Land use/land cover change in Orange County, North Carolina from 1955 to 2001
Add to collection, downloadable content.
- Land use land cover change in Orange County, North Carolina from 1955 to 2001
- March 21, 2019
- Affiliation: College of Arts and Sciences, Department of Geography
- Land use/land cover change (LULCC) has significant implications in terrestrial ecosystem goods and services. Current studies on LULCC are primarily based on space-borne satellite images. These studies are limited by the data availability. In this study, I extend the land-use land cover change analysis back to 1955 for Orange County, North Carolina based on historical aerial photos. I also analyzed the spatial configuration of the landscapes based on pattern matrix analysis and geospatial analysis. Results show that the urban area increased from 11.31 km2 to 128.15 km2 from; the agricultural area decreased from 335.53 km2 to 259.81 km2; the forest area decreased from 676.95 km2 to 632.45 km2. The LULCC change is associated with land configuration and composition changes. Pattern metrics analysis shows that in 2001, Orange County has more scattered land use patches and smaller patch sizes than in 1955 and 1975. Semi-vairograms generated for conifer and hardwoods are changing both in shape and the key characteristic parameters with time. These results can provide essential information for land management and planning to achieve sustainable development of Orange County in the future.
- August 2010
- https://doi.org/10.17615/awjy-zt84
- Masters Thesis
- In Copyright
- "... in partial fulfillment of the requirements for the degree of Master of Arts in the Department of Geography."
- Song, Conghe
- University of North Carolina at Chapel Hill
- Chapel Hill, NC
- Open access
- March 18, 2013
This work has no parents.
Thumbnail | Title | Date Uploaded | Visibility | Actions |
---|---|---|---|---|
2019-04-12 | Public |

Select type of work
Master's papers.
Deposit your masters paper, project or other capstone work. Theses will be sent to the CDR automatically via ProQuest and do not need to be deposited.
Scholarly Articles and Book Chapters
Deposit a peer-reviewed article or book chapter. If you would like to deposit a poster, presentation, conference paper or white paper, use the “Scholarly Works” deposit form.
Undergraduate Honors Theses
Deposit your senior honors thesis.
Scholarly Journal, Newsletter or Book
Deposit a complete issue of a scholarly journal, newsletter or book. If you would like to deposit an article or book chapter, use the “Scholarly Articles and Book Chapters” deposit option.
Deposit your dataset. Datasets may be associated with an article or deposited separately.
Deposit your 3D objects, audio, images or video.
Poster, Presentation, Protocol or Paper
Deposit scholarly works such as posters, presentations, research protocols, conference papers or white papers. If you would like to deposit a peer-reviewed article or book chapter, use the “Scholarly Articles and Book Chapters” deposit option.
- Open access
- Published: 14 March 2024
Assessment of land use land cover change and its effects using artificial neural network-based cellular automation
- Nishant Mehra ORCID: orcid.org/0000-0001-6069-8103 1 &
- Janaki Ballav Swain 1
Journal of Engineering and Applied Science volume 71 , Article number: 70 ( 2024 ) Cite this article
1569 Accesses
6 Citations
Metrics details
The challenge of urban growth and land use land cover (LULC) change is particularly critical in developing countries. The use of remote sensing and GIS has helped to generate LULC thematic maps, which have proven immensely valuable in resource and land-use management, facilitating sustainable development by balancing developmental interests and conservation measures. The research utilized socio-economic and spatial variables such as slope, elevation, distance from streams, distance from roads, distance from built-up areas, and distance from the center of town to determine their impact on the LULC of 2016 and 2019. The research integrates Artificial Neural Network with Cellular Automta to forecast and establish potential land use changes for the years 2025 and 2040. Comparison between the predicted and actual LULC maps of 2022 indicates high agreement with kappa hat of 0.77 and a percentage of correctness of 86.83%. The study indicates that the built-up area will increase by 8.37 km 2 by 2040, resulting in a reduction of 7.08 km 2 and 1.16 km 2 in protected and agricultural areas, respectively. These findings will assist urban planners and lawmakers to adopt management and conservation strategies that balance urban expansion and conservation of natural resources leading to the sustainable development of the cities.
Introduction
The demographic projections suggest that the Central and Southern Asia are poised to emerge as the world’s most populous region by 2037 [ 1 ]. Furthermore, India surpassed China to become the most populous country in the year 2023, and prevailing indications anticipate the persistence of this demographic trend for several decades [ 2 ]. The unrestrained expansion of built-up areas is majorly propelled by a substantial increase in population which ultimately leads to land use land cover (LULC) changes [ 3 , 4 , 5 ].
The significant characteristics of urban sprawl are a rapid decrease in vegetated areas [ 6 , 7 ], random and unplanned growth [ 8 , 9 ], increased economic activities in higher elevations [ 10 , 11 , 12 ], land cover change in agricultural areas [ 13 , 14 , 15 , 16 ], and increase in urban heat island [ 17 , 18 , 19 ]. This has created environmental, ecological, economic, and social challenges [ 8 ]. The changes, geographical and climatic, occurring in Himalayan cities call for special attention due to the geo-morphological, topographical, and seismic constraints [ 7 , 10 , 20 , 21 ]. Thus, the monitoring of spatio-temporal expansion of the cities and accurate prediction of LULC change is vital for ecosystem conservation and sustainable development management strategies to be implemented in these regions [ 22 ]. As per the year-wise records shared by the Department of Economics and Statistics, State Government of Himachal Pradesh in India, the class III cities having a population of less than 50,000 in the state were found to be more vulnerable to urban sprawl due to saturation in capital city Shimla, and thus, there is a pressing need to balance economic development with sustainable environmental practices.
The integrated use of remote sensing and GIS has helped immensely in the management of land and natural resources and in understanding the complex linkages between spatial patterns and processes responsible for change [ 7 , 23 , 24 , 25 ]. Thus, the modeling and accurate prediction of urban sprawl has been inviting the attention of various researchers [ 26 , 27 ], and the use of modern self-learning algorithms has further improved the accuracy of these models [ 28 , 29 , 30 , 31 ]. The understanding of dynamic changes occurring in the region and the incorporation of driving factors also improves the accuracy of these models [ 26 ].
Cellular automata (CA)-based models are spatially explicit models (SEM) that work on a simple premise that the future state of a land cover type is dependent on the past local interactions between the different land covers [ 22 , 26 ]. The model’s popularity in GIS grew immensely in the 1980s, catalyzed by pivotal contributions from Wolfarm [ 32 ], Michael Batty and Xie [ 33 ], and Batty et al. [ 34 ]. The accuracy of the model was dependent upon the temporal scale of maps, neighboring cells, and transition rules [ 35 , 36 ]. Batty [ 34 ], Leao [ 37 ], and Lagarias [ 38 ] found them to be powerful spatial dynamic models. The open structure, simplicity, good spatial resolution, and integration with other knowledge-driven models make it an appropriate choice for urban sprawl studies [ 22 , 26 , 35 , 39 ]. However, the model is dependent upon spatial data only and is limited in implementing driving forces which is important for complex processes and accurate simulation [ 22 , 26 ]. The non-uniform cell space, dynamic neighborhood classes, and non-stationary transition rules offer opportunities for modification in the original CA structure to make it applicable for real-time complex urban sprawl studies [ 22 , 35 ]. This makes it necessary to integrate CA with other models.
To address the inherent constraints in the individual models, various researchers have employed hybrid models like CA–Markov model [ 40 ] and CA-ANN model [ 41 ]. The integration of spatial patterns with the processes responsible for causing changes in landforms is imperative for the accurate prediction and modeling of land cover changes [ 24 ]. Artificial neural networks (ANN) can identify and analyze the complex inter-relationship between causative factors and complex patterns [ 26 , 42 ]. The architecture of ANN simulates and behaves in a similar pattern as the human brain and nervous system [ 43 , 44 , 45 ]. ANN can deal with incomplete data, does not assume the distribution of input data, and can detect potential inter-dependencies between driving factors [ 46 , 47 ]. Multi-layer perceptron (MLP)-ANN, consists of input layers, hidden layers, and an output layer, and is the widely used model in ANN because it is fast, accurate, and can infer and forecast outcomes derived from inputs that it has not encountered previously, exhibiting the capacity for extrapolation and prognostication [ 48 ]. Researchers have adeptly employed CA-ANN models to address spatial-dynamic complexities and driving factors, enhancing the robustness and realism of modeling for accurate prediction and estimation of land cover changes [ 18 , 39 , 42 , 49 , 50 ].
The study aims to model LULC change using MLP-ANN and cellular automation simulation in the city of Dharamshala, one of the fastest-growing cities in the state of Himachal Pradesh, India. The results are expected to act as a road map for urban planners and policymakers for sustainable development of the city. The research used the MOLUSCE plugin, as a tool to predict and assess the transformations occurring in each LULC type in the study area. In the study, LULC maps of 2016 and 2019 were used as independent variables in the model to simulate and validate the LULC map of 2022, and thereafter, LULC maps of 2025 and 2040 were predicted.
The research locale encompasses Dharamshala, situated in the state of Himachal Pradesh, India, as illustrated in Fig. 1 . Positioned within the Western Himalayas, the city graces the southern inclines of the principal regional Dhauladhar mountain range (V. Gupta et al., [ 51 ]). Geographically, the study vicinity spans from 32° 9′ 52″ N to 32° 15′ 58″ N in latitude and 76° 17′ 22″ E to 76° 23′ 09″ E in longitude, encompassing an expanse of 42.7 km 2 . Elevation within this area exhibits variability, ranging from 790 m in the southwest to an altitude of 2130 m above mean sea level (AMSL) in the north. The region has a humid subtropical climate and experiences a mean annual temperature of about 19.1 ± 0.5 °C. The zenith of temperature occurs in June with an average of 32 °C, while the nadir registers in January with an average of 10 °C. The northern parts of the region also receive heavy snowfall during winter. Geologically, the region forms a part of the Outer Himalayas with a predominant geological composition comprising sandstone, characterized by alternating bands of clays, shale, and siltstones (V. Gupta et al., [ 51 ]).
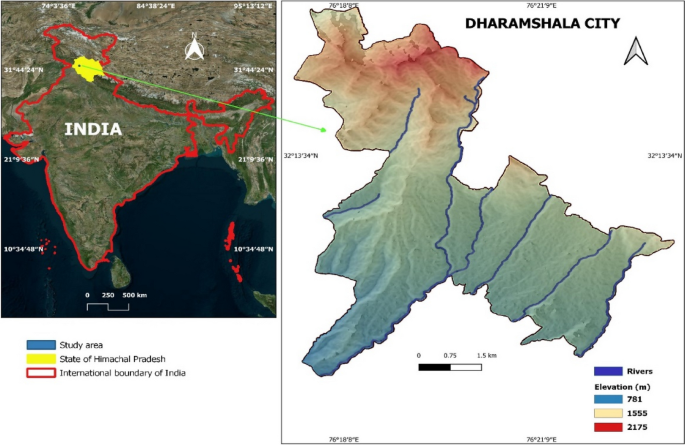
Study area, Dharamshala city
The city is the winter capital of the state of Himachal Pradesh and the headquarters of the Central Tibetan Administration. The city is a famous hill station destination, both for national and international visitors. Further, it is also the administrative headquarters of Kangra district. The city was declared a municipal corporation in the year 2015 by merging 9 adjacent villages and has ever since witnessed rapid urbanization. It is one among the 100 cities in India and the only city in the state of Himachal Pradesh chosen in the year 2016 to be developed under the National Smart Cities Mission by the Government of India.
A dramatic rise in urban spaces has been witnessed in the city from the year 2016 onwards, and there exists an inherent imperative to address the recent alterations that have manifested within this geographical area through a scientific lens. The time scale chosen in the study corresponds to the maximum socio-economic changes occurring in the city due to the formation of municipal limits, hosting of international cricket matches and also serving as the residence of His Holiness Dalai Lama.
The simulation’s correctness is determined by the quality of the data and criteria used in the investigation [ 26 , 35 , 39 ]. The month of May is characterized by sunny days with no or little rainfall in the region; thus, all the temporal satellite imageries were chosen from this month to negate the impacts of phenological effects and cloudy pixels [ 52 ]. The ancillary data included a draft town and country planning (TCP) report of Dharamshala city and ground truth points (using GPS) for assistance and validation in image classification.
The study incorporated LULC maps of 2016, 2019, and 2022 and digital elevation model (DEM), the details of which are given in Table 1 . Multi-temporal Landsat 8 Operational land Imager (OLI) satellite imageries for the years 2016, 2019, and 2022 were used, the description of which is shown in Table 2 . A hybrid approach involving a Maximum Likelihood Classifier (MLC) and thereafter adopting post-classificaton improvement measures using vegetation indices was used in the research study to create LULC maps of 2016, 2019, and 2022 with each LULC map attaining an overall accuracy surpassing 85% and kappa hat showing substantial agreement. The selection of the Maximum Likelihood Classifier was based on the topographical challenges and spectrally homogeneous attributes of the land cover classes under investigation. The correction of the land cover classes through visual interpretation becomes essential by utilizing high-resolution satellite imagery obtained from Google Earth and Planet Scope [ 53 , 54 ].
The riverine sources, in this part of the Himalayan region, are characterized by the presence of boulders and cobbles, and thus, the chances of overlapping spectral characteristics for the built-up areas and water bodies were likely. The Strahler order algorithm available in SAGA was used to accurately delineate the water bodies.
Various researchers have included slope, elevation, and aspect, as geospatial parameters; population density as the socio-economic parameter; and spatial variables such as distance from the water bodies, roads, built-up areas, and from the center of town for simulation [ 18 , 30 , 31 , 39 , 42 , 49 , 50 ]. After checking different combinations of socio-economic and physical factors, the simulated LULC map of 2022 showed the best performance by considering five parameters that included slope, distance from streams, distance from roads, distance from built-up areas, and distance from the center of town. The explanatory maps having the shp data format were converted to a raster and then Euclidean distance was calculated in QGIS to create a raster data type. The explanatory maps in GeoTIFF format were also created using Euclidean distance in QGIS.
The methodological workflow for the area under investigation is summarized in Fig. 2 . The MOLUSCE plugin available in QGIS 2.18 was used for the simulation of land cover change in 2022.
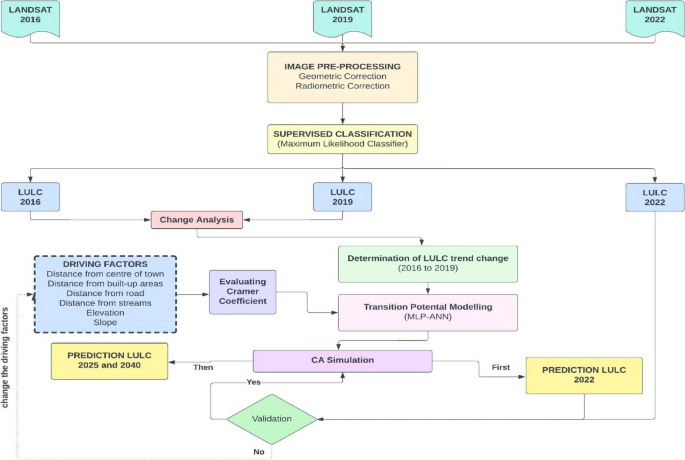
Methodological workflow and data analysis
The transition probabilities derived from MLP-ANN learning processes are fed into CA to predict and estimate the LULC changes in this hybrid model of CA-ANN [ 31 , 49 ].
Image pre-processing
The satellite imageries of 2016, 2019, and 2022 were transformed to spectral radiance values, and the Dark Object Subtraction (DOS) in the semi-automatic classification (SCP) plugin in QGIS was used for performing atmospheric correction. Thereafter, the images were mosaicked, and an image subset was performed using the shapefile of the municipal corporation limits of Dharamshala city. The shape file of municipal limits was geometrically corrected with the use of ground control points (GCP) selected using GPS. This was executed in a manner that ensured the Root mean Squared Error (RMSE) attained a value of less than half of a pixel [ 55 ].
Modified Anderson’s LULC classification system was adopted to produce thematic maps comprising five LULC classes, Protected areas (PA), Agricultural areas (AA), Built-up Areas (BA), Barren land (BL), and Water bodies (WB), as shown in Table 3 , for the years 2016, 2019, and 2022. Supervised classification using MLC was used for the creation of the five land cover classes [ 7 , 20 , 53 , 56 , 57 ]. The forests are protected under Indian Forest Act, 1927, and the tea plantations are protected under Himachal Pradesh Ceiling on Land Holdings Act, 1972, and thus were classified under the protected areas (PA).
The LULC maps for 2016 and 2019 are taken as input and establish the spatio-temporal dynamics of the region. The MOLUSCE plugin was used to create a transition map between 2016 and 2019 showing the percentage change occurring in each of the five land cover types for the period from 2016 to 2019.
For using the CA model, the region should be a discrete grided area, with each cell specifying a land cover type. The driving factors could be categorized as having different spatial attributes, such as distance parameters, physical properties, and neighborhood relationships [ 58 ]. The distance parameter includes distance from the streams, roads, built-up areas, and from the center of town. Physical properties include slope and elevation. Neighborhood relationships involve the percentage area of a land cover type around the cell of interest. The explanatory maps are extracted in a raster format (Fig. 3 ).
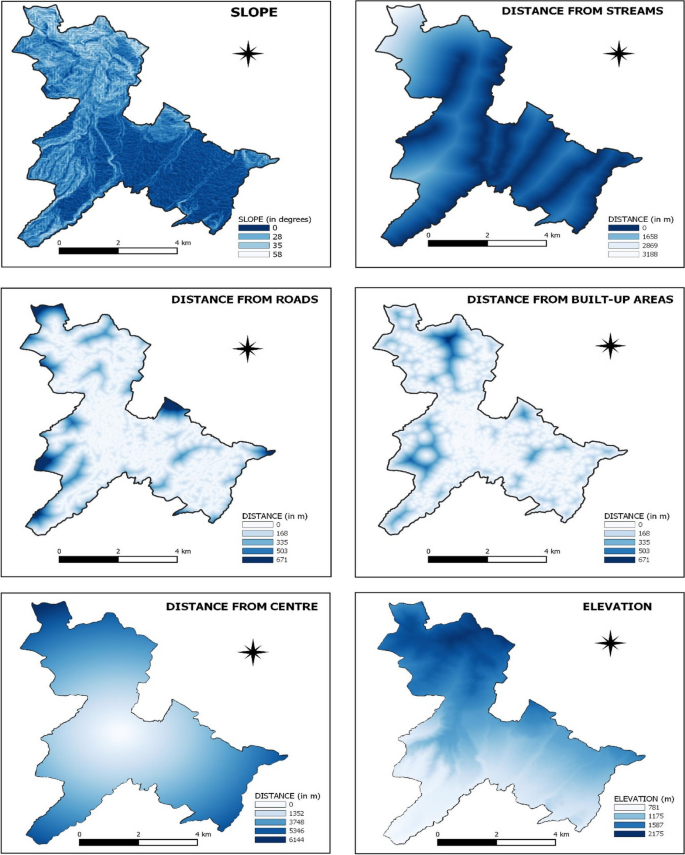
Explanatory map: slope, distance from streams, distance from roads, distance from built-up areas, distance from the center, and elevation
The transition functions are non-linear and represent the relationship between driving factors and transformation probabilities of land cover type [ 26 , 39 ]. ANN model is trained on explanatory maps, and then the transition probabilities are established for the CA model. The prediction of transition probabilities from the current land use type to different LULC categories at the subsequent time point, denoted as “ t + 1,” was determined by taking into account the current LULC classification of a specific cell as well as the neighboring cells at time t .
Based on spatio-temporal dynamics and the impact of driving factors, the simulation is initially performed for the year 2022, and based on the performance of the model, the predictions are thereafter made for the years 2025 and 2040 in the iterative steps of two and six, respectively, in the model.
Evaluating correlation and transition analysis
The examination of correlation among the driving factors was executed using the Cramer coefficient, also known as the Cramer V method, particularly suitable for contingency tables larger than 2 × 2. The outcomes span a range of 0 to 1, where elevated values signify a heightened correlation amid the driving factors. A coefficient surpassing 0.15 indicates a substantial explanatory potency of variables [ 49 ]. The correlation matrix is shown in Table 4 .
The changes (in area and percentage) occurring in the land cover classes for the period 2016 to 2019 are shown in Table 5 . The transition matrix, shown in Table 6 , helps compare and understand temporal transformations occurring in the region, without the impact of physical and socio-economic driving factors. Within the matrix’s diagonal, the constituent elements signify the magnitude of class constancy, portraying the persistence of specific land cover categories. Conversely, the off-diagonal entries encapsulate the dimensions of shifts occurring between distinct classes [ 18 ]. The values proximate to 1 are present in the diagonal entries, signifying the stability of the corresponding land cover types for the chosen period.
Transition potential modeling
The transformations occurring in a region are a highly complex process dependent on spatio-temporal changes and driving factors responsible for the changes [ 26 , 31 ]. The geographical phenomenon although non-linear and stochastic but have fractal properties [ 59 ] and machine learning algorithms, like MLP-ANN, can be very useful in the identification of these changes [ 45 , 60 ]. The transition function pertaining to the alteration in LULC delineates the association linking the driving factors with the probabilities of conversion, specifically discerning whether cells will shift towards a particular land use/cover classification. The multi-layer feed-forward approach of the model is trained using the error back propagation, wherein the network parameters are modified as per the output error demands [ 48 , 58 , 61 ]. The learning curve for the ANN-MLP is shown in Fig. 4 .
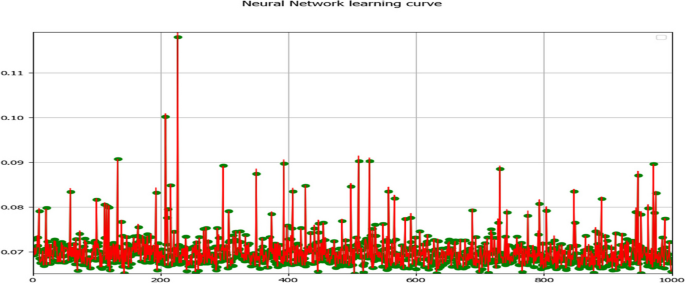
Neural network learning curve
In LULC simulation, the cross-tabulation matrix, also referred to as a contingency table, error matrix, or confusion matrix, stands as an extensively utilized approach for the evaluation of outcomes [ 62 ]. Cross-tabulation facilitates a comparative analysis between the outcomes projected by the model and the observed outcomes [ 63 ]. In this matrix, each row corresponds to the anticipated category, while each column signifies the factual category, thereby showcasing discrepancies in the cells, often expressed as errors represented in percentages or areas [ 27 , 64 ].
The assessment of accuracy was conducted utilizing overall accuracy and kappa hat statistics as the metrics of evaluation. Both metrics use the confusion matrix for calculation purposes. The determination of overall accuracy involves the consideration of diagonal elements only within the confusion matrix, while the kappa hat also considers non-diagonal elements and thus incorporates omission and commission errors [ 64 ]. Kappa hat evaluates the land modeling performance excluding chance agreement [ 65 ], with values ranging from 0.41 to 0.60 categorized as “moderate agreement” and 0.61 to 0.80 as “substantial agreement” [ 27 , 66 ].
Several simulations with different combinations of exploratory maps were performed, as shown in Table 7 . The combination consisting of the parameters distance from built-up areas, distance from roads, distance from the center of town, elevation, slope, and distance from streams showed the maximum accuracy and was chosen in the research study to prognosticate the LULC for the year 2022. The simulated and actual maps were compared with the accuracy metric kappa having a value of 0.77 denoting a notable concordance between both the maps and accuracy was found to be 86.83%. It can be concluded from these that the explanatory variables chosen had a great influence on the prediction of LULC classes. The maps for the years 2025 and 2040 were predicted after running two and seven iterations in CA, respectively.
Results and discussion
The LULC distribution for the years 2016, 2019, and 2022 is shown in Table 8 . Table 9 shows the transition undergoing area-wise and percentage-wise for each LULC class from 2016 to 2019 and 2019 to 2022. The positive values show the increase in that land cover class, while the negative values indicate the decrease for a particular land cover class. The spatio-temporal distribution of LULC classes for the years 2016, 2019, and 2022 are shown in Fig. 5 . It can be observed that protected areas had undergone the maximum transition from the year 2016 to 2022 with a reduction of 11.85% and a decrease of 5.04 km 2 in area. The built-up areas had increased considerably by 14.54% and 6.18 km 2 in area. The agricultural areas had also decreased by 2.73% and 1.16 km 2 in area and a slight increase in barren land is also observed. This signifies the impact of anthropogenic and socio-economic activities in the city and the rapid conversion of this hill station into a concrete jungle. The results also indicate widespread encroachments and abeyance of legislation.
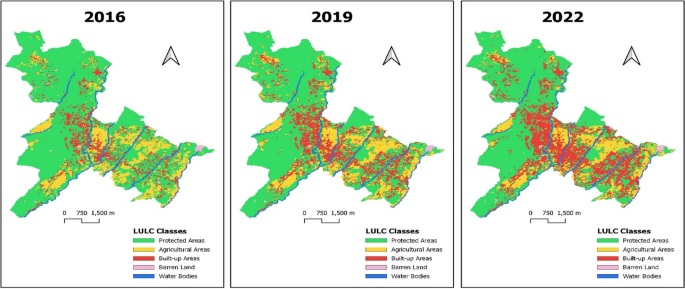
LULC maps for the years 2016, 2019, and 2022
The increase in built-up areas and barren land for the period 2016–2022 is primarily related to the increasing human population and tourist inflow in the city, leading to additional need for residential and commercial spaces. This led to high pressure on the protected areas and agricultural areas, which had suffered maximum depreciation for this period.
The region lying at an altitude of less than 1500 m remained the most critical with maximum changes in LULC classes being witnessed there. The built-up areas, agricultural areas, and protected areas showed maximum transition in this region. The main reason for this could be attributed to the better transportation facilities, road connectivity, suitable climatic conditions for living and agricultural practices, commercial establishments, and more population concentration in this region. Higher altitude regions, because of terrain and other geographical constraints, are less vulnerable to built-up areas. Thus, the city requires greater concern and attention from policymakers and environmentalists to pave the way for a balanced, holistic, and sustainable development model.
The simulation and accurate prediction of LULC become necessary to understand the trend and direction of urban sprawl. The LULC maps of 2025 and 2040 were prepared using CA modeling, and the spatial distribution of these LULC maps is shown in Fig. 6 . Six driving factors, distance from built-up areas, distance from roads, distance from the center of town, elevation, slope, and distance from streams, were chosen for the modeling.
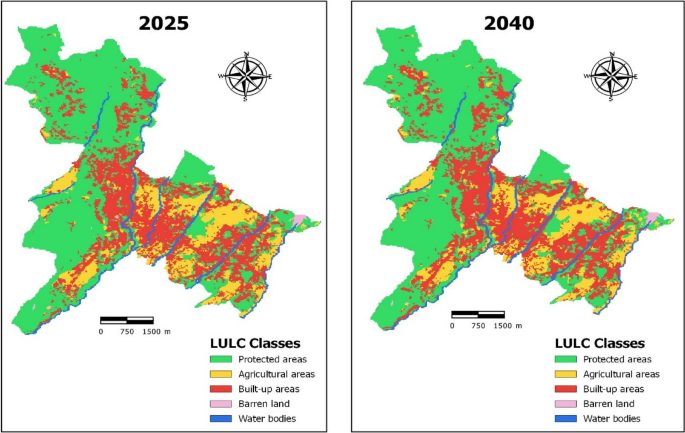
Predicted LULC maps for the years 2025 and 2040
The LULC change analysis of the maps from 2016 to 2025 and 2016 to 2040 is shown in Tables 10 and 11 . The results indicate the continuation of the trend of increase in the built-up areas and a decrease in protected areas for the year 2025. However, the increase in built-up areas will saturate after 2025, and the percentage increase in built-up areas for 3 years will be reduced as compared to the previous 3-year transition. This could be attributed to the fact that most of the usable and productive areas for construction will be exhausted.
The hilly areas offer geographical and topographical constraints for construction, and thus, the ideal locations for construction are usually those located at mid-altitudes and having less slope. The seismicity of the area is another challenge. All these factors will lead to construction in high seismic and landslide-prone areas, which would present a significant impediment to the well-being and security of the inhabitants. Another important observation from the findings was that the transition of built-up areas on the temporal scale is usually restricted to mid and south-eastern regions of the study area. The region has witnessed urban sprawl in these pockets and will remain a critical region in the future.
The swift expansion of urbanized regions, stemming from demographic expansion and the influx of tourists, emphasizes the critical significance of implementing sustainable urban planning strategies. Effective land-use management strategies should be implemented by policymakers and urban planners involving the promotion of efficient land use, reducing urban sprawl, and preserving green spaces, contributing to the attainment of Sustainable Development Goal (SDG) 11, which focuses on creating sustainable cities and communities.
The decline in protected areas is a matter of concern as it poses a threat to biodiversity and ecosystems. Strict implementation of legislation, with the involvement of environmentalists and policymakers, can help protect and restore these areas, thus preserving biodiversity and ensuring the long-term sustainability of natural resources. This effort directly relates to SDG 15, which focuses on maintaining and enhancing life on land.
Land-use planning plays a crucial role in fostering responsible consumption and production patterns. By optimizing land use and preventing further encroachment on protected areas, policymakers can contribute to sustainable resource management and reduce the environmental impact of human activities, which aligns with the objectives of SDG 12, aiming to ensure responsible consumption and production.
The increasing population and tourists will remain the major driving factors for the change. The decrease in agricultural areas indicates a shift in agriculture practice, which lately has been the preferred occupation of the residents. Further, the decrease in protected areas indicates the persistent encroachments and abeyance of legislation. In order to address the decreasing agricultural areas, it is crucial to promote sustainable farming practices and increase agricultural productivity to address the escalating requirements of sustenance. This can be accomplished through the implementation of innovative techniques, support for small-scale farmers, and ensuring food security for all, thereby working towards achieving Zero Hunger (SDG-2).
Conclusions
The study applied ANN-based CA approach for prediction of land cover classes which showed substantial agreement between the simulated and the actual LULC map, with the accuracy metric kappa showing a value of 0.77. The model incorporated six driving factors, out of which four were socio-economic spatial parameters, distance from built-up areas, roads, center of town, and streams; while two were geospatial parameters, elevation, and slope. These criteria combinations performed the best in the CA-ANN model showing the highest value of accuracy of 86.83%.
The selection of these factors was based on their potential influence on the study’s outcomes. For instance, proximity to built-up areas may impact pollution levels and development rates, while distance from roads may correlate with traffic noise and urbanization patterns. Elevation and slope could affect water resource accessibility, and proximity to streams might indicate water source quality.
The study predicts that the built-up areas will increase by 17.84% in the year 2025 and 19.69% by the year 2040. The protected areas will decrease by 14.75% and 16.66%, agricultural areas by 2.81% and 2.72%, and barren land by 0.29% and 0.31% for the years 2025 and 2040, respectively.
The rapid increase in population and tourism has led to a significant rise in built-up areas, creating an urgent demand for more land and putting undue pressure on protected areas and agricultural areas. Strict implementation of legislation is necessary to prevent further encroachments in the protected areas. Studying the critical land-use classes in terms of socio-ecological and environmental concerns is valuable for balancing environmental pressures and conservation interventions. The findings can offer guidance to administrators, policymakers, agricultural practitioners, and urban planners in formulating methodologies for sustainable land-use planning and management, fostering the optimal utilization of natural resources.
