids. IEEE Access 7 , 80778–80788 (2019). https://doi.org/10.1109/ACCESS.2019.2920326
J.J.Q. Yu, Y. Hou, V.O.K. Li, Online false data injection attack detection with wavelet transform and deep neural networks. IEEE Trans. Ind. Informat. 14 (7), 3271–3280 (2018). https://doi.org/10.1109/TII.2018.2825243
A. Al-Abassi, H. Karimipour, A. Dehghantanha, R.M. Parizi, An ensemble deep learning-based cyber-attack detection in industrial control system. IEEE Access 8 , 83965–83973 (2020). https://doi.org/10.1109/ACCESS.2020.2992249
S. Soltan, P. Mittal, H.V. Poor, Line failure detection after a cyber-physical attack on the grid using Bayesian regression. IEEE Trans. Power Syst. 34 (5), 3758–3768 (2019). https://doi.org/10.1109/TPWRS.2019.2910396
F.C. Schweppe, J. Wildes, Power system static-state estimation, part i: Exact model. IEEE Trans. Power Apparatus Syst. 59 (1), 120–125 (1970)
A.J. Wood, B.F. Wollenberg, Power Generation Operation and Control (Wiley, New York, 2003)
K. Chatterjee, V. Padmini, S.A. Khaparde, Review of cyber attacks on power system operations, in IEEE Region 10 Symposium, Conference Paper , (2017)
D. P. Kothari and I. J. Padmini, Power System Engineering, New Delhi: Tata McGraw Hill Education, 2008
P.M. Esfahani, M. Vrakopoulou, K. Margellos, J. Lygeros, G. Andersson, Cyber Attack in a Two-Area Power System : Impact Identification using Reachability, In Proceedings of the 2010 American control conference, pp. 962–967. IEEE (2010)
B.F. Wollenberg, Power system operation and control, in Power System Stability and Control , 3rd edn., (CRC Press, 2017). https://doi.org/10.4324/b12113
H. Bevrani, Robust Power System Frequency Control (Power Electronics and Power Systems) (Springer, New York, 2009)
MATH Google Scholar
A. Moradzadeh, K. Pourhossein, B. Mohammadi-Ivatloo, F. Mohammadi, Locating inter-turn faults in transformer windings using isometric feature mapping of frequency response traces. IEEE Trans. Ind. Informat., 17 , 1–1 (2020). https://doi.org/10.1109/tii.2020.3016966
Z.A. Obaid, L.M. Cipcigan, L. Abrahim, M.T. Muhssin, Frequency control of future power systems: Reviewing and evaluating challenges and new control methods. J. Mod. Power Syst. Clean Energy 7 (1), 9–25 (2019). https://doi.org/10.1007/s40565-018-0441-1
F. Teng, Y. Mu, H. Jia, J. Wu, P. Zeng, G. Strbac, Challenges of primary frequency control and benefits of primary frequency response support from electric vehicles. Energy Procedia 88 , 985–990 (2016). https://doi.org/10.1016/j.egypro.2016.06.123
M.J. Bryant, R. Ghanbari, M. Jalili, P. Sokolowski, L. Meegahapola, Frequency Control Challenges in Power Systems with High Renewable Power Generation: An Australian Perspective, RMIT University (2019)
H.T. Nguyen, G. Yang, A.H. Nielsen, P.H. Jensen, Challenges and research opportunities of frequency control in low inertia systems, in E3S Web of Conferences , vol. 115, (2019). https://doi.org/10.1051/e3sconf/201911502001
