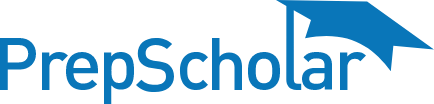

Choose Your Test
Sat / act prep online guides and tips, 113 great research paper topics.
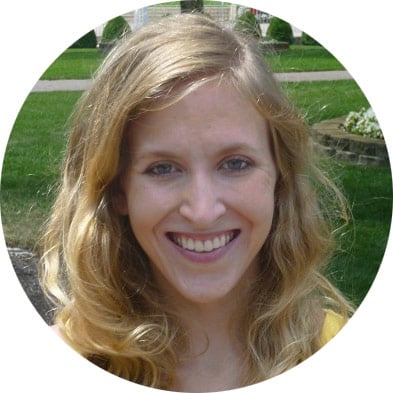
General Education
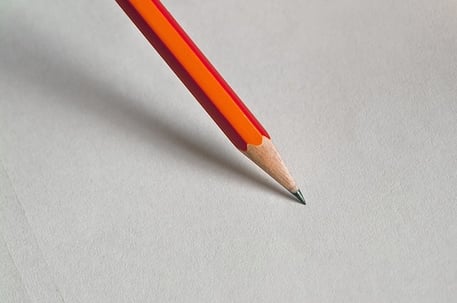
One of the hardest parts of writing a research paper can be just finding a good topic to write about. Fortunately we've done the hard work for you and have compiled a list of 113 interesting research paper topics. They've been organized into ten categories and cover a wide range of subjects so you can easily find the best topic for you.
In addition to the list of good research topics, we've included advice on what makes a good research paper topic and how you can use your topic to start writing a great paper.
What Makes a Good Research Paper Topic?
Not all research paper topics are created equal, and you want to make sure you choose a great topic before you start writing. Below are the three most important factors to consider to make sure you choose the best research paper topics.
#1: It's Something You're Interested In
A paper is always easier to write if you're interested in the topic, and you'll be more motivated to do in-depth research and write a paper that really covers the entire subject. Even if a certain research paper topic is getting a lot of buzz right now or other people seem interested in writing about it, don't feel tempted to make it your topic unless you genuinely have some sort of interest in it as well.
#2: There's Enough Information to Write a Paper
Even if you come up with the absolute best research paper topic and you're so excited to write about it, you won't be able to produce a good paper if there isn't enough research about the topic. This can happen for very specific or specialized topics, as well as topics that are too new to have enough research done on them at the moment. Easy research paper topics will always be topics with enough information to write a full-length paper.
Trying to write a research paper on a topic that doesn't have much research on it is incredibly hard, so before you decide on a topic, do a bit of preliminary searching and make sure you'll have all the information you need to write your paper.
#3: It Fits Your Teacher's Guidelines
Don't get so carried away looking at lists of research paper topics that you forget any requirements or restrictions your teacher may have put on research topic ideas. If you're writing a research paper on a health-related topic, deciding to write about the impact of rap on the music scene probably won't be allowed, but there may be some sort of leeway. For example, if you're really interested in current events but your teacher wants you to write a research paper on a history topic, you may be able to choose a topic that fits both categories, like exploring the relationship between the US and North Korea. No matter what, always get your research paper topic approved by your teacher first before you begin writing.
113 Good Research Paper Topics
Below are 113 good research topics to help you get you started on your paper. We've organized them into ten categories to make it easier to find the type of research paper topics you're looking for.
Arts/Culture
- Discuss the main differences in art from the Italian Renaissance and the Northern Renaissance .
- Analyze the impact a famous artist had on the world.
- How is sexism portrayed in different types of media (music, film, video games, etc.)? Has the amount/type of sexism changed over the years?
- How has the music of slaves brought over from Africa shaped modern American music?
- How has rap music evolved in the past decade?
- How has the portrayal of minorities in the media changed?
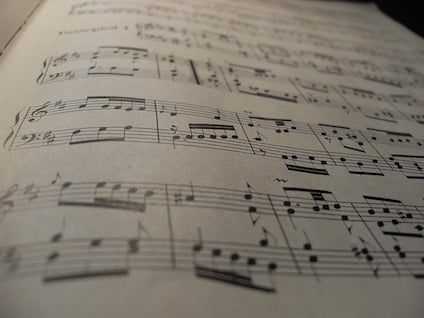
Current Events
- What have been the impacts of China's one child policy?
- How have the goals of feminists changed over the decades?
- How has the Trump presidency changed international relations?
- Analyze the history of the relationship between the United States and North Korea.
- What factors contributed to the current decline in the rate of unemployment?
- What have been the impacts of states which have increased their minimum wage?
- How do US immigration laws compare to immigration laws of other countries?
- How have the US's immigration laws changed in the past few years/decades?
- How has the Black Lives Matter movement affected discussions and view about racism in the US?
- What impact has the Affordable Care Act had on healthcare in the US?
- What factors contributed to the UK deciding to leave the EU (Brexit)?
- What factors contributed to China becoming an economic power?
- Discuss the history of Bitcoin or other cryptocurrencies (some of which tokenize the S&P 500 Index on the blockchain) .
- Do students in schools that eliminate grades do better in college and their careers?
- Do students from wealthier backgrounds score higher on standardized tests?
- Do students who receive free meals at school get higher grades compared to when they weren't receiving a free meal?
- Do students who attend charter schools score higher on standardized tests than students in public schools?
- Do students learn better in same-sex classrooms?
- How does giving each student access to an iPad or laptop affect their studies?
- What are the benefits and drawbacks of the Montessori Method ?
- Do children who attend preschool do better in school later on?
- What was the impact of the No Child Left Behind act?
- How does the US education system compare to education systems in other countries?
- What impact does mandatory physical education classes have on students' health?
- Which methods are most effective at reducing bullying in schools?
- Do homeschoolers who attend college do as well as students who attended traditional schools?
- Does offering tenure increase or decrease quality of teaching?
- How does college debt affect future life choices of students?
- Should graduate students be able to form unions?
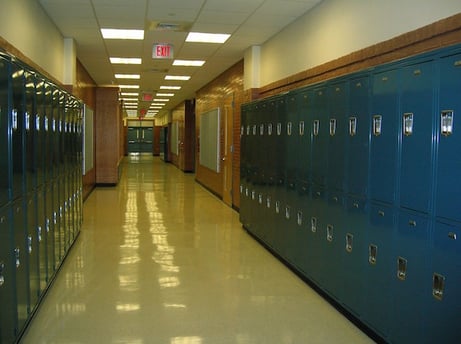
- What are different ways to lower gun-related deaths in the US?
- How and why have divorce rates changed over time?
- Is affirmative action still necessary in education and/or the workplace?
- Should physician-assisted suicide be legal?
- How has stem cell research impacted the medical field?
- How can human trafficking be reduced in the United States/world?
- Should people be able to donate organs in exchange for money?
- Which types of juvenile punishment have proven most effective at preventing future crimes?
- Has the increase in US airport security made passengers safer?
- Analyze the immigration policies of certain countries and how they are similar and different from one another.
- Several states have legalized recreational marijuana. What positive and negative impacts have they experienced as a result?
- Do tariffs increase the number of domestic jobs?
- Which prison reforms have proven most effective?
- Should governments be able to censor certain information on the internet?
- Which methods/programs have been most effective at reducing teen pregnancy?
- What are the benefits and drawbacks of the Keto diet?
- How effective are different exercise regimes for losing weight and maintaining weight loss?
- How do the healthcare plans of various countries differ from each other?
- What are the most effective ways to treat depression ?
- What are the pros and cons of genetically modified foods?
- Which methods are most effective for improving memory?
- What can be done to lower healthcare costs in the US?
- What factors contributed to the current opioid crisis?
- Analyze the history and impact of the HIV/AIDS epidemic .
- Are low-carbohydrate or low-fat diets more effective for weight loss?
- How much exercise should the average adult be getting each week?
- Which methods are most effective to get parents to vaccinate their children?
- What are the pros and cons of clean needle programs?
- How does stress affect the body?
- Discuss the history of the conflict between Israel and the Palestinians.
- What were the causes and effects of the Salem Witch Trials?
- Who was responsible for the Iran-Contra situation?
- How has New Orleans and the government's response to natural disasters changed since Hurricane Katrina?
- What events led to the fall of the Roman Empire?
- What were the impacts of British rule in India ?
- Was the atomic bombing of Hiroshima and Nagasaki necessary?
- What were the successes and failures of the women's suffrage movement in the United States?
- What were the causes of the Civil War?
- How did Abraham Lincoln's assassination impact the country and reconstruction after the Civil War?
- Which factors contributed to the colonies winning the American Revolution?
- What caused Hitler's rise to power?
- Discuss how a specific invention impacted history.
- What led to Cleopatra's fall as ruler of Egypt?
- How has Japan changed and evolved over the centuries?
- What were the causes of the Rwandan genocide ?
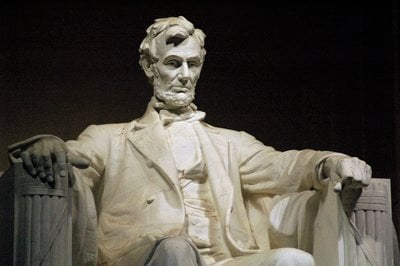
- Why did Martin Luther decide to split with the Catholic Church?
- Analyze the history and impact of a well-known cult (Jonestown, Manson family, etc.)
- How did the sexual abuse scandal impact how people view the Catholic Church?
- How has the Catholic church's power changed over the past decades/centuries?
- What are the causes behind the rise in atheism/ agnosticism in the United States?
- What were the influences in Siddhartha's life resulted in him becoming the Buddha?
- How has media portrayal of Islam/Muslims changed since September 11th?
Science/Environment
- How has the earth's climate changed in the past few decades?
- How has the use and elimination of DDT affected bird populations in the US?
- Analyze how the number and severity of natural disasters have increased in the past few decades.
- Analyze deforestation rates in a certain area or globally over a period of time.
- How have past oil spills changed regulations and cleanup methods?
- How has the Flint water crisis changed water regulation safety?
- What are the pros and cons of fracking?
- What impact has the Paris Climate Agreement had so far?
- What have NASA's biggest successes and failures been?
- How can we improve access to clean water around the world?
- Does ecotourism actually have a positive impact on the environment?
- Should the US rely on nuclear energy more?
- What can be done to save amphibian species currently at risk of extinction?
- What impact has climate change had on coral reefs?
- How are black holes created?
- Are teens who spend more time on social media more likely to suffer anxiety and/or depression?
- How will the loss of net neutrality affect internet users?
- Analyze the history and progress of self-driving vehicles.
- How has the use of drones changed surveillance and warfare methods?
- Has social media made people more or less connected?
- What progress has currently been made with artificial intelligence ?
- Do smartphones increase or decrease workplace productivity?
- What are the most effective ways to use technology in the classroom?
- How is Google search affecting our intelligence?
- When is the best age for a child to begin owning a smartphone?
- Has frequent texting reduced teen literacy rates?
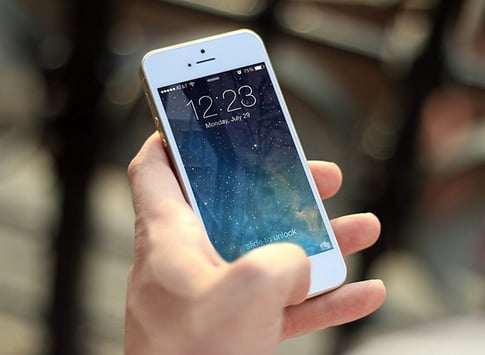
How to Write a Great Research Paper
Even great research paper topics won't give you a great research paper if you don't hone your topic before and during the writing process. Follow these three tips to turn good research paper topics into great papers.
#1: Figure Out Your Thesis Early
Before you start writing a single word of your paper, you first need to know what your thesis will be. Your thesis is a statement that explains what you intend to prove/show in your paper. Every sentence in your research paper will relate back to your thesis, so you don't want to start writing without it!
As some examples, if you're writing a research paper on if students learn better in same-sex classrooms, your thesis might be "Research has shown that elementary-age students in same-sex classrooms score higher on standardized tests and report feeling more comfortable in the classroom."
If you're writing a paper on the causes of the Civil War, your thesis might be "While the dispute between the North and South over slavery is the most well-known cause of the Civil War, other key causes include differences in the economies of the North and South, states' rights, and territorial expansion."
#2: Back Every Statement Up With Research
Remember, this is a research paper you're writing, so you'll need to use lots of research to make your points. Every statement you give must be backed up with research, properly cited the way your teacher requested. You're allowed to include opinions of your own, but they must also be supported by the research you give.
#3: Do Your Research Before You Begin Writing
You don't want to start writing your research paper and then learn that there isn't enough research to back up the points you're making, or, even worse, that the research contradicts the points you're trying to make!
Get most of your research on your good research topics done before you begin writing. Then use the research you've collected to create a rough outline of what your paper will cover and the key points you're going to make. This will help keep your paper clear and organized, and it'll ensure you have enough research to produce a strong paper.
What's Next?
Are you also learning about dynamic equilibrium in your science class? We break this sometimes tricky concept down so it's easy to understand in our complete guide to dynamic equilibrium .
Thinking about becoming a nurse practitioner? Nurse practitioners have one of the fastest growing careers in the country, and we have all the information you need to know about what to expect from nurse practitioner school .
Want to know the fastest and easiest ways to convert between Fahrenheit and Celsius? We've got you covered! Check out our guide to the best ways to convert Celsius to Fahrenheit (or vice versa).
These recommendations are based solely on our knowledge and experience. If you purchase an item through one of our links, PrepScholar may receive a commission.
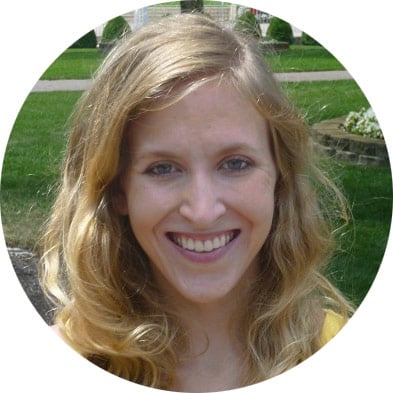
Christine graduated from Michigan State University with degrees in Environmental Biology and Geography and received her Master's from Duke University. In high school she scored in the 99th percentile on the SAT and was named a National Merit Finalist. She has taught English and biology in several countries.
Student and Parent Forum
Our new student and parent forum, at ExpertHub.PrepScholar.com , allow you to interact with your peers and the PrepScholar staff. See how other students and parents are navigating high school, college, and the college admissions process. Ask questions; get answers.

Ask a Question Below
Have any questions about this article or other topics? Ask below and we'll reply!
Improve With Our Famous Guides
- For All Students
The 5 Strategies You Must Be Using to Improve 160+ SAT Points
How to Get a Perfect 1600, by a Perfect Scorer
Series: How to Get 800 on Each SAT Section:
Score 800 on SAT Math
Score 800 on SAT Reading
Score 800 on SAT Writing
Series: How to Get to 600 on Each SAT Section:
Score 600 on SAT Math
Score 600 on SAT Reading
Score 600 on SAT Writing
Free Complete Official SAT Practice Tests
What SAT Target Score Should You Be Aiming For?
15 Strategies to Improve Your SAT Essay
The 5 Strategies You Must Be Using to Improve 4+ ACT Points
How to Get a Perfect 36 ACT, by a Perfect Scorer
Series: How to Get 36 on Each ACT Section:
36 on ACT English
36 on ACT Math
36 on ACT Reading
36 on ACT Science
Series: How to Get to 24 on Each ACT Section:
24 on ACT English
24 on ACT Math
24 on ACT Reading
24 on ACT Science
What ACT target score should you be aiming for?
ACT Vocabulary You Must Know
ACT Writing: 15 Tips to Raise Your Essay Score
How to Get Into Harvard and the Ivy League
How to Get a Perfect 4.0 GPA
How to Write an Amazing College Essay
What Exactly Are Colleges Looking For?
Is the ACT easier than the SAT? A Comprehensive Guide
Should you retake your SAT or ACT?
When should you take the SAT or ACT?
Stay Informed

Get the latest articles and test prep tips!
Looking for Graduate School Test Prep?
Check out our top-rated graduate blogs here:
GRE Online Prep Blog
GMAT Online Prep Blog
TOEFL Online Prep Blog
Holly R. "I am absolutely overjoyed and cannot thank you enough for helping me!”
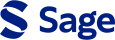
- Request new password
- Create a new account
Marketing Research: Planning, Process, Practice
Student resources, multiple choice quizzes.
Try these quizzes to test your understanding.
1. Research analysis is the last critical step in the research process.
2. The final research report where a discussion of findings and limitations is presented is the easiest part for a researcher.
3. Two different researchers may be presented with the same data analysis results and discuss them differently, uncovering alternative insights linked to the research question, each using a different lens.
4. A reliable research is essentially valid, but a valid research is not necessarily reliable.
5. A valid research refers to the degree to which it accurately measures what it intends to measure.
6. Keeping an envisioned original contribution to knowledge in mind, the research report in appearance and content should highlights the outcomes and link back to objectives.
7. A good conclusion chapter should (please select ALL answers that apply) ______.
- have a structure that brings back what the research set out to do
- discuss the researcher’s own assumptions and ideas about the topic under study
- makes logical links between the various parts of the arguments starting from the hypotheses
Answer: A & C
8. Research implications presented in a study must be either theoretical only or practical only.
9. Good researchers should aim for a perfect research, with no limitations or restrictions.
10. Examples of research limitations include (please select the answer that DOESN’T apply) ______.
- access to the population of interest
- the study’s coverage of possible contributory factors
- the researcher’s poor analysis skills
- the sampling technique used
11. A good structure outlining an effective research report starts with the ‘Analysis and Results’ section.
12. A good research study can just focus on its key outcomes without highlighting areas for future research.
13. If some of the research questions were not answered or some research objectives could not be achieved, then the final report must explain and reflect on the reasons why this is the case.
14. The importance of being critically reflective in presenting the future research section is that it allows for the advent of new arenas of thought that you or other researchers can develop on.
15. A weak future research section and weak discussion of the research limitations does not make the study fragile/lacking rigour and depth.
16. Once a research specifies a study’s limitations, this discredits all research efforts exerted in it.
17. Reporting research is about presenting the research journey through clear and evidence-based arguments of design, process and outcomes, not just describing it.
18. It is not important to present in every research report the ethical considerations that were anticipated or have ascended in the study.
19. Verbal and visual presentations of research aid in the dissemination of its outcomes and value, and allow for its strengths to be revealed.
20. In oral presentations, the audience expects you as a researcher to present your work in full detail even if they will ask further questions in the follow-up discussion.
Scientific Writing: Peer Review and Scientific Journals
by Natalie H. Kuldell
- Peer review can best be summarized as: a process for evaluating the safety of boat docks. a process by which independent scientists evaluate the technical merit of scientific research papers. a process by which a scientist's friends can give him or her advice. a method of typesetting in publishing.
- The process of peer review always ensures that a scientific paper is correct. true false
- One of the main purposes for including a "Materials and Methods" section in a paper is: to advertise scientific products. to demonstrate that your methods are superior to other scientists' methods. to allow other scientists to reproduce your findings. for no reason; most journals do not require this section.