Availability of data and materials
The data used in the study was downloaded from USGS ( https://earthexplorer.usgs.gov/ ) and is available openly. It is further declared that the data related to the study will be shared upon request.
It is further certified that the research complies with ethical standards, there was no funding for this research, and there are no potential conflicts of interest (financial or non-financial).
Abbreviations
Land use land cover
Cellular automata
Artificial neural network
Multi-layer perceptron
Operational Land Imager
Thermal infrared sensor
Protected areas
Agricultural areas
Built-up areas
Barren land
Water bodies
Maximum likelihood classifier
Modules for land use change
Sustainable Development Goal
Gaigbe-Togbe V, Bassarsky L, Gu D, Spoorenberg T, Zeifman L (2022) World population prospects, Department of Economic and Social Affairs, Population Division: New York, NY, USA
Hertog S, Gerland P, Wilmoth J (2023) India overtakes China as the world’s most populous country. Department of Economic and Social Affairs, United Nations. Policy Brief No. 153
Jat MK, Garg PK, Khare D (2008) Monitoring and modelling of urban sprawl using remote sensing and GIS techniques. Int J Appl Earth Obs Geoinf 10(1):26–43
Google Scholar
Mondal I, Thakur S, Ghosh P, De TK, Bandyopadhyay J (2019) Land use/land cover modeling of Sagar Island, India using remote sensing and GIS techniques. In Emerging Technologies in Data Mining and Information Security: Proceedings of IEMIS 2018:771–785
Mushtaq F, Pandey AC (2014) Assessment of land use/land cover dynamics vis-à-vis hydrometeorological variability in Wular Lake environs Kashmir Valley, India using multitemporal satellite data. Arab J Geosci 7:4707–4715
Chowdhury M, Hasan ME, Abdullah-Al-Mamun MM (2020) Land use/land cover change assessment of Halda watershed using remote sensing and GIS. Egypt J Remote Sens Sp Sci 23(1):63–75
Rawat JS, Kumar M (2015) Monitoring land use/cover change using remote sensing and GIS techniques: a case study of Hawalbagh block, district Almora, Uttarakhand, India. Egypt J Remote Sens Sp Sci 18(1):77–84
Aithal BH, Ramachandra TV (2016) Visualization of urban growth pattern in Chennai using geoinformatics and spatial metrics. J Indian Soc Remote Sens 44:617–633
Article Google Scholar
Fazal S (2000) Urban expansion and loss of agricultural land-a GIS based study of Saharanpur City. India Environ Urban 12(2):133–149
Deka J, Tripathi OP, Khan ML, Srivastava VK (2019) Study on land-use and land-cover change dynamics in Eastern Arunachal Pradesh, NE India using remote sensing and GIS. Trop Ecol 60:199–208
Ghosh S, Sen KK, Rana U, Rao KS, Saxena KG (1996) Application of GIS for land-use/land-cover change analysis in a mountainous terrain. J Indian Soc Remote Sens 24:193–202
Bisht BS, Kothyari BP (2001) Land-cover change analysis of Garur Ganga watershed using GIS/remote sensing technique. J Indian Soc Remote Sens 29:137–141
Berberoglu S, Akin A (2009) Assessing different remote sensing techniques to detect land use/cover changes in the eastern Mediterranean. Int J Appl Earth Obs Geoinf 11(1):46–53
Hegazy IR, Kaloop MR (2015) Monitoring urban growth and land use change detection with GIS and remote sensing techniques in Daqahlia governorate Egypt. Int J Sustain Built Environ 4(1):117–124
Liping C, Yujun S, Saeed S (2018) Monitoring and predicting land use and land cover changes using remote sensing and GIS techniques—a case study of a hilly area, Jiangle. China. PLoS One 13(7):e0200493
Article PubMed Google Scholar
Meshesha TW, Tripathi SK, Khare D (2016) Analyses of land use and land cover change dynamics using GIS and remote sensing during 1984 and 2015 in the Beressa Watershed Northern Central Highland of Ethiopia. Model Earth Syst Environ 2:1–12
Mathew A, Chaudhary R, Gupta N, Khandelwal S, Kaul N (2015) Study of urban heat island effect on Ahmedabad City and its relationship with urbanization and vegetation parameters. Int J Comput Math Sci 4:126–135
Muhammad R, Zhang W, Abbas Z, Guo F, Gwiazdzinski L (2022) Spatiotemporal change analysis and prediction of future land use and land cover changes using QGIS MOLUSCE Plugin and Remote Sensing Big Data: a case study of Linyi. China. Land 11(3):419
Zhao S, Liu S, Zhou D (2016) Prevalent vegetation growth enhancement in urban environment. Proc Natl Acad Sci USA 113(22):6313–6318
Article CAS PubMed PubMed Central ADS Google Scholar
Saha AK, Arora MK, Csaplovics E, Gupta RP (2005) Land cover classification using IRS LISS III image and DEM in a rugged terrain: a case study in Himalayas. Geocarto Int 20(2):33–40
Article ADS Google Scholar
Wang SW, Gebru BM, Lamchin M, Kayastha RB, Lee WK (2020) Land use and land cover change detection and prediction in the Kathmandu district of Nepal using remote sensing and GIS. Sustainability 12(9):3925
Gharaibeh A, Shaamala A, Obeidat R, Al-Kofahi S (2020) Improving land-use change modeling by integrating ANN with cellular automata-Markov chain model. Heliyon 6(9):e05092
Article PubMed PubMed Central Google Scholar
Bhattacharya RK, Das Chatterjee N, Das K (2021) Land use and land cover change and its resultant erosion susceptible level: an appraisal using RUSLE and logistic regression in a tropical plateau basin of West Bengal. India Environ Dev Sustain 23(2):1411–1446
Nagendra H, Munroe DK, Southworth J (2004) From pattern to process: landscape fragmentation and the analysis of land use/land cover change. Agric Ecosyst Environ. 101:111–115
Thakkar AK, Desai VR, Patel A, Potdar MB (2017) Post-classification corrections in improving the classification of land use/land cover of arid region using RS and GIS: the case of Arjuni watershed, Gujarat, India. Egypt J Remote Sens Sp Sci 20(1):79–89
Aburas MM, Ho YM, Ramli MF, Ash’aari ZH, (2016) The simulation and prediction of spatio-temporal urban growth trends using cellular automata models: a review. Int J Appl Earth Obs Geoinf 52:380–389
Tong X, Feng Y (2020) A review of assessment methods for cellular automata models of land-use change and urban growth. Int J Geogr Inf Sci 34(5):866–898
Al-doski J, Mansor SB, Zulhaidi H, Shafri M (2013) Image classification in remote sensing. Int J Sens Netw 3(10):141–148
Bruzzone L, Serpico SB (1997) Classification of imbalanced remote-sensing data by neural networks. Pattern Recognit Lett 18(11–13):1323–1328
Buğday E, Erkan Buğday S (2019) Modeling and simulating land use/cover change using artificial neural network from remotely sensing data. Cerne 25(2):246–254
Kafy AA, Shuvo RM, Naim MN, Sikdar MS, Chowdhury RR, Islam MA, Sarker MH, Khan MH, Kona MA (2021) Remote sensing approach to simulate the land use/land cover and seasonal land surface temperature change using machine learning algorithms in a fastest-growing megacity of Bangladesh. Remote Sens Appl Soc Environ 21:100463
Wolfram S (1984) Cellular automata as models of complexity. Nature 311(5985):419–424
Batty M, Xie Y (1994) Research article modelling inside GIS: part 1. Model structures, exploratory spatial data analysis and aggregation. Int J Geogr Inf Syst 8(3):291–307
Batty M, Xie Y, Sun Z (1999) Modeling urban dynamics through GIS-based cellular automata. Comput Environ Urban Syst 23(3):205–233
Santé I, García AM, Miranda D, Crecente R (2010) Cellular automata models for the simulation of real-world urban processes: a review and analysis. Landsc Urban Plan 96(2):108–122
Sarkar P (2000) A brief history of cellular automata. ACM Comput Surv 32(1):80–107
Leao S, Bishop I, Evans D (2004) Simulating urban growth in a developing nation’s region using a cellular automata-based model. J Urban Plan Dev 130(3):145–158
Lagarias A (2012) Urban sprawl simulation linking macro-scale processes to micro-dynamics through cellular automata, an application in Thessaloniki, Greece. Appl Geogr 34:146–160
Kamaraj M, Rangarajan S (2022) Predicting the future land use and land cover changes for Bhavani basin, Tamil Nadu, India, using QGIS MOLUSCE plugin. Environ Sci Pollut Res 29(57):86337–86348
Atef I, Ahmed W, Abdel-Maguid RH (2023) Future land use land cover changes in El-Fayoum governorate: a simulation study using satellite data and CA-Markov model. Stoch Environ Res Risk Assess 26:1–14
Asori M, Adu P (2023) Modeling the impact of the future state of land use land cover change patterns on land surface temperatures beyond the frontiers of greater Kumasi: a coupled cellular automaton (CA) and Markov chains approaches. Remote Sens Appl Soc Environ 29:100908
Saputra MH, Lee HS (2019) Prediction of land use and land cover changes for North Sumatra, Indonesia, using an artificial-neural-network-based cellular automaton. Sustain 11(11):1–16
Gupta N (2013) Artificial neural network Netw Complex Syst 3(1):24–28
Kadam AK, Wagh VM, Muley AA, Umrikar BN, Sankhua RN (2019) Prediction of water quality index using artificial neural network and multiple linear regression modelling approach in Shivganga River basin, India. Model Earth Syst Environ 5:951–962
Wu X, Liu X, Zhang D, Zhang J, He J, Xu X (2022) Simulating mixed land-use change under multi-label concept by integrating a convolutional neural network and cellular automata: a case study of Huizhou. China GIsci Remote Sens 59(1):609–632
Shafizadeh-Moghadam H, Tayyebi A, Helbich M (2017) Transition index maps for urban growth simulation: application of artificial neural networks, weight of evidence and fuzzy multi-criteria evaluation. Environ Monit Assess 189:1–4
Tayyebi A, Pijanowski BC (2014) Modeling multiple land use changes using ANN, CART and MARS: comparing tradeoffs in goodness of fit and explanatory power of data mining tools. Int J Appl Earth Obs Geoinf 28:102–116
Atkinson PM, Tatnall AR (1997) Introduction neural networks in remote sensing. Int J Remote Sens 18(4):699–709
Hakim AM, Baja S, Rampisela DA, Arif S (2019) Spatial dynamic prediction of landuse/landcover change (case study: Tamalanrea sub-district, Makassar city). IOP Conf Ser Earth Environ Sci 280(1):012023
Mzava P, Nobert J, Valimba P (2019) Land cover change detection in the urban catchments of Dar es Salaam, Tanzania using remote sensing and GIS techniques. Tanz J Sci 45(3):315–329
Gupta V, Ram BK, Kumar S, Sain K (2022) A case study of the 12 July 2021 Bhagsunath (McLeod Ganj) flash flood in Dharamshala, Himachal Pradesh: A warning against constricting natural drainage. J Geol Soc India 98(5):607–610
Weng Q, Fu P (2014) Modeling annual parameters of clear-sky land surface temperature variations and evaluating the impact of cloud cover using time series of Landsat TIR data. Remote Sens Environ 140:267–278
Mishra PK, Rai A, Rai SC (2020) Land use and land cover change detection using geospatial techniques in the Sikkim Himalaya, India. Egypt J Remote Sens Sp Sci 23(2):133–143
Phinzi K, Ngetar NS, Pham QB, Chakilu GG, Szabó S (2023) Understanding the role of training sample size in the uncertainty of high-resolution LULC mapping using random forest. Earth Sci Inform 16(4):3667–3677
Gebreslasie MT, Ahmed FB, Van Aardt JA, Blakeway F (2011) Individual tree detection based on variable and fixed window size local maxima filtering applied to IKONOS imagery for even-aged Eucalyptus plantation forests. Int J Remote Sens 32(15):4141–4154
Kumar D (2017) Monitoring and assessment of land use and land cover changes (1977–2010) in Kamrup District of Assam, India using remote sensing and GIS techniques. Appl Ecol Environ Res 15(3):221–239
Article MathSciNet Google Scholar
Zaz SN, Romshoo SA (2012) Assessing the geoindicators of land degradation in the Kashmir Himalayan region, India. Nat Hazards 64:1219–1245
Deng Z, Zhang X, Li D, Pan G (2015) Simulation of land use/land cover change and its effects on the hydrological characteristics of the upper reaches of the Hanjiang Basin. Environ Earth Sci 73:1119–1132
Yeh AG, Li X (1998) Sustainable land development model for rapid growth areas using GIS. Int J Geogr Inf Sci 12(2):169–189
Martins VS, Kaleita AL, Gelder BK, da Silveira HL, Abe CA (2020) Exploring multiscale object-based convolutional neural network (multi-OCNN) for remote sensing image classification at high spatial resolution. ISPRS J Photogramm Remote Sens 168:56–73
Chettry V, Surawar M (2021) Delineating urban growth boundary using remote sensing, ANN-MLP and CA model: a case study of Thiruvananthapuram urban agglomeration. India J Indian Soc Remote Sens 49(10):2437–2450
Bozkaya AG, Balcik FB, Goksel C, Esbah H (2015) Forecasting land-cover growth using remotely sensed data: a case study of the Igneada protection area in Turkey. Environ Monit Assess 187:1–8
Feng Y (2017) Modeling dynamic urban land-use change with geographical cellular automata and generalized pattern search-optimized rules. Int J Geogr Inf Sci 31(6):1198–1219
Lillesand T, Kiefer RW, Chipman J (2015) Remote sensing and image interpretation. John Wiley & Sons
Van Vliet J, Bregt AK, Hagen-Zanker A (2011) Revisiting kappa to account for change in the accuracy assessment of land-use change models. Ecol Modell 222(8):1367–1375
Rwanga SS, Ndambuki JM (2017) Accuracy assessment of land use/land cover classification using remote sensing and GIS. Int J Geosci 8(04):611
Download references
Acknowledgements
Not applicable.
The authors, Nishant Mehra and Dr. Janaki Ballav Swain, hereby certify that no funding was received.
Author information
Authors and affiliations.
School of Civil Engineering, Lovely Professional University, Punjab, 144001, India
Nishant Mehra & Janaki Ballav Swain
You can also search for this author in PubMed Google Scholar
Contributions
It is certified that both the authors had contributed equally in the preparation of the manuscript. It is also certified that all authors have read and approved the manuscript.
Corresponding author
Correspondence to Nishant Mehra .
Ethics declarations
Competing interests.
The authors, Nishant Mehra and Dr. Janaki Ballav Swain, hereby certify that they have no affiliations with or involvement in any organization or entity with any financial interest (such as honoraria; educational grants; participation in speakers’ bureaus; membership, employment, consultancies, stock ownership, or other equity interest; and expert testimony or patent-licensing arrangements), or non-financial interest (such as personal or professional relationships, affiliations, knowledge or beliefs) in the subject matter or materials discussed in this manuscript.
Additional information
Publisher’s note.
Springer Nature remains neutral with regard to jurisdictional claims in published maps and institutional affiliations.
Rights and permissions
Open Access This article is licensed under a Creative Commons Attribution 4.0 International License, which permits use, sharing, adaptation, distribution and reproduction in any medium or format, as long as you give appropriate credit to the original author(s) and the source, provide a link to the Creative Commons licence, and indicate if changes were made. The images or other third party material in this article are included in the article's Creative Commons licence, unless indicated otherwise in a credit line to the material. If material is not included in the article's Creative Commons licence and your intended use is not permitted by statutory regulation or exceeds the permitted use, you will need to obtain permission directly from the copyright holder. To view a copy of this licence, visit http://creativecommons.org/licenses/by/4.0/ . The Creative Commons Public Domain Dedication waiver ( http://creativecommons.org/publicdomain/zero/1.0/ ) applies to the data made available in this article, unless otherwise stated in a credit line to the data.
Reprints and permissions
About this article
Cite this article.
Mehra, N., Swain, J.B. Assessment of land use land cover change and its effects using artificial neural network-based cellular automation. J. Eng. Appl. Sci. 71 , 70 (2024). https://doi.org/10.1186/s44147-024-00402-0
Download citation
Received : 03 November 2023
Accepted : 27 February 2024
Published : 14 March 2024
DOI : https://doi.org/10.1186/s44147-024-00402-0
Share this article
Anyone you share the following link with will be able to read this content:
Sorry, a shareable link is not currently available for this article.
Provided by the Springer Nature SharedIt content-sharing initiative
- Artificial neural network (ANN)
- Supervised classification
- Cellular automata (CA)
- Land use land cover (LULC)
Click through the PLOS taxonomy to find articles in your field.
For more information about PLOS Subject Areas, click here .
Loading metrics
Open Access
Peer-reviewed
Research Article
Monitoring and predicting land use and land cover changes using remote sensing and GIS techniques—A case study of a hilly area, Jiangle, China
Roles Data curation, Formal analysis, Methodology, Software, Validation, Writing – original draft, Writing – review & editing
Affiliation State Forestry Administration Key Laboratory of Forest Resources & Environmental Management, Beijing Forestry University, Beijing, China
Roles Funding acquisition, Investigation, Project administration, Supervision, Visualization, Writing – review & editing
* E-mail: [email protected]
Roles Writing – original draft, Writing – review & editing
- Chen Liping,
- Sun Yujun,
- Sajjad Saeed
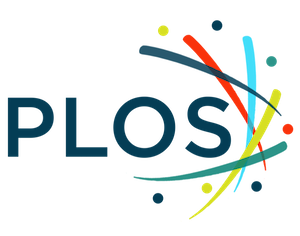
- Published: July 13, 2018
- https://doi.org/10.1371/journal.pone.0200493
- Reader Comments
Land use and land cover change research has been applied to landslides, erosion, land planning and global change. Based on the CA-Markov model, this study predicts the spatial patterns of land use in 2025 and 2036 based on the dynamic changes in land use patterns using remote sensing and geographic information system. CA-Markov integrates the advantages of cellular automata and Markov chain analysis to predict future land use trends based on studies of land use changes in the past. Based on Landsat 5 TM images from 1992 and 2003 and Landsat 8 OLI images from 2014, this study obtained a land use classification map for each year. Then, the genetic transition probability from 1992 to 2003 was obtained by IDRISI software. Based on the CA-Markov model, a predicted land use map for 2014 was obtained, and it was validated by the actual land use results of 2014 with a Kappa index of 0.8128. Finally, the land use patterns of 2025 and 2036 in Jiangle County were determined. This study can provide suggestions and a basis for urban development planning in Jiangle County.
Citation: Liping C, Yujun S, Saeed S (2018) Monitoring and predicting land use and land cover changes using remote sensing and GIS techniques—A case study of a hilly area, Jiangle, China. PLoS ONE 13(7): e0200493. https://doi.org/10.1371/journal.pone.0200493
Editor: Andreas Westergaard-Nielsen, University of Copenhagen, DENMARK
Received: November 4, 2017; Accepted: June 27, 2018; Published: July 13, 2018
Copyright: © 2018 Liping et al. This is an open access article distributed under the terms of the Creative Commons Attribution License , which permits unrestricted use, distribution, and reproduction in any medium, provided the original author and source are credited.
Data Availability: Remote sensing data is available from the USGS ( http://glovis.usgs.gov ) for free. ASTER GDEM is available from the Geospatial Data Cloud ( http://www.gscloud.cn/ ) for free.
Funding: This study was supported by the Introduce Project of Forest Multifunction Management Science and Technology of Forplan System (No.2015-4-31). The funders had no role in study design, data collection and analysis, decision to publish, or preparation of the manuscript.
Competing interests: The authors have declared that no competing interests exist.
Introduction
Land use research programs at a global scale have become central to international climate and environmental change research since the launch of land use and land cover (LULC) change project[ 1 ]. LULC has two separate terminologies that are often used interchangeably[ 2 ]. Land cover refers to the biophysical characteristics of earth’s surface, including the distribution of vegetation, water, soil, and other physical features of the land. While land use refers to the way in which land has been used by humans and their habitat, usually with an emphasis on the functional role of land for economic activities[ 3 – 5 ]. For instance, in terms of urbanization, a large amount of agricultural / forestry land has been transformed into urban land, and mining activities / oil exploitation have occurred worldwide to meet the demands of people and can directly and obviously lead to the LUCC[ 6 , 7 ]. In past studies, global environmental changes such as emissions of greenhouse gases, global climate change, loss of biodiversity, and loss of soil resources have been closely linked to LULC changes[ 8 ]. Land use and land cover change (LULCC) is the conversion of different land use types and is the result of complex interactions between humans and the physical environment[ 9 ]. LULCC is a major driver of global change and has a significant impact on ecosystem processes, biological cycles and biodiversity[ 7 , 10 , 11 ]. Moreover, LULCC is also closely related to the sustainable development of the social economy[ 12 , 13 ]. Vast areas of the earth’s terrestrial surface have undergone LULCC[ 14 – 16 ]. With rapid economic development, land uses change more rapidly, and the contrast among land use types also increases[ 17 ].
Various techniques of LULC change detection analysis were discussed by Lu et al [ 18 ]. It is possible to establish a model to predict the trends in land uses in a certain period of time through the study of past land use changes, which could provide some basis for scientific and effective land use planning, management and ecological restoration in a study area and guidance for regional socio-economic development. Therefore, accurate and up-to-date land cover change information is necessary for understanding and assessing LULC changes. Remote sensing (RS) and geographic information system (GIS) are essential tools in obtaining accurate and timely spatial data of land use and land cover, as well as analyzing the changes in a study area[ 19 – 21 ]. Remote sensing images can effectively record land use situations and provide an excellent source of data, from which updated LULC information and changes can be extracted, analyzed and simulated efficiently through certain means[ 22 , 23 ]. Therefore, remote sensing is widely used in the detection and monitoring of land use at different scales[ 24 – 27 ]. GIS provides a flexible environment for collecting, storing, displaying and analyzing digital data necessary for change detection[ 19 , 28 , 29 ].
Land cover change modeling means time interpolation or extrapolation when the modeling exceeds the known period[ 30 ]. Commonly used models for estimating land cover changes are analytical equation-based models[ 31 ], statistical models[ 32 ], evolutionary models[ 33 ], cellular models[ 34 ], Markov models[ 35 ], hybrid models[ 36 ], expert system models[ 37 ] and multi-agent models[ 38 ]. At present, the most widely used models in land use change monitoring and prediction are cellular and agent-based models or the mixed model based on these two types of models[ 39 – 42 ]. The Markov chain and Cellular Automata (CA-Markov) model, one of a mixed models, is the hybrid of the Cellular Automata and Markov models. This model effectively combines the advantages of the long-term predictions of the Markov model and the ability of the Cellular Automata (CA) model to simulate the spatial variation in a complex system, and this mixed model can effectively simulate land cover change[ 43 ]. A CA model is a dynamic model with local interactions that reflect the evolution of a system, where space and time are considered as discrete units, and space is often represented as a regular lattice of two dimensions[ 44 ]. CA-based models have a strong ability to represent nonlinear, spatial and stochastic processes. However, CA model cannot represent macro-scale social, economic and cultural driving forces that influence urban expansion well. Thus, an integration of agents into CA models results in the improved CA-Markov model[ 40 ]. In the Markov model, the change in an area is summarized by a series of transition probabilities from one state to another over a specified period of time. These probabilities can be subsequently used to predict the land use properties at specific future time points[ 45 ]. The use of the CA-Markov model in LULCC studies has advantages such as its dynamic simulation capability; high efficiency with data, scarcity and simple calibration; and ability to simulate multiple land covers and complex patterns[ 17 , 46 ]. Many researchers have applied the CA-Markov model to monitor land use and landscape changes and predictions[ 23 , 36 , 47 , 48 ]. Therefore, we adopted this method to obtain reliable results for Jiangle. In this study, the 2025 and 2036 LULCs were predicted based on the state of 2003 and 2014 LULCs.
In recent decades, rapid population migration from rural to urban regions and improved economic conditions in China have resulted in unprecedented LULC changes and urban expansion rates[ 6 ]. Drivers of urbanization and changes in urban planning should be taken into account[ 48 ]. Many studies have focused on the LULCC at the scale of large cities to provinces in terms of surface runoff, urban impervious surface, surface urban heat islands, etc.[ 1 , 6 , 8 , 49 , 50 ]. While there are few studies on small cities such as Jiangle, a county that is west of Fujian Province. Fujian Province, one of the most economically developed provinces in China and located in the southeastern hilly area, plays an important role in China. Moreover, Fujian Province is the core area of the “the Belt and Road Initiative” policy[ 51 ]. Jiangle is the representative county of the hilly area and owns a state-owned forest farm. In the “National Wood Strategic Reserve Production Base Construction Plan (2013–2020)”, Jiangle is one of the bases. Therefore, understanding the LULC in this area and predicting future LULC can be of great importance.
This study seeks to utilize remotely sensed data and GIS tools to analyze the LULCC in Jiangle County in Fujian, China for the purpose of detecting changes in the area by comparing images between two years. Based on the Markov model, the transfer probability was established based on the data from 1992 and 2003, and the predicted data of 2014 was processed using the transfer probability and suitability maps in the CA model. After validation, the land use and land cover in 2025 and 2036 were predicted. Finally, a scientific basis for decision-making for the region's ecological protection and optimal allocation of resources is provided.
Jiangle, located in the western part of Fujian Province, has a latitude between "26°25’ 31”~27°4’8” N and a longitude between 117°5’2”~117°39’56”E ( Fig 1 ). The study area is in the subtropical monsoon climate zone, with marine and continental climate characteristics. The annual average temperature is 18.7°C. The annual average rainfall during 2011 to 2015 was 1802.16 mm, and the frost-free period is approximately 273 days[ 52 ]. The precipitation during April—August accounts for more than 60% of the annual precipitation. The study area is in the middle of the main section of the Wuyi Mountains, with an average elevation of 540 m, and its highest peak in the southwest is Longxi Mountain with a height of 1620 m. The altitude in the center of the study area is lower than the altitude around the edge of the area. The terrain is tilted from northwest to southeast. The terrain is complex, and 90% of the region is the mountain hilly landform. The Jinxi River runs across the county. The main soil type in the study area is red soil. The main vegetation types are natural secondary forest, artificial fir, Pinus massoniana and Phyllostachys pubescens .
- PPT PowerPoint slide
- PNG larger image
- TIFF original image
https://doi.org/10.1371/journal.pone.0200493.g001
Data collection and research methods
Fig 2 illustrates the framework of the prediction process. The major steps include (1) data preparation; (2) determination of the classification results of three years; and (3) the application of the CA-Markov model to obtain the predictions the LULCs in the years 2025 and 2036.
https://doi.org/10.1371/journal.pone.0200493.g002
Data collection
In this study, Landsat satellite remote sensing images from 1992, 2003 and 2014 are used, with a resolution of 30 m and track numbers of 120 / 41, 120 / 42. The detailed data are shown in Table 1 . Documents such as the "Land use status classification" from the national standards and "Fujian Environmental Bulletin" and "Fujian Statistical Yearbook" are used.
https://doi.org/10.1371/journal.pone.0200493.t001
Remote sensing image preprocessing and accuracy verification
The remote sensing image data of the years 1992, 2003 and 2014 were radially calibrated and atmospherically corrected. The relative geometric corrections of the three images were conducted to remove geometric distortion caused by the sensor or the Earth rotation. Due to the differences between the TM and OLI sensors, the geometric correction of the year 2002 was based on the DEM data of the study area. Then, the 1992 and 2014 images were georeferenced to the 2002 image[ 48 ]. The errors were less than 1 pixel. Finally, terrain correction and image stitching were conducted. Considering the "China Land Classification System" and the goal of this study, the land use types were divided into five categories: farmland (including dry land and paddy field), woodland (the forest area), water, construction land (including settlements and roads) and bare land. Based on Google Earth images, Forest Management Inventory data and Landsat data of different periods, the training samples and the validation data of different periods were selected. With the help of the maximum likelihood method, classification was carried out on these three images. Precision testing was conducted using the Kappa index and the overall accuracy for the classification[ 53 , 54 ]. Image processing was based on the UTM WGS 1984 (50N) projection system. The software ENVI 5.1 and ArcGIS 10.2 were used.
Prediction of future LULC dynamics
Markov chain analysis..
Cellular automata model.
CA-Markov model.
There are no spatial variables in the Markov model, while the status for cells in the CA model is closely related to the spatial variables. The CA-Markov model integrates the CA model's ability to simulate the spatial variation in complex systems and the advantages of the long-term predictions of the Markov model. The Markov chain model component controls the temporal dynamics among the LULC classes based on transition probabilities, while the spatial dynamics are controlled by local rules determined either by the CA spatial filter or transition potential maps. The transition probabilities matrix produced by the Markov chain model is one of the inputs of the CA model[ 23 ]. The CA Markov model effectively combines the advantages of the Markov model and the CA model. The spatial prediction accuracy can be effectively simulated at the same time[ 60 ]. The process of prediction with the CA-Markov model is 1) building the suitability atlas based on the MCE, 2) generating the transfer matrix and the state transition probability matrix using the Markov model, and 3) predicting the future LULC using the CA model.
Suitability maps preparation.
To use Cellular Automata, a suitability atlas for all the classes is considered as a prerequirement[ 17 ]. The suitability atlas contains a series of suitability maps, which are usually built through the multi-criteria evaluation (MCE). The basic point of MCE is to integrate different rules to derive a single index of evaluation[ 61 ]. The MCE includes two parts: the constraints (the hard rules) and the factors (the soft rules). The constraints are the criteria that limit the expansion of classes. The constraints are expressed in the form of Boolean maps where the areas that are not suitable will be set a value of 0, while the suitable areas will be set a value of 1. The factors give a degree of suitability for an area to change (mostly on distance basis) [ 62 ]. The process of data preparation is outlined in Fig 2 . There are 3 steps: (1) Identification and development of the criteria, (2) standardization of the criteria and (3) aggregation of the criteria to obtain the suitability map for each class.
Due to the complexity of the terrain, social development and the Soil and Water Conservation Work Regulations, the existing slope, road, construction area, water are adopted to build the rules. The water and construction area were derived from the LULC maps. The road was downloaded from the OpenStreetMap and was checked according to the image data of each year. The slope was derived from the DEM data of study area with a resolution of 30×30 m. Factor and constraint images were first prepared in ArcGIS 10.0. Then, the images were imported into the IDRISI 17.0 for further processing. Using the Decision Wizard module in IDRISI, the suitability maps (Water, Construction, Bare land, Woodland and Farm land) were derived ( Fig 3 ). First, the constraints for the year 2014 were standardized into Boolean maps. Here, we utilized two constraints, the water and existing construction, because no changes can take place in the waterbodies and existing construction areas. Second, the Fuzzy function combined with the Weighted Linear Combination (WLC) was used to process the standard factors. During the standardization, the factors were stretched from 0 to 255 using different fuzzy functions and control points. For different type of factors, the fuzzy functions can be Sigmoidal, J-shaped and Linear, with monotonically increasing/decreasing or symmetric. For the control points, we set them according to the statistical results or regulations from the government. The weights of the factors were derived with the AHP function in the WLC module. Third, the suitability map of a certain class was processed in the MCE module with the constraints, factors, and weights. Finally, the suitability atlas was obtained using Collection Editor in IDRISI.
Construction (b), Bare land (c), Woodland (d), Farmland(e) and Water (a) are suitability maps. Slope (f), Elevation (g), Road (h) and Construction (i) are the input data.
https://doi.org/10.1371/journal.pone.0200493.g003

LULC change prediction.