Chapter Google Scholar
P.W. Sauer, Reactive power and voltage control issues in electric power systems, in Applied Mathematics for Restructured Electric Power Systems. Power Electronics and Power Systems , ed. by J. H. Chow, F. F. Wu, J. Momoh, (Springer, Boston, 2005)
Download references
Author information
Authors and affiliations.
Electrical Engineering Department, Sharif University of Technology, Tehran, Iran
Ali Ardeshiri, Amir Lotfi & Ashkan Barzkar
Electrical Engineering Department, Amirkabir University of Technology, Tehran, Iran
Reza Behkam
Faculty of Electrical and Computer Engineering, University of Tabriz, Tabriz, Iran
Arash Moradzadeh
You can also search for this author in PubMed Google Scholar
Corresponding author
Correspondence to Ali Ardeshiri .
Editor information
Editors and affiliations.
Department of Architectural Engineering, Pennsylvania State University, University Park, PA, USA
Morteza Nazari-Heris
Somayeh Asadi
Department of Energy Technology Aalborg University, Aalborg, Denmark
Behnam Mohammadi-Ivatloo
Deakin University, Geelong, VIC, Australia
Moloud Abdar
Houtan Jebelli
Milad Sadat-Mohammadi
Rights and permissions
Reprints and permissions
Copyright information
© 2021 The Author(s), under exclusive license to Springer Nature Switzerland AG
About this chapter
Ardeshiri, A., Lotfi, A., Behkam, R., Moradzadeh, A., Barzkar, A. (2021). Introduction and Literature Review of Power System Challenges and Issues. In: Nazari-Heris, M., Asadi, S., Mohammadi-Ivatloo, B., Abdar, M., Jebelli, H., Sadat-Mohammadi, M. (eds) Application of Machine Learning and Deep Learning Methods to Power System Problems. Power Systems. Springer, Cham. https://doi.org/10.1007/978-3-030-77696-1_2
Download citation
DOI : https://doi.org/10.1007/978-3-030-77696-1_2
Published : 21 October 2021
Publisher Name : Springer, Cham
Print ISBN : 978-3-030-77695-4
Online ISBN : 978-3-030-77696-1
eBook Packages : Energy Energy (R0)
Share this chapter
Anyone you share the following link with will be able to read this content:
Sorry, a shareable link is not currently available for this article.
Provided by the Springer Nature SharedIt content-sharing initiative
- Publish with us
Policies and ethics
- Find a journal
- Track your research
Got any suggestions?
We want to hear from you! Send us a message and help improve Slidesgo
Top searches
Trending searches
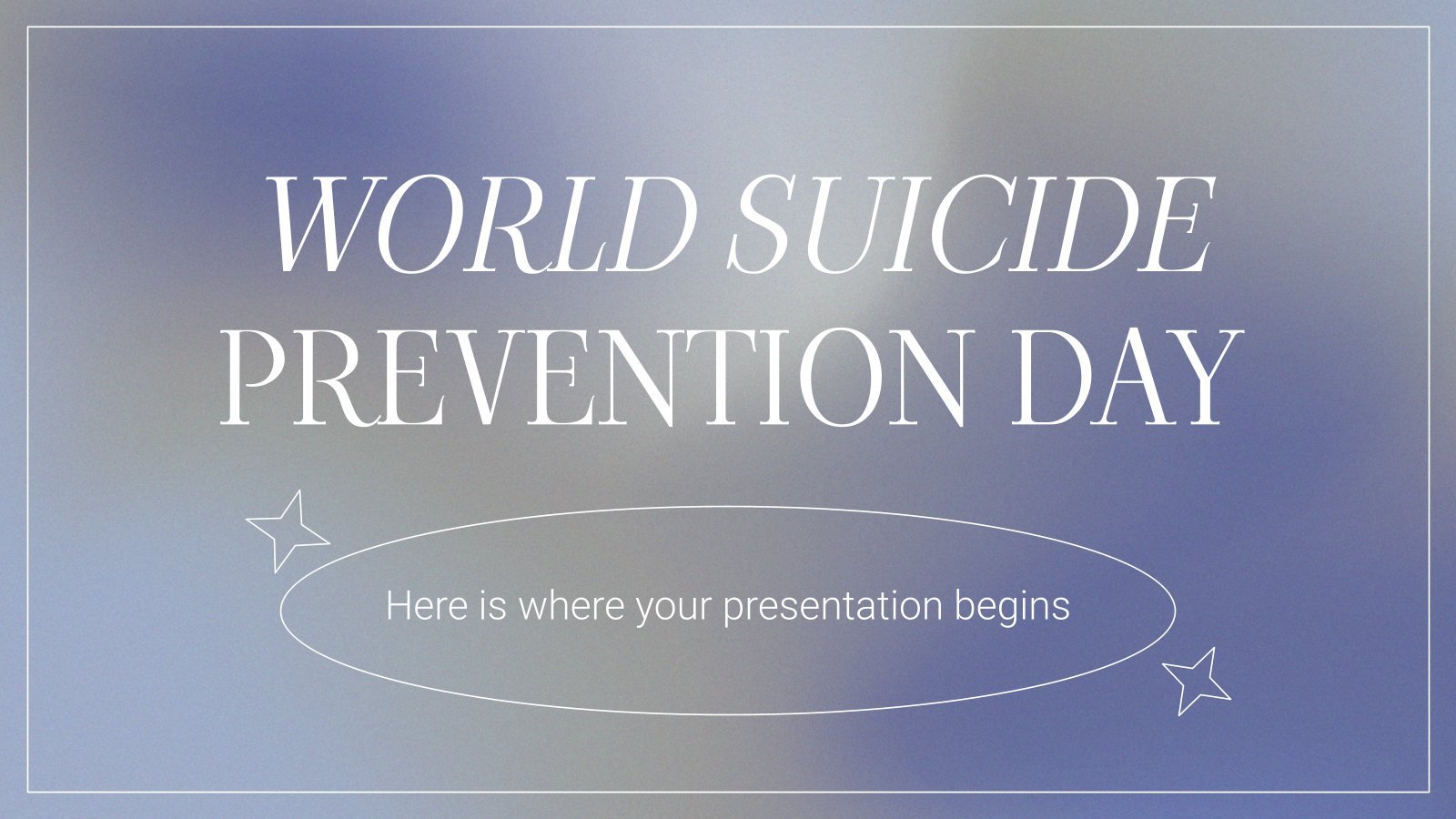
suicide prevention
8 templates
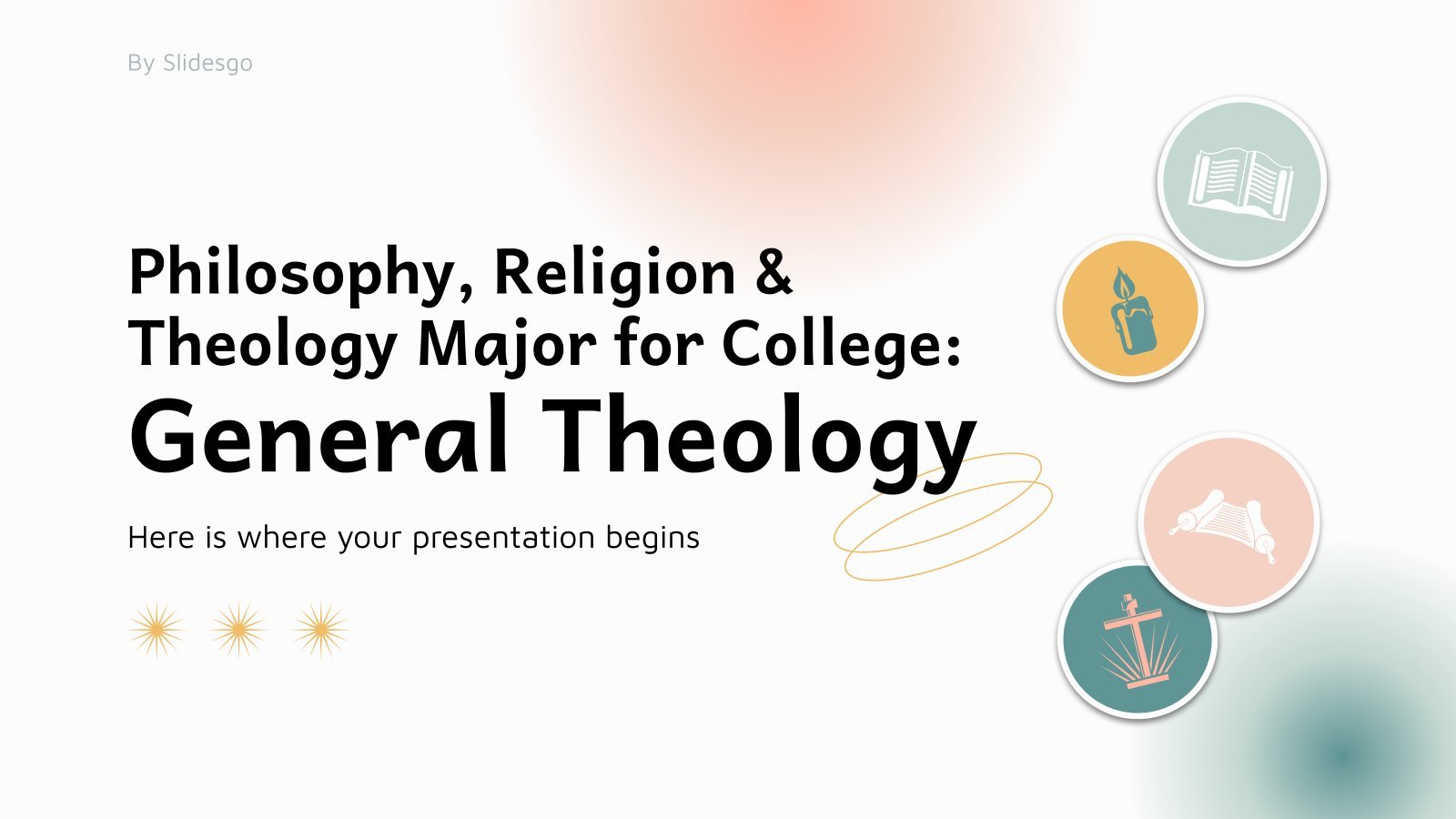
46 templates

cybersecurity
6 templates
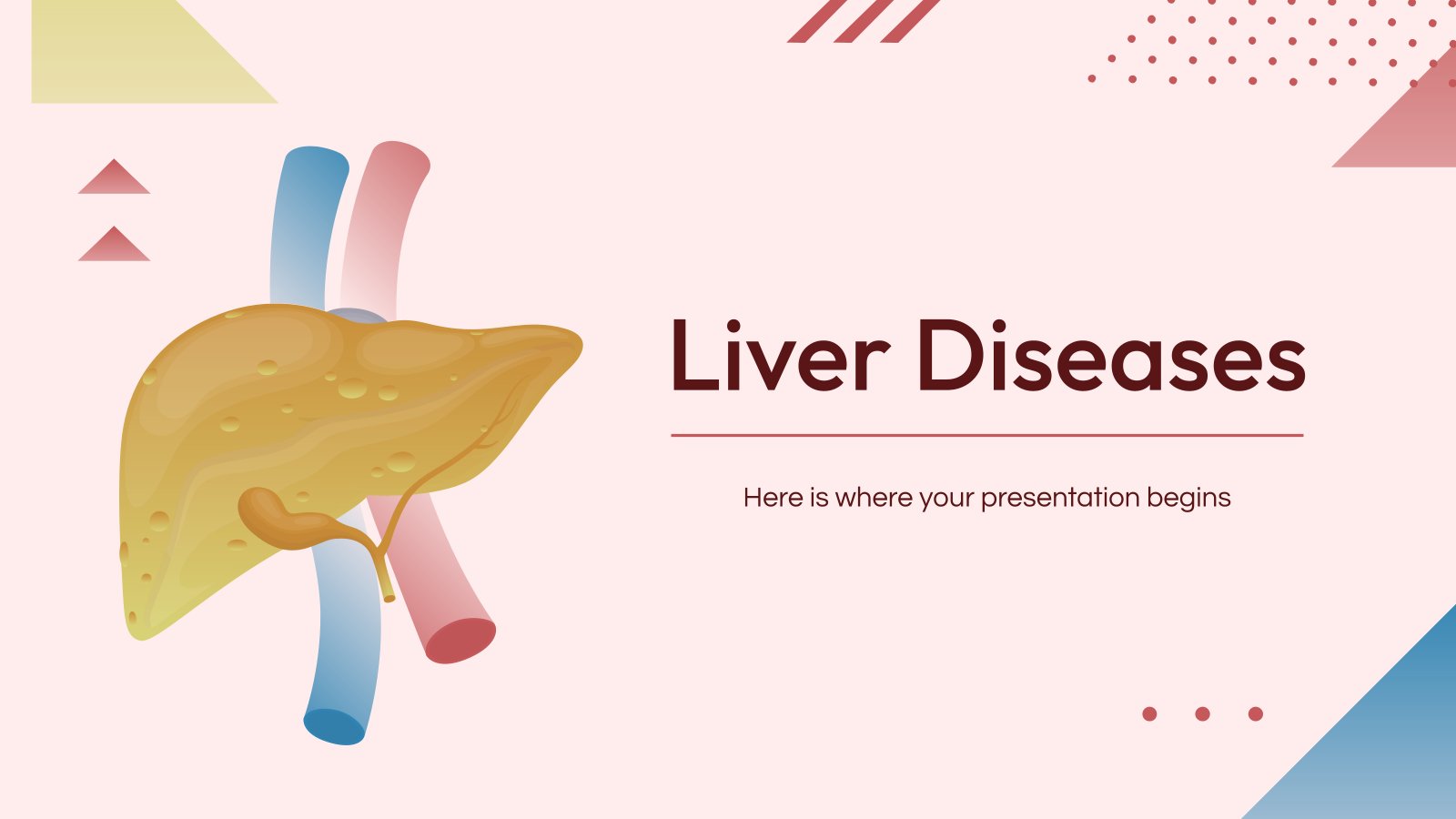
10 templates
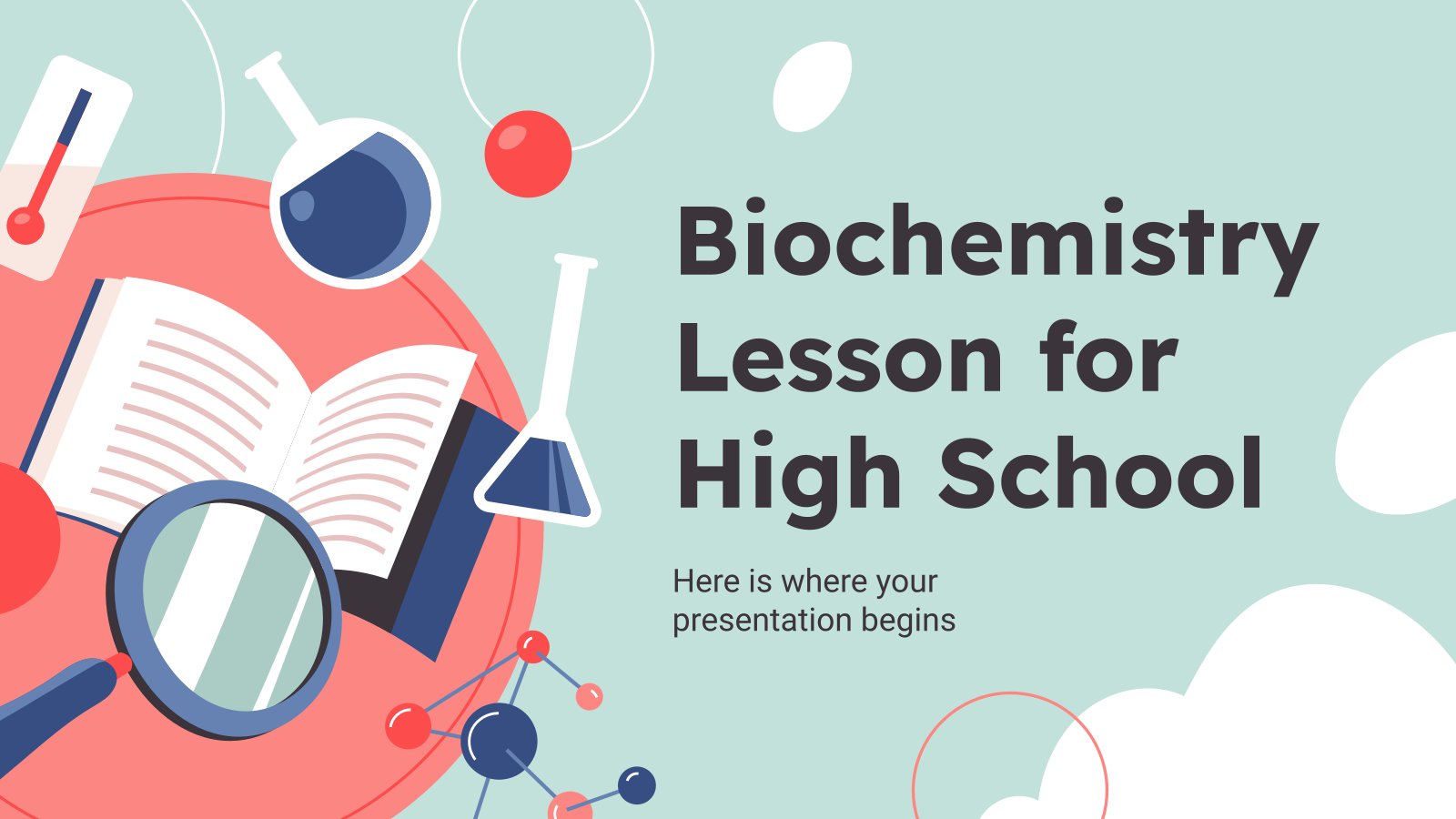
biochemistry
37 templates
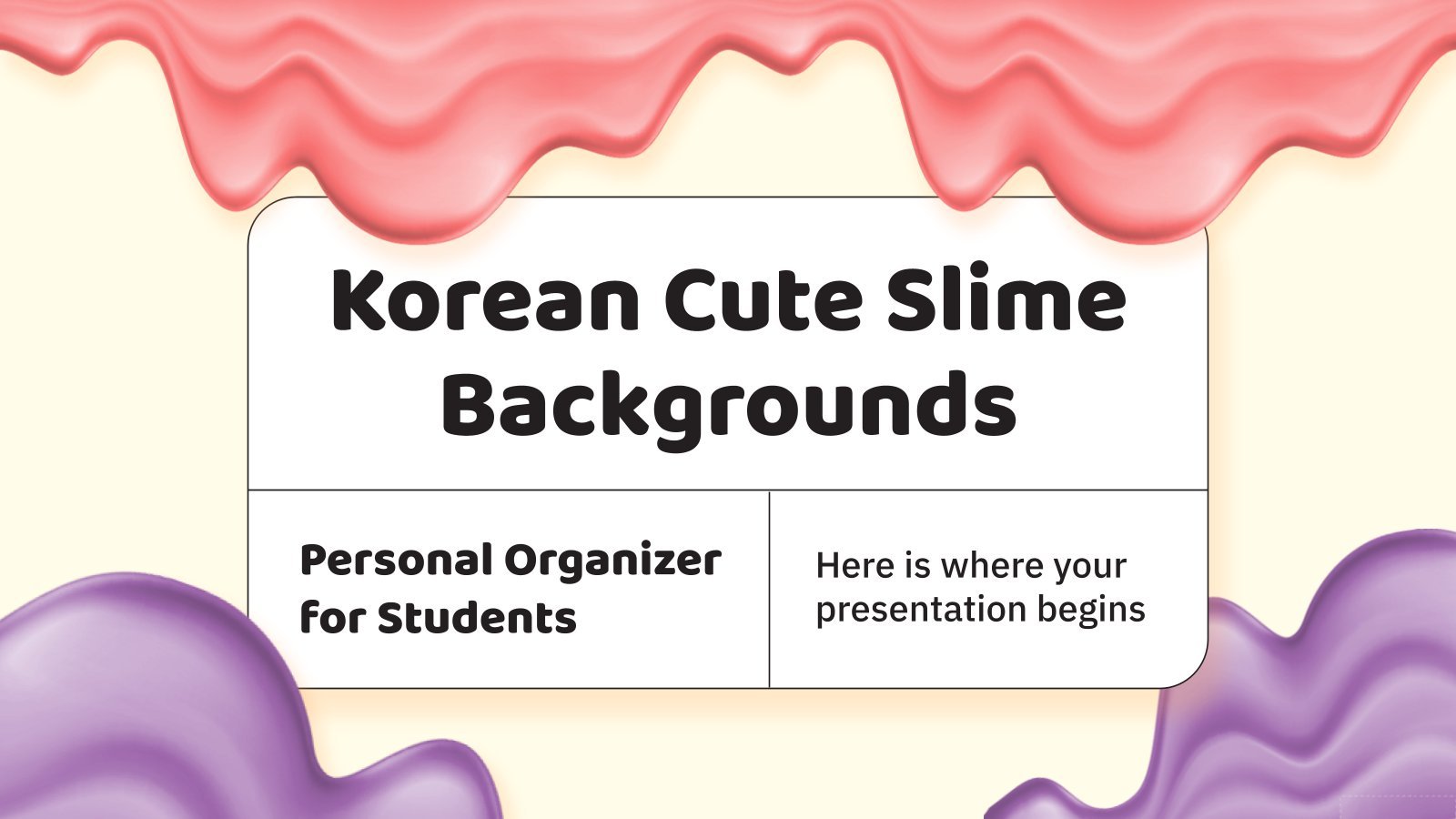
18 templates
Literature Review
Literature review presentation, free google slides theme, powerpoint template, and canva presentation template.