- The main purpose of a "References" section in a scientific paper: is to acknowledge your colleagues who gave you advice. is to present other papers that the reader might want to consult. is to provide a list of scientists who have repeated your research. is to acknowledge research and concepts upon which your work builds.
- Tables and figures are used in a scientific paper to present and explain research results. true false
- Often, one of the best places to start reading an article is: at the end, in the "Discussion" section. at a random spot in the middle of the article. in the "Materials and Methods" section. in the "References" section.
Have a language expert improve your writing
Run a free plagiarism check in 10 minutes, generate accurate citations for free.
- Knowledge Base
- Starting the research process
- 10 Research Question Examples to Guide Your Research Project
10 Research Question Examples to Guide your Research Project
Published on October 30, 2022 by Shona McCombes . Revised on October 19, 2023.
The research question is one of the most important parts of your research paper , thesis or dissertation . It’s important to spend some time assessing and refining your question before you get started.
The exact form of your question will depend on a few things, such as the length of your project, the type of research you’re conducting, the topic , and the research problem . However, all research questions should be focused, specific, and relevant to a timely social or scholarly issue.
Once you’ve read our guide on how to write a research question , you can use these examples to craft your own.
Note that the design of your research question can depend on what method you are pursuing. Here are a few options for qualitative, quantitative, and statistical research questions.
Other interesting articles
If you want to know more about the research process , methodology , research bias , or statistics , make sure to check out some of our other articles with explanations and examples.
Methodology
- Sampling methods
- Simple random sampling
- Stratified sampling
- Cluster sampling
- Likert scales
- Reproducibility
Statistics
- Null hypothesis
- Statistical power
- Probability distribution
- Effect size
- Poisson distribution
Research bias
- Optimism bias
- Cognitive bias
- Implicit bias
- Hawthorne effect
- Anchoring bias
- Explicit bias
Cite this Scribbr article
If you want to cite this source, you can copy and paste the citation or click the “Cite this Scribbr article” button to automatically add the citation to our free Citation Generator.
McCombes, S. (2023, October 19). 10 Research Question Examples to Guide your Research Project. Scribbr. Retrieved April 10, 2024, from https://www.scribbr.com/research-process/research-question-examples/
Is this article helpful?
Shona McCombes
Other students also liked, writing strong research questions | criteria & examples, how to choose a dissertation topic | 8 steps to follow, evaluating sources | methods & examples, "i thought ai proofreading was useless but..".
I've been using Scribbr for years now and I know it's a service that won't disappoint. It does a good job spotting mistakes”
QuizFun: Mobile based quiz game for learning
Ieee account.
- Change Username/Password
- Update Address
Purchase Details
- Payment Options
- Order History
- View Purchased Documents
Profile Information
- Communications Preferences
- Profession and Education
- Technical Interests
- US & Canada: +1 800 678 4333
- Worldwide: +1 732 981 0060
- Contact & Support
- About IEEE Xplore
- Accessibility
- Terms of Use
- Nondiscrimination Policy
- Privacy & Opting Out of Cookies
A not-for-profit organization, IEEE is the world's largest technical professional organization dedicated to advancing technology for the benefit of humanity. © Copyright 2024 IEEE - All rights reserved. Use of this web site signifies your agreement to the terms and conditions.
- Open access
- Published: 18 March 2021
Automatic question generation and answer assessment: a survey
- Bidyut Das ORCID: orcid.org/0000-0002-8588-1913 1 ,
- Mukta Majumder 2 ,
- Santanu Phadikar 3 &
- Arif Ahmed Sekh 4
Research and Practice in Technology Enhanced Learning volume 16 , Article number: 5 ( 2021 ) Cite this article
31k Accesses
36 Citations
8 Altmetric
Metrics details
Learning through the internet becomes popular that facilitates learners to learn anything, anytime, anywhere from the web resources. Assessment is most important in any learning system. An assessment system can find the self-learning gaps of learners and improve the progress of learning. The manual question generation takes much time and labor. Therefore, automatic question generation from learning resources is the primary task of an automated assessment system. This paper presents a survey of automatic question generation and assessment strategies from textual and pictorial learning resources. The purpose of this survey is to summarize the state-of-the-art techniques for generating questions and evaluating their answers automatically.
Introduction
Online learning facilitates learners to learn through the internet via a computer or other digital device. Online learning is classified into three general categories depends on the learning materials: textual learning, visual learning, and audio-video learning. Online learning needs two things: the learning resources and the assessment of learners from the learning resources. The learning resources are available, and learners can able to learn from many sources on the web. On the other hand, the manual questions from the learning materials are required for the learner’s assessment. To the best of our knowledge, no generic assessment system has been proposed in the literature to test the learning gap of learners from the e-reading documents. Therefore, automatic question generation and evaluation strategies can help to automate the assessment system. This article presents several techniques for automatic question generation and their answer assessment. The main contributions of this article are as follows:
This article at first presents a few survey articles that are available in this research area. Table 1 lists the majority of the existing review articles, which described several approaches for question generation. Table 2 presents the survey articles on learner’s answer evaluation techniques.
The second contribution is to summarize the related existing datasets. We also critically analyzed various purposes and limitations of the use of these datasets.
The third contribution is to discuss and summarize the existing and possible question generation methods with corresponding evaluation techniques used to automate the assessment system.
The arrangement of the rest of the article is as follows. In the “ Question Generation and Learner’s Assessment ” section, we describe the overview of question generation and assessment techniques. The “ Related datasets ” section describes the datasets used by researchers for different applications. The “ Objective Question Generation ” section presents the different types of objective question generation techniques. In the “ Subjective Question Generation and Evaluation ” section, we illustrate the existing methods of subjective question generation and their answer evaluation. The “ Visual Question-Answer Generation ” section describes methods of image-based question and answer generation. Finally, we present a few challenges in the “ Challenges in Question Generation and Answer Assessment ” section and conclude the paper in the “ Conclusion ” section.
Question Generation and Learner’s Assessment
Automatic question generation (AQG) performs a significant role in educational assessment. Handmade question creation takes much labor, time and cost, and manual answer assessment is also a time-consuming task. Therefore, to build an automatic system has attracted the attention of researchers in the last two decades for generating questions and evaluating the answers of learners ( Divate and Salgaonkar 2017 ). All question types are broadly divided into two groups: objective question and subjective question. The objective-question asks learners to pick the right answer from two to four alternative options or provides a word/multiword to answer a question or to complete a sentence. Multiple-choice, matching, true-false, and fill-in-the-blank are the most popular assessment items in education ( Boyd 1988 ). On the other side, the subjective question requires an answer in terms of explanation that allows the learners to compose and write a response in their own words. The two well-known examples of the subjective question are short-answer type question and long-answer type question ( Clay 2001 ). The answer to a short question requires a sentence or two to three sentences, and a long-type question needs more than three sentences or paragraphs. However, both subjective and objective questions are necessary for good classroom test ( Heaton 1990 ). Figure 1 shows the overall diagram of different question generation and answer evaluation methods for automatic assessment system. We initially categorized the online learning techniques into three different types, namely text-based, audio and video-based, and image-based. We emphasized mainly text-based approaches and further extended the modality towards assessment methods. We discussed audio-video and image-based learning in this article, but the extensive analysis of such learning methods is out of the scope of this article.
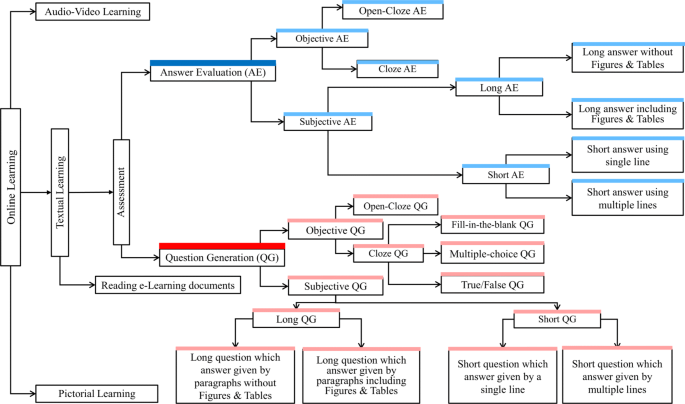
Different modalities of question generation and assessment methods reported in literature
The objective question becomes popular as an automated assessment tool in the examination system due to its fast and reliable evaluation policies ( Nicol 2007 ). It involves the binary mode of assessment that has only one correct answer. On the other side, the subjective examination has obtained the attention of the evaluators to evaluate a candidate’s deep knowledge and understanding of the traditional education system for centuries ( Shaban 2014 ). Individually, each university has followed different patterns of subjective examination. Due to the rapid growth of e-learning courses, we need to consider such assessments and evaluations done by the automated appraisal system. The computer-based assessment of subjective questions is challenging, and the accuracy of it has not achieved adequate results. Hopefully, the research on automatic evaluation of subjective-questions in examination discovers new tools to help schools and teachers. An automated tool can able to resolve the problem of hand-scoring thousands of written answers in the subjective-examination. Today’s computer-assisted examination excludes the subjective-questions by MCQs, which are not able to assess the writing skills and critical reasoning of the students due to its unreliable accuracy of evaluation. Table 3 shows the different types of questions and compares the level of difficulties to generate questions and evaluate the learner’s answers.
ACL, IEEE, Mendeley, Google Scholar, and Semantic Scholar are searched to collect high-quality journals and conferences for this survey. The search has involved a combination and variation of the keywords such as automatic question generation, multiple-choice questions generation, cloze questions generation, fill-in-the-blank questions generation, visual question generation, subjective answer evaluation, short answer evaluation, and short answer grading. A total of 78 articles are included in this study. Figure 2 shows the statistics of articles for different question generation and learners’ answer evaluation that found in the last 10 years in the literature.
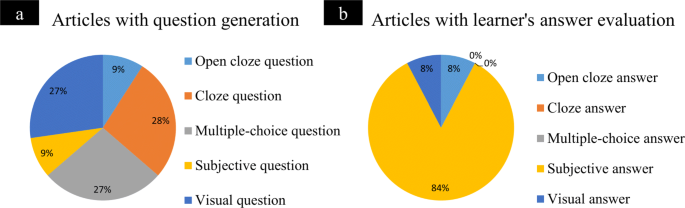
a Statistics of question generation articles appeared in the last decade. b Statistics of answer evaluation articles appeared in the last decade
Related datasets
In 2010, a question generation system QGSTEC used a dataset that contains overall 1000 questions (generated by both humans and machines). The system generated a few questions for each question type (which, what, who, when, where, and how many). Five fixed criteria were used to measure the correctness of the generated questions—relevance, question type, grammatically correct, and ambiguity. Both the relevancy and the syntactic correctness measures did not score well. The agreement between the two human judges was quite low.
The datasets SQuAD, 30MQA, MS MARCO, RACE, NewsQA, TriviaQA, and NarrativeQA contain question-answer pairs and are mainly developed for machine-reading comprehension or question answering models. These datasets are not designed for direct question-generation from textual documents. The datasets are also not suited for educational assessment due to their limited number of topics or insufficient information for generating questions and further answer the questions.
TabMCQ dataset contains large scale crowdsourced MCQs covering the facts in the tables. This dataset is designed for not only the task of question answering but also information extraction, question parsing, answer-type identification, and lexical-semantic modeling. The facts of the tables are not adequate to generate MCQs. The SciQ dataset also consists of a large set of crowdsourced MCQs with distractors and an additional passage that provides the clue for the correct answer. This passage does not contain sufficient information to generate MCQs or distractors. Therefore, both the TabMCQ and SciQ datasets are not applicable for multiple-choice question generation as well as distractors generation.
MCQL dataset is designed for automatic distractors generation. Each MCQ associates with four fields: sentence, answer, distractors, and the number of distractors. We observed that the sentence is not sufficient for generating MCQs for all times. The dataset does not include the source text from where it collects the MCQs and distractors. Distractors not only depend on the question, sentence, and correct answer but also the source text. Therefore, the MCQL dataset is not applicable when it needs to generate questions, answers, and distractors from the same source text or study materials.
LearningQ dataset covers a wide range of learning subjects as well as the different levels of cognitive complexity and contains a large-set of document-question pairs and multiple source sentences for question generation. The dataset decreases the performance of question generation when the length of source sentences increases. Therefore, the dataset is helpful to forward the research on automatic question generation in education.
Table 4 presents the existing datasets which contain question-answer pairs and related to question-answer generation. Table 5 includes the detail description of each dataset.
Objective Question Generation
The study of literature review shows that most of the researchers paid attention to generate objective-type questions, automatically or semi-automatically. They confined their works to generate multiple-choice or cloze questions. A limited number of approaches are found in the literature that shows interest in open-cloze question generation.
Pino and Eskenazi (2009 ) provided the hint in an open-cloze question. They noted the first few letters of a missing word gave a clue about the missing word. Their goal was to vary the number of letters in hint to change the difficulty level of questions that facilitate the students to learn vocabulary. Agarwal (2012) developed an automated open-cloze question generation method. Their approach composed of two steps—selected relevant and informative sentences and identified keywords from the selected sentences. His proposed system had taken cricket-news articles as input and generated factual open-cloze questions as output. Das and Majumder (2017) described a system for open-cloze question generation to evaluate the factual knowledge of learners. They computed the evaluation score using a formula that depends on the number of hints used by the learners to give the right answers. The multiword answer to the open-cloze question makes the system more attractive.
Coniam (1997 ) proposed one of the oldest techniques of cloze test item generation. He applied word frequencies to analyze the corpus in various phases of development, such as obtain the keys for test items, generate test item alternatives, construct cloze test items, and identify good and bad test items. He matched word frequency and parts-of-speech of each test item key with a similar word class and word frequency to construct test items. Brown et al. (2005) revealed an automated system to generate vocabulary questions. They applied WordNet ( Miller 1995 ) for obtaining the synonym, antonym, and hyponym to develop the question key and the distractors. Chen et al. (2006) developed a semi-automated method using NLP techniques to generate grammatical test items. Their approach implied handcraft patterns to find authentic sentences and distractors from the web that transform into grammar-based test items. Their experimental results showed that the method had generated 77% meaningful questions. Hoshino and Nakagawa (2007) introduced a semi-automated system to create cloze test items from online news articles to help teachers. Their test items removed one or more words from a passage, and learners were asked to fill those omitted words. Their system generated two types of distractors: grammatical distractors and vocabulary distractors. The human-based evaluation revealed that their system produced 80% worthy cloze test items. Pino et al. (2008) employed four selection criteria: well-defined context, complexity, grammaticality, and length to give a weighted score for each sentence. They selected a sentence as informative if the score was higher than a threshold for generating a cloze question. Agarwal and Mannem (2011) presented a method to create gap-fill-questions from a biological-textbook. The authors adopted several features to generate the questions: sentence length, the sentence position in a document, is it the first sentence, is the sentence contains token that appears in the title, the number of nouns and pronouns in the sentence, is it holds abbreviation or superlatives. They did not report the optimum value of these features or any relative weight among features or how the features combined. Correia et al. (2012) applied supervised machine learning to select stem for cloze questions. They employed several features to run the classifier of SVM: the length of sentence, the position of the word in a sentence, the chunk of the sentence, verb, parts-of-speech, named-entity, known-word, unknown-word, acronym, etc. Narendra et al. (2013) directly employed a summarizer (MEAD) Footnote 1 to select the informative sentences for automatic CQs generation. Flanagan et al. (2013) described an automatic-method for generating multiple-choice and fill-in-the-blanks e-Learning quizzes.
Mitkov et al. (2006 ) proposed a semi-automated system for generating MCQs from a linguistic-textbook. They employed several NLP approaches for question generation—shallow parsing, key term extraction, semantic distance, sentence transformation, and ontology such as WordNet. Aldabe et al. 2010 presented a system to generate MCQ in the Basque language. They suggested different methods to find semantic similarities between the right answer and its distractors. A corpus-based strategy was applied to measure the similarities. Papasalouros et al. (2008) revealed a method to generate MCQs from domain ontologies. Their experiment used five different domain ontologies for multiple-choice question generation. Bhatia et al. (2013) developed a system for automatic MCQ generation from Wikipedia. They proposed a potential sentence selection approach using the pattern of existing questions on the web. They also suggested a technique for generating distractors using the named entity. Majumder and Saha (2014) applied named entity recognition and syntactic structure similarity to select sentences for MCQ generation. Majumder and Saha (2015) alternately used topic modeling and parse tree structure similarity to choose informative sentences for question formation. They picked the keywords using topic-word and named-entity and applied a gazetteer list-based approach to select distractors.
Subjective Question Generation and Evaluation
Limited research works found in the literature that focused on subjective question generation. Rozali et al. (2010) presented a survey of dynamic question generation and qualitative evaluation and a description of related methods found in the literature. Dhokrat et al. (2012) proposed an automatic system for subjective online examination using a taxonomy that coded earlier into the system. Deena et al. (2020) suggested a question generation method using NLP and bloom’s taxonomy that generated subjective questions dynamically and reduced the occupation of memory.
Proper scoring is the main challenge of subjective assessment. Therefore, automatic subjective-answer evaluation is a current trend of research in the education system ( Burrows et al. 2015 ). It reduces the assessment time and effort in the education system. Objective-type answer evaluation is easy and requires a binary mode of assessment (true/false) to test the correct option. But, the subjective answer evaluation does not achieve adequate results due to its complex nature. The next paragraph discusses some related works of subjective-answer evaluation and grading techniques.
Leacock and Chodorow (2003 ) proposed an answer grading system C-rater that deals with semantic information of the text. They adopted a method to recognize paraphrase to grad the answers. Their approach achieved 84% accuracy with the manual evaluation of human graders. Bin et al. (2008) employed the K-nearest neighbor (KNN) classifier for automated essay scoring using the text categorization model. The Vector Space Model was used to express each essay. They used words, phrases, and arguments as essay features and represented each vector using the TF-IDF weight. The cosine similarity was applied to calculate the score of essays and achieved 76% average accuracy using the different methods of feature selection, such as term frequency (TF), term frequency-inverse document frequency (TF-IDF), and information gain (IG). Kakkonen et al. (2008) recommended an automatic essay grading system that compares learning materials with the teacher graded essays using three methods: Latent Semantic Analysis (LSA), Probabilistic LSA (PLSA), and Latent Dirichlet Allocation (LDA). Their system performed better than the k-NN based grading system. Noorbehbahani and Kardan (2011) introduced a method for judging free text answers of students using a modified Bilingual Evaluation Understudy (M-BLEU) algorithm. The M-BLEU recognized the most similar reference answer to a student answer and estimated a score to judge the answers. Their method achieved higher accuracy than the other evaluation methods, like latent semantic analysis and n-gram co-occurrence. Dhokrat et al. (2012) proposed an appraisal system for evaluating the student’s answer. The system used a centralized file that includes the model answer with the reference material for each question. Their system found overall 70% accuracy. Islam and Hoque (2010) presented an automatic essay grading system using the generalized latent semantic analysis (GLSA). The GLSA based system used word-ordering in the sentences by including the word n-gram for grading essays. The GLSA based system performs better than the LSA-based grading system and overcomes the limitations of the LSA based system, where the LSA does not consider word-order of sentences in a document. Ramachandran et al. (2015) described a unique technique for scoring short answers. They introduced word ordering graphs to recognize the useful patterns from handcraft rubric texts and the best responses of students. The method also employed semantic metrics to manage related-words for alternative answer options. Sakaguchi et al. (2015) used different sources of information for scoring content-based short answers. Their approach extracted features from the responses (word and character n-grams). Their reference-based method found the similarity between the response features with the information from the scoring guidelines. Their model outperformed when the training data is limited.
