- Research Process
- Manuscript Preparation
- Manuscript Review
- Publication Process
- Publication Recognition
- Language Editing Services
- Translation Services
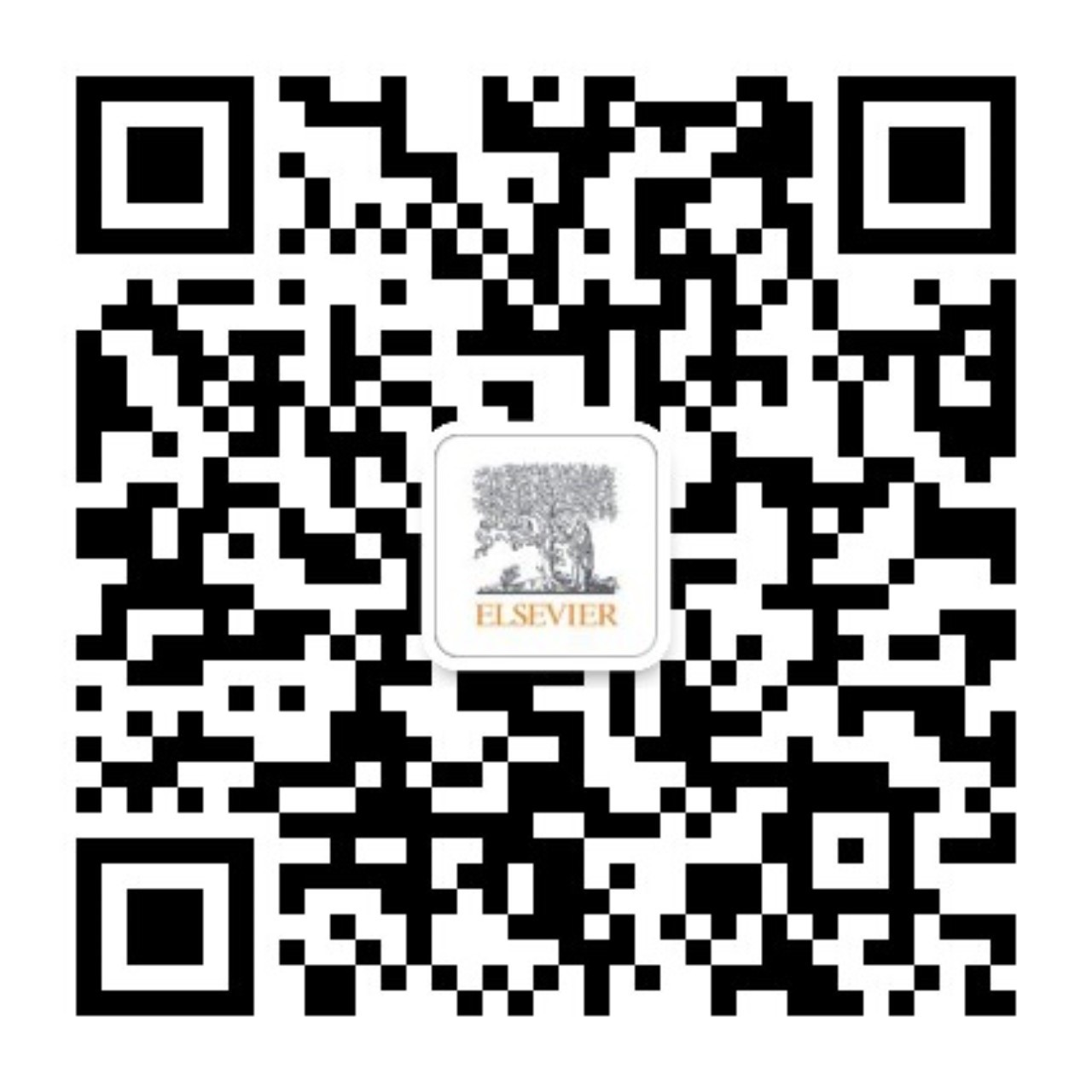

Why is data validation important in research?
- 3 minute read
- 63.1K views
Table of Contents
Data collection and analysis is one of the most important aspects of conducting research. High-quality data allows researchers to interpret findings accurately, act as a foundation for future studies, and give credibility to their research. As such, research often needs to go under the scanner to be free of suspicions of fraud and data falsification . At times, even unintentional errors in data could be viewed as research misconduct. Hence, data integrity is essential to protect your reputation and the reliability of your study.
Owing to the very nature of research and the sheer volume of data collected in large-scale studies, errors are bound to occur. One way to avoid “bad” or erroneous data is through data validation.
What is data validation?
Data validation is the process of examining the quality and accuracy of the collected data before processing and analysing it. It not only ensures the accuracy but also confirms the completeness of your data. However, data validation is time-consuming and can delay analysis significantly. So, is this step really important?
Importance of data validation
Data validation is important for several aspects of a well-conducted study:
- To ensure a robust dataset: The primary aim of data validation is to ensure an error-free dataset for further analysis. This is especially important if you or other researchers plan to use the dataset for future studies or to train machine learning models.
- To get a clearer picture of the data: Data validation also includes ‘cleaning-up’ of data, i.e., removing inputs that are incomplete, not standardized, or not within the range specified for your study. This process could also shed light on previously unknown patterns in the data and provide additional insights regarding the findings.
- To get accurate results: If your dataset has discrepancies, it will impact the final results and lead to inaccurate interpretations. Data validation can help identify errors, thus increasing the accuracy of your results.
- To mitigate the risk of forming incorrect hypotheses: Only those inferences and hypotheses that are backed by solid data are considered valid. Thus, data validation can help you form logical and reasonable speculations .
- To ensure the legitimacy of your findings: The integrity of your study is often determined by how reproducible it is. Data validation can enhance the reproducibility of your findings.
Data validation in research
Data validation is necessary for all types of research. For quantitative research, which utilizes measurable data points, the quality of data can be enhanced by selecting the correct methodology, avoiding biases in the study design, choosing an appropriate sample size and type, and conducting suitable statistical analyses.
In contrast, qualitative research , which includes surveys or behavioural studies, is prone to the use of incomplete and/or poor-quality data. This is because of the likelihood that the responses provided by survey participants are inaccurate and due to the subjective nature of observational studies. Thus, it is extremely important to validate data by incorporating a range of clear and objective questions in surveys, bullet-proofing multiple-choice questions, and setting standard parameters for data collection.
Importantly, for studies that utilize machine learning approaches or mathematical models, validating the data model is as important as validating the data inputs. Thus, for the generation of automated data validation protocols, one must rely on appropriate data structures, content, and file types to avoid errors due to automation.
Although data validation may seem like an unnecessary or time-consuming step, it is absolutely critical to validate the integrity of your study and is absolutely worth the effort. To learn more about how to validate data effectively, head over to Elsevier Author Services !
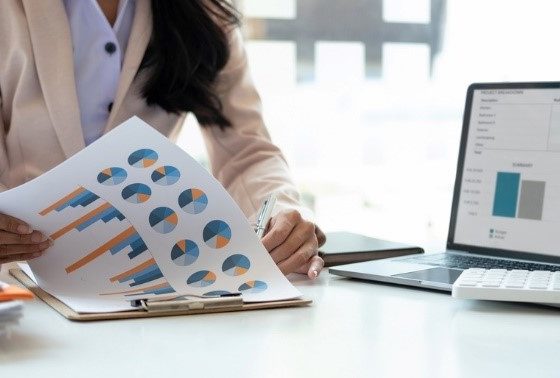
How to write the results section of a research paper
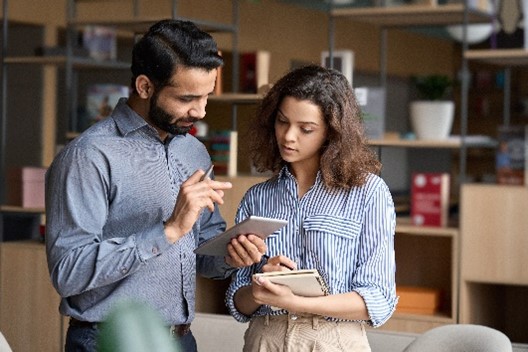
Choosing the Right Research Methodology: A Guide for Researchers
You may also like.
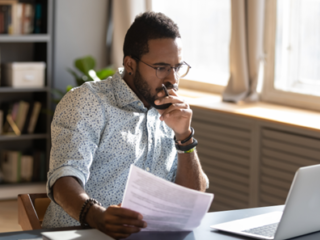
Descriptive Research Design and Its Myriad Uses
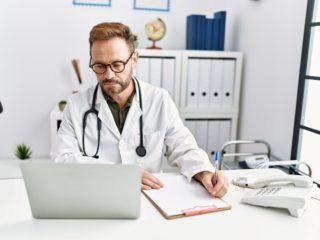
Five Common Mistakes to Avoid When Writing a Biomedical Research Paper
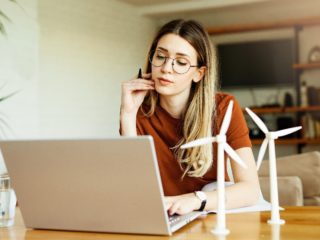
Making Technical Writing in Environmental Engineering Accessible
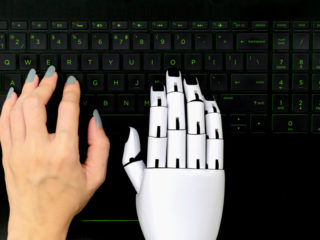
To Err is Not Human: The Dangers of AI-assisted Academic Writing
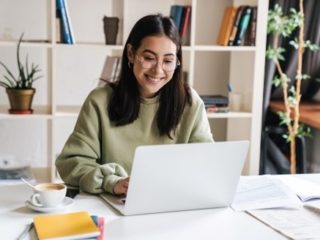
When Data Speak, Listen: Importance of Data Collection and Analysis Methods
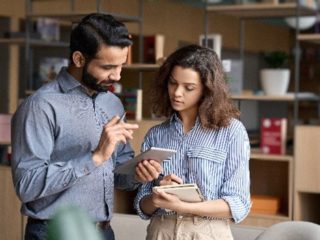
Writing a good review article
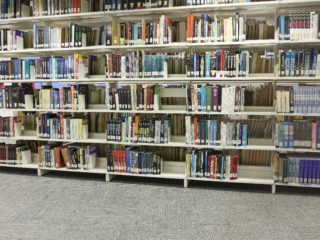
Scholarly Sources: What are They and Where can You Find Them?
Input your search keywords and press Enter.
- Search Menu
Sign in through your institution
- Browse content in Arts and Humanities
- Browse content in Archaeology
- Anglo-Saxon and Medieval Archaeology
- Archaeological Methodology and Techniques
- Archaeology by Region
- Archaeology of Religion
- Archaeology of Trade and Exchange
- Biblical Archaeology
- Contemporary and Public Archaeology
- Environmental Archaeology
- Historical Archaeology
- History and Theory of Archaeology
- Industrial Archaeology
- Landscape Archaeology
- Mortuary Archaeology
- Prehistoric Archaeology
- Underwater Archaeology
- Zooarchaeology
- Browse content in Architecture
- Architectural Structure and Design
- History of Architecture
- Residential and Domestic Buildings
- Theory of Architecture
- Browse content in Art
- Art Subjects and Themes
- History of Art
- Industrial and Commercial Art
- Theory of Art
- Biographical Studies
- Byzantine Studies
- Browse content in Classical Studies
- Classical Numismatics
- Classical Literature
- Classical Reception
- Classical History
- Classical Philosophy
- Classical Mythology
- Classical Art and Architecture
- Classical Oratory and Rhetoric
- Greek and Roman Archaeology
- Greek and Roman Epigraphy
- Greek and Roman Law
- Greek and Roman Papyrology
- Late Antiquity
- Religion in the Ancient World
- Social History
- Digital Humanities
- Browse content in History
- Colonialism and Imperialism
- Diplomatic History
- Environmental History
- Genealogy, Heraldry, Names, and Honours
- Genocide and Ethnic Cleansing
- Historical Geography
- History by Period
- History of Agriculture
- History of Education
- History of Emotions
- History of Gender and Sexuality
- Industrial History
- Intellectual History
- International History
- Labour History
- Legal and Constitutional History
- Local and Family History
- Maritime History
- Military History
- National Liberation and Post-Colonialism
- Oral History
- Political History
- Public History
- Regional and National History
- Revolutions and Rebellions
- Slavery and Abolition of Slavery
- Social and Cultural History
- Theory, Methods, and Historiography
- Urban History
- World History
- Browse content in Language Teaching and Learning
- Language Learning (Specific Skills)
- Language Teaching Theory and Methods
- Browse content in Linguistics
- Applied Linguistics
- Cognitive Linguistics
- Computational Linguistics
- Forensic Linguistics
- Grammar, Syntax and Morphology
- Historical and Diachronic Linguistics
- History of English
- Language Variation
- Language Families
- Language Acquisition
- Language Evolution
- Language Reference
- Lexicography
- Linguistic Theories
- Linguistic Typology
- Linguistic Anthropology
- Phonetics and Phonology
- Psycholinguistics
- Sociolinguistics
- Translation and Interpretation
- Writing Systems
- Browse content in Literature
- Bibliography
- Children's Literature Studies
- Literary Studies (Modernism)
- Literary Studies (Asian)
- Literary Studies (European)
- Literary Studies (Eco-criticism)
- Literary Studies (Romanticism)
- Literary Studies (American)
- Literary Studies - World
- Literary Studies (1500 to 1800)
- Literary Studies (19th Century)
- Literary Studies (20th Century onwards)
- Literary Studies (African American Literature)
- Literary Studies (British and Irish)
- Literary Studies (Early and Medieval)
- Literary Studies (Fiction, Novelists, and Prose Writers)
- Literary Studies (Gender Studies)
- Literary Studies (Graphic Novels)
- Literary Studies (History of the Book)
- Literary Studies (Plays and Playwrights)
- Literary Studies (Poetry and Poets)
- Literary Studies (Postcolonial Literature)
- Literary Studies (Queer Studies)
- Literary Studies (Science Fiction)
- Literary Studies (Travel Literature)
- Literary Studies (War Literature)
- Literary Studies (Women's Writing)
- Literary Theory and Cultural Studies
- Mythology and Folklore
- Shakespeare Studies and Criticism
- Browse content in Media Studies
- Browse content in Music
- Applied Music
- Dance and Music
- Ethics in Music
- Ethnomusicology
- Gender and Sexuality in Music
- Medicine and Music
- Music Cultures
- Music and Culture
- Music and Religion
- Music and Media
- Music Education and Pedagogy
- Music Theory and Analysis
- Musical Scores, Lyrics, and Libretti
- Musical Structures, Styles, and Techniques
- Musicology and Music History
- Performance Practice and Studies
- Race and Ethnicity in Music
- Sound Studies
- Browse content in Performing Arts
- Browse content in Philosophy
- Aesthetics and Philosophy of Art
- Epistemology
- Feminist Philosophy
- History of Western Philosophy
- Metaphysics
- Moral Philosophy
- Non-Western Philosophy
- Philosophy of Action
- Philosophy of Law
- Philosophy of Religion
- Philosophy of Science
- Philosophy of Language
- Philosophy of Mind
- Philosophy of Perception
- Philosophy of Mathematics and Logic
- Practical Ethics
- Social and Political Philosophy
- Browse content in Religion
- Biblical Studies
- Christianity
- East Asian Religions
- History of Religion
- Judaism and Jewish Studies
- Qumran Studies
- Religion and Education
- Religion and Health
- Religion and Politics
- Religion and Science
- Religion and Law
- Religion and Art, Literature, and Music
- Religious Studies
- Browse content in Society and Culture
- Cookery, Food, and Drink
- Cultural Studies
- Customs and Traditions
- Ethical Issues and Debates
- Hobbies, Games, Arts and Crafts
- Natural world, Country Life, and Pets
- Popular Beliefs and Controversial Knowledge
- Sports and Outdoor Recreation
- Technology and Society
- Travel and Holiday
- Visual Culture
- Browse content in Law
- Arbitration
- Browse content in Company and Commercial Law
- Commercial Law
- Company Law
- Browse content in Comparative Law
- Systems of Law
- Competition Law
- Browse content in Constitutional and Administrative Law
- Government Powers
- Judicial Review
- Local Government Law
- Military and Defence Law
- Parliamentary and Legislative Practice
- Construction Law
- Contract Law
- Browse content in Criminal Law
- Criminal Procedure
- Criminal Evidence Law
- Sentencing and Punishment
- Employment and Labour Law
- Environment and Energy Law
- Browse content in Financial Law
- Banking Law
- Insolvency Law
- History of Law
- Human Rights and Immigration
- Intellectual Property Law
- Browse content in International Law
- Private International Law and Conflict of Laws
- Public International Law
- IT and Communications Law
- Jurisprudence and Philosophy of Law
- Law and Society
- Law and Politics
- Browse content in Legal System and Practice
- Courts and Procedure
- Legal Skills and Practice
- Legal System - Costs and Funding
- Primary Sources of Law
- Regulation of Legal Profession
- Medical and Healthcare Law
- Browse content in Policing
- Criminal Investigation and Detection
- Police and Security Services
- Police Procedure and Law
- Police Regional Planning
- Browse content in Property Law
- Personal Property Law
- Restitution
- Study and Revision
- Terrorism and National Security Law
- Browse content in Trusts Law
- Wills and Probate or Succession
- Browse content in Medicine and Health
- Browse content in Allied Health Professions
- Arts Therapies
- Clinical Science
- Dietetics and Nutrition
- Occupational Therapy
- Operating Department Practice
- Physiotherapy
- Radiography
- Speech and Language Therapy
- Browse content in Anaesthetics
- General Anaesthesia
- Browse content in Clinical Medicine
- Acute Medicine
- Cardiovascular Medicine
- Clinical Genetics
- Clinical Pharmacology and Therapeutics
- Dermatology
- Endocrinology and Diabetes
- Gastroenterology
- Genito-urinary Medicine
- Geriatric Medicine
- Infectious Diseases
- Medical Oncology
- Medical Toxicology
- Pain Medicine
- Palliative Medicine
- Rehabilitation Medicine
- Respiratory Medicine and Pulmonology
- Rheumatology
- Sleep Medicine
- Sports and Exercise Medicine
- Clinical Neuroscience
- Community Medical Services
- Critical Care
- Emergency Medicine
- Forensic Medicine
- Haematology
- History of Medicine
- Medical Ethics
- Browse content in Medical Dentistry
- Oral and Maxillofacial Surgery
- Paediatric Dentistry
- Restorative Dentistry and Orthodontics
- Surgical Dentistry
- Browse content in Medical Skills
- Clinical Skills
- Communication Skills
- Nursing Skills
- Surgical Skills
- Medical Statistics and Methodology
- Browse content in Neurology
- Clinical Neurophysiology
- Neuropathology
- Nursing Studies
- Browse content in Obstetrics and Gynaecology
- Gynaecology
- Occupational Medicine
- Ophthalmology
- Otolaryngology (ENT)
- Browse content in Paediatrics
- Neonatology
- Browse content in Pathology
- Chemical Pathology
- Clinical Cytogenetics and Molecular Genetics
- Histopathology
- Medical Microbiology and Virology
- Patient Education and Information
- Browse content in Pharmacology
- Psychopharmacology
- Browse content in Popular Health
- Caring for Others
- Complementary and Alternative Medicine
- Self-help and Personal Development
- Browse content in Preclinical Medicine
- Cell Biology
- Molecular Biology and Genetics
- Reproduction, Growth and Development
- Primary Care
- Professional Development in Medicine
- Browse content in Psychiatry
- Addiction Medicine
- Child and Adolescent Psychiatry
- Forensic Psychiatry
- Learning Disabilities
- Old Age Psychiatry
- Psychotherapy
- Browse content in Public Health and Epidemiology
- Epidemiology
- Public Health
- Browse content in Radiology
- Clinical Radiology
- Interventional Radiology
- Nuclear Medicine
- Radiation Oncology
- Reproductive Medicine
- Browse content in Surgery
- Cardiothoracic Surgery
- Gastro-intestinal and Colorectal Surgery
- General Surgery
- Neurosurgery
- Paediatric Surgery
- Peri-operative Care
- Plastic and Reconstructive Surgery
- Surgical Oncology
- Transplant Surgery
- Trauma and Orthopaedic Surgery
- Vascular Surgery
- Browse content in Science and Mathematics
- Browse content in Biological Sciences
- Aquatic Biology
- Biochemistry
- Bioinformatics and Computational Biology
- Developmental Biology
- Ecology and Conservation
- Evolutionary Biology
- Genetics and Genomics
- Microbiology
- Molecular and Cell Biology
- Natural History
- Plant Sciences and Forestry
- Research Methods in Life Sciences
- Structural Biology
- Systems Biology
- Zoology and Animal Sciences
- Browse content in Chemistry
- Analytical Chemistry
- Computational Chemistry
- Crystallography
- Environmental Chemistry
- Industrial Chemistry
- Inorganic Chemistry
- Materials Chemistry
- Medicinal Chemistry
- Mineralogy and Gems
- Organic Chemistry
- Physical Chemistry
- Polymer Chemistry
- Study and Communication Skills in Chemistry
- Theoretical Chemistry
- Browse content in Computer Science
- Artificial Intelligence
- Computer Architecture and Logic Design
- Game Studies
- Human-Computer Interaction
- Mathematical Theory of Computation
- Programming Languages
- Software Engineering
- Systems Analysis and Design
- Virtual Reality
- Browse content in Computing
- Business Applications
- Computer Games
- Computer Security
- Computer Networking and Communications
- Digital Lifestyle
- Graphical and Digital Media Applications
- Operating Systems
- Browse content in Earth Sciences and Geography
- Atmospheric Sciences
- Environmental Geography
- Geology and the Lithosphere
- Maps and Map-making
- Meteorology and Climatology
- Oceanography and Hydrology
- Palaeontology
- Physical Geography and Topography
- Regional Geography
- Soil Science
- Urban Geography
- Browse content in Engineering and Technology
- Agriculture and Farming
- Biological Engineering
- Civil Engineering, Surveying, and Building
- Electronics and Communications Engineering
- Energy Technology
- Engineering (General)
- Environmental Science, Engineering, and Technology
- History of Engineering and Technology
- Mechanical Engineering and Materials
- Technology of Industrial Chemistry
- Transport Technology and Trades
- Browse content in Environmental Science
- Applied Ecology (Environmental Science)
- Conservation of the Environment (Environmental Science)
- Environmental Sustainability
- Environmentalist Thought and Ideology (Environmental Science)
- Management of Land and Natural Resources (Environmental Science)
- Natural Disasters (Environmental Science)
- Nuclear Issues (Environmental Science)
- Pollution and Threats to the Environment (Environmental Science)
- Social Impact of Environmental Issues (Environmental Science)
- History of Science and Technology
- Browse content in Materials Science
- Ceramics and Glasses
- Composite Materials
- Metals, Alloying, and Corrosion
- Nanotechnology
- Browse content in Mathematics
- Applied Mathematics
- Biomathematics and Statistics
- History of Mathematics
- Mathematical Education
- Mathematical Finance
- Mathematical Analysis
- Numerical and Computational Mathematics
- Probability and Statistics
- Pure Mathematics
- Browse content in Neuroscience
- Cognition and Behavioural Neuroscience
- Development of the Nervous System
- Disorders of the Nervous System
- History of Neuroscience
- Invertebrate Neurobiology
- Molecular and Cellular Systems
- Neuroendocrinology and Autonomic Nervous System
- Neuroscientific Techniques
- Sensory and Motor Systems
- Browse content in Physics
- Astronomy and Astrophysics
- Atomic, Molecular, and Optical Physics
- Biological and Medical Physics
- Classical Mechanics
- Computational Physics
- Condensed Matter Physics
- Electromagnetism, Optics, and Acoustics
- History of Physics
- Mathematical and Statistical Physics
- Measurement Science
- Nuclear Physics
- Particles and Fields
- Plasma Physics
- Quantum Physics
- Relativity and Gravitation
- Semiconductor and Mesoscopic Physics
- Browse content in Psychology
- Affective Sciences
- Clinical Psychology
- Cognitive Neuroscience
- Cognitive Psychology
- Criminal and Forensic Psychology
- Developmental Psychology
- Educational Psychology
- Evolutionary Psychology
- Health Psychology
- History and Systems in Psychology
- Music Psychology
- Neuropsychology
- Organizational Psychology
- Psychological Assessment and Testing
- Psychology of Human-Technology Interaction
- Psychology Professional Development and Training
- Research Methods in Psychology
- Social Psychology
- Browse content in Social Sciences
- Browse content in Anthropology
- Anthropology of Religion
- Human Evolution
- Medical Anthropology
- Physical Anthropology
- Regional Anthropology
- Social and Cultural Anthropology
- Theory and Practice of Anthropology
- Browse content in Business and Management
- Business History
- Business Strategy
- Business Ethics
- Business and Government
- Business and Technology
- Business and the Environment
- Comparative Management
- Corporate Governance
- Corporate Social Responsibility
- Entrepreneurship
- Health Management
- Human Resource Management
- Industrial and Employment Relations
- Industry Studies
- Information and Communication Technologies
- International Business
- Knowledge Management
- Management and Management Techniques
- Operations Management
- Organizational Theory and Behaviour
- Pensions and Pension Management
- Public and Nonprofit Management
- Social Issues in Business and Management
- Strategic Management
- Supply Chain Management
- Browse content in Criminology and Criminal Justice
- Criminal Justice
- Criminology
- Forms of Crime
- International and Comparative Criminology
- Youth Violence and Juvenile Justice
- Development Studies
- Browse content in Economics
- Agricultural, Environmental, and Natural Resource Economics
- Asian Economics
- Behavioural Finance
- Behavioural Economics and Neuroeconomics
- Econometrics and Mathematical Economics
- Economic Methodology
- Economic Systems
- Economic History
- Economic Development and Growth
- Financial Markets
- Financial Institutions and Services
- General Economics and Teaching
- Health, Education, and Welfare
- History of Economic Thought
- International Economics
- Labour and Demographic Economics
- Law and Economics
- Macroeconomics and Monetary Economics
- Microeconomics
- Public Economics
- Urban, Rural, and Regional Economics
- Welfare Economics
- Browse content in Education
- Adult Education and Continuous Learning
- Care and Counselling of Students
- Early Childhood and Elementary Education
- Educational Equipment and Technology
- Educational Strategies and Policy
- Higher and Further Education
- Organization and Management of Education
- Philosophy and Theory of Education
- Schools Studies
- Secondary Education
- Teaching of a Specific Subject
- Teaching of Specific Groups and Special Educational Needs
- Teaching Skills and Techniques
- Browse content in Environment
- Applied Ecology (Social Science)
- Climate Change
- Conservation of the Environment (Social Science)
- Environmentalist Thought and Ideology (Social Science)
- Management of Land and Natural Resources (Social Science)
- Natural Disasters (Environment)
- Pollution and Threats to the Environment (Social Science)
- Social Impact of Environmental Issues (Social Science)
- Sustainability
- Browse content in Human Geography
- Cultural Geography
- Economic Geography
- Political Geography
- Browse content in Interdisciplinary Studies
- Communication Studies
- Museums, Libraries, and Information Sciences
- Browse content in Politics
- African Politics
- Asian Politics
- Chinese Politics
- Comparative Politics
- Conflict Politics
- Elections and Electoral Studies
- Environmental Politics
- Ethnic Politics
- European Union
- Foreign Policy
- Gender and Politics
- Human Rights and Politics
- Indian Politics
- International Relations
- International Organization (Politics)
- Irish Politics
- Latin American Politics
- Middle Eastern Politics
- Political Theory
- Political Methodology
- Political Communication
- Political Philosophy
- Political Sociology
- Political Behaviour
- Political Economy
- Political Institutions
- Politics and Law
- Politics of Development
- Public Administration
- Public Policy
- Qualitative Political Methodology
- Quantitative Political Methodology
- Regional Political Studies
- Russian Politics
- Security Studies
- State and Local Government
- UK Politics
- US Politics
- Browse content in Regional and Area Studies
- African Studies
- Asian Studies
- East Asian Studies
- Japanese Studies
- Latin American Studies
- Middle Eastern Studies
- Native American Studies
- Scottish Studies
- Browse content in Research and Information
- Research Methods
- Browse content in Social Work
- Addictions and Substance Misuse
- Adoption and Fostering
- Care of the Elderly
- Child and Adolescent Social Work
- Couple and Family Social Work
- Direct Practice and Clinical Social Work
- Emergency Services
- Human Behaviour and the Social Environment
- International and Global Issues in Social Work
- Mental and Behavioural Health
- Social Justice and Human Rights
- Social Policy and Advocacy
- Social Work and Crime and Justice
- Social Work Macro Practice
- Social Work Practice Settings
- Social Work Research and Evidence-based Practice
- Welfare and Benefit Systems
- Browse content in Sociology
- Childhood Studies
- Community Development
- Comparative and Historical Sociology
- Disability Studies
- Economic Sociology
- Gender and Sexuality
- Gerontology and Ageing
- Health, Illness, and Medicine
- Marriage and the Family
- Migration Studies
- Occupations, Professions, and Work
- Organizations
- Population and Demography
- Race and Ethnicity
- Social Theory
- Social Movements and Social Change
- Social Research and Statistics
- Social Stratification, Inequality, and Mobility
- Sociology of Religion
- Sociology of Education
- Sport and Leisure
- Urban and Rural Studies
- Browse content in Warfare and Defence
- Defence Strategy, Planning, and Research
- Land Forces and Warfare
- Military Administration
- Military Life and Institutions
- Naval Forces and Warfare
- Other Warfare and Defence Issues
- Peace Studies and Conflict Resolution
- Weapons and Equipment