Whether you're a student or an academic, mastering the literature review is a key skill in scholarly writing. This fully customizable Google Slides and PowerPoint template can assist you in structuring your review seamlessly. Featuring a vibrant yellow design with captivating book illustrations, this template is designed to facilitate the organization and presentation of your research. Navigate your audience through chapters, themes, and references with ease and clarity using this versatile academic tool. Utilize this tool to craft an impressive literature review that leaves a lasting impression!
Features of this template
- 100% editable and easy to modify
- 35 different slides to impress your audience
- Contains easy-to-edit graphics such as graphs, maps, tables, timelines and mockups
- Includes 500+ icons and Flaticon’s extension for customizing your slides
- Designed to be used in Google Slides and Microsoft PowerPoint
- 16:9 widescreen format suitable for all types of screens
- Includes information about fonts, colors, and credits of the resources used
How can I use the template?
Am I free to use the templates?
How to attribute?
Attribution required If you are a free user, you must attribute Slidesgo by keeping the slide where the credits appear. How to attribute?
Related posts on our blog.
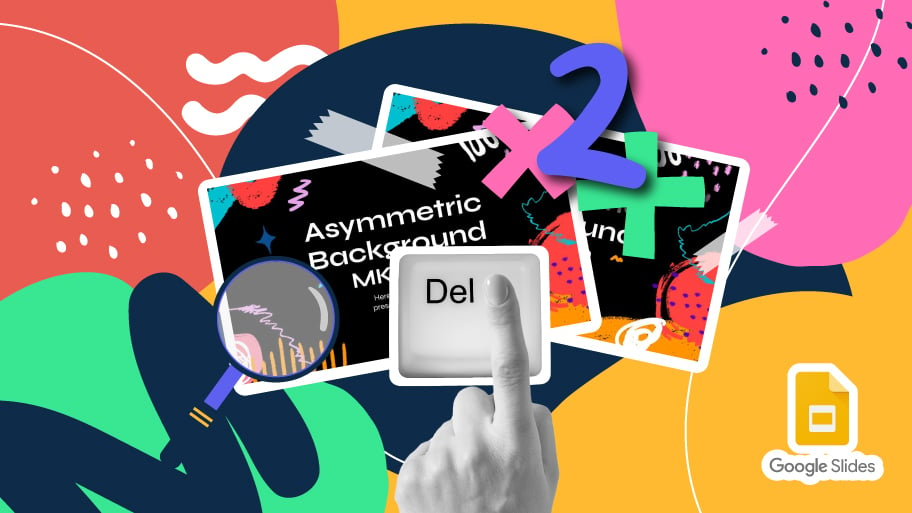
How to Add, Duplicate, Move, Delete or Hide Slides in Google Slides
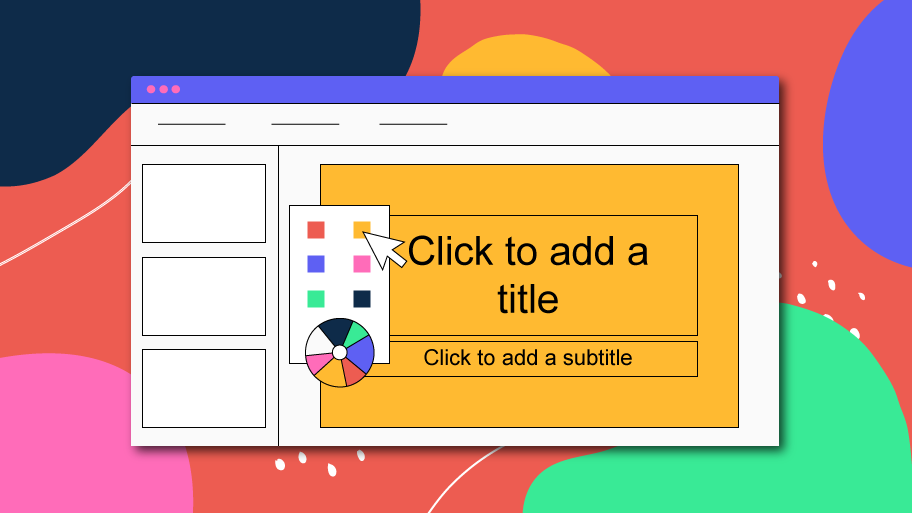
How to Change Layouts in PowerPoint
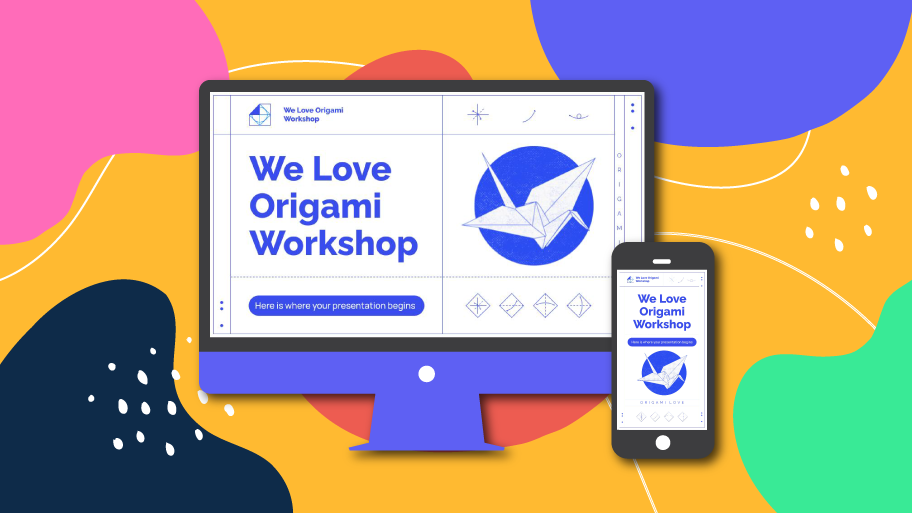
How to Change the Slide Size in Google Slides
Related presentations.