Recent progress in deep learning-based NLP has also shown a promising future in answer assessment. Sentiment-based assessment techniques Nassif et al. 2020 ; Abdi et al 2019 used in many cases because of the generalized representation of sentiment in NLP. The success of recurrent neural networks (RNN) such as Long short-term memory (LSTM) becomes popular in sequence analysis and applied in various answer assessment ( Du et al. 2017 ; Klein and Nabi 2019 ).
Visual Question-Answer Generation
Recently, question generation has been included in the field of computer vision to generate image-based questions ( Gordon et al. 2018 ; Suhr et al. 2019 ; Santoro et al. 2018 ). The most recent approaches use human-annotated question-answer pairs to train machine learning algorithms for generating multiple questions per image, which were labor-intensive and time-consuming ( Antol et al. 2015 ; Gao et al. 2015 ). One of the recent examples, Zhu et al. 2016 manually created seven wh-type questions such as when, where, and what. People also investigated automatic visual question generation by using rules. Yu et al. (2015) proposed the question generation as a task of removing a content word (answer) from an image caption and reforms the caption sentence as a question. Similarly, Ren et al. 2015 suggested a rule to reformulate image captions into limited types of questions. Some considered model-based methods to overwhelm the diversity issue of question types. Simoncelli and Olshausen (2001) trained a model using a dataset of image captions and respective visual questions. But, their model could not generate multiple questions per image. Mora et al. (2016) proposed an AI model to generate image-based questions with respective answers simultaneously. Mostafazadeh et al. (2016) collected the first visual question generation dataset, where their model generated several questions per image. Zhang et al. (2017) proposed an automatic model for generating several visually grounded questions from a single image. Johnson et al. (2016) suggested a framework named Densecap for generating region captions, which are the additional information to supervise the question generation. Jain et al. (2017) combined the variational auto-encoders and LSTM networks to generate numerous types of questions from a given image. The majority of these image-based question-answers were related to image understanding and reasoning in real-world images.
Visual Question-Answer Dataset
Figure 3 a shows a few examples where various pattern identification and reasoning tests used synthetic images. Johnson et al. (2017) proposed a diagnostic dataset CLEVR, which has a collection of 3D shapes and used to test the skill of visual reasoning. The dataset is used for question-answering about shapes, positions, and colors. Figure 3 b presents Raven progressive matrices based visual-reasoning that is used to test shape, count, and relational visual reasoning from an image sequence ( Bilker et al. 2012 ). Figure 3 c is an example of NLVR dataset. The dataset used the concepts of 2D shapes and color to test visual reasoning. The dataset is used to generate questions related to the knowledge of shape, size, and color. Figure 3 d is an example of visual question answering dataset (VQA). The dataset consists of a large volume of real-world images and is used to generate questions and corresponding answers related to objects, color, and counting. Figure 3 e is also a similar dataset related to event and actions. All these datasets are used to generate image-specific questions and also used in various assessments.
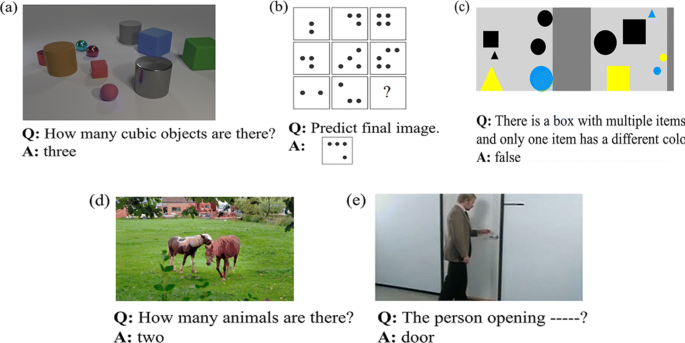
Different datasets and questions used in visual question answering. a CLEVR ( Johnson et al. 2017 ) dataset, b abstract reasoning dataset ( Santoro et al. 2018 ), c NLVR ( Suhr et al. 2019 ) dataset, d VQA ( Antol et al. 2015 ) dataset, and e IQA ( Gordon et al. 2018 ) dataset
Challenges in Question Generation and Answer Assessment
Informative-simple-sentence extraction.
Questions mainly depend on informative sentences. An informative-sentence generates a quality question to assess learners. We found that text-summarization, sentence-simplification, and some rule-based techniques in the literature exacted the informative-sentences from an input text. Most of the previous articles did not focus adequately on the step of informative-sentence selection. But it is a useful-step for generating quality questions. Generate simple-sentences from complex and compound sentences are also complex. A simple-sentence eliminates the ambiguity between multiple answers to a particular question. Therefore, a generic technique is needed to extract the informative-simple-sentences from the text for generating questions ( Das et al. 2019 ). The popular NLP packages like NLTK, spaCy, PyNLPl, and CoreNLP did not include any technique for extracting informative-sentences from a textual document. It is a future direction of research to incorporate it into the NLP packages.
Question generation from multiple sentences
Different question generation techniques generate different questions that assess the knowledge of learners in different ways. An automated system generates questions from study material or learning content based on informative keywords or sentences and multiple sentences or a passage. Generate questions from multiple sentences or a paragraph is difficult and consider a new research direction for automatic question generation. It requires the inner relation between sentences using natural language understanding concepts.
Short and long-type answer assessment
We found many works in the last decade for automatic grading short answers or free-text answers. But the unreliable results of previous research indicates that it is not practically useful in real life. Therefore, most of the exams conduct using MCQs and exclude the short type and long type answers. We found only one research that evaluates long-answers in the literature. Therefore, future research expects a reliable and real-life system for short answer grading as well as long type answer evaluation that fully automate the education system.
Answer assessment standard
Question generation and assessment depend on many factors such as learning domain, type of questions for assessments, difficulty level, question optimization, scoring techniques, and overall scoring. Several authors proposed different evaluation techniques depend on their application, and the scoring scale is also different. Therefore, an answer assessment standard is required in the future to evaluate and compare the learner’s knowledge and compare the research results.
Question generation and assessment from video lectures
We found that the majority of question generation and assessment systems focus on generating questions from the textual document to automate the education system. We found a limited number of works in the literature that generate questions from the visual content for the learner’s assessment. Assessment from video lectures by generating questions from video content is a future research direction. Audio-video content improves the learning process ( Carmichael et al. 2018 ). Automated assessments from video content can help learners to learn quickly in a new area.
Question generation and assessment using machine learning
Due to the many advantages of the machine learning method, recent works focus on it to generate questions and evaluate answers. Most of the textual question generation used natural language processing (NLP) techniques. The advancement of NLP is natural language understanding (NLU) and natural language generation (NLG) that used a deep learning neural network ( Du et al. 2017 ; Klein and Nabi 2019 ). The visual question generation method mainly used machine learning to generate image captions. Image caption translates into a question using NLP techniques. VQG is a combined application of computer vision and NLP. In some articles used sequence-to-sequence modeling for generating questions. Limited works found in the literature that assess the learners using a machine learning approach. More research works need to focus on this area in the future.
Due to the advances in online learning, automatic question generation and assessment are becoming popular in the intelligent education system. The article first includes a collection of review articles in the last decade. Next, it discusses the state-of-the-art methods of various automatic question generation as well as different assessment techniques that summarizes the progress of research. It also presents a summary of related existing datasets found in the literature. This article critically analyzed the methods of objective question generation, subjective question generation with the learner’s response evaluation, and a summarizing of visual question generation methods.
Availability of data and materials
Not applicable
http://www.summarization.com/mead/
Abbreviations
Artificial intelligence
Automatic question generation
Bilingual evaluation understudy
Generalized latent semantic analysis
K-nearest neighbor
Latent Dirichlet allocation
Latent semantic analysis
Long short term memory
Multiple choice question
Natural language processing
Probabilistic latent semantic analysis
Term frequency-inverse document frequency
Visual question answering
Visual question generation
Abdi, A., Shamsuddin, S.M., Hasan, S., Piran, J. (2019). Deep learning-based sentiment classification of evaluative text based on multi-feature fusion. Information Processing & Management , 56 (4), 1245–1259.
Article Google Scholar
Agarwal, M. (2012). Cloze and open cloze question generation systems and their evaluation guidelines. Master’s thesis. International Institute of Information Technology, (IIIT), Hyderabad, India .
Agarwal, M., & Mannem, P. (2011). Automatic gap-fill question generation from text books. In Proceedings of the 6th Workshop on Innovative Use of NLP for Building Educational Applications . Association for Computational Linguistics, Portland, (pp. 56–64).
Google Scholar
Aldabe, I., & Maritxalar, M. (2010). Automatic distractor generation for domain specific texts. In Proceedings of the 7th International Conference on Advances in Natural Language Processing . Springer-Verlag, Berlin, (pp. 27–38).
Chapter Google Scholar
Alruwais, N., Wills, G., Wald, M. (2018). Advantages and challenges of using e-assessment. International Journal of Information and Education Technology , 8 (1), 34–37.
Amidei, J., Piwek, P., Willis, A. (2018). Evaluation methodologies in automatic question generation 2013-2018. In Proceedings of The 11th International Natural Language Generation Conference . Association for Computational Linguistics, Tilburg University, (pp. 307–317).
Antol, S., Agrawal, A., Lu, J., Mitchell, M., Batra, D., Lawrence Zitnick, C., Parikh, D. (2015). Vqa: Visual question answering. In Proceedings of the IEEE International Conference on Computer Vision , (pp. 2425–2433).
Bhatia, A.S., Kirti, M., Saha, S.K. (2013). Automatic generation of multiple choice questions using wikipedia. In Proceedings of the Pattern Recognition and Machine Intelligence . Springer-Verlag, Berlin, (pp. 733–738).
Bilker, W.B., Hansen, J.A., Brensinger, C.M., Richard, J., Gur, R.E., Gur, R.C. (2012). Development of abbreviated nine-item forms of the Raven’s standard progressive matrices test. Assessment , 19 (3), 354–369.
Bin, L., Jun, L., Jian-Min, Y., Qiao-Ming, Z. (2008). Automated essay scoring using the KNN algorithm. In 2008 International Conference on Computer Science and Software Engineering , (Vol. 1. IEEE, Washington, DC, pp. 735–738).
Boyd, R.T. (1988). Improving your test-taking skills. Practical Assessment, Research & Evaluation , 1 (2), 3.
Brown, J.C., Frishkoff, G.A., Eskenazi, M. (2005). Automatic question generation for vocabulary assessment. In Proceedings of the Conference on Human Language Technology and Empirical Methods in Natural Language Processing . Association for Computational Linguistics, Vancouver, (pp. 819–826).
Burrows, S., Gurevych, I., Stein, B. (2015). The eras and trends of automatic short answer grading. International Journal of Artificial Intelligence in Education , 25 (1), 60–117.
Carmichael, M., Reid, A., Karpicke, J.D. (2018). Assessing the impact of educational video on student engagement, critical thinking and learning: The Current State of Play , (pp. 1–21): A SAGE Whitepaper, Sage Publishing.
Ch, D.R., & Saha, S.K. (2018). Automatic multiple choice question generation from text: A survey. IEEE Transactions on Learning Technologies , 13 (1), 14–25. https://doi.org/10.1109/TLT.2018.2889100 .
Chen, C.-Y., Liou, H.-C., Chang, J.S. (2006). Fast–an automatic generation system for grammar tests. In Proceedings of the COLING/ACL on Interactive Presentation Sessions . Association for Computational Linguistics, Sydney, (pp. 1–4).
Chen, G., Yang, J., Hauff, C., Houben, G.-J. (2018). Learningq: A large-scale dataset for educational question generation. In Twelfth International AAAI Conference on Web and Social Media , (pp. 481–490).
Clay, B. (2001). A short guide to writing effective test questions. Lawrence: Kansas Curriculum Center, University of Kansas . https://www.k-state.edu/ksde/alp/resources/Handout-Module6.pdf .
Coniam, D. (1997). A preliminary inquiry into using corpus word frequency data in the automatic generation of English language cloze tests. Calico Journal , 14 (2-4), 15–33.
Correia, R., Baptista, J., Eskenazi, M., Mamede, N. (2012). Automatic generation of cloze question stems. In Computational Processing of the Portuguese Language . Springer-Verlag, Berlin, (pp. 168–178).
Das, B., & Majumder, M. (2017). Factual open cloze question generation for assessment of learner’s knowledge. International Journal of Educational Technology in Higher Education , 14 (1), 1–12.
Das, B., Majumder, M., Phadikar, S., Sekh, A.A. (2019). Automatic generation of fill-in-the-blank question with corpus-based distractors for e-assessment to enhance learning. Computer Applications in Engineering Education , 27 (6), 1485–1495.
Deena, G., Raja, K., PK, N.B., Kannan, K. (2020). Developing the assessment questions automatically to determine the cognitive level of the E-learner using NLP techniques. International Journal of Service Science, Management, Engineering, and Technology (IJSSMET) , 11 (2), 95–110.
Dhokrat, A., Gite, H., Mahender, C.N. (2012). Assessment of answers: Online subjective examination. In Proceedings of the Workshop on Question Answering for Complex Domains , (pp. 47–56).
Divate, M., & Salgaonkar, A. (2017). Automatic question generation approaches and evaluation techniques. Current Science , 113 (9), 1683–1691.
Du, X., Shao, J., Cardie, C. (2017). Learning to Ask: Neural Question Generation for Reading Comprehension. In Proceedings of the 55th Annual Meeting of the Association for Computational Linguistics (Volume 1: Long Papers) . Association for Computational Linguistics, Vancouver, (pp. 1342–1352).
Flanagan, B., Yin, C., Hirokawa, S., Hashimoto, K., Tabata, Y. (2013). An automated method to generate e-learning quizzes from online language learner writing. International Journal of Distance Education Technologies (IJDET) , 11 (4), 63–80.
Gao, H., Mao, J., Zhou, J., Huang, Z., Wang, L., Xu, W. (2015). Are you talking to a machine? Dataset and methods for multilingual image question. In Advances in Neural Information Processing Systems , (pp. 2296–2304).
Gordon, D., Kembhavi, A., Rastegari, M., Redmon, J., Fox, D., Farhadi, A. (2018). Iqa: Visual question answering in interactive environments. In Proceedings of the IEEE Conference on Computer Vision and Pattern Recognition , (pp. 4089–4098).
Hasanah, U., Permanasari, A.E., Kusumawardani, S.S., Pribadi, F.S. (2016). A review of an information extraction technique approach for automatic short answer grading. In 2016 1st International Conference on Information Technology, Information Systems and Electrical Engineering (ICITISEE) . IEEE, Yogyakarta, (pp. 192–196).
Heaton, J.B. (1990). Classroom testing .
Hoshino, A., & Nakagawa, H. (2007). Assisting cloze test making with a web application. In Society for Information Technology & Teacher Education International Conference . Association for the Advancement of Computing in Education (AACE), Waynesville, NC USA, (pp. 2807–2814).
Islam, M.M., & Hoque, A.L. (2010). Automated essay scoring using generalized latent semantic analysis. In 2010 13th International Conference on Computer and Information Technology (ICCIT) . IEEE, Dhaka, (pp. 358–363).
Jain, U., Zhang, Z., Schwing, A.G. (2017). Creativity: Generating diverse questions using variational autoencoders. In Proceedings of the IEEE Conference on Computer Vision and Pattern Recognition , (pp. 6485–6494).
Jauhar, S.K., Turney, P., Hovy, E. (2015). TabMCQ: A Dataset of General Knowledge Tables and Multiple-choice Questions. https://www.microsoft.com/en-us/research/publication/tabmcq-a-dataset-of-general-knowledge-tables-and-multiple-choice-questions/ .
Johnson, J., Hariharan, B., van der Maaten, L., Fei-Fei, L., Lawrence Zitnick, C., Girshick, R. (2017). Clevr: A diagnostic dataset for compositional language and elementary visual reasoning. In Proceedings of the IEEE Conference on Computer Vision and Pattern Recognition , (pp. 2901–2910).
Johnson, J., Karpathy, A., Fei-Fei, L. (2016). Densecap: Fully convolutional localization networks for dense captioning. In Proceedings of the IEEE Conference on Computer Vision and Pattern Recognition , (pp. 4565–4574).
Joshi, M., Choi, E., Weld, D.S., Zettlemoyer, L. (2017). TriviaQA: A Large Scale Distantly Supervised Challenge Dataset for Reading Comprehension. In Proceedings of the 55th Annual Meeting of the Association for Computational Linguistics (Volume 1: Long Papers) . Association for Computational Linguistics, Vancouver, (pp. 1601–1611).
Kakkonen, T., Myller, N., Sutinen, E., Timonen, J. (2008). Comparison of dimension reduction methods for automated essay grading. Journal of Educational Technology & Society , 11 (3), 275–288.
Klein, T., & Nabi, M. (2019). Learning to Answer by Learning to Ask: Getting the Best of GPT-2 and BERT Worlds. ArXiv , abs/1911.02365 .
Kočiskỳ, T., Schwarz, J., Blunsom, P., Dyer, C., Hermann, K.M., Melis, G., Grefenstette, E. (2018). The narrativeqa reading comprehension challenge. Transactions of the Association for Computational Linguistics , 6 , 317–328.
Kurdi, G., Leo, J., Parsia, B., Sattler, U., Al-Emari, S. (2020). A systematic review of automatic question generation for educational purposes. International Journal of Artificial Intelligence in Education , 30 (1), 121–204.
Lai, G., Xie, Q., Liu, H., Yang, Y., Hovy, E. (2017). RACE: Large-scale ReAding Comprehension Dataset From Examinations. In Proceedings of the 2017 Conference on Empirical Methods in Natural Language Processing . Association for Computational Linguistics, Copenhagen, (pp. 785–794).
Le, N.-T., Kojiri, T., Pinkwart, N. (2014). Automatic question generation for educational applications–the state of art. In Advanced Computational Methods for Knowledge Engineering , (pp. 325–338).
Leacock, C., & Chodorow, M. (2003). C-rater: Automated scoring of short-answer questions. Computers and the Humanities , 37 (4), 389–405.
Liang, C., Yang, X., Dave, N., Wham, D., Pursel, B., Giles, C.L. (2018). Distractor generation for multiple choice questions using learning to rank. In Proceedings of the Thirteenth Workshop on Innovative Use of NLP for Building Educational Applications , (pp. 284–290).
Majumder, M., & Saha, S.K. (2014). Automatic selection of informative sentences: The sentences that can generate multiple choice questions. Knowledge Management and E-Learning: An International Journal , 6 (4), 377–391.
Majumder, M., & Saha, S.K. (2015). A system for generating multiple choice questions: With a novel approach for sentence selection. In Proceedings of the 2nd Workshop on Natural Language Processing Techniques for Educational Applications . Association for Computational Linguistics, Beijing, (pp. 64–72).
Miller, G.A. (1995). WordNet: A lexical database for English. Communications of the ACM , 38 (11), 39–41.
Mitkov, R., LE An, H., Karamanis, N. (2006). A computer-aided environment for generating multiple-choice test items. Natural Language Engineering , 12 (2), 177–194.
Mora, I.M., de la Puente, S.P., Nieto, X.G. (2016). Towards automatic generation of question answer pairs from images. In Proceedings of the IEEE Conference on Computer Vision and Pattern Recognition Workshops , (pp. 1–2).