- < Previous
- Next chapter >
1 Validity and Validation in Research and Assessment
- Published: October 2013
- Cite Icon Cite
- Permissions Icon Permissions
This chapter first sets out the book's purpose, namely to further define validity and to explore the factors that should be considered when evaluating claims from research and assessment. It then discusses validity theory and its philosophical foundations, with connections between the philosophical foundations and specific ways validation is considered in research and measurement. An overview of the subsequent chapters is also presented.
Personal account
- Sign in with email/username & password
- Get email alerts
- Save searches
- Purchase content
- Activate your purchase/trial code
- Add your ORCID iD
Institutional access
Sign in with a library card.
- Sign in with username/password
- Recommend to your librarian
- Institutional account management
- Get help with access
Access to content on Oxford Academic is often provided through institutional subscriptions and purchases. If you are a member of an institution with an active account, you may be able to access content in one of the following ways:
IP based access
Typically, access is provided across an institutional network to a range of IP addresses. This authentication occurs automatically, and it is not possible to sign out of an IP authenticated account.
Choose this option to get remote access when outside your institution. Shibboleth/Open Athens technology is used to provide single sign-on between your institution’s website and Oxford Academic.
- Click Sign in through your institution.
- Select your institution from the list provided, which will take you to your institution's website to sign in.
- When on the institution site, please use the credentials provided by your institution. Do not use an Oxford Academic personal account.
- Following successful sign in, you will be returned to Oxford Academic.
If your institution is not listed or you cannot sign in to your institution’s website, please contact your librarian or administrator.
Enter your library card number to sign in. If you cannot sign in, please contact your librarian.
Society Members
Society member access to a journal is achieved in one of the following ways:
Sign in through society site
Many societies offer single sign-on between the society website and Oxford Academic. If you see ‘Sign in through society site’ in the sign in pane within a journal:
- Click Sign in through society site.
- When on the society site, please use the credentials provided by that society. Do not use an Oxford Academic personal account.
If you do not have a society account or have forgotten your username or password, please contact your society.
Sign in using a personal account
Some societies use Oxford Academic personal accounts to provide access to their members. See below.
A personal account can be used to get email alerts, save searches, purchase content, and activate subscriptions.
Some societies use Oxford Academic personal accounts to provide access to their members.
Viewing your signed in accounts
Click the account icon in the top right to:
- View your signed in personal account and access account management features.
- View the institutional accounts that are providing access.
Signed in but can't access content
Oxford Academic is home to a wide variety of products. The institutional subscription may not cover the content that you are trying to access. If you believe you should have access to that content, please contact your librarian.
For librarians and administrators, your personal account also provides access to institutional account management. Here you will find options to view and activate subscriptions, manage institutional settings and access options, access usage statistics, and more.
Our books are available by subscription or purchase to libraries and institutions.
Month: | Total Views: |
---|---|
October 2022 | 16 |
November 2022 | 10 |
December 2022 | 9 |
January 2023 | 3 |
February 2023 | 10 |
March 2023 | 31 |
April 2023 | 14 |
May 2023 | 15 |
June 2023 | 3 |
July 2023 | 6 |
August 2023 | 10 |
September 2023 | 10 |
October 2023 | 9 |
November 2023 | 7 |
December 2023 | 7 |
January 2024 | 11 |
February 2024 | 10 |
March 2024 | 12 |
April 2024 | 24 |
May 2024 | 29 |
June 2024 | 6 |
July 2024 | 5 |
- About Oxford Academic
- Publish journals with us
- University press partners
- What we publish
- New features
- Open access
- Rights and permissions
- Accessibility
- Advertising
- Media enquiries
- Oxford University Press
- Oxford Languages
- University of Oxford
Oxford University Press is a department of the University of Oxford. It furthers the University's objective of excellence in research, scholarship, and education by publishing worldwide
- Copyright © 2024 Oxford University Press
- Cookie settings
- Cookie policy
- Privacy policy
- Legal notice
This Feature Is Available To Subscribers Only
Sign In or Create an Account
This PDF is available to Subscribers Only
For full access to this pdf, sign in to an existing account, or purchase an annual subscription.
How to Improve Data Validation in Five Steps
Mercatus Working Paper Series
18 Pages Posted: 26 Mar 2021
Danilo Freire
Independent Researcher
Date Written: March 25, 2021
Social scientists are awash with new data sources. Though data preprocessing and storage methods have developed considerably over the past few years, there is little agreement on what constitutes the best set of data validation practices in the social sciences. In this paper I provide five simple steps that can help students and practitioners improve their data validation processes. I discuss how to create testable validation functions, how to increase construct validity, and how to incorporate qualitative knowledge in statistical measurements. I present the concepts according to their level of abstraction, and I provide practical examples on how scholars can add my suggestions to their work.
Keywords: computational social science, computer programming, content validity, data validation, tidy data
JEL Classification: A20, C80, C88
Suggested Citation: Suggested Citation
Danilo Freire (Contact Author)
Independent researcher ( email ), do you have a job opening that you would like to promote on ssrn, paper statistics, related ejournals, mercatus center research paper series.
Subscribe to this free journal for more curated articles on this topic
Innovation Educator: Courses, Cases & Teaching eJournal
Subscribe to this fee journal for more curated articles on this topic
Sociology of Innovation eJournal
Information systems ejournal, other innovation research & policy ejournal.
Thank you for visiting nature.com. You are using a browser version with limited support for CSS. To obtain the best experience, we recommend you use a more up to date browser (or turn off compatibility mode in Internet Explorer). In the meantime, to ensure continued support, we are displaying the site without styles and JavaScript.
- View all journals
- Explore content
- About the journal
- Publish with us
- Sign up for alerts
- Open access
- Published: 22 April 2024
Practical approaches in evaluating validation and biases of machine learning applied to mobile health studies
- Johannes Allgaier ORCID: orcid.org/0000-0002-9051-2004 1 &
- Rüdiger Pryss ORCID: orcid.org/0000-0003-1522-785X 1
Communications Medicine volume 4 , Article number: 76 ( 2024 ) Cite this article
844 Accesses
1 Altmetric
Metrics details
- Health services
- Medical research
Machine learning (ML) models are evaluated in a test set to estimate model performance after deployment. The design of the test set is therefore of importance because if the data distribution after deployment differs too much, the model performance decreases. At the same time, the data often contains undetected groups. For example, multiple assessments from one user may constitute a group, which is usually the case in mHealth scenarios.
In this work, we evaluate a model’s performance using several cross-validation train-test-split approaches, in some cases deliberately ignoring the groups. By sorting the groups (in our case: Users) by time, we additionally simulate a concept drift scenario for better external validity. For this evaluation, we use 7 longitudinal mHealth datasets, all containing Ecological Momentary Assessments (EMA). Further, we compared the model performance with baseline heuristics, questioning the essential utility of a complex ML model.
Hidden groups in the dataset leads to overestimation of ML performance after deployment. For prediction, a user’s last completed questionnaire is a reasonable heuristic for the next response, and potentially outperforms a complex ML model. Because we included 7 studies, low variance appears to be a more fundamental phenomenon of mHealth datasets.
Conclusions
The way mHealth-based data are generated by EMA leads to questions of user and assessment level and appropriate validation of ML models. Our analysis shows that further research needs to follow to obtain robust ML models. In addition, simple heuristics can be considered as an alternative for ML. Domain experts should be consulted to find potentially hidden groups in the data.
Plain Language Summary
Computational approaches can be used to analyse health-related data collected using mobile applications from thousands of participants. We tested the impact of some participants being represented multiple times or some not being counted properly within the analysis. In this context, we label a multi-represented participant a group. We find that ignoring such groups can lead to false estimation of health-related predictions. In some cases, simpler quantitative methods can outperform complex computational models. This highlights the importance of monitoring and validating results conducted by complex computational models and confers the use of simpler analytical methods in its place.
Similar content being viewed by others
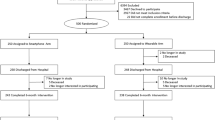
Using remotely monitored patient activity patterns after hospital discharge to predict 30 day hospital readmission: a randomized trial
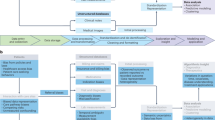
Harnessing EHR data for health research
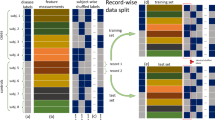
Detecting the impact of subject characteristics on machine learning-based diagnostic applications
Introduction.
When machine learning models are applied to medical data, an important question is whether the model learns subject-specific characteristics (not desired effect) or disease-related characteristics (desired effect) between an input and output. A recent paper by Kunjan et al. 1 describes this very well at the example of classification and EEG disease diagnosis. In the Kunjan et al. paper, this is discussed using different variants of cross-validation. It is well shown that the type of validation can cause extreme differences. Older work has evaluated different cross-validation techniques on datasets with different recommendations for the number of optimal folds 2 , 3 . We transfer and adapt this idea to mHealth data and the application of machine-learning-based classification and raise new questions about this. To this end, we will briefly explain the background. Using simple, understandable models rather than complex black box models is a clamor of Rudin et. al., which motivates us to evaluate simple heuristics against complex models 4 . The Cross-Industry Standard Process for Data Mining (CRISP-DM) highlights the importance of subject matter experts to get familiar with a dataset 5 . In turn, familiarity with the dataset is necessary to detect hidden groups in the dataset. In our mHealth use cases, one app user that fills out more several questionnaires constitutes a group.
We have developed numerous applications in mobile health in recent years (e.g. 6 , 7 ) and the issue of disease-related or subject-specific characteristics is particularly pronounced in these applications. mHealth applications very often use the principles of Patient-reported Outcome Measures (PROMs) or/and Ecological Momentary Assessments (EMAs) 8 . EMAs have the major goal that users record symptoms several times a day over a longer period. As a result, users of an mHealth solution generate longitudinal data with many assessments. Since not all users respond equally frequently in the applications (as shown by many applications that have been in operation for a long time 9 ), the result is a very different number of assessments per user. Therefore, the question arises in the application of machine learning, how the actual learning takes place. In learning, should we group the ratings per user so that a user only appears in either the training set or the testing set, which is correct by design. Or, can we accept that a user’s ratings appear in both the training and test sets, since users with many ratings have such a high variance in ratings. Finally, individual users may undergo concept drift in the way they answer questions in many assessments over a long period of time. In such a case, the question also arises as to whether it makes sense to use an individual’s ratings separately in the training and testing sets.
In this context, we also see another question as relevant that is not given enough attention: What is an appropriate baseline for a machine learning outcome in studies? As mentioned earlier, some mHealth users fill out thousands of assessments, and do so for years. In this case, there may be questions about whether a previous assessment can reliably predict the next one, and the use of machine learning may be poorly targeted.
With respect to the above research questions, we use another component to further promote the results. We selected seven studies from the pool of developed apps that we will use for the analysis of this paper. Since a total of 7 studies are used, a more representative picture should emerge. However, since the studies do not all have the same research goals, classification tasks need to be found per app to make the overall results comparable. The studies also do not all have the same duration. Even though the studies are not always directly comparable, the setting is very promising as the results will show in the end. Before deriving specific research questions against this background, related work and technical background information will be briefly discussed.
This section surveys relevant literature to contextualize our contributions within the broader field of study. Cawley et al. also address the question of how to minimize the error in the estimator of performance in ground truth. Using synthetic data sets, they argue that overfitting a model is as problematic as selection bias in the training data 10 . However, they do not address the phenomenon of groups in the data. Refaeilzadeh et al. give an overview of common cross-validation techniques such as leave-one-out, repeated k-fold, or hold-out validation 11 . They discuss pros and cons of each kind and mention an underestimated performance variance for repeated k-fold cross-validation, but they also do not address the problem with (unknown) groups in the dataset 11 . Schratz et. al. focus on spatial auto-correlation and spatial cross-validation rather than on groups and splitting approaches 12 . Spatial cross-validation is sometimes also referred to as block cross-validation13. They observe large performance differences in the use or non-use of spatial cross validation. By random sampling of train and test samples, a train and test sample might be too close to each other on a geographical space, which induces a selection bias and thus an overoptimistic estimate of the generalization error. They then use spatial cross-validation. We would like to briefly differentiate between space and group . Two samples belong to the same space if they are geographically close to each other 13 . They belong to the same group if a domain expert assigns them to a group. In our work, multiple assessments belonging to one user form a group. Meyer et al. also evaluate using a spatial cross-validation approach, but also add a time dimension using Leave-Time-Out cross-validation where samples belong to one fold if they fall into a specific time range 14 . This leave-time-out approach is like our time-cut approach, which will be introduced in the methods section. Yet, we are not aware of any related approach on mHealth data like the one we are pursuing in this work.
As written at the beginning of the introduction, we want to evaluate how much the model’s performance depends on specific users (syn. subjects, patients, persons ) that are represented several times within our dataset, but with a varying number of assessments per user. From previous work, we already know that so-called power-users with many more assessments than most of the other users have a high impact on the models training procedure 15 . We would further like to investigate whether a simple heuristic can outperform complex ensemble methods. Simple heuristics are interesting because they are easy to understand, have a low maintenance requirement, and have low variance, but also generate high bias.
Technically, across studies (i.e., across the seven studies), we investigate simple heuristics at the user and assessment level and compare them to tree-based non-tuned ML ensembles. Tree-based methods have already been proven in the literature on the specific mHealth data used, that is why we use only tree-based methods. The reason for not tuning these models is that we want to be more comparable across the used studies. With these levels of consideration, we would like to elaborate on the following research two questions: First, what is the variance in performance when using different splitting methods for train and test set of mHealth data (RQ1)? Second, in which cases is the development, deployment and maintenance of a ML model compared to a simple baseline heuristic worthwhile when being used on mHealth data?
The present work compares the performance of a tree-based ensemble method if the split of the data happens on two different levels: User and assessment. It further compares this performance to non-ML approaches that uses simple heuristics to also predict the target on a user- or assessment level. To summarize the major findings: First, ignoring users in datasets during cross-validation leads to an overestimation of the model’s performance and robustness. Second, for some use cases, simple heuristics are as good as complicated tree-based ensemble methods. Within this domain, heuristics are more advantageous if they are trained or applied at the user level. ML models also work at the assessment level. And third, sorting users can simulate concept drift in training if the time span of data collection is large enough. The results in the test set change due to the shuffling of users.
In this section, we first describe how Ecological Momentary Assessments work and how they differentiate from assessments that are collected within a clinical environment. Second, we present the studies and ML use cases for each dataset. Next, we introduce the non-ML baseline heuristics and explain the ML preprocessing steps. Finally, we describe existing train-test-split approaches (cross-validation) and the splitting approaches at the user- and assessment levels.
Ecological momentary assessments
Within this context, ecological means “within the subject’s natural environment", and momentary “within this moment" and ideally, in real time 16 . Assessments collected in research or clinical environments may cause recall bias of the subject’s answers and are not primarily designed to track changes in mood or behavior longitudinally. Ecological Momentary Assessments (EMA) thus increase validity and decrease recall bias. They are suitable for asking users in their daily environment about their state of being, which can change over time, by random or interval time sampling. Combining EMAs and mobile crowdsensing sensor measurements allows for multimodal analyses, which can gain new insights in, e.g., chronic diseases 8 , 15 . The datasets used within this work have EMA in common and are described in the following subsection.
The ML use cases
From ongoing projects of our team, we are constantly collecting mHealth data as well as Ecological Momentary Assessments 6 , 17 , 18 , 19 . To investigate how the machine learning performance varies based on the splits, we wanted different datasets with different use cases. However, to increase comparability between the use cases, we created multi-class classification tasks.
We train each model using historical assessments, the oldest assessment was collected at time t s t a r t , the latest historical assessment at time t l a s t . A current assessment is created and collected at time t n o w , a future assessment at time t n e x t . Depending on the study design, the actual point of time t n e x t may be in some hours or in a few weeks from t n o w . For each dataset and for each user, we want to predict a feature (synonym, a question of an assessment) at time t n e x t using the features at time t n o w . This feature at time t n e x t is then called the target. For each use case, a model is trained using data between t s t a r t and t l a s t , and given the input data from t n o w , it predicts the target at t n e x t . Figure 1 gives a schematic representation of the relevant points of time t s t a r t , t l a s t , t n o w , and t n e x t .
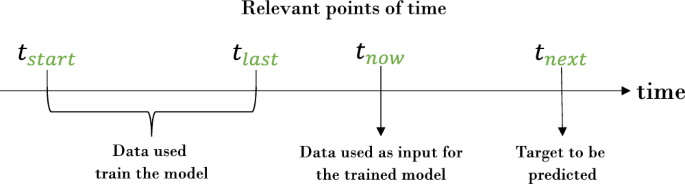
At time t s t a r t , the first assessment is given; t l a s t is the last known assessment used for training, whereas t n o w is the currently available assessment as input for the classifier and the target is predicted at time t t e x t .
To increase comparability between the approaches, we used the same model architecture with the same pseudo-random initialisation. The model is a Random Forest classifier with 100 trees and the Gini impurity as the splitting criterion. The whole coding was in Python 3.9, using mostly scikit-learn , pandas and Jupyter Notebooks . Details can be found on GitHub in the supplementary material.
The included apps and studies in more detail
For all datasets that we used in this study, we have ethical approvals (UNITI No. 20-1936-101, TYT No. 15-101-0204, Corona Check No. 71/20-me, and Corona Health No. 130/20-me). The following section provides an overview of the studies, the available datasets with characteristics, and then describes each use case in more detail. An brief overview is given in Table 1 with baseline statistics for each dataset in Table 2 .
To provide some more background info about the studies: The analyses happen with all apps on the so-called EMA questionnaires (synonym: assessment), i.e., the questionnaires that are filled out multiple times in all apps and the respective studies. This can happen several times a day (e.g., for the tinnitus study TrackYourTinnitus (TYT)) or at weekly intervals (e.g., studies in the Corona Health (CH) app). Nevertheless, the analysis happens on the recurring questionnaires, which collect symptoms over time and in the real environment through unforeseen (i.e., random) notifications.
The TrackYourTinnitus (TYT) dataset has the most filled-out assessments with more than 110,000 questionnaires as by 2022-10-24. The Corona Check (CC) study has the most users. This is because each time an assessment is filled out, a new user can optionally be created. Notably, this app has the largest ratio of non-German users and the youngest user group with the largest standard deviation. The Corona Health (CH) app with its studies Mental health for adults, adolescents and physical health for adults has the highest proportion of German users because it was developed in collaboration with the Robert Koch Institute and was primarily promoted in Germany. Unification of treatments and Interventions for Tinnitus patients (UNITI) is a European Union-wide project, which overall aim is to deliver a predictive computational model based on existing and longitudinal data 19 . The dataset from the UNITI randomized controlled trial is described by Simoes et al. 20 .
TrackYourTinnitus (TYT)
With this app, it is possible to record the individual fluctuations in tinnitus perception. With the help of a mobile device, users can systematically measure the fluctuations of their tinnitus. Via the TYT website or the app, users can also view the progress of their own data and, if necessary, discuss it with their physician.
The ML task at hand is a classification task with target variable Tinnitus distress at time t n o w and the questions from the daily questionnaire as the features of the problem. The target’s values range in [0, 1] on a continuous scale. To make it a classification task, we created bins with step size of 0.2 resulting in 5 classes. The features are perception , loudness , and stressfulness of tinnitus, as well as the current mood , arousal and stress level of a user, the concentration level while filling out the questionnaire, and perception of the worst tinnitus symptom . A detailed description of the features was already done in previous works 21 . Of note, the time delta of two assessments of one user at t n e x t and t n o w varies between users. Its median value is 11 hours.
Unification of Treatments and Interventions for Tinnitus Patients (UNITI)
The overall goal of UNITI is to treat the heterogeneity of tinnitus patients on an individual basis. This requires understanding more about the patient-specific symptoms that are captured by EMA in real time.
The use case we created at UNITI is like that of TYT. The target variable encumbrance, coded as cumberness , which was also continuously recorded, was divided into an ordinal scale from 0 to 1 in 5 steps. Features also include momentary assessments of the user during completion, such as jawbone, loudness, movement, stress, emotion , and questions about momentary tinnitus. The data was collected using our mobile apps 7 . Here, of note: on average, the median time gap between two assessment is 24 hours for each user.
Corona Check (CC)
At the beginning of the COVID-19 pandemic, it was not easy to get initial feedback about an infection, given the lack of knowledge about the novel virus and the absence of widely available tests. To assist all citizens in this regard, we launched the mobile health app Corona Check together with the Bavarian State Office for Health and Food Safety 22 .
The Corona Check dataset predicts whether a user has a Covid infection based on a list of given symptoms 23 . It was developed in the early pandemic back in 2020 and helped people to get quick estimate for an infection without having an antigen test. The target variable has four classes: First, “suspected coronavirus (COVID-19) case", second, “symptoms, but no known contact with confirmed corona case", third, “contact with confirmed corona case, but currently no symptoms", and last, “neither symptoms nor contact".
The features are a list of Boolean variables, which were known at this time to be typically related with a Covid infection, such as fever, a sore throat, a runny nose, cough, loss of smell, loss of taste, shortness of breath, headache, muscle pain, diarrhea, and general weakness. Depending on the answers given by a user, the application programming interface returned one of the classes. The median time gap of two assessments for the same user is 8 hours on average with a much larger standard deviation of 24.6 days.
Corona Health ∣ Mental health for adults (CHA)
The last four use cases are all derived from a bigger Covid-related mHealth project called Corona Health 6 , 24 . The app was developed in collaboration with the Robert Koch-Institute and was primarily promoted in Germany, it includes several studies about the mental or physical health, or the stress level of a user. A user can download the app and then sign up for a study. He or she will then receive a baseline one-time questionnaire, followed by recurring follow-ups with between-study varying time gaps. The follow-up assessment of CHA has a total of 159 questions including a full PHQ9 questionnaire 25 . We then used the nine questions of PHQ9 as features at t n o w to predict the level of depression for this user for t n e x t . Depression levels are ordinally scaled from None to Severe in a total of 5 classes. The median time gap of two assessments for the same user is 7.5 days. That is, the models predict the future in this time interval.
Corona Health ∣ Mental health for adolescents (CHY)
Similar to the adult cohort, the mental health of adolescents during the pandemic and its lock-downs is also captured by our app using EMA.
A lightweight version of the mental health questionnaire for adults was also offered to adolescents. However, this did not include a full PHQ9 questionnaire, so we created a different use case. The target variable to be classified on a 4-level ordinal scale is perceived dejection coming from the PHQ instruments, features are a subset of quality of live assessments and PHQ questions, such as concernment, tremor, comfort, leisure quality, lethargy, prostration, and irregular sleep. For this study, the median time gap of two follow up assessments is 7.3 days.
Corona Health ∣ Physical health for adults (CHP)
Analogous to the mental health of adults, this study aims to track how the physical health of adults changes during the pandemic period.
Adults had the option to sign up for a study with recurring assessments asking for their physical health. The target variable to be classified asks about the constraints in everyday life that arise due to physical pain at t n e x t . The features for this use case include aspects like sport, nutrition, and pain at t n o w . The median time gap of two assessments for the same user is 14.0 days.
Corona Health ∣ Stress (CHS)
This additional study within the Corona Health app asks users about their stress level on a weekly basis. Both features and target are assessed on a five-level ordinal scale from never to very often . The target asks for the ability of stress management, features include the first nine questions of the perceived stress scale instrument 26 . The median time gap of two assessments for the same user on average is 7.0 days.
Baseline heuristics instead of complex ML models?
We also want to compare the ML approaches with a baseline heuristic ( synonym: Baseline model ). A baseline heuristic can be a simple ML model like a linear regression or a small Decision Tree, or alternatively, depending on the use case, it could also be a simple statement like “The next value equals the last one". The typical approach for improving ML models is to estimate the generalization error of the model on a benchmark data set when compared to a baseline heuristic. However, it is often not clear, which baseline heuristic to consider, i.e.: The same model architecture as the benchmark model, but without tuned hyperparameters? A simple, intrinsically explainable model with or without hyperparameter tuning? A random guess? A naive guess, in which the majority class is predicted? Since we have approaches on a user-level (i.e., we consider users when splitting) and on an assessment-level (i.e., we ignore users when splitting), we also should create baseline heuristics on both levels. We additionally account for within-user variance in Ecological Momentary Assessments by averaging a user’s previously known assessments. Previously known here means that we calculate the mode or median of all assessments of a user that are older than the given timestamp. In total, this leads to four baseline heuristics (user-level latest, user-level average, assessment-level latest, assessment-level average) that do not use any machine learning but simple heuristics. On the assessment-level, the latest known target or the mean of all known targets so far is taken to predict the next target, no matter of the user-id of this assessment. On the user-level, either the last known, or median, or mode value of this user is taken to predict the target. This, in turn, leads to a cold-start problem for users that appear for the first time in a dataset. In this case, either the last known, or mode, or median of all assessments that are known so far are taken to predict the target.