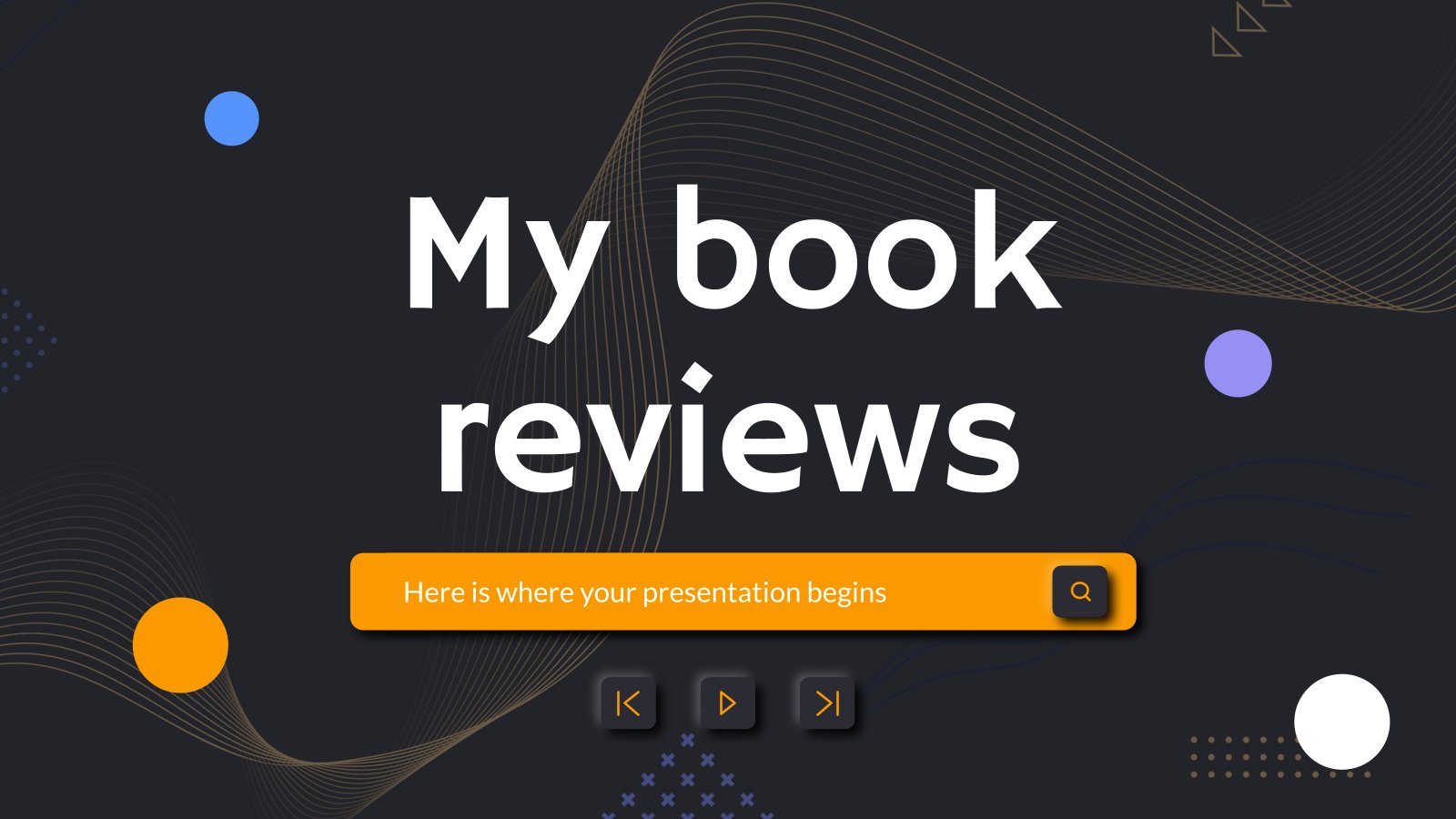
Premium template
Unlock this template and gain unlimited access
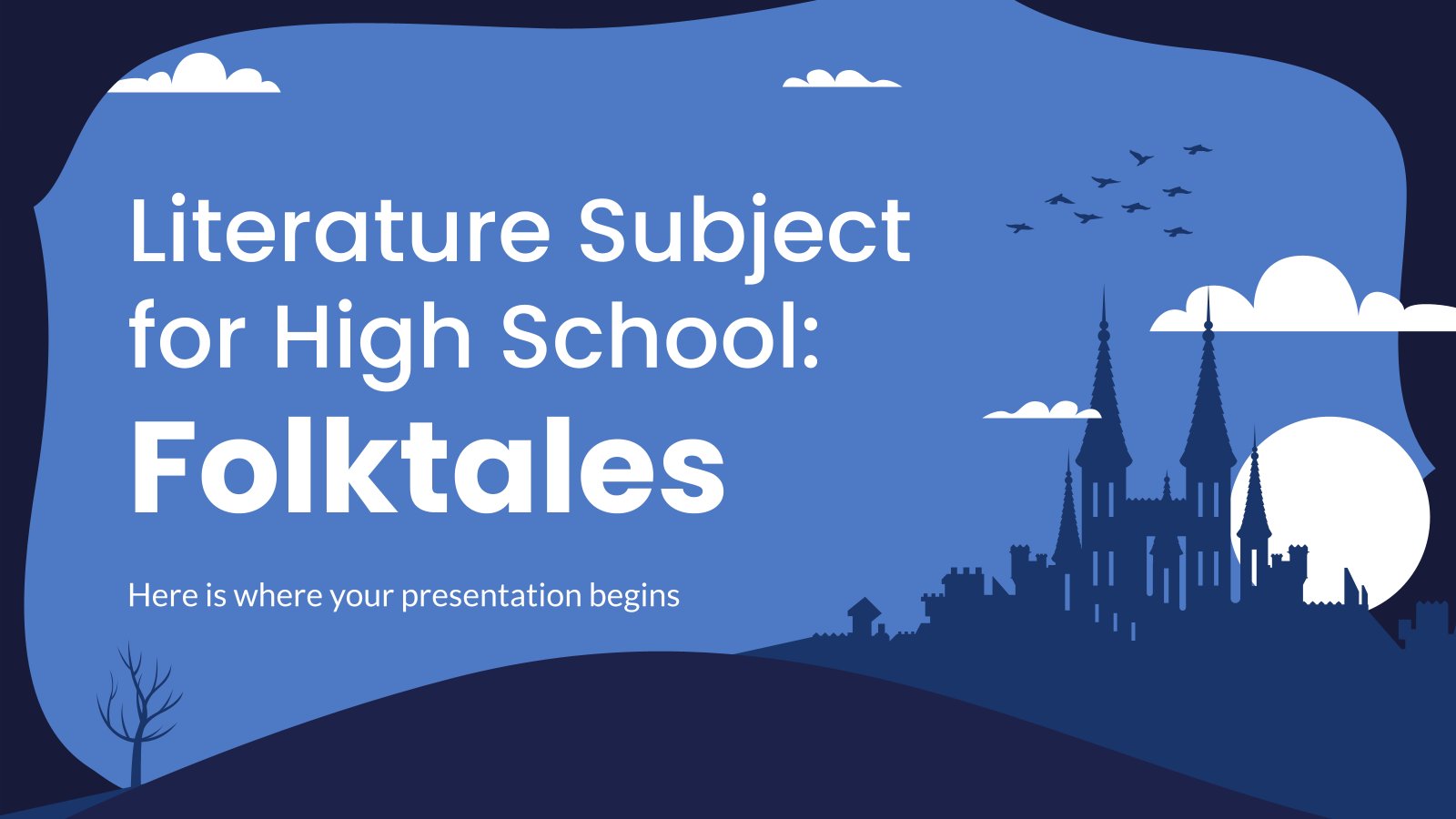
Register for free and start editing online
Dynamic Security Analysis on Android: A Systematic Literature Review
Ieee account.
- Change Username/Password
- Update Address
Purchase Details
- Payment Options
- Order History
- View Purchased Documents
Profile Information
- Communications Preferences
- Profession and Education
- Technical Interests
- US & Canada: +1 800 678 4333
- Worldwide: +1 732 981 0060
- Contact & Support
- About IEEE Xplore
- Accessibility
- Terms of Use
- Nondiscrimination Policy
- Privacy & Opting Out of Cookies
A not-for-profit organization, IEEE is the world's largest technical professional organization dedicated to advancing technology for the benefit of humanity. © Copyright 2024 IEEE - All rights reserved. Use of this web site signifies your agreement to the terms and conditions.
- Reference Manager
- Simple TEXT file
People also looked at
Original research article, application of mixed reality navigation technology in primary brainstem hemorrhage puncture and drainage surgery: a case series and literature review.

- 1 Department of Neurosurgery, Chongqing Emergency Medical Center, Chongqing University Central Hospital, Chongqing, China
- 2 Pre-hospital Emergency Department, Chongqing Emergency Medical Center, Chongqing University Central Hospital, Chongqing, China
- 3 Qinying Technology Co., Ltd., Chongqing, China
Objective: The mortality rate of primary brainstem hemorrhage (PBH) is high, and the optimal treatment of PBH is controversial. We used mixed reality navigation technology (MRNT) to perform brainstem hematoma puncture and drainage surgery in seven patients with PBH. We shared practical experience to verify the feasibility and safety of the technology.
Method: We introduced the surgical procedure of brainstem hematoma puncture and drainage surgery with MRNT. From January 2021 to October 2022, we applied the technology to seven patients. We collected their clinical and radiographic indicators, including demographic indicators, preoperative and postoperative hematoma volume, hematoma evacuation rate, operation time, blood loss, deviation of the drainage tube target, depth of implantable drainage tube, postoperative complications, preoperative and 1-month postoperative GCS, etc.
Result: Among seven patients, with an average age of 56.71 ± 12.63 years, all had underlying diseases of hypertension and exhibited disturbances of consciousness. The average evacuation rate of hematoma was 50.39% ± 7.71%. The average operation time was 82.14 ± 15.74 min, the average deviation of the drainage tube target was 4.58 ± 0.72 mm, and the average depth of the implantable drainage tube was 62.73 ± 0.94 mm. Among all seven patients, four patients underwent external ventricular drainage first. There were no intraoperative deaths, and there was no complication after surgery in seven patients. The 1-month postoperative GCS was improved compared to the preoperative GCS.
Conclusion: It was feasible and safe to perform brainstem hematoma puncture and drainage surgery by MRNT. The technology could evacuate about half of the hematoma and prevent hematoma injury. The advantages included high precision in dual-plane navigation technology, low cost, an immersive operation experience, etc. Furthermore, improving the matching registration method and performing high-quality prospective clinical research was necessary.
Introduction
Primary brainstem hemorrhage (PBH) is spontaneous brainstem bleeding associated with hypertension unrelated to cavernous hemangioma, arteriovenous malformation, and other diseases. Hypertension is the leading risk factor for PBH, and other elements include anticoagulant therapy, cerebral amyloid angiopathy, et al. PBH is the deadliest subtype of intracerebral hemorrhage (ICH), accounting for 6%–10% of all ICH with an annual incidence of approximately 2–4/100,000 people [ 1 – 3 ]. The clinical characteristics of PBH are acute onset, rapid deterioration, poor prognosis, and high mortality (30%–90%) [ 1 , 4 , 5 ].
The inclusion criteria of previous ICH research all excluded PBH, such as STICH and MISTIE trials. There is no clear evidence for the optimal treatment of PBH, and the view of surgical treatment has noticeable regional differences. European and North American countries generally believe that severe disability or survival in a vegetative state is a high mental and economic burden for PBH patients and their families. These countries do not favor surgical treatment. However, many PBH surgical treatments have been carried out in China, Japan, and South Korea. Surgical treatment methods, surgical effects, monitoring methods, and complications have been investigated, and much experience has been accumulated.
In 1998, Korean scholars performed the first craniotomy to evacuate the brainstem hematoma [ 6 ]. However, in 1989, the Japanese scholar Takahama performed stereotactic brainstem hematoma aspiration surgery [ 7 ]. In our opinion, microsurgery craniotomy requires high electrophysiological monitoring and surgical skills, and these limitations are not conductive to popularization. Minimally invasive surgery has the characteristics of a simple operation, minimally invasive, and short operation time, and it is believed to reduce the damage to critical brainstem structures and protect brainstem function as much as possible. More and more minimally invasive treatments have been adopted to improve the precision of PBH puncture, including stereotactic frameworks, robotic-assisted navigation systems, 3D printing techniques, and even laser combined with CT navigation techniques.
Mixed reality navigation technology (MRNT) is based on virtual and augmented reality development. The technology uses CT images to construct a 3D head model and design an individual hematoma puncture trajectory. The actual environmental position is captured by a camera during surgery and was fused with 3D head model synchronously. MRNT not only display the model image combined with actual environment but also navigate the puncture trajectory in real time, allowing the surgeon to precisely control puncture angle and depth to achieve a perfect procedure. This technology makes the head utterly transparent during the surgery and brings an immersive experience to the surgeon.
MRNT has broad application prospects. However, it is still in its infancy, and its application in neurosurgery has rarely been reported. Furthermore, there is no report on application of MRNT in the surgical treatment of PBH. In this study, we used MRNT to perform brainstem hematoma puncture and drainage surgery in seven patients with PBH to share practical experience to verify the feasibility and safety of the technology.
Materials and methods
General information.
With the approval of the Ethics Committee of the Chongqing Emergency Medical Center, we included seven patients diagnosed with PBH from January 2021 to October 2022. All underwent brainstem hematoma puncture and drainage surgery with MRNT under general anesthesia. Indications for surgery were patients who 1) were 18–80 years of age; 2) had hematoma volume greater than 5 mL and less than 15 mL; 3) had a diameter of the hematoma greater than 2 cm; 4) had hematoma deviating toward one side or the dorsal side; 5) had GCS less than 8; and 6) had surgery within 6–24 h after onset. Family members were informed and signed the consent form [ 8 ]. Exclusion criteria were patients who had 1) brainstem hemorrhage caused by cavernous hemangioma, arteriovenous malformation, and other diseases; 2) GCS >12; 3) bilateral pupil dilation; 4) unstable vital signs; 5) severe underlying disease; or 6) coagulation dysfunction.