In this study, the cell is the image grid cell, the unit size is 30×30 mm, and the whole land use spatial pattern is the cell space. The interval time is 11 years, so the number of cycles for the cellular automaton is 11. First, the land use transfer matrix and state transition probability matrix from 1992 to 2003 and 2003 to 2014 are calculated using the Markov module of IDRISI 17.0. Based on the MCE suitability map, the multi-objective decision-making module (multicriteria evaluation, MCE) in IDRISI is used to determine the suitability[ 63 ]. After comparing the results derived under the 3×3 filter with the 5×5 filter in CA model process, we adopted the 5×5 filter, meaning that the change in status for a central cell will be affected by the 5×5 neighbor cells. Finally, the land use predictions for 2025 and 2036 based on the data in 2003 and 2014 were carried out using the CA-Markov module integrated in IDRISI.
CA-Markov model validation.
Model calibration and validation is an important step in the process of model prediction. The usefulness of a model depends on the output of the validation model. The Kappa index is one of the most popular used methods for quantifying the predictive power of a model[ 23 ]. That is to compare the predicted data with reference data using the VALIDATE module. Using the CROSSTAB module in IDRISI, the predicted LULC of 2014 is compared with the 2014 observed results to obtain the Kappa index. When the Kappa index is acceptable, the land use and land cover in 2025 and 2036 can be predicted.
Analysis on dynamic change rate of land use
The rate of land use change reflects the severity of land use change in the study area in a given time period. The standard of measurement is divided into a single land use dynamic degree and a comprehensive land use dynamic degree[ 64 – 66 ]. In this study, we adopt a single land use dynamic degree and a comprehensive land use dynamic degree. In addition, a spatial analysis model of land use dynamic change on the basis of the dynamic degree proposed by Liu Shenghe and Shu Jin is also used to compare the differences between these two dynamic evaluation methods[ 67 ]. The formulas are as follows:
Results and discussion
Results of classification and analysis.
Classification results of the preprocessed images in 1992, 2003 and 2014 are presented in Fig 4 . According to the classification results, the statistics for the three years of different types of land use areas and their proportions are shown in Table 2 . It can be drawn from Table 2 and Fig 4 that the area of woodland is the largest in the study area. The woodland area are 2012.78 km 2 , 2020.76 km 2, and 1997.88 km 2 in 1992, 2003 and 2014, respectively. Construction land increases gradually during the 22 years from 1992 to 2014, which is characteristic of the Chinese urbanization process. The water area shows a trend of decreasing first and then increasing. According to the images of the years and relevant data, water bodies were developed and dredged. Substantial sand excavation equipment existed in the early stage and sediment built up. The river width was narrower in 2014 than 1992, so the water area decreased sharply. Woodland and bare land changes correlate with urban expansion and wood cutting. The Jiangle state-owned forest farm is dominated by Chinese Fir, which is cut and planted for a fixed period.
https://doi.org/10.1371/journal.pone.0200493.g004
https://doi.org/10.1371/journal.pone.0200493.t002
In this study, 163 polygons for Landsat TM and 172 polygons for Landsat OLI are randomly selected to assess classification accuracy. The validation data are randomly and manually chosen based on Google Maps and the Forest Management Inventory. Table 3 contains the evaluation results of the three periods of images. Producer’s accuracy and user’s accuracy are obtained by a confusion matrix. Overall classification accuracy in 1992, 2003 and 2014 are 94.94%, 92.12% and 92.33%, respectively, with Kappa indexes of 0.9254, 0.8964 and 0.8746, respectively.
https://doi.org/10.1371/journal.pone.0200493.t003
Analysis of land use change
Fig 5 is the schematic diagram of each land use in the periods of 1992–2003 ( Fig 5(A) ), 2003–2014 ( Fig 5(B) ) and 1992–2014 ( Fig 5(C) ), and the diagrams show the increase and decrease of each land use. Table 4 is the statistical table of land use change in Jiangle. Considering Fig 5 and Table 4 , it can be concluded that land use change is obvious in the three periods. Fig 6 is a sketch map of the increases and decreases in different land use types from 1992 to 2014. For a certain land use and land cover type, the green cells mean during that period, the LULC type of a cell is transferred from another LULC type into that specific LULC type. In contrast, the red cells mean that the certain land use type of LULC transferred out to other types. Fig 7 demonstrates the mutual transformation of different land use types from 1992 to 2014. In Fig 7 , each color represents one kind of transformation.
https://doi.org/10.1371/journal.pone.0200493.g005
https://doi.org/10.1371/journal.pone.0200493.g006
https://doi.org/10.1371/journal.pone.0200493.g007
https://doi.org/10.1371/journal.pone.0200493.t004
From 1992–2003, the net changes in water area and farmland area are relatively large, at more than 30%. The amount of water area and farmland area that converts to other land use types are 6.32 km 2 and 30.20 km 2 , accounting for 44.58% and 53.50%, respectively. The percent of other land use types that transfer into water and farmland are 19.35% and 32.77%, respectively. According to the remote sensing images and the material, sand excavation and mine exploration existed around Jinxi River, which flowed through Jiangle County; therefore, Jinxi River became narrower. Farmland transfers into construction land and bare land under the affection of urbanization process. According to the Table 4 , construction land area has a net increase of 9.21 km 2 , accounting for 17.05%. In addition, the percent of this construction land that transfers to other land use types is 57.55%, and the amount of area that transfers into construction land is 63.74%. The net increase in bare land is 19.33 km 2 , accounting for 27.08% of the area, and the percent of area that transfers into and from bare land are 76.26% and 69.93%, respectively. The net increase in area of woodland is 7.98 km 2 , accounting for 0.4% of the area. The amount of area that transfers into and from woodland are 3.26% and 2.87%, respectively. Because of forest maturity and economy development, the increasing percent of the bare land area is relatively high in this period.
Of the area that changes during 2003 to 2014, water has the largest change, at 45.34%, with a net increase in area of 6.29 km 2 . The percent of area that transfers from water into other land use type is 9.42%, and the percent of area that transfers into water is 37.68%. According to Jinagle County annals, the water area increases sharply because of a series of improvements, sand cleaning, a decrease in sand excavation, etc., which were part of dredging the Jinxi River. The area of farmland increases by 28.93% compared with that of 2003, with a net area increase of 19.6 km 2 . The percent of area that transfers into farmland and from farmland are 65.08% and 55.08%, respectively. Construction land area increased slowly, with a net increase of 5.86 km 2 , accounting for 9.27%. The percent of area that transfers into and from construction land are 56.31% and 55.08%, respectively. The area of bare land and woodland both decrease. Bare land area decreases 8.86 km 2 , accounting for 9.77%. The percent of area that transfers into and from bare land area are 66.80% and 70.08%, respectively. Woodland area decreases 22.88 km 2 , accounting for 1.13%. The percent of area that transfers into and from woodland area are 2.72% and 3.81%, respectively.
According to the changes from 1992 to 2014, the water area is flat, and the changes from 1992 to 2003 and from 2003 to 2014 demonstrate dynamic changes due to improvements to the Jinxi River. Generally, the construction area increased from 54.01 km 2 to 69.08 km 2 , accounting for 27.09% of the increasing area. The percent of the area that transfers into and from the construction are 66.06% and 56.57%, respectively. The bare land area increases from 71.36 km 2 to 81.83 km 2 , accounting for 14.67%, and the percent of area that changed into and from bare land are 87.72% and 85.98%, respectively, and these changes are the result of economic development and forest cutting. The farmland area decreases from 97.94 km 2 to 87.34 km 2 , accounting for 10.82% of the decreasing area. The percent of area that transfers into and from farmland area are 54.13% and 59.18%, respectively, and these changes are the comprehensive results of forest cutting and economic development. The percent of the woodland area changed little at 0.74%.
Analysis of change rate between two different models
Table 5 and Table 6 are the statistical tables of the land use dynamic changes in Jiangle in 1992–2003 and 2003–2014. The fastest change rate from 1992 to 2003 is bare land, which is 15.17 km 2 .a -1 , and its transfer rate and gain rate are 6.36 km 2 .a -1 and 8.82 km 2 .a -1 , respectively. The bare land dynamic index is 6.36 km 2 .a -1 . The second fastest change rate is construction land area, which is 12.01 km 2 .a -1 . Construction land area transfer rate and gain rate are 5.23 km 2 .a -1 and 6.78 km 2 .a -1 , respectively, and the construction land dynamic rate is 5.23 km 2 .a -1 . Change rates for water and farmland are low, namely, 5.26 km 2 .a -1 and 6.92 km 2 .a -1 , respectively. However, their dynamic rates are the highest, namely, 4.05 km 2 .a -1 and 4.86 km 2 .a -1 , respectively. The change rate and dynamic rate of woodland are lowest, namely, 0.56 km 2 .a -1 and 0.26 km 2 .a -1 , respectively. For the period of 2003–2014, farmland has the fastest change rate, which is 12.64 km 2 .a -1 , and its transfer rate and gain rate are 5.01 km 2 .a -1 and 7.64 km 2 .a -1 , respectively. The farmland dynamic rate is 5.01 km 2 .a -1 . The next fastest change rate is for bare land, which is 11.86 km 2 .a -1 . The transfer rate and gain rate of bare land are 6.37 km 2 .a -1 and 5.48 km 2 .a -1 , respectively. The bare land dynamic rate is 6.37 km 2 .a -1 . The construction land change rate is 10.34 km 2 .a -1 , and its dynamic rate is 4.75 km 2 .a -1 . The change rates of water and woodland are lower, which are 5.84 km 2 .a -1 and 0.59 km 2 .a -1 , respectively. Their dynamic rates are 0.86 km 2 .a -1 and 0.35 km 2 .a -1 , respectively.
The following conclusions can be drawn: the rate of change is larger than the dynamic rate. During these two periods, the transfer rate, gain rate, rate of change and the dynamic rate are all relatively large, meaning that the LULC change is intense. However, the rate of change is significantly larger than dynamic rate, so the single land use dynamic rate cannot properly describe the dynamic changes of the LULCC. For the whole area, the dynamic rate is the same as the rate of change.
https://doi.org/10.1371/journal.pone.0200493.t005
https://doi.org/10.1371/journal.pone.0200493.t006
Analysis of land use change matrices
Table 7 and Table 8 are the land use change matrix and transfer matrix, respectively. Markov’s transfer matrix reveals different types of transfer probabilities while quantitatively demonstrating the land use transfer process. The rows of the table signify the land use status and transferring out situation in the preliminary state t1 of land use change, while the columns of the table represent the land use status and transferring in situation in the final state. As is shown in the tables, the highest proportion of net increase in area is bare land, whose net increase area is 19.33 km 2 , and its net increase area accounts for 27.08%. The main reason for the increase is the transfer from woodland and farmland, and the amounts of their transfer areas are 35.51 km 2 and 23.93 km 2 , respectively, with state transition probabilities of 0.1065 and 0.2761, respectively. The second highest proportion of net increase in area is construction, which accounts for 17.05% of the net increasing area, with a net increased area of 9.21 km 2 . The net increase area of construction land is mainly from woodland transfer-in and farmland transfer-in, and their transfer-in areas are 19.61 km 2 and 12.99 km 2 , respectively, with state transition probabilities of 0.2263 and 0.0393, respectively. The woodland net increase area is 7.98 km 2 , and it has the lowest net increase percent at 0.40%. The woodland net increase area is mainly from bare land transfer-in, whose transfer-in area and transfer probability are 43.37 km 2 and 0.6472, respectively. Water and farmland areas both decrease. Water area decreased 6.32 km 2 and has a decrease proportion of 31.29%. Water mainly transfers into construction land, whose transfer-out area is 4.52 km 2 with a probability of 0.0266. Farmland transfer-out area is 30.20 km 2 , accounting for 30.83%. Farmland mainly transfers into bare land and construction land, whose areas are 23.93 km 2 and 19.6 km 2 , respectively, with state transition probabilities of 0.1065 and 0.0393, respectively.
https://doi.org/10.1371/journal.pone.0200493.t007
https://doi.org/10.1371/journal.pone.0200493.t008
Table 9 and Table 10 are the land use change transfer matrix and transition probability matrix, respectively. The preliminary stage and the final state of changes are 2003 and 2014 with an 11-year interval. As can be seen from the table, water has the highest net area percent for the land use changes during 2003–2014. The net increase area is 6.29 km 2 , with a proportion of 45.34%. The net increase area mainly comes from construction, which is 3.77 km 2 , and the state transition probability is 0.0677. The second highest net area percent is farmland, which accounts for 29.93%. The farmland increase area is 19.60 km 2 , which mainly comes from woodland and bareland with transferring areas of 26.47 km 2 and 18.27 km 2 , respectively, and with transition probabilities of 0.0628 and 0.2127, respectively. Construction land has a net increase proportion of 9.27% and its net increase area is 5.86 km 2 . Construction land increases mainly come from the transfer-in of woodland and farmland, and their transfer-in areas are 21.14 km 2 and 10.51 km 2 , respectively, with state transition probabilities of 0.0501 and 0.1744, respectively. For the bare land, the net decrease proportion is 9.77%, with a net decrease of 8.86 km 2 . Bare land mainly transfers into woodland, farmland and construction land, with transfer-out areas of 38.57 km 2 , 18.27 km 2 and 6.10 km 2 , respectively, and transition probabilities of 0.4538, 0.2127 and 0.0718, respectively. The woodland net area change proportion is the lowest, with a decrease proportion of 1.13%. However, woodland net area change amount is the highest, at 22.88 km 2 . Woodland mainly transfers into bare land, farmland and construction land, whose areas are 28.01, 26.47 and 21.14 km 2 , respectively. The corresponding transition probabilities are 0.0657, 0.0628 and 0.0501, respectively.
https://doi.org/10.1371/journal.pone.0200493.t009
https://doi.org/10.1371/journal.pone.0200493.t010
Results validation (observed LULC of 2014, simulated LULC of 2014)
The state transition area matrix and state transition probability matrix are created according to land use maps in 1992 and 2003, which can be obtained by running the CA-Markov model in IDRISI software based on the suitability atlas that has already been created. The predictive results map for 2014 is obtained with a 5×5 contiguity filter, whose running cycle is 11 years. Fig 8 is the predicted map of 2014. The next step is to use the CROSSTAB module in IDRISI. Predictive results are analyzed by overlaying the land use map of 2014 that is truly classified. Commonly, if the Kappa index is less than or equal to 0.4, then the land uses changed greatly and with poor consistency between the two images. If the Kappa index is 0.4–0.75, then there are general consistencies and obvious changes between the two images. Otherwise, there is high consistency between two images[ 58 ]. Usually, the Kappa values range from 0 to 1. Values of 0.61–0.80 means substantial, while 0.81–1 means almost perfect[ 68 , 69 ]. Here, the Kappa index between the predicted map and the observed map of 2014 is 0.8128, which is above 0.75, illustrating that the results are reliable. There is high consistency between the actual observed results and predictive results. The precision for correct predictions is relatively high; therefore, this method can be used to predict the results in 2025 and 2036.
https://doi.org/10.1371/journal.pone.0200493.g008
Results prediction
The state transition area matrix and state transition probability matrix are created by land use maps for 2003 and 2014, and the results for 2025 and 2036 are predicted by the same method ( Fig 9 ). For the prediction of 2025, the interval time is 11 years, while for the year of 2036, the interval time is 22 years. Table 11 is the statistical table based on the predictive results in 2025 and 2036. In general, in 2025 and 2036, the woodland area decreased greatly, and the remaining land area increased by a certain amount, especially in 2036, and the area of woodland decreased significantly. Therefore, to consider ecology, the protection of woodland is necessary in planning.
Predicted results of 2025 (left) and 2036 (right).
https://doi.org/10.1371/journal.pone.0200493.g009
https://doi.org/10.1371/journal.pone.0200493.t011
In recent years, with economic development and the impact of human activities, the county's land use has experienced substantial changes since the 1990s. In this study, Landsat5 TM and Landsat8 OLI image data were used to obtain land use maps for 1992, 2003 and 2014. Then, the land use structure of the study area was simulated and predicted based on the CA-Markov model.
According to the results of the classification, the forest coverage rate of Jiangle County was high, and the forest areas in 1992, 2003 and 2014 were 2012.78, 2020.76 and 977.88 km 2 , respectively. Construction land increased from 1992 to 2014 year by year. Water, bare land, and farmland area changes were closely related to human activities.
Under the influence of human activities, the land use changes in Jiangle County from 1992 to 2014 were obvious. The water area decreased first and then increased. In 1992–2003, a large amount of sand mining equipment was built in the Jinxi River, and a large amount of sediment was deposited on the river bank, so that the water area was drastically reduced. In 2003–2014, river sand mining equipment had reduced significantly, and the river was cleared, which led to the gradual restoration of the water area. The woodland area is large in size, although the changed area is large, and the proportion is small. Changes in the woodland area are mainly related to timber harvesting and urban expansion. The results showed that in 2025 and 2036, the area of woodland decreased drastically. Taking into account of the ecological functions of woodland, we should pay attention to the amount of woodland harvest in planning.
In the experimental process, there were some points that affected the prediction results. First, there were some difficulties in data acquisition due to the location of the study area. In addition, the study area is in the hilly area, where the ground changes in altitude, which has a certain impact on the image pixel value and ultimately leads to inaccuracy of the classification results. Second, the setting of the suitability data set had some influence on the LULC predictions. Finally, there was some human impacts on the land use types, especially the construction land changes. Therefore, through improving the quality of the input data and the setting of related parameters, the accuracy of the predicted LULC scenarios can be increased.
Acknowledgments
The authors thank the editors and the anonymous reviewers for their useful input. In addition, thanks to Sydney M. Greenfield for English language revision and editing.
- View Article
- Google Scholar
- 3. McConnell WJ. Land Change: The Merger of Land Cover and Land use Dynamics A2—Wright, James D. International Encyclopedia of the Social & Behavioral Sciences (Second Edition). Oxford: Elsevier; 2015. p. 220–3.
- 5. Arsanjani JJ. Dynamic Land Use / Cover Change Modelling : Geosimulation and Agent-Based Modelling. Vienna: University of Vienna; 2011.
- PubMed/NCBI
Assessment of Climate Change Impact on Land Use-Land Cover Using Geospatial Technology
- First Online: 14 February 2023
Cite this chapter
- Syeda Mishal Zahra 7 , 8 , 9 ,
- Muhammad Adnan Shahid 7 , 8 ,
- Rabeea Noor 9 ,
- M. Aali Misaal 10 , 11 ,
- Fahd Rasul 12 ,
- Sikandar Ali 7 ,
- M. Imran 7 , 9 ,
- M. Tasawar 7 , 9 &
- Sidra Azam 7
Part of the book series: Springer Climate ((SPCL))
718 Accesses
Monitoring land cover variations is imperative for global resource management. Climatic variation influences land use–land cover (LULC) distribution steadily. Geospatial techniques are among the most comprehensive and efficient approaches for developing LULC categorization maps, which greatly enhance the overall utilization of agricultural, industrial, and urban areas of any region. The primary causes of LU change are urbanization and variation in temperature and precipitation leading to climate change. People have the innate desire to be close to nature, which drives them to relocate from densely populated places toward less heavily populated regions. Therefore, as a result, agricultural lands are being replaced by new communities created by deforestation and disrupting overall ecology. In the perspective of urbanization and variation in climatic parameters, i.e., precipitation and temperature, the present and previous LULC have been examined in this study by using geospatial techniques. Geographic information systems enable the examination of the changing patterns of LULC through monitoring by satellites. The key categorization indices in this study are the normalized difference vegetation index (NDVI) ranging between −0.28 and 0.74. As per analysis, the forthcoming trend of climate change and its impact on LULC has been detected for the better management of land resources. The outcomes of this research will aid in the formulation of mitigation as a result of climate change.
This is a preview of subscription content, log in via an institution to check access.
Access this chapter
Subscribe and save.
- Get 10 units per month
- Download Article/Chapter or eBook
- 1 Unit = 1 Article or 1 Chapter
- Cancel anytime
- Available as PDF
- Read on any device
- Instant download
- Own it forever
- Available as EPUB and PDF
- Compact, lightweight edition
- Dispatched in 3 to 5 business days
- Free shipping worldwide - see info
- Durable hardcover edition
Tax calculation will be finalised at checkout
Purchases are for personal use only
Institutional subscriptions
Similar content being viewed by others
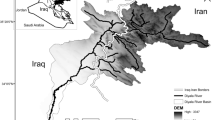
Geo-informatics techniques for detecting changes in land use and land cover in response to regional weather variation
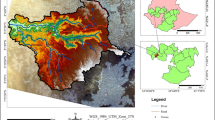
Land use/land cover change analysis using geospatial techniques: a case of Geba watershed, western Ethiopia
Analyzing Trends of Urbanization and Concomitantly Increasing Environmental Cruciality—A Case of the Cultural City, Kolkata
Abdul Athick ASM, Shankar K, Naqvi HR (2019) Data on time series analysis of land surface temperature variation in response to vegetation indices in twelve Wereda of Ethiopia using mono window, split window algorithm and spectral radiance model. Data Br 27:104773
Google Scholar
Ahmad F (2012) A review of remote sensing data change detection algorithms: comparison of Faisalabad and Multan districts, Punjab Province, Pakistan. J Geogr Reg Plan 5:236–251
Akar, Güngör O (2015) Integrating multiple texture methods and NDVI to the random forest classification algorithm to detect tea and hazelnut plantation areas in northeast Turkey. Int J Remote Sens 36:442–464
Aslam M (2016) Agricultural productivity current scenario, constraints and future prospects in Pakistan. Sarhad J Agric 32:289–303
Ayele GT, Tebeje AK, Demissie SS, Belete MA, Jemberrie MA, Teshome WM, Mengistu DT, Teshale EZ (2018) Time series land cover mapping and change detection analysis using geographic information system and remote sensing, Northern Ethiopia. Air Soil Water Res 11:117862211775160
Choudhury D, Das K, Das A (2019) Assessment of land use land cover changes and its impact on variations of land surface temperature in Asansol-Durgapur Development Region. Egypt J Remote Sens Sp Sci 22:203–218
Dhinwa PS, Pathan SK, Sastry SVC, Rao M, Majumder KL, Chotani ML, Singh JP, Sinha RLP (1992) Land use change analysis of Bharatpur district using GIS. J Indian Soc Remote Sens 20:237–250
Fan F, Weng Q, Wang Y (2007) Land use and land cover change in Guangzhou, China, from 1998 to 2003, based on Landsat TM/ETM+ imagery. Sensors 7:1323–1342
PubMed Central Google Scholar
Farooq A, Qurat-ul-ain F (2012) Algorithm and n-dimensional visualization for ETM+ image analysis: a case of district Vehari. Glob J Hum Soc Sci Arts Humanit 12:23–32
Fensholt R, Sandholt I, Stisen S (2006) Evaluating MODIS, MERIS, and VEGETATION vegetation indices using in situ measurements in a semiarid environment. IEEE Trans Geosci Remote Sens 44:1774–1786
Finance Division, G. of P (2019) Pakistan economic survey 2017–18. Pakistan Econ Surv, pp 115–131
Harris A, Carr AS, Dash J (2014) Remote sensing of vegetation cover dynamics and resilience across southern Africa. Int J Appl Earth Obs Geoinf 28:131–139
Hashim AM, Elkelish A, Alhaithloul HA, El-hadidy SM, Farouk H (2020) Environmental monitoring and prediction of land use and land cover spatio-temporal changes: a case study from El-Omayed Biosphere Reserve, Egypt. Environ Sci Pollut Res 27:42881–42897
Hassan Z, Shabbir R, Ahmad SS, Malik AH, Aziz N, Butt A, Erum S (2016) Dynamics of land use and land cover change (LULCC) using geospatial techniques: a case study of Islamabad Pakistan. Springerplus 5:812
PubMed PubMed Central Google Scholar
Hussain S (2020) Land Use/Land Cover Classification by Using Satellite NDVI Tool for Sustainable Water and Climate Change in Southern Punjab By MS Thesis COMSATS University Islamabad (CUI). https://doi.org/10.13140/RG.2.2.32363.69923
Ibrahim GRF (2017) Urban land use land cover changes and their effect on land surface temperature: case study using Dohuk City in the Kurdistan Region of Iraq. Climate 5:13
CAS Google Scholar
Jabbar A, Othman AA, Merkel B, Hasan SE (2020) Change detection of glaciers and snow cover and temperature using remote sensing and GIS: a case study of the Upper Indus Basin, Pakistan. Remote Sens Appl Soc Environ 18:100308
Jacquin A, Sheeren D, Lacombe JP (2010) Vegetation cover degradation assessment in Madagascar savanna based on trend analysis of MODIS NDVI time series. Int J Appl Earth Obs Geoinf 12:3–10
Karuppannan S (2021) Land use/land cover changes and their impact on land surface temperature using remote sensing technique in district Khanewal, Punjab Pakistan. Geol Ecol Landscapes 00:1–13
Kawo NS, Hordofa AT, Karuppannan S (2021) Performance evaluation of GPM-IMERG early and late rainfall estimates over Lake Hawassa catchment, Rift Valley Basin, Ethiopia. Arab J Geosci 14:256
Kefi C, Mabrouk A, Halouani N, Ismail H (2021) Comparison of pixel-based and object-oriented classification methods for extracting built-up areas in coastal zone. Environ Sci Eng 8:2151–2155
Kidane M, Tolessa T, Bezie A, Kessete N, Endrias M (2019) Evaluating the impacts of climate and land use/land cover (LU/LC) dynamics on the Hydrological Responses of the Upper Blue Nile in the Central Highlands of Ethiopia. Spat Inf Res 27:151–167
Lambin EF, Geist HJ, Lepers E (2003) Dynamics of land-use and land-cover change in tropical regions. Annu Rev Environ Resour 28:205–241
Lu D, Li G, Moran E, Hetrick S (2013) Spatiotemporal analysis of land-use and land-cover change in the Brazilian Amazon. Int J Remote Sens 34:5953–5978
Majeed M, Tariq A, Anwar MM, Khan AM, Arshad F, Mumtaz F, Farhan M, Zhang L, Zafar A, Aziz M, Abbasi S, Rahman G, Hussain S, Waheed M, Fatima K, Shaukat S (2021) Monitoring of land use–land cover change and potential causal factors of climate change in Jhelum district, Punjab, Pakistan, through GIS and multi-temporal satellite data. Land 10:1026
Maviza A, Ahmed F (2020) Analysis of past and future multi-temporal land use and land cover changes in the semi-arid Upper-Mzingwane sub-catchment in the Matabeleland south province of Zimbabwe. Int J Remote Sens 41:5206–5227
Näschen K, Diekkrüger B, Evers M, Höllermann B, Steinbach S, Thonfeld F (2019) The impact of land use/land cover change (LULCC) on water resources in a tropical catchment in Tanzania under different climate change scenarios. Sustainability 11(24):7083
Nowacki GJ, Abrams MD (2015) Is climate an important driver of post-European vegetation change in the Eastern United States? Glob Chang Biol 21:314–334
PubMed Google Scholar
Pande CB, Moharir K, Khadri SFR, Patil S (2018) Study of land use classification in Aried region using multispectral satellite images. Appl Water Sci (Springer J)., ISSN 2190-5487 8(5):1–11
Pande CB, Moharir KN, Khadri SFR (2021) Assessment of land-use and land-cover changes in Pangari watershed area (MS), India, based on the remote sensing and GIS techniques. Appl Water Sci. Impact factor: 3.87, Five Year Impact Factor: 4.39. 11:96. https://doi.org/10.1007/s13201-021-01425-1
Pasha SV, Reddy CS, Jha CS, Rao PVVP, Dadhwal VK (2016) Assessment of land cover change hotspots in Gulf of Kachchh, India using multi-temporal remote sensing data and GIS. J Indian Soc Remote Sens 44:905–913
Qureshi A, Mahessar AA (2016) Time – dependent flow through asymmetric contraction and expansion channel. Sindh Univ Res J (Sci Ser) 45:153–157
Rahman MTU, Tabassum F, Rasheduzzaman M, Saba H, Sarkar L, Ferdous J, Uddin SZ, Zahedul Islam AZM (2017) Temporal dynamics of land use/land cover change and its prediction using CA-ANN model for southwestern coastal Bangladesh. Environ Monit Assess 189:565
Rahman G, Rahman AU, Ullah S, Dawood M, Moazzam MFU, Lee BG (2021) Spatio-temporal characteristics of meteorological drought in Khyber Pakhtunkhwa, Pakistan. PLoS One 16:1–16
Rizvi SH, Fatima H, Alam K, Iqbal MJ (2021) The surface urban heat island intensity and urban expansion: a comparative analysis for the coastal areas of Pakistan. Environ Dev Sustain 23:5520–5537
Sapuro JT (2016) No 主観的健康感を中心とした在宅高齢者における 健康関連指標に関する共分散構造分析Title. Euphytica 18:22280
Shahid M, Rahman KU, Haider S et al (2021) Quantitative assessment of regional land use and climate change impact on runoff across Gilgit watershed. Environ Earth Sci 80:743. https://doi.org/10.1007/s12665-021-10032-x
Srivastava A, Chinnasamy P (2021) Investigating impact of land-use and land cover changes on hydro-ecological balance using GIS: insights from IIT Bombay, India. SN Appl Sci 3:343. https://doi.org/10.1007/s42452-021-04328-7
Tariq A, Riaz I, Ahmad Z, Yang B, Amin M, Kausar R, Andleeb S, Farooqi MA, Rafiq M (2020) Land surface temperature relation with normalized satellite indices for the estimation of spatio-temporal trends in temperature among various land use land cover classes of an arid Potohar region using Landsat data. Environ Earth Sci 79:1–15
Tewabe D, Fentahun T (2020) Assessing land use and land cover change detection using remote sensing in the Lake Tana Basin, Northwest Ethiopia. Cogent Environ Sci 6:E09267
Ullah S, Tahir AA, Akbar TA, Hassan QK, Dewan A, Khan AJ, Khan M (2019) Remote sensing-based quantification of the relationships between land use land cover changes and surface temperature over the lower Himalayan region. Sustainability 11(19):5492
Use L, Cover L, Using C (2020) CA-Markov chain analysis of seasonal land surface temperature and land use land cover change using optical multi-temporal satellite data of Faisalabad, Pakistan. Remote Sens 12:3402
Wakdok SS, Bleischwitz R (2021) Climate change, security, and the resource nexus: case study of Northern Nigeria and Lake Chad. Sustainability 13:1–18
Wang SW, Gebru BM, Lamchin M, Kayastha RB, Lee WK (2020) Land use and land cover change detection and prediction in the Kathmandu district of Nepal using remote sensing and GIS. Sustainability 12(9):3925
Zoungrana BJB, Conrad C, Thiel M, Amekudzi LK, Da ED (2018) MODIS NDVI trends and fractional land cover change for improved assessments of vegetation degradation in Burkina Faso, West Africa. J Arid Environ 153:66–75
Download references
Author information
Authors and affiliations.