ML preprocessing
Before the data and approaches could be compared, it was necessary to homogenize them. In order for all approaches to work on all data sets, at least the following information is necessary: Assessment_id, user_id, timestamp, features, and the target. Any other information such as GPS data, or additional answers to questions of the assessment, we did not include into the ML pipeline. Additionally, targets that were collected on a continuous scale, had to be binned into an ordinal scale of five classes. For an easier interpretation and readability of the outputs, we also created label encodings for each target. To ensure consistency of the pre-processing, we created helper utilities within Python to ensure that the same function was applied on each dataset. For missing values, we created a user-wise missing value treatment. More precisely, if a user skipped a question in an assessment, we filled the missing value with the mean or mode ( mode = most common value) of all other answers of this user for this assessment. If a user had only one assessment, we filled it with the overall mean for this question.
For each dataset and for each script, we set random states and seeds to enhance reproducibility. For the outer validation set, we assigned the first 80 % of all users that signed up for a study to the train set, the latest 20% to the test set. To ensure comparability, the test users were the same for all approaches. We did not shuffle the users to simulate a deployment scenario where new users join the study. This would also add potential concept drift from the train to the test set and thus improve the simulation quality.
For the cross-validation within the training set, which we call internal validation, we chose a total of 5 folds with 1 validation fold. We then applied the four baseline heuristics (on user level and assessment level with either latest target or average target as prediction) to calculate the within-train-set performance standard deviation and the mean of the weighted F1 scores for each train fold. The mean and standard deviation of the weighted F1 score are then the estimator of the performance of our model in the test set.
We call one approach superior to another if the final score is higher. The final score to evaluate an approach is calculated as:
If the standard deviation between the folds during training is large, the final score is lower. The test set must not contain any selection bias against the underlying population. The pre-factor α of the standard deviation is another hyperparameter. The more important model robustness for the use case, the higher α should be set.
Existing train-test-split approaches
Within cross-validation, there exist several approaches on how to split up the data into folds and validate them, such as the k -fold approach with k as the number of folds in the training set. Here, k − 1 folds form the training folds and one fold is the validation fold 27 . One can then calculate k performance scores and their standard deviation to get an estimator for the performance of the model in the test set, which itself is an estimator for the model’s performance after deployment (see also Fig. 2 ).
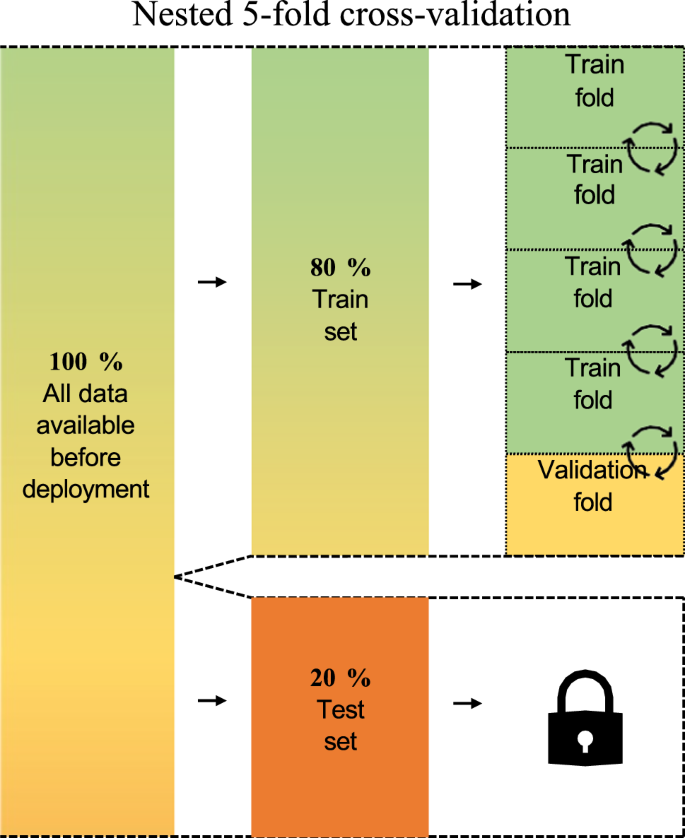
Schematic visualisation of the steps required to perform a k -fold cross-validation, here with k = 5.
In addition, there exist the following strategies: First, (repeated) stratified k -fold, in which the target distribution is retained in each fold, which can also be seen in Fig. 3 . After shuffling the samples, the stratified split can be repeated 3 . Second, leave- one -out cross-validation 28 , in which the validation fold contains only one sample while the model has been trained on all other samples. And third, leave- p -out cross-validation, in which \(\left(\begin{array}{c}n\\ p\end{array}\right)\) train-test-pairs are created with n equals number of assessments (synonym sample ) 29 .
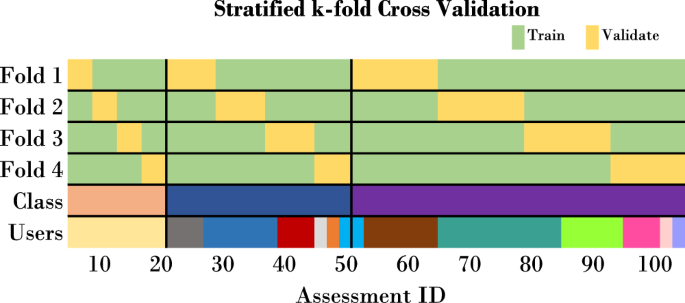
While this approach retains the class distribution in each fold, it still ignores user groups. Each color represents a different class or user id.
These approaches, however, do not always focus on samples that might belong to our mHealth data peculiarities. To be more specific, they do not account for users (syn. groups, subjects) that generate daily assessments (syn. samples) with a high variance.
Splitting approaches related to EMA
To precisely explain the splitting approaches, we would like to differentiate between the terms folds and sets . We call a chunk of samples (synonym: assessments, filled-out questionnaires) a set on the outer split of the data, for which we cut-off the final test set . However, within the training set, we then split further to create training and validation folds . That is, using the term fold , we are in the context of cross validation. When we use the term set , then we are in the outer split of the ML pipeline. Figure 4 visualizes this approach. Following this, we define 4 different approaches to split the data. For one of them we ignore the fact that there are users, for the other three we do not. We call these approaches user-cut, average-user, user-wise and time-cut . All approaches have in common that the first 80 % of all users are always in the training set and the remaining 20 % are in the test set. A schematic visualization of the splitting approaches is shown in Fig. 5 . Within the training set, we then split on user-level for the approaches user-cut, average-user and user-wise , and on assessment-level for the approach time-cut .
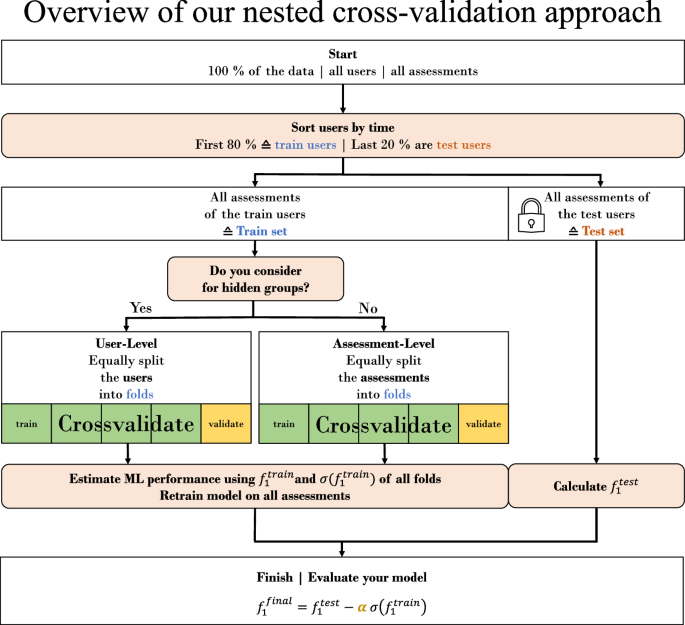
In the second step, users are ordered by their study registration time, with the initial 80 % designated as training users and the remaining 20 % as test users. Subsequently, assessments by training users are allocated to the training set, and those by test users to the test set. Within the training set, user grouping dictates the validation approach: group-cross-validation is applied if users are declared as a group, otherwise, standard cross-validation is utilized. We compute the average f 1 score, \({f}_{1}^{train}\) , from training folds and the f 1 score on the test set, \({f}_{1}^{test}\) . The standard deviation of \({f}_{1}^{train},\sigma ({f}_{1}^{train})\) , indicates model robustness. The hyperparameter α adjusts the emphasis on robustness, with higher α values prioritizing it. Ultimately, \({f}_{1}^{final}\) , which is a more precise estimate if group-cross-validation is applied, offers a refined measure of model performance in real-world scenarios.
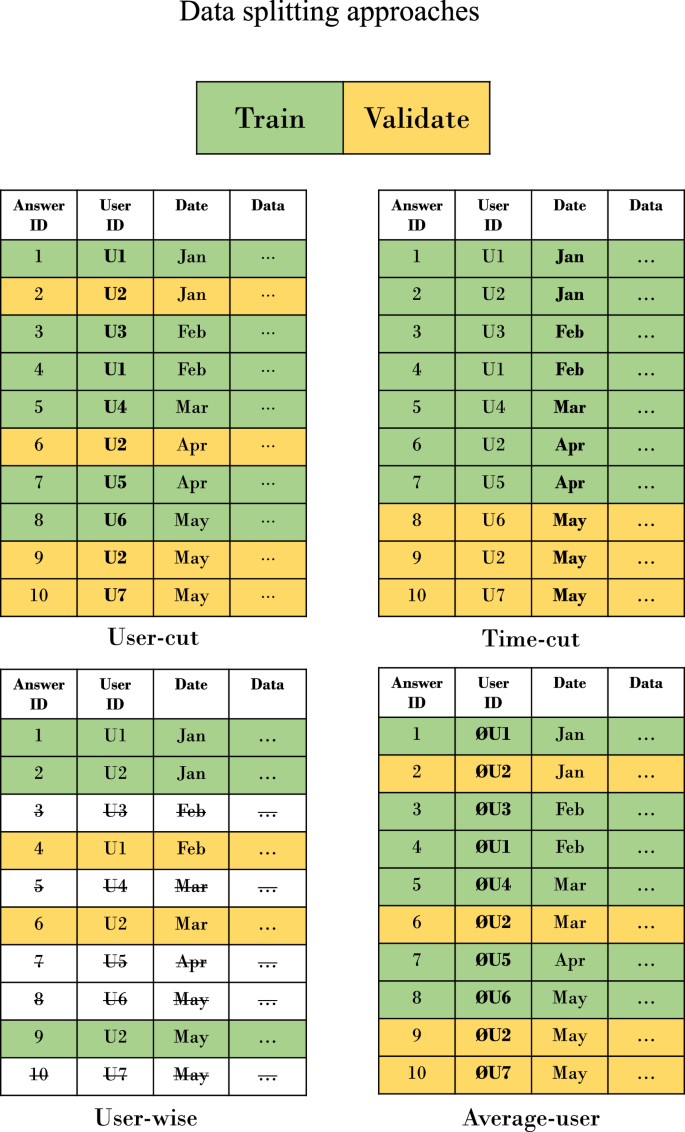
Yellow means that this sample is part of the validation fold, green means it is part of a training fold. Crossed out means that the sample has been dropped in that approach because it does not meet the requirements. Users can be sorted by time to accommodate any concept drift.
In the following section, we will explain the splitting approaches in more detail. The time-cut approach ignores the fact of given groups in the dataset and simply creates validation folds based on the time the assessments arrive in the database. In this example, the month, in which a sample was collected, is known. More precisely, all samples from January until April are in the training set while May is in the test set. The user-cut approach shuffles all user ids and creates five data folds with distinct user-groups. It ignores the time dimension of the data, but provides user-distinct training and validation folds, which is like the GroupKFold cross-validation approach as implemented in scikit-learn 30 . The average-user approach is very similar to the user-cut approach. However, each answer of a user is replaced by the median or mode answer of this user up to the point in question to reduce within-user-variance. While all the above-mentioned approaches require only one single model to be trained, the user-wise approach requires as many models as distinct users are given in the dataset. Therefore, for each user, 80 % of his or her assessments are used to train a user-specific model, and the remaining 20% of the time-sorted assessments are used to test the model. This means that for this approach, we can directly evaluate on the test set as each model is user specific and we solved the cold-start problem by training the model on the first assessments of this user. If a user has less than 10 assessments, he or she is not evaluated on that approach.
Approval for the UNITI randomized controlled trial and the UNITI app was obtained by the Ethics Committee of the University Clinic of Regensburg (ethical approval No. 20-1936-101). All users read and approved the informed consent before participating in the study. The study was carried out in accordance with relevant guidelines and regulations. The procedures used in this study adhere to the tenets of the Declaration of Helsinki. The Track Your Tinnitus (TYT) study was approved by the Ethics Committee of the University Clinic of Regensburg (ethical approval No. 15-101-0204). The Corona Check (CH) study was approved by the Ethics Committee of the University of Würzburg (ethical approval no. 71/20-me) and the university’s data protection officer and was carried out in accordance with the General Data Protection Regulations of the European Union. The procedures used in the Corona Health (CH) study were in accordance with the 1964 Helsinki declaration and its later amendments and was approved by the ethics committee of the University of Würzburg, Germany (No. 130/20-me). Ethical approvals include secondary use. The data from this study are available on request from the corresponding author. The data are not publicly available, as the informed consent of the participants did not provide for public publication of the data.
Reporting summary
Further information on research design is available in the Nature Portfolio Reporting Summary linked to this article.
We will see in this results section that ignoring users in training leads to an underestimation of the generalizability of the model, the standard deviation is then too small. To further explain, a model is ranked first in the comparison of all computations if it has the highest final score, and last if it has the lowest final score. We recall the formula of the final score from the methods section: \({f}_{1}^{final}={f}_{1}^{test}-0.5{\sigma }\left(\,{f}_{1}^{train}\right)\) . For these use cases, we set α = 0.5. The greater the emphasis on model robustness and the increased concerns regarding concept drift, the greater the alpha value should be set.
RQ1: What is the variance in performance when using different splitting methods for train and test set?
Considering performance aspects and ignoring the user groups in the data, the time cut approach has on average the best performance on assessment level. As an additional variant, we have sorted users once by time and once by random. When sorting by time, the baseline heuristic with the last known assessment of a user follows at rank 2, whereas with randomly sorted users, the user cut approach takes rank 2. The baseline heuristic with all known assessments on the user-level has the highest standard deviation in ranks, which means that this approach is highly dependent on the use case: For some datasets, it works better, for other it does not. The user-wise model approach has also a higher standard deviation in the ranking score, which means that the success of this approach is more use-case specific. As we set the threshold of users to be included into this approach to a minimum of 10 assessments, we have a high chance of a selection bias for the train-test split for users with only a few assessments, which could be a reason for the larger variance in performance. Details for the result are given in Table 3 .
Could there be a selection bias of users that are sorted and split by time? To answer this, we randomly draw 5 different user test sets for the whole pipeline and compared the approaches’ rankings with the variation where users were sorted by time. The approaches’ ranking changes by .44, which is less than one rank and can be calculated from Table 3 . This shows that there is no easily classifiable group of test users.
Cross-validation within the train helps to estimate the generalization error of the model for unseen data. On assessment-level, the standard deviations of the weighted F1 score within the train set for all datasets varies between 0.25 % for TrackYourTinnitus and 1.29 % for Corona Health Stress. On user-level, depending on the splitting approach, the standard deviation varies from 1.42 % to 4.69 %. However, on the test set, the estimator of the generalization error (i.e., the standard deviation of the F1 scores of the validation folds within the train set) is too low for all 7 datasets on assessment-level. On user-level, the estimator of the generalization error is too low for 4 out of 7 datasets. We define the estimator of the generalization error as in range if its smaller or equals the performance drop between validation and test set. Details for the result are given in Table 4 .
Both approaches, user- and assessment, overestimate the performance of the model during training. However, the quality of estimator of the generalization error increases if users are split on user-level.
RQ2: In which cases is the development, deployment and maintenance of a ML model compared to a simple baseline heuristic worthwhile?
For our 7 datasets, the baseline heuristics on a user-level perform better than those on assessment-level. For the datasets Corona Check (CC), Corona Health Stress (CH), TrackYourTinnitus (TYT) and UNITI , the last known user assessment is the best predictor within the baseline heuristics. For the psychological Corona Health study with adolescents (CHY) and adults (CHA), and physical health for adults (CHP), the average of the historic assessments is the best baseline predictor. The last known assessment on an assessment-level as a baseline heuristic performs worse for each dataset compared to the assessment level. The average of all so far known assessment as a predictor for the next assessment - independent from the user - has worst performance within the baseline heuristics for all datasets except CHA. Notably, the larger the number of assessments, the more the all-instances-approach on assessment-level converts to the mean of the target, which has high bias and minimum variance.
These results lead us to conclude that recognizing user groups in datasets leads to an improved baseline when trying to predict future ones from historical assessments. When these non-machine-learning baseline heuristics are then compared to machine learning models without hyperparameter tuning, it is found that they sometimes outperform or similarly outperform the machine learning model.
The approaches ranking in Table 5 shows the general overestimation of the performance of the time-cut approach as this approach is ranked best on average. It can be also seen that these approaches are ranked closely to each other. We chose α to be 0.5. Because we only subtract 0.5 (0.5 = α , our hyperparameter to control importance of model robustness) of the standard deviation of the f 1 scores of the validation folds, approaches with a higher standard deviation are less punished. This means, in turn, that the overestimation of the performance of the splits on assessment-level would be higher if α was higher. Another reason for the similarity of the approaches is that the same model architecture has been finally trained on all assessments of all train users to be evaluated on the test set. Thus, the only difference of the rankings results from the standard deviation of the f 1 scores of the validation folds.
To answer the question whether it is worthwhile to turn a prediction task into an ML project, further constraints should be considered. The above analysis shows that the baseline heuristics are competitive to the non-tuned random forest with much lower complexity. At the same time, the overall results are an f1 score between 55 and 65 for a multi-class classification with potential for improvement. Thus, the question should be additionally asked, from which f 1 score can be deployed, which depends on the use case, and in addition it is not clear whether the ML approach can be significantly improved by a different model or the right tuning.
The present work compared the performance of a tree-based ensemble method if the split of the data happens on two different levels: User and assessment. It further compared this performance to non-ML approaches that uses simple heuristics to also predict the target on a user- or assessment level. We quickly summarize the findings and then discuss them in more detail in the sections below. Neglecting user data during cross-validation may result in an inflated estimation of model performance and robustness, a phenomenon critical to the integrity of model evaluation. In specific scenarios, empirical evidence suggests that straightforward heuristic approaches can rival the efficacy of complex tree-based ensemble methodologies. Particularly, heuristics tailored or applied at the user level manifest a distinct advantage, while machine learning models maintain efficacy at the assessment level. Additionally, the methodological sorting of users in the dataset can serve as a proxy for concept drift in longitudinal studies, given a sufficiently extensive data collection period. This manipulation affects the test set outcomes, underscoring the influence of temporal user behavior variations on model validation.
The - still - small number of 7 use cases itself has a risk of selection bias in the data, features, or variables. This limits the generalizability of the statements. However, it is also arguable whether the trends found turn in a different direction when more use cases are included in the analysis. We do not believe that the tendencies would turn. We restricted the ML model to be a random forest classifier with a default hyperparameter set up to increase the degree of comparability between use cases. We are aware that each use case is different and direct comparability is not possible. Furthermore, we could have additionally evaluated the entire pipeline on other ML models that are not tree-based. However, this would have added another dimension to the comparison and further complicated the comparison of the results. Therefore, we cannot preclude that the results would have been substantially different for non-tree-based methods, which can be investigated further in future analyses.
Future research of this user-vs.-assessment-level comparison could include a hyperparameter tuning of the model on each use case, a change of model kind (i.e., from a random forest to a support vector machine) to see whether this changes the ranking. The overarching goal remains to obtain the most accurate estimate of the model’s performance after deployment.
We cannot give a final answer to what can be chosen as a common baseline heuristic. In machine learning projects, a majority vote is typically used for classification tasks, and a simple model such as a linear regression can be used for regression tasks. These approaches can also be called naive approaches since they often do not do justice to the complexity of the use case. Nevertheless, the power of a simple non-ML heuristic should not be underestimated. If only a few percentage points more performance can be achieved by the maintenance- and development-intensive ML approach, it is worth considering whether the application of a simple heuristic such as “the next assessment will be the same as the last one" is sufficient for a use case. Notably, Cawley and Talbot argue that it might be easier to build domain expert knowledge into hierarchical models, which could also function as a baseline heuristic 10 .
To retain consistency and reproducibility, we kept the users sorted by sign-up date to draw train and test users. The advantage of sorting the users is that one can simulate potential concept drift during training. The disadvantage, however, is an inherent risk of a selection bias towards users that signed up earlier for a study. From Figure 3 , we can see that the overfitting of users increases when we shuffle them. We conclude this from the fact that the difference between the average ranks of the approaches time cut and user cut increases. The advantage of shuffling users is that the splitting methods seem to depend less on the dataset. This can be deduced from the reduced standard deviation of the ranks compared to the sorted users.
Regardless of the level of splitting (user- or assessment-level), one can expect a performance drop if unknown users with unknown assessments are withheld from the model in the test set. When splitting at the user-level, the performance drop is lower during training and validation compared to the assessment-level. However, it remains questionable why we see this performance drop in the test set at all, because both, the validation folds and the test set contain unknown users with unknown assessments. A possible cause could be simple overfitting of the training data with the large random forest classifier and its 100 trees. But, also a single tree with max depth = number of features and balanced class weights has this performance drop from the validation to the test set. One explanation for the defiant performance drop could be that during cross validation information leaks from training folds to validation folds, but not to the test set.
A simple heuristic is not always trivial to beat by an ML model, depending on the use case and the complexity of the search space. Thinking of the complexity that a ML model adds to a project, a heuristic might be a valuable start to see how well the model fits into the workflow and improves the outcome. A frequent communication with the domain expert of the use case helps to set up a heuristic as a baseline heuristic. In a second step, it can be evaluated whether the performance gain from an ML model justifies the additional development effort.
Data availability
In relation to the individual data sets used (see Table 2 ), the availability is as follows: (1) TYT: The data presented in this study are available on request from the corresponding author. The data are not publicly available for data protection reasons. (2) UNITI, Corona Check, Corona Health: The investigators have access to the study data. Raw data (de-identified) can be made available on request from the corresponding author. Furthermore, only the mHealth data was used in this study on UNITI, but the entire UNITI RCT study contains even more data, which can be found here 20 .
Code availability
All code to replicate the results, models, numbers, figures, and tables is publicly available to anyone on https://github.com/joa24jm/UsAs 32 , DOI = 10.5281/zenodo.10401660.
Kunjan, S. et al. The necessity of leave one subject out (loso) cross validation for eeg disease diagnosis. In Brain Informatics: 14th International Conference, BI 2021, Virtual Event, September 17–19, 2021, Proceedings vol. 14, 558–567 (Springer, 2021).
Kohavi, R. A study of cross-validation and bootstrap for accuracy estimation and model selection. In Proceedings of the 14th International Joint Conference on Artificial Intelligence (IJCAI) , vol. 14, 1137–1145 (Montreal, Canada, 1995).
Dietterich, T. G. Approximate statistical tests for comparing supervised classification learning algorithms. Neural Comput 10 , 1895–1923 (1998).
Article CAS PubMed Google Scholar
Rudin, C. Stop explaining black box machine learning models for high stakes decisions and use interpretable models instead. Nat. Mach. Intell. 1 , 206–215 (2019).
Article PubMed PubMed Central Google Scholar
Chapman, P. et al. Crisp-dm 1.0: Step-by-step data mining guide. SPSS Inc 9 , 1–73 (2000).
Google Scholar
Beierle, F. et al. Corona health–a study-and sensor-based mobile app platform exploring aspects of the covid-19 pandemic. Int. J. Environ. Res. Public Health 18 , 7395 (2021).
Article CAS PubMed PubMed Central Google Scholar
Vogel, C., Schobel, J., Schlee, W., Engelke, M. & Pryss, R. Uniti mobile–emi-apps for a large-scale european study on tinnitus. In 2021 43rd Annual International Conference of the IEEE Engineering in Medicine & Biology Society (EMBC) , vol. 43, 2358–2362 (IEEE, 2021).
Kraft, R. et al. Combining mobile crowdsensing and ecological momentary assessments in the healthcare domain. Front. Neurosci. 14 , 164 (2020).
Schleicher, M. et al. Understanding adherence to the recording of ecological momentary assessments in the example of tinnitus monitoring. Sci. Rep. 10 , 22459 (2020).
Cawley, G. C. & Talbot, N. L. On over-fitting in model selection and subsequent selection bias in performance evaluation. J. Mach. Learn. Res. 11 , 2079–2107 (2010).
Refaeilzadeh, P., Tang, L. & Liu, H. Cross-validation. Encyclopedia Database Syst. 5 , 532–538 (2009).
Article Google Scholar
Schratz, P., Muenchow, J., Iturritxa, E., Richter, J. & Brenning, A. Hyperparameter tuning and performance assessment of statistical and machine-learning algorithms using spatial data. Ecolog. Model. 406 , 109–120 (2019).
Shao, J. Linear model selection by cross-validation. J. Am. Stat. Associat. 88 , 486–494 (1993).
Meyer, H., Reudenbach, C., Hengl, T., Katurji, M. & Nauss, T. Improving performance of spatio-temporal machine learning models using forward feature selection and target-oriented validation. Environ. Model. Software 101 , 1–9 (2018).
Allgaier, J., Schlee, W., Probst, T. & Pryss, R. Prediction of tinnitus perception based on daily life mhealth data using country origin and season. J. Clin. Med. 11 , 4270 (2022).
Shiffman, S., Stone, A. A. & Hufford, M. R. Ecological momentary assessment. Annu. Rev. Clin. Psychol. 4 , 1–32 (2008).
Article PubMed Google Scholar
Holfelder, M. et al. Medical device regulation efforts for mhealth apps during the covid-19 pandemic–an experience report of corona check and corona health. J 4 , 206–222 (2021).
Pryss, R., Reichert, M., Herrmann, J., Langguth, B. & Schlee, W. Mobile crowd sensing in clinical and psychological trials–a case study. In 2015 IEEE 28th international symposium on computer-based medical systems , 23–24 (IEEE, 2015).
Schlee, W. et al. Towards a unification of treatments and interventions for tinnitus patients: The eu research and innovation action uniti. Progress Brain Res. 260 , 441–451 (2021).
Simoes, J. P. et al. The statistical analysis plan for the unification of treatments and interventions for tinnitus patients randomized clinical trial (uniti-rct). Trials 24 , 472 (2023).
Allgaier, J., Schlee, W., Langguth, B., Probst, T. & Pryss, R. Predicting the gender of individuals with tinnitus based on daily life data of the trackyourtinnitus mhealth platform. Sci. Rep. 11 , 1–14 (2021).
Beierle, F. et al. Self-assessment of having covid-19 with the corona check mhealth app. IEEE J Biomed Health Inform. 27 , 2794–2805 (2023).
Humer, E. et al. Associations of country-specific and sociodemographic factors with self-reported covid-19–related symptoms: Multivariable analysis of data from the coronacheck mobile health platform. JMIR Public Health Surveil. 9 , e40958 (2023).
Wetzel, B. et al. "How come you don’t call me?” Smartphone communication app usage as an indicator of loneliness and social well-being across the adult lifespan during the COVID-19 pandemic. Int. Environ. Res. Public Health 18 , 6212 (2021).
Article CAS Google Scholar
Kroenke, K., Spitzer, R. L. & Williams, J. B. The phq-9: validity of a brief depression severity measure. J. General Internal Med. 16 , 606–613 (2001).
Cohen, S., Kamarck, T. & Mermelstein, R. et al. Perceived stress scale. Measur. Stress: Guider Health Social Scient. 10 , 1–2 (1994).
Stone, M. Cross-validatory choice and assessment of statistical predictions. J. Royal Stat. Society: Series B (Methodological) 36 , 111–133 (1974).
Lachenbruch, P. A. & Mickey, M. R. Estimation of error rates in discriminant analysis. Technometrics 10 , 1–11 (1968).
Geisser, S. The predictive sample reuse method with applications. J. Am. Stat. Associa. 70 , 320–328 (1975).
Pedregosa, F. et al. Scikit-learn: Machine learning in python. J. Mach. Learn. Res. 12 , 2825–2830 (2011).
Schlee, W. et al. Innovations in doctoral training and research on tinnitus: The european school on interdisciplinary tinnitus research (esit) perspective. Front. Aging Neurosci 9 , 447 (2018).
Allgaier, J. Github repository ∣ from hidden groups to robust models: How to better estimate performance of mobile health models. Zenodo https://doi.org/10.5281/zenodo.10401660 (2023).
Download references
Acknowledgements
This work was partly funded by the ESIT (European School for Interdisciplinary Tinnitus Research 31 ) project, which is financed by European Union’s Horizon 2020 research and innovation programme under the Marie Sklodowska-Curie grant agreement number 722046 and the UNITI (Unification of Treatments and Interventions for Tinnitus Patients) project financed by the European Union’s Horizon 2020 Research and Innovation Programme, Grant Agreement Number 848261 19 . J.A. and R.P. are supported by grants in the projects COMPASS and NAPKON. The COMPASS and NAPKON projects are part of the German COVID-19 Research Network of University Medicine ("Netzwerk Universitätsmedizin”), funded by the German Federal Ministry of Education and Research (funding reference 01KX2021). This publication was supported by the Open Access Publication Fund of the University of Wuerzburg.
Open Access funding enabled and organized by Projekt DEAL.
Author information
Authors and affiliations.
Institute of Clinical Epidemiology and Biometry, Julius-Maximilians-University Würzburg, Josef-Schneider-Straße 2, Würzburg, Germany
Johannes Allgaier & Rüdiger Pryss
You can also search for this author in PubMed Google Scholar
Contributions
J.A. primarily wrote this paper, created the figures, tables and plots, and trained the machine learning algorithms. R.P. supervised and revised the paper.
Corresponding author
Correspondence to Johannes Allgaier .
Ethics declarations
Competing interests.
The authors declare no competing interests.
Peer review
Peer review information.
Communications Medicine thanks Mostafa Rezapour, Koushik Howlader, and Wenyu Gao for their contribution to the peer review of this work. A peer review file is available.
Additional information
Publisher’s note Springer Nature remains neutral with regard to jurisdictional claims in published maps and institutional affiliations.
Supplementary information
Peer review file, reporting summary, rights and permissions.
Open Access This article is licensed under a Creative Commons Attribution 4.0 International License, which permits use, sharing, adaptation, distribution and reproduction in any medium or format, as long as you give appropriate credit to the original author(s) and the source, provide a link to the Creative Commons licence, and indicate if changes were made. The images or other third party material in this article are included in the article’s Creative Commons licence, unless indicated otherwise in a credit line to the material. If material is not included in the article’s Creative Commons licence and your intended use is not permitted by statutory regulation or exceeds the permitted use, you will need to obtain permission directly from the copyright holder. To view a copy of this licence, visit http://creativecommons.org/licenses/by/4.0/ .
Reprints and permissions
About this article
Cite this article.
Allgaier, J., Pryss, R. Practical approaches in evaluating validation and biases of machine learning applied to mobile health studies. Commun Med 4 , 76 (2024). https://doi.org/10.1038/s43856-024-00468-0
Download citation
Received : 21 March 2023
Accepted : 27 February 2024
Published : 22 April 2024
DOI : https://doi.org/10.1038/s43856-024-00468-0
Share this article
Anyone you share the following link with will be able to read this content:
Sorry, a shareable link is not currently available for this article.
Provided by the Springer Nature SharedIt content-sharing initiative
Quick links
- Explore articles by subject
- Guide to authors
- Editorial policies
Sign up for the Nature Briefing newsletter — what matters in science, free to your inbox daily.