Mixed reality navigation technology (MRNT)
All patients preparing for surgery were required to wear sticky analysis markers in the parieto-occipital region and undergo a CT scan before surgery. CT image scanning was performed with a 64-slice CT scanner (Lightspeed VCT 6, General Electric Company, United States of America). The image parameters included in the exposure were 3 mAS, the thickness was 5mm, and the image size was 512 × 512. The DICOM data were analyzed to construct the 3D model of the hematoma and head, and the volume of brainstem preoperative hematoma was calculated using software (Medical Modeling and Design System). In addition, the hematoma puncture trajectory was designed according to the constructed head model.
After general anesthesia, the sticky analysis markers were replaced with bone nail markers, keeping the same position [ 9 ]. Based on the principle of near-infrared optical navigation, the camera captured the actual space position in real-time, fused it with the markers of the 3D head model (HSCM3D DICOM), and transmitted the information to the wearable device (HoloLens). During surgery, the camera continuously tracked the position of the puncture needle to achieve navigation function. In short, the image processing software matched and fused information from camera systems and wearable device through multiple markers. When controlling the movement of surgical tools, the software also processed the dynamic tool position data and fused it with the virtual model through wireless transmission.
Surgical procedures
Hydrocephalus patients were first treated with external ventricular drainage (EVD), and the frontal Kocher point was selected as the cranial entry point. The procedures were cutting the skin, drilling the skull, cutting the dura mater, puncturing in the direction of the plane of binaural connection, fixing the drainage tube, and suturing it layer by layer.
The patient was placed in a prone position with the head frame fixed. The puncture point was 2 cm below the transverse sinus and 3 cm lateral to the midline of the hematoma side. After cutting the skin, the muscle was separated. The dura mater was cut through a drilled hole. Wearing HoloLens, the surgeon synchronously observed actual head structure and fused puncture trajectory from multiple angles and used dual-plane navigation technology [ 9 ] for hematoma puncture. After watching that the drainage tube was in place, the puncture needle was removed, and a 5 mL empty syringe was connected for suction. The drainage tube was fixed and sutured layer by layer. The head CT was reviewed immediately after the surgery, and the decision whether to inject urokinase according to the drainage tube’s position and the residual hematoma volume. Urokinase was injected from a drainage tube for 2-3 w units every 12 h, usually 4–6 times, and kept for 1.5 h before opening the tube. The retention time of the drainage tube was no more than 72 h after the surgery. The surgical procedure to apply MRNT is shown in Figure 1 .
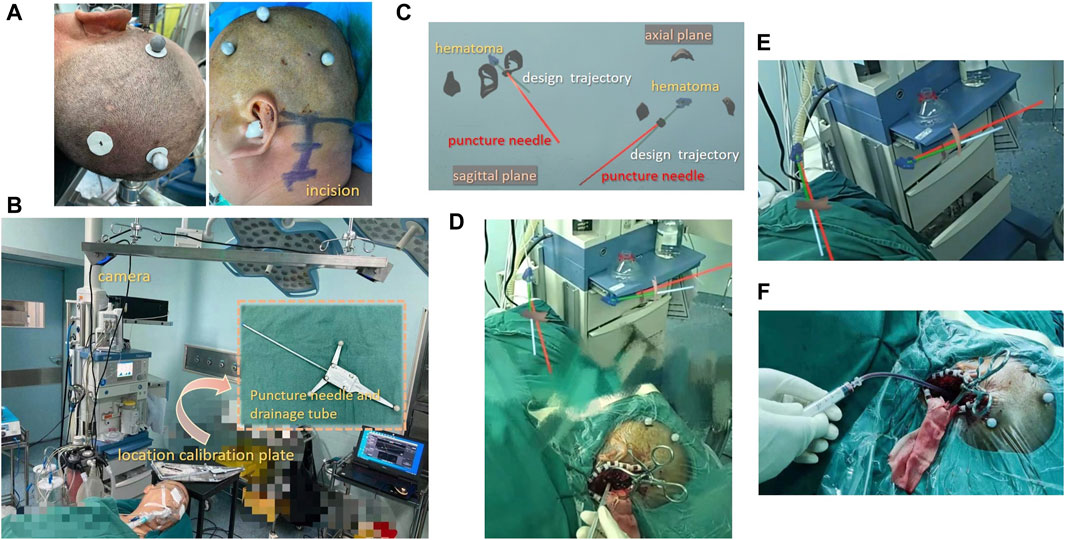
Figure 1 . Surgical procedure for brainstem hematoma puncture and drainage surgery with MRNT (A) Patients were required to wear sticky analysis markers in the parieto-occipital region. (B) The camera captured the real space position of the calibration plate, puncture needle, and head. (C) Wearing HoloLens, the surgeon viewed the two planes of the image. (D) MRNT displays the model image and the actual environment synchronously, allowing the surgeon to perform precise surgery. (E) The real-time navigation of MRNT showed that the puncture needle was close to the hematoma target. (F) The surgeon was aspirating the hematoma.
Clinical and radiographic indicators
The indicators for analysis included: demographic indicators, preoperative and postoperative hematoma volume, hematoma evacuation rate, operation time, blood loss, deviation of the drainage tube target, depth of implantable drainage tube, postoperative complications, and preoperative and 1-month postoperative GCS, etc.
The deviation of the drainage tube target was defined as the distance between the tip of the drainage tube and the planned puncture hematoma target. The deviation calculation was done with the BLENDER 2.93.3 software, which used the 3D global coordinate system to visualize the distance.
The head CT examination was reviewed within 24 h after surgery, and the postoperative hematoma volume was measured by non-operators using previous software (Medical Modeling and Design System). Hematoma evacuation rate = (preoperative hematoma volume - postoperative hematoma volume)/preoperative hematoma volume.
Statistical analysis
All statistical analyses were performed with SPSS (version 21, IBM, Chicago, IL, United States). Quantitative variables are presented as means ± standard deviations. The normality of quantitative variables was assessed through the Kolmogorov-Smirnov test. If the distribution was found to be normal, paired t -test were performed. The categorical variables are presented as percentages and tested by χ2 or Fisher’s test. A p -value less than 0.05 was considered statistically significant.
From January 2021 to October 2022, seven patients were diagnosed with PBH and underwent brainstem hematoma puncture and drainage surgery with MRNT. A summary of the demographic and clinical characteristics of the patients was provided in Table 1 . Among the seven patients, five were men, with an average age of 56.71 ± 12.63 years (37–74 years). The seven cases had underlying hypertension, and four cases had diabetes. The average time from onset to admission was 4.2 ± 1.47 h. Seven patients had prominent disturbances of consciousness, four required ventilator assistance, and three had a high fever.
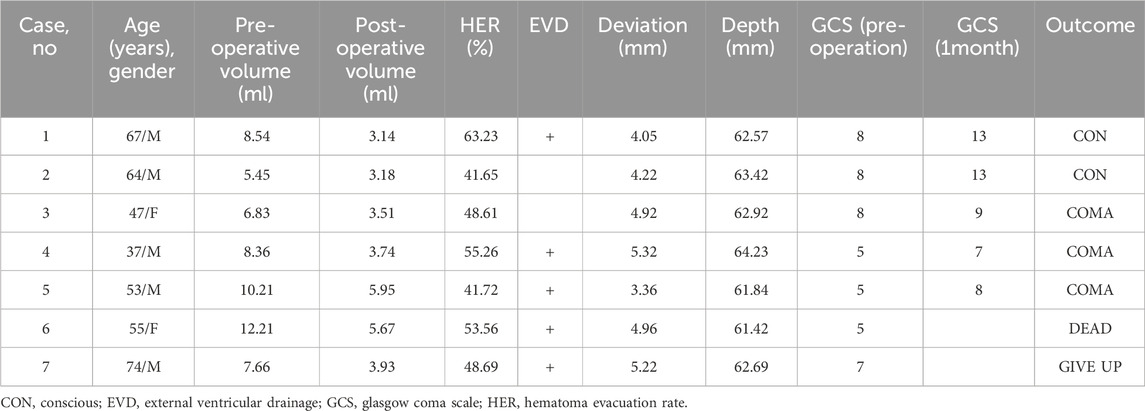
Table 1 . Demographic and clinical characteristics of seven patients.
According to the brainstem hematoma classification advocated by Chung [ 10 ], 2 cases belonged to small unilateral tegmental type, 4 cases belonged to basal-tegmental type, and other 1 case belonged to bilateral tegmental type. The average volume of preoperative brainstem hematoma was 8.47 ± 2.22 mL (range, 5.45–12.2 mL), the average volume of postoperative brainstem hematoma was 4.16 ± 1.17 mL (range, 3.14–5.95 mL), and the differences were significant. The average hematoma evacuation rate was 50.39% ± 7.71% (range, 41.65%–63.23%). Four of the seven patients underwent EVD first (57.1%), and one underwent EVD 2 days after hematoma puncture and drainage surgery. The average operation time was 82.14 ± 15.74 min, the average blood loss was 32.2 ± 8.14 mL, the average deviation of the drainage tube target was 4.58 ± 0.72 mm (range, 3.36–5.32 mm), and the average depth of the implantable drainage tube was 62.73 ± 0.94 mm (range, 61.42–64.23 mm). Three patients were injected with urokinase after surgery, and the average retention time of the drainage tube was 53.56 ± 7.83 h.
There were no intraoperative deaths in seven patients. Two patients had slight intraoperative fluctuations in vital signs. The most common postoperative comorbidity was pneumonia (7/7, 100%), followed by gastrointestinal bleeding (5/7, 71.43%). There were no rebleeding incidents, ischemic stroke, intracranial infection, or epilepsy within 2 weeks after surgery. The preoperative high fever symptoms were relieved after surgery. Only one patient died due to pneumonia 12 days after surgery, one patient gave up 20 days after surgery. Two patients were conscious and three patients were still in a coma 1 month after surgery.
The average preoperative GCS was 6.57 ± 1.51, and the average postoperative GCS was 10.00 ± 2.83 1 month after surgery. The improvement was statistically significant. The representative cases are shown in Figure 2 and Figure 3 .
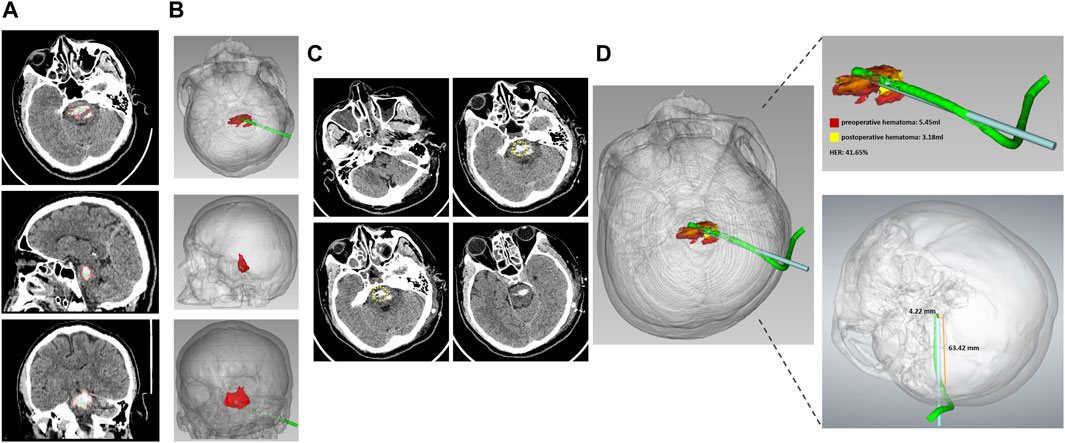
Figure 2 . The representative case 2 (A) Preoperative CT showed PBH in the axial, sagittal, and coronal planes. (B) The 3D model constructed from CT images showed hematoma and designed the puncture trajectory from the axial, sagittal, and coronary positions. (C) Postoperative CT of the axial plane showed that the drainage tube location was precise. The yellow circle indicated the tip of the drainage tube. (D) Fusion of preoperative and postoperative 3D model showed that the preoperative hematoma volume was 5.45 mL, the postoperative hematoma volume was 3.18 mL, the hematoma evacuation rate was 41.65%, the deviation of the target drainage tube was 4.22 mm, and the depth of the implantable drainage tube was 63.42 mm.