Department of Irrigation and Drainage, Faculty of Agricultural Engineering and Technology, University of Agriculture, Faisalabad, Pakistan
Syeda Mishal Zahra, Muhammad Adnan Shahid, Sikandar Ali, M. Imran, M. Tasawar & Sidra Azam
Agricultural Remote Sensing Lab (ARSL), University of Agriculture, Faisalabad, Pakistan
Syeda Mishal Zahra & Muhammad Adnan Shahid
Department of Agricultural Engineering, Bahauddin Zakariya University, Multan, Pakistan
Syeda Mishal Zahra, Rabeea Noor, M. Imran & M. Tasawar
Department of Farm Machinery & Power, Faculty of Agricultural Engineering and Technology, University of Agriculture, Faisalabad, Pakistan
M. Aali Misaal
Department of Farm Machinery & Precision Engineering, Faculty of Agricultural Engineering and Technology, PMAS-Arid Agriculture University, Rawalpindi, Pakistan
Department of Agriculture, University of Agriculture, Faisalabad, Pakistan
You can also search for this author in PubMed Google Scholar
Editor information
Editors and affiliations.
Indian Institute of Tropical Meteorology (IITM), Pune, Maharashtra, India
Chaitanya B. Pande
Department of Earth Science, Banasthali University, Aliyabad, Rajasthan, India
Kanak N. Moharir
K. Banerjee Centre of Atmospheric and Ocean Studies, University of Allahabad, Allahabad, Uttar Pradesh, India
Sudhir Kumar Singh
Faculty of Natural Sciences, Institute of Earth Sciences, University of Silesia in Katowice, Sosnowiec, Poland
Quoc Bao Pham
Department of Agricultural Engineering, Mansoura University, Al Manşūrah, Egypt
Ahmed Elbeltagi
Rights and permissions
Reprints and permissions
Copyright information
© 2023 The Author(s), under exclusive license to Springer Nature Switzerland AG
About this chapter
Zahra, S.M. et al. (2023). Assessment of Climate Change Impact on Land Use-Land Cover Using Geospatial Technology. In: Pande, C.B., Moharir, K.N., Singh, S.K., Pham, Q.B., Elbeltagi, A. (eds) Climate Change Impacts on Natural Resources, Ecosystems and Agricultural Systems. Springer Climate. Springer, Cham. https://doi.org/10.1007/978-3-031-19059-9_17
Download citation
DOI : https://doi.org/10.1007/978-3-031-19059-9_17
Published : 14 February 2023
Publisher Name : Springer, Cham
Print ISBN : 978-3-031-19058-2
Online ISBN : 978-3-031-19059-9
eBook Packages : Earth and Environmental Science Earth and Environmental Science (R0)
Share this chapter
Anyone you share the following link with will be able to read this content:
Sorry, a shareable link is not currently available for this article.
Provided by the Springer Nature SharedIt content-sharing initiative
- Publish with us
Policies and ethics
- Find a journal
- Track your research
PhD-Thesis- Chapter-4: Land Use/ Land Cover Mapping And Spatial Analysis
- November 2015
- In book: Urban Settlement, Planning and Environmental study of Jodhpur City using Remote Sensing and GIS Technologies.

- MBM University
Discover the world's research
- 25+ million members
- 160+ million publication pages
- 2.3+ billion citations
No full-text available

To read the full-text of this research, you can request a copy directly from the author.
- Recruit researchers
- Join for free
- Login Email Tip: Most researchers use their institutional email address as their ResearchGate login Password Forgot password? Keep me logged in Log in or Continue with Google Welcome back! Please log in. Email · Hint Tip: Most researchers use their institutional email address as their ResearchGate login Password Forgot password? Keep me logged in Log in or Continue with Google No account? Sign up
Academia.edu no longer supports Internet Explorer.
To browse Academia.edu and the wider internet faster and more securely, please take a few seconds to upgrade your browser .
Enter the email address you signed up with and we'll email you a reset link.
- We're Hiring!
- Help Center
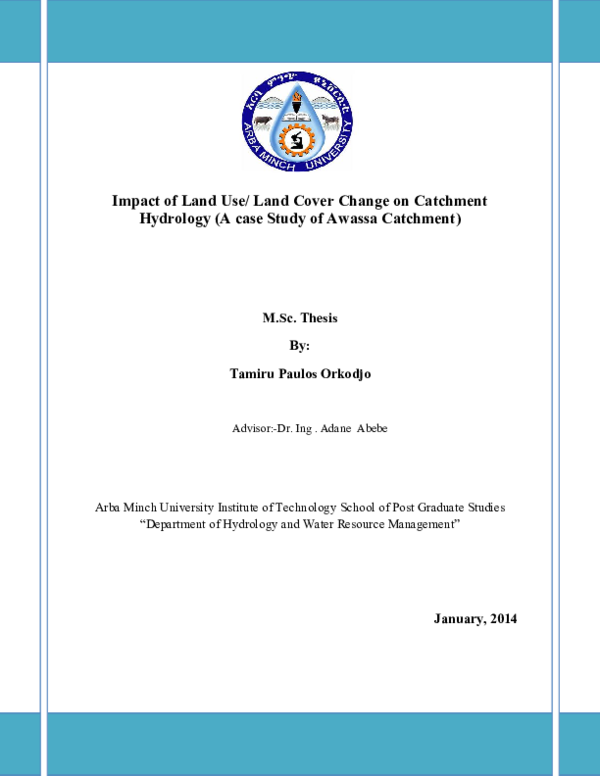
Impact of Land Use/ Land Cover Change on Catchment Hydrology (A case Study of Awassa Catchment)
Abstract Land use/land cover change has been one of factors responsible for altering the hydrologic response of watersheds leading to impacting river flows. Various water resources projects’ planning and implementation will require knowledge of the extent of these changes on catchment hydrology. The main objective of this study was to assess the impact of the environmental changes on the flow of the Awassa catchment which is located in the Main Ethiopian Rift valley in Ethiopia. In particular, the study analyses the land cover change between the 1986, 2000 and 2011, and the effect these changes have had on flows in the catchment. Land use maps of 1986, 2000 and 2011 were derived from satellite images using ERDAS Imagine 9.1 software. The land cover changes within the catchment were examined through classification of satellite images with integrated use of image and spatial analysis. The Soil and Water Assessment Tool (SWAT) model was used to investigate the impact of land cover change on stream flow. The model was calibrated and validated using historical flow data. Land cover change analysis has shown an increment in the proportion of that cultivated land from 9.5% to 17.6%, agriculture land 9.1% to 19%, and built‐up areas 5.2% to 14.4%, while a decrement in the forest area from 31.15 to 19%, water body, from 7.2 to 7% shrub from15.9% to 8.2%, wet land from 8.8 to 4.9 and grass land from 13.2 to 9.6% decreased between 1986 and 2011. Sensitivity analysis has shown that the curve number is the most sensitive parameter that affects the hydrology of the watershed. The model was calibrated for the year using flow data from 1992 to 1999 and validated from 2000 to 2002. The R 2 and Nash‐Sutcliffe Efficiency (NSE) values for the watershed were 0.90 and 0.89 for calibration, and 0.88 and 0.87 for validation, respectively. The evaluation of the SWAT model response to the land cover has shown that the mean wet monthly flow for 2011 land cover increased by 39% compared to the 1986 land cover. On the other hand, dry average monthly flow decreased by 46% in 2011 compared to 1986 land cover. According to the uncertainty analysis carried out, the significance of the LULC impacts for the catchment was analyzed. As a result it might be possible to conclude that for the catchments the impacts were significant. Therefore, it can be deduced that LULC impact for the study area might be the most sensitive than the propagated uncertainty on catchment flow. Key words: Land Use Change, SWAT Modeling, Remote Sensing, Awassa Catchment.
Related Papers
African Journal of Environmental Science and Technology
Anmut E N A W G A W Kassie , Negash Wagesho
For human beings, land and water are vital. To enhance agricultural productivity and socioeconomic development in the agricultural catchment, irrigation development is an important issue. The present study aims to evaluate the stream flow under land use land cover (LULC) change for surface irrigation in Chemoga catchment part of Abay River Basin. To accomplish the objectives of this study, the watershed model SWAT (Soil and Water Assessment Tool) was used based on organization and website data sources. LULC classification was performed using ERDAS imagine 2014 which was used for further analysis of streamflow in SWAT to evaluate surface irrigation. The selected LULC years, 1994 and 2013 were used to assess the impact on streamflow. The result depicts that in wet season streamflow increases and in the dry season the stream flow decreases respectively for the LULC of 1994 and 2013. The performance of the model during calibration and validation period was good. So watershed management approach should be done in the catchment to improve surface irrigation potential.
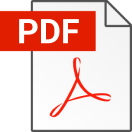
Takele Dufera
International journal of scientific research
TSEGAYESUS DILNESA
Land use /land cover change have been responsible for altering the hydrological responses of watersheds leading to impact of the stream flows. Various water resource project planning and implementation will require knowledge of the extents of these changes on watershed hydrology. This study is mainly focusing on the investigation of the impacts of land use / land cover changes on the stream flow of Muga watershed which is located in the East Choke Mountains watersheds, Upper Blue Nile Basin, East Gojjam Zone, Amhara Region, Ethiopia. Soil and Water Assessment Tool (SWAT) model were used it investigate the impact of land cover change on the stream flow. For this study SWAT Simulation is used in identifying the most vulnerable sub basins to the stream flow and sediment load changes of Muga watershed. The model was calibrated and validated using historic Stream flow data. The model was calibrated using stream flow data from 1993 to1998, validated from 1999 to2002. The R2 and NSE values...
Tesfa G Andualem , Minichil Jemberie
Land use land cover change have an impact on hydrology of the watershed on theDedissa sun basin. The study mainly focused on estimating land use change and stream flow under different land use land cover changes of the sub basin. Land use land cove maps of 1986 and 2010 were developed using satellite image throughmaximum likelihood algorithm of supervised classification using ERDAS Imagine 2014. Aphysical-based, semi-distributed hydrological model, SWAT was used to simulate LULCeffects on the hydrological response of Didessa sub-basin.During the study period the land use land cover has changed due to population growth. The cultivatedland increased by 12% and whileforestland decreased by 3.61%. The simulatedStream flow results were utilized to analyze seasonal variability stream flow due to land use and land cover changes. The performance ofthe SWAT model was evaluated through sensitivity analysis, calibration, and validation.Both the calibration and validation result shows good agreement between observed andsimulated stream flow with NSE and R 2 values of 0.7 and 0.79 respectively. Sensitivity analysis using the SWAT modelhas pointed out some crucial parameters that control the stream flow and sediment yieldin the catchment. The result of this study indicated that mean monthly stream flow wereincreased by 29.44m 3 /s for wet season and decreased by 4.44m 3 /s in dry season over 25 years period.
Applied and Environmental Soil Science
Tewodros Assefa Nigussie
Applied Water Science
Temesgen Manderso
Changes in Land Use Land Cover (LULC) are currently one of the greatest pressing issues facing the watershed, its hydrological properties of soil, and water management in catchment areas. One of the most important elements impacting streamflow in watersheds is LULC change. The main objective of this study was to evaluate the effect and future predication of LULC change on streamflow of the Fetam watershed by using Cellular Automata (CA)-Markov in IDRISI software. To analyze the impact of land use/cover change on streamflow, the Soil and Water Assessment Tool (SWAT) calibration and validation model was used. LULC map was developed by using Cellular Automata (CA)-Markov in IDRISI software, and the coverage of LULCs was including parameters of cropland, vegetation, grassland, Built-up area/Urban and water body. The findings of this study showed that the major challenges of land use/cove changes were rapid population increase, farming, and industrial activity. During the study period (2...
Temesgen Gashaw
engida nigussie
ABSTRACT Land use land cover change has a direct effect in catchment hydrologic response. It is caused by human intervention to enhance and diversify their livelihood needs, and at the same time get economic benefits from the land resources. The effects of the land cover changes have impacted on the stream flow of the watershed by changing the magnitude of surface runoff and ground water flow. This study is mainly focusing on the assessment of the impacts of the land cover changes on the stream flow by changing SUR_Q(mm), Water yield WYLD(mm), Ground water flow GW_Q (mm), Lateral flow (LATQ(mm), Evapotranspiration ET(mm) for the study period. ERDAS Imagine 2014 used to generate land use and cover maps from Landsat TM and Landsat 8 acquired, respectively, in 1995, 2001 and 2015. The land cover maps were generated using the Maximum Likelihood Algorithm of Supervised Classification. Based on analyses, the results clearly indicate that the overall accuracy and Kappa coefficient value of 0.9 and 0.87 were obtained from 2015 LULC map. The total persistence and unchanged land use land cover of the study area were reached 43.24%, 38.08% and 56.77 %, 61.94% in the study period (1995 to 2001) and (2001 to 2015) respectively. The result of this analysis showed that the chat plantation has expanded during the study period of 1995 to 2001 and 2001 to 2015. Using the three generated land cover maps, three SWAT models set up were run to evaluate the impacts the land use and cover changes on the stream flow of the study sub-basin. The performance of the SWAT model was evaluated through sensitivity analysis, calibration, and validation were run for land use land cover map of 2015.11 flow parameters were identified to be sensitive for the stream flow of the study area and used for model calibration from 24 parameters. Both the calibration and validation results showed good match between measured and simulated stream flow data with the coefficient of determination (R2) of 0.85,Nash-Sutcliffe efficiency (NSE) of 0.79 and Root Square Ratio(RSR) of 0.47 for the calibration, and R2 of 0.81, NSE of 0.77 and Root Square Ratio(RSR) of 0.48 for validation period. The result indicates that the wet mean monthly flow increased by 9.72% between 1995 to 2001, while from 2001 to 2015, it decreased by 2.63%. On the other hand small rainy season mean monthly flow was increased by 17.65% and decreased by 8.73% between 1995 to 2001.Similarly dry mean monthly flow also indicate similar stream flow characteristics like other wet and small rainy season .during this dry month, the monthly stream flow increased by 26.28% from 1995 to 2001 and it lowered by 11.72% from 2001 to 2015. Simulated stream flow for LULC 1995 to 2001 increased by 13.94% and begin to decrease LULC 2001 to 2015 by 2.57% when compared to the LULC 1995 to 2001.Thefore, decrement of the stream flow occurred due to different LULC change frequently takes places (especially, dramatically increment of mixed forest & chat plantation) in Erer river Sub-basin according to investigations and data analysis. The new patterns and trends in the use and intensity of land use reaffirmed the need for studies on the updated status of natural resources in the sub-basin. This work, we believe, is a technical tool to support the sustainable management and the decision-making processes on land use land cover.
Murad Mohammed
ABSTRACT In more recent times, land use land cover with a growing demand of population growth and urbanization has become the major concern for the proper functioning of hydrological system and environment degradations. In this context, this study was aimed to assess the impacts of LULC dynamics on surface water resources in order to have the updated quantitative data on LULC dynamics and its impacts on hydrological components of the area which is important as inputs to those who developed future directional action strategies on water resources and land resources management. For this assessment the SWAT model were used. The LULC change analysis was carried out using GIS and ERDAS Imagine 2015 using a maximum likelihood classification system for three satellite images of the years (1996, 2005, and 2014). The performance of the SWAT model in simulating the historical streamflow of the study area was evaluated through sensitivity analysis, calibration, and validation using statistical model performance indices such as coefficient of determination (R2 ), Nash-Sutcliffe coefficient of efficiency (NSE), and percentage of bias (PBIAS), and the root mean square error-observed standard ratio (RSR). Five meteorological data such as; precipitation, minimum and maximum temperature, solar radiation, relative humidity, and wind speed, and three spatial data; such as, land use land cover map, soil map, and digital elevation model (DEM) were used as input for SWAT model. The result revealed that agriculture land decreased by -1.91%, barren land increased by 3.34%, forest land decreased by -5.1%, and range grasses land increased by 0.68% during period from 1996-2014. SWAT model performs very well in simulating historical streamflow during both calibration and validation periods with NSE (0.82), R2 (0.88), Pbias (-11.1), and RSR (0.43) values during calibration and R2 (0.94), NSE (0.85), Pbias (-16.3), and RSR (0.43) values during validation. The simulated and observed streamflow of the study area during calibration and validation had a very good relationship. As a result of LULC changes in the last two decades, the water balance components (surface runoff and evapotranspiration) were increased by 12.23% and 0.47%, respectively while lateral flow, baseflow, and total water yield were decreased by -0.77%%, -4.74%, and -0.1172%, respectively. In general, LULC dynamics in the study area were significantly affected the surface water resources. Therefore, integrated and participatory water resources management strategies are suggested for exercise in the Laga- Arba watershed to mitigate the problem faced. Keywords: Awash River basin, ERDAS imagine, Laga-Arba watershed, LULC change, Streamflow, SWAT model
Cogent Environmental Science
atinkut Mezgebu
Loading Preview
Sorry, preview is currently unavailable. You can download the paper by clicking the button above.
RELATED PAPERS
Journal of African Earth Sciences
Haddush Goitom
International Soil and Water Conservation Research
Bogale Gebremariam N (PhD)
Abdata Wakjira
Mihret ULSIDO
Journal of Environment and Earth Science
TJPRC Publication
EditorIJEE RajuAedla
Research Square (Research Square)
Wassie Abuhay
ashenafi nigusie
Fitsum Alemayehu
IAEME Publication
IJCMES EDITOR
International Journal of Innovative Technology and Exploring Engineering
Tesfahun Addisu
International SWAT Conference at IIT Madras in Chennai, India
Leelambar Singh
Journal of Spatial Hydrology
Mustafa Mohamed
Anwar Surur
Misganaw Choto Choramo
Kidane Welde Reda
Hydrology and Earth System Sciences Discussions
Dagnachew Legesse
Proceedings of the International Association of Hydrological Sciences
Celso Santos
International Journal of Technology Enhancements and Emerging Engineering Research
Alene Moshe
THESESAASTU-2019-088.pdf
shemeles meshesha
RELATED TOPICS
- We're Hiring!
- Help Center
- Find new research papers in:
- Health Sciences
- Earth Sciences
- Cognitive Science
- Mathematics
- Computer Science
- Academia ©2024
- Bibliography
- More Referencing guides Blog Automated transliteration Relevant bibliographies by topics
- Automated transliteration
- Relevant bibliographies by topics
- Referencing guides
Dissertations / Theses on the topic 'Land cover land use change'
Create a spot-on reference in apa, mla, chicago, harvard, and other styles.
Consult the top 50 dissertations / theses for your research on the topic 'Land cover land use change.'
Next to every source in the list of references, there is an 'Add to bibliography' button. Press on it, and we will generate automatically the bibliographic reference to the chosen work in the citation style you need: APA, MLA, Harvard, Chicago, Vancouver, etc.
You can also download the full text of the academic publication as pdf and read online its abstract whenever available in the metadata.
Browse dissertations / theses on a wide variety of disciplines and organise your bibliography correctly.
Maluki, Peter Masavi. "MAPPING LAND COVER LAND USE CHANGE IN MBEERE DISTRICT, KENYA." Miami University / OhioLINK, 2007. http://rave.ohiolink.edu/etdc/view?acc_num=miami1187030316.
Bates-Lanclos, Melissa Marie. "Assessing urban land use/land cover change in Springfield, Missouri 1972-2000 /." free to MU campus, to others for purchase, 2004. http://wwwlib.umi.com/cr/mo/fullcit?p1426046.
Chan, Cheung-Wai Jonathan. "A neural network approach to land use/land cover change detection /." Hong Kong : University of Hong Kong, 1998. http://sunzi.lib.hku.hk/hkuto/record.jsp?B21415067.
陳章偉 and Cheung-Wai Jonathan Chan. "A neural network approach to land use/land cover change detection." Thesis, The University of Hong Kong (Pokfulam, Hong Kong), 1998. http://hub.hku.hk/bib/B31238166.
Guardiola-Claramonte, Maria Teresa. "EFFECTS OF LAND USE / LAND COVER CHANGE ON THE HYDROLOGICAL PARTITIONING." Diss., The University of Arizona, 2009. http://hdl.handle.net/10150/145730.
Knorn, Jan. "Studying land-use and land-cover change with high resolution data." Doctoral thesis, Humboldt-Universität zu Berlin, Mathematisch-Naturwissenschaftliche Fakultät II, 2012. http://dx.doi.org/10.18452/16513.
Batunacun. "Modelling land use and land cover change on the Mongolian Plateau." Doctoral thesis, Humboldt-Universität zu Berlin, 2020. http://dx.doi.org/10.18452/21796.
Saxena, Rishu. "Towards a Polyalgorithm for Land Use and Land Cover Change Detection." Thesis, Virginia Tech, 2018. http://hdl.handle.net/10919/93177.
Lawrence, Peter. "Climate impacts of Australian land cover change /." [St. Lucia, Qld.], 2004. http://www.library.uq.edu.au/pdfserve.php?image=thesisabs/absthe18055.pdf.
Bradley, Andrew Vincent. "Land-use and land-cover change in the Chapare region of Bolivia." Thesis, University of Leicester, 2005. http://hdl.handle.net/2381/9586.
Tizora, Petronella Chenayi. "Modelling land use and land cover change in the Western Cape Province." Diss., University of Pretoria, 2018. http://hdl.handle.net/2263/65948.
Anibas, Kyle Lawrence. "Land cover, land use and habitat change in Volyn, Ukraine : 1986-2011." Thesis, Kansas State University, 2014. http://hdl.handle.net/2097/17682.
Leach, Nicholas Persak. "Hydrologic response of land use and land cover changes." Thesis, University of Iowa, 2015. https://ir.uiowa.edu/etd/1870.
Bhattarai, Shreejana. "Understanding the relationship between land use/land cover and malaria in Nepal." Thesis, Virginia Tech, 2018. http://hdl.handle.net/10919/96214.
Pearlman, Daniel I. "Patterns and Processes of Land Use/Land Cover Change, 1975-2011, at Mt. Kasigau, Kenya." Miami University / OhioLINK, 2014. http://rave.ohiolink.edu/etdc/view?acc_num=miami1416571718.
Ek, Edgar. "Monitoring Land Use and Land Cover Changes in Belize, 1993-2003: A Digital Change Detection Approach." Ohio University / OhioLINK, 2004. http://www.ohiolink.edu/etd/view.cgi?ohiou1102520727.
Alavi, Shoushtari Niloofar. "Land Use and Land Cover Change Detection in Isfahan, Iran Using Remote Sensing Techniques." Thèse, Université d'Ottawa / University of Ottawa, 2012. http://hdl.handle.net/10393/22848.
Carneiro, Tiago Garcia de Senna. "Nested-ca: a foundation for multiscale modelling of land use and land cover change." Instituto Nacional de Pesquisas Espaciais, 2006. http://urlib.net/sid.inpe.br/mtc-m17@80/2007/01.03.11.57.
Zhou, Yushuang. "Development of integrated prognostic models of land use/land cover change case studies in Brazil and China /." access full-text online access from Digital dissertation consortium, 2002. http://libweb.cityu.edu.hk/cgi-bin/er/db/ddcdiss.pl?3053828.
English, Amanda M. "Land Cover Change Analysis of the Mississippi Gulf Coast from 1975 to 2005 using Landsat MSS and TM Imagery." ScholarWorks@UNO, 2011. http://scholarworks.uno.edu/td/1306.
Day, Karis L. "Assessing the impact of highway development on land use/land cover change in Appalachian Ohio." Ohio : Ohio University, 2006. http://www.ohiolink.edu/etd/view.cgi?ohiou1149852252.
Wen, Yuming. "Spatial diffusion model for simulation of urban land cover change /." View online ; access limited to URI, 2004. http://0-wwwlib.umi.com.helin.uri.edu/dissertations/dlnow/3147805.
Rodríguez, Eraso Nelly. "Land-cover and land-use change and deforestation in Colombia: spatial dynamics, drivers and modelling." Doctoral thesis, Universitat Autònoma de Barcelona, 2011. http://hdl.handle.net/10803/84004.
Yin, He. "Understanding land use and land cover change in Inner Mongolia using remote sensing time series." Doctoral thesis, Humboldt-Universität zu Berlin, Mathematisch-Naturwissenschaftliche Fakultät II, 2014. http://dx.doi.org/10.18452/17081.
Khan, Abdul Sattar. "Urban expansion, land use land cover change and human impacts : a case study of Rawalpindi." Thesis, Durham University, 2013. http://etheses.dur.ac.uk/8480/.
Gibson, Glen R. "War and Agriculture: Three Decades of Agricultural Land Use and Land Cover Change in Iraq." Diss., Virginia Tech, 2012. http://hdl.handle.net/10919/27671.
Kandel, Hari P. "Land Use /Land Cover Driven Surface Energy Balance and Convective Rainfall Change in South Florida." FIU Digital Commons, 2015. http://digitalcommons.fiu.edu/etd/2198.
Lopez, Robert Daniel. "Land-cover change, fragmentation, and agriculture in southwest Puerto Rico 1982-2002 /." [Gainesville, Fla.] : University of Florida, 2006. http://purl.fcla.edu/fcla/etd/UFE0013702.
Yorke, Charles. "Analyzing land use and land cover change in Densu River Basin in Ghana a remote sensing and GIS approach /." Diss., Online access via UMI:, 2006.
Ampomah, Richard Owusu. "Sediment Harvesting, Beneficial Use and the Impact of Climate and Land-Use/Land-Cover Change on Sediment Load." University of Akron / OhioLINK, 2014. http://rave.ohiolink.edu/etdc/view?acc_num=akron1403478256.
Qi, Zhixin, and 齐志新. "Short-interval monitoring of land use and land cover change using RADARSAT-2 polarimetric SAR images." Thesis, The University of Hong Kong (Pokfulam, Hong Kong), 2012. http://hdl.handle.net/10722/194623.
John, Ranjeet. "The Effects of Land cover/Land Use Change on Ecosystem Functions in Semi-arid Inner Mongolia." University of Toledo / OhioLINK, 2011. http://rave.ohiolink.edu/etdc/view?acc_num=toledo1294335117.
Liu, Qingling, and Fanting Gong. "Monitoring land use and land cover change: a combining approach of change detection to analyze urbanization in Shijiazhuang, China." Thesis, Högskolan i Gävle, Avdelningen för Industriell utveckling, IT och Samhällsbyggnad, 2013. http://urn.kb.se/resolve?urn=urn:nbn:se:hig:diva-13715.
Michaels, Amanda Paige. "Land Use and Land Cover Change in the Crown of the Continent Ecosystem, Montana, USA from 1992-2011." Thesis, Virginia Tech, 2016. http://hdl.handle.net/10919/72842.
Oguz, Hakan. "Modeling urban growth and land use/land cover change in the Houston Metropolitan Area from 2002 - 2030." Diss., Texas A&M University, 2003. http://hdl.handle.net/1969.1/2231.
Mendoza, Ponce Alma Virgen. "Vulnerability of biodiversity to land use change and climate change in Mexico." Thesis, University of Edinburgh, 2016. http://hdl.handle.net/1842/21701.
Samaniego, Eguiguren Luis E. "Hydrological consequences of land use, land cover and climatic changes in mesoscale catchments." Stuttgart : Inst. für Wasserbau, 2003. http://deposit.ddb.de/cgi-bin/dokserv?idn=968806724.
Samaniego-Eguiguren, Luis Eduardo. "Hydrological consequences of land use - land cover and climatic changes in mesoscale catchments." [S.l. : s.n.], 2003. http://www.bsz-bw.de/cgi-bin/xvms.cgi?SWB10720630.
Xi, Xin. "Examination of mineral dust variability and linkages to climate and land-cover/land-use change over Asian drylands." Diss., Georgia Institute of Technology, 2014. http://hdl.handle.net/1853/53433.
Humagain, Kamal. "Examining Land Use/Land Cover Change and Potential Causal Factors in the Context of Climate Change in Sagarmatha National Park, Nepal." TopSCHOLAR®, 2012. http://digitalcommons.wku.edu/theses/1218.
Trammell, Erick Jaime. "Groundwater, land use and land cover change in the Ash Meadows National Wildlife Refuge from 1948 to 1998." abstract and full text PDF (free order & download UNR users only), 2006. http://0-gateway.proquest.com.innopac.library.unr.edu/openurl?url_ver=Z39.88-2004&rft_val_fmt=info:ofi/fmt:kev:mtx:dissertation&res_dat=xri:pqdiss&rft_dat=xri:pqdiss:1433352.
Witmer, Frank D. W. "The effects of war on land-use/land-cover change: An analysis of Landsat imagery for northeast Bosnia." Connect to online resource, 2007. http://gateway.proquest.com/openurl?url_ver=Z39.88-2004&rft_val_fmt=info:ofi/fmt:kev:mtx:dissertation&res_dat=xri:pqdiss&rft_dat=xri:pqdiss:3256403.
Rahamtallah, Abualgasim Majdaldin. "Mapping and Assessing Impacts of Land Use and Land Cover Change by Means of Advanced Remote Sensing Approach:." Doctoral thesis, Saechsische Landesbibliothek- Staats- und Universitaetsbibliothek Dresden, 2017. http://nbn-resolving.de/urn:nbn:de:bsz:14-qucosa-230455.
Mhangara, Paidamwoyo. "Land use/cover change modelling and land degradation assessment in the Keiskamma catchment using remote sensing and GIS." Thesis, Nelson Mandela Metropolitan University, 2011. http://hdl.handle.net/10948/1467.
Adepoju, Matthew Olumide. "Land use and land cover change detection with remote sensing and GIS at metropolitan Lagos, Nigeria (1984-2002)." Thesis, University of Leicester, 2007. http://hdl.handle.net/2381/30414.
Johnson, Adam Bradford. "THE USE OF REMOTE SENSING AND GEOGRAPHICAL INFORMATION SYSTEMS TO CREATE LAND USE AND LAND COVER MAPS AND TO DETERMINE THE CHANGES IN THE LAND USE AND LAND COVER OVER A TEN YEAR PERIOD." MSSTATE, 2005. http://sun.library.msstate.edu/ETD-db/theses/available/etd-07072005-193332/.
Moore, Marissa Lenée. "An examination of contributing factors to land use/land cover change in southern Belize and the use of satellite image analysis to track changes." [Ames, Iowa : Iowa State University], 2007.
Ruckthongsook, Warangkana. "The Impact Of Land Use And Land Cover Change On The Spatial Distribution Of Buruli Ulcer In Southwest Ghana." Thesis, University of North Texas, 2011. https://digital.library.unt.edu/ark:/67531/metadc103385/.
Almutairi, Abdullah. "Monitoring land-cover change detection in an arid urban environment a comparison of change detection techniques /." Morgantown, W. Va. : [West Virginia University Libraries], 2000. http://etd.wvu.edu/templates/showETD.cfm?recnum=1410.
Valdez-Zamudio, Diego 1953. "Land cover and land use change detection in northwestern Sonora, Mexico using geographic information system and remote sensing techniques." Thesis, The University of Arizona, 1994. http://hdl.handle.net/10150/278469.