Mostafazadeh, N., Misra, I., Devlin, J., Mitchell, M., He, X., Vanderwende, L. (2016). Generating Natural Questions About an Image. In Proceedings of the 54th Annual Meeting of the Association for Computational Linguistics, (Volume 1: Long Papers), Berlin, Germany , (pp. 1802–1813).
Narendra, A., Agarwal, M., Shah, R. (2013). Automatic cloze-questions generation. In Proceedings of Recent Advances in Natural Language Processing . INCOMA Ltd. Shoumen, BULGARIA (ACL 2013), Hissar, (pp. 511–515).
Nassif, A.B., Elnagar, A., Shahin, I., Henno, S. (2020). Deep learning for arabic subjective sentiment analysis: Challenges and research opportunities. Applied Soft Computing , 106836.
Bajaj, P., Campos, D., Craswell, N., Deng, L., Gao, J., Liu, X., Majumder, R., McNamara, A., Mitra, B., Nguyen, T., Rosenberg, M., Song, X., Stoica, A., Tiwary, S., Wang, T. (2016). MS MARCO: A Human Generated MAchine Reading COmprehension Dataset. arXiv , arXiv:1611.09268. https://ui.adsabs.harvard.edu/abs/2016arXiv161109268B .
Nicol, D. (2007). E-assessment by design: Using multiple-choice tests to good effect. Journal of Further and higher Education , 31 (1), 53–64.
Noorbehbahani, F., & Kardan, A.A. (2011). The automatic assessment of free text answers using a modified BLEU algorithm. Computers & Education , 56 (2), 337–345.
Papasalouros, A., Kanaris, K., Kotis, K. (2008). Automatic generation of multiple choice questions from domain ontologies. In Proceedings of the e-Learning , (pp. 427–434).
Pino, J., & Eskenazi, M. (2009). Measuring hint level in open cloze questions. In Proceedings of the 22nd International Florida Artificial Intelligence Research Society Conference(FLAIRS) . The AAAI Press, Florida, (pp. 460–465).
Pino, J., Heilman, M., Eskenazi, M. (2008). A selection strategy to improve cloze question quality. In Proceedings of the Workshop on Intelligent Tutoring Systems for Ill-Defined Domains, 9th International Conference on Intelligent Tutoring Systems . Springer, Montreal, (pp. 22–34).
Rajpurkar, P., Zhang, J., Lopyrev, K., Liang, P. (2016). SQuAD: 100,000+ Questions for Machine Comprehension of Text. In Proceedings of the 2016 Conference on Empirical Methods in Natural Language Processing . Association for Computational Linguistics, Austin, (pp. 2383–2392).
Ramachandran, L., Cheng, J., Foltz, P. (2015). Identifying patterns for short answer scoring using graph-based lexico-semantic text matching. In Proceedings of the Tenth Workshop on Innovative Use of NLP for Building Educational Applications , (pp. 97–106).
Ren, M., Kiros, R., Zemel, R. (2015). Exploring models and data for image question answering. In Advances in Neural Information Processing Systems , (pp. 2953–2961).
Roy, S., Narahari, Y., Deshmukh, O.D. (2015). A perspective on computer assisted assessment techniques for short free-text answers. In International Computer Assisted Assessment Conference . Springer, Zeist, (pp. 96–109).
Rozali, D.S., Hassan, M.F., Zamin, N. (2010). A survey on adaptive qualitative assessment and dynamic questions generation approaches. In 2010 International Symposium on Information Technology , (Vol. 3. IEEE, Kuala Lumpur, pp. 1479–1484).
Rus, V., Wyse, B., Piwek, P., Lintean, M., Stoyanchev, S., Moldovan, C. (2012). A detailed account of the first question generation shared task evaluation challenge. Dialogue & Discourse , 3 (2), 177–204.
Sakaguchi, K., Heilman, M., Madnani, N. (2015). Effective feature integration for automated short answer scoring. In Proceedings of the 2015 Conference of the North American Chapter of the Association for Computational Linguistics: Human Language Technologies , (pp. 1049–1054).
Santoro, A., Hill, F., Barrett, D., Morcos, A., Lillicrap, T. (2018). Measuring abstract reasoning in neural networks. In International Conference on Machine Learning , (pp. 4477–4486).
Serban, I.V., García-Durán, A., Gulcehre, C., Ahn, S., Chandar, S., Courville, A., Bengio, Y. (2016). Generating Factoid Questions With Recurrent Neural Networks: The 30M Factoid Question-Answer Corpus. In Proceedings of the 54th Annual Meeting of the Association for Computational Linguistics (Volume 1: Long Papers) . Association for Computational Linguistics, Berlin, (pp. 588–598).
Shaban, A.-M.S. (2014). A comparison between objective and subjective tests. Journal of the College of Languages , 30 , 44–52.
Shermis, M.D., & Burstein, J. (2013). Handbook of automated essay evaluation: Current applications and new directions .
Simoncelli, E.P., & Olshausen, B.A. (2001). Natural image statistics and neural representation. Annual Review of Neuroscience , 24 (1), 1193–1216.
Suhr, A., Zhou, S., Zhang, A., Zhang, I., Bai, H., Artzi, Y. (2019). A Corpus for Reasoning about Natural Language Grounded in Photographs. In Proceedings of the 57th Annual Meeting of the Association for Computational Linguistics . Association for Computational Linguistics, Florence, (pp. 6418–6428).
Trischler, A., Wang, T., Yuan, X., Harris, J., Sordoni, A., Bachman, P., Suleman, K. (2017). NewsQA: A Machine Comprehension Dataset. In Proceedings of the 2nd Workshop on Representation Learning for NLP . Association for Computational Linguistics, Vancouver, (pp. 191–200).
Welbl, J., Liu, N.F., Gardner, M. (2017). Crowdsourcing multiple choice science questions. In Proceedings of the 3rd Workshop on Noisy User-generated Text , (pp. 94–106).
Yu, L., Park, E., Berg, A.C., Berg, T.L. (2015). Visual madlibs: Fill in the blank description generation and question answering. In Proceedings of the IEEE International Conference on Computer Vision , (pp. 2461–2469).
Zhang, S., Qu, L., You, S., Yang, Z., Zhang, J. (2017). Automatic generation of grounded visual questions. In Proceedings of the 26th International Joint Conference on Artificial Intelligence . The AAAI Press, Melbourne, (pp. 4235–4243).
Zhu, Y., Groth, O., Bernstein, M., Fei-Fei, L. (2016). Visual7w: Grounded question answering in images. In Proceedings of the IEEE Conference on Computer Vision and Pattern Recognition , (pp. 4995–5004).
Download references
Acknowledgements
This research was supported/partially supported by Indian Center for Advancement of Research and Education (ICARE), Haldia
This study is not funded from anywhere.
Author information
Authors and affiliations.
Department of Information Technology, Haldia Institute of Technology, Haldia, India
Department of Computer Science and Application, University of North Bengal, Darjeeling, India
Mukta Majumder
Department of Computer Science and Engineering, Maulana Abul Kalam Azad University of Technology, West Bengal, India
Santanu Phadikar
Department of Physics and Technology, UiT The Arctic University of Norway, Tromsø, Norway
Arif Ahmed Sekh
You can also search for this author in PubMed Google Scholar
Contributions
All authors equally contributed and approved the final manuscript.
Corresponding author
Correspondence to Bidyut Das .
Ethics declarations
Ethics approval and consent to participate.
This article does not contain any studies with human participants or animals performed by any of the authors.
Additional information
Publisher’s note.
Springer Nature remains neutral with regard to jurisdictional claims in published maps and institutional affiliations.
Rights and permissions
Open Access This article is licensed under a Creative Commons Attribution 4.0 International License, which permits use, sharing, adaptation, distribution and reproduction in any medium or format, as long as you give appropriate credit to the original author(s) and the source, provide a link to the Creative Commons licence, and indicate if changes were made. The images or other third party material in this article are included in the article’s Creative Commons licence, unless indicated otherwise in a credit line to the material. If material is not included in the article’s Creative Commons licence and your intended use is not permitted by statutory regulation or exceeds the permitted use, you will need to obtain permission directly from the copyright holder. To view a copy of this licence, visit http://creativecommons.org/licenses/by/4.0/ .
Reprints and permissions
About this article
Cite this article.
Das, B., Majumder, M., Phadikar, S. et al. Automatic question generation and answer assessment: a survey. RPTEL 16 , 5 (2021). https://doi.org/10.1186/s41039-021-00151-1
Download citation
Received : 10 July 2020
Accepted : 24 February 2021
Published : 18 March 2021
DOI : https://doi.org/10.1186/s41039-021-00151-1
Share this article
Anyone you share the following link with will be able to read this content:
Sorry, a shareable link is not currently available for this article.
Provided by the Springer Nature SharedIt content-sharing initiative
- Question generation
- Automatic assessment
- Self learning
- Self assessment
- Educational assessment
- Create A Quiz
- Relationship
- Personality
- Harry Potter
- Online Exam
- Entertainment
- Training Maker
- Survey Maker
- Brain Games
- ProProfs.com
Research Quizzes, Questions & Answers
Top trending quizzes.

Popular Topics
Recent quizzes.
« Previous 1 2 3 4 Next »
Popular Quizzes
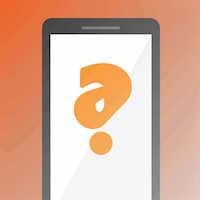
Parts of research paper
Quiz by Jean Daryl Ampong
Feel free to use or edit a copy
includes Teacher and Student dashboards
Measure skills from any curriculum
Tag the questions with any skills you have. Your dashboard will track each student's mastery of each skill.
- edit the questions
- save a copy for later
- start a class game
- automatically assign follow-up activities based on students’ scores
- assign as homework
- share a link with colleagues
- print as a bubble sheet
- Q 1 / 9 Score 0 Which of the following is NOT a part of a research paper? 29 Introduction Acknowledgment Title page Acknowledgment
Our brand new solo games combine with your quiz, on the same screen
Correct quiz answers unlock more play!
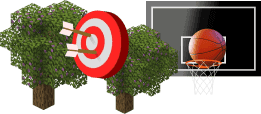
- Q 1 Which of the following is NOT a part of a research paper? Introduction Acknowledgment Title page Acknowledgment 30 s
- Q 2 Which of the following sections comes first in a research paper? Abstract Conclusion Methodology Introduction 30 s
- Q 3 Which of the following is the section where you highlight the key findings of your research paper? Results Introduction Conclusion Methodology 30 s
- Q 4 Which of the following is NOT one of the main sections of a research paper? Conclusion Appendix Introduction Results 30 s
- Q 5 Which section of a research paper describes the methods and procedures used in the research? Results Introduction Methodology Conclusion 30 s
- Q 6 Which section of a research paper summarizes the main points and provides recommendations based on the research findings? Results Methodology Introduction Conclusion 30 s
- Q 7 Which of the following is the correct order of the sections in a research paper? Abstract, Conclusion, Introduction, Methodology, References, Title page, Results, Discussion Title page, Abstract, Introduction, Methodology, Results, Discussion, Conclusion, References Introduction, Results, Methodology, Abstract, Conclusion, References, Title page, Discussion Results, Introduction, Methodology, Conclusion, Title page, Abstract, References, Discussion 30 s
- Q 8 Which section of a research paper lists the sources cited in the paper? Results Introduction Conclusion References 30 s
- Q 9 Which of the following is NOT one of the key elements of a research paper? Methodology Glossary Abstract Introduction 30 s
Teachers give this quiz to your class
A Systematic Review of Automatic Question Generation for Educational Purposes
- Open access
- Published: 21 November 2019
- Volume 30 , pages 121–204, ( 2020 )

Cite this article
You have full access to this open access article
- Ghader Kurdi ORCID: orcid.org/0000-0003-1745-5581 1 ,
- Jared Leo 1 ,
- Bijan Parsia 1 ,
- Uli Sattler 1 &
- Salam Al-Emari 2
44k Accesses
154 Citations
15 Altmetric
Explore all metrics
While exam-style questions are a fundamental educational tool serving a variety of purposes, manual construction of questions is a complex process that requires training, experience, and resources. This, in turn, hinders and slows down the use of educational activities (e.g. providing practice questions) and new advances (e.g. adaptive testing) that require a large pool of questions. To reduce the expenses associated with manual construction of questions and to satisfy the need for a continuous supply of new questions, automatic question generation (AQG) techniques were introduced. This review extends a previous review on AQG literature that has been published up to late 2014. It includes 93 papers that were between 2015 and early 2019 and tackle the automatic generation of questions for educational purposes. The aims of this review are to: provide an overview of the AQG community and its activities, summarise the current trends and advances in AQG, highlight the changes that the area has undergone in the recent years, and suggest areas for improvement and future opportunities for AQG. Similar to what was found previously, there is little focus in the current literature on generating questions of controlled difficulty, enriching question forms and structures, automating template construction, improving presentation, and generating feedback. Our findings also suggest the need to further improve experimental reporting, harmonise evaluation metrics, and investigate other evaluation methods that are more feasible.
Similar content being viewed by others
Qgen: An Automatic Question Paper Generator
Automating Question Generation From Educational Text
Towards Generalized Methods for Automatic Question Generation in Educational Domains
Avoid common mistakes on your manuscript.
Introduction
Exam-style questions are a fundamental educational tool serving a variety of purposes. In addition to their role as an assessment instrument, questions have the potential to influence student learning. According to Thalheimer ( 2003 ), some of the benefits of using questions are: 1) offering the opportunity to practice retrieving information from memory; 2) providing learners with feedback about their misconceptions; 3) focusing learners’ attention on the important learning material; 4) reinforcing learning by repeating core concepts; and 5) motivating learners to engage in learning activities (e.g. reading and discussing). Despite these benefits, manual question construction is a challenging task that requires training, experience, and resources. Several published analyses of real exam questions (mostly multiple choice questions (MCQs)) (Hansen and Dexter 1997 ; Tarrant et al. 2006 ; Hingorjo and Jaleel 2012 ; Rush et al. 2016 ) demonstrate their poor quality, which Tarrant et al. ( 2006 ) attributed to a lack of training in assessment development. This challenge is augmented further by the need to replace assessment questions consistently to ensure their validity, since their value will decrease or be lost after a few rounds of usage (due to being shared between test takers), as well as the rise of e-learning technologies, such as massive open online courses (MOOCs) and adaptive learning, which require a larger pool of questions.
Automatic question generation (AQG) techniques emerged as a solution to the challenges facing test developers in constructing a large number of good quality questions. AQG is concerned with the construction of algorithms for producing questions from knowledge sources, which can be either structured (e.g. knowledge bases (KBs) or unstructured (e.g. text)). As Alsubait ( 2015 ) discussed, research on AQG goes back to the 70’s. Nowadays, AQG is gaining further importance with the rise of MOOCs and other e-learning technologies (Qayyum and Zawacki-Richter 2018 ; Gaebel et al. 2014 ; Goldbach and Hamza-Lup 2017 ).
In what follows, we outline some potential benefits that one might expect from successful automatic generation of questions. AQG can reduce the cost (in terms of both money and effort) of question construction which, in turn, enables educators to spend more time on other important instructional activities. In addition to resource saving, having a large number of good-quality questions enables the enrichment of the teaching process with additional activities such as adaptive testing (Vie et al. 2017 ), which aims to adapt learning to student knowledge and needs, as well as drill and practice exercises (Lim et al. 2012 ). Finally, being able to automatically control question characteristics, such as question difficulty and cognitive level, can inform the construction of good quality tests with particular requirements.
Although the focus of this review is education, the applications of question generation (QG) are not limited to education and assessment. Questions are also generated for other purposes, such as validation of knowledge bases, development of conversational agents, and development of question answering or machine reading comprehension systems, where questions are used for training and testing.
This review extends a previous systematic review on AQG (Alsubait 2015 ), which covers the literature up to the end of 2014. Given the large amount of research that has been published since Alsubait’s review was conducted (93 papers over a four year period compared to 81 papers over the preceding 45-year period), an extension of Alsubait’s review is reasonable at this stage. To capture the recent developments in the field, we review the literature on AQG from 2015 to early 2019. We take Alsubait’s review as a starting point and extend the methodology in a number of ways (e.g. additional review questions and exclusion criteria), as will be described in the sections titled “ Review Objective ” and “ Review Method ”. The contribution of this review is in providing researchers interested in the field with the following:
a comprehensive summary of the recent AQG approaches;
an analysis of the state of the field focusing on differences between the pre- and post-2014 periods;
a summary of challenges and future directions; and
an extensive reference to the relevant literature.
Summary of Previous Reviews
There have been six published reviews on the AQG literature. The reviews reported by Le et al. 2014 , Kaur and Bathla 2015 , Alsubait 2015 and Rakangor and Ghodasara ( 2015 ) cover the literature that has been published up to late 2014 while those reported by Ch and Saha ( 2018 ) and Papasalouros and Chatzigiannakou ( 2018 ) cover the literature that has been published up to late 2018. Out of these, the most comprehensive review is Alsubait’s, which includes 81 papers (65 distinct studies) that were identified using a systematic procedure. The other reviews were selective and only cover a small subset of the AQG literature. Of interest, due to it being a systematic review and due to the overlap in timing with our review, is the review developed by Ch and Saha ( 2018 ). However, their review is not as rigorous as ours, as theirs only focuses on automatic generation of MCQs using text as input. In addition, essential details about the review procedure, such as the search queries used for each electronic database and the resultant number of papers, are not reported. In addition, several related studies found in other reviews on AQG are not included.
Findings of Alsubait’s Review
In this section, we concentrate on summarising the main results of Alsubait’s systematic review, due to its being the only comprehensive review. We do so by elaborating on interesting trends and speculating about the reasons for those trends, as well as highlighting limitations observed in the AQG literature.
Alsubait characterised AQG studies along the following dimensions: 1) purpose of generating questions, 2) domain, 3) knowledge sources, 4) generation method, 5) question type, 6) response format, and 7) evaluation.
The results of the review and the most prevalent categories within each dimension are summarised in Table 1 . As can be seen in Table 1 , generating questions for a specific domain is more prevalent than generating domain-unspecific questions. The most investigated domain is language learning (20 studies), followed by mathematics and medicine (four studies each). Note that, for these three domains, there are large standardised tests developed by professional organisations (e.g. Test of English as a Foreign Language (TOEFL), International English Language Testing System (IELTS) and Test of English for International Communication (TOEIC) for language, Scholastic Aptitude Test (SAT) for mathematics and board examinations for medicine). These tests require a continuous supply of new questions. We believe that this is one reason for the interest in generating questions for these domains. We also attribute the interest in the language learning domain to the ease of generating language questions, relative to questions belonging to other domains. Generating language questions is easier than generating other types of questions for two reasons: 1) the ease of adopting text from a variety of publicly available resources (e.g. a large number of general or specialised textual resources can be used for reading comprehension (RC)) and 2) the availability of natural language processing (NLP) tools for shallow understanding of text (e.g. part of speech (POS) tagging) with an acceptable performance, which is often sufficient for generating language questions. To illustrate, in Chen et al. ( 2006 ), the distractors accompanying grammar questions are generated by changing the verb form of the key (e.g. “write”, “written”, and “wrote” are distractors while “writing” is the key). Another plausible reason for interest in questions on medicine is the availability of NLP tools (e.g. named entity recognisers and co-reference resolvers) for processing medical text. There are also publicly available knowledge bases, such as UMLS (Bodenreider 2004 ) and SNOMED-CT (Donnelly 2006 ), that are utilised in different tasks such as text annotation and distractor generation. The other investigated domains are analytical reasoning, geometry, history, logic, programming, relational databases, and science (one study each).