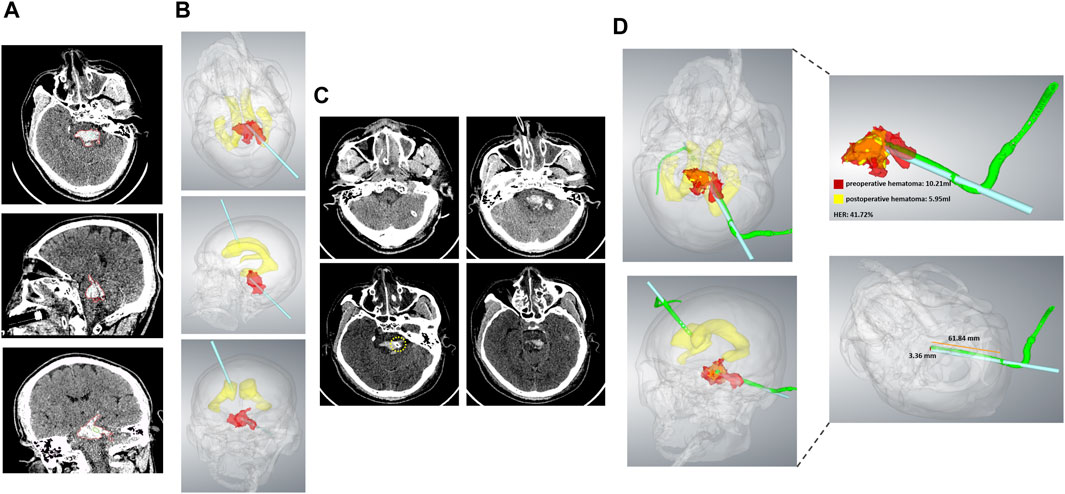
Figure 3 . The representative case 5. (A) Preoperative CT showed PBH in the axial, sagittal, and coronal planes. (B) The 3D model constructed from CT images showed hematoma, lateral ventricular, and a designed puncture trajectory from axial, sagittal, and coronary positions. (C) Postoperative CT of the axial plane showed that the drainage tube location was precise. The yellow circle indicated the tip of the drainage tube. (D) Fusion of the preoperative and postoperative 3D model showed that the preoperative hematoma volume was 10.21 mL, the postoperative hematoma volume was 5.95 mL, the hematoma evacuation rate was 41.72%, the deviation of the drainage tube target was 3.36 mm. The depth of the implantable drainage tube was 61.84 mm.
The brainstem is small, deep in the skull, and includes the midbrain, pons, and medulla oblongata. The brainstem is the center of life, controlling respiration, heart rate, blood pressure, and body temperature. About 60%–80% of PBH occurs in the pons due to the rupture of the perforating vessels of the basilar artery [ 1 , 2 ]. Hypertension is one of the most common causes of severe cerebrovascular disease. By causing mechanical and chemical damage to essential structures in the brainstem, such as the nucleus clusters and the reticular system, the hematoma quickly induces clinical symptoms such as coma, central hyperthermia, tachycardia, abnormal pupils, and hypotension. The prognosis is extremely poor, which presents a challenge to existing treatment methods.
The conservative treatment strategy for PBH is mainly related to the hypertensive treatment strategy for ICH [ 11 ]. Since the primary damage of PBH is irreversible, surgical treatment is believed to relieve mechanical compression of the hematoma and prevent secondary injury, improving prognosis [ 1 , 12 , 13 ]. However, there have been some controversies about surgical treatment. Due to the high mortality and disability rate of PBH, it is necessary to strictly evaluate the indications for surgery. Indications for surgery proposed by Shresha included a hematoma volume greater than 5 mL, a relatively concentrated hematoma, GCS less than 8, progressive neurological dysfunction, and uneventful vital signs, particularly requiring ventilatory assistance [ 14 ]. Huang established a brainstem hemorrhage scoring system and suggested patients with a score of 2–3 might benefit from surgical treatment. A score of 4 was a contraindication to surgical treatment [ 15 ]. A review of 10 cohort studies showed that the patients in the surgical group were 45–65 years old, unconscious, with a GCS of 3–8, and the hematoma volume was approximately 8 mL. The surgical group had a better prognosis and lower mortality than the conservative treatment group. The research also suggested that older age and coma were not contraindications for brainstem hemorrhage surgery [ 16 ]. According to the Chinese guidelines for brainstem hemorrhage, we specified the following surgical indications: age 18–80 years old, hematoma volume greater than 5 mL and less than 15 mL, hematoma diameter greater than 2 cm, hematoma deviated to one side or the dorsal side, GCS less than 8, surgery performed within 6–24 h after onset, and family consent [ 8 ].
The surgical treatments for PBH included microscopic craniotomy to evacuate the hematoma, which removed the hematoma as much as possible, performed hemostasis, and removed the fourth ventricular hematoma to smooth the circulation of cerebrospinal fluid. However, this technology required various intraoperative monitoring methods and proficient surgical skills. The most widely chosen method was stereotactic hematoma puncture and drainage surgery. To achieve precise puncture of the brainstem hematoma, surgeons had used invasive stereotaxic frames [ 17 ], robot-assisted navigation systems [ 18 ], the 3D printing technology navigation method [ 19 ], and laser combined with CT navigation technology [ 13 ]. The above techniques had shortcomings, including invasive placement positioning framework, the risk of skull bleeding and infection, expensive costs of robot-assisted and neuronavigation systems, the lengthy procedure of 3D printing technology, etc.
We innovatively used MRNT to perform brainstem hematoma puncture and drainage surgery. Our team used this technology to successfully perform intracranial foreign body removal [ 20 ] and minimally invasive puncture surgery for deep ICH, with a deviation of the drainage tube target of 5.76 ± 0.80 mm [ 9 ]. Based on previous experience and technical improvement, we applied technology to perform brainstem hematoma puncture and drainage surgery. The average volume of preoperative brainstem hematoma was 8.47 ± 2.22 mL, postoperative brainstem hematoma was 4.16 ± 1.17 mL, and the average hematoma evacuation rate was 50.39% ± 7.71%, which prevented hematoma primary compression and secondary injury. The surgical procedure under general anesthesia took an average of 82.14 ± 15.74 min, the average target deviation was 4.58 ± 0.72 mm, and the average depth of the implantable drainage tube was 62.73 ± 0.94 mm. The depth of the drainage tube was longer than that in the application of deep ICH, which required higher precision. Moreover, we found MRNT was safe in seven patients.
A comparison of the precision of augmented reality technology, mixed reality technology, and traditional stereotactic methods have been discussed in previous literature. Van Doormaal et al. conducted a holographic navigation study using augmented reality technology. They found that the fiducial registration error was 7.2 mm in a plastic head model, and the fiducial registration error was 4.4 mm in three patients [ 21 ]. A meta-analysis was conducted to systematically review the accuracy of augmented reality neuronavigation and compare it with conventional infrared neuronavigation. In 35 studies, the average target registration error of 2.5 mm in augmented reality technology was no different from that of 2.6 mm in traditional infrared navigation [ 22 ]. Moreover, In the study of neuronavigation using mixed reality technology, the researchers received a target deviation range of 4–6 mm [ 23 – 25 ].
The augmented reality technology application scenarios mainly involve intracranial tumors and rarely involve ICH. Qi et al. used mixed reality navigation technology to perform ICH surgery. They also used markers for point registration and image fusion. The results showed that the occipital hematoma puncture deviation was 5.3 mm due to the prone and supine position, and the deviation in the basal ganglia was 4.0 mm [ 26 ]. Zhou et al. also presented a novel multi-model mixed reality navigation system for hypertensive ICH surgery. The results of the phantom experiments revealed a mean registration error of 1.03 mm. The registration error was 1.94 mm in clinical use, which showed that the system was sufficiently accurate and effective for clinical application [ 27 ]. A summary of the deviations in the application of MR or AR was provided in Table 2 .
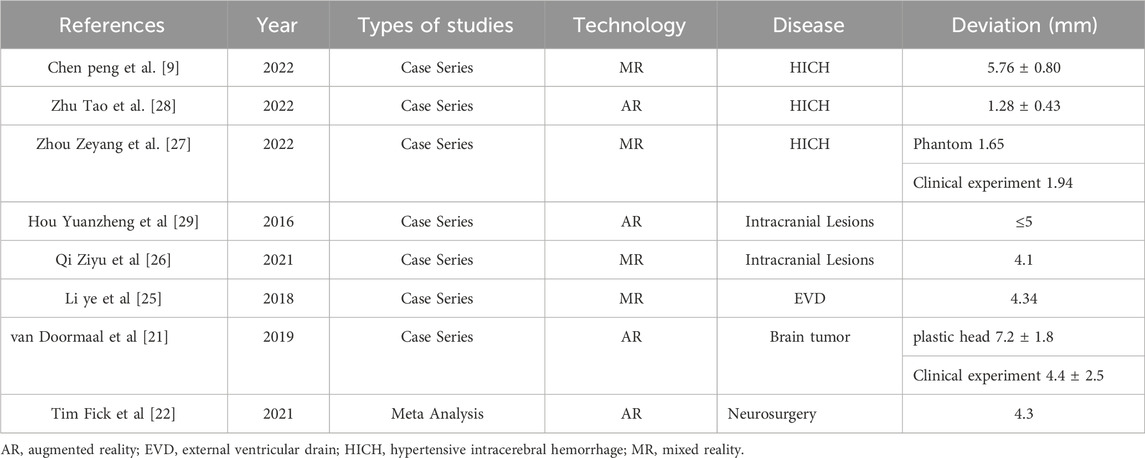
Table 2 . Reported cases of deviations in the application of MR or AR in neurosurgery.
In addition to precision puncture and hematoma drainage, surgical treatment of PBH also required further discussion on the timing of surgery, external ventricular drainage, and fibrinolytic drugs. Shrestha et al. found that surgical treatment within 6 h after onset was associated with a good prognosis [ 14 ]. The ultra-early operation alleviated the hematoma mass effect and reduced secondary injury. In particular, for patients with a severe condition, early hematoma aspiration could immediately eliminate harmful effects and prevent worse clinical outcomes [ 17 ] However, many primary hospitals are not equipped with PBH surgical treatment abilities. Patients have to waste a lot of time in the transfer process, which is a big challenge in clinical treatment. PBH can also cause cerebrospinal fluid circulation disorder that induces patients to become unconscious. External ventricular drainage is beneficial in improving cerebrospinal fluid circulation, managing intracranial pressure, and facilitating patient recovery [ 17 ]. In our study, external ventricular drainage was performed in five cases of seven patients. Previous research investigating the effects of rtPA on ICH and ventricular hemorrhage by MISTIE and CLAEA demonstrated that fibrinolytic drug administration did not increase the risk of hemorrhage [ 30 – 33 ]. Currently, there is no evidence and consensus to verify the effects of the thrombolytic drug used in PBH. We also found that urokinase did not increase the risk of bleeding and improve drainage efficiency, as reported in previous literature [ 13 , 18 ].