M.Sc, Thesis On: Impact of Land-Use/Land-Cover Changes on Land Surface Temperature in Adama Zuria Woreda, Ethiopia Using Geospatial Tools: May 2017
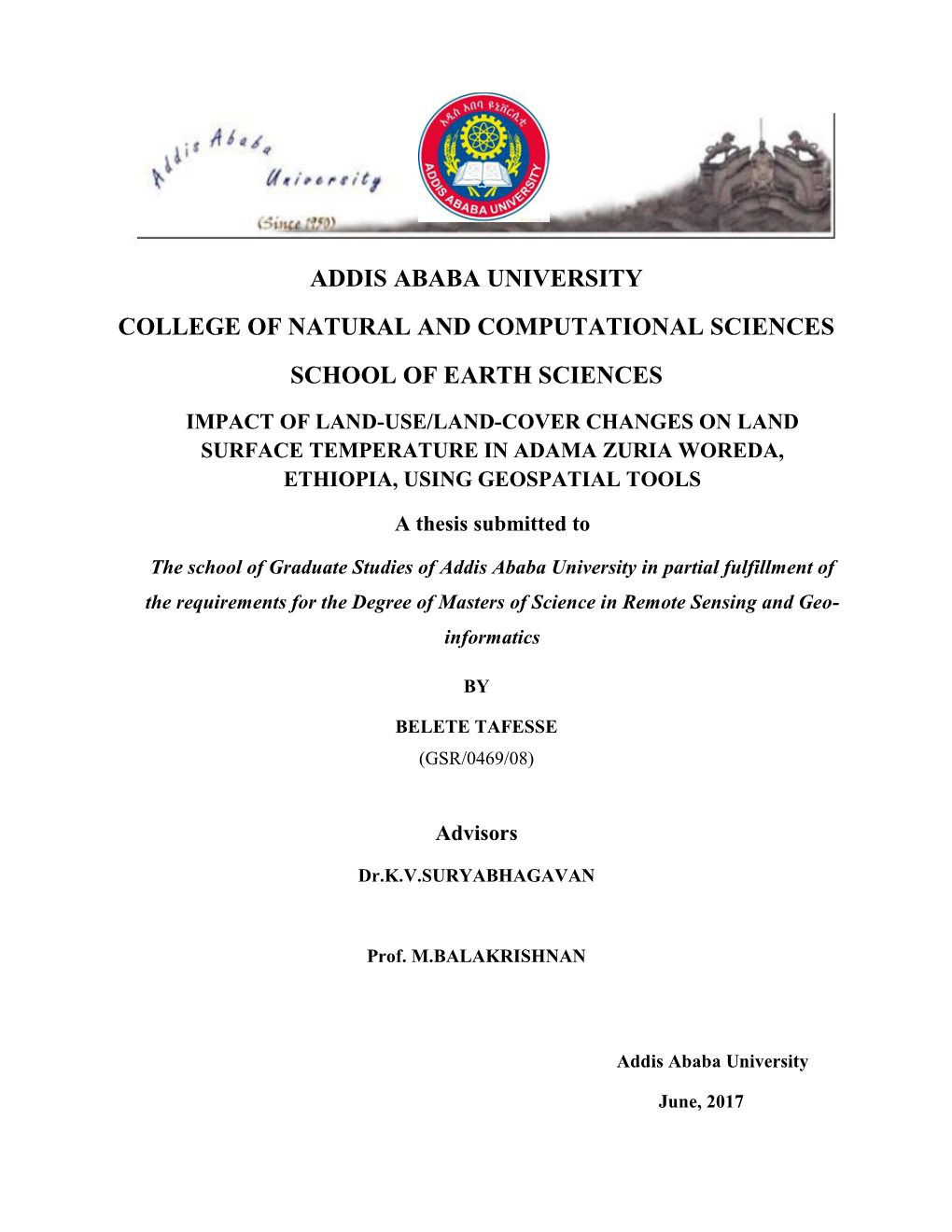
ADDIS ABABA UNIVERSITY COLLEGE OF NATURAL AND COMPUTATIONAL SCIENCES SCHOOL OF EARTH SCIENCES
IMPACT OF LAND-USE/LAND-COVER CHANGES ON LAND SURFACE TEMPERATURE IN ADAMA ZURIA WOREDA, ETHIOPIA , USING GEOSPATIAL TOOLS
A thesis submitted to
The school of Graduate Studies of Addis Ababa University in partial fulfillment of the requirements for the Degree of Masters of Science in Remote Sensing and Geo- informatics
BELETE TAFESSE (GSR/0469/08)
Dr.K.V.SURYABHAGAVAN
Prof. M.BALAKRISHNAN
Addis Ababa University
IMPACT OF LAND-USE/LAND-COVER CHANGES ON LAND SURFACE TEMPERATURE IN ADAMA ZURIA WOREDA, ETHIOPIA, USING GEOSPATIAL TOOLS
A THESIS SUBMITTED TO THE SCHOOL OF GRADUATE STUDIES OFADDIS ABABA UNIVERSITY IN PARTIAL FULFILLMENT OF THE REQUIREMENTS FOR THE DEGREE OF MASTERS OF SCIENCE IN REMOTE SENSING AND GEO- INFORMATICS
BELETE TAFESSE HABTEWOLD
(GSR/0469/08)
School of Graduate Studies
This is to certify the thesis prepared by Belete Tafesse entitled as “Impact of land-use/land- cover changes on land surface temperature in Adama Zuria Woreda, Ethiopia, using Geospatial tools” is submitted in partial fulfillment of the requirements for the Degree of Master of Science in Remote Sensing and Geo-informatics compiles with the regulations of the University and meets the accepted standards with respect to originality and quality.
Signed by the Examining Committee:
Dr. K.V.Suryabhagavan ______/______/______
Advisor Signature Date
Prof. M.Balakrishnan ______/______/______
Co-advisor Signature Date
Dr. Binyam Tesfaw ______/______/______
Chairman Signature Date
Dr. Ameha Atnafu ______/______/______
Examiner Signature Date
Head, School of Earth sciences
______/______/______
Signature Date
Acknowledgments
First and for most, I would like to thank the “Almighty God” who made it possible and for strength and patience that he gave me to complete my study successfully within the given short period of time. Next to “God”, many people and organizations deserve heartfelt thanks for their precious contributions to this study.
I express my heartfelt gratitude and indebtedness to my advisor Dr. K.V.Suryabhagavan and to my Co-advisor Prof. M.Balakrishnan, who helped me from the very beginning of the research to its compilation and for sharing me their time for valuable discussions and giving me their kind helpful guidance throughout this study.
Further, I would like to extend my thanks to all Earth Science Department staff members and Remote Sensing and Geo-informatics stream staff members for securing lab. facility and unreserved support.
I have a great gratitude to acknowledge the Ethiopian Mapping Agency, Central Statistical Agency, Ethiopian Geological Survey and Ethiopian Meteorology Agency for providing me the necessary data and information to carry out this study.
Special thanks go to all my family members and my classmates for their appreciation and continuous support. Finally, I want to thank all of those people who, once upon time, were my teachers, Friends and others, who helped me, to begin this long journey. Their names are too numerous to list, but many of them inspired me to learn and upgrade.
Table of Contents Acknowledgments ...... i
List of Tables ...... vi
List of Figures ...... vii
List of Appendices ...... ix
List of Acronyms ...... x
Abstract ...... xi
CHAPTER ONE ...... 1
1. INTRODUCTION...... 1
1.1. Background of the study ...... 1
1.2 Statement of the problem ...... 3
1.3. Objectives of the study ...... 3
1.3.1. General Objective ...... 4
1.3.2 Specific Objectives ...... 4
1.4. Research Questions ...... 4
1.5. Scope of the study ...... 4
1.6. Significance of the study ...... 5
1.7. Limitations of the study...... 5
1.8. Structure of the thesis ...... 5
CHAPTER TWO ...... 7
2. LITERATURE REVIEW ...... 7
2.1. Concept of land-use/land-cover change ...... 7
2.2. Causes of land-use and land-cover changes ...... 7
2.3. Land-use and land-cover change in Ethiopia ...... 8
2.4. Remote Sensing ...... 9
2.5. Geographic Information System (GIS) ...... 9
2.6. Role of Remote Sensing and GIS in Land-use and Land-cover change ...... 10
2.7. Land surface temperature ...... 11
2.8. Urban heat island ...... 11
2.9. The impact of land-use/land-cover change on land surface temperature ...... 12
2.10. Normalized Difference Vegetation Index ...... 12
CHAPTER THREE ...... 14
3. MATERIALS AND METHODS ...... 14
3.1. Description of the study area ...... 14
3.1.1. Location ...... 14
3.1.2. Topography ...... 15
3.1.4. Population ...... 18
3.2.1. Primary data ...... 19
3.2.2. Remote Sensing data acquisition ...... 19
3.2.3. Field data ...... 23
3.2.4. Secondary data ...... 23
3.2.5. Data description and source ...... 24
3.2.6. Software Packages used ...... 24
3.3. Methods...... 25
3.3.1. Data Preparation and Analyzing ...... 27
3.3.2. Digital Image Processing (DIP) ...... 27
3.3.3. Image enhancement ...... 27
3.3.4. Image classification ...... 28
3.3.5. Classification accuracy assessment ...... 29
3.3.6. Land-use/Land-cover change detection ...... 33
3.4. Derivation of Normalized Difference Vegetation Index and Land surface temperature ... 33
3.4.1. Derivation of Normalized Difference Vegetation Index ...... 33
3.4.2. Derivation of land surface temperature ...... 34
3.4.3. Radiometric correction ...... 35
3.4.4. Conversion at sensor spectral radiance ...... 35
3.4.5. Conversion to top of atmosphere (TOA) reflectance ...... 36
3.4.6. Conversion of radiance into brightness temperature ...... 37
CHAPTER FOUR ...... 42
4. RESULTS ...... 42
4.1. Land-use/land-cover in 1989, 1999 and 2016 ...... 42
4.2. Spatial extent of land-use/land-cover ...... 44
Table 4.3: Land transformation for Adama Zuria Woreda (1989––2016)...... 46
4.3. Settlement expansion during 1989–2016 ...... 46
4.4. Accuracy assessment ...... 47
4.5. Normalized difference vegetation index...... 48
4.6. Relationship between land-use/land-cover and Normalized difference vegetation index ...... 50
4.7. Spatial pattern of land surface temperature in Adama Zuria Woreda ...... 50
4.8. Impact of land-use/land-cover change on land surface temperature ...... 52
4.9. Relationship between land-use/land-cover and land surface temperature ...... 54
4.10. Relationship between normalized difference vegetation index and land surface temperature ...... 55
4.11. Comparisons of LST distribution during 1989, 1999 and 2016 ...... 57
4.12. Verification of the result for land surface temperature ...... 58
CHAPTR FIVE ...... 60
5. DISCUSSION ...... 60
5.1. Land-use/land-cover status of Adama Zuria Woreda ...... 60
5.2. Normalized difference vegetation index ...... 60
5.3. Land surface temperature ...... 60
CHAPTR SIX ...... 63
6. CONCLUSION AND RECOMMENDATIONS ...... 63
6.1. Conclusion ...... 63
6.2. Recommendations ...... 63
References ...... 65
Appendices ...... 71
List of Tables Table 3.1 Remote sensing data used in the study...... 19 Table 3.2: Landsat 4 Thematic Mapper sensor bands and description...... 21 Table 3.3: Landsat 7 Enhanced Thematic Mapper Plus bands and description...... 22 Table 3.4: Landsat 8 Operational Land Imager and Thermal infrared Sensor bands and description...... 23 Table 3.5: Data Description and source used in this study...... 24 Table 3.6: Land-use/land-cover classes and description of the study area...... 30 Table 3.7: Emissivity constant value of Landsat 8...... 35 Table 3.8: Thermal band calibration constant of Landsat 8...... 38 Table 3.9: Split window algorithm constant value...... 39 Table 3.10: Thematic mapper (TM) and Enhanced Thematic Mapper Plus (ETM+) thermal band calibration constant...... 39 Table 4.1: Land-use/land-cover classes and area coverage of 1989, 1999 and 2016 in Adama Zuria Woreda, Ethiopia ...... 42 Table 4.2: Land-use/land-cover distribution and net changes during 1989–2016...... 45 Table 4.3: Land transformation for Adama Zuria Woreda (1989––2016)...... 46 Table 4.4: Statistical information of accuracy assessment for the year 1989, 1999 and 2016.. .. 48 Table 4.5: Statistical information of NDVI value for the years 1989, 1999 and 2016...... 48 Table 4.6: Zonal statistical description of LST in 1989, 1999 and 2016 over different LU/LC classes...... 52 Table 4.7: Mean temperature of 1989, 1999, 2016 and changes in temperature during 1989−2016 in the study area...... 54 Table 4.8: Trends of land surface temperature distribution during the study period in Adama Zuria Woreda during 1989–2016...... 57
List of Figures Figure 3.1: Location map of the study area...... 14 Figure 3. 2: (a) Elevation and (b) Slope map of the Study area...... 15 Figure 3.3: Monthly average rainfall distribution of the Study Area...... 16 Figure 3.4: Distribution of annual rainfall in the study area (1989–2016)...... 17 Figure 3.5: Maximum, minimum and mean monthly Temperature during 1989–2016...... 17 Figure 3.6: Population of Adama Zuria Woreda...... 18 Figure 3.7: Landsat Images of 1989 TM, 1999 ETM+ and 2016 OLI and TIRS...... 20 Figure 3.8: Methodological flow chart of the study…………………………………………….26
Figure 3.9: Interpretation of Landsat image for LU/LC classification...... 31 Figure 3.10: Steps and procedures followed to classify land-use/land-cover from a Landsat image...... 32 Figure 4.1: Land-use/land-cover maps of the study area of the years of 1989, 1999 and 2016. 43 Figure 4.2: Land-use/land-cover distribution and changes in the study area during the period 1989–2016...... 44 Figure 4.3: Land-use/land-cover changes during 1989–2016 in the Adama Zuria Woreda...... 45 Figure 4.4: The trend of expansion of settlement area from the year 1989 to 2016 in Adama Zuria Woreda...... 47 Figure 4.5: Normalized difference vegetation index maps of the study area (1989, 1999 and 2016)...... 49 Figure 4.6: Zonal statistical description of NDVI in 1989, 1999 and 2016 over different LU/LC classes in the study area...... 50 Figure 4.7: Land surface temperature maps of the study area of the years 1989, 1999 and 2016...... 51 Figure 4.8: Different views of land surface temperature of the study area for 2016...... 53 Figure 4.9: Comparisons of mean land surface temperature in different land-use/land-cover classes during the study period in Adama Zuria Woreda...... 54 Figure 4.10: Land surface temperature and normalized difference vegetation index correlation for the years 1989, 1999 and 2016 for the study area...... 56 Figure 4.11: Comparisons between LST distributions of the years 1989, 1999 and 2016 in Adama Zuria Woreda...... 57
Figure 4.12: a) Interpolated map of rainfall and b) Interpolated map of temperature of Adama Zuria Woreda...... 58
List of Appendices Appendix 1: Classification accuracy assessment report for the year 1989...... 71
Appendix 2: Classification accuracy assessment report for the year 1999...... 72
Appendix 3: Classification accuracy assessment report for the year 2016...... 73
Appendix 4.Plate 1: Sample of different LU/LC photographs...... 74
Appendix 5.map 1: Location of meteorological stations map...... 75
Appendix 6 map 2: GPS point data map...... 76
List of Acronyms CSA Central Statistical Agency
DEM Digital Elevation Model
DIP Digital Image Processing
DN Digital Number
ENVI Environment for Visualizing Images
ERDAS Earth Resources Data Analysis System
ETM+ Enhanced Thematic Mapper Plus
FAO Food and Agricultural Organization
GIS Geographic Information System
GPS Global Positioning System
LST Land Surface Temperature
LU/LC Land-Use/Land-Cover
LU/LCC Land-Use/Land-Cover Change
NDVI Normalized Difference Vegetation Index
OLI Operational Land Imager
QGIS Quantum GIS
TIRS Thermal Infrared Sensor
TM Thematic Mapper
TOA Top of Atmosphere
UHI Urban Heat Island
UNEP United Nations Environmental Program
USGS United States Geological Survey
UTM Universal Transverse Mercator
Abstract Land-use/land-cover change is one of the main environmental problems and challenges, which strongly influence the process of urbanization and agricultural development. The world has faced with the problem of overwhelming increase in land surface temperature (LST) as compared from year to year. The present study has investigated the impact of land-use/land-cover (LU/LC) change on LST. The research was conducted in Adama Zuria Woreda, located East Shewa Zone of Oromia region, in the main Ethiopian rift valley. Land-use/land-cover, LST and NDVI were extracted from Landsat TM (1989), Landsat ETM + (1999), Landsat 8 OLI/TIRS (2016) using GIS and remote sensing tools. Remote sensing and GIS were found to be a robust technique to quantify and map the LU/LC changes and its drawback on LST. Land surface temperature was done using split window algorism. Changes in LU/LC, which occurred between 1989 and 2016 in the study area was evaluated and analyzed using geospatial tools and verified by field data. The result of LU/LC indicated that farmland covered more than 60% during the study periods (1989–2016) and followed by shrub land covering more than 12%. The study indicated that most areas having lower LST in 1989 were changed to higher LST in 1999 and 2016.This happened due to the increased in different LU/LC changes especially the decreasing of vegetation cover in the study area. By linking the LU/LC classes and LST parameter using zonal statistics as table, it has been found that, LST has negative relationship with vegetation cover. Land surface temperature result showed that the northwestern, south (Wenji showa sugarcane plantation), lake Koka area and along Awash River have relatively low value ranged 9ºC–21ºC. This happened because of high NDVI value. While the eastern, Adama Town and western part had high LST value reached up to 42ºC. Therefore, the visual comparison of 1989, 1999 and 2016 images showed that the LU/LC type and NDVI status play a great role for variability of LST values. Land-use/land-cover change could not be stopped easily. However, different measures have to be taken by environmental experts and the concerned bodies to minimize the influence of changes on the LST and environments. At last, this study implies that the use of geospatial tools as time saving and cost effective methods for LST analysis and evaluation.
Key words: LU/LC, LST, NDVI, Landsat image, GIS, Remote sensing
M.Sc, Thesis on: Impact of land-use/land-cover changes on land surface temperature in Adama Zuria Woreda, Ethiopia using geospatial tools: may 2017
CHAPTER ONE
1. INTRODUCTION
1.1. Background of the study The planet earth has been in a state of continuous change since a long time ago and has faced with the problem of overwhelming increase in LST as compared from year to year. Land-use/land-cover (LU/LC) changes caused by natural and human processes have played a major role in global as well as regional scale patterns of the climate and other aspect of the earth (Ramachandra et al., 2012). Now a day’s global warming makes massive LU/LC changes, which affects many aspects of the natural environment. All over the world the change in LU/LC leads to environmental changes, rainy season fluctuation, sea level rise and increase land surface temperature (LST). Massive LU/LC changes are result of the need of land for settlement and agricultural in relation to the increasing human population. Land is a scarce (limited) natural resource, which cannot be changed when the population increases. Land-use should correspond to land capacity and it should respect the overall climate condition and the environment (FAO/UNEP, 1999). Human population is increasing and it causes transformation of natural setting/environment into human landscapes. Human settlements, the need for farmland, and especially industrial areas and large urban areas significantly modify their environment. Changing from permeable and moist land uses to impermeable and dry one with paving and building material can have a negative effect on LST and energy budget (Guo et al., 2012), and also, many other surface properties such as the surface infiltration, runoff rate, evaporation and drainage system. Therefore, it is critical to study to have detailed information, spatial-temporal LU/LC dynamics and the rate of changes.
One of the main environmental problems in both rural and urban areas is the increase of LST due to conversion of vegetated surfaces into a settlement, bare land and agricultural land. Land surface temperature is one of the main variables measured using thermal bands of different sensors such as AVHRR, MODIS, Landsat-5TM, Landsat-7ETM+ and Landsat-8TIRS (Gebrekidan, 2016). Land surface temperature is the temperature of the land derived from solar radiation (Kumar and Singh, 2016). It is also defined as the temperature of the skin of earth surface phenomena and the feeling of how much hot the surface of the earth as derived from direct measurements/ from remotely sensed information (Kayet et al., 2016).
AAU.Remote Sensing and Geo-informatics stream: By Belete Tafesse: [email protected] 1
According to Rajeshwari and Mani (2014), LST is the temperature emitted by the surface and measured in Kelvin/Celsius. In remote sensing language, LST is the surface radiometric temperatures emitted by the land surfaces and adhere/observed by a sensor at instance viewing angles (Prata and Caselles, 1995; Schmugge et al., 1998). It is highly influenced by increasing greenhouse gases in the atmosphere. The changes of LST integrated with many factors, such as dynamics in land-use, seasonal variation of rainfall, weather condition and socio-economic development (Jiang and Guangjin, 2010). Urban thermal is sway out by the physical characteristics of the earth surface and by human socio-economic activities. Also to the rural area, it influenced by way of land-use and agricultural activities (Yue et al., 2007). The thermal environment can be considered to be the main and significant indicator for describing the urban vegetation and environment (Yue et al., 2007).The total energy-balance of urban areas is similar to the rural areas; however, there is difference in the ratio of shortwave and long wave radiation (Orsolya et al., 2016).
Being a less developed society, the adhered dynamics of the land are rapid in Africa than in developed continents. Environmental degradation (deterioration) is a main phenomenon in Ethiopia since agricultural activities were begun (Orsolya et al., 2016). Increasing number of population or High population pressure, low production, decline land holding size, loss of soil fertility and increasing demand for fuel energy and construction are some of the expected challenges for Ethiopia for the future. Modification and conversion of environment have impact on ecology and foster pressure on the living standard of the society. As Asubonteng (2007), stated land-use and rapid modification of land-cover have adept or practiced implications for human survival.
Changes in the land-cover occurs predominantly because of fire (Nunes et al., 2005) and deforestation for agriculture and settlement (urban expansion) (Huang and Siegert, 2006). A Land- cover change further disturbs the biogeochemical cycling that induces global warming, erosion of soil and land-use patterns.
Remote sensing and GIS or geospatial tools are now providing new tools for advanced environmental management. Satellite data facilitate synoptic dissection of the earth system, patterning and changes from local to global scales over time. Therefore, attempt has been made in this study to evaluate, analyze and to map out the status of LU/LC and LST in Adama Zuria Woreda Ethiopia. Remote sensing and GIS also used for evaluating and processing meteorological data such
AAU.Remote Sensing and Geo-informatics stream: By Belete Tafesse: [email protected] 2
as rainfall and temperature and also used to generate important information for the concerned body to use as input in environmental management.
1.2 Statement of the problem Global climate appears to be changing at an alarming rate (Naissan and Lily, 2016). Both urban and rural areas are experiencing warm temperature condition and it is increasing from time to time. The earth’s environment is a dynamic system, including many interacting components (physical, chemical, biological and human) that are continuously varying (Emilio, 2008). Due to the increase of population, industrialization and other natural and human activities, its land-use/land-cover patterns are changing. One of the main factors that responsible for the increment of LST is land-use land-cover change. In the recent time, global warming and environmental problems are a headache for both within developing and developed countries. Practices such as overgrazing, deforestation, unplanned land-use for settlement and other activities lead our environment to warm temperature. In East Shewa (Misrak shewa) zone, Adama Zuria (surrounding) Woreda LST has been increasing from season to season. Therefore, what signifies the relevance of this study is a wide array of applications that gives information of LST and LU/LC status of the area as an input for planning and decision-making. According to Aires et al. (2001) LST is a main parameter in land surface processes, not only acting as an index of climate change, but also to control the upward terrestrial radiation, and consequently, the control of the surface sensible and latent heat flux exchange with the atmosphere.
In this study, RS and GIS approach or geospatial tools were used to examine and better understand LU/LC, the relationship between LU/LCC and LST of Adama Zuria Woreda. In addition, thermal infrared remote sensing is a part of the electromagnetic spectrum and one of the best observation tools for calculating and quantifying LST.
The basic argument why this study is proposed and done in Oromia national, regional estate, East Shewa Zone Adama Zuria Woreda is that, the area is characterized diverse vegetation, climate, and topographic pattern and different land-use/land-cover.
1.3. Objectives of the study This study was carried out in the Adama Zuria Woreda, Oromia national, regional estate, Ethiopia and was intended to meet the following general and specific objectives.
AAU.Remote Sensing and Geo-informatics stream: By Belete Tafesse: [email protected] 3
1.3.1. General Objective The general objective of this research was to investigate the impact of LU/LC dynamics on LST change in Adama Zuria Woreda, Ethiopia based on Landsat imagery using geospatial tools.
1.3.2 Specific Objectives To achieve the general objective, the following three specific objectives were formulated. These are:
To examine temporal and spatial changes in the LST in relation to land-use/land-cover dynamics in the study area To analyze the relationship between LST and NDVI over time. To produce maps of land-use/land-cover, NDVI and LST from multi temporal Landsat satellite images.
1.4. Research Questions Understanding the impact of LU/LC and vegetation on LST could serve to be useful for land management and planning strategies focused on LST mitigation and the adaptation of the study area to the challenges of climate change. All the activities that are to be based on the above general and specific objectives should correlate with the following research question.
What are the spatiotemporal patterns of LU/LC changes of the study area? What is the major LU/LC class of the study area? What is the role of NDVI in LST? What is the correlation between LU/LC and LST in the study area? What is the impact of LU/LC dynamics on LST change in the study area?
1.5. Scope of the study The present study was conducted in the Adama Zuria Woreda (Adama surroundings Woreda) Oromia Region; Ethiopia. The study areas have different land-use/land-cover patterns and the LU/LC has been changing from time to time. As a result, LST of the Woreda has been rising from time to time. However, the increase of LST is not supported by research rather than perception of local communities. In order to know and compute the trend of LU/LC change, to know the correlation between LST and Land-use/land-cover (LU/LC) and to analysis, the impact of LU/LC dynamics on LST change. For each land-use/land-cover classes the LST values assessed and LU/LC classification should be supported by field verification. Increasing LST leads to environmental
AAU.Remote Sensing and Geo-informatics stream: By Belete Tafesse: [email protected] 4
problem such as climate change and seasonal fluctuation. Unless land for Settlements and farmland properly managed, it can affect and have a negative impact on the environment. Therefore, to overcome this problem, the present study would contribute for decision makers as information and identifying different LU/LC classes and changes.
1.6. Significance of the study The significance of the study could be, 1) Give information about the trend of LU/LC and LST Change of the area. 2) It could be used as an input for government policy makers, urban and rural land management, natural resources managers, environmental experts and other concerned bodies for their decision making processes related to how LU/LC and LST changes through time. In addition to this, it can be a reference or initial step and use as input for coming researchers based on the analysis of the study. In addition, it helps to quantify the relationship between LST and LU/LC, and can be an important input to predict future land warming.
The output of the study would provide better information about the changes in urban areas and surrounding integrated application of GIS and remote sensing techniques or geospatial tools and its applicability, which is time and cost effective for analysis and impact of LU/LC dynamics on LST. It also provides the opportunity to understand the trends of changes and its driving factors.
1.7. Limitations of the study The present study was attempted with all possible efforts in acquiring required inputs in the form of primary and ancillary data collection, interpretation and analysis. However, the study has encountered certain limitations. One of the limitations was routine process to get data from different organizations.
1.8. Structure of the thesis The first Chapter introduces introduction, statement of problem, objectives of the study, research questions, the scope of the study, significance of the study and limitations of the study. Chapter two concentrates on literature review related to this study. This section presents a brief understanding of land-use/land-cover changes, LST and NDVI in general. The third chapter focuses on the general methodology followed, the data used in the study and detail explanation of the study area. Chapter four explains the results, which presents the detailed results from image classification and collected data. In this section, land-use/ land-cover maps generated using maximum likelihood classification,
AAU.Remote Sensing and Geo-informatics stream: By Belete Tafesse: [email protected] 5
LST and NDVI result presented. Moreover, change analysis of LU/LC and LST, spatial map was prepared for comparison of changes in each year. Chapter five presents the discussion part and the last chapter six presents’ conclusions and recommendations. In this section, key findings and critical points that need further treatment has been forwarded as a recommendation for decision makers and for future research.
AAU.Remote Sensing and Geo-informatics stream: By Belete Tafesse: [email protected] 6
CHAPTER TWO
2. LITERATURE REVIEW This chapter presents the basic concepts and meaning of LU/LC, LST, NDVI, RS and GIS. Furthermore, it tries to explore the findings of relevant studies that have been studied previously in different area.
2.1. Concept of land-use/land-cover change The earth’s surface has been changed considerably in the past decades by human’s as a result human induced factors of deforestation, agricultural activities and urbanization. Land is the ultimate resource of the biosphere and the definition LU/LC has been used as one in different research. However, these two terms explain two different issues and have different meanings. Land-cover refers to the observed biophysical cover on the earth’s surface, including water bodies, vegetation, soil and hard surfaces. Land-use is the exploitation/utilization of the land by human activities for the purpose of settlements, agriculture, forestry, and by pasture altering land surface processes including biogeochemistry, hydrology and biodiversity (Di Gregorio and Jansen, 2000). In this context, as variation in the surface component of the landscape and is only considered to occur if the surface has a different appearance when viewed on at least two successive occasions (Lemlem, 2007). The definition also given by FAO (1999) for land-use is as the arrangements, activities and inputs people undertake in a certain land cover type to produce change or to maintain it. According to Lambin and Meyfroidt (2010), transition in LU/LC can be caused negative socio-ecological feedback that comes from a rigorous (severe) degradation in ecosystem services/ as a result of from socio-economic changes and innovations.
2.2. Causes of land-use and land-cover changes Changes in the land-use reflect the history and, perhaps, the future of humankind. Such changes are influenced by a variety of factors related to human population growth, economic development, technology and environmental changes (Houghton, 1994). Land-cover changes, which is conversion of the land-cover from one type of to another and modification of the conditions within a category and land-use change occurs initially at the level of land parcels when land managers decide that a change towards another land utilization type is desirable (Meyer and Turner, 1992). Population growth is one of the major factors for LU/LC change. People are the most important natural resources, which is mutually inter-related and interdependent for their sustainable development
AAU.Remote Sensing and Geo-informatics stream: By Belete Tafesse: [email protected] 7
(Santa, 2011). However, Land-use reflects the importance of land as a key and finite resource for most human activities such as forestry, agriculture, industry, energy production, recreation, settlement and water catchment and storage (http://www.ciesin.org/docs/002-105/002-105b.html ). During the past 3 centuries, the extent of earths cultivated land has grown by more than 45% increasing from 2.65 million km² to 15 million km² and at the same time, other natural resources (land-cover) such as forest has been shrinking due to agricultural land expansion and urbanization(Santa, 2011). High rate of deforestation in many developing countries is most commonly associated with population growth and poverty (Mather and Needle, 2000).
Land-use/land-cover changes have become major problems for the world, and it is a significant driving agent of global environmental changes (FAO, 1999). Such a large-scale land-use classes through the increase of agricultural land at rural area, deforestation (clearance of trees), urbanization and other natural phenomena and human activities are inducing changes in global systems and cycles. However, the major change in land-use, historically, has been the worldwide increase in agricultural land (Houghton, 1994). Climate change refers to long term or permanent shift in climate of the area. Some of the evidence for climate change includes increased frequency of the occurrence of drought, global temperature rise, tropical cyclones, flood, and reduced annual rainfall reduction in glacial cover over mountain and rising sea levels (Alemayehu, 2008).
United States Environmental Protection Agency (USEPA, 2004), identified the general causes of LU/LCCs are:
Natural processes, such as climate and atmospheric changes, wildfire and pest infestation. Direct effect of human activity such as road and illegal house construction and deforestation (clearance of trees). Indirect effects of human activity is such as, water diversion leading to lowering of the water table.
2.3. Land-use and land-cover change in Ethiopia In Ethiopia, the availability of natural resources changes and management differs significantly from place to place. This variability is because difference in biogeography and topography climate. Land- use/land-cover changes are accelerating, by human actions, but also producing changes that affect humans (Agarwal et al., 2002). The dynamics of LU/LC alters the existence of different biophysical
AAU.Remote Sensing and Geo-informatics stream: By Belete Tafesse: [email protected] 8
resources, including water, vegetation, soil, animal feed and other (Ali, 2009). Previous studies reported that, in Ethiopia there have been considerable LU/LC changes in different part of the country and the expansion of cultivated land at the expense of forestland and it were stretched into sloppy area due to scarcity of land. Especially in the highlands of Ethiopia, agricultural practices and human settlement have a long history and recently a highland population pressure including depletion of natural resources and unsustainable practices (Miheretu andYimer, 2017).