With regard to knowledge sources, the most commonly used source for question generation is text (Table 1 ). A similar trend was also found by Rakangor and Ghodasara ( 2015 ). Note that 19 text-based approaches, out of the 38 text-based approaches identified by Alsubait ( 2015 ), tackle the generation of questions for the language learning domain, both free response (FR) and multiple choice (MC). Out of the remaining 19 studies, only five focus on generating MCQs. To do so, they incorporate additional inputs such as WordNet (Miller et al. 1990 ), thesaurus, or textual corpora. By and large, the challenge in the case of MCQs is distractor generation. Despite using text for generating language questions, where distractors can be generated using simple strategies such as selecting words having a particular POS or other syntactic properties, text often does not incorporate distractors, so external, structured knowledge sources are needed to find what is true and what is similar. On the other hand, eight ontology-based approaches are centred on generating MCQs and only three focus on FR questions.
Simple factual wh-questions (i.e. where the answers are short facts that are explicitly mentioned in the input) and gap-fill questions (also known as fill-in-the-blank or cloze questions) are the most generated types of questions with the majority of them, 17 and 15 respectively, being generated from text. The prevalence of these questions is expected because they are common in language learning assessment. In addition, these two types require relatively little effort to construct, especially when they are not accompanied by distractors. In gap-fill questions, there are no concerns about the linguistic aspects (e.g. grammaticality) because the stem is constructed by only removing a word or a phrase from a segment of text. The stem of a wh-question is constructed by removing the answer from the sentence, selecting an appropriate wh-word, and rearranging words to form a question. Other types of questions such as mathematical word problems, Jeopardy-style questions, Footnote 1 and medical case-based questions (CBQs) require more effort in choosing the stem content and verbalisation. Another related observation we made is that the types of questions generated from ontologies are more varied than the types of questions generated from text.
Limitations observed by Alsubait ( 2015 ) include the limited research on controlling the difficulty of generated questions and on generating informative feedback. Existing difficulty models are either not validated or only applicable to a specific type of question (Alsubait 2015 ). Regarding feedback (i.e. an explanation for the correctness/incorrectness of the answer), only three studies generate feedback along with the questions. Even then, the feedback is used to motivate students to try again or to provide extra reading material without explaining why the selected answer is correct/incorrect. Ungrammaticality is another notable problem with auto-generated questions, especially in approaches that apply syntactic transformations of sentences (Alsubait 2015 ). For example, 36.7% and 39.5% of questions generated in the work of Heilman and Smith ( 2009 ) were rated by reviewers as ungrammatical and nonsensical, respectively. Another limitation related to approaches to generating questions from ontologies is the use of experimental ontologies for evaluation, neglecting the value of using existing, probably large, ontologies. Various issues can arise if existing ontologies are used, which in turn provide further opportunities to enhance the quality of generated questions and the ontologies used for generation.
Review Objective
The goal of this review is to provide a comprehensive view of the AQG field since 2015. Following and extending the schema presented by Alsubait ( 2015 ) (Table 1 ), we have structured our review around the following four objectives and their related questions. Questions marked with an asterisk “*” are those proposed by Alsubait ( 2015 ). Questions under the first three objectives (except question 5 under OBJ3) are used to guide data extraction. The others are analytical questions to be answered based on extracted results.
Providing an overview of the AQG community and its activities
What is the rate of publication?*
What types of papers are published in the area?
Where is research published?
Who are the active research groups in the field?*
Summarising current QG approaches
What is the purpose of QG?*
What method is applied?*
What tasks related to question generation are considered?
What type of input is used?*
Is it designed for a specific domain? For which domain?*
What type of questions are generated?* (i.e., question format and answer format)
What is the language of the questions?
Does it generate feedback?*
Is difficulty of questions controlled?*
Does it consider verbalisation (i.e. presentation improvements)?
Identifying the gold-standard performance in AQG
Are there any available sources or standard datasets for performance comparison?
What types of evaluation are applied to QG approaches?*
What properties of questions are evaluated? Footnote 2 and What metrics are used for their measurement?
How does the generation approach perform?
What is the gold-standard performance?
Tracking the evolution of AQG since Alsubait’s review
Has there been any progress on feedback generation?
Has there been progress on generating questions with controlled difficulty?
Has there been progress on enhancing the naturalness of questions (i.e. verbalisation)?
One of our motivations for pursuing these objectives is to provide members of the AQG community with a reference to facilitate decisions such as what resources to use, whom to compare to, and where to publish. As we mentioned in the Summary of Previous Reviews , Alsubait ( 2015 ) highlighted a number of concerns related to the quality of generated questions, difficulty models, and the evaluation of questions. We were motivated to know whether these concerns have been addressed. Furthermore, while reviewing some of the AQG literature, we made some observations about the simplicity of generated questions and about the reporting being insufficient and heterogeneous. We want to know whether these issues are universal across the AQG literature.
Review Method
We followed the systematic review procedure explained in (Kitchenham and Charters 2007 ; Boland et al. 2013 ).
Inclusion and Exclusion Criteria
We included studies that tackle the generation of questions for educational purposes (e.g. tutoring systems, assessment, and self-assessment) without any restriction on domains or question types. We adopted the exclusion criteria used in Alsubait ( 2015 ) (1 to 5) and added additional exclusion criteria (6 to 13). A paper is excluded if:
it is not in English
it presents work in progress only and does not provide a sufficient description of how the questions are generated
it presents a QG approach that is based mainly on a template and questions are generated by substituting template slots with numerals or with a set of randomly predefined values
it focuses on question answering rather than question generation
it presents an automatic mechanism to deliver assessments, rather than generating assessment questions
it presents an automatic mechanism to assemble exams or to adaptively select questions from a question bank
it presents an approach for predicting the difficulty of human-authored questions
it presents a QG approach for purposes other than those related to education (e.g. training of question answering systems, dialogue systems)
it does not include an evaluation of the generated questions
it is an extension of a paper published before 2015 and no changes were made to the question generation approach
it is a secondary study (i.e. literature review)
it is not peer-reviewed (e.g. theses, presentations and technical reports)
its full text is not available (through the University of Manchester Library website, Google or Google scholar).
Search Strategy
Data sources.
Six data sources were used, five of which were electronic databases (ERIC, ACM, IEEE, INSPEC and Science Direct), which were determined by Alsubait ( 2015 ) to have good coverage of the AQG literature. We also searched the International Journal of Artificial Intelligence in Education (AIED) and the proceedings of the International Conference on Artificial Intelligence in Education for 2015, 2017, and 2018 due to their AQG publication record.
We obtained additional papers by examining the reference lists of, and the citations to, AQG papers we reviewed (known as “snowballing”). The citations to a paper were identified by searching for the paper using Google Scholar, then clicking on the “cited by” option that appears under the name of the paper. We performed this for every paper on AQG, regardless of whether we had decided to include it, to ensure that we captured all the relevant papers. That is to say, even if a paper was excluded because it met some of the exclusion criteria (1-3 and 8-13), it is still possible that it refers to, or is referred to by, relevant papers.
We used the reviews reported by Ch and Saha ( 2018 ) and Papasalouros and Chatzigiannakou ( 2018 ) as a “sanity check” to evaluate the comprehensiveness of our search strategy. We exported all the literature published between 2015 and 2018 included in the work of Ch and Saha ( 2018 ) and Papasalouros and Chatzigiannakou ( 2018 ) and checked whether they were included in our results (both search results and snowballing results).
Search Queries
We used the keywords “question” and “generation” to search for relevant papers. Actual search queries used for each of the databases are provided in the Appendix under “ Search Queries ”. We decided on these queries after experimenting with different combinations of keywords and operators provided by each database and looking at the ratio between relevant and irrelevant results in the first few pages (sorted by relevance). To ensure that recall was not compromised, we checked whether relevant results returned using different versions of each search query were still captured by the selected version.
The search results were exported to comma-separated values (CSV) files. Two reviewers then looked independently at the titles and abstracts to decide on inclusion or exclusion. The reviewers skimmed the paper if they were not able to make a decision based on the title and abstract. Note that, at this phase, it was not possible to assess whether all papers had satisfied the exclusion criteria 2, 3, 8, 9, and 10. Because of this, the final decision was made after reading the full text as described next.
To judge whether a paper’s purpose was related to education, we considered the title, abstract, introduction, and conclusion sections. Papers that mentioned many potential purposes for generating questions, but did not state which one was the focus, were excluded. If the paper mentioned only educational applications of QG, we assumed that its purpose was related to education, even without a clear purpose statement. Similarly, if the paper mentioned only one application, we assumed that was its focus.
Concerning evaluation, papers that evaluated the usability of a system that had a QG functionality, without evaluating the quality of generated questions, were excluded. In addition, in cases where we found multiple papers by the same author(s) reporting the same generation approach, even if some did not cover evaluation, all of the papers were included but counted as one study in our analyses.
Lastly, because the final decision on inclusion/exclusion sometimes changed after reading the full paper, agreement between the two reviewers was checked after the full paper had been read and the final decision had been made. However, a check was also made to ensure that the inclusion/exclusion criteria were interpreted in the same way. Cases of disagreement were resolved through discussion.
Data Extraction
Guided by the questions presented in the “ Review Objective ” section, we designed a specific data extraction form. Two reviewers independently extracted data related to the included studies. As mentioned above, different papers that related to the same study were represented as one entry. Agreement for data extraction was checked and cases of disagreement were discussed to reach a consensus.
Papers that had at least one shared author were grouped together if one of the following criteria were met:
they reported on different evaluations of the same generation approach;
they reported on applying the same generation approach to different sources or domains;
one of the papers introduced an additional feature of the generation approach such as difficulty prediction or generating distractors without changing the initial generation procedure.
The extracted data were analysed using a code written in R markdown. Footnote 3
Quality Assessment
Since one of the main objectives of this review is to identify the gold standard performance, we were interested in the quality of the evaluation approaches. To assess this, we used the criteria presented in Table 2 which were selected from existing checklists (Downs and Black 1998 ; Reisch et al. 1989 ; Critical Appraisal Skills Programme 2018 ), with some criteria being adapted to fit specific aspects of research on AQG. The quality assessment was conducted after reading a paper and filling in the data extraction form.
In what follows, we describe the individual criteria (Q1-Q9 presented in Table 2 ) that we considered when deciding if a study satisfied said criteria. Three responses are used when scoring the criteria: “yes”, “no” and “not specified”. The “not specified” response is used when either there is no information present to support the criteria, or when there is not enough information present to distinguish between a “yes” or “no” response.
Q1-Q4 are concerned with the quality of reporting on participant information, Q5-Q7 are concerned with the quality of reporting on the question samples, and Q8 and Q9 describe the evaluative measures used to assess the outcomes of the studies.
When a study reports the exact number of participants (e.g. experts, students, employees, etc.) used in the study, Q1 scores a “yes”. Otherwise, it scores a “no”. For example, the passage “20 students were recruited to participate in an exam …” would result in a “yes”, whereas “a group of students were recruited to participate in an exam …” would result in a “no”.
Q2 requires the reporting of demographic characteristics supporting the suitability of the participants for the task. Depending on the category of participant, relevant demographic information is required to score a “yes”. Studies that do not specify relevant information score a “no”. By means of examples, in studies relying on expert reviews, those that include information on teaching experience or the proficiency level of reviewers would receive a “yes”, while in studies relying on mock exams, those that include information about grade level or proficiency level of test takers would also receive a “yes”. Studies reporting that the evaluation was conducted by reviewers, instructors, students, or co-workers without providing any additional information about the suitability of the participants for the task would be considered neglectful of Q2 and score a “no”.
For a study to score “yes” for Q3, it must provide specific information on how participants were selected/recruited, otherwise it receives a score of “no”. This includes information on whether the participants were paid for their work or were volunteers. For example, the passage “7th grade biology students were recruited from a local school.” would receive a score of “no” because it is not clear whether or not they were paid for their work. However, a study that reports “Student volunteers were recruited from a local school …” or “Employees from company X were employed for n hours to take part in our study… they were rewarded for their services with Amazon vouchers worth $n” would receive a “yes”.
To score “yes” for Q4, two conditions must be met: the study must 1) score “yes” for both Q2 and Q3 and 2) only use participants that are suitable for the task at hand. Studies that fail to meet the first condition score “not specified” while those that fail to meet the second condition score “no”. Regarding the suitability of participants, we consider, as an example, native Chinese speakers suitable for evaluating the correctness and plausibility of options generated for Chinese gap-fill questions. As another example, we consider Amazon Mechanical Turk (AMT) co-workers unsuitable for evaluating the difficulty of domain-specific questions (e.g. mathematical questions).
When a study reports the exact number of questions used in the experimentation or evaluation stage, Q5 receives a score of “yes”, otherwise it receives a score of “no”. To demonstrate, consider the following examples. A study reporting “25 of the 100 generated questions were used in our evaluation …” would receive a score of “yes”. However, if a study made a claim such as “Around half of the generated questions were used …”, it would receive a score of “no”.
Q6a requires that the sampling strategy be not only reported (e.g. random, proportionate stratification, disproportionate stratification, etc.) but also justified to receive a “yes”, otherwise, it receives a score of “no”. To demonstrate, if a study only reports that “We sampled 20 questions from each template …” would receive a score of “no” since no justification as to why the stratified sampling procedure was used is provided. However, if it was to also add “We sampled 20 questions from each template to ensure template balance in discussions about the quality of generated questions …” then this would be considered as a suitable justification and would warrant a score of “yes”. Similarly, Q6b requires that the sample size be both reported and justified.
Our decision regarding Q7 takes into account the following: 1) responses to Q6a (i.e. a study can only score “yes” if the score to Q6a is “yes”, otherwise, the score would be “not specified”) and 2) representativeness of the population. Using random sampling is, in most cases, sufficient to score “yes” for Q7. However, if multiple types of questions are generated (e.g. different templates or different difficulty levels), stratified sampling is more appropriate in cases in which the distribution of questions is skewed.
Q8 considers whether the authors provide a description, a definition, or a mathematical formula for the evaluation measures they used as well as a description of the coding system (if applicable). If so, then the study receives a score of “yes” for Q8, otherwise it receives a score of “no”.
Q9 is concerned with whether questions were evaluated by multiple reviewers and whether measures of the agreement (e.g., Cohen’s kappa or percentage of agreement) were reported. For example, studies reporting information similar to “all questions were double-rated and inter-rater agreement was computed…” receive a score of “yes”, whereas studies reporting information similar to “Each question was rated by one reviewer…” receive a score of “no” .
To assess inter-rater reliability, this activity was performed by two reviewers (the first and second authors), who are proficient in the field of AQG, independently on an exploratory random sample of 27 studies. Footnote 4 The percentage of agreement and Cohen’s kappa were used to measure inter-rater reliability for Q1-Q9. The percentage of agreement ranged from 73% to 100%, while Cohen’s kappa was above .72 for Q1-Q5, demonstrating “substantial to almost perfect agreement”, and equal to 0.42 for Q9, Footnote 5
Results and Discussion
Search and screening results.
Searching the databases and AIED resulted in 2,012 papers and we checked 974. Footnote 7 The difference is due to ACM which provided 1,265 results and we only checked the first 200 results (sorted by relevance) because we found that subsequent results became irrelevant. Out of the search results, 122 papers were considered relevant after looking at their titles and abstracts. After removing duplicates, 89 papers remained. This set was further reduced to 36 papers after reading the full text of the papers. Checking related work sections and the reference lists identified 169 further papers (after removing duplicates). After we read their full texts, we found 46 to satisfy our inclusion criteria. Among those 46, 15 were captured by the initial search. Tracking citations using Google Scholar provided 204 papers (after removing duplicates). After reading their full text, 49 were found to satisfy our inclusion criteria. Among those 49, 14 were captured by the initial search. The search results are outlined in Table 3 . The final number of included papers was 93 (72 studies after grouping papers as described before). In total, the database search identified 36 papers while the other sources identified 57. Although the number of papers identified through other sources was large, many of them were variants of papers already included in the review.
The most common reasons for excluding papers on AQG were that the purpose of the generation was not related to education or there was no evaluation. Details of papers that were excluded after reading their full text are in the Appendix under “ Excluded Studies ”.
Data Extraction Results
In this section, we provide our results and outline commonalities and differences with Alsubait’s results (highlighted in the “ Findings of Alsubait’s Review ” section). The results are presented in the same order as our research questions. The main characteristics of the reviewed literature can be found in the Appendix under “ Summary of Included Studies ”.
Rate of Publication
The distribution of publications by year is presented in Fig. 1 . Putting this together with the results reported by Alsubait ( 2015 ), we notice a strong increase in publication starting from 2011. We also note that there were three workshops on QG Footnote 8 in 2008, 2009, and 2010, respectively, with one being accompanied by a shared task (Rus et al. 2012 ). We speculate that the increase starting from 2011 is because workshops on QG have drawn researchers’ attention to the field, although the participation rate in the shared task was low (only five groups participated). The increase also coincides with the rise of MOOCs and the launch of major MOOC providers (Udacity, Udemy, Coursera and edX, which all started up in 2012 (Baturay 2015 )) which provides another reason for the increasing interest in AQG. This interest was further boosted from 2015. In addition to the above speculations, it is important to mention that QG is closely related to other areas such as NLP and the Semantic Web. Being more mature and providing methods and tools that perform well have had an effect on the quantity and quality of research in QG. Note that these results are only related to question generation studies that focus on educational purposes and that there is a large volume of studies investigating question generation for other applications as mentioned in the “ Search and Screening Results ” section.
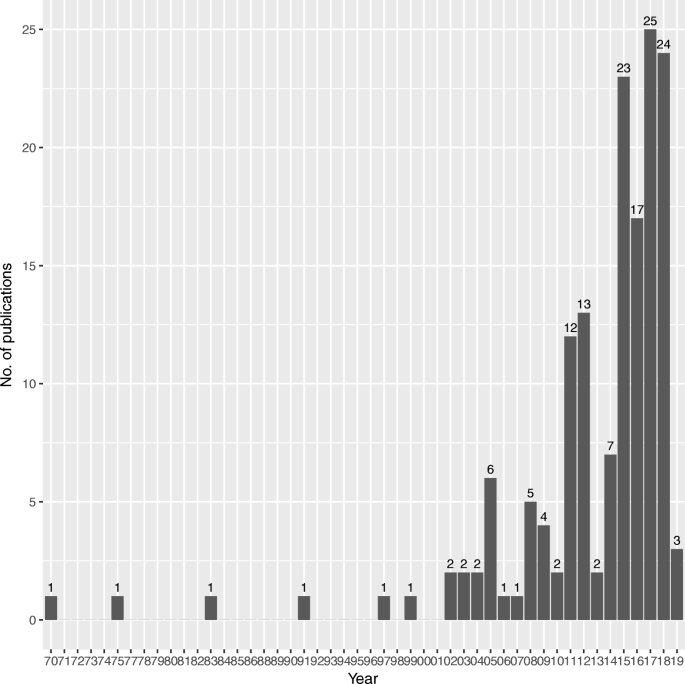
Publications per year
Types of Papers and Publication Venues
Of the papers published in the period covered by this review, conference papers constitute the majority (44 papers), followed by journal articles (32 papers) and workshop papers (17 papers). This is similar to the results of Alsubait ( 2015 ) with 34 conference papers, 22 journal papers, 13 workshop papers, and 12 other types of papers, including books or book chapters as well as technical reports and theses. In the Appendix, under “ Publication Venues ”, we list journals, conferences, and workshops that published at least two of the papers included in either of the reviews.