Compared with the expensive neuronavigation system, mixed reality navigation technology was an independent research and development project, the equipment of the technology was simple, and the cost was low. The effect of the technology met the clinical application of intracerebral hemorrhage surgery, and was beneficial to popularization for primary hospital.
There were also some limitations in our technology. Firstly, in order to introduce our innovative mixed reality navigation technology earlier and faster, we reported few cases, so there are not enough data to verify the advancement of the technology. At present, it was difficult to perform a cohort study because of the small number of patients enrolled. We plan to carry out clinical study with other centers in the future. Secondly, navigation technology was mainly based on point-matching technology, which enabled the fusion of the image model with the actual space through markers. Implementing invasive markers in the skull might carry potential risks of bleeding or infection. Moreover, the procedure required CT examinations before surgery, which delayed surgery time, and increased costs. Some researchers proposed the face registration plan, but the target deviation of the face registration was higher than that of the point registration, and the clinical practicability was poor [ 34 ]. Clinical practice must explore a precise, simple, fast, and noninvasive matching and fusion innovative solution.
It was feasible and safe to perform brainstem hematoma puncture and drainage by MRNT. Early minimally invasive precise surgery could prevent hematoma primary and secondary injury, and improve the prognosis of patients with PBH. The advantages included high precision in dual-plane navigation technology, low cost, an immersive operation experience, etc. Furthermore, improving the matching registration method and performing high-quality prospective clinical research was necessary.
Data availability statement
The original contributions presented in the study are included in the article/supplementary material, further inquiries can be directed to the corresponding authors.
Ethics statement
The studies involving humans were approved by Ethics Committee of the Chongqing Emergency Medical Center. The studies were conducted in accordance with the local legislation and institutional requirements. The participants provided their written informed consent to participate in this study.
Author contributions
XT: Writing–original draft, Data curation, Software. YaW: Writing–original draft. GT: Conceptualization, Project administration, Writing–original draft. YiW: Investigation, Resources, Software, Writing–original draft. WX: Resources, Formal Analysis, Writing–original draft, Writing–review and editing. YL: Methodology, Writing–original draft. YD: Writing–review and editing. PC: Writing–review and editing, Conceptualization, Writing–original draft.
The author(s) declare that financial support was received for the research, authorship, and/or publication of this article. This study was financially supported by the Fundamental Research Funds for the Central Universities (2022CDJYGRH-015) and Medical Research Project of Science and Technology Bureau and Health Commission, Chongqing, China (2023MSXM076).
Conflict of interest
Author YiW was employed by Qinying Technology Co., Ltd.
The remaining authors declare that the research was conducted in the absence of any commercial or financial relationships that could be construed as a potential conflict of interest.
Publisher’s note
All claims expressed in this article are solely those of the authors and do not necessarily represent those of their affiliated organizations, or those of the publisher, the editors and the reviewers. Any product that may be evaluated in this article, or claim that may be made by its manufacturer, is not guaranteed or endorsed by the publisher.
1. Chen P, Yao H, Tang X, Wang Y, Zhang Q, Liu Y, et al. Management of primary brainstem hemorrhage: a review of outcome prediction, surgical treatment, and animal model. Dis Markers (2022) 2022:1–8. doi:10.1155/2022/4293590
CrossRef Full Text | Google Scholar
2. Chen D, Tang Y, Nie H, Zhang P, Wang W, Dong Q, et al. Primary brainstem hemorrhage: a review of prognostic factors and surgical management. Front Neurol (2021) 12:727962. doi:10.3389/fneur.2021.727962
PubMed Abstract | CrossRef Full Text | Google Scholar
3. van Asch CJ, Luitse MJ, Rinkel GJ, van der Tweel I, Algra A, Klijn CJ. Incidence, case fatality, and functional outcome of intracerebral haemorrhage over time, according to age, sex, and ethnic origin: a systematic review and meta-analysis. Lancet Neurol (2010) 9:167–76. doi:10.1016/s1474-4422(09)70340-0
4. Behrouz R. Prognostic factors in pontine haemorrhage: a systematic review. Eur Stroke J (2018) 3:101–9. doi:10.1177/2396987317752729
5. Balci K, Asil T, Kerimoglu M, Celik Y, Utku U. Clinical and neuroradiological predictors of mortality in patients with primary pontine hemorrhage. Clin Neurol Neurosurg (2005) 108:36–9. doi:10.1016/j.clineuro.2005.02.007
6. Hong JT, Choi SJ, Kye DK, Park CK, Lee SW, Kang JK. Surgical outcome of hypertensive pontine hemorrhages: experience of 13 cases. J Korean Neurosurg Soc (1998) 27:59–65.
Google Scholar
7. Takahama H, Morii K, Sato M, Sekiguchi K, Sato S. Stereotactic aspiration in hypertensive pontine hemorrhage: comparative study with conservative therapy. No Shinkei Geka (1989) 17:733–9.
PubMed Abstract | Google Scholar
8. Chen L, Chen T, Mao G, Chen B, Li M, Zhang H, et al. Clinical neurorestorative therapeutic guideline for brainstem hemorrhage (2020 China version). J Neurorestoratology (2020) 8:232–40. doi:10.26599/jnr.2020.9040024
9. Peng C, Yang L, Yi W, Yidan L, Yanglingxi W, Qingtao Z, et al. Application of fused reality holographic image and navigation technology in the puncture treatment of hypertensive intracerebral hemorrhage. Front Neurosci (2022) 16:850179. doi:10.3389/fnins.2022.850179
10. Chung CS, Park CH. Primary pontine hemorrhage: a new CT classification. Neurology (1992) 42(4):830–4. doi:10.1212/wnl.42.4.830
11. Greenberg SM, Ziai WC, Cordonnier C, Dowlatshahi D, Francis B, Goldstein JN, et al. 2022 guideline for the management of patients with spontaneous intracerebral hemorrhage: a guideline from the American heart association/American stroke association. Stroke (2022) 53:e282–e361. doi:10.1161/str.0000000000000407
12. Balami JS, Buchan AM. Complications of intracerebral haemorrhage. Lancet Neurol (2012) 11:101–18. doi:10.1016/s1474-4422(11)70264-2
13. Wang Q, Guo W, Zhang T, Wang S, Li C, Yuan Z, et al. Laser navigation combined with XperCT technology assisted puncture of brainstem hemorrhage. Front Neurol (2022) 13:905477. doi:10.3389/fneur.2022.905477
14. Shrestha BK, Ma L, Lan Z, Li H, You C. Surgical management of spontaneous hypertensive brainstem hemorrhage. Interdiscip Neurosurg (2015) 2:145–8. doi:10.1016/j.inat.2015.06.005
15. Huang K, Ji Z, Sun L, Gao X, Lin S, Liu T, et al. Development and validation of a grading Scale for primary pontine hemorrhage. Stroke (2017) 48:63–9. doi:10.1161/strokeaha.116.015326
16. Zheng WJ, Shi SW, Gong J. The truths behind the statistics of surgical treatment for hypertensive brainstem hemorrhage in China: a review. Neurosurg Rev (2022) 45:1195–204. doi:10.1007/s10143-021-01683-2
17. Du L, Wang JW, Li CH, Gao BL. Effects of stereotactic aspiration on brainstem hemorrhage in a case series. Front Surg (2022) 9:945905. doi:10.3389/fsurg.2022.945905
18. Zhang S, Chen T, Han B, Zhu W. A retrospective study of puncture and drainage for primary brainstem hemorrhage with the assistance of a surgical robot. Neurologist (2023) 28:73–9. doi:10.1097/nrl.0000000000000445
19. Wang Q, Guo W, Liu Y, Shao W, Li M, Li Z, et al. Application of a 3D-printed navigation mold in puncture drainage for brainstem hemorrhage. J Surg Res (2020) 245:99–106. doi:10.1016/j.jss.2019.07.026
20. Li Y, Huang J, Huang T, Tang J, Zhang W, Xu W, et al. Wearable mixed-reality holographic navigation guiding the management of penetrating intracranial injury caused by a nail. J Digit Imaging (2021) 34:362–6. doi:10.1007/s10278-021-00436-3
21. van Doormaal TPC, van Doormaal JAM, Mensink T. Clinical accuracy of holographic navigation using point-based registration on augmented-reality glasses. Oper Neurosurg (Hagerstown) (2019) 17:588–93. doi:10.1093/ons/opz094
22. Fick T, van Doormaal JAM, Hoving EW, Willems PWA, van Doormaal TPC. Current accuracy of augmented reality neuronavigation systems: systematic review and meta-analysis. World Neurosurg (2021) 146:179–88. doi:10.1016/j.wneu.2020.11.029
23. Incekara F, Smits M, Dirven C, Vincent A. Clinical feasibility of a wearable mixed-reality device in neurosurgery. World Neurosurg (2018) 118:e422–7. doi:10.1016/j.wneu.2018.06.208
24. McJunkin JL, Jiramongkolchai P, Chung W, Southworth M, Durakovic N, Buchman CA, et al. Development of a mixed reality platform for lateral skull base anatomy. Otol Neurotol (2018) 39:e1137–42. doi:10.1097/mao.0000000000001995
25. Li Y, Chen X, Wang N, Zhang W, Li D, Zhang L, et al. A wearable mixed-reality holographic computer for guiding external ventricular drain insertion at the bedside. J Neurosurg (2018) 1–8. doi:10.3171/2018.4.JNS18124
26. Qi Z, Li Y, Xu X, Zhang J, Li F, Gan Z, et al. Holographic mixed-reality neuronavigation with a head-mounted device: technical feasibility and clinical application. Neurosurg Focus (2021) 51:E22. doi:10.3171/2021.5.focus21175
27. Zhou Z, Yang Z, Jiang S, Zhuo J, Zhu T, Ma S. Surgical navigation system for hypertensive intracerebral hemorrhage based on mixed reality. J Digit Imaging (2022) 35:1530–43. doi:10.1007/s10278-022-00676-x
28. Zhu T, Jiang S, Yang Z, Zhou Z, Li Y, Ma S, et al. A neuroendoscopic navigation system based on dual-mode augmented reality for minimally invasive surgical treatment of hypertensive intracerebral hemorrhage. Comput Biol Med (2022) 140:105091. doi:10.1016/j.compbiomed.2021.105091
29. Hou Y, Ma L, Zhu R, Chen X, Zhang J. A low-cost iPhone-assisted augmented reality solution for the localization of intracranial lesions. PLoS One (2016) 11(7):e0159185. doi:10.1371/journal.pone.0159185
30. Hanley DF, Thompson RE, Rosenblum M, Yenokyan G, Lane K, McBee N, et al. Efficacy and safety of minimally invasive surgery with thrombolysis in intracerebral haemorrhage evacuation (MISTIE III): a randomised, controlled, open-label, blinded endpoint phase 3 trial. Lancet (2019) 393:1021–32. doi:10.1016/s0140-6736(19)30195-3
31. Hanley DF, Lane K, McBee N, Ziai W, Tuhrim S, Lees KR, et al. Thrombolytic removal of intraventricular haemorrhage in treatment of severe stroke: results of the randomised, multicentre, multiregion, placebo-controlled CLEAR III trial. Lancet (2017) 389:603–11. doi:10.1016/s0140-6736(16)32410-2
32. Montes JM, Wong JH, Fayad PB, Awad IA. Stereotactic computed tomographic-guided aspiration and thrombolysis of intracerebral hematoma: protocol and preliminary experience. Stroke (2000) 31:834–40. doi:10.1161/01.str.31.4.834
33. Vespa P, McArthur D, Miller C, O'Phelan K, Frazee J, Kidwell C, et al. Frameless stereotactic aspiration and thrombolysis of deep intracerebral hemorrhage is associated with reduction of hemorrhage volume and neurological improvement. Neurocrit Care (2005) 2:274–81. doi:10.1385/ncc:2:3:274
34. Mongen MA, Willems PWA. Current accuracy of surface matching compared to adhesive markers in patient-to-image registration. Acta Neurochir (Wien) (2019) 161:865–70. doi:10.1007/s00701-019-03867-8
Keywords: primary brainstem hemorrhage, mixed reality navigation technology, brainstem hematoma puncture and drainage surgery, neuronavigation, deviation
Citation: Tang X, Wang Y, Tang G, Wang Y, Xiong W, Liu Y, Deng Y and Chen P (2024) Application of mixed reality navigation technology in primary brainstem hemorrhage puncture and drainage surgery: a case series and literature review. Front. Phys. 12:1390236. doi: 10.3389/fphy.2024.1390236
Received: 23 February 2024; Accepted: 26 March 2024; Published: 17 April 2024.