2.4. Remote Sensing Remote sensing is the capability to gather information without being in direct contact with it (Lillesand and Kiefer, 2000). The modern use of the term remote sensing has more to do with technical ways of collecting airborne and space born information. The earth observation from airborne platforms has 150 year of history, although the majority of the innovation and development has taken place in the recent decade’s years (Zubair, 2006). The first earth observation-using balloons in the 1860s were regarded as an important benchmark in the history of remote sensing (Lillesand and Kiefer, 2000).
Information is gathered by instruments at the natural level by our naked eyes, or by cameras (radiometric which measure radiation). Satellite based remote sensing provides valuable information that can be used in the assessment of the various aspect of atmospheric environment, climatology, meteorology, ecology, agronomy and environmental protection (Kern, 2011). The essence of remote sensing is measuring and recording of the electromagnetic radiation emitted or reflected from the earth’s surface (Hardegree, 2006). This technique enables us to investigate and know the tendency of LU/LC change through time.
2.5. Geographic Information System (GIS) Geographic Information System is a system designed to capture, store, manipulate, analyze, manage and present spatial or geographic data (Coppin and Bauer, 1996). The data type in GIS can be classified into two major groups as spatial and non-spatial data. The spatial data are the data that have location value and that non-spatial data are a data, which describe more the spatial data in the form of a table. According to Burrough (1990), data in GIS is composed of three dimensions that mean spatial (geographic), time and attribute. Some people believe that geographic information system as the system of hardware and software, which contribute to analyze applications or information processing (Maguire, 1991). Geographic information system is not only digital store of
AAU.Remote Sensing and Geo-informatics stream: By Belete Tafesse: [email protected] 9
spatial objectives (areas, points and lines) but also capable of spatial analysis based on the relation between these objects, including the r/ship between objects defined by their location and geometry.
According to Foresman (1998), the combination of computer technology and cartography in the 1960s paved the way for the possibility of using techniques of superimposing and overlaying maps in fields other than cartography. The power multiplication, which results from the integration of climate, environment, terrain, agronomic, economic, social and institutional management data, makes available for managers and scientists alike a new and powerful modeling.
2.6. Role of Remote Sensing and GIS in Land-use and Land-cover change Remote sensing and geographic information system techniques have been widely used over the world for the study of historical changes in LU/LC and LST analysis. Remote sensing has been used to identify vegetation cover, air pollution, LST and other surface characteristics (Zha, 2012; Weng, 2004). Furthermore, understanding the correlation between LST and LU/LC is important to manage the land. It provides a large variety and amount of data about the earth’s surface for detailed analysis, change detection with the help of various airborne, and space born. With the availability of historical remote sensing data, the reduction in data cost and increased resolution from satellite platforms, remote sensing technology appears ready to make an even greater impact on monitoring land-cover change. Land-use/land-cover changes can be analyzed over a period using Landsat sensors such as Landsat Multi Scanner (MSS) data and Landsat Thematic Mapper (TM) data by image classification techniques (Gumindoga, 2010).
Since 1972, Landsat satellites have provided repetitive, synoptic, global coverage of high-resolution multispectral imageries. Their long history and reliability have made them a popular source for documenting changes in LU/LC over time(Turner et al., 2003) and their evolution is further marked by the launch of Landsat7 (Enhanced Thematic Mapper Plus sensors) by the United State in 1999.
According to Macleod and Congation (1998), there the following are four LU/LCs change detection (aspects of change detection), which are important when monitoring natural resources:
Distinguishing the nature of the change Detection/finding of the changes that have occurred Measuring the area extent of the change Assessing and investigating spatial pattern of the change
AAU.Remote Sensing and Geo-informatics stream: By Belete Tafesse: [email protected] 10
The basis of using remote sensing data for change detection is that changes in land-cover result in changes in radiance values, which can be remotely sensed.
2.7. Land surface temperature Land surface temperature denotes the temperature on the surface of the earth or it is the skin temperature of the earth surface phenomena (Kayet et al., 2016). From the satellite’s point of view the ‘surface ‘looks different for different area at different times (Kumar and Singh, 2016). Remote Sensing and geospatial tools play crucial role in quantifying and estimating LST. Land surface temperature is derived from geometrically corrected Landsat Thermal Infrared (TIR) band 6 and Landsat 8 thermal infrared (TIR) band 10 and 11(Khin et al., 2012). Land surface temperature of a given area can be determined based on its brightness temperature and the land surface emissivity, which is calculated through applying the split window algorithm (Rajeshwari and Mani, 2014, Md Shahid, 2014). According to Kerr et al. (2004) land surface temperature gives information about the difference of the surface equilibrium state and vigorous/vital for many applications. LST also defined as, the monitoring of surface temperature based on pixel derived observation through remote sensing (Paramasivam, 2016). The characteristics of urban land surface temperature is depending up on its surface energy balance, which is governed by its properties such as orientation, sky and wind, openness to the sun and radiative ability to reflect solar and infrared and also ability to emit infrared availability of surface moisture to evaporate and roughness of the surface (Voogt, 2000). Land-use/land-cover changes due to changes in surface temperature (ST) which makes both urban and rural managers to estimate the urban surface temperature and its surrounding rural area for urban planning as well land management in general (Becker et al., 1990).
2.8. Urban heat island Urban heat island (UHI) is an urban area or metropolitan area that is significantly warmer than its surrounding rural areas due to human activities. The term heat island describes built up areas that are hotter than nearby rural areas (Sobrino et al., 2012). Urban heat island also defined as phenomenon/events that occurs when air and surface temperatures (ST) in urban areas became significantly greater than those experienced in nearby area and land-cover change has become a central component in current strategies for managing natural resources and monitoring and environmental changes (Sobrino et al., 2012). According to the Intergovernmental Panel on Climate Change (IPCC) Report, climate change has contributed to a significant increase in the global mean
AAU.Remote Sensing and Geo-informatics stream: By Belete Tafesse: [email protected] 11
temperature (IPCC, 2014). There are number of contributor factors, which play significant role in creation of urban heat island such as low albedo materials, wind blocking, air pollutants, human gathering, distractions of trees, and increased use of air conditioner (Nuruzzaman, 2015).
2.9. The impact of land-use/land-cover change on land surface temperature One of the major factors that are responsible for the increase of land surface temperature is LU/LCC and Different researchers agree with that land-use change and unplanned use of land resources lead to increasing land surface temperature. Oluseyi et al., 2011 have studied that spatially there are correlations with changes as reflected in the characteristics of individual land-use classes or categories. This study emphasize the changes in LST of the various land-uses between 1995 and 2006 in the case of Anyigba Town; Kogi State, Nigeria. It shows that there was 1ºC variation and increase in surface temperature of vacant land, built-up area and stream, while cultivated land and vegetation also had increases of 0.95ºC ,respectively. The influence of LU/LC changes on LST is different at different latitude, for example in South Asia and East Asia tropical temperate regions (Shukla, 1990). The relationship between land-use changes, biodiversity and land degradation across East Africa shows that, land cover were transformed to grazing farmland and settlement area (Matimal et al., 2009).
According to Yue et al. (2007), the relationship between NDVI and LST with integrated remote sensing application to quantify Shanghai Landsat7 ETM+ data was used. The result shows that different LU/LC classes have significantly different impacts on land surface temperature and normalized difference vegetation index calculated by the Enhanced Thematic Mapper Plus sensor in the Shanghai urban environment.
2.10. Normalized Difference Vegetation Index Normalized Difference Vegetation Index is the difference of near infrared and visible red reflectance values normalized over reflectance and calculated from reflectance measurements in the near infrared (NIR) and red portion of the spectrum (Burgan and Hartford, 1993). To calculate the Normalized Difference Vegetation Index, subtracting the red band from near infrared and then dividing to near infrared plus red band. The value is ranging from -1 to1, the negative values are indicative of water, snow, clouds, non-reflective surface and other non-vegetated, while the positive value expresses reflective surfaces such as vegetated area (Burgan and Hartford 1993). Vegetation has a direct match/correspondence with thermal, moisture and radiative properties of the earth’s
AAU.Remote Sensing and Geo-informatics stream: By Belete Tafesse: [email protected] 12
surface that determine LST (Weng, 2004). In addition to NDVI, Normalized Difference Moisture Index (NDMI) also used as an alternative indicator of surface urban heat island effects in Landsat imagery by investigating the r/ships between land surface temperature and NDVI. The index is expressed as NDMI= (NIR-IR)/ (NIR+IR), it evaluates the different content of humidity from the landscape elements, especially in soils, rocks and vegetations and it is an excellent indicator of dryness. Values greater than 0.1 are symbolized light colors and they signal high humidity level, whereas values close to -1 symbolized by dark colors represents low-level humidity level (Mihai, 2012).
Previously, different researchers outside Ethiopia did researches in relation to the impact of LU/LC changes on land surface temperature. However, in Ethiopia there are some papers related to the proposed title. For example, Gebrekidan,2016 studied modeling land surface temperature from satellite data, the case of Addis Ababa, which presented in Africa hall, United Nations conference centre Addis Ababa; Ethiopia (ESRI Eastern Africa Education GIS conference which held from 23−24 September, 2016). The study mainly focuses on modeling LST of Addis Ababa city, which acquired Landsat 5 and 8, from 1985 and 2015. Finally, the results show that negative correlation was found between Normalized difference vegetation index and Land Surface Temperature and the study indicates the need for urban greening and plans to increase vegetations covers to sustain the ecosystem of the city and to minimize urban heat island effect.
According to streutker (2003), one of the promising of studying urban surface temperature is using remote sensing or air born technology. Evaluation of land surface temperature from remotely sensed data is common and typically used in studies of evapotranspiration and desertification processes. Further, (Walsh et al., 2011) stated that urban area such as buildings and roads and infrastructures or anthropogenic factors contribute to increase atmospheric temperature. The wide use of land surface temperature for environmental studies, have made remote sensing of land surface temperature important academic issue during the last decades. Indeed, one of the most important parameters in all surface atmosphere interactions and fluxes between the land and the atmospheric is land surface temperature (Buyadi et al, 2013).
AAU.Remote Sensing and Geo-informatics stream: By Belete Tafesse: [email protected] 13
CHAPTER THREE
3. MATERIALS AND METHODS
3.1. Description of the study area
3.1.1. Location Adama Zuria Woreda (Adama and surrounding Woreda) is one of the Woredas in the East Shewa Zone of the Oromia, Regional State of Ethiopia. The Administrative center of the Woreda is Adama, which is located southeast of Addis Ababa the capital city of Ethiopia approximately about 90 km at latitude and longitude of 8° 14′ 0″−8° 43′ 0″N and 39° 6′ 0″−39° 25′ 0″ E, covering a total area of 901.5 km2 (Fig.3.1). Altitude of the area range from 1415 to 2505 m above sea level and it is located within the Great Ethiopian Rift Valley.
Figure 3.1: Location map of the study area.
AAU.Remote Sensing and Geo-informatics stream: By Belete Tafesse: [email protected] 14
3.1.2. Topography Topography is the study of the shape and feature of the surface of the earth and other related phenomenon or it is an integral part of the land surface. It includes such as landforms, elevation, latitude, longitude and topographic maps. The Adama Zuria Woreda has a broad flat area. However, in its northwest part there is relatively rugged topography. It also bordered on the south by the Arsi Zone , on the Southwest by Koka reservoir , which separates it from Dugda Bora on the west by Lome on the north by the Amhara Region , and on the East by Boset ; the Awash River, the only important river in this Woreda, defines the Woreda boundaries on the east and south. The elevation of the study area is indicated in (Fig. 3.2 a).
Slope of Adama Zuria Woreda shows that, 61.73% of the area is between 0 and 3 º. 26.55% of the area is between 3 and 7 º, 8% of the area between 7 and 14 º, 3% of the area 15 between 24 º and 0.62 % of the area is more than 24 º of slope. Slope of the area with greater than 24 º is located in the northern, northwest, southwest ridge part of the area. The slope of the area is indicated in (Fig.3.2 b).
Figure 3.2: (a) Elevation and (b) Slope map of the Study area.
AAU.Remote Sensing and Geo-informatics stream: By Belete Tafesse: [email protected] 15
3.1.3. Climate and Vegetation A. Rainfall A long-term rainfall record from1989 to 2016 at the Adama meteorological station shows an average annual rainfall of 844. 20 mm and the maximum monthly average rainfall was 259.8 mm in the month of July (Fig.3.3). On the average, most rainfall or rainy season is June, July, August and September. Whereas, the dry month is in January, October, November and December. On average, the warmest month is May and coolest month is July. Among all month, the driest month is December.
100 Rainfall (mm) Rainfall
Figure 3.3: Monthly average rainfall distribution of the Study Area.
The average rainfall recorded between 1989 and 1999 was 709.9 mm, while 1999 and 2010; it was 833.24 mm and 745 for the years between 2010 and 2016. The distribution of annual rainfall from (1989–2016) is presented in (Fig.3.4).
AAU.Remote Sensing and Geo-informatics stream: By Belete Tafesse: [email protected] 16
Rainfall (mm Rainfall 200
2002 1989 1990 1991 1992 1993 1994 1995 1996 1997 1998 1999 2000 2001 2003 2004 2005 2006 2007 2008 2009 2010 2011 2012 2013 2014 2015 2016
Figure 3.4: Distribution of annual rainfall in the study area (1989–2016).
B. Temperature The mean annual temperature of the Adama Zuria Woreda is 27.8 . It can be classified as semi- humid to semi-arid climate, which characterizes the altitude range between 1,500–2,400 m above mean sea level. In the study area, the hottest month with maximum mean temperature of 30.7ºC was May. The detailed information presented in (Fig. 3.5).
C) C) 30 ° 25 20 15 Temp Max
10 Temp Min Temperature ( Temperature 5 Mean
Figure 3.5: Maximum, minimum and mean monthly Temperature during 1989–2016.
AAU.Remote Sensing and Geo-informatics stream: By Belete Tafesse: [email protected] 17
C. Vegetation The vegetation distribution of the area is mainly dependent on the climate condition of the area. The climate condition of the study area is characterized as tropical. On these types of climate, vegetation is scarce and typical example that is found in the area is shrub, Acacia and scattered trees of Eucalyptus. Eucalyptus trees, which is, grown by local communities in soil conservation program that is applied in the Main Ethiopian rift to protect soil from erosion. The local people cultivate, some types of crops cultivated in the area are Teff, wheat, Barley, maize and sorghum. The harvesting season is between October and December at which the rain is very low.
3.1.4. Population According to CSA (2007), the total human population of Adama Zuria Woreda including Adama Town is about 375,561. Out of this, 187,676 are women and 187,885 are men. While in 2014 CSA projected data (estimated population) the total population is about 473,385. From this, about 237,541 are women and 237844 are men. The detail information is presented in (Fig.3.6).
500,000 450,000 400,000 350,000 300,000 250,000
200,000 Population 150,000 100,000 50,000 0 Female Male Rural Urban Total population population population population population 2007 187,676 187,885 129,027 246,534 375,561 2014 237,541 237,844 153,501 319,884 473,385
Figure 3.1: Population of Adama Zuria Woreda.
3.2. Data and software
Data used for this research comprise both primary and secondary (Ancillary). The most important source was the primary data (satellite data). Ancillary source of data was collected from governmental and nongovernmental organization and published information.
AAU.Remote Sensing and Geo-informatics stream: By Belete Tafesse: [email protected] 18
3.2.1. Primary data
The primary data includes Landsat satellite image (Landsat data): Thematic Mapper (TM) 1989, Enhanced Thematic Mapper Plus (ETM+) 1999 and Operational Land Imager/Thermal Infrared (OLI/TIRS) obtained from USGS. Digital Elevation Model (DEM) data with the resolution of 30*30m for mapping the elevation and slope of the area and field data, GPS point and photo of different classes of LULC were used.
3.2.2. Remote Sensing data acquisition One scene of Landsat 4 TM (1989), Landsat7 ETM+ (1999) and Landsat 8 OLI/TIRS (2016) cloud free image of the study area with the path of 168 and row 054 were acquired (downloaded) from the website of earth explorer. usgs.gov, United States Geological survey (USGS) and Landsat8 from https://libra.developmentseed.org.The-acquired data is world datum (WGS84) projection system.
Remote sensing images used to calculate LST, NDVI and LULC in Adama Zuria Woreda are shown in the following (Table 3.1) and multispectral band in (Fig.3.7).
Table 3.1: Remote sensing data used in the study.
Spatial Spectral Date of Multispectra Resolution Thermal range Acquisition Sensor Path Row l Band (Pixel Source Band (micromete Spacing) rs) 11/21/1989 TM 168 054 1 to5 and7 6 10.45-12.45 120
USGS ETM+ 168 054 1 to5 and7 6 10.45-12.51 60 12/3/1999 OLI 10 and 11/23/2016 and 168 054 1 to7 and9 10.60-12.51 100 11 TIRS
AAU.Remote Sensing and Geo-informatics stream: By Belete Tafesse: [email protected] 19
Figure 3.7: Landsat Images of 1989 TM, 1999 ETM+ and 2016 OLI and TIRS.
AAU.Remote Sensing and Geo-informatics stream: By Belete Tafesse: [email protected] 20
A. Landsat Thematic Mapper (TM)
The Landsat Thematic Mapper (TM) sensor was carried on-board four and five from July 1982 to May 2012 with 16-day repeat cycle. Thematic Mapper sensor has seven spectral bands; three in the visible range and four in the infrared range. Band 6 is specifically sensitive to thermal infrared radiation to measure surface temperature. Detailed information is shown in Table 3.2.
Table 3.2: Landsat 4 Thematic Mapper sensor bands and description.
Spatial Wavelength Repeating Bands Description resolution Source (Micrometers) time (m) Band 1 Blue 0.452-0.518 Band 2 Green 0.528-0.609 Landsat4 Band 3 Red 0.626-0.693 Thematic Near Infrared 30 Band 4 0.776-0.904 Mapper (NIR) 1 16 days USGS (TM) Near Infrared Band 5 1.567-1.784 (NIR) 2 Band 6 Thermal 10.45-12.45 120 Middle Infrared Band 7 2.097-2.349 30 (MIR)
B. Landsat 7 Enhanced Thematic Mapper plus (ETM+)
The Landsat Enhanced Thematic Mapper Plus sensor onboard the Landsat 7 satellite has acquired images of the Earth nearly continuously since July 1999,with a 16 day repeat cycle. Landsat 7 ETM+ images consist of eight spectral bands with a spatial resolution of 30m for bands 1-5 and 7 where as the thermal band 6 and panchromatic band 8 has a resolution of 60 and 15 m, respectively. Detail information is shown in Table 3.3.
AAU.Remote Sensing and Geo-informatics stream: By Belete Tafesse: [email protected] 21
Table 3.3: Landsat 7 Enhanced Thematic Mapper Plus bands and description.
Spatial Repeati Satellite Bands Description Wavelength resolution (m) ng time Source /sensor (micrometer ) Band 1 Blue 0.452-0.514 Landsat 7 Band 2 Green 0.519-0.601 Enhanced Band 3 Red 0.631-0.692 30 Thematic Band 4 Near Infrared 0.772-0.898 Mapper (NIR) 1 Plus Band 5 Near Infrared 16 days USGS 1.547-1.748 (ETM+) (NIR) 2 Band 6 Thermal 10.31-12.36 60 Band 7 Middle 2.065-2.346 30 Infrared (MIR) Band 8 Panchromatic 0.515-0.896 15
C. Landsat 8 Operational Land Imager (OLI) and Thermal Infrared Sensor (TIRS)
Images consist of nine spectral bands with a spatial resolution of 30 m for band 1-7, 9, and TIRS with two bands (band 10 and 11). New band 1 (ultra-blue) is useful for coastal and aerosols studies. Another new band 9 is useful for cirrus cloud detection. Landsat 8 was launched in February 11, 2013 (Table 3.4).
AAU.Remote Sensing and Geo-informatics stream: By Belete Tafesse: [email protected] 22
Table 3.4: Landsat 8 Operational Land Imager and Thermal infrared Sensor bands and description.
Satellite/ Wavelength Resolutio Repeating Source Band Description sensor (micrometers) n (m) time Band 1 Coastal aerosol 0.43-0.45 Band 2 Blue 0.45-0.51 Band 3 Green 0.53-0.59 Band 4 Red 0.64-0.67 Near Infrared 30 Band 5 0.85-0.88 (NIR) Landsat Short -Wave Band 6 1.57-1.65 8 Infrared (SWIR) 1 16 days USGS OLI/TIR Short -Wave Band 7 2.11-2.29 S Infrared (SWIR) 2 Band 8 Panchromatic 0.50-0.68 15 Band 9 Cirrus 1.36-1.38 30 Thermal Infrared Band 10 10.60-11.19 (TIRS) 1 100 Thermal Infrared Band 11 11.50-12.51 (TIRS) 2
3.2.3. Field data Field data used for this research was from GPS data and used for accurately checking the LU/LC classified image into different classes. After Random point, generated GPS point was collected and pictures captured showing different LU/LC classes. Field data was used for validation accuracy assessment based on ground truth.
3.2.4. Secondary data Secondary data used in this study comprises meteorological data such as average monthly temperature (1989–2016) and average monthly rainfall (1989–2016).These meteorological data was used for evaluation and validation. In addition to this, the other data used in this study were
AAU.Remote Sensing and Geo-informatics stream: By Belete Tafesse: [email protected] 23
geological map from Ethiopian Geological Survey to see the geological setting of the area and population data from CSA to understand the status of the population of the study area (CSA, 2007).
3.2.5. Data description and source Primary and ancillary data that used in this study was collected from different sources as indicated in Table 3.5.
Table 3.5: Data Description and source used in this study.
GIS Data layer Data Description Data source Vector (polygon) Woreda (Woreda) Boundary CSA,2008 Vector (line) River and Roads CSA,2008 Vector (point) Major Town Ethio-GIS Raster Elevation and Slope DEM 30m SRTM Attribute table Rainfall and Temperature National Metrological Agency Attribute table Population CSA,2007
Raster Satellite Image USGS 1989,1999 and 2016 1989 Landsat4 TM 1999 Landsat7ETM+ 2016 Landsat 8 OLI and TIRS
Ground truth Ground truth 2016 Field survey
3.2.6. Software Packages used Software packages used for this study were ArcGIS 10.3 for image analysis; calculate LST, NDVI and map preparation, ERDAS (Earth Resources Data Analysis System) Imagine 2014 for RS application in order to process satellite images including image enhancement, preprocessing and for LU/LC classification.
PCI Geomatica 2015 was also used for top of atmospheric correction, change detection and export to Google earth. Google earth also used to check and compare results with ground truth, especially
AAU.Remote Sensing and Geo-informatics stream: By Belete Tafesse: [email protected] 24
for the year 1989 and 1999 LU/LC verification. Quantum GIS (QGIS): open source software was used for raster manipulation include neighborhood analysis and map algebra. In addition to ERDAS Imagine software, ENVI software used for classification to compare and contrast the results. Microsoft office, Microsoft Excel and Microsoft power point was used to create word documents, analyze spreadsheets, to do graphs, manage databases and for presentation.
3.3. Methods In this study, the methods used to achieve the objectives of the research were begun with acquisition of Landsat imagery for the year 1989, 1999 and 2016 from website of earth explorer (USGS) and Landsat 8 from https://libra.developmentseed.org. The reason behind that these years chosen were because of the availability of the data. The image acquired or downloaded was during the dry season and was cloud free image. Figure 3.8 show the general methodological flow chart of the study.
AAU.Remote Sensing and Geo-informatics stream: By Belete Tafesse: [email protected] 25
Landsat satellite imagery Ancillary L4 TM (1989), L7 ETM+ Data (1999), L8 OLI/TIRS 2016
Multispectral Thermal Band infrared Band Clipping of thermal bands Meteorologic Image Pre-processing: al data Geometric correction, subsetting,Enhancement
Band Rationing Conversion to at sensor Selecting Thematic Radiance classes and Training samples
NDVI using NIR At sensor Image &red band brightness classification Statistical temperature analysis& interpolation Maximum Supervised Likelihood classification method If not Land surface NDVI Emissivity Accuracy Ground assessment Truthing
If yes Spatial projected LST Map of Change metrological map 1989, 1999 & Detection NDVI 2016 1989, 1999&2016 LULC Map of Zonal 1989,1999 & Validation statistics 2016
Comparison of LST and LULC
Figure 3.8: Methodological flow chart of the study.
AAU.Remote Sensing and Geo-informatics stream: By Belete Tafesse: [email protected] 26
3.3.1. Data Preparation and Analyzing Satellite image by its nature have some distortion, noise, haze and stripes. Therefore, before processing the data, image pre-processing activities were done. Preprocessing includes importing, layer stacking, and subsetting of the image based on the boundary of Adama Zuria Woreda, geometric correction, radiometric correction, and removal of stripes, pan sharpening and other image enhancement techniques. Radiometric correction is a removal of atmospheric noise to make more representatives of the ground truth conditions based on the sensors. These all previously mentioned activities done were to improve visible interpretability of an image by increasing apparent distinction between the features in the scene.
In addition, the image was also georeferenced-using boundary of the Woreda. During georeferencing and reprojecting process, Adindan UTM Zone 37N coordinate system was followed for raster and vector data in the study to maintain uniformity. Adindan UTM Zone 37N is local datum that Ethiopia used.
3.3.2. Digital Image Processing (DIP) A digital image is a numeric representation of (binary) a two dimensional image or digital image is a sampled quantized numeric representation of the scenes and made up of picture element called pixels. It involves the manipulation and interpretation of digital images with the aid of computers. In remote sensing digital image, processing historically stems from two principal application areas, the improvement of the information for human interpretation and the processing of image data for computer-assisted interpretation. The whole activities of DIP revolve around increasing spectral separability of the objects on the image.
3.3.3. Image enhancement Image enhancement is the procedure applied to image data in order to make more effectively display or record the data for subsequent visual interpretation. Normally, image enhancement involves techniques for increasing the visual distinction between features in the scene (Billah and Rahman, 2004).
The main purpose of image enhancement is to improve the interpretability of information in images for human viewers, or to provide better input for other automated image processing techniques.
AAU.Remote Sensing and Geo-informatics stream: By Belete Tafesse: [email protected] 27
3.3.4. Image classification In remote sensing, Image classification is the task of extracting information classes from a multiband raster image or extracting information based on the reflectance of the object and it serves specific aims; which is converting image data into thematic data. Digital image classification techniques assemble pixels to represent LU/LC classes. Image classification uses the reflectance statistics for individual pixels. Pixels were grouped based on the reflectance properties of pixels called clusters. The users identify the number of clusters to generate and which bands to use. With this information, the image classification software generates clusters. In this research supervised classification techniques is used.
A. Supervised classification
Supervised classification is the techniques most often used for the quantitative analysis of RS image data depending on their reflectance properties. It uses the spectral signature obtained from training samples to classify an image. Image classification toolbar, can easily create training samples to represent classes. With supervised classification, it can be identified sample of information classes (any land-cover type) of interest in the image. The supervised classification image of each year involves pixel categorizations by taking training area for each class of LU/LC. After the training area assigned for each class classification activity was performed. For bare land, farmland, shrub land and settlement LU/LC types taken 20 training site, where as for plantation and water body LU/LC types was taken 15 training areas as sample. Areas in digital images were marked as signature of individual identity and the field truth verification was adapted to represent LU/LC class (Coppin and Bauer, 1996).
Using Multispectral Band from band 1 to 5 and 7 for TM 1989 and ETM+ 1999 and OLI 2016 1 to 7 Bands of the preprocessed images the land-use/ land-cover pattern mapped was by supervised classification with the likelihood classification algorithm of ERDAS Imagine 2014 software. In supervised classification, with the help of image processing techniques, the user specified type of the land-use land-cover classes. The six major classes studied in Adama Zuria Woreda were Farm Land, bare Land, settlement, shrub land, plantation and water body. The advantage of the supervised classification was development of information classes, self-assessment using training sites and training sites reusable. However, information classes may not match spectral classes, the signature homogeneity, uniformity of information classes may be varies.
AAU.Remote Sensing and Geo-informatics stream: By Belete Tafesse: [email protected] 28
B. Maximum Likelihood classification
Maximum likelihood classification (MLC) is one of the most known methods of classification in remote sensing, in which a pixel with the MLC is classified into the matching classes/categories. It is a statistical decision measure to assist in the classification of overlapping signatures; pixels are assigned to the class (categories) of the highest probability. It was considered more accurate than parallelepiped classification. However, it is slower to extract computations. The MLC classification tool considers both the variances of the class signatures when assigning each cell to one of the classless represented in the signature file.
C. Ground Truthing
A ground truthing activity was carried out in the study area, in which different LU/LC classes were validated. The observed LU/LC includes: farmland (small scale agriculture),shrub land, sugarcane plantation (large scale agriculture),water body ,bare land and settlement (urban and rural settlement).These LU/LC classes were used in producing the map legends and with the assist of GPS, training set data used for image classification were acquired. During this ground truthing activities, photos of scenes of interest and coordinates from sampled LU/LC classes were captured.
3.3.5. Classification accuracy assessment Assessing classification accuracy requires the collection of some original data or a prior knowledge about some parts of the terrain, which can then be compared with the RS derived classification map. Thus, to assess classification accuracy, it is necessary to compare the following two-classification map:
The remote sensing (RS) derived map Assumed true map (it may contain some error).
The supposed true map may be derived from in situ examination or quite often from the interpretation of remotely sensed data obtained at a larger scale or higher resolution. Shortly, accuracy assessment is performed by comparing the created by RS analysis to a reference map based on a different information sources. Using hand held Global Positioning System (GPS) field survey was conducted in the study area and about 60 points identified. The field survey and Google Earth were used as a ground for evaluation the LU/LC classification accuracy. The final output of
AAU.Remote Sensing and Geo-informatics stream: By Belete Tafesse: [email protected] 29
classification accuracy was calculated for the years 1989, 1999, 2016 Land-use/Land-cover Map. Land-use and Land-cover classes and its description are presented in Table 3.6.
Table 3.6: Land-use/land-cover classes and description of the study area.
LU/LC classes Description
Areas covered with shrubs, bushes and small trees, with little useful Shrubs land wood, mixed with some grasses and less dense than forests.
Areas used for crop cultivation, both annual perennials, and the Farm land scattered rural.
Area occupied by houses buildings including road network residential, Settlement commercial, industrial, transportation, roads, mixed urban and other facilities.
Areas covered by natural and manmade small dams, like pond, lake Water body and river.
Plantation agriculture is a form of commercial farming where crops Plantation were grown for profit. it includes sugarcane, sweet potato and tobacco.
Areas of has thin soil, sand/rocks and includes deserts, dry
Bare land Salt flats, beaches, sand dunes, exposed rocks, stripe mines, queries and gravel pits/non-vegetated area dominated by rock out crops, roads, eroded and degraded lands.
AAU.Remote Sensing and Geo-informatics stream: By Belete Tafesse: [email protected] 30
To determine and classify LU/LC of the study area; prior knowledge about the area is important. During field observation, there were six major LU/LC class identified. Based on the coordinate point Sample of LU/LC class and LST values of each LU/LC of the study area was shown in figure 3.9.
Bare land Shrub land
Settlement Sugarcane Plantation
Water body Farmland
Figure 3.9: Interpretation of Landsat image for LU/LC classification.