Research Groups
Overall, 358 researchers are working in the area (168 identified in Alsubait’s review and 205 identified in this review with 15 researchers in common). The majority of researchers have only one publication. In Appendix “ Active Research Groups ”, we present the 13 active groups defined as having more than two publications in the period of both reviews. Of the 174 papers identified in both reviews, 64 were published by these groups. This shows that, besides the increased activities in the study of AQG, the community is also growing.
Purpose of Question Generation
Similar to the results of Alsubait’s review (Table 1 ), the main purpose of generating questions is to use them as assessment instruments (Table 4 ). Questions are also generated for other purposes, such as to be employed in tutoring or self-assisted learning systems. Generated questions are still used in experimental settings and only Zavala and Mendoza ( 2018 ) have reported their use in a class setting, in which the generator is used to generate quizzes for several courses and to generate assignments for students.
Generation Methods
Methods of generating questions have been classified in the literature (Yao et al. 2012 ) as follows: 1) syntax-based, 2) semantic-based, and 3) template-based. Syntax-based approaches operate on the syntax of the input (e.g. syntactic tree of text) to generate questions. Semantic-based approaches operate on a deeper level (e.g. is-a or other semantic relations). Template-based approaches use templates consisting of fixed text and some placeholders that are populated from the input. Alsubait ( 2015 ) extended this classification to include two more categories: 4) rule-based and 5) schema-based. The main characteristic of rule-based approaches, as defined by Alsubait ( 2015 ), is the use of rule-based knowledge sources to generate questions that assess understanding of the important rules of the domain. As this definition implies that these methods require a deep understanding (beyond syntactic understanding), we believe that this category falls under the semantic-based category. However, we define the rule-based approach differently, as will be seen below. Regarding the fifth category, according to Alsubait ( 2015 ), schemas are similar to templates but are more abstract. They provide a grouping of templates that represent variants of the same problem. We regard this distinction between template and schema as unclear. Therefore, we restrict our classification to the template-based category regardless of how abstract the templates are.
In what follows, we extend and re-organise the classification proposed by Yao et al. ( 2012 ) and extended by Alsubait ( 2015 ). This is due to our belief that there are two relevant dimensions that are not captured by the existing classification of different generation approaches: 1) the level of understanding of the input required by the generation approach and 2) the procedure for transforming the input into questions. We describe our new classification, characterise each category and give examples of features that we have used to place a method within these categories. Note that these categories are not mutually exclusive.
Level of understanding
Syntactic: Syntax-based approaches leverage syntactic features of the input, such as POS or parse-tree dependency relations, to guide question generation. These approaches do not require understanding of the semantics of the input in use (i.e. entities and their meaning). For example, approaches that select distractors based on their POS are classified as syntax-based.
Semantic: Semantic-based approaches require a deeper understanding of the input, beyond lexical and syntactic understanding. The information that these approaches use are not necessarily explicit in the input (i.e. they may require reasoning to be extracted). In most cases, this requires the use of additional knowledge sources (e.g., taxonomies, ontologies, or other such sources). As an example, approaches that use either contextual similarity or feature-based similarity to select distractors are classified as being semantic-based.
Procedure of transformation
Template: Questions are generated with the use of templates. Templates define the surface structure of the questions using fixed text and placeholders that are substituted with values to generate questions. Templates also specify the features of the entities (either syntactic, semantic, or both), that can replace the placeholders.
Rule: Questions are generated with the use of rules. Rules often accompany approaches using text as input. Typically, approaches utilising rules annotate sentences with syntactic and/or semantic information. They then use these annotations to match the input to a pattern specified in the rules. These rules specify how to select a suitable question type (e.g. selecting suitable wh-words) and how to manipulate the input to construct questions (e.g. converting sentences into questions).
Statistical methods: This is where question transformation is learned from training data. For example, in Gao et al. ( 2018 ), question generation has been dealt with as a sequence-to-sequence prediction problem in which, given a segment of text (usually a sentence), the question generator forms a sequence of text representing a question (using the probabilities of co-occurrence that are learned from the training data). Training data has also been used in Kumar et al. ( 2015b ) for predicting which word(s) in the input sentence is/are to be replaced by a gap (in gap-fill questions).
Regarding the level of understanding, 60 papers rely on semantic information and only ten approaches rely only on syntactic information. All except three of the ten syntactic approaches (Das and Majumder 2017 ; Kaur and Singh 2017 ; Kusuma and Alhamri 2018 ) tackle the generation of language questions. In addition, templates are more popular than rules and statistical methods, with 27 papers reporting the use of templates, compared to 16 and nine for rules and statistical methods, respectively. Each of these three approaches has its advantages and disadvantages. In terms of cost, all three approaches are considered expensive. Templates and rules require manual construction, while learning from data often requires a large amount of annotated data which is unavailable in many specific domains. Additionally, questions generated by rules and statistical methods are very similar to the input (e.g. sentences used for generation), while templates allow the generating of questions that differ from the surface structure of the input, in the use of words for example. However, questions generated from templates are limited in terms of their linguistic diversity. Note that some of the papers were classified as not having a method of transforming the input into questions because they only focused on distractor generation or gap-fill questions for which the stem is the same input statement with a word or a phrase being removed. Readers interested in studies that belong to a specific approach are referred to the “ Summary of Included Studies ” in the Appendix.
Generation Tasks
Tasks involved in question generation are explained below. We grouped the tasks into the stages of preprocessing, question construction, and post-processing. For each task, we provide a brief description, mention its role in the generation process, and summarise different approaches that have been applied in the literature. The “ Summary of Included Studies ” in the Appendix shows which tasks have been tackled in each study.
Preprocessing
Two types of preprocessing are involved: 1) standard preprocessing and 2) QG-specific preprocessing. Standard preprocessing is common to various NLP tasks and is used to prepare the input for upcoming tasks; it involves segmentation, sentence splitting, tokenisation, POS tagging, and coreference resolution. In some cases, it also involves named entity recognition (NER) and relation extraction (RE). The aim of QG-specific preprocessing is to make or select inputs that are more suitable for generating questions. In the reviewed literature, three types of QG-specific preprocessing are employed:
Sentence simplification: This is employed in some text-based approaches (Liu et al. 2017 ; Majumder and Saha 2015 ; Patra and Saha 2018b ). Complex sentences, usually sentences with appositions or sentences joined with conjunctions, are converted into simple sentences to ease upcoming tasks. For example, Patra and Saha ( 2018b ) reported that Wikipedia sentences are long and contain multiple objects; simplifying these sentences facilitates triplet extraction (where triples are used later for generating questions). This task was carried out by using sentence simplification rules (Liu et al. 2017 ) and relying on parse-tree dependencies (Majumder and Saha 2015 ; Patra and Saha 2018b ).
Sentence classification: In this task, sentences are classified into categories, which is, according to Mazidi and Tarau ( 2016a ) and Mazidi and Tarau ( 2016b ), a key to determining the type of question to be asked about the sentence. This classification was carried out by analysing POS and dependency labels, as in Mazidi and Tarau ( 2016a ) and Mazidi and Tarau ( 2016b ) or by using a machine learning (ML) model and a set of rules, as in Basuki and Kusuma ( 2018 ). For example, in Mazidi and Tarau( 2016a , 2016b ), the pattern “S-V-acomp” is an adjectival complement that describes the subject and is therefore matched to the question template “Indicate properties or characteristics of S?”
Content selection: As the number of questions in examinations is limited, the goal of this task is to determine important content, such as sentences, parts of sentences, or concepts, about which to generate questions. In the reviewed literature, the majority approach is to generate all possible questions and leave the task of selecting important questions to exam designers. However, in some settings such as self-assessment and self-learning environments, in which questions are generated “on the fly”, leaving the selection to exam designers is not feasible.
Content selection was of interest for those approaches that utilise text more than for those that utilise structured knowledge sources. Several characterisations of important sentences and approaches for their selection have been proposed in the reviewed literature which we summarise in the following paragraphs.
Huang and He ( 2016 ) defined three characteristics for selecting sentences that are important for reading assessment and propose metrics for their measurement: keyness (containing the key meaning of the text), completeness (spreading over different paragraphs to ensure that test-takers grasp the text fully), and independence (covering different aspects of text content). Olney et al. ( 2017 ) selected sentences that: 1) are well connected to the discourse (same as completeness) and 2) contain specific discourse relations. Other researchers have focused on selecting topically important sentences. To that end, Kumar et al. ( 2015b ) selected sentences that contain concepts and topics from an educational textbook, while Kumar et al. ( 2015a ) and Majumder and Saha ( 2015 ) used topic modelling to identify topics and then rank sentences based on topic distribution. Park et al. ( 2018 ) took another approach by projecting the input document and sentences within it into the same n-dimensional vector space and then selecting sentences that are similar to the document, assuming that such sentences best express the topic or the essence of the document. Other approaches selected sentences by checking the occurrence of, or measuring the similarity to, a reference set of patterns under the assumption that these sentences convey similar information to sentences used to extract patterns (Majumder and Saha 2015 ; Das and Majumder 2017 ). Others (Shah et al. 2017 ; Zhang and Takuma 2015 ) filtered sentences that are insufficient on their own to make valid questions, such as sentences starting with discourse connectives (e.g. thus, also, so, etc.) as in Majumder and Saha ( 2015 ).
Still other approaches to content selection are more specific and are informed by the type of question to be generated. For example, the purpose of the study reported in Susanti et al. ( 2015 ) is to generate “closest-in-meaning vocabulary questions” Footnote 9 which involve selecting a text snippet from the Internet that contains the target word, while making sure that the word has the same sense in both the input and retrieved sentences. To this end, the retrieved text was scored on the basis of metrics such as the number of query words that appear in the text.
With regard to content selection from structured knowledge bases, only one study focuses on this task. Rocha and Zucker ( 2018 ) used DBpedia to generate questions along with external ontologies; the ontologies describe educational standards according to which DBpedia content was selected for use in question generation.
Question Construction
This is the main task and involves different processes based on the type of questions to be generated and their response format. Note that some studies only focus on generating partial questions (only stem or distractors). The processes involved in question construction are as follows:
Stem and correct answer generation: These two processes are often carried out together, using templates, rules, or statistical methods, as mentioned in the “ Generation Methods ” Section. Subprocesses involved are:
transforming assertive sentences into interrogative ones (when the input is text);
determination of question type (i.e. selecting suitable wh-word or template); and
selection of gap position (relevant to gap-fill questions).
Incorrect options (i.e. distractor) generation: Distractor generation is a very important task in MCQ generation since distractors influence question quality. Several strategies have been used to generate distractors. Among these are selection of distractors based on word frequency (i.e. the number of times distractors appear in a corpus is similar to the key) (Jiang and Lee 2017 ), POS (Soonklang and Muangon 2017 ; Susanti et al. 2015 ; Satria and Tokunaga 2017a , 2017b ; Jiang and Lee 2017 ), or co-occurrence with the key (Jiang and Lee 2017 ). A dominant approach is the selection of distractors based on their similarity to the key, using different notions of similarity, such as syntax-based similarity (i.e. similar POS, similar letters) (Kumar et al. 2015b ; Satria and Tokunaga 2017a , 2017b ; Jiang and Lee 2017 ), feature-based similarity (Wita et al. 2018 ; Majumder and Saha 2015 ; Patra and Saha 2018a , 2018b ; Alsubait et al. 2016 ; Leo et al. 2019 ), or contextual similarity (Afzal 2015 ; Kumar et al. 2015a , 2015b ; Yaneva et al. 2018 ; Shah et al. 2017 ; Jiang and Lee 2017 ). Some studies (Lopetegui et al. 2015 ; Faizan and Lohmann 2018 ; Faizan et al. 2017 ; Kwankajornkiet et al. 2016 ; Susanti et al. 2015 ) selected distractors that are declared in a KB to be siblings of the key, which also implies some notion of similarity (siblings are assumed to be similar). Another approach that relies on structured knowledge sources is described in Seyler et al. ( 2017 ). The authors used query relaxation, whereby queries used to generate question keys are relaxed to provide distractors that share some of the key features. Faizan and Lohmann ( 2018 ) and Faizan et al. ( 2017 ) and Stasaski and Hearst ( 2017 ) adopted a similar approach for selecting distractors. Others, including Liang et al. ( 2017 , 2018 ) and Liu et al. ( 2018 ), used ML-models to rank distractors based on a combination of the previous features.
Again, some distractor selection approaches are tailored to specific types of questions. For example, for pronoun reference questions generated in Satria and Tokunaga ( 2017a , 2017b ), words selected as distractors do not belong to the same coreference chain as this would make them correct answers. Another example of a domain specific approach for distractor selection is related to gap-fill questions. Kumar et al. ( 2015b ) ensured that distractors fit into the question sentence by calculating the probability of their occurring in the question.
Feedback generation: Feedback provides an explanation of the correctness or incorrectness of responses to questions, usually in reaction to user selection. As feedback generation is one of the main interests of this review, we elaborate more fully on this in the “ Feedback Generation ” section.
Controlling difficulty: This task focuses on determining how easy or difficult a question will be. We elaborate more on this in the section titled “ Difficulty ” .
Post-processing
The goal of post-processing is to improve the output questions. This is usually achieved via two processes:
Verbalisation: This task is concerned with producing the final surface structure of the question. There is more on this in the section titled “ Verbalisation ”.
Question ranking (also referred to as question selection or question filtering): Several generators employed an “over-generate and rank” approach whereby a large number of questions are generated, and then ranked or filtered in a subsequent phase. The ranking goal is to prioritise good quality questions. The ranking is achieved by the use of statistical models as in Blšták ( 2018 ), Kwankajornkiet et al. ( 2016 ), Liu et al. ( 2017 ), and Niraula and Rus ( 2015 ).
In this section, we summarise our observations on which input formats are most popular in the literature published after 2014. One question we had in mind is whether structured sources (i.e. whereby knowledge is organised in a way that facilitates automatic retrieval and processing) are gaining more popularity. We were also interested in the association between the input being used and the domain or question types. Specifically, are some inputs more common in specific domains? And are some inputs more suitable for specific types of questions?
As in the findings of Alsubait (Table 1 ), text is still the most popular type of input with 42 studies using it. Ontologies and resource description framework (RDF) knowledge bases come second, with eight and six studies, respectively, using these. Note that these three input formats are shared between our review and Alsubit’s review. Another input, used by more than one study, are question stems and keys, which feature in five studies that focus on generating distractors. See the Appendix “ Summary of Included Studies ” for types of inputs used in each study.
The majority of studies reporting the use of text as the main input are centred around generating questions for language learning (18 studies) or generating simple factual questions (16 studies). Other domains investigated are medicine, history, and sport (one study each). On the other hand, among studies utilising Semantic Web technologies, only one tackles the generation of language questions and nine tackle the generation of domain-unspecific questions. Questions for biology, medicine, biomedicine, and programming have also been generated using Semantic Web technologies. Additional domains investigated in Alsubait’s review are mathematics, science, and databases (for studies using the Semantic Web). Combining both results, we see a greater variety of domains in semantic-based approaches.
Free-response questions are more prevalent among studies using text, with 21 studies focusing on this question type, 18 on multiple-choice, three on both free-response and multiple-choice questions, and one on verbal response questions. Some studies employ additional resources such as WordNet (Kwankajornkiet et al. 2016 ; Kumar et al. 2015a ) or DBpedia (Faizan and Lohmann 2018 ; Faizan et al. 2017 ; Tamura et al. 2015 ) to generate distractors. By contrast, MCQs are more prevalent in studies using Semantic Web technologies, with ten studies focusing on the generation of multiple-choice questions and four studies focusing on free-response questions. This result is similar to those obtained by Alsubait (Table 1 ) with free-response being more popular for generation from text and multiple-choice more popular from structured sources. We have discussed why this is the case in the “ Findings of Alsubait’s Review ” Section.
Domain, Question Types and Language
As Alsubait found previously (“ Findings of Alsubait’s Review ” section), language learning is the most frequently investigated domain. Questions generated for language learning target reading comprehension skills, as well as knowledge of vocabulary and grammar. Research is ongoing concerning the domains of science (biology and physics), history, medicine, mathematics, computer science, and geometry, but there are still a small number of papers published on these domains. In the current review, no study has investigated the generation of logic and analytical reasoning questions, which were present in the studies included in Alsubait’s review. Sport is the only new domain investigated in the reviewed literature. Table 5 shows the number of papers in each domain and the types of questions generated for these domains (for more details, see the Appendix, “ Summary of Included Studies ”). As Table 5 illustrates, gap-fill and wh-questions are again the most popular. The reader is referred to the section “ Findings of Alsubait’s Review ” for our discussion of reasons for the popularity of the language domain and the aforementioned question types.
With regard to the response format of questions, both free- and selected-response questions (i.e. MC and T/F questions) are of interest. In all, 35 studies focus on generating selected-response questions, 32 on generating free-response questions, and four studies on both. These numbers are similar to the results reported in Alsubait ( 2015 ), which were 33 and 32 papers on generation of free- and selected-response questions respectively (Table 1 ). However, which format is more suitable for assessment is debatable. Although some studies that advocate the use of free-response argue that these questions can test a higher cognitive level, Footnote 10 most automatically generated free-response questions are simple factual questions for which the answers are short facts explicitly mentioned in the input. Thus, we believe that it is useful to generate distractors, leaving to exam designers the choice of whether to use the free-response or the multiple-choice version of the question.
Concerning language, the majority of studies focus on generating questions in English (59 studies). Questions in Chinese (5 studies), Japanese (3 studies), Indonesian (2 studies), as well as Punjabi and Thai (1 study each) have also been generated. To ascertain which languages have been investigated before, we skimmed the papers identified in Alsubait ( 2015 ) and found three studies on generating questions in languages other than English: French in Fairon ( 1999 ), Tagalog in Montenegro et al. ( 2012 ), and Chinese, in addition to English, in Wang et al. ( 2012 ). This reflects an increasing interest in generating questions in other languages, which possibly accompanies interest in NLP research in these domains. Note that there may be studies on other languages or more studies on the languages we have identified that we were not able to capture, because we excluded studies written in languages other than English.
Feedback Generation
Feedback generation concerns the provision of information regarding the response to a question. Feedback is important in reinforcing the benefits of questions especially in electronic environments in which interaction between instructors and students is limited. In addition to informing test takers of the correctness of their responses, feedback plays a role in correcting test takers’ errors and misconceptions and in guiding them to the knowledge they must acquire, possibly with reference to additional materials.
This aspect of questions has been neglected in early and recent AQG literature. Among the literature that we reviewed, only one study, Leo et al. ( 2019 ), has generated feedback, alongside the generated questions. They generate feedback as a verbalisation of the axioms used to select options. In cases of distractors, axioms used to generate both key and distractors are included in the feedback.
We found another study (Das and Majumder 2017 ) that has incorporated a procedure for generating hints using syntactic features, such as the number of words in the key, the first two letters of a one-word key, or the second word of a two-words key.