Ask Yale Library
My Library Accounts
Find, Request, and Use
Help and Research Support
Visit and Study
Explore Collections
Research Data Management: Validate Data
- Plan for Data
- Organize & Document Data
- Store & Secure Data
- Validate Data
- Share & Re-use Data
- Data Use Agreements
- Research Data Policies
What is Data Validation?
Data validation is important for ensuring regular monitoring of your data and assuring all stakeholders that your data is of a high quality that reliably meets research integrity standards — and also a crucial aspect of Yale's Research Data and Materials Policy, which states "The University deems appropriate stewardship of research data as fundamental to both high-quality research and academic integrity and therefore seeks to attain the highest standards in the generation, management, retention, preservation, curation, and sharing of research data."
Data Validation Methods
Basic methods to ensure data quality — all researchers should follow these practices :
- Be consistent and follow other data management best practices, such as data organization and documentation
- Document any data inconsistencies you encounter
- Check all datasets for duplicates and errors
- Use data validation tools (such as those in Excel and other software) where possible
Advanced methods to ensure data quality — the following methods may be useful in more computationally-focused research :
- Establish processes to routinely inspect small subsets of your data
- Perform statistical validation using software and/or programming languages
- Use data validation applications at point of deposit in a data repository
Additional Resources for Data Validation
Data validation and quality assurance is often discipline-specific, and expectations and standards may vary. To learn more about data validation and data quality assurance, consider the information from the following U.S. government entities producing large amounts of public data:
- U.S. Census Bureau Information Quality Guidelines
- U.S. Geological Survey Data-Quality Management
- << Previous: Store & Secure Data
- Next: Share & Re-use Data >>
- Last Updated: Sep 27, 2023 1:15 PM
- URL: https://guides.library.yale.edu/datamanagement