AAU.Remote Sensing and Geo-informatics stream: By Belete Tafesse: [email protected] 31
Steps or processes that were followed to classify land-use/land-cover from a Landsat image were presented in Fig 3.10.
Landsat imagery 1989 TM,
1999 ETM+ & 2016 OLI
Geometrically corrected
Land-use land-cover categories /class determined
Selecting Training area for ground truthing and visual interpretation Primary data
Cross check using Supervised classification Google earth on ERDAS Imagine 2014
environment Field data If not Field observation Edit/evaluate signatures Secondary/ancillary data If yes
Classify image Toposheet
Evaluate classification
Final data / map of land-use /land-cover 1989, 1999 & 2016
Figure 3.10: Steps and procedures followed to classify land-use/land-cover from a Landsat image.
AAU.Remote Sensing and Geo-informatics stream: By Belete Tafesse: [email protected] 32
3.3.6. Land-use/Land-cover change detection Land-use/land-cover change detection was done by involving images of 1989, 1999 and 1999 and 2016. Using GIS techniques thematic image was compared. The cross operation process of mapping LU/LCC over time began with mapping the recent 2016-satellite imagery, then looking back in time to map the 1989 imagery. Post classification is among the most widely used approach for change detection purpose (Chen, 2000). The analysis of LU/LCC maps involved technical procedures of integration using the ArcGIS software techniques. The first task was to develop a table indicating the area coverage in square kilometers and the percentage change for each year 1989, 1999 and 2016 measured against each LU/LC classes. Therefore, to calculate LU/LCC in percentage equation (eq.3.1) were used (Lambin et al, 2001).
Percentage change = ×100 Eq. (3.1)
3.4. Derivation of Normalized Difference Vegetation Index and Land surface temperature
3.4.1. Derivation of Normalized Difference Vegetation Index According to Farooq (2012), the reason NDVI relates to vegetation is that, the one which is well vegetated reflects better in the near infrared part of the spectrum. Green leaves have a reflectance of 20% or less in the 0.5 to 0.7 range and about 60% in the 0.7 to 1.0 micrometer range. The value of NDVI is between -1and 1. Normalized difference vegetation index (NDVI) was acquired from spectral reflectance measurements in the visible (RED) and near infrared regions (NIR) in the ArcGIS environment.
The index was defined by the following equation 3.2.
NDVI = Eq. (3.2)
NDVI=Normalized Difference Vegetation Index
NIR= is the near infrared band 4, R= is the red band 3. Equation 3.2 is used to calculate NDVI for the sensor TM 1989, ETM+ 1999 and OLI 2016.But in case of Landsat 8 NIR is band 5 and the red band is a band 4 (Weng et al, 2004).
AAU.Remote Sensing and Geo-informatics stream: By Belete Tafesse: [email protected] 33
To calculate fractional vegetation cover (FVC) the following Eq. 3.3 was used.
FVC= Eq. (3.3)
FVC=Fractional Vegetation cover
NDVI= Normalized Difference Vegetation Index
The above equation (FVC) was used to get fraction an area with vegetation cover using NDVI value.
= is NDVI for soil and NDVI for vegetation
Equation 3.4 was used to calculate Proportion of Vegetation that helps in calculating Landsat 8 land surface emissivity (LSE).
Pv = (NDVI-NDVImin/NDVImax-NDVImin Eq. (3.4)
Pv =Proportion of Vegetation
NDVI min= Normalized Difference Vegetation Index minimum value
NDVImax= Normalized Difference Vegetation Index maximum value
3.4.2. Derivation of land surface temperature Calculating land surface temperature passes different steps.
To calculate the LSE, it is important know the inherent characteristics of the earth’s surface and change the thermal radiance energy during calculating LST (Sobrino et al., 2014). The emissivity constant values for vegetation and soil are stated in Table 3.7.
AAU.Remote Sensing and Geo-informatics stream: By Belete Tafesse: [email protected] 34
Table 3.7: Emissivity constant value of Landsat 8.
Emissivity Band 10 Band 11
0.971 0.977
0.987 0.989
The following equation 3.5, 3.6 and 3.7 used to compute Land surface Emissivity, mean of LST and change of LSE of Band 10 and 11, respectively.
LSE=0.004Pv+0.986 Eq. (3.5)
Mean of LST= Eq. (3.6)
Difference of land surface emissivity (LSE) =LSE10-LSE11 Eq. (3.7)
3.4.3. Radiometric correction Radiometric correction requires converting a remote sensing digital number to spectral radiance values and data for comparisms. Image processing procedures that are used to correct errors, converting digital number (DN) values to radiance and then reflectance was categorized as a Radiometric correction (Parente, 2013). To perform the conversion of digital number to spectral radiance equation (3.8) was used.
L λ =Lmin+ (Lmax-Lmin)*DN/255 Eq. (3.8)
Where, L λ=spectral radiance Lmin=spectral radiance of DN value 1 Lmax=spectral radiance of DN value of 255 DN=Digital Number Equation 3.8 the above, was used to convert digital number into spectral radiance
3.4.4. Conversion at sensor spectral radiance In radiometric calibration, pixel values, which were represented by Q in remote sensing raw data
AAU.Remote Sensing and Geo-informatics stream: By Belete Tafesse: [email protected] 35
and unprocessed image data, were changed into absolute radiance values.
The equation 3.9 was used to perform the conversion at sensor spectral radiance or satellite data scaled into 8 bits.
λ λ Lλ= ) (Qcal-Qcalmin) +LMINλ Eq. (3.9)
L λ=Spectral radiance at sensors aperture or the calculated radiance associated to the ground area enclosed in the pixel and referred to the λ wavelength range of specific band.
Lmaxλ=spectral at Sensor Radiance that is scaled to Qcalmax
Lminλ=Spectral at Sensor Radiance that is scaled to Qcalmin
Qcalmax=Maximum Quantized Calibrated Pixel values corresponding to Lmaxλ
Qcalmin=Minimum Quantized Calibrated Pixel values corresponding to Lminλ
Qcal=Quantized Calibration Pixel value (DN)
3.4.5. Conversion to top of atmosphere (TOA) reflectance Landsat Thematic Mapper top of atmosphere reflectance data must be corrected and processed .because of variation in solar zenith angle due to time difference between data acquisition. The gained output also calibrated to reflectance value. Equation 3.10 was used to compute TOA.
λ Pλ= Eq. (3.10) λ
Pλ=planetary top of atmosphere (TOA) reflectance, which has no unit or it is unit less
=mathematical constant approximately equal to 3.14159. It is also unit less
Lλ=spectral radiance at the sensors aperture
d=earth -sun distance/distance from the earth to the sun astronomical units
AAU.Remote Sensing and Geo-informatics stream: By Belete Tafesse: [email protected] 36
ESUNλ=mean exoatmospheric solar irradiation (from meta data)
Solar zenith angle
3.4.6. Conversion of radiance into brightness temperature Thermal infrared data can be converted from atmosphere reflectance (Lλ) to effective sensor brightness temperature (TB) using thermal constants provided in the Meta data file.
Remote sensing data (Landsat imagery) thermal band that is band 6 on thematic mapper and enhanced thematic mapper plus needs to be converted from at sensor spectral radiance to effective at sensor brightness temperature. Brightness temperature is the radiance travelling upward from the top of earth atmosphere. To covert Lλ (spectral radiance) to TB (brightness temperature) equation 3.11 was used Rajeshwari andMani, 2014).
TB= Eq. (3.11)
TB=effective at satellite brightness temperature (unit in Kelvin)
K2=calibration constant 2
Ln=natural logarithm
K1=calibration constant 1
λ=spectral radiance at sensors aperture
Landsat 8 has two thermal bands (band 10 and band 11). Table.3.8 shows the calibration constant used during performing the formula for brightness temperature.
AAU.Remote Sensing and Geo-informatics stream: By Belete Tafesse: [email protected] 37
Table 3.8: Thermal band calibration constant of Landsat 8.
Satellite sensors Categories Band 10 Band 11
K1 777.8853 480.8883
Landsat 8 OLI/TIRS K2 1321.0789 1202.1442
Radiance_ MULT_BAND 0.0003342 0.0003342
Radiance_ADD_BAND 0.01 0.01
LST was derived from Landsat TIRS using band 10 and band 11 based on split window algorithm, which was proposed for the first time by Mc Millin in1975. As follows (eq.3.12). LST=TB10+C1 (TB10-TB11) +C2 (TB10-TB11)2 +C0+ (C3+C4W) (1-m) + (C5+C6W) m
LST=Land Surface Temperature
C0 up to C6=Split Window Coefficient Value
TB10=Brightness Temperature of band 10
TB11=Brightness Temperature of band11
m=Mean Land Surface Emissivity of thermal infrared bands (mean of band 10 and band11)
W=Atmospheric water vapor content (0.005) from Earth science reference table (ESRT) Relative humidity table.
m=Difference in Land Surface Emissivity (LSE)
During performing/calculating LST of given area, using Landsat 8 thermal bands (Table 3.9) was used.
AAU.Remote Sensing and Geo-informatics stream: By Belete Tafesse: [email protected] 38
Table 3.9: Split window algorithm constant value.
Constant Value
The calibration constant for Landsat 4 and Landsat 7 different from Landsat 8. For the case of Landsat 4 and Landsat 7 the following table 3.10 was used.
Table 3.10: Thematic mapper (TM) and Enhanced Thematic Mapper Plus (ETM+) thermal band calibration constant.
satellite sensors constant value
TM K1 607.76 Landsat 4 K2 1260.56
K1 666.09 Landsat 7 ETM+ K2 1282.71
Source: Landsat7 handbook
AAU.Remote Sensing and Geo-informatics stream: By Belete Tafesse: [email protected] 39
Temperature was converted into degree Celsius, by subtracting 272.15 from the result, which was in degree Kelvin. Therefore, to convert degree Kelvin into degree Celsius the following formula was used (eq.3.13).
C=K-272.15 Eq. (3.13)
C: result in degree Celsius
K: result in degree Kelvin
Generally, to calculate LST for the sensor TM and ETM+ subtracting 272.15 from the existed result that is performed from effective at satellite temperature formula in Kelvin.
Zonal statistics
A zone is defined as all areas in the input that have the same value. Zonal statistics function summarizes the value of a raster within the zones of another dataset (either raster or vector) and reports the results as a Table. Maps of LU/LC, NDVI and LST were prepared for the year 1989, 1999 and 2016. To examine the spatial difference of LST according to varies LU/LC; the result was summarized using the zonal statistics tool of the ArcGIS 10. 3. subsequently, summarized LU/LC, NDVI and LST map data were analyzed using excel. Zonal statistics as table is one of important methods that used to examine the correlation between LULC and LST.
Spatial interpolation
Spatial interpolation is the procedure of using points with known values to evaluate values at other unknown points. For instance, to map rainfall and temperature of given area based up on nearby weather station.
In present study, meteorological data was used to interpolate and see the result and compare with the LST values that were done from Landsat thermal infrared bands. To interpolate rainfall and temperature data Inverse Distance Weighted (IDW) interpolation method was used. Inverse distance weighted is one of the interpolation methods in which the sample points are weighted during interpolation such that the impact of one point to another with distance declines from the unknown
AAU.Remote Sensing and Geo-informatics stream: By Belete Tafesse: [email protected] 40
point. Inverse Distance Weighted is the simplest interpolation method and deterministic models. Deterministic models include IDW, rectangular, natural neighbours and spine. Interpolation uses vector points with known values to estimate unknown locations to create raster surface covering an entire area (Legates and Wilmont, 1990).
The advantages of IDW interpolations are:
Different distances are integrated in the estimation The distance weighting is able to precisely regulate the impact of the distances It allows very fast and simple calculation
Therefore, in the present study rainfall and temperature data was interpolated using sample points in ArcGIS 10.3 environment and finally the interpolated maps were used for validation.
AAU.Remote Sensing and Geo-informatics stream: By Belete Tafesse: [email protected] 41
CHAPTER FOUR
4.1. Land-use/land-cover in 1989, 1999 and 2016
Land-use/land-cover classification result of 1989 showed that the dominant LU/LC classes were farmland and shrub land. These classes accounted for 82.7% of the overall area coverage. From the total area of 901.50 km2, farmland accounted for 556.65 km2 (61.7%) and shrub land accounted for 189.72 km2 (21%). The other LU/LC the bare land, plantation, settlement and water body together accounted for 155.13 km2 (17.3%) of the total area. The water bodies covered the smallest area than all than other classes. Analysis of 1999 image also showed that farmland constitutes the largest proportion of land in the study area with the value of 631 km2 (70%), followed by shrub land, which accounted 108.94 km2 (12.5%). Other LU/LC classes such as bare land, plantation, settlement and water body together accounted for 17.5% of the total area. In 1999, also water body covered the smallest area than all other classes. Land-use/land-cover classification results of 2016 image revealed that the dominant LU/LC categories were farmland and shrub land together accounted for 81.55% of the total area coverage. Farmland accounted for 623.4 km2 (69.15%) and shrub land accounted for 110.99 km2 (12.40%). Other LU/LC classes were bare land, plantation, settlement and water body, which together accounted 18.45% of the total area. However, the extent of farmland 2016 decreased by 0.85 from 1999. Generally, farmland is the major LU/LC of the area in relation to area coverage, followed by shrub land. Detailed statistical data for each of these classes and LU/LC map of the study period are shown in Table 4.1and Figure 4.1.
Table 4.1: Land-use/land-cover classes and area coverage of 1989, 1999 and 2016 in Adama Zuria Woreda, Ethiopia No LU/LC classes 1989 1999 2016 Area , Area, Area , Area, Area, Area, (%) (km2) (%) (km2) (%) (km2) 1 Farm land 556.65 61.7 631 70 623.4 69.15 2 Bare land 80.02 8.89 67.98 7.22 35.86 3.98 3 Plantation 66.21 7.41 68.97 7.57 72.90 8.00 4 Settlement 6.48 0.73 20.97 2.28 55.30 6.11 5 Shrub land 189.72 21 108.94 12.50 110.99 12.43 6 Water body 2.42 0.27 3.64 0.40 3.05 0.33
AAU.Remote Sensing and Geo-informatics stream: By Belete Tafesse: [email protected] 42
Figure 4.1: Land-use/land-cover maps of the study area of the years of 1989, 1999 and 2016.
AAU.Remote Sensing and Geo-informatics stream: By Belete Tafesse: [email protected] 43
Farmland habitat had the largest extent of all LU/LC in the study area during 1989–2016. The extent of farmland increased 1989 from 555.65 km2 to 631 km2 by 1999 but slightly decreased 623.4 km2
by 2016 (Fig 4.2).
1989 1999 2016
Area ( Area
3.05 2.42 0 Farm Land Bare Land Plantation Settlement Shrub Land Water Body
LU/LC catagories
Figure 4.2: Land-use/land-cover distribution and changes in the study area during the period 1989– 2016.
4.2. Spatial extent of land-use/land-cover
Land-use/land-cover patterns in the study area have indicated a significant change in between 1989 and 2016 years. Among the six major LU/LC classes, farmland and shrub land covered more than 80% of the area in all the study years. Settlement area has been increasing from 1989 to 2016. It has increased from 0.73% in 1989 to 2.28% by 1999 and 6.11% by 2016. Plantation also increased from 1989 to 2016. It increased from 7.41% by 1989 to 7.51% by 1999 and 8 % by 2016. Shrub land and water body showed fluctuations from 1989 to 2016. Shrub land was 21% in 1989, which was decreased to 12.0% by 1999 and then increased to 12.4% by 2016. Water body also has changed from 0.27% in 1989 to 0.40% by 1999 and then decreased to 0.33% by 2016. Farmland increased from 61.7% by 1989 to 70% by 1999 and decreased to 69.15% in 2016. Bare land showed continuous decrement from 1989–2016. It was 8.89% in 1989, which decreased to 7.20% by 1999 and 3.98% by 2016 (Table 4.2).
AAU.Remote Sensing and Geo-informatics stream: By Belete Tafesse: [email protected] 44
Table 4.2: Land-use/land-cover distribution and net changes during 1989–2016.
1999 2016 LU/LC 1989 Net changes during N Classes 2 Area Area, Area, Area , Area , Area , 1989–2016, km o 2 2 ,km2 % km % km %
1 Farmland 556.65 61.7 631 70 623.6 69.15 +66.75 2 Bare land 80.02 8.89 67.98 7.22 35.86 3.98 -44.16 3 Plantation 66.21 7.41 68.97 7.57 72.90 8.00 +6.69 4 Settlement 6.48 0.73 20.97 2.28 55.30 6.11 +48.82 5 Shrub land 189.72 21 108.94 12.50 110.99 12.4 -78.73 6 Water body 2.42 0.27 3.64 0.40 3.05 0.33 +0.63 Total 901.5 100 901.5 100 901.5 100
The LU/LC change trends of the study area showed that shrub land and bare land have decreased by 78.73 km2 and 44.16 km2, respectively. Other classes showed increasing and fluctuating area coverage. Figure 4.3.illustrated the LU/LC changes in the study area.
700 1989 600 1999 500
2 400 Net change (1989–2016)
Area Area 200
0 Farm Land Bare Land Plantation Settlement Shrub Land Water Body -100 LU/LC catagories
Figure 4.3: Land-use/land-cover changes during 1989––2016 in the Adama Zuria Woreda.
AAU.Remote Sensing and Geo-informatics stream: By Belete Tafesse: [email protected] 45
Table 4.3: Land transformation for Adama Zuria Woreda (1989––2016). 2016 LU/LC Farm Bare Shrub Class Total Plantation Settlement Water Classes land land land body
Farm land 423.6147 23.9958 14.6853 47.6505 39.3093 0.9207 550.1763
Bare land 61.7499 8.9118 1.9269 6.0228 2.673 0.0963 81.3807
Plantation 0.693 0.9522 43.1469 1.8711 19.5426 0.5148 66.7206
1989 Settlement 0.5499 0.2088 0.5031 4.2822 0.5967 0.1584 6.2991
Shrub land 98.6634 6.957 12.0681 14.4972 56.988 0.4311 190.6048
Water 0.3204 0.1521 0.2007 0.5472 0.3447 0.7245 2.2896 body Class Total 585.8451 41.202 72.5598 78.885 119.5173 2.8737 901.429
4.3. Settlement expansion during 1989–2016 An overlay analysis of settlement area detected from 1989, 1999 and 2016 satellite image of Adama Zuria Woreda revealed that the farmland, shrub land and bare land have been changed to settlement area in different direction of the Woreda. The spread of settlement area towards other LU/LC classes is shown in Figure 4.4.
AAU.Remote Sensing and Geo-informatics stream: By Belete Tafesse: [email protected] 46
Figure 4.4: The trend of expansion of settlement area from the year 1989 to 2016 in Adama Zuria Woreda.
4.4. Accuracy assessment
The accuracy assessment of LU/LC for the years 1989, 1999 and 2016 recorded the overall classification accuracy of 88.33%, 90.00% and 88.33%, respectively. The classification Kappa statistics for 1989 is 0.85. For the years, 1999 and 2016 Kappa values are 0.87 and 0.84, respectively. The detailed information of producers and users accuracy is indicated in Table 4.4.
AAU.Remote Sensing and Geo-informatics stream: By Belete Tafesse: [email protected] 47
Table 4.4: Statistical information of accuracy assessment for the year 1989, 1999 and 2016.
1989 1999 2016 class name producers users producers users producers users accuracy accuracy accuracy accuracy accuracy accuracy Plantation 87.50% 87.50% 77.78% 87.50% 100.00% 80.00% Settlement 81.82% 90.00% 100.00% 90.00% 93.75% 88.24% Water body 90.91% 90.91% 100.00% 90.91% 75.00% 100.00% Farm land 92.31% 85.71% 92.86% 92.86% 89.47% 94.44% Shrub land 90.00% 90.00% 90.00% 90.00% 66.67% 80.00% Bare land 85.71% 85.71% 75.00% 85.71% 90.91% 83.33% Overall
classification 88.33% 90.00% 88.33% accuracy Overall
Kappa 0.8588 0.8790 0.8496 statistics
4.5. Normalized difference vegetation index
In this study, it has been observed that the vegetation cover was more in 1989 and 1999 with maximum NDVI values of 0.66 and 0.55, respectively. The highest value shows healthy vegetation. Vegetation cover has decreased for the year 2016 with the NDVI value of 0.48. Normalized difference vegetation index result for the years 1989, 1999 and 2016 are presented in Figure 4.5.
The normalized difference vegetation index result of 1989, 1999 and 2016 showed that the northern and northwest part of the study area resulted higher NDVI value and the area of Boku ridge and Wonji sugarcane plantation showed highest NDVI value. However, settlement area along Awash River and most parts in eastern region have relatively low NDVI values. As indicated in figure 4.5, vegetation cover has decreased and non-vegetated area has been increasing gradually over the study period. However, in 2016 sugarcane plantation, area has slightly increased due to the expansion of Wenji Shewa Sugarcane Plantation. Table 4.5 showed statistical information of NDVI value for the year 1989, 1999 and 2016.
Table 4.5: Statistical information of NDVI value for the years 1989, 1999 and 2016.
Year Min Max Mean Std 1989 -0.40 0.66 0.159 0.123 1999 -0.61 0.55 -0.099 0.138 2016 -0.14 0.48 0.140 0.069 Where, Min = Minimum, Max = Maximum, Std = Standard deviation
AAU.Remote Sensing and Geo-informatics stream: By Belete Tafesse: [email protected] 48
Figure 4.5: Normalized difference vegetation index maps of the study area (1989, 1999 and 2016).
AAU.Remote Sensing and Geo-informatics stream: By Belete Tafesse: [email protected] 49
4.6. Relationship between land-use/land-cover and Normalized difference vegetation index
Normalized difference vegetation index maps extracted from near infrared and red-bands of the study periods indicated that different LU/LC has different NDVI values. As NDVI is related with the vegetation condition, the value varies from area to area based on vegetation intensity of the sites. Plantation and shrub land have the highest NDVI value than other classes (Fig. 4.6).
Farm Land Shrub Land Settlement Plantation Bare Land Water Body NDVI -0.2
-0.8 LU/LC catagories
Figure 4.6: Zonal statistical description of NDVI in 1989, 1999 and 2016 over different LU/LC classes in the study area.
4.7. Spatial pattern of land surface temperature in Adama Zuria Woreda
The spatial distribution of LST of the study area was extracted and quantified using Landsat TM of 1989, ETM+ of 1999 and OLI/TIRS thermal bands. The analysis from such images indicated that the LST value of the study period ranged from 9°C to 42°C. As it was observed from the processed thermal images, the east, northeast and southeast parts of the study area exhibited relatively high temperature. This is mainly due to altitude, slope and LU/LC types. On the other hand, Northwest part and Wenji Shewa sugarcane plantation area exhibited relatively low LST values. The Northwestern part of the study area had high altitude ranges from 2,118 to 2,505 meters a.s.l, and the southwestern part had altitude ranges from 1758 to 2,118 meters a.s.l. For each period, LST distribution is shown in Figure.4.7.
AAU.Remote Sensing and Geo-informatics stream: By Belete Tafesse: [email protected] 50
Figure 4.7: Land surface temperature maps of the study area of the years 1989, 1999 and 2016.
AAU.Remote Sensing and Geo-informatics stream: By Belete Tafesse: [email protected] 51
4.8. Impact of land-use/land-cover change on land surface temperature
Land-use/land-cover dynamics has an impact on land surface temperature. The changes LU/LC resulted in alteration of land surface temperature, particularly in the settlement, farmland (after harvested) and bare land. The reason behind farmland LU/LC classes of high LST was that the image used for the analysis was taken during harvesting time and dry weather condition. For each of LU/LC classes LST value varies from place to place because of vegetation and climate condition. The conversions of farmland and other types of LU into settlement area also contributed to the increase of LST. As observed in Figure 4.4 (Expansion of settlement area from the year 1989 to 2016), the settlement area has highly increased especially the northern and southern parts of Adama town and along the main gate of town (Addis Ababa, Wenji Shewa, Asella and Metehara). In addition to that, settlement area has increased in small towns of the Woreda (Table 4.6).
Table 4.6: Zonal statistical description of LST in 1989, 1999 and 2016 over different LU/LC classes.
LU/LC classes Min Max Mean Std Min Max Mean Std Min Max Mean Std
10.4 14.9 38.3 21.2 Farm land 32.82 25.49 2.32 31.11 2.36 41.86 35.55 2.12 4 6 7 4
13.6 35.0 19.8 Shrub land 9.02 32.12 22.55 3.30 26.47 2.95 39.75 31.79 3.27 2 0 3
12.7 17.6 38.7 20.8 Settlement 31.76 23.05 2.43 28.46 2.46 41.17 31.01 2.48 8 0 3 6
14.0 32.7 19.7 Plantation 9.78 28.55 18.55 2.65 22.73 1.34 38.00 31.48 3.03 4 1 1
16.4 18.9 38.3 20.9 Bare land 32.47 26.15 1.80 31.34 1.74 41.18 33.68 2.50 3 0 7 3
Water body 16.7 28.8 19.9 9.96 23.39 15.72 1.98 21.23 2.15 39.91 29.20 3.54 3 1 0 Where, Min = Minimum, Max = Maximum, Std = Standard deviation.
AAU.Remote Sensing and Geo-informatics stream: By Belete Tafesse: [email protected] 52
Most of the study area was under farmland cover, it covered more than 61% in all study period. The LST value has increased from the mean of 25.49ºC in 1989 to 35.55ºC in 2016. Figure 4.8 showed how LU/LCs changes affect the variability of LST.
Figure 4.8: Different views of land surface temperature of the study area for 2016.
AAU.Remote Sensing and Geo-informatics stream: By Belete Tafesse: [email protected] 53
4.9. Relationship between land-use/land-cover and land surface temperature
Land-use/land-cover and LST map of the study area revealed how the categories and corresponding land surface temperature have changed during the study period. Land-use/land-cover categories that received minimum temperature was water body, plantation and shrub land, respectively. Water body and Vegetated area played crucial role in minimizing the LST. Vegetated area and trees in different part of the area provide shade, which help lower land surface temperatures and reduce air temperature through evapotranspiration, in which plant release water to the surrounding air. Relatively, the highest LST value was recorded in farmland, settlement and bare land. The LST value was high in farmland during the acquisition time of the image.
From 1989 to 2016, the mean LST of Adama Zuria Woreda has increased by 9.66 °C. Table 4.5 indicates that all major LU/LC categories have recorded increase in the LST over the study period. The highest mean LST was recorded in farmland, bare land and settlement area. Whereas, the lowest mean LST was recorded in water body, plantation and shrub land area. Comparisons of mean LST with LU/LC classes for the study period are indicated in Figure 4.9. The land surface temperature in the study area has increased considerably from 1989 to 2016 (Table 4.7).
30 25 20 1989 15 1999 10 2016
Mean LST (ºC) LST Mean 5 0 Farm Land Shrub Settlement Plantation Bare Land Water Land Body LU/LC categories
Figure 4.9: Comparisons of mean land surface temperature in different land-use/land-cover classes during the study period in Adama Zuria Woreda.
AAU.Remote Sensing and Geo-informatics stream: By Belete Tafesse: [email protected] 54
Table 4.7: Mean temperature of 1989, 1999, 2016 and changes in temperature during 1989−2016 in the study area.
1989–1999 1999–2016 LU/LC 1989 1999 2016 1989–2016 Change in Change in Classes Mean Mean Mean Change in (ºC) (ºC) (ºC) Farm land 25.49 31.11 35.15 5.62 4.04 9.66
Shrub land 22.55 26.47 31.52 3.92 5.05 8.97
Settlement 23.05 28.46 31.67 5.41 3.21 8.62
Plantation 18.55 22.73 31.48 4.18 8.75 12.93
Bare land 26.15 31.34 33.68 5.19 2.34 7.53
Water body 15.72 21.23 29.20 5.41 7.97 13.48
4.10. Relationship between normalized difference vegetation index and land surface temperature The analyzed Landsat images of 1989, 1999 and 2016 indicated that NDVI and LST have indirect relationships. A Low NDVI value has high LST and high NDVI values have low LST. However, the relationship between NDVI and LST were direct/positive relationship for the case of water body, because both NDVI and LST value was less for water body. The R² values of the study period between NDVI and LST correlations indicated 96.08%, 97.52% and 98.58%, respectively. These results show that, in (1989) 96.08% value of LST variability was due to the difference in NDVI value, the coefficient variation values are also the same for 1999 and 2016. Generally, negative correlation was found between NDVI values with LST. Land surface temperature and NDVI correlation for the years 1989, 1999 and 2016 are shown in Figure 4.10.
AAU.Remote Sensing and Geo-informatics stream: By Belete Tafesse: [email protected] 55
Figure 4.10: Land surface temperature and normalized difference vegetation index correlation for the years 1989, 1999 and 2016 for the study area.
AAU.Remote Sensing and Geo-informatics stream: By Belete Tafesse: [email protected] 56
4.11. Comparisons of LST distribution during 1989, 1999 and 2016 The results of the study indicated that throughout all the study period the LST value increased drastically. Land surface temperatures were classified into six groups ranging from 9 ºC –42ºC.The LST distribution statuses during study period are given in Table 4.8.
Table 4.8: Trends of land surface temperature distribution during the study period in Adama Zuria Woreda during 1989–2016.
Area coverage during study period (km2) LST 1989 1999 2016 36 0.347769 117.7 137.6997 Total 901.5 901.5 901.5
The trend of increase in LST shows that in Adama Zuria Woreda LST was increasing from year to year. A comparison between LST distributions of the selected years are given in Figure 4.11.
400 350 LST 1989
300 LST 1999
250 LST 2016 200
150 Area (km²) Area 100 50 0 36 LST (ºC)
Figure 4.11: Comparisons between LST distributions of the years 1989, 1999 and 2016 in Adama Zuria Woreda.
AAU.Remote Sensing and Geo-informatics stream: By Belete Tafesse: [email protected] 57
4.12. Verification of the result for land surface temperature The land surface temperature extracted from Landsat thermal band of the study area and the interpolated atmospheric temperature have shown the direct relationships. However, LST trend attained in this study was from Landsat imagery acquired during dry weather condition and harvesting time. Considering the existing metrological and acquired time of Landsat, the spatial distribution of the LST was validated. Land surface temperature was verified using meteorological data and LU/LC classes of the study area, which validated with GPS point collected from field and Google Earth. Interpolation of rainfall and temperature were done based on the meteorological data collected from five stations within and nearby the study area. These stations were; Mojo, Koka Dam, Nazareth, Sodere and Welenchiti . There is strong relationship between LST and atmospheric temperature, which was interpolated from five stations. Remotely sensed LST is most valuable in characterization and prediction of spatial temporal patterns of atmospheric temperature. Therefore, LST can be used as indicator of atmospheric temperature. Interpolated map of rainfall and atmospheric temperature are given in Figure 4.12a and 4. 12b.
Figure 4.12: a) Interpolated map of rainfall and b) Interpolated map of temperature of Adama Zuria Woreda.
AAU.Remote Sensing and Geo-informatics stream: By Belete Tafesse: [email protected] 58
As observed from the interpolated map of the study area, both rainfall and temperature results was low at the eastern part. However, rainfall map of the northern and western parts have high values than eastern and southern parts. Interpolated map of temperature result showed that the southern and the northern part have higher value in ºC than east and western relatively.