Difficulty is a fundamental property of questions that is approximated using different statistical measures, one of which is percentage correct (i.e the percentage of examinees who answered a question correctly). Footnote 11 Lack of control over difficulty poses issues such as generating questions of inappropriate difficulty (inappropriately easy or difficult questions). Also, searching for a question with a specific difficulty among a huge number of generated questions is likely to be tedious for exam designers.
We structure this section around three aspects of difficulty models: 1) their generality, 2) features underlying them, and 3) evaluation of their performance.
Despite the growth in AQG, only 14 studies have dealt with difficulty. Eight of these studies focus on the difficulty of questions belonging to a particular domain, such as mathematical word problems (Wang and Su 2016 ; Khodeir et al. 2018 ), geometry questions (Singhal et al. 2016 ), vocabulary questions (Susanti et al. 2017a ), reading comprehension questions (Gao et al. 2018 ), DFA problems (Shenoy et al. 2016 ), code-tracing questions (Thomas et al. 2019 ), and medical case-based questions (Leo et al. 2019 ; Kurdi et al. 2019 ). The remaining six focus on controlling the difficulty of non-domain-specific questions (Lin et al. 2015 ; Alsubait et al. 2016 ; Kurdi et al. 2017 ; Faizan and Lohmann 2018 ; Faizan et al. 2017 ; Seyler et al. 2017 ; Vinu and Kumar 2015a , 2017a ; Vinu et al. 2016 ; Vinu and Kumar 2017b , 2015b ).
Table 6 shows the different features proposed for controlling question difficulty in the aforementioned studies. In seven studies, RDF knowledge bases or OWL ontologies were used to derive the proposed features. We observe that only a few studies account for the contribution of both stem and options to difficulty.
Difficulty control was validated by checking agreement between predicted difficulty and expert prediction in Vinu and Kumar ( 2015b ), Alsubait et al. ( 2016 ), Seyler et al. ( 2017 ), Khodeir et al. ( 2018 ), and Leo et al. ( 2019 ), by checking agreement between predicted difficulty and student performance in Alsubait et al. ( 2016 ), Susanti et al. ( 2017a ), Lin et al. ( 2015 ), Wang and Su ( 2016 ), Leo et al. ( 2019 ), and Thomas et al. ( 2019 ), by employing automatic solvers in Gao et al. ( 2018 ), or by asking experts to complete a survey after using the tool (Singhal et al. 2016 ). Expert reviews and mock exams are equally represented (seven studies each). We observe that the question samples used were small, with the majority of samples containing less than 100 questions (Table 7 ).
In addition to controlling difficulty, in one study (Kusuma and Alhamri 2018 ), the author claims to generate questions targeting a specific Bloom level. However, no evaluation of whether generated questions are indeed at a particular Bloom level was conducted.
Verbalisation
We define verbalisation as any process carried out to improve the surface structure of questions (grammaticality and fluency) or to provide variations of questions (i.e. paraphrasing). The former is important since linguistic issues may affect the quality of generated questions. For example, grammatical inconsistency between the stem and incorrect options enables test takers to select the correct option with no mastery of the required knowledge. On the other hand, grammatical inconsistency between the stem and the correct option can confuse test takers who have the required knowledge and would have been likely to select the key otherwise. Providing different phrasing for the question text is also of importance, playing a role in keeping test takers engaged. It also plays a role in challenging test takers and ensuring that they have mastered the required knowledge, especially in the language learning domain. To illustrate, consider questions for reading comprehension assessment; if the questions match the text with a very slight variation, test takers are likely to be able to answer these questions by matching the surface structure without really grasping the meaning of the text.
From the literature identified in this review, only ten studies apply additional processes for verbalisation. Given that the majority of the literature focuses on gap-fill question generation, this result is expected. Aspects of verbalisation that have been considered are pronoun substitutions (i.e. replacing pronouns by their antecedents) (Huang and He 2016 ), selection of a suitable auxiliary verb (Mazidi and Nielsen 2015 ), determiner selection (Zhang and VanLehn 2016 ), and representation of semantic entities (Vinu and Kumar 2015b ; Seyler et al. 2017 ) (see below for more on this). Other verbalisation processes that are mostly specific to some question types are the following: selection of singular personal pronouns (Faizan and Lohmann 2018 ; Faizan et al. 2017 ), which is relevant for Jeopardy questions; selection of adjectives for predicates (Vinu and Kumar 2017a ), which is relevant for aggregation questions; and ordering sentences and reference resolution (Huang and He 2016 ), which is relevant for word problems.
For approaches utilising structured knowledge sources, semantic entities, which are usually represented following some convention such as using camel case (e.g anExampleOfCamelCase) or using underscore as a word separator, need to be represented in a natural form. Basic processing which includes word segmentation, adaptation of camel case, underscores, spaces, punctuation, and conversion of the segmented phrase into a suitable morphological form (e.g. “has pet” to “having pet”), has been reported in Vinu and Kumar ( 2015b ). Seyler et al. ( 2017 ) used Wikipedia to verbalise entities, an entity-annotated corpus to verbalise predicates, and WordNet to verbalise semantic types. The surface form of Wikipedia links was used as verbalisation for entities. The annotated corpus was used to collect all sentences that contain mentions of entities in a triple, combined with some heuristic for filtering and scoring sentences. Phrases between the two entities were used as verbalisation of predicates. Finally, as types correspond to WordNet synsets, the authors used a lexicon that comes with WordNet for verbalising semantic types.
Only two studies (Huang and He 2016 ; Ai et al. 2015 ) have considered paraphrasing. Ai et al. ( 2015 ) employed a manually created library that includes different ways to express particular semantic relations for this purpose. For instance, “wife had a kid from husband” is expressed as “from husband, wife had a kid”. The latter is randomly chosen from among the ways to express the marriage relation as defined in the library. The other study that tackles paraphrasing is Huang and He ( 2016 ) in which words were replaced with synonyms.
In this section, we report on standard datasets and evaluation practices that are currently used in the field (considering how QG approaches are evaluated and what aspects of questions such evaluation focuses on). We also report on issues hindering comparison of the performance of different approaches and identification of the best-performing methods. Note that our focus is on the results of evaluating the whole generation approach, as indicated by the quality of generated questions, and not on the results of evaluating a specific component of the approach (e.g. sentence selection or classification of question types). We also do not report on evaluations related to the usability of question generators (e.g. evaluating ease of use) or efficiency (i.e. time taken to generate questions). For approaches using ontologies as the main input, we consider whether they use existing ontologies or experimental ones (i.e. created for the purpose of QG), since Alsubait ( 2015 ) has concerns related to using experimental ontologies in evaluations (see “ Findings of Alsubait’s Review ” section). We also reflect on further issues in the design and implementation of evaluation procedures and how they can be improved.
Standard Datasets
In what follows, we outline publicly available question corpora, providing details about their content, as well as how they were developed and used in the context of QG. These corpora are grouped on the basis of the initial purpose for which they were developed. Following this, we discuss the advantages and limitations of using such datasets and call attention to some aspects to consider when developing similar datasets.
The identified corpora are developed for the following three purposes:
Machine reading comprehension
The Stanford Question Answering Dataset (SQuAD) Footnote 12 (Rajpurkar et al. 2016 ) consists of 150K questions about Wikipedia articles developed by AMT co-workers. Of those, 100K questions are accompanied by paragraph-answer pairs from the same articles and 50K questions have no answer in the article. This dataset was used by Kumar et al. ( 2018 ) and Wang et al. ( 2018 ) to perform a comparison among variants of the generation approach they developed and between their approach and an approach from the literature. The comparison was based on the metrics BLEU-4, METEOR, and ROUGE-L which capture the similarity between generated questions and the SQuAD questions that serve as ground truth questions (there is more information on these metrics in the next section). That is, questions were generated using the 100K paragraph-answer pairs as input. Then, the generated questions were compared with the human-authored questions that are based on the same paragraph-answer pairs.
NewsQA Footnote 13 is another crowd-sourced dataset of about 120K question-answer pairs about CNN articles. The dataset consists of wh-questions and is used in the same way as SQuAD.
Training question-answering (QA) systems
The 30M factoid question-answer corpus (Serban et al. 2016 ) is a corpus of questions automatically generated from Freebase. Footnote 14 Freebase triples (of the form: subject, relationship, object) were used to generate questions where the correct answer is the object of the triple. For example, the question: “What continent is bayuvi dupki in?” is generated from the triple (bayuvi dupki, contained by, europe). The triples and the questions generated from them are provided in the dataset. A sample of the questions was evaluated by 63 AMT co-workers, each of whom evaluated 44-75 examples; each question was evaluated by 3-5 co-workers. The questions were also evaluated by automatic evaluation metrics. Song and Zhao ( 2016a ) performed a qualitative analysis comparing the grammaticality and naturalness of questions generated by their approach and questions from this corpus (although the comparison is not clear).
SciQ Footnote 15 (Welbl et al. 2017 ) is a corpus of 13.7K science MCQs on biology, chemistry, earth science, and physics. The questions target a broad cohort, ranging from elementary to college introductory level. The corpus was created by AMT co-workers at a cost of $10,415 and its development relied on a two-stage procedure. First, 175 co-workers were shown paragraphs and asked to generate questions for a payment of $0.30 per question. Second, another crowd-sourcing task in which co-workers validate the questions developed and provide them with distractors was conducted. A list of six distractors was provided by a ML-model. The co-workers were asked to select two distractors from the list and to provide at least one additional distractor for a payment of $0.20. For evaluation, a third crowd-sourcing task was created. The co-workers were provided with 100 question pairs, each pair consisting of an original science exam question and a crowd-sourced question in a random order. They were instructed to select the question likelier to be the real exam question. The science exam questions were identified in 55% of the cases. This corpus was used by Liang et al. ( 2018 ) to develop and test a model for ranking distractors. All keys and distractors in the dataset were fed to the model to rank. The authors assessed whether ranked distractors were among the original distractors provided with the questions.
Question generation
The question generation shared task challenge (QGSTEC) dataset Footnote 16 (Rus et al. 2012 ) is created for the QG shared task. The shared task contains two challenges: question generation from individual sentences and question generation from a paragraph. The dataset contains 90 sentences and 65 paragraphs collected from Wikipedia, OpenLearn, Footnote 17 and Yahoo! Answers, with 180 and 390 questions generated from the sentences and paragraphs, respectively. A detailed description of the dataset, along with the results achieved by the participants, is given in Rus et al. ( 2012 ). Blšták and Rozinajová ( 2017 , 2018 ) used this dataset to generate questions and compare their performance on correctness to the performance of the systems participating in the shared task.
Medical CBQ corpus (Leo et al. 2019 ) is a corpus of 435 case-based, auto-generated questions that follow four templates (“What is the most likely diagnosis?”, “What is the drug of choice?”, “What is the most likely clinical finding?”, and “What is the differential diagnosis?”). The questions are accompanied by experts’ ratings of appropriateness, difficulty, and actual student performance. The data was used to evaluate an ontology-based approach for generating case-based questions and predicting their difficulty.
MCQL is a corpus of about 7.1K MCQs crawled from the web, with an average of 2.91 distractors per question. The domains of the questions are biology, physics, and chemistry, and they target Cambridge O-level and college-level. The dataset was used in Blšták and Rozinajová ( 2017 ) to develop and evaluate a ML-model for ranking distractors.
Several datasets were used for assessing the ability of question generators to generate similar questions (see Table 8 for an overview). Note that the majority of these datasets were developed for purposes other than education and, as such, the educational value of the questions has not been validated. Therefore, while use of these datasets supports the claim of being able to generate human-like questions, it does not indicate that the generated questions are good or educationally useful. Additionally, restricting the evaluation of generation approaches to the criterion of being able to generate questions that are similar to those in the datasets does not capture their ability to generate other good quality questions that differ in surface structure and semantics.
Some of these datasets were used to develop and evaluate ML-models for ranking distractors. However, being written by humans does not necessarily mean that these distractors are good. This is, in fact, supported by many studies on the quality of distractors in real exam questions (Sarin et al. 1998 ; Tarrant et al. 2009 ; Ware and Vik 2009 ). If these datasets were to be used for similar purposes, distractors would need to be filtered based on their functionality (i.e. being picked by test takers as answers to questions).
We also observe that these datasets have been used in a small number of studies (1-2). This is partially due to the fact that many of them are relatively new. In addition, the design space for question generation is large (i.e. different inputs, question types, and domains). Therefore, each of these datasets is only relevant for a small set of question generators.
Types of Evaluation
The most common evaluation approach is expert-based evaluation (n = 21), in which experts are presented with a sample of generated questions to review. Given that expert review is also a standard procedure for selecting questions for real exams, expert rating is believed to be a good proxy for quality. However, it is important to note that expert review only provides initial evidence for the quality of questions. The questions also need to be administered to a sample of students to obtain further evidence of their quality (empirical difficulty, discrimination, and reliability), as we will see later. However, invalid questions must be filtered first, and expert review is also utilised for this purpose, whereby questions indicated by experts to be invalid (e.g. ambiguous, guessable, or not requiring domain knowledge) are filtered out. Having an appropriate question set is important to keep participants involved in question evaluation motivated and interested in solving these questions.
One of our observations on expert-based evaluation is that only in a few studies were experts required to answer the questions as part of the review. We believe this is an important step to incorporate since answering a question encourages engagement and triggers deeper thinking about what is required to answer. In addition, expert performance on questions is another indicator of question quality and difficulty. Questions answered incorrectly by experts can be ambiguous or very difficult.
Another observation on expert-based evaluation is the ambiguity of instructions provided to experts. For example, in an evaluation of reading comprehension questions (Mostow et al. 2017 ), the authors reported different interpretations of the instructions for rating the overall question quality, whereby one expert pointed out that it is not clear whether reading the preceding text is required in order to rate the question as being of good quality. Researchers have also measured question acceptability, as well as other aspects of questions, using scales with a large number of categories (up to a 9-point scale) without a clear categorisation for each category. Zhang ( 2015 ) found that reviewers perceive scale differently and not all categories of scales are used by all reviewers. We believe that these two issues are reasons for low inter-rater agreement between experts. To improve the accuracy of the data obtained through expert review, researchers must precisely specify the criteria by which to evaluate questions. In addition, a pilot test needs to be conducted with experts to provide an opportunity for validating the instructions and ensuring that instructions and questions are easily understood and interpreted as intended by different respondents.
The second most commonly employed method for evaluation is comparing machine-generated questions (or parts of questions) to human-authored ones (n = 15), which is carried out automatically or as part of the expert review. This comparison is utilised to confirm different aspects of question quality. Zhang and VanLehn ( 2016 ) evaluated their approach by counting the number of questions in common between those that are human- and machine-generated. The authors used this method under the assumption that humans are likely to ask deep questions about topics (i.e. questions of higher cognitive level). On this ground, the authors claimed that an overlap means the machine was able to mimic this in-depth questioning. Other researchers have compared machine-generated questions with human-authored reference questions using metrics borrowed from the fields of text summarisation (ROUGE (Lin 2004 )) and machine translation (BLEU (Papineni et al. 2002 ) and METEOR (Banerjee and Lavie 2005 )). These metrics measure the similarity between two questions generated from the same text segment or sentence. Put simply, this is achieved by counting matching n-grams in the gold-standard question to n-grams in the generated question with some focusing on recall (i.e. how much of the reference question is captured in the generated question) and others focusing on precision (i.e. how much of the generated question is relevant). METEOR also considers stemming and synonymy matching. Wang et al. ( 2018 ) claimed that these metrics can be used as initial, inexpensive, large-scale indicators of the fluency and relevancy of questions. Other researchers investigated whether machine-generated questions are indistinguishable from human-authored questions by mixing both types and asking experts about the source of each question (Chinkina and Meurers 2017 ; Susanti et al. 2015 ; Khodeir et al. 2018 ). Some researchers evaluated their approaches by investigating the ability of the approach to assemble human-authored distractors. For example, Yaneva et al. ( 2018 ) only focused on generating distractors given a question stem and key. However, given the published evidence of the poor quality of human-generated distractors, additional checks need to be performed, such as the functionality of these distractors.
Crowd-sourcing has also been used in ten of the studies. In eight of these, co-workers were employed to review questions while in the remaining three, they were employed to take mock tests. To assess the quality of their responses, Chinkina et al. ( 2017 ) included test questions to make sure that the co-workers understood the task and were able to distinguish low-quality from high-quality questions. However, including a process for validating the reliability of co-workers has been neglected in most studies (or perhaps not reported). Another validation step that can be added to the experimental protocol is conducting a pilot to test the capability of co-workers for review. This can also be achieved by adding validated questions to the list of questions to be reviewed by the co-workers (given the availability of a validated question set).
Similarly, students have been employed to review questions in nine studies and to take tests in a further ten. We attribute the low rate of question validation through testing with student cohorts to it being time-consuming and to the ethical issues involved in these experiments. Experimenters must ensure that these tests do not have an influence on students’ grades or motivations. For example, if multiple auto-generated questions focus on one topic, students could perceive this as an important topic and pay more attention to it while studying for upcoming exams, possibly giving less attention to other topics not covered by the experimental exam. Difficulty of such experimental exams could also affect students. If an experimental test is very easy, students could expect upcoming exams to be the same, again paying less attention when studying for them. Another possible threat is a drop in student motivation triggered by an experimental exam being too difficult.
Finally, for ontology-based approaches, similar to the findings reported in the section “ Findings of Alsubait’s Review ”, most ontologies used in evaluations were hand-crafted for experimental purposes and the use of real ontologies was neglected, except in Vinu and Kumar ( 2015b ), Leo et al. ( 2019 ), and Lopetegui et al. ( 2015 ).
Quality Criteria and Metrics
Table 9 shows the criteria used for evaluating the quality of questions or their components. Some of these criteria concern the linguistic quality of questions, such as grammatical correctness, fluency, semantic ambiguity, freeness from errors, and distractor readability. Others are educationally oriented, such as educational usefulness, domain relevance, and learning outcome. There are also standard quality metrics for assessing questions, such as difficulty, discrimination, and cognitive level. Most of the criteria can be used to evaluate any type of question and only a few are applicable to a specific class of questions, such as the quality of blank (i.e. a word or a phrase that is removed from a segment of text) in gap-fill questions. As can be seen, human-based measures are the most common compared to automatic scoring and statistical procedures. More details about the measurement of these criteria and the results achieved by generation approaches can be found in the Appendix “ Evaluation ”.
Performance of Generation Approaches and Gold Standard Performance
We started this systematic review hoping to identify standard performance and the best generation approaches. However, a comparison between the performances of various approaches was not possible due to heterogeneity in the measurement of quality and reporting of results. For example, scales that consist of different number of categories were used by different studies for measuring the same variables. We were not able to normalise these scales because most studies have only reported aggregated data without providing the number of observations in each rating scale category. Another example of heterogeneity is difficulty based on examinee performance. While some studies use percentage correct, others use Rasch difficulty without providing the raw data to allow the other metric to be calculated. Also, essential information that is needed to judge the trustability and generality of the results, such as sample size and selection method, was not reported in multiple studies. All of these issues preclude a statistical analysis of, and a conclusion about, the performance of generation approaches.
Quality Assessment Results
In this section, we describe and reflect on the state of experimental reporting in the reviewed literature.