Site Navigation
P.O. BOX 208240 New Haven, CT 06250-8240 (203) 432-1775
Yale's Libraries
Bass Library
Beinecke Rare Book and Manuscript Library
Classics Library
Cushing/Whitney Medical Library
Divinity Library
East Asia Library
Gilmore Music Library
Haas Family Arts Library
Lewis Walpole Library
Lillian Goldman Law Library
Marx Science and Social Science Library
Sterling Memorial Library
Yale Center for British Art
SUBSCRIBE TO OUR NEWSLETTER
@YALELIBRARY

Yale Library Instagram
Accessibility Diversity, Equity, and Inclusion Giving Privacy and Data Use Contact Our Web Team
© 2022 Yale University Library • All Rights Reserved
Data Validation for Machine Learning
Research areas.
Data Management
Machine Intelligence
Learn more about how we conduct our research
We maintain a portfolio of research projects, providing individuals and teams the freedom to emphasize specific types of work.
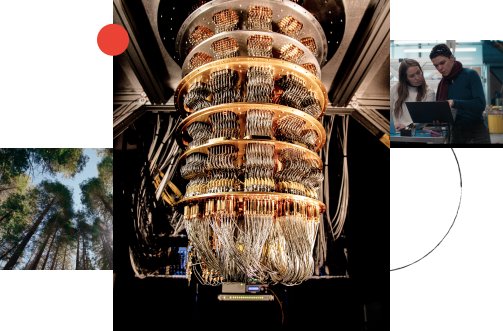
Validity in research: a guide to measuring the right things
Last updated
27 February 2023
Reviewed by
Cathy Heath
Short on time? Get an AI generated summary of this article instead
Validity is necessary for all types of studies ranging from market validation of a business or product idea to the effectiveness of medical trials and procedures. So, how can you determine whether your research is valid? This guide can help you understand what validity is, the types of validity in research, and the factors that affect research validity.

Make research less tedious
Dovetail streamlines research to help you uncover and share actionable insights
- What is validity?
In the most basic sense, validity is the quality of being based on truth or reason. Valid research strives to eliminate the effects of unrelated information and the circumstances under which evidence is collected.
Validity in research is the ability to conduct an accurate study with the right tools and conditions to yield acceptable and reliable data that can be reproduced. Researchers rely on carefully calibrated tools for precise measurements. However, collecting accurate information can be more of a challenge.
Studies must be conducted in environments that don't sway the results to achieve and maintain validity. They can be compromised by asking the wrong questions or relying on limited data.
Why is validity important in research?
Research is used to improve life for humans. Every product and discovery, from innovative medical breakthroughs to advanced new products, depends on accurate research to be dependable. Without it, the results couldn't be trusted, and products would likely fail. Businesses would lose money, and patients couldn't rely on medical treatments.
While wasting money on a lousy product is a concern, lack of validity paints a much grimmer picture in the medical field or producing automobiles and airplanes, for example. Whether you're launching an exciting new product or conducting scientific research, validity can determine success and failure.
- What is reliability?
Reliability is the ability of a method to yield consistency. If the same result can be consistently achieved by using the same method to measure something, the measurement method is said to be reliable. For example, a thermometer that shows the same temperatures each time in a controlled environment is reliable.
While high reliability is a part of measuring validity, it's only part of the puzzle. If the reliable thermometer hasn't been properly calibrated and reliably measures temperatures two degrees too high, it doesn't provide a valid (accurate) measure of temperature.
Similarly, if a researcher uses a thermometer to measure weight, the results won't be accurate because it's the wrong tool for the job.
- How are reliability and validity assessed?
While measuring reliability is a part of measuring validity, there are distinct ways to assess both measurements for accuracy.
How is reliability measured?
These measures of consistency and stability help assess reliability, including:
Consistency and stability of the same measure when repeated multiple times and conditions
Consistency and stability of the measure across different test subjects
Consistency and stability of results from different parts of a test designed to measure the same thing
How is validity measured?
Since validity refers to how accurately a method measures what it is intended to measure, it can be difficult to assess the accuracy. Validity can be estimated by comparing research results to other relevant data or theories.
The adherence of a measure to existing knowledge of how the concept is measured
The ability to cover all aspects of the concept being measured
The relation of the result in comparison with other valid measures of the same concept
- What are the types of validity in a research design?
Research validity is broadly gathered into two groups: internal and external. Yet, this grouping doesn't clearly define the different types of validity. Research validity can be divided into seven distinct groups.
Face validity : A test that appears valid simply because of the appropriateness or relativity of the testing method, included information, or tools used.
Content validity : The determination that the measure used in research covers the full domain of the content.
Construct validity : The assessment of the suitability of the measurement tool to measure the activity being studied.
Internal validity : The assessment of how your research environment affects measurement results. This is where other factors can’t explain the extent of an observed cause-and-effect response.
External validity : The extent to which the study will be accurate beyond the sample and the level to which it can be generalized in other settings, populations, and measures.
Statistical conclusion validity: The determination of whether a relationship exists between procedures and outcomes (appropriate sampling and measuring procedures along with appropriate statistical tests).
Criterion-related validity : A measurement of the quality of your testing methods against a criterion measure (like a “gold standard” test) that is measured at the same time.
- Examples of validity
Like different types of research and the various ways to measure validity, examples of validity can vary widely. These include:
A questionnaire may be considered valid because each question addresses specific and relevant aspects of the study subject.
In a brand assessment study, researchers can use comparison testing to verify the results of an initial study. For example, the results from a focus group response about brand perception are considered more valid when the results match that of a questionnaire answered by current and potential customers.
A test to measure a class of students' understanding of the English language contains reading, writing, listening, and speaking components to cover the full scope of how language is used.
- Factors that affect research validity
Certain factors can affect research validity in both positive and negative ways. By understanding the factors that improve validity and those that threaten it, you can enhance the validity of your study. These include:
Random selection of participants vs. the selection of participants that are representative of your study criteria
Blinding with interventions the participants are unaware of (like the use of placebos)
Manipulating the experiment by inserting a variable that will change the results
Randomly assigning participants to treatment and control groups to avoid bias
Following specific procedures during the study to avoid unintended effects
Conducting a study in the field instead of a laboratory for more accurate results
Replicating the study with different factors or settings to compare results
Using statistical methods to adjust for inconclusive data
What are the common validity threats in research, and how can their effects be minimized or nullified?
Research validity can be difficult to achieve because of internal and external threats that produce inaccurate results. These factors can jeopardize validity.
History: Events that occur between an early and later measurement
Maturation: The passage of time in a study can include data on actions that would have naturally occurred outside of the settings of the study
Repeated testing: The outcome of repeated tests can change the outcome of followed tests
Selection of subjects: Unconscious bias which can result in the selection of uniform comparison groups
Statistical regression: Choosing subjects based on extremes doesn't yield an accurate outcome for the majority of individuals
Attrition: When the sample group is diminished significantly during the course of the study
Maturation: When subjects mature during the study, and natural maturation is awarded to the effects of the study
While some validity threats can be minimized or wholly nullified, removing all threats from a study is impossible. For example, random selection can remove unconscious bias and statistical regression.
Researchers can even hope to avoid attrition by using smaller study groups. Yet, smaller study groups could potentially affect the research in other ways. The best practice for researchers to prevent validity threats is through careful environmental planning and t reliable data-gathering methods.
- How to ensure validity in your research
Researchers should be mindful of the importance of validity in the early planning stages of any study to avoid inaccurate results. Researchers must take the time to consider tools and methods as well as how the testing environment matches closely with the natural environment in which results will be used.
The following steps can be used to ensure validity in research:
Choose appropriate methods of measurement
Use appropriate sampling to choose test subjects
Create an accurate testing environment
How do you maintain validity in research?
Accurate research is usually conducted over a period of time with different test subjects. To maintain validity across an entire study, you must take specific steps to ensure that gathered data has the same levels of accuracy.
Consistency is crucial for maintaining validity in research. When researchers apply methods consistently and standardize the circumstances under which data is collected, validity can be maintained across the entire study.
Is there a need for validation of the research instrument before its implementation?
An essential part of validity is choosing the right research instrument or method for accurate results. Consider the thermometer that is reliable but still produces inaccurate results. You're unlikely to achieve research validity without activities like calibration, content, and construct validity.
- Understanding research validity for more accurate results
Without validity, research can't provide the accuracy necessary to deliver a useful study. By getting a clear understanding of validity in research, you can take steps to improve your research skills and achieve more accurate results.
Should you be using a customer insights hub?
Do you want to discover previous research faster?
Do you share your research findings with others?
Do you analyze research data?
Start for free today, add your research, and get to key insights faster
Editor’s picks
Last updated: 18 April 2023
Last updated: 27 February 2023
Last updated: 6 February 2023
Last updated: 6 October 2023
Last updated: 5 February 2023
Last updated: 16 April 2023
Last updated: 9 March 2023
Last updated: 12 December 2023
Last updated: 11 March 2024
Last updated: 4 July 2024
Last updated: 6 March 2024
Last updated: 5 March 2024
Last updated: 13 May 2024
Latest articles
Related topics, .css-je19u9{-webkit-align-items:flex-end;-webkit-box-align:flex-end;-ms-flex-align:flex-end;align-items:flex-end;display:-webkit-box;display:-webkit-flex;display:-ms-flexbox;display:flex;-webkit-flex-direction:row;-ms-flex-direction:row;flex-direction:row;-webkit-box-flex-wrap:wrap;-webkit-flex-wrap:wrap;-ms-flex-wrap:wrap;flex-wrap:wrap;-webkit-box-pack:center;-ms-flex-pack:center;-webkit-justify-content:center;justify-content:center;row-gap:0;text-align:center;max-width:671px;}@media (max-width: 1079px){.css-je19u9{max-width:400px;}.css-je19u9>span{white-space:pre;}}@media (max-width: 799px){.css-je19u9{max-width:400px;}.css-je19u9>span{white-space:pre;}} decide what to .css-1kiodld{max-height:56px;display:-webkit-box;display:-webkit-flex;display:-ms-flexbox;display:flex;-webkit-align-items:center;-webkit-box-align:center;-ms-flex-align:center;align-items:center;}@media (max-width: 1079px){.css-1kiodld{display:none;}} build next, decide what to build next.

Users report unexpectedly high data usage, especially during streaming sessions.

Users find it hard to navigate from the home page to relevant playlists in the app.

It would be great to have a sleep timer feature, especially for bedtime listening.