The result of LST extracted from Landsat thermal bands, also revealed that the eastern part had the highest value in each of the study periods, where as the northern part had low value. However, in the area of Wenji Shewa sugarcane plantation, LST was low. For instance, Wenji Sugarcane plantation area; the LST was very low but in the interpolated map it shows relatively medium temperature. The mean LST, which was extracted from thermal bands of the study period, was ranged from 25.49ºC to 35.15 ºC. Whereas, the interpolated atmospheric temperature result ranged from 23.35 ºC – 33.82 ºC.
AAU.Remote Sensing and Geo-informatics stream: By Belete Tafesse: [email protected] 59
CHAPTR FIVE
5. DISCUSSION 5.1. Land-use/land-cover status of Adama Zuria Woreda The LU/LC changes experienced across Adama Zuria Woreda shows as a sign of population growth and the increase of needs of land for settlement and agriculture. These changes have brought about a series of adverse impacts on the environment. Houghton (1994) revealed that human population growth, economic development, technology and environmental changes are major factor responsible for LU/LC variability. According to FAO (1999), LU/LC changes have become major problems and it is a significant driving agent of environmental changes. Alemayehu (2008) also showed some of the evidence for climate change including occurrence of drought, rising temperature, flood, reduced annual rainfall and rising sea levels. The present study revealed that during the study period there were drastic changes in LU/LC. In 1989, the settlement area was 6.48 km2 and then it reached 55.30 km2 in 2016. It is one of the evidences for the increasing needs of settlement. The extent of sugarcane plantation also increased from 66.21 km2 in 1989 km2 to 72.90 km2 in 2016 and bare land decreased from 80.02 km2 in 1989 to 35.86 km2 in 2016. Farmland, shrub land and water body showed fluctuation. In general, the classified satellite image indicates that there is a change in LU/LC.
5.2. Normalized difference vegetation index Normalized difference vegetation index is a measure of vegetation condition and used to determine LST. According to Mihai (2012) and Weng (2004), NDVI is found as an acceptable indicator of LST and dryness. The findings of the present study also revealed that NDVI varied from south towards to the north of the Woreda. As farmland and shrub land classes dominate the present study area, the vegetation cover also varied. The NDVI and LST correlation coefficient was evaluated in this study for 2016 was R2=0.98, which indicate how the vegetation condition determine the LST of the area and NDVI accounts 98.58% in distribution of LST. In line with this, the present study revealed that NDVI have strong relation with LST (with the exception of water body class).
5.3. Land surface temperature A thermal band of Landsat image was important in quantifying the LST of the area. In the present study farmland, settlement and bare land have high LST. The main reason behind less NDVI and high LST values in the area of farmland LU/LC class is that the image was taken during the dry
AAU.Remote Sensing and Geo-informatics stream: By Belete Tafesse: [email protected] 60
season and during harvesting time November and December. In Ethiopia, the main harvesting season is November, December and January. Due to this, farmland, bare land and settlement classes had high surface reflectance. After harvest of crops, the land was very dry and at the same time, land reflectance high. Normalized Difference Vegetation Index value is less for water body, bare land, settlement and farmland classes. However, in the case of plantation and shrub land, the values were relatively high. To evaluate the thermal status of land surface by satellite, it is important to find the relation between LU/LC type and LST. Normalized difference vegetation index play a great role in assessing and investigating of LST. Land surface temperature and NDVI have indirect relationships in different LU/LC categories except in the case of water body. Water body LU/LC class has low NDVI and LST value. Vegetation condition (NDVI value) and types of LU/LC have impacts on LST value. However, it does not mean that the type of LU/LC is the only factor for the increase of LST. There are a number of contributing factors for increasing or decreasing the LST value. For instance, types of soil, geothermal energy, geological setting, altitude and climate condition in the area.
Different researchers used thermal bands to study LST. Gebrekidan Worku (2016) used Landsat satellite imagery to study LST situation of Addis Ababa city. The study investigated the relationship between LU/LC, LST and NDVI. Gebrekidan Worku (2016) found negative linkage in NDVI and LST values. However, in this case, water body should be considered as exception, as water body has low NDVI and LST value. The result of present study shows that different LU/LC has different NDVI and LST values during the study period.
Maitima et al. (2009), Khin et al. (2012) and Kerr et al. (2004) agree with that the main factor for increase of LST in rural as well as urban areas land-cover changes and unplanned use of land resources. In less developed areas the relation between Land-cover change, biodiversity and land deterioration is very high, because of that land-cover are transformed to human settlements, farmlands and grazing lands at the expense of forest and vegetation (Maitima et al. 2009). With regard to LU/LC changes in the study area, with the expansion of settlements, plantations (sugarcane plantation) and farmland the shrub land and open space have highly declined. This is clearly observed, especially at Adama town area. In this area, due to the expansion of settlement area, small-scale farmers moved and start to settled in open space and shrub land. In addition to this, Wonji Shewa sugarcane plantation also extended its area towards the previously open space, shrub
AAU.Remote Sensing and Geo-informatics stream: By Belete Tafesse: [email protected] 61
land and farmland. In 1989, at the Boku ridge area, there were some sparsely vegetated area but through time, this area was totally changed into farmland and settlement. Thus, the study area is highly degraded and exposed to natural disasters such as soil erosion. As evident from the classified satellite images. However, sometimes LU/LC has a positive impact on environment. For instance, the conversion of open space/bare land/ to plantation LU/LC type. This might help to control increasing LST.
Normalized difference vegetation index and LST have indirect/negative/ relationship. However, that could be a positive/direct/ relationship, especially in water body and high potential geothermal areas. According to Burgan and Hartford (1993), the negative values are indicative of water, snow, clouds, non-reflective surface and other non-vegetated areas while positive values expresses reflective surfaces such as vegetated area. However, it does not mean that all negative values of NDVI have high LST value. Land surface temperature depends up on the reflective conditions of the surface of the earth. In general, area of non-vegetated and non-reflective surface, have high LST than those of vegetated and water body.
According to the result of this study, there are six major LU/LC classes and for each class LST value varies. Water body, plantation and shrub land have relatively low LST than settlement, farmland and bare land LU/LC class. Spatial and temporal distribution of LST during 1989,1999 and 2016 revealed that during the study period there was drastic changes took place in the area. In 1989, the distribution of LST was 9ºC and 33ºC, minimum and maximum, respectively, where as in 1999 and 2016, the minimum and maximum LST ranges were 13ºC–39ºC and 19ºC–42ºC, respectively.
AAU.Remote Sensing and Geo-informatics stream: By Belete Tafesse: [email protected] 62
6. CONCLUSION AND RECOMMENDATIONS
6.1. Conclusion Satellite data are useful for different applications such as LU/LC change detection and environmental management programs. Landsat imageries have the potential to examine the LU/LC dynamics and to analyze the spatio-temporal distribution of LST. The present study has used Landsat TM 4, ETM+7 and Landsat OLI/TIRS images to evaluate and analyze the impact of LU/LC changes on LST. Land surface temperature and LU/LC derived from such data provides important information to monitor human activities and environmental changes. It was reveals that Landsat images are very useful in quantifying and mapping LU/LC change, NDVI and LST in Adama Zuria Woreda. The LU/LC pattern of the study area has attributed to socio-economic and natural factors and their exploitation in time and space. Water body and vegetation classes (Plantation and shrub land) have lowest LST value where as settlement, bare land and farmland class have highest LST. Land-use/land-cover has been changing from time to time in the present study area. Land-use/land- cover change has an impact on LST. For each year, the minimum and maximum LST has increased. Hence, Calculated LST acts an important role or functions of LU/LC and alters temperature condition of the area.
The findings of this study showed that LSTs of Adama Zuria Woreda has increased during period of 1989, 1999 and 2016. This was because of LU/LC changes, human activities and climate variability of the area. The land surface temperature of the Woreda indicated a high variation in LST between different LU/LC types. The derived LST values from satellite data were found to be in fine harmony with interpolated temperature values from the weather stations used. The derived NDVI values from satellite data also somehow a good indicator of LST status of the study area. Therefore, the use of GIS remote sensing data to investigate the differences in LST and different LU/LC class pattern in the study area illustrates that it suggest a quicker and cost effective technique with the advantage of covering large area.
6.2. Recommendations
The focus of this study was to assess and investigate the impact of LU/LC changes on LST in Adama Zuria Woreda based on Landsat imagery of 1989, 1999 and 2016. The study showed that the
AAU.Remote Sensing and Geo-informatics stream: By Belete Tafesse: [email protected] 63
conversion of LU/LC types to another type of LU/LC types such as from vegetation area to farmland, farmland to settlement and the value of LST was different for each LU/LC class. However, there is case in which the change could make a positive impact on LST. For instance, Wenji sugarcane plantation had a positive contribution for the low LST value. Therefore, based on the result of this study, the following recommendations have drawn.
Land-use/land-cover change becomes a central component in current strategies for managing resources and monitoring environmental changes. Therefore, the governmental and non-governmental bodies should give high attention for proper land-use management and ecological effect of each LU/LC. Conservation activities have to be taken on both rural and urban green areas of the study area and recommended to plant trees and delineate green area, especially at high settlement and bare land areas. Land managers and environmental experts should control LU/LC changes through different measures to minimize the influence on the environments. The output of this research can serve as an input for future researchers who want to study the impact of LU/LC changes on environmental issues.
AAU.Remote Sensing and Geo-informatics stream: By Belete Tafesse: [email protected] 64
References Agarwal, C., Green, G. M., Grove, J. M., Evans, T. P., and Schweik, C. M. (2002). A review and assessment of land-use change models: dynamics of space, time, and human choice.
Aires, F., Prigent, C., Rossow, W. B., and Rothstein, M. (2001). A new neural network approach including first guess for retrieval of atmospheric water vapor, cloud liquid water path, surface temperature, and emissivities over land from satellite microwave observations. Journal of Geophysical Research: Atmospheres, 106(D14), 14887–14907.
Alemayehu Mengistu (2008). Climate Variability and change. Ethiopian journal of Animal production.8 (1), 94–98.
Ali, H. (2009). Land use and land cover change, drivers and its impact: A comparative study from Kuhar Michael and Lenche Dima of Blue Nile and Awash Basins of Ethiopia (Doctoral dissertation, Cornell University).
Asubonteng, K. O. (2007). Identification of land use/cover transfer hotspots in the Ejisu-Juabeng Woreda, Ghana. International Institute for Geo-information Science and Earth Observation Enschede, Netherlands.
Becker, F., and Li, Z. L. (1990). Towards a local split window method over land surfaces. Remote Sensing, 11(3), 369–393.
Billah, M., and Rahman, G. A. (2004). Land-cover mapping of Khulna city applying remote sensing technique. In Proceedings of the 12th International Conference on Geoinformatics (Sweden: Geospatial Information Research: Bridging the Pacific and Atlantic, University of Gävle) pp. 707– 714.
Bonini, M., Corti, G., Innocenti, F., Manetti, P., Mazzarini, F., Abebe, T., and Pecskay, Z. (2005). Evolution of the Main Ethiopian Rift in the frame of Afar and Kenya rifts propagation. Tectonics 24, TC1007.
Burgan, R. E., and Hartford, R. A. (1993). Monitoring vegetation greenness with satellite data.
Burrough, P.A., (1990). Principles of Geographic information systems.Pp.333.Oxford University press, UK.
Buyadi, S. N. A., Mohd, W. M. N. W., and Misni, A. (2013). Impact of land use changes on the surface temperature distribution of area surrounding the National Botanic Garden, Shah Alam. Procedia-Social and Behavioral Sciences, 101, 516–525.
CSA. (2007) Central Statistical Agency. Summary and statistical report of the population and housing census. Addis Ababa, Ethiopia.
Chen, X., (2000). Using RS and GIS to analyze land-cover change and its impacts on the Regional Sustainable Development. International Journal Remote Sensing. (23), 107–113.
AAU.Remote Sensing and Geo-informatics stream: By Belete Tafesse: [email protected] 65
Coppin, P. R., and Bauer, M. E. (1996). Digital change detection in forest ecosystems with remote sensing imagery. Remote sensing reviews, 13(3-4), 207–234.
Di Gregorio, A., and Jansen L.J., (2000). Land-cover classification: classification concepts and user manual, Food and Agriculture Organization (FAO) of the United Nations Rome.
Emilio, C. (2008). The role of satellite RS in monitoring global environment “department of Geography University of Alkala Spain, PP. 1–2.
FAO/UNEP., (1999).Terminology for integrated resources planning and management. Food and agriculture organization/United Nations Environmental program, Rome, Italy and Nairobi, Kenya.
Farooq, A. (2012). Landsat ETM+ and MODIS EVI/NDVI data products for climate variation and Agricultural Measurements in Holliston Desert. Global Journal of Humanities, social science: Geography and Environmental Geosciences, 12 :( 13).111.
Foresman, T. W. (Ed.). (1998). The history of geographic information systems: perspectives from the pioneers. Prentice Hall.
Gebrekidan, Werku (2016). Modeling land surface temperature from satellite data, the case of Addis Ababa. ES RI, Eastern Africa Education GIS conference. Held at Africa hall, United Nations conference center from23-24 sept. 2016, Addis Ababa.
Gumindoga, W. (2010). Hydrologic Impact of Land-Use Change in The Upper Gilgel Abay River Basin, Ethiopia; TOPMODEL Application (Doctoral dissertation, M. Sc. Thesis, ITC Netherlands).
Guo, Z., Wang, S. D., Cheng, M. M., and Shu, Y. (2012). Assess the effect of different degrees of urbanization on land surface temperature using remote sensing images. Procedia Environmental Sciences, 13, 935–942.
Hardegree, L. C. (2006). Spatial Characteristics Of The Remotely-sensed Surface Urban Heat Island in Baton Rouge, LA: 1988-2003 (Doctoral dissertation).
Houghton, R. A. (1994). The worldwide extent of land-use change. BioScience, 44(5), 305-313.
Huang, S., and Siegert, F. (2006). Land cover classification optimized to detect areas at risk of desertification in North China based on SPOT VEGETATION imagery. Journal of Arid Environments, 67(2), 308–327.
IPCC. (2014). Climate Change 2014–Impacts, Adaptation and Vulnerability: Regional Aspects. Cambridge University Press.
Jiang, J., and Guangjin, T. (2010). Analysis of the impact of land use/land cover change on land surface temperature with remote sensing. Procedia environmental sciences, 2, 571–575.
AAU.Remote Sensing and Geo-informatics stream: By Belete Tafesse: [email protected] 66
Kayet, N., Pathak, K., Chakrabarty, A., and Sahoo, S. (2016). Spatial impact of land use/land cover change on surface temperature distribution in Saranda Forest, Jharkhand. Modeling Earth Systems and Environment, 2(3), 1–10.
Kazmin, V., and Seifemichael, B. (1978). Ethiopian institution of geological survey; Geology and Development of the Nazret area, Northern Ethiopian Rift, Addis Ababa.
Kern, A. (2011). Deriving Normalized Difference Vegetation Index based on remotely sensed AVHRR and MODIS data (Doctoral dissertation, Eötvös Loránd University).
Kerr, Y.H., Lagouarde, J.P., Nerry, F., Ottle, C. (2004). Land surface retrieval techniques and applications: case of AVHRR, in: Quatrocci, D.A., Luvall, J. C. Thermal remote sensing in land surface processes. CRC press, Florida, USA, 33–109.
Khin, M.Y., Shin D.Y., Park, J.J., Choi C.C., (2012). Land surface temperature changes by LU changes of Inlay Lake area, Myanmar. Department of Spatial Information Engineering, Pukyong National University, South Korea.
Kumar, R., and Singh, S. (2016). Case Study for Change Detection Analysis and Land Surface Temperature Retrieval in Uttrakhand Region and their correlation. Imperial Journal of Interdisciplinary Research, 2(9).
Lambin, E. F., Turner, B. L., Geist, H. J., Agbola, S. B., Angelsen, A., Bruce, J. W., ... and George, P. (2001). The causes of land-use and land-cover change: moving beyond the myths. Global environmental change, 11(4), 261–269.
Lambin, E.F., and Meyfroidt, P. (2010). Land-use transitions: socio-ecological feedback versus socioeconomic change, land-use policy, 27,108–118.
Legates, D. R., and Willmott, C. J. (1990). Mean seasonal and spatial variability in global surface air temperature. Theoretical and applied climatology, 41(1), 11–21.
Lemlem Abraha (2007). Assessing the Impact of Land Use and Land Cover Change on Groundwater Recharge using Rs and GIS; a Case of Awassa Catchement, Southern Ethiopia (Doctoral dissertation, AAU), Addis Ababa.
Lillesand, T., Kiefer, R. W., and Chipman, J. (2014). Remote sensing and image interpretation. John Wiley and Sons, New Delhi.
Macleod, R. D., and Congalton, R. G. (1998). A quantitative comparison of change-detection algorithms for monitoring eelgrass from remotely sensed data. Photogrammetric engineering and remote sensing, 64(3), 207–216.
Maguire, D.J., (1991). An overview and definition of Geographic information system. In geography information systems: principles and applications. London Longman, Vol.1,9–20.
AAU.Remote Sensing and Geo-informatics stream: By Belete Tafesse: [email protected] 67
Maitima,J.M.,Mgatha,S.M.,Reid,R.S.,Gachimbi,L.N.,Majule,A.,Lyaru,H.,Pomery,D.,Mathai,S.,and Mugisha,S.,(2009). The linkage between land-use change, land degradation and biodiversity across East Africa. Africa Journal of environmental science and technology, 3 (10), 310–326.
Mather, A. S., and Needle, C. L. (2000). The relationships of population and forest trends. TheGeographical Journal, 166(1), 2–13.
Md Shahid, L., (2014).Land Surface Temperature Retrieval of Landsat 8 Data using split window Algorithm a case study Ranchi Woreda. International Journal of Engineering Development and Research, 2(4), 3840–3849.
Meyer, W. B., and Turner, B. L. (1992). Human population growth and global land-use/cover change. Annual review of ecology and systematics, 23(1), 39-61.
Mihai,V.H.(2012).Using Satellite Images Landsat TM For calculating Normalized Difference Indexes for the landscape of Parang mountains.Lect.Ph.D.Eng.Banat University of Agricultural sciences and Veterinary medicine, Romania.
Miheretu Birhan and Assefa Abegaz (2017). Land use/land cover changes and their environmental implications in the Gelana sub-watershed of Northern highlands of Ethiopia. Environmental Systems Research, 6(1), 7.
Naissan, O.W., and Lily,T.(2016). Aesthetc Dermatology,effects of climate change on skin,Dermatology news USA, May 16, 2016.
Nunes, M. C., Vasconcelos, M. J., Pereira, J. M., Dasgupta, N., Alldredge, R. J., and Rego, F. C. (2005). Land cover type and fire in Portugal: do fires burn land cover selectively?. Landscape Ecology, 20(6), 661–673.
Nuruzzaman, M. (2015). Urban Heat Island: Causes, Effects and Mitigation Measures-A Review. International Journal of Environmental Monitoring and Analysis, 3(2), 67.
Oluseyi, I. O., Danlami, M. S., and Olusegun, A. J. (2011). Managing Land Use Transformation and Land Surface Temperature Change in Anyigba Town, Kogi State, Nigeria. Journal of Geography and Geology, 3(1), 77.
Orsolya, G., Zalan T., Boudewijn V.L. (2016). Satellite based Analysis of surface UHI intensity. Journal of Environmental Geography, 9(2), 23–30.
Paramasivam, C.R. (2016). Remote Sensing and spectral studies of magnesite mining area, Salem, India. For the award of the Degree of Doctor of Philosophy in Geology thesis submitted to Periyar University, Salem, India. Prata, A.J., and Caselles, C., (1995). Thermal remote sensing of land surface temperature from satellites: status and future prospects. Remote sensing rev.12, 175–223.
AAU.Remote Sensing and Geo-informatics stream: By Belete Tafesse: [email protected] 68
Rajeshwari, A., and Mani, N. D. (2014). Estimation of land surface temperature of Dindigul Woreda using Landsat 8 data. International Journal of Research in Engineering and Technology, 3(5), 122– 126.
Ramachandra, T. V., Aithal, B. H., and Sanna, D. (2012). Land Surface Temperature Analysis in an Urbanising Landscape through Multi-Resolution Data. Research and Reviews: Journal of Space Science and Technology, 1(1), 1–10.
Santa, P. (2011). Forest cover and land-use changes: a study of Laljihadi forest (corridor), far western development region, Nepal.MSc Thesis Tribhuvan University.
Schmugge, T., Hook, S. J., and Coll, C. (1998). Recovering surface temperature and emissivity from thermal infrared multispectral data. Remote Sensing of Environment, 65(2), 121–131.
Shukla, J., Nobre, C., and Sellers, P. (1990). Amazon deforestation and climate change. Science (Washington), 247(4948), 1322–1325.
Sobrino, J.A., Rosa, O., Guillen, S., Juan, C.J., Belen.F. Victoria.H., Cristian, M., Yves,J.,Juan,C.,Mireia,R.,Antonio,G.J.,Eduardo,D.M.,Remo,B.,andMarc,p.(2012).Evaluation of the surface UHI effect in the city of Madrid by thermal RS. International journal of remote sensing, 34, 910.
Streutker, D. R. (2003). Satellite-measured growth of the urban heat island of Houston, Texas. Remote Sensing of Environment, 85(3), 282–289.
Turner, W., Spector, S., Gardiner, N., Fladeland, M., Sterling, E., and Steininger, M. (2003). Remote sensing for biodiversity science and conservation. Trends in ecology and evolution, 18(6), 306–314.
USAPA, (2004). A Summary of Models for assessing the effects of Community Growth and change on land-use patterns.EPA.600.R-00.098.U.S.Environmental protection Agency office of research and development , pp. 260.
Voogt, J., (2000). How researchers measure UHI. Glossary of Meteorology, 2nd edition, Canada.
Walsh, C. L., Dawson, R. J., Hall, J. W., Barr, S. L., Batty, M., Bristow, A. L., and Tight, M. R. (2011). Assessment of climate change mitigation and adaptation in cities. Proceedings of the Institution of Civil Engineers-Urban Design and Planning, 164(2), 75–84.
Weng, Q.H. (2004). A remote sensing and GIS evaluation of urban expansion and its impact on surface temperature in the Zhujiang Delta, China. International journal of remote sensing 22(10), 1999-2014. Weng, Q., Lu, D., and Schubring, J. (2004). Estimation of land surface temperature–vegetation abundance relationship for urban heat island studies. Remote sensing of Environment, 89(4), 467– 483.
AAU.Remote Sensing and Geo-informatics stream: By Belete Tafesse: [email protected] 69
Yue, W., Xu, J., Tan, W., and Xu, L. (2007). The relationship between land surface temperature and NDVI with remote sensing: application to Shanghai Landsat 7 ETM+ data. International Journal of Remote Sensing, 28(15), 3205–3226.
Zha, Y., Gao, J., Jiang, J., Lu, H., and Huang, J. (2012). Normalized difference haze index: a new spectral index for monitoring urban air pollution. International journal of remote sensing, 33(1), 309–321.
Zubair, A.O. (2006). Change detection in LU/LC using RS data and GIS a case study of Ilorin and its environments in Kwara State. Department of geography, University of Ibadan.
AAU.Remote Sensing and Geo-informatics stream: By Belete Tafesse: [email protected] 70
Appendix 1: Classification accuracy assessment report for the year 1989.
AAU.Remote Sensing and Geo-informatics stream: By Belete Tafesse: [email protected] 71
Appendix 2: Classification accuracy assessment report for the year 1999.
AAU.Remote Sensing and Geo-informatics stream: By Belete Tafesse: [email protected] 72
Appendix 3: Classification accuracy assessment report for the year 2016.
AAU.Remote Sensing and Geo-informatics stream: By Belete Tafesse: [email protected] 73
Appendix 4. Plate 1: Sample of different LU/LC photographs.
Plantation Settlement
Water body Bare land
Farmland Shrub land
AAU.Remote Sensing and Geo-informatics stream: By Belete Tafesse: [email protected] 74
Appendix 5. map 1: Location of meteorological stations map.
AAU.Remote Sensing and Geo-informatics stream: By Belete Tafesse: [email protected] 75
Appendix 6.map 2: GPS point data map.
AAU.Remote Sensing and Geo-informatics stream: By Belete Tafesse: [email protected] 76
Declaration of originality
I, undersigned, declare that this thesis entitled “IMPACT OF LAND-USE/LAND-COVER CHANGES ON LAND SURFACE TEMPERATURE IN ADAMA ZURIA WOREDA, ETHIOPIA, USING GEOSPATIAL TOOLS” is my original work and has not been presented for a degree in any other university and that all sources of materials used for this thesis have been interestingly acknowledged.
Belete Tafesse Habtewold
Signature ______
Date ______
Addis Ababa
This is certified that the thesis entitled “Impact of land-use/land-cover changes on land surface temperature in Adama Zuria Woreda, Ethiopia, using geospatial tools” is original work of Mr. Belete Tafesse Habtewold for the partial fulfillment of the Degree of Masters of science in remote sensing and Geo-informatics from Addis Ababa University under our guidance and supervision.
Dr.K.V.Suryabhagavan Prof. M. Balakrishnan
Signature ______Signature ______
Date ______Date ______
School of earth science

IMAGES
VIDEO
COMMENTS
Watershed and Soil Conservation Thesis and Dissertations 2022-07 Land Use/Land Cover Change Detection and Assessment on Community Livelihoods in Enqulal Watershed, North Western Ethiopia ... Changes in the condition and composition of the land cover affects climate changes in biogeochemical cycles and energy fluxes (Melillo et al., 2003) ...
Land is a vital natural resource for human survival and the base for all terrestrial ecosystem services [1, 2].However, land degradation in the form of land use/land cover (LULC) changes has been a severe problem in the world [2, 3].LULC changes have gained as the main cause of ecosystem service change at global scale and Africa is experiencing substantial changes across the continent [4,5,6,7].
492 Journal of the Indian Institute of Science VOL 90:4 Oct-Dec 2010 journal.library.iisc.ernet.in. Land use and land cover change in India: A remote sensing & GIS prespective REVIEW. Figure 2 ...
Land use land cover change in Orange County, North Carolina from 1955 to 2001; Last Modified. March 21, 2019; Creator. Zhang, Su. Affiliation: College of Arts and Sciences, Department of Geography; Abstract. Land use/land cover change (LULCC) has significant implications in terrestrial ecosystem goods and services.
To identify land cover changes detection; remote sensing data, satellite imagery and image processing techniques had done within three dates of 1986, 2002 and 2018 using Land sat TM 30 m resolution images. ENVI and Arc GIS soft wares had used to identify the changes. The classification had done using six land cover (water body, bushland ...
Understanding the determinants of Land-Use and Land-Cover change as a basis for conservation management in the Warren River Catchment Achieve International Excellence Thayse Nery de Figueiredo M.Sc. Ecology University of Basel, Switzerland This thesis is presented for the degree of Doctor of Philosophy of The University of Western Australia
1. Introduction. Land use and land cover (LULC) change is a primary driver of environmental challenges in many developing countries (Matlhodi et al., Citation 2019).The expansion of agriculture, human settlements, urban and industrial areas are among the main factors accelerating LULC changes (Mariye et al., Citation 2022; Seyam et al., Citation 2022).
d use map.Chapter 5 Conclusion of the study is done in this chapter.1.5.1 AIM OF THE STUDY:The aim of the stud. land cover and land use changes in Sukinda valley at in last three decades.1.5.2 OB. The following specific objectives will be pursued in order to achieve the aim above. To create a land use land cover classification scheme.
The challenge of urban growth and land use land cover (LULC) change is particularly critical in developing countries. The use of remote sensing and GIS has helped to generate LULC thematic maps, which have proven immensely valuable in resource and land-use management, facilitating sustainable development by balancing developmental interests and conservation measures. The research utilized ...
This paper describes a methodology for systematic land use/land cover classification on a regional scale, with emphasis on a low cost and highly automatized approach. This methodology is based on ...
This thesis study was conducted to capture the land use and land cover (LULC) change dynamics in Kiskatinaw River Watershed, BC, Canada. A combination of remote sensing, GIS and modeling approach was utilized for this purpose. Landsat TM and ETM+ satellite images of the years 1984, 1999 and 2010
Land use and land cover change research has been applied to landslides, erosion, land planning and global change. Based on the CA-Markov model, this study predicts the spatial patterns of land use in 2025 and 2036 based on the dynamic changes in land use patterns using remote sensing and geographic information system. CA-Markov integrates the advantages of cellular automata and Markov chain ...
Land use/land cover (LULC) changes play a significant role in ecosystem services, impacting ecosystem functioning, local toward global scales (Sahana et al., Citation 2016), and human variables like environment and government planning (Athick & Shankar, Citation 2019; Sinha et al., Citation 2012).
1.4.2 Objectives. The following specific objectives will be pursued in order to achieve the aim above. To create a land use land cover classification scheme. To determine the trend, nature, rate, location and magnitude of land use land cover change. To forecast the future pattern of land use land cover in the area.
Monitoring land cover variations is imperative for global resource management. Climatic variation influences land use-land cover (LULC) distribution steadily. ... Cover Classification by Using Satellite NDVI Tool for Sustainable Water and Climate Change in Southern Punjab By MS Thesis COMSATS University Islamabad (CUI).
This is to certify that the thesis entitled impact of land use land cover change on soil erosion risk in Limu-Seka watershed of Didessa sub- basin‚ south west Ethiopia using GIS and RS technique. Submitted to graduate studies of Jimma University for the award of Master of
LAND USE AND LAND COVER CHANGE, DRIVERS AND ITS IMPACT: A COMPARATIVE STUDY FROM KUHAR MICHAEL AND LENCHE DIMA OF BLUE NILE AND AWASH BASINS OF ETHIOPIA A Thesis Presented to the Faculty of the Graduate School of Cornell University In Partial Fulfillment of the Requirements for the Degree of
Abstract. Chapter four deals with the urban land use and urban land use changes in the study. It elaborates upon how the land has been used for various reasons and subsequent change in the pattern ...
As research thesis advisors, we hereby certify that we have read and evaluated this thesis entitled The Impact of Land Use-Land Cover Change on Rangeland Ecosystem Services in Shinile District, Siti Zone, Somali Regional State Prepared, ... Table 1: Summary of the land cover and land cover changes in Darito site. 12
Urban areas became the land use type exhibits the fastest growth, being doubled in a 21-year period. Forest conversion, farm loss, and rapid uncontrollable settlement development appear to be the challenges for land use planning. SWAT-based assessment results showed that the LULC
The land cover changes within the catchment were examined through classification of satellite images with integrated use of image and spatial analysis. The Soil and Water Assessment Tool (SWAT) model was used to investigate the impact of land cover change on stream flow. The model was calibrated and validated using historical flow data.
Geographic information system and remote sensing technologies have shown their great capabilities to solve the study issues like land use and land cover changes. The aim of this thesis is to produce maps of land use and land cover of Shijiazhuang on year 1993, 2000 and 2009 to monitor the possible changes that may occur particularly in ...
ADDIS ABABA UNIVERSITY COLLEGE OF NATURAL AND COMPUTATIONAL SCIENCES SCHOOL OF EARTH SCIENCES . IMPACT OF LAND-USE/LAND-COVER CHANGES ON LAND SURFACE TEMPERATURE IN ADAMA ZURIA WOREDA, ETHIOPIA, USING GEOSPATIAL TOOLS . A thesis submitted to . The school of Graduate Studies of Addis Ababa University in partial fulfillment of the requirements for the Degree of Masters of Science in Remote ...