Reviewed by:
Copyright © 2024 Tang, Wang, Tang, Wang, Xiong, Liu, Deng and Chen. This is an open-access article distributed under the terms of the Creative Commons Attribution License (CC BY). The use, distribution or reproduction in other forums is permitted, provided the original author(s) and the copyright owner(s) are credited and that the original publication in this journal is cited, in accordance with accepted academic practice. No use, distribution or reproduction is permitted which does not comply with these terms.
*Correspondence: Yongbing Deng, [email protected] ; Peng Chen, [email protected]
† These authors share first authorship
This article is part of the Research Topic
Multi-Sensor Imaging and Fusion: Methods, Evaluations, and Applications – Volume II

- SUGGESTED TOPICS
- The Magazine
- Newsletters
- Managing Yourself
- Managing Teams
- Work-life Balance
- The Big Idea
- Data & Visuals
- Reading Lists
- Case Selections
- HBR Learning
- Topic Feeds
- Account Settings
- Email Preferences
6 Common Leadership Styles — and How to Decide Which to Use When
- Rebecca Knight

Being a great leader means recognizing that different circumstances call for different approaches.
Research suggests that the most effective leaders adapt their style to different circumstances — be it a change in setting, a shift in organizational dynamics, or a turn in the business cycle. But what if you feel like you’re not equipped to take on a new and different leadership style — let alone more than one? In this article, the author outlines the six leadership styles Daniel Goleman first introduced in his 2000 HBR article, “Leadership That Gets Results,” and explains when to use each one. The good news is that personality is not destiny. Even if you’re naturally introverted or you tend to be driven by data and analysis rather than emotion, you can still learn how to adapt different leadership styles to organize, motivate, and direct your team.
Much has been written about common leadership styles and how to identify the right style for you, whether it’s transactional or transformational, bureaucratic or laissez-faire. But according to Daniel Goleman, a psychologist best known for his work on emotional intelligence, “Being a great leader means recognizing that different circumstances may call for different approaches.”

- RK Rebecca Knight is a journalist who writes about all things related to the changing nature of careers and the workplace. Her essays and reported stories have been featured in The Boston Globe, Business Insider, The New York Times, BBC, and The Christian Science Monitor. She was shortlisted as a Reuters Institute Fellow at Oxford University in 2023. Earlier in her career, she spent a decade as an editor and reporter at the Financial Times in New York, London, and Boston.
Partner Center

IMAGES
VIDEO
COMMENTS
Accordingly, researchers have turned to various forms of power analyses (Cohen, 1988) and precision-based approaches (e.g., Rothman & Greenland, 2018) to select and evaluate sample sizes.In this article, we review existing facts and controversies about power and sample size adequacy, review different kinds of sample size determination methods, discuss standards for reporting them, and review ...
Power analysis is not logical when performed after the data have been collected because the probability of the outcome to be detected is no longer random. In a simulation study, Zhang et al. showed that post hoc power estimates are misleading and can be very different from true power estimates. ... Conduct a thorough literature review to get ...
The power analysis is performed by some specific tests and they aim to find the exact number of population for a clinical or experimental study . In fact, there are two situations while testing the hypothesis in a clinical trial. These are null hypothesis (H 0) and alternative hypothesis (H 1). Null hypothesis always argues that there is no ...
The general literature review is a synthesis and analysis of published research on a relevant clinical issue, and is a common format for academic theses at the bachelor's and master's levels in nursing, physiotherapy, occupational therapy, public health and other related fields. ... - Experimental study - Power calculation used to estimate ...
Examples of literature reviews. Step 1 - Search for relevant literature. Step 2 - Evaluate and select sources. Step 3 - Identify themes, debates, and gaps. Step 4 - Outline your literature review's structure. Step 5 - Write your literature review.
The total sample size for the study with r = 1 (equal sample size), a = 5% and power at 80% and 90% were computed as and for 90% of statistical power, the sample size will be 32. In unequal sample size of 1: 2 ( r = 0.5) with 90% statistical power of 90% at 5% level significance, the total sample size required for the study is 48.
There are at least three ways to guestimate the values that are needed to do a power analysis: a literature review, a pilot study and using Cohen's recommendations. We will review the pros and cons of each of these methods. For this discussion, we will focus on finding the effect size, as that is often the most difficult number to obtain and ...
a description of the publication. a summary of the publication's main points. an evaluation of the publication's contribution to the topic. identification of critical gaps, points of disagreement, or potentially flawed methodology or theoretical approaches. indicates potential directions for future research.
Lecture 17 Inputs for Power Analysis: Literature Review 2 Measurements taken from a given patient at months zero, six, and 12 were correlated with one another. To design a study, specify four types of information 4 1. Design 2. Statistical test 3. Criterion 4. Five key inputs for power or sample size analysis Kreidler et al., 2013
A formal literature review is an evidence-based, in-depth analysis of a subject. There are many reasons for writing one and these will influence the length and style of your review, but in essence a literature review is a critical appraisal of the current collective knowledge on a subject. Rather than just being an exhaustive list of all that ...
Plan your literature review and document your progress. Identify questions of interest, such as unknown correlations or standards of design in a given field. Be careful to not reuse an inappropriate design or analysis, such Measurements as ignoring taken from clustering. a given patient at months zero, six, and 12 were correlated with one another.
Statistical power and sample size analysis provides both numeric and graphical results, as shown below. The text output indicates that we need 15 samples per group (total of 30) to have a 90% chance of detecting a difference of 5 units. The dot on the Power Curve corresponds to the information in the text output.
A literature review can broadly be described as a more or less systematic way of collecting and synthesizing previous research (Baumeister & Leary, 1997; ... a literature review can address research questions with a power that no single study has. ... designing the review, (2) conducting the review, (3) analysis and (4) writing up the review.
A literature review is an integrated analysis-- not just a summary-- of scholarly writings and other relevant evidence related directly to your research question. That is, it represents a synthesis of the evidence that provides background information on your topic and shows a association between the evidence and your research question.
4.0 Inputs for Power Analysis: Literature Review. In this lesson, we will talk about what inputs are needed for power and sample size analysis and discuss how to find these inputs through a literature review process. Some questions to consider while completing this lesson include: How many key inputs are there for power or sample size analysis?
In what appears to be the first article in nursing on integrative reviews, Ganong 9 described this methodology as including stages of problem formulation, literature search, data evaluation, data analysis, and presentation of findings. Ganong emphasized that these reviews needed to be held to the same standards of quality as primary research.
The lit review is an important genre in many disciplines, not just literature (i.e., the study of works of literature such as novels and plays). When we say "literature review" or refer to "the literature," we are talking about the research (scholarship) in a given field. You will often see the terms "the research," "the ...
A literature review is a critical analysis and synthesis of existing research on a particular topic. It provides an overview of the current state of knowledge, identifies gaps, and highlights key findings in the literature. 1 The purpose of a literature review is to situate your own research within the context of existing scholarship, demonstrating your understanding of the topic and showing ...
A review of the literature and evaluation of studies conducted in recent years shows that many solutions have been introduced to cyberattacks detection in the power systems so that machine learning and deep learning methods have found a special place in this field [79,80,81,82,83].
Introduction and Literature Review of Power System Challenges and Issues. October 2021. DOI: 10.1007/978-3-030-77696-1_2. In book: Application of Machine Learning and Deep Learning Methods to ...
The analysis was conducted during 20 days between February and March 2019 by an external consultant. It consists of an internal and external literature review of previous gender analysis, assessments and country profiles focusing on the socio-economic empowerment of women and girls,
This fully customizable Google Slides and PowerPoint template can assist you in structuring your review seamlessly. Featuring a vibrant yellow design with captivating book illustrations, this template is designed to facilitate the organization and presentation of your research. Navigate your audience through chapters, themes, and references ...
This study proposes a semi-systematic literature review of the available knowledge on the adoption of artificial intelligence (AI) in the higher education system. It presents a stakeholder-centric analysis to explore multiple perspectives, including pedagogical, managerial, technological, governmental, external, and social ones.
Literature reviews are essential in moving our evidence-base forward. "A literature review makes a significant contribution when the authors add to the body of knowledge through providing new insights" (Bearman, 2016, p. 383).Although there are many methods for conducting a literature review (e.g., systematic review, scoping review, qualitative synthesis), some commonalities in ...
This paper conducts a literature analysis through Citespace on carbon emission, carbon emissions in power systems, and the theoretical models of the carbon emission analysis method for power systems. ... The exclusion criteria for journal articles or reviews on carbon emissions and carbon flows in power systems published through peer review are ...
Aspect-based sentiment analysis (ABSA) is a fine-grained type of sentiment analysis; it works on an aspect level. It mainly focuses on extracting aspect terms from text or reviews, categorizing the aspect terms, and classifying the sentiment polarities toward each aspect term and aspect category. Aspect term extraction (ATE) and aspect category detection (ACD) are interdependent and closely ...
In this study, based on an approach integrating bibliometrics and a literature review, we systematically analyzed peatland research from a literature perspective. ... Wang, Yao Xiao, Guojie Hu, and et al. 2024. "Research Progress in the Field of Peatlands in 1990-2022: A Systematic Analysis Based on Bibliometrics" Land 13, no. 4: 549 ...
Dynamic analysis is a technique that is used to fully understand the internals of a system at runtime. On Android, dynamic security analysis involves real-time assessment and active adaptation of an app's behaviour, and is used for various tasks, including network monitoring, system-call tracing, and taint analysis. The research on dynamic analysis has made significant progress in the past ...
Figure 1.Surgical procedure for brainstem hematoma puncture and drainage surgery with MRNT (A) Patients were required to wear sticky analysis markers in the parieto-occipital region.(B) The camera captured the real space position of the calibration plate, puncture needle, and head.(C) Wearing HoloLens, the surgeon viewed the two planes of the image.(D) MRNT displays the model image and the ...
6 Common Leadership Styles — and How to Decide Which to Use When. by. Rebecca Knight. April 09, 2024. Carol Yepes/Getty Images. Summary. Research suggests that the most effective leaders adapt ...