I need better filters to find the songs or artists I’m looking for.
Log in or sign up
Get started for free
Advertisement
The Role of Academic Validation in Developing Mattering and Academic Success
- Published: 24 March 2022
- Volume 63 , pages 1368–1393, ( 2022 )
Cite this article
- Elise Swanson ORCID: orcid.org/0000-0002-4529-9646 1 &
- Darnell Cole 2
1535 Accesses
2 Citations
2 Altmetric
Explore all metrics
We use survey data from three four-year campuses to explore the relationship between academic validation and student outcomes during students’ first 3 years in college using structural equation modeling. We examine both a psychosocial outcome (mattering to campus) and an academic outcome (cumulative GPA). We find that both frequency of interactions with faculty and feelings of academic validation from faculty are positively related to students’ feelings of mattering to campus and cumulative GPA in their third year. Our results suggest that academic validation, beyond the frequency of faculty–student interactions, is an important predictor of students’ psychosocial and academic success.
This is a preview of subscription content, log in via an institution to check access.
Access this article
Subscribe and save.
- Get 10 units per month
- Download Article/Chapter or eBook
- 1 Unit = 1 Article or 1 Chapter
- Cancel anytime
Price includes VAT (Russian Federation)
Instant access to the full article PDF.
Rent this article via DeepDyve
Institutional subscriptions
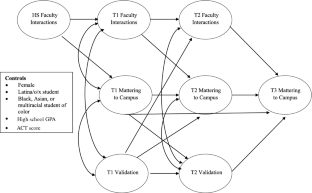
Similar content being viewed by others
Developing a student conception of academic rigor.
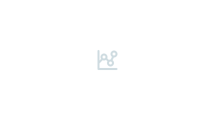
From Policies to Principles: The Effects of Campus Climate on Academic Integrity, a Mixed Methods Study
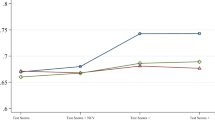
The Role of Non-cognitive Variables in Identifying Community College Students in Need of Targeted Supports
Data availability.
The data used for this analysis are restricted-used and under the purview of the Promoting At-promise Student Success project. Interested researchers may apply to access the data. The survey used was for this research was compiled by researchers at the Pullias Center for Higher Education. Certain scales on the survey were used with permission from other research organizations; the survey instrument used for this study may not be used without appropriate permissions for all scales on the survey.
Code Availability
All analyses were conducted in Stata; code is available from the authors upon request.
A concern with this modeling decision is that our estimates of the relationships between validation and faculty interactions, respectively, and third-year GPA may include the indirect relationship between prior (e.g., T1) validation and faculty as well as the direct relationship between the T2 measurements and third-year GPA. When we include students’ high school, first semester, first year, second year, and third year GPA, we find no significant relationship between students’ first-year faculty interactions and second-year GPA and a small, marginally significant relationship between first-year validation and second-year GPA, mitigating this concern. We also estimate the model including lagged direct paths between first-year validation and faculty interactions and third-year GPA; we find similar results to those presented below affirming the importance of second-year validation for predicting third-year GPA, again mitigating concerns of bias in our main estimates. However, a conservative interpretation of our results is as the cumulative relationship between second-year student-initiated interactions with faculty and feelings of academic validation with GPA. Goodness-of-fit measures are similar across specifications.
Angrist, J., Autor, D., Hudson, S., & Pallais, A. (2016). Evaluating post-secondary aid: Enrollment, persistence, and projected completion effects. NBER Working Paper 23015. National Bureau of Economic Research. https://www.nber.org/papers/w23015
Astin, A. W. (1984). Student involvement: A developmental theory for higher education. Journal of College Student Personnel, 25 (4), 297–308.
Google Scholar
Astin, A. (1985). Achieving educational excellence: a critical assessment of priorities and practices in higher education . Jossey-Bass.
Astin, A. W. (1993). What matters in college: Four critical years revisited . Jossey-Bass.
Aud, S., Fox, M., & KewalRamani, A. (2010). Status and trends in the education of racial and ethnic groups (NCES 2010-015). U.S. Department of Education, National Center for Education Statistics. U.S. Government Printing Office.
Auerbach, R., Alonso, J., Axinn, W., Cuijpers, P., Ebert, D., Green, J., Hwang, I., Kessler, R., Liu, H., Mortier, P., Nock, M., Pinder-Amaker, S., Sampson, N., Aguilar-Gaxiola, S., Al-Hamzawi, A., Andrade, L., Benjet, C., Caldas-de-Almeida, J., Demyttenaere, K., Florescu, S., … Buffaerts, R. (2016). Mental disorders among college students in the World Health Organization World Mental Health Surveys. Psychological Medicine, 46 (2016), 2955–2970. https://doi.org/10.1017/S0033291716001665
Barnett, E. (2006). Validation experiences and persistence among urban community college students. Dissertation Abstracts International, 68 (02) A. (UMI No. 3250210).
Barnett, E. (2011). Validation experiences and persistence among community college students. The Review of Higher Education, 34 (2), 193–230. https://doi.org/10.1353/rhe.2010.0019
Article Google Scholar
Bean, J. P., & Kuh, G. D. (1984). The relationship between student–faculty interaction and undergraduate grade point average. Research in Higher Education, 21 , 461–477.
Belenky, M. F., Clinchy, B. M., Goldberger, N. R., & Tarule, J. M. (1986). Women’s ways of knowing: The development of self, voice, and mind . Basic Books.
Bettinger, T., & Long, B. (2005). Do faculty serve as role models? The impact of instructor gender on female students. American Economic Association, 95 (2), 152–157.
Brown McNair, T., Albertine, S., Cooper, M. A., McDonald, N., & Major, T. (2016). Becoming a student-ready college: A new culture of leadership for student success . Jossey-Bass.
Brownback, A., & Sadoff, S. (2019). Improving college instruction through incentives. UC San Diego Working Paper. Retrieved from https://rady.ucsd.edu/docs/Brownback_Sadoff_College_Instructor_Incentives_20190501.pdf
Cole, D., & Griffin, K. A. (2013). Advancing the study of student-faculty interaction: A focus on diverse students and faculty. In Higher education: Handbook of theory and research (pp. 561–611). Springer.
Cole, D., Kitchen, J., & Kezar, A. (2018). Examining a comprehensive college transition program: An account of an iterative mixed methods longitudinal design. Research in Higher Education, 60 , 392–413. https://doi.org/10.1007/s11162-018-9515-1
Dee, T. (2005). A teacher like me: Does race, ethnicity, or gender matter? American Economic Review, 95 (2), 158–165.
Dixon Rayle, A., & Chung, K. (2007). Revisiting first year college students’ mattering: Social support, academic stress, and the mattering experience. Journal of College Student Retention, 9 (1), 21–37.
Dykes, M. (2011). Appalachian bridges to the baccalaureate: Mattering perceptions and transfer persistence of low-income, first-generation community college students (Doctoral Dissertation). Retrieved from University of Kentucky Doctoral Dissertations (844). https://uknowledge.uky.edu/gradschool_diss/844
Eagen, K., & Jaeger, A. (2009). Effects of exposure to part-time faculty on community college transfer. Research in Higher Education, 50 (2), 168–188.
Egalite, A., Kisida, B., & Winters, M. (2015). Representation in the classroom: The effect of own-race teachers on student achievement. Economics of Education Review, 45 (2015), 44–52.
Eisenberg, D., Hunt, J., Speer, N., & Zivin, K. (2011). Mental health service utilization among college students in the United States. The Journal of Nervous and Mental Disease, 199 (5), 301–308. https://doi.org/10.1097/NMD.0b013e3182175123
Gershenfeld, S., Hood, D., & Zhan, M. (2015). The role of first-semester GPA in predicting graduation rates of underrepresented students. Journal of College Student Retention: Research, Theory & Practice, 17 (4), 469–488.
Gershenson, S., Holt, S., & Papageorge, N. (2016). Who believes in me? The effect of student-teacher demographic match on teacher expectations. Economics of Education Review, 52 (2016), 209–224.
Gildersleeve, R. (2011). Toward a neo-critical validation theory: participatory action research and Mexican migrant student success. Enrollment Management Journal, 5 (2), 72–96.
Hallett, R., Bettencourt, G. M., Kezar, A., Kitchen, J. A., Perez, R., & Reason, R. (2021). Re-envisioning campuses to holistically support students: The ecological validation model of student success [Brief]. USC Pullias Center for Higher Education.
Hallett, R. E., Kezar, A., Perez, R. J., & Kitchen, J. A. (2019). A typology of college transition and support programs: Situating a 2-year comprehensive college transition program within college access. American Behavioral Scientist, 64 (3), 230–252.
Hallett, R. E., Reason, R. D., Toccoli, J., Kitchen, J. A., & Perez, R. J. (2019). The process of academic validation within a comprehensive college transition program. American Behavioral Scientist, 64 (3), 253–275.
Hart, T. (2017). The relationship between online students’ use of services and their feelings of mattering (Doctoral Dissertation). Retrieved from The University of New Mexico Digital Repository. https://digitalrepository.unm.edu/oils_etds/43
Hu, L., & Bentler, P. (1999). Cutoff criteria for fit indexes in covariance structure analysis: Conventional criteria versus new alternatives. Structural Equation Modeling: A Multidisciplinary Journal, 6 (1), 1–55.
Hunt, J., & Eisenberg, D. (2010). Mental health problems and help-seeking behavior among college students. Journal of Adolescent Health, 46 (1), 3–10. https://doi.org/10.1016/j.jadohealth.2009.08.008
Hurtado, S., Alvarado, A. R., & Guillermo-Wann, C. (2015). Creating inclusive environments: The mediating effect of faculty and staff validation on the relationship of discrimination/bias to students’ sense of belonging. JCSCORE, 1 (1), 59–81.
Hurtado, S., Cuellar, M., & Guillermo-Wann, C. (2011). Quantitative measures of students’ sense of validation: Advancing the study of diverse learning environments. Enrollment Management Journal, 5 (2), 53–71.
Jaschik, S. (March 2016). Grade inflation, higher and higher. Inside Higher Ed . https://www.insidehighered.com/news/2016/03/29/survey-finds-grade-inflationcontinues-rise-four-year-colleges-not-communitycollege#:~:text=Grade%20point%20averages%20at%20four,than%2042%20percent%20of%20grades
Ketchen Lipson, S., Kern, A., Eisenberg, D., & Breland-Noble, A. (2018). Mental health disparities among students of color. Journal of Adolescent Health, 63 (2018), 348–356. https://doi.org/10.1016/j.jadohealth.2018.04.014
Kitchen, J. A., Perez, R., Hallett, R., Kezar, A., & Reason, R. (2020, November 21). Ecology of validation: A new student support model for promoting college success among lowincome, first-generation, and racially minoritized students [Conference presentation]. Association for the Study of Higher Education. https://www.ashe.ws//Files/Past%20Conferences/ASHE%202020%20Program%20Book.pdf
Klasik, D. (2012). The college application gauntlet: A systematic analysis of the steps to four-year college enrollment. Research in Higher Education, 53 (5), 506–549.
Kline, R. (2015). Principles and practice of structural equation modeling (4th ed.). Guilford Press.
Kuh, G. D., Schuh, J. H., Whitt, E. J., & Associates (1991). Involving colleges: Successful approaches to fostering student learning and development outside the classroom . Jossey-Bass.
Kuh, G. D., & Hu, S. (2001). The effects of student-faculty interaction in the 1990s. The Review of Higher Education, 24 (3), 309–332.
Linares, L. I. R., & Muñoz, S. M. (2011). Revisiting validation theory: Theoretical foundations, applications, and extensions. Enrollment Management Journal, 2 (1), 12–33.
Lindsay, C., & Hart, C. (2017). Teacher race and school discipline: Are students suspended less often when they have a teacher of the same race? Education next, 17 (1), 72–78.
Lozano, A. (2010). Culture centers: Providing a sense of belonging and promoting student success. In L. D. Patton (Ed.), Culture centers in higher education: Perspectives on identity, theory, and practice. Stylus.
Mayhew, M., Rockenbach, A., Bowman, N., Seifert, T., Wolniak, G., Pascarella, E., & Terenzini, P. (2016). How college affects students Volume 3: 21st century evidence that higher education works . Jossey-Bass.
Melguizo, T., Martorell, P., Swanson, E., Chi, E., Park, E., & Kezar, A. (2021). Expanding student success: The impact of a comprehensive college transition program on psychosocial outcomes. Journal of Research on Educational Effectiveness, 14 (4), 835–860.
Nora, A., Urick, A., & Cerecer, P. D. Q. (2011). Validating students: A conceptualization and overview of its impact on student experiences and outcomes. Enrollment Management Journal, 5 (2), 34–52.
O’Shea, S., & Delahunty, J. (2018). Getting through the day and still having a smile on my face! How do students define success in the university learning environment? Higher Education Research & Development, 37 (5), 1062–1075.
Pascarella, E., & Terenzini, P. (1991). How college affects students: Findings and insights from twenty years of research . Jossey-Bass.
Pattison, E., Grodsky, E., & Muller, C. (2013). Is the sky falling? Grade inflation and the signaling power of grades. Educational Researcher, 42 (5), 259–265. https://doi.org/10.3102/0013189X13481382
Perez, R. J., Acuña, A., & Reason, R. D. (2021). Pedagogy of validation: Autobiographical reading and writing courses for first year, low-income students. Innovative Higher Education . https://doi.org/10.1007/s10755-021-09555-9
Quaye, S. J., & Chang, S. H. (2012). Fostering cultures of inclusion in the classroom: From marginality to mattering. In S. D. Museus & U. M. Jayakumar (Eds.), Creating campus cultures: Fostering success among racially diverse student populations. Routledge.
Rendon, L. (1994). Validating culturally diverse students: Toward a new model of learning and student development. Innovative Higher Education, 19 (1), 33–51.
Rendon, L. (2002). Community College Puente: A validating model of education. Educational Policy, 16 (4), 642–667.
Rendon, L., & Munoz, S. M. (2011). Revisiting validation theory: Theoretical foundations, applications, and extensions. Enrollment Management Journal, 5 (2), 12–33.
Robinson-Cimpian, J. P., Lubienski, S. T., Ganley, C. M., & Copur-Gencturk, Y. (2014). Teachers’ perceptions of students’ mathematics proficiency may exacerbate early gender gaps in achievement. Developmental Psychology, 50 (4), 1262–1281.
Rodriquez, R. (1975). Coming home again: The new American scholarship boy. American Scholar, 44 (1), 15–28.
Rosenberg, M., & McCullough, B. C. (1981). Mattering: Inferred significance and mental health among adolescents. Research in Community and Mental Health, 2 , 163–182.
Saggio, J., & Rendòn, L. (2004). Persistence among American Indians and Alaska Natives at a bible college: The importance of family, spirituality, and validation. Christian Higher Education, 3 (3), 223–240.
Schlossberg, N. K. (1989). Marginality and mattering: Key issues in building community. New Directions for Student Services, 1989 (48), 5–15. https://doi.org/10.1002/ss.37119894803
Stanton-Salazar, R. D. (2011). A social capital framework for the study of institutional agents and their role in the empowerment of low-status students and youth. Youth & Society, 43 (3), 1066–1109.
Tinto, V. (1993). Leaving college: Rethinking the causes and cures of student attrition (2nd ed.). University of Chicago Press.
Vasquez, M., Gonzalez, A., Cataño, Y., & Garcia, F. (2021). Exploring the role of women as validating agents for Latino men in their transfer success. Community College Journal of Research and Practice . https://doi.org/10.1080/10668926.2021.1873874
Zhang, Y. (2016). An overlooked population in community college: International students’ (in)validation experiences with academic advising. Community College Review, 44 (2), 153–170.
Download references
This project received support from the Susan Thompson Buffett Foundation.
Author information
Authors and affiliations.
Harvard University, 50 Church St, Fourth Floor, Cambridge, MA, 02138, USA
Elise Swanson
University of Southern California, Los Angeles, USA
Darnell Cole
You can also search for this author in PubMed Google Scholar
Corresponding author
Correspondence to Elise Swanson .
Ethics declarations
Conflict of interest.
The authors have no conflicts of interest.
Additional information
Publisher's note.
Springer Nature remains neutral with regard to jurisdictional claims in published maps and institutional affiliations.
We would like to thank Adrianna Kezar, Tatiana Melguizo, Ronald Hallett, Gwendelyn Rivera, KC Culver, Joseph Kitchen, Rosemary Perez, Robert Reason, Matt Soldner, Mark Masterton, Evan Nielsen, Cameron McPhee, Samantha Nieman, and all the other members of the broader mixed-methods evaluation team for designing and implementing the Longitudinal Survey of Thompson Scholars, for helping us get a better understanding of the program and providing feedback on previous versions of this manuscript. We would also like to thank Gregory Hancock for his assistance with the structural equation modeling. Finally, we would also like to thank the staff at the Thompson Scholars Learning Communities for their reflections and continued work to support at-promise students. This study received financial support from the Susan Thompson Buffett Foundation. Opinions are those of the authors alone and do not necessarily reflect those of the granting agency or of the authors’ home institutions.
See Tables 5 , 6 , and 7 .
Rights and permissions
Reprints and permissions
About this article
Swanson, E., Cole, D. The Role of Academic Validation in Developing Mattering and Academic Success. Res High Educ 63 , 1368–1393 (2022). https://doi.org/10.1007/s11162-022-09686-8
Download citation
Received : 03 March 2021
Accepted : 08 March 2022
Published : 24 March 2022
Issue Date : December 2022
DOI : https://doi.org/10.1007/s11162-022-09686-8
Share this article
Anyone you share the following link with will be able to read this content:
Sorry, a shareable link is not currently available for this article.
Provided by the Springer Nature SharedIt content-sharing initiative
- Academic achievement
- Longitudinal analysis
- Structural equation modeling
- Find a journal
- Publish with us
- Track your research
- Open Source Software
- Business Software
- SourceForge Podcast
- Site Documentation
- Subscribe to our Newsletter
- Support Request
Learn Key Reasons Why Data Validation is Essential in the Research Process

Key Reasons Why Data Validation is Essential in the Research Process
In the ever-evolving world of scientific inquiry and data-driven decision-making, the integrity of research findings hinges on the robustness of the data upon which they are based. Data validation, a critical yet often overlooked aspect of the research process, ensures that the data collected and analyzed can truly support the conclusions drawn. This article explores the vital importance of data validation, providing insights into why it is indispensable for accuracy, reliability, credibility, and much more.
Introduction to Data Validation in Research
Data validation refers to the process of ensuring that the data used for research are accurate, complete, and reliable. It involves various checks and balances to detect and correct errors and inconsistencies in the data. The significance of data validation extends beyond mere error correction; it is foundational to maintaining the integrity and credibility of the research itself. Without rigorous validation, the conclusions of any study may be fundamentally flawed, leading to misguided decisions and tarnished reputations.
Why Data Validation is Essential
Enhancing accuracy.
At its core, data validation serves to enhance the accuracy of research findings. Errors in data can arise from numerous sources, including manual data entry , faulty data collection instruments, and misinterpretation of data due to cultural or linguistic barriers. By implementing stringent validation protocols, researchers can identify and rectify these errors before they impact the analysis. For instance, in epidemiological research, accurate data on disease incidence rates are crucial. Simple validation methods like range checks (ensuring values fall within a plausible range) and consistency checks (ensuring data across variables make sense together) can prevent misdiagnoses and incorrect reporting of disease prevalence.
Ensuring Reliability and Credibility
Data that has been thoroughly validated not only supports the reliability of the research findings but also bolsters the credibility of the study among peers and the broader community. In academic publishing, for example, the reproducibility of results is a cornerstone of scientific integrity. Validated data assures readers and reviewers that the findings are based on solid ground, fostering trust and facilitating further scholarly engagement.
Facilitating Reproducibility
A study’s reproducibility is directly tied to the rigor of its data validation process. When data is properly validated, other researchers can replicate the study under similar conditions and expect to reach similar conclusions. This reproducibility is essential for the advancement of science, as it allows subsequent researchers to build on existing knowledge confidently. Techniques such as cross-validation, where data is divided into subsets to test and validate the model’s assumptions, play a critical role in ensuring that the findings are not just a result of peculiarities in the data.
Supporting Decision Making
In fields ranging from healthcare to public policy, the decisions made based on research findings can have profound impacts. Validated data ensures that these decisions are based on the most accurate information available, thereby minimizing risks and enhancing the effectiveness of interventions. For instance, data-driven policy making in public health relies heavily on validated data to strategize interventions during a health crisis such as a pandemic.
Compliance with Ethical and Legal Standards
Data validation is also crucial in meeting ethical and legal standards, especially in research involving human subjects. Ensuring data accuracy and integrity helps protect participants’ privacy and rights, as inaccurate data can lead to wrongful conclusions that might affect their lives. Moreover, in many fields, regulatory frameworks require rigorous data validation to ensure compliance with legal standards and avoid penalties.
Improving Data Integration
In today’s interdisciplinary and collaborative research environment, projects often involve integrating data from multiple sources. Validation is key to ensuring that this integrated data is consistent and compatible. For example, combining patient data from different hospitals without proper validation can lead to errors in medical research and treatment outcomes.
Practical Aspects of Data Validation
Data validation employs a variety of methods, from simple manual checks to complex automated systems. Tools such as SQL for database management, Python for scripting automated checks, and specialized software for statistical analysis (like R or SAS) are commonly used. Choosing the right tools and methods depends largely on the data’s nature and the specific requirements of the research project.
Researchers often face significant challenges in data validation, including dealing with large datasets or high-dimensional data. Techniques such as parallel processing for big data or employing machine learning algorithms for anomaly detection can help address these issues effectively.
The meticulous process of data validation is a cornerstone of scientific rigor. It is a critical step that should never be bypassed in the rush to achieve results. By ensuring that data validation is integrated into the research process, researchers can uphold the highest standards of accuracy, reliability, and integrity in their work.
Posted in General | Comments disabled
Comments are closed.

An official website of the United States government
The .gov means it’s official. Federal government websites often end in .gov or .mil. Before sharing sensitive information, make sure you’re on a federal government site.
The site is secure. The https:// ensures that you are connecting to the official website and that any information you provide is encrypted and transmitted securely.
- Publications
- Account settings
Preview improvements coming to the PMC website in October 2024. Learn More or Try it out now .
- Advanced Search
- Journal List
- Saudi J Anaesth
- v.11(Suppl 1); 2017 May
Guidelines for developing, translating, and validating a questionnaire in perioperative and pain medicine
Department of Epidemiology, Columbia University, New York, NY, USA
Colin F. Royse
1 Department of Surgery, University of Melbourne, Melbourne, Victoria, Australia
2 Department of Anesthesia and Pain Management, The Royal Melbourne Hospital, Parkville, Victoria, Australia
Abdullah Sulieman Terkawi
3 Department of Anesthesiology, University of Virginia, Charlottesville, VA, USA
4 Department of Anesthesiology, King Fahad Medical City, Riyadh, Saudi Arabia
5 Outcomes Research Consortium, Cleveland, OH, USA, USA
The task of developing a new questionnaire or translating an existing questionnaire into a different language might be overwhelming. The greatest challenge perhaps is to come up with a questionnaire that is psychometrically sound, and is efficient and effective for use in research and clinical settings. This article provides guidelines for the development and translation of questionnaires for application in medical fields, with a special emphasis on perioperative and pain medicine. We provide a framework to guide researchers through the various stages of questionnaire development and translation. To ensure that the questionnaires are psychometrically sound, we present a number of statistical methods to assess the reliability and validity of the questionnaires.
Introduction
Questionnaires or surveys are widely used in perioperative and pain medicine research to collect quantitative information from both patients and health-care professionals. Data of interest could range from observable information (e.g., presence of lesion, mobility) to patients’ subjective feelings of their current status (e.g., the amount of pain they feel, psychological status). Although using an existing questionnaire will save time and resources,[ 1 ] a questionnaire that measures the construct of interest may not be readily available, or the published questionnaire is not available in the language required for the targeted respondents. As a result, investigators may need to develop a new questionnaire or translate an existing one into the language of the intended respondents. Prior work has highlighted the wealth of literature available on psychometric principles, methodological concepts, and techniques regarding questionnaire development/translation and validation. To that end, this article is not meant to provide an exhaustive review of all the related statistical concepts and methods. Rather, this article aims to provide straightforward guidelines for the development or translation of questionnaires (or scales) for use in perioperative and pain medicine research for readers who may be unfamiliar with the process of questionnaire development and/or translation. Readers are recommended to consult the cited references to further examine these techniques for application.
This article is divided into two main sections. The first discusses issues that investigators should be aware of in developing or translating a questionnaire. The second section of this paper illustrates procedures to validate the questionnaire after the questionnaire is developed or translated. A model for the questionnaire development and translation process is presented in Figure 1 . In this special issue of the Saudi journal of Anesthesia we presented multiple studies of development and validation of questionnaires in perioperative and pain medicine, we encourage readers to refer to them for practical experience.

Questionnaire development and translation processes
Preliminary Considerations
It is crucial to identify the construct that is to be assessed with the questionnaire, as the domain of interest will determine what the questionnaire will measure. The next question is: How will the construct be operationalized? In other words, what types of behavior will be indicative of the domain of interest? Several approaches have been suggested to help with this process,[ 2 ] such as content analysis, review of research, critical incidents, direct observations, expert judgment, and instruction.
Once the construct of interest has been determined, it is important to conduct a literature review to identify if a previously validated questionnaire exists. A validated questionnaire refers to a questionnaire/scale that has been developed to be administered among the intended respondents. The validation processes should have been completed using a representative sample, demonstrating adequate reliability and validity. Examples of necessary validation processes can be found in the validation section of this paper. If no existing questionnaires are available, or none that are determined to be appropriate, it is appropriate to construct a new questionnaire. If a questionnaire exists, but only in a different language, the task is to translate and validate the questionnaire in the new language.
Developing a Questionnaire
To construct a new questionnaire, a number of issues should be considered even before writing the questionnaire items.
Identify the dimensionality of the construct
Many constructs are multidimensional, meaning that they are composed of several related components. To fully assess the construct, one may consider developing subscales to assess the different components of the construct. Next, are all the dimensions equally important? or are some more important than others? If the dimensions are equally important, one can assign the same weight to the questions (e.g., by summing or taking the average of all the items). If some dimensions are more important than others, it may not be reasonable to assign the same weight to the questions. Rather, one may consider examining the results from each dimension separately.
Determine the format in which the questionnaire will be administered
Will the questionnaire be self-administered or administered by a research/clinical staff? This decision depends, in part, on what the questionnaire intends to measure. If the questionnaire is designed to measure catastrophic thinking related to pain, respondents may be less likely to respond truthfully if a research/clinical staff asked the questions, whereas they may be more likely to respond truthfully if they are allowed to complete the questionnaire on their own. If the questionnaire is designed to measure patients’ mobility after surgery, respondents may be more likely to overreport the amount of mobility in an effort to demonstrate recovery. To obtain a more accurate measure of mobility after surgery, it may be preferable to obtain objective ratings by clinical staff.
If respondents are to complete the questionnaire by themselves, the items need to be written in a way that can be easily understood by the majority of the respondents, generally about Grade 6 reading level.[ 3 ] If the questionnaire is to be administered to young respondents or respondents with cognitive impairment, the readability level of the items should be lowered. Questionnaires intended for children should take into consideration the cognitive stages of young people[ 4 ] (e.g., pictorial response choices may be more appropriate, such as pain faces to assess pain[ 5 ]).
Determine the item format
Will the items be open ended or close ended? Questions that are open ended allow respondents to elaborate upon their responses. As more detailed information may be obtained using open-ended questions, these items are best suited for situations in which investigators wish to gather more information about a specific domain. However, these responses are often more difficult to code and score, which increases the difficulty of summarizing individuals’ responses. If multiple coders are included, researchers have to address the additional issue of inter-rater reliability.
Questions that are close ended provide respondents a limited number of response options. Compared to open-ended questions, these items are easier to administer and analyze. On the other hand, respondents may not be able to clarify their responses, and their responses may be influenced by the response options provided.
If close-ended items are to be used, should multiple-choice, Likert-type scales, true/false, or other close-ended formats be used? How many response options should be available? If a Likert-type scale is to be adopted, what scale anchors are to be used to indicate the degree of agreement (e.g., strongly agree, agree, neither, disagree, strongly degree), frequency of an event (e.g., almost never, once in a while, sometimes, often, almost always), or other varying options? To make use of participants’ responses for subsequent statistical analyses, researchers should keep in mind that items should be scaled to generate sufficient variance among the intended respondents.[ 6 , 7 ]
Item development
A number of guidelines have been suggested for writing items.[ 7 ] Items should be simple, short, and written in language familiar to the target respondents. The perspective should be consistent across items; items that assess affective responses (e.g., anxiety, depression) should not be mixed with those that assess behavior (e.g., mobility, cognitive functioning).[ 8 ] Items should assess only a single issue. Items that address more than one issue, or “double-barreled” items (e.g., “My daily activities and mood are affected by my pain.”), should not be used. Avoid leading questions as they may result in biased responses. Items that all participants would respond similarly (e.g., “I would like to reduce my pain.”) should not be used, as the small variance generated will provide limited information about the construct being assessed. Table 1 summarizes important tips on writing questions.
Tips on writing questions[ 15 , 16 ]

The issue of whether reverse-scored items should be used remains debatable. Since reverse-scored items are negatively worded, it has been argued that the inclusion of these items may reduce response set bias.[ 9 ] On the other hand, others have found a negative impact on the psychometric properties of scales that included negatively worded items.[ 10 ] In recent years, an increasing amount of literature reports problems with reverse-scored items.[ 11 , 12 , 13 , 14 ] Researchers who decide to include negatively worded items should take extra steps to ensure that the items are interpreted as intended by the respondents, and that the reverse-coded items have similar psychometric properties as the other regularly coded items.[ 7 ]
Determine the intended length of questionnaire
There is no rule of thumb for the number of items that make up a questionnaire. The questionnaire should contain sufficient items to measure the construct of interest, but not be so long that respondents experience fatigue or loss of motivation in completing the questionnaire.[ 17 , 18 ] Not only should a questionnaire possess the most parsimonious (i.e., simplest) structure,[ 19 ] but it also should consist of items that adequately represent the construct of interest to minimize measurement error.[ 20 ] Although a simple structure of questionnaire is recommended, a large pool of items is needed in the early stages of the questionnaire's development as many of these items might be discarded throughout the development process.[ 7 ]
Review and revise initial pool of items
After the initial pool of questionnaire items are written, qualified experts should review the items. Specifically, the items should be reviewed to make sure they are accurate, free of item construction problems, and grammatically correct. The reviewers should, to the best of their ability, ensure that the items do not contain content that may be perceived as offensive or biased by a particular subgroup of respondents.
Preliminary pilot testing
Before conducting a pilot test of the questionnaire on the intended respondents, it is advisable to test the questionnaire items on a small sample (about 30–50)[ 21 ] of respondents.[ 17 ] This is an opportunity for the questionnaire developer to know if there is confusion about any items, and whether respondents have suggestions for possible improvements of the items. One can also get a rough idea of the response distribution to each item, which can be informative in determining whether there is enough variation in the response to justify moving forward with a large-scale pilot test. Feasibility and the presence of floor (almost all respondents scored near the bottom) or ceiling effects (almost all respondents scored near the top) are important determinants of items that are included or rejected at this stage. Although it is possible that participants’ responses to questionnaires may be affected by question order,[ 22 , 23 , 24 ] this issue should be addressed only after the initial questionnaire has been validated. The questionnaire items should be revised upon reviewing the results of the preliminary pilot testing. This process may be repeated a few times before finalizing the final draft of the questionnaire.
So far, we highlighted the major steps that need to be undertaken when constructing a new questionnaire. Researchers should be able to clearly link the questionnaire items to the theoretical construct they intend to assess. Although such associations may be obvious to researchers who are familiar with the specific topic, they may not be apparent to other readers and reviewers. To develop a questionnaire with good psychometric properties that can subsequently be applied in research or clinical practice, it is crucial to invest the time and effort to ensure that the items adequately assess the construct of interest.
Translating a Questionnaire
The following section summarizes the guidelines for translating a questionnaire into a different language.
Forward translation
The initial translation from the original language to the target language should be made by at least two independent translators.[ 25 , 26 ] Preferably, the bilingual translators should be translating the questionnaire into their mother tongue, to better reflect the nuances of the target language.[ 27 ] It is recommended that one translator be aware of the concepts the questionnaire intend to measure, to provide a translation that more closely resembles the original instrument. It is suggested that a naïve translator, who is unaware of the objective of the questionnaire, produce the second translation so that subtle differences in the original questionnaire may be detected.[ 25 , 26 ] Discrepancies between the two (or more) translators can be discussed and resolved between the original translators, or with the addition of an unbiased, bilingual translator who was not involved in the previous translations.
Backward translation
The initial translation should be independently back-translated (i.e., translate back from the target language into the original language) to ensure the accuracy of the translation. Misunderstandings or unclear wordings in the initial translations may be revealed in the back-translation.[ 25 ] As with the forward translation, the backward translation should be performed by at least two independent translators, preferably translating into their mother language (the original language).[ 26 ] To avoid bias, back-translators should preferably not be aware of the intended concepts the questionnaire measures.[ 25 ]
Expert committee
Constituting an expert committee is suggested to produce the prefinal version of the translation.[ 25 ] Members of the committee should include experts who are familiar with the construct of interest, a methodologist, both the forward and backward translators, and if possible, developers of the original questionnaires. The expert committee will need to review all versions of the translations and determine whether the translated and original versions achieve semantic, idiomatic, experiential, and conceptual equivalence.[ 25 , 28 ] Any discrepancies will need to be resolved, and members of the expert committee will need to reach a consensus on all items to produce a prefinal version of the translated questionnaire. If necessary, the process of translation and back-translation can be repeated.
As with developing a new questionnaire, the prefinal version of the translated questionnaire should be pilot tested on a small sample (about 30–50)[ 21 ] of the intended respondents.[ 25 , 26 ] After completing the translated questionnaire, the respondent is asked (verbally by an interviewer or via an open-ended question) to elaborate what they thought each questionnaire item and their corresponding response meant. This approach allows the investigator to make sure that the translated items retained the same meaning as the original items, and to ensure there is no confusion regarding the translated questionnaire. This process may be repeated a few times to finalize the final translated version of the questionnaire.
In this section, we provided a template for translating an existing questionnaire into a different language. Considering that most questionnaires were initially developed in one language (e.g., English when developed in English-speaking countries[ 25 ]), translated versions of the questionnaires are needed for researchers who intend to collect data among respondents who speak other languages. To compare responses across populations of different language and/or culture, researchers need to make sure that the questionnaires in different languages are assessing the equivalent construct with an equivalent metric. Although the translation process is time consuming and costly, it is the best method to ensure that a translated measure is equivalent to the original questionnaire.[ 28 ]
Validating a Questionnaire
Initial validation.
After the new or translated questionnaire items pass through preliminary pilot testing and subsequent revisions, it is time to conduct a pilot test among the intended respondents for initial validation. In this pilot test, the final version of the questionnaire is administered to a large representative sample of respondents for whom the questionnaire is intended. If the pilot test is conducted for small samples, the relatively large sampling errors may reduce the statistical power needed to validate the questionnaire.[ 2 ]
Reliability
The reliability of a questionnaire can be considered as the consistency of the survey results. As measurement error is present in content sampling, changes in respondents, and differences across raters, the consistency of a questionnaire can be evaluated using its internal consistency, test-retest reliability, and inter-rater reliability, respectively.
Internal consistency
Internal consistency reflects the extent to which the questionnaire items are inter-correlated, or whether they are consistent in measurement of the same construct. Internal consistency is commonly estimated using the coefficient alpha,[ 29 ] also known as Cronbach's alpha. Given a questionnaire x , with k number of items, alpha ( α ) can be computed as:

Cronbach's alpha ranges from 0 to 1 (when some items are negatively correlated with other items in the questionnaire, it is possible to have negative values of Cronbach's alpha). When reverse-scored items are [incorrectly] not reverse scored, it can be easily remedied by correctly scoring the items. However, if a negative Cronbach's alpha is still obtained when all items are correctly scored, there are serious problems in the original design of the questionnaire), with higher values indicating that items are more strongly interrelated with one another. Cronbach's α = 0 indicates no internal consistency (i.e., none of the items are correlated with one another), whereas α = 1 reflects perfect internal consistency (i.e., all the items are perfectly correlated with one another). In practice, Cronbach's alpha of at least 0.70 has been suggested to indicate adequate internal consistency.[ 30 ] A low Cronbach's alpha value may be due to poor inter-relatedness between items; as such, items with low correlations with the questionnaire total score should be discarded or revised. As alpha is a function of the length of the questionnaire, alpha will increase with the number of items. In addition, alpha will increase if the variability of each item is increased. It is, therefore, possible to increase alpha by including more related items, or adding items that have more variability to the questionnaire. On the other hand, an alpha value that is too high ( α ≥ 0.90) suggests that some questionnaire items may be redundant;[ 31 ] investigators may consider removing items that are essentially asking the same thing in multiple ways.
It is important to note that Cronbach's alpha is a property of the responses from a specific sample of respondents.[ 31 ] Investigators need to keep in mind that Cronbach's alpha is not “the” estimate of reliability for a questionnaire under all circumstances. Rather, the alpha value only indicates the extent to which the questionnaire is reliable for “a particular population of examinees.”[ 32 ] A questionnaire with excellent reliability with one sample may not necessarily have the same reliability in another. Therefore, the reliability of a questionnaire should be estimated each time the questionnaire is administered, including pilot testing and subsequent validation stages.
Test-retest reliability
Test-retest reliability refers to the extent to which individuals’ responses to the questionnaire items remain relatively consistent across repeated administration of the same questionnaire or alternate questionnaire forms.[ 2 ] Provided the same individuals were administered the same questionnaires twice (or more), test-retest reliability can be evaluated using Pearson's product moment correlation coefficient (Pearson's r ) or the intraclass correlation coefficient.
Pearson's r between the two questionnaires’ responses can be referred to as the coefficient of stability. A larger stability coefficient indicates stronger test-retest reliability, reflecting that measurement error of the questionnaire is less likely to be attributable to changes in the individuals’ responses over time.
Test-retest reliability can be considered the stability of respondents’ attributes; it is applicable to questionnaires that are designed to measure personality traits, interest, or attitudes that are relatively stable across time, such as anxiety and pain catastrophizing. If the questionnaires are constructed to measure transitory attributes, such as pain intensity and quality of recovery, test-retest reliability is not applicable as the changes in respondents’ responses between assessments are reflected in the instability of their responses. Although test-retest reliability is sometimes reported for scales that are intended to assess constructs that change between administrations, researchers should be aware that test-retest reliability is not applicable and does not provide useful information about the questionnaires of interest. Researchers should also be critical when evaluating the reliability estimates reported in such studies.
An important question to consider in estimating test-retest reliability is how much time should lapse between questionnaire administrations? If the duration between time 1 and time 2 is too short, individuals may remember their responses in time 1, which may overestimate the test-retest reliability. Respondents, especially those recovering from major surgery, may experience fatigue if the retest is administered shortly after the first administration, which may underestimate the test-retest reliability. On the other hand, if there is a long period of time between questionnaire administrations, individuals’ responses may change due to other factors (e.g., a respondent may be taking pain management medications to treat chronic pain condition). Unfortunately, there is no single answer. The duration should be long enough to allow the effects of memory to fade and to prevent fatigue, but not so long as to allow changes to take place that may affect the test-retest reliability estimate.[ 17 ]
Inter-rater reliability
For questionnaires in which multiple raters complete the same instrument for each examinee (e.g., a checklist of behavior/symptoms), the extent to which raters are consistent in their observations across the same group of examinees can be evaluated. This consistency is referred to as the inter-rater reliability, or inter-rater agreement, and can be estimated using the kappa statistic.[ 33 ] Suppose two clinicians independently rated the same group of patients on their mobility after surgery (e.g., 0 = needs help of 2+ people; 1 = needs help of 1 person; 2 = independent), kappa ( к ) can be computed as follows:

Where, P o is the observed proportion of observations in which the two raters agree, and P e is the expected proportion of observations in which the two raters agree by chance. Accordingly, к is the proportion of agreement between the two raters, after factoring out the proportion of agreement by chance. к ranges from 0 to 1, where к = 0 indicates all chance agreements and к =1 represents perfect agreement between the two raters. Others have suggested к = 0 as no agreement, к = 0.01 − 0.20 as poor agreement, к = 0.21 − 0.40 as slight agreement, к = 0.41 − 0.60 as fair agreement, к = 0.61 − 0.80 as good agreement, к = 0.81 − 0.92 as very good agreement, and к = 0.93 − 1 as excellent agreement.[ 34 , 35 ] If more than two raters are used, an extension of Cohen's к statistic is available to compute the inter-rater reliability across multiple raters.[ 36 ]
The validity of a questionnaire is determined by analyzing whether the questionnaire measures what it is intended to measure. In other words, are the inferences and conclusions made based on the results of the questionnaire (i.e., test scores) valid?[ 37 ] Two major types of validity should be considered when validating a questionnaire: content validity and construct validity.
Content validity
Content validity refers to the extent to which the items in a questionnaire are representative of the entire theoretical construct the questionnaire is designed to assess.[ 17 ] Although the construct of interest determines which items are written and/or selected in the questionnaire development/translation phase, content validity of the questionnaire should be evaluated after the initial form of the questionnaire is available.[ 2 ] The process of content validation is particularly crucial in the development of a new questionnaire.
A panel of experts who are familiar with the construct that the questionnaire is designed to measure should be tasked with evaluating the content validity of the questionnaire. The experts judge, as a panel, whether the questionnaire items are adequately measuring the construct intended to assess, and whether the items are sufficient to measure the domain of interest. Several approaches to quantify the judgment of content validity across experts are also available, such as the content validity ratio[ 38 ] and content validation form.[ 39 , 40 ] Nonetheless, as the process of content validation depends heavily on how well the panel of experts can assess the extent to which the construct of interest is operationalized, the selection of appropriate experts is crucial to ensure that content validity is evaluated adequately. Example items to assess content validity include:[ 41 ]
- The questions were clear and easy
- The questions covered all the problem areas with your pain
- You would like the use of this questionnaire for future assessments
- The questionnaire lacks important questions regarding your pain
- Some of the questions violate your privacy.
A concept that is related to content validity is face validity. Face validity refers to the degree to which the respondents or laypersons judge the questionnaire items to be valid. Such judgment is based less on the technical components of the questionnaire items, but rather on whether the items appear to be measuring a construct that is meaningful to the respondents. Although this is the weakest way to establish the validity of a questionnaire, face validity may motivate respondents to answer more truthfully. For example, if patients perceive a quality of recovery questionnaire to be evaluating how well they are recovering from surgery, they may be more likely to respond in ways that reflect their recovery status.
Construct validity
Construct validity is the most important concept in evaluating a questionnaire that is designed to measure a construct that is not directly observable (e.g., pain, quality of recovery). If a questionnaire lacks construct validity, it will be difficult to interpret results from the questionnaire, and inferences cannot be drawn from questionnaire responses to a behavior domain. The construct validity of a questionnaire can be evaluated by estimating its association with other variables (or measures of a construct) with which it should be correlated positively, negatively, or not at all.[ 42 ] In practice, the questionnaire of interest, as well as the preexisting instruments that measure similar and dissimilar constructs, is administered to the same groups of individuals. Correlation matrices are then used to examine the expected patterns of associations between different measures of the same construct, and those between a questionnaire of a construct and other constructs. It has been suggested that correlation coefficients of 0.1 should be considered as small, 0.3 as moderate, and 0.5 as large.[ 43 ]
For instance, suppose a new scale is developed to assess pain among hospitalized patients. To provide evidence of construct validity for this new pain scale, we can examine how well patients’ responses on the new scale correlate with the preexisting instruments that also measure pain. This is referred to as convergent validity. One would expect strong correlations between the new questionnaire and the existing measures of the same construct, since they are measuring the same theoretical construct.
Alternatively, the extent to which patients’ responses on the new pain scale correlate with instruments that measure unrelated constructs, such as mobility or cognitive function, can be assessed. This is referred to as divergent validity. As pain is theoretically dissimilar to the constructs of mobility or cognitive function, we would expect zero, or very weak, correlation between the new pain questionnaire and instruments that assess mobility or cognitive function. Table 2 describes different validation types and important definitions.
Questionnaire-related terminology[ 16 , 44 , 45 ]

Subsequent validation
The process described so far defines the steps for initial validation. However, the usefulness of the scale is the ability to discriminate between different cohorts in the domain of interest. It is advised that several studies investigating different cohorts or interventions should be conducted to identify whether the scale can discriminate between groups. Ideally, these studies should have clearly defined outcomes where the changes in the domain of interest are well known. For example, in subsequent validation of the Postoperative Quality of Recovery Scale, four studies were constructed to show the ability to discriminate recovery and cognition in different cohorts of participants (mixed cohort, orthopedics, and otolaryngology), as well as a human volunteer study to calibrate the cognitive domain.[ 46 , 47 , 48 , 49 ]
Sample size
Guidelines for the respondent-to-item ratio ranged from 5:1[ 50 ] (i.e., fifty respondents for a 10-item questionnaire), 10:1,[ 30 ] to 15:1 or 30:1.[ 51 ] Others suggested that sample sizes of 50 should be considered as very poor, 100 as poor, 200 as fair, 300 as good, 500 as very good, and 1000 or more as excellent.[ 52 ] Given the variation in the types of questionnaire being used, there are no absolute rules for the sample size needed to validate a questionnaire.[ 53 ] As larger samples are always better than smaller samples, it is recommended that investigators utilize as large a sample size as possible. The respondent-to-item ratios can be utilized to further strengthen the rationale for the large sample size when necessary.
Other considerations
Even though data collection using questionnaires is relatively easy, researchers should be cognizant about the necessary approvals that should be obtained prior to beginning the research project. Considering the differences in regulations and requirements in different countries, agencies, and institutions, researchers are advised to consult the research ethics committee at their agencies and/or institutions regarding the necessary approval needed and additional considerations that should be addressed.
In this review, we provided guidelines on how to develop, validate, and translate a questionnaire for use in perioperative and pain medicine. The development and translation of a questionnaire requires investigators’ thorough consideration of issues relating to the format of the questionnaire and the meaning and appropriateness of the items. Once the development or translation stage is completed, it is important to conduct a pilot test to ensure that the items can be understood and correctly interpreted by the intended respondents. The validation stage is crucial to ensure that the questionnaire is psychometrically sound. Although developing and translating a questionnaire is no easy task, the processes outlined in this article should enable researchers to end up with questionnaires that are efficient and effective in the target populations.
Financial support and sponsorship
Siny Tsang, PhD, was supported by the research training grant 5-T32-MH 13043 from the National Institute of Mental Health.
Conflicts of interest
There are no conflicts of interest.
Have a language expert improve your writing
Run a free plagiarism check in 10 minutes, generate accurate citations for free.
- Knowledge Base
Methodology
Reliability vs. Validity in Research | Difference, Types and Examples
Published on July 3, 2019 by Fiona Middleton . Revised on June 22, 2023.
Reliability and validity are concepts used to evaluate the quality of research. They indicate how well a method , technique. or test measures something. Reliability is about the consistency of a measure, and validity is about the accuracy of a measure.opt
It’s important to consider reliability and validity when you are creating your research design , planning your methods, and writing up your results, especially in quantitative research . Failing to do so can lead to several types of research bias and seriously affect your work.
Reliability | Validity | |
---|---|---|
What does it tell you? | The extent to which the results can be reproduced when the research is repeated under the same conditions. | The extent to which the results really measure what they are supposed to measure. |
How is it assessed? | By checking the consistency of results across time, across different observers, and across parts of the test itself. | By checking how well the results correspond to established theories and other measures of the same concept. |
How do they relate? | A reliable measurement is not always valid: the results might be , but they’re not necessarily correct. | A valid measurement is generally reliable: if a test produces accurate results, they should be reproducible. |
Table of contents
Understanding reliability vs validity, how are reliability and validity assessed, how to ensure validity and reliability in your research, where to write about reliability and validity in a thesis, other interesting articles.
Reliability and validity are closely related, but they mean different things. A measurement can be reliable without being valid. However, if a measurement is valid, it is usually also reliable.
What is reliability?
Reliability refers to how consistently a method measures something. If the same result can be consistently achieved by using the same methods under the same circumstances, the measurement is considered reliable.
What is validity?
Validity refers to how accurately a method measures what it is intended to measure. If research has high validity, that means it produces results that correspond to real properties, characteristics, and variations in the physical or social world.
High reliability is one indicator that a measurement is valid. If a method is not reliable, it probably isn’t valid.
If the thermometer shows different temperatures each time, even though you have carefully controlled conditions to ensure the sample’s temperature stays the same, the thermometer is probably malfunctioning, and therefore its measurements are not valid.
However, reliability on its own is not enough to ensure validity. Even if a test is reliable, it may not accurately reflect the real situation.
Validity is harder to assess than reliability, but it is even more important. To obtain useful results, the methods you use to collect data must be valid: the research must be measuring what it claims to measure. This ensures that your discussion of the data and the conclusions you draw are also valid.
Prevent plagiarism. Run a free check.
Reliability can be estimated by comparing different versions of the same measurement. Validity is harder to assess, but it can be estimated by comparing the results to other relevant data or theory. Methods of estimating reliability and validity are usually split up into different types.
Types of reliability
Different types of reliability can be estimated through various statistical methods.
Type of reliability | What does it assess? | Example |
---|---|---|
The consistency of a measure : do you get the same results when you repeat the measurement? | A group of participants complete a designed to measure personality traits. If they repeat the questionnaire days, weeks or months apart and give the same answers, this indicates high test-retest reliability. | |
The consistency of a measure : do you get the same results when different people conduct the same measurement? | Based on an assessment criteria checklist, five examiners submit substantially different results for the same student project. This indicates that the assessment checklist has low inter-rater reliability (for example, because the criteria are too subjective). | |
The consistency of : do you get the same results from different parts of a test that are designed to measure the same thing? | You design a questionnaire to measure self-esteem. If you randomly split the results into two halves, there should be a between the two sets of results. If the two results are very different, this indicates low internal consistency. |
Types of validity
The validity of a measurement can be estimated based on three main types of evidence. Each type can be evaluated through expert judgement or statistical methods.
Type of validity | What does it assess? | Example |
---|---|---|
The adherence of a measure to of the concept being measured. | A self-esteem questionnaire could be assessed by measuring other traits known or assumed to be related to the concept of self-esteem (such as social skills and ). Strong correlation between the scores for self-esteem and associated traits would indicate high construct validity. | |
The extent to which the measurement of the concept being measured. | A test that aims to measure a class of students’ level of Spanish contains reading, writing and speaking components, but no listening component. Experts agree that listening comprehension is an essential aspect of language ability, so the test lacks content validity for measuring the overall level of ability in Spanish. | |
The extent to which the result of a measure corresponds to of the same concept. | A is conducted to measure the political opinions of voters in a region. If the results accurately predict the later outcome of an election in that region, this indicates that the survey has high criterion validity. |
To assess the validity of a cause-and-effect relationship, you also need to consider internal validity (the design of the experiment ) and external validity (the generalizability of the results).
The reliability and validity of your results depends on creating a strong research design , choosing appropriate methods and samples, and conducting the research carefully and consistently.
Ensuring validity
If you use scores or ratings to measure variations in something (such as psychological traits, levels of ability or physical properties), it’s important that your results reflect the real variations as accurately as possible. Validity should be considered in the very earliest stages of your research, when you decide how you will collect your data.
- Choose appropriate methods of measurement
Ensure that your method and measurement technique are high quality and targeted to measure exactly what you want to know. They should be thoroughly researched and based on existing knowledge.
For example, to collect data on a personality trait, you could use a standardized questionnaire that is considered reliable and valid. If you develop your own questionnaire, it should be based on established theory or findings of previous studies, and the questions should be carefully and precisely worded.
- Use appropriate sampling methods to select your subjects
To produce valid and generalizable results, clearly define the population you are researching (e.g., people from a specific age range, geographical location, or profession). Ensure that you have enough participants and that they are representative of the population. Failing to do so can lead to sampling bias and selection bias .
Ensuring reliability
Reliability should be considered throughout the data collection process. When you use a tool or technique to collect data, it’s important that the results are precise, stable, and reproducible .
- Apply your methods consistently
Plan your method carefully to make sure you carry out the same steps in the same way for each measurement. This is especially important if multiple researchers are involved.
For example, if you are conducting interviews or observations , clearly define how specific behaviors or responses will be counted, and make sure questions are phrased the same way each time. Failing to do so can lead to errors such as omitted variable bias or information bias .
- Standardize the conditions of your research
When you collect your data, keep the circumstances as consistent as possible to reduce the influence of external factors that might create variation in the results.
For example, in an experimental setup, make sure all participants are given the same information and tested under the same conditions, preferably in a properly randomized setting. Failing to do so can lead to a placebo effect , Hawthorne effect , or other demand characteristics . If participants can guess the aims or objectives of a study, they may attempt to act in more socially desirable ways.
It’s appropriate to discuss reliability and validity in various sections of your thesis or dissertation or research paper . Showing that you have taken them into account in planning your research and interpreting the results makes your work more credible and trustworthy.
Section | Discuss |
---|---|
What have other researchers done to devise and improve methods that are reliable and valid? | |
How did you plan your research to ensure reliability and validity of the measures used? This includes the chosen sample set and size, sample preparation, external conditions and measuring techniques. | |
If you calculate reliability and validity, state these values alongside your main results. | |
This is the moment to talk about how reliable and valid your results actually were. Were they consistent, and did they reflect true values? If not, why not? | |
If reliability and validity were a big problem for your findings, it might be helpful to mention this here. |
If you want to know more about statistics , methodology , or research bias , make sure to check out some of our other articles with explanations and examples.
- Normal distribution
- Degrees of freedom
- Null hypothesis
- Discourse analysis
- Control groups
- Mixed methods research
- Non-probability sampling
- Quantitative research
- Ecological validity
Research bias
- Rosenthal effect
- Implicit bias
- Cognitive bias
- Selection bias
- Negativity bias
- Status quo bias
Cite this Scribbr article
If you want to cite this source, you can copy and paste the citation or click the “Cite this Scribbr article” button to automatically add the citation to our free Citation Generator.
Middleton, F. (2023, June 22). Reliability vs. Validity in Research | Difference, Types and Examples. Scribbr. Retrieved July 27, 2024, from https://www.scribbr.com/methodology/reliability-vs-validity/
Is this article helpful?
Fiona Middleton
Other students also liked, what is quantitative research | definition, uses & methods, data collection | definition, methods & examples, get unlimited documents corrected.
✔ Free APA citation check included ✔ Unlimited document corrections ✔ Specialized in correcting academic texts

IMAGES
VIDEO
COMMENTS
This paper is structured as follows: Section 1 provides the introduction to the need for a validation format for research, and the fundamentals of validation and the factors involved in validation from various literature studies are discussed in Section 2. Section 3 presents the methodology used in framing the validation format.
Given (1) the historical context for the terms verification and validation in software and hardware standards, regulations, and guidances, and (2) the separated concepts of analytical and clinical ...
Consequently, validation challenges have been well observed in the earlier research, and our aim in this paper is to study the validation methods that resolve or alleviate these challenges. Gao et al. (2019) also argue that there is a deficiency in supporting tools for validating AI systems. Readily available tools are not non-existent.
Importance of data validation. Data validation is important for several aspects of a well-conducted study: To ensure a robust dataset: The primary aim of data validation is to ensure an error-free dataset for further analysis. This is especially important if you or other researchers plan to use the dataset for future studies or to train machine ...
The purpose of this book is to further define validity and to explore the factors that should be considered when evaluating claims from research and assessment. 2 Those who write about validity generally focus on either assessment or research. However, research and assessment are inextricably related to one another.
The internal validation processes on the instrument (i.e., the evaluation dimensions, subitems, and scales of subitems) followed a revised Delphi method [18 - 22], which included transparent and open discussions (via face-to-face meetings, emails, and complementary video conferences) among the research team. The external validation consisted ...
Though data preprocessing and storage methods have developed considerably over the past few years, there is little agreement on what constitutes the best set of data validation practices in the social sciences. In this paper I provide five simple steps that can help students and practitioners improve their data validation processes.
Meyer et al. also evaluate using a spatial cross-validation approach, but also add a time dimension using Leave-Time-Out cross-validation where samples belong to one fold if they fall into a ...
Data validation is important for ensuring regular monitoring of your data and assuring all stakeholders that your data is of a high quality that reliably meets research integrity standards — and also a crucial aspect of Yale's Research Data and Materials Policy, which states "The University deems appropriate stewardship of research data as fundamental to both high-quality research and ...
In this paper, we tackle this problem and present a data validation system that is designed to detect anomalies specifically in data fed into machine learning pipelines. This system is deployed in production as an integral part of TFX (Baylor, 2017) -- an end-to-end machine learning platform at Google. It is used by hundreds of product teams ...
This guide is solely about the production of a valid survey for research purposes. ‡Boateng et al. (2018) offers a similar practical guide but from a different perspective with somewhat different coverage. § Much of what is in this practical guide can also be applied to the development and validation of interview protocols.
Validation studies, in which an investigator compares the accuracy of a measure with a gold standard measure, are an important way to understand and mitigate this bias. More attention is being paid to the importance of validation studies in recent years, yet they remain rare in epidemiologic research and, in our experience, they remain poorly ...
These few authors argue that the broad and abstract concepts of reliability and validity can be applied to all research because the goal of finding plausible and credible outcome explanations is central to all research (Hammersley, 1992; Kuzel & Engel, 2001; Yin, 1994). We are concerned, nonetheless, that the focus on evaluation strategies that ...
This notion is based on a three-step early validation process. This paper shares the same philosophy regarding the importance of validity at all stages of the systems engineering life cycle but differs in the treatment of these artifacts. ... logistics, and how evidence is presented to stakeholders. Further research will address these ...
This paper provides an A-Z guide for sampling and census within an integrated framework of data collection and validation. It provides worked examples and inter-disciplinary exemplifications of essential methods, techniques and formulas for each step of the framework. Real research application tricks are disentangled for non-statisticians.
Peer debriefing is a form of external evaluation of the qualitative research process. Lincoln and Guba (1985, p. 308) describe the role of the peer reviewer as the "devil's advocate.". It is a person who asks difficult questions about the procedures, meanings, interpretations, and conclusions of the investigation.
Validity in research is the ability to conduct an accurate study with the right tools and conditions to yield acceptable and reliable data that can be reproduced. Researchers rely on carefully calibrated tools for precise measurements. However, collecting accurate information can be more of a challenge. Studies must be conducted in environments ...
We use survey data from three four-year campuses to explore the relationship between academic validation and student outcomes during students' first 3 years in college using structural equation modeling. We examine both a psychosocial outcome (mattering to campus) and an academic outcome (cumulative GPA). We find that both frequency of interactions with faculty and feelings of academic ...
Important stages in validation The action identifying with validation studies can be categorized mainly into three stages: Stage 1 This includes pre-validation qualification stage which covers all exercises identifying with product studies and improvement, formulation pilot batch testing, scale-up research, exchange of
Compare your paper to billions of pages and articles with Scribbr's Turnitin-powered plagiarism checker. ... The 4 Types of Validity in Research | Definitions & Examples. ... Concurrent validity is a validation strategy where the the scores of a test and the criterion are obtained at the same time.
Whenever I write a paper, review a paper a key item that I look for is whether the methodology and equipment has been validated, demonstrating the effectiveness of the research. Without validation ...
Data validation, a critical yet often overlooked aspect of the research process, ensures that the data collected and analyzed can truly support the conclusions drawn. This article explores the vital importance of data validation, providing insights into why it is indispensable for accuracy, reliability, credibility, and much more.
The second section of this paper illustrates procedures to validate the questionnaire after the questionnaire is developed or translated. ... To develop a questionnaire with good psychometric properties that can subsequently be applied in research or clinical practice, it is crucial to invest the time and effort to ensure that the items ...
Joseph A. Kitchen is an assistant professor of higher education at the University of Miami in Miami, Florida. Dr. Kitchen conducts quantitative, qualitative, and mixed-methods research and his research agenda spans several areas, with a central focus on the role of college transition, outreach, and support programs and interventions in promoting equitable outcomes and college success among ...
Reliability is about the consistency of a measure, and validity is about the accuracy of a measure.opt. It's important to consider reliability and validity when you are creating your research design, planning your methods, and writing up your results, especially in quantitative research. Failing to do so can lead to several types of research ...
from research organizations. ... the researchers are pursuing more animal studies to validate an advanced blood pressure management approach that uses this method. ... Dahleh and Brown, the paper ...