Overall, the experimental reporting is unsatisfactory. Essential information that is needed to assess the strength of a study is not reported, raising concerns about trustability and generalisability of the results. For example, the number of evaluated questions, the number of participants involved in evaluations, or both of these numbers are not mentioned in five, ten and five studies, respectively. Information about sampling strategy and how sample size was determined is almost never reported (see the Appendix, “ Quality assessment ”).
A description of the participants’ characteristics, whether experts, students, or co-workers, is frequently missing (neglected by 23 studies). Minimal information that needs to be reported about experts involved in reviewing questions, in addition to their numbers, is their teaching and exam construction experience. Reporting whether experts were paid or not is important for the reader to understand possible biases involved. However, this is not reported in 51 studies involving experiments with human subjects. Other additional helpful information to report is the time taken to review, because this would assist researchers to estimate the number of experts to recruit given a particular sample size, or to estimate the number of questions to sample given the available number of experts.
Characteristics of students involved in evaluations, such as their educational level and experience with the subject under assessment, are important for replication of studies. In addition, this information can provide a basis for combining evidence from multiple studies. For example, we could gain stronger evidence about the effect of specific features on question difficulty by combining studies investigating the same features with different cohorts. In addition, the characteristics of the participants are a possible justification for the difference in difficulty between studies. Similarly, criteria used for the selection of co-workers such as imposing a restriction on which countries they are from, or the number and accuracy of previous tasks in which they participated is important.
Some studies neglect to report on the total number of generated questions and the distribution of questions per categories (question types, difficulty levels, and question sources, when applicable), which are necessary to assess the suitability of sampling strategies. For example, without reporting the distribution of question types, making a claim based on random sampling that “70% of questions are appropriate to be used in exams” would be misleading if the distribution of question types is skewed. This is due to the sample not being representative of question types with a low number of questions. Similarly, if the majority of generated questions are easy, using a random sample will result in the underrepresentation of difficult questions, consequently precluding any conclusion about difficult questions or any comparison between easy and difficult questions.
With regard to measurement descriptions, 10 studies fail to report information sufficient for replication, such as instructions given to participants and a description of the rating scales. Another limitation concerning measurements is the lack of assessment of inter-rater reliability (not reported by 43 studies). In addition, we observed a lack of justification for experimental decisions. Examples of this are the sources from which questions were generated, when particular texts or knowledge sources were selected without any discussion of whether these sources were representative and of what they were representative. We believe that generation challenges and question quality issues that might be encountered when using different sources need to be raised and discussed.
Conclusion and Future Work
In this paper, we have conducted a comprehensive review of 93 papers addressing the automatic generation of questions for educational purposes. In what follows, we summarise our findings in relation to the review objectives.
Providing an Overview of the AQG Community and its Activities
We found that AQG is an increasing activity of a growing community. Through this review, we identified the top publication venues and the active research groups in the field, providing a connection point for researchers interested in the field.
Summarising Current QG Approaches
We found that the majority of QG systems focus on generating questions for the purpose of assessment. The template-based approach was the most common method employed in the reviewed literature. In addition to the generation of complete questions or of question components, a variety of pre- and post-processing tasks that are believed to improve question quality have been investigated. The focus was on the generation of questions from text and for the language domain. The generation of both multiple-choice and free-response questions was almost equally investigated with a large number of studies focusing on wh-word and gap-fill questions. We also found increased interest in generating questions in languages other than English. Although extensive research has been carried out on QG, only a small proportion of these tackle the generation of feedback, verbalisation of questions, and the control of question difficulty.
Identifying Gold Standard performance in AQG
Incomparability of the performance of generation approaches is an issue we identified in the reviewed literature. This issue is due to the heterogeneity in both measurement of quality and reporting of results. We suggest below how the evaluation of questions and reporting of results can be improved to overcome this issue.
Tracking the Evolution of AQG Since Alsubait’s Review
Our results are consistent with the findings of Alsubait ( 2015 ). Based on these findings, we suggest that research in the area can be extended in the following directions (starting at the question level before moving on to the evaluation and research in closely related areas):
Improvement at the Question Level
Generating questions with controlled difficulty.
As mentioned earlier, there is little research on question difficulty and what there is mostly focuses on either stem or distractor difficulty. The difficulty of both stem and options plays a role in overall difficulty and therefore needs to be considered together and not in isolation. Furthermore, controlling MCQ difficulty by varying the similarity between key and distractors is a common feature found in multiple studies. However, similarity is only one facet of difficulty and there are others that need to be identified and integrated into the generation process. Thus, the formulation of a theory behind an intelligent automatic question generator capable of both generating questions and accurately controlling their difficulty is at the heart of AQG research. This would be used for improving the quality of generated questions by filtering inappropriately easy or difficult questions which is especially important given the large number of questions.
Enriching Question Forms and Structures
One of the main limitations of existing works is the simplicity of generated questions, which has also been highlighted in Song and Zhao ( 2016b ). Most generated questions consist of a few terms and target lower cognitive levels. While these questions are still useful, there is a potential for improvement by exploring the generation of other, higher order and more complex, types of questions.
Automating Template Construction
The template library is a major component of question generation systems. At present, the process of template construction is largely manual. The templates are either developed through analysing a set of hand-written questions manually or through consultation with domain experts. While one of the main motivations for generating questions automatically is cost reduction, both of these template acquisition techniques are costly. In addition, there is no evidence that the set of templates defined by a few experts is typical of the set of questions used in assessments. We attribute part of the simplicity of the current questions to the cost, both in terms of time and resources, of both template acquisition techniques.
The cost of generating questions automatically could be reduced further by automatically constructing templates. In addition, this would contribute to the development of more diverse questions.
Employing natural language generation and processing techniques in order to present questions in natural and correct forms and to eliminate errors that invalidate questions, such as syntactic clues, are important steps to take before questions can be used beyond experimental settings for assessment purposes.
As has been seen in both reviews, work on feedback generation is almost non-existent. Developing mechanisms for producing rich, effective feedback is one of the features that needs to be integrated into the generation process. This includes different types of feedback, such as formative, summative, interactive, and personalised feedback.
Improvement of Evaluation Methods
Using human-authored questions for evaluation.
Evaluating question quality, whether by means of expert review or mock exams, is an expensive and time consuming process. Analysing existing exam performance data is a potential source for evaluating question quality and difficulty prediction models. Translating human-authored questions to a machine-processable representation is a possible method for evaluating the ability of generation approaches to generate human-like questions. Regarding the evaluation of difficulty models, this can be done by translating questions to a machine-processable representation, computing the features of these questions, and examining their effect on difficulty. This analysis also provides an understanding of pedagogical content knowledge (i.e. concepts that students often find difficult and usually have misconceptions about). This knowledge can be integrated into difficulty prediction models, or used for question selection and feedback generation.
Standardisation and Development of Automatic Scoring Procedures
To ease comparison between different generation approaches, which was difficult due to heterogeneity in measurement and reporting as well as ungrounded heterogeneity needs to be eliminated. The development of standard and well defined scoring procedures is important to reduce heterogeneity and improve inter-rater reliability. In addition, developing automatic scoring procedures that correlate with human ratings are also important since this will reduce evaluation cost and heterogeneity.
Improvement of Reporting
We also emphasise the need for good experimental reporting. In general, authors should improve reporting on their generation approaches and on evaluation, which are both essential for other researchers who wish to compare their approaches with existing approaches. At a minimum, data extracted in this review (refer to questions under OBJ2 and OBJ3) should be reported in all publications on AQG. To ensure quality, journals can require authors to be complete a checklist prior to peer review, which has shown to improve the reporting quality (Han et al. 2017 ). Alternatively, text-mining techniques can be used for assessing the reporting quality by targeting key information in AQG literature, as has been proposed in Flórez-Vargas et al. ( 2016 ).
Other Areas of Improvement and Further Research
Assembling exams from the generated questions.
Although there is a large amount of work that needs to be done at the question level before moving to the exam level, further work in extending the difficulty models, enriching question form and structure, and improving presentation are steps towards this goal. Research in these directions will open new opportunities for AQG research to move towards assembling exams automatically from generated questions. One of the challenges in exam generation is the selection of a question set that is of appropriate difficulty with good coverage of the material. Ensuring that questions do not overlap or provide clues for other questions also needs to be taken into account. The AQG field could adopt ideas from the question answering field in which question entailment has been investigated (for example, see the work of Abacha and Demner-Fushman ( 2016 )). Finally, ordering questions in a way that increases motivation and maximises the accuracy of scores is another interesting area.
Mining Human-Authored Questions
While existing researchers claim that the questions they generate can be used for educational purposes, these claims are not generally supported. More attention needs to be given to the educational value of generated questions.
In addition to potential use in evaluation, analysing real, good quality exams can help to gain insights into what questions need to be generated so that the generation addresses real life educational needs. This will also help to quantify the characteristics of real questions (e.g. number of terms in real questions) and direct attention to what needs to be done and where the focus should be in order to move to exam generation. Additionally, exam questions reflect what should be included in similar assessments that, in turn, can be further used for content selection and the ranking of questions. For example, concepts extracted from these questions can inform the selection of existing textual or structured sources and the quantifying of whether or not the contents are of educational relevance.
Other potential advantages that the automatic mining of questions offers are the extraction of question templates, a major component of automatic question generators, and improving natural language generation. Besides, mapping the information contained in existing questions to an ontology permits modification of these questions, prediction of their difficulty, and the formation of theories about different aspects of the questions such as their quality.
Similarity Computation and Optimisation
A variety of similarity measures have been used in the context of QG to select content for questions, to select plausible distractors and to control question difficulty (see “ Generation Tasks ” section for examples). Similarity can also be employed in suggesting a diverse set of generated questions (i.e. questions that do not entail the same meaning regardless of their surface structure). Improving computation of the similarity measures (i.e. speed and accuracy) and investigating other types of similarity that might be needed for other question forms are all considered sidelines that have direct implications for improving the current automatic question generation process. Evaluating the performance of existing similarity measures in comparison to each other and whether or not cheap similarity measures can approximate expensive ones are further interesting objects of study.
Source Acquisition and Enrichment
As we have seen in this review, structured knowledge sources have been a popular source for question generation, either by themselves or to complement texts. However, knowledge sources are not available for many domains, while those that are developed for purposes other than QG might not be rich enough to generate good quality questions. Therefore, they need to be adapted or extended before they can be used for QG. As such, investigating different approaches for building or enriching structured knowledge sources and gaining further evidence for the feasibility of obtaining good quality knowledge sources that can be used for question generation, are crucial ingredients for their successful use in question generation.
Limitations
A limitation of this review is the underrepresentation of studies published in languages other than English. In addition, ten papers were excluded because of the unavailability of their full texts.
Questions like those presented in the T.V. show “Jeopardy!”. These questions consist of statements that give hints about the answer. See Faizan and Lohmann ( 2018 ) for an example.
Note that evaluated properties are not necessarily controlled by the generation method. For example, an evaluation could focus on difficulty and discrimination as an indication of quality.
The code and the input files are available at: https://github.com/grkurdi/AQG_systematic_review
The required sample size was calculated using the N.cohen.kappa function (Gamer et al. 2019 ).
This due to the initial description of Q9 being insufficient. However, the agreement improved after refining the description of Q9. demonstrating “moderate agreement”. Footnote 6 Note that Cohen’s kappa was unsuitable for assessing the agreement on the criteria Q6-Q8 due to the unbalanced distribution of responses (e.g. the majority of responses to Q6a were “no”). Since the level of agreement between both reviewers was high, the quality of the remaining studies was assessed by the first author.
Cohen’s kappa was interpreted according to the interpretation provided by Viera et al. ( 2005 ).
The last update of the search was on 3-4-2019.
http://www.questiongeneration.org/
Questions consisting of a text segment followed by a stem of the form: “The word X in paragraph Y is closest in meaning to:” and a set of options. See Susanti et al. ( 2015 ) for more details.
This relates to the processes required to answer questions as characterised in known taxonomies such as Bloom’s taxonomy (Bloom et al. 1956 ), SOLO taxonomy (Biggs and Collis 2014 ) or Webb’s depth of knowledge (Webb 1997 ).
A percentage of 0 means that no one answered the question correctly (highly difficult question), while 100% means that everyone answered the question correctly (extremely easy question).
This can be found at https://rajpurkar.github.io/SQuAD-explorer/
This can be found at https://datasets.maluuba.com/NewsQA
This is a collaboratively created knowledge base.
Available at http://allenai.org/data.html
The dataset can be obtained from https://github.com/bjwyse/QGSTEC2010/blob/master/QGSTEC-Sentences-2010.zip
OpenLearn is an online repository that provides access to learning materials from The Open University.
Abacha, AB, & Demner-Fushman, D. (2016). Recognizing question entailment for medical question answering. In: the AMIA annual symposium, American medical informatics association, p. 310.
Adithya, SSR, & Singh, PK. (2017). Web authoriser tool to build assessments using Wikipedia articles. In: TENCON 2017 - 2017 IEEE region 10 conference, pp. 467–470. https://doi.org/10.1109/TENCON.2017.8227909 .
Afzal, N. (2015). Automatic generation of multiple choice questions using surface-based semantic relations. International Journal of Computational Linguistics (IJCL) , 6 (3), 26–44. https://doi.org/10.1007/s00500-013-1141-4 .
Google Scholar
Afzal, N, & Mitkov, R. (2014). Automatic generation of multiple choice questions using dependency-based semantic relations. Soft Computing , 18 (7), 1269–1281. https://doi.org/10.1007/s00500-013-1141-4 .
Article Google Scholar
Afzal, N, Mitkov, R, Farzindar, A. (2011). Unsupervised relation extraction using dependency trees for automatic generation of multiple-choice questions. In: Canadian conference on artificial intelligence, Springer, pp. 32–43. https://doi.org/10.1007/978-3-642-21043-3_4 .
Ai, R, Krause, S, Kasper, W, Xu, F, Uszkoreit, H. (2015). Semi-automatic generation of multiple-choice tests from mentions of semantic relations. In: the 2nd Workshop on Natural Language Processing Techniques for Educational Applications, pp. 26–33.
Alsubait, T. (2015). Ontology-based question generation . PhD thesis: University of Manchester.
Alsubait, T, Parsia, B, Sattler, U. (2012a). Automatic generation of analogy questions for student assessment: an ontology-based approach. Research in Learning Technology 20. https://doi.org/10.3402/rlt.v20i0.19198 .
Alsubait, T, Parsia, B, Sattler, U. (2012b). Mining ontologies for analogy questions: A similarity-based approach. In: OWLED.
Alsubait, T, Parsia, B, Sattler, U. (2012c). Next generation of e-assessment: automatic generation of questions. International Journal of Technology Enhanced Learning , 4 (3-4), 156–171.
Alsubait, T, Parsia, B, Sattler, U. (2013). A similarity-based theory of controlling MCQ difficulty. In 2013 2Nd international conference on e-learning and e-technologies in education (pp. 283–288). ICEEE: IEEE.. https://doi.org/10.1109/ICeLeTE.2013.664438
Alsubait, T, Parsia, B, Sattler, U. (2014a). Generating multiple choice questions from ontologies: Lessons learnt. In: OWLED, Citeseer, pp. 73–84.
Alsubait, T, Parsia, B, Sattler, U. (2014b). Generating multiple questions from ontologies: How far can we go? In: the 1st International Workshop on Educational Knowledge Management (EKM 2014), Linköping University Electronic Press, pp. 19–30.
Alsubait, T, Parsia, B, Sattler, U. (2016). Ontology-based multiple choice question generation. KI - Kü,nstliche Intelligenz , 30 (2), 183–188. https://doi.org/10.1007/s13218-015-0405-9 .
Araki, J, Rajagopal, D, Sankaranarayanan, S, Holm, S, Yamakawa, Y, Mitamura, T. (2016). Generating questions and multiple-choice answers using semantic analysis of texts. In The 26th international conference on computational linguistics (COLING , (Vol. 2016 pp. 1125–1136).
Banerjee, S, & Lavie, A. (2005). METEOR: An automatic metric for MT evaluation with improved correlation with human judgments. In: the ACL Workshop on Intrinsic and Extrinsic Evaluation Measures for Machine Translation and/or Summarization, pp. 65–72.
Basuki, S, & Kusuma, S F. (2018). Automatic question generation for 5w-1h open domain of Indonesian questions by using syntactical template-based features from academic textbooks. Journal of Theoretical and Applied Information Technology , 96 (12), 3908–3923.
Baturay, M H. (2015). An overview of the world of MOOCs. Procedia - Social and Behavioral Sciences , 174 , 427–433. https://doi.org/10.1016/j.sbspro.2015.01.685 .
Beck, JE, Mostow, J, Bey, J. (2004). Can automated questions scaffold children’s reading comprehension? In: International Conference on Intelligent Tutoring Systems, Springer, pp. 478–490.
Bednarik, L, & Kovacs, L. (2012a). Automated EA-type question generation from annotated texts, IEEE, SACI. https://doi.org/10.1109/SACI.2012.6250000 .
Bednarik, L, & Kovacs, L. (2012b). Implementation and assessment of the automatic question generation module, IEEE, CogInfoCom. https://doi.org/10.1109/CogInfoCom.2012.6421938 .
Biggs, J B, & Collis, KF. (2014). Evaluating the quality of learning: The SOLO taxonomy (Structure of the Observed Learning Outcome) . Cambridge: Academic Press.
Bloom, B S, Engelhart, M D, Furst, E J, Hill, W H, Krathwohl, D R. (1956). Taxonomy of educational objectives, handbook i: The cognitive domain vol 19 . New York: David McKay Co Inc.
Blšták, M. (2018). Automatic question generation based on sentence structure analysis. Information Sciences & Technologies: Bulletin of the ACM Slovakia , 10 (2), 1–5.
Blšták, M., & Rozinajová, V. (2017). Machine learning approach to the process of question generation. In Blšták, M., & Rozinajová, V. (Eds.) Text, speech, and dialogue (pp. 102–110). Cham: Springer International Publishing.. https://doi.org/10.1007/978-3-319-64206-2_12
Blšták, M., & Rozinajová, V. (2018). Building an agent for factual question generation task. In 2018 World symposium on digital intelligence for systems and machines (DISA) (pp. 143–150). IEEE.. https://doi.org/10.1109/DISA.2018.8490637
Bodenreider, O. (2004). The unified medical language system (UMLS): integrating biomedical terminology. Nucleic acids research , 32 (suppl_1), D267–D270. https://doi.org/10.1093/nar/gkh061 .
Boland, A, Cherry, M G, Dickson, R. (2013). Doing a systematic review: A student’s guide. Sage.
Ch, DR, & Saha, SK. (2018). Automatic multiple choice question generation from text: A survey. IEEE Transactions on Learning Technologies https://doi.org/10.1109/TLT.2018.2889100 , in press.
Chen, CY, Liou, HC, Chang, JS. (2006). Fast: an automatic generation system for grammar tests. In: the COLING/ACL on interactive presentation sessions, association for computational linguistics, pp. 1–4.
Chinkina, M, & Meurers, D. (2017). Question generation for language learning: From ensuring texts are read to supporting learning. In: the 12th workshop on innovative use of NLP for building educational applications, pp. 334–344.
Chinkina, M, Ruiz, S, Meurers, D. (2017). Automatically generating questions to support the acquisition of particle verbs: evaluating via crowdsourcing. In: CALL in a climate of change: adapting to turbulent global conditions, pp. 73–78.
Critical Appraisal Skills Programme. (2018). CASP qualitative checklist. https://casp-uk.net/wp-content/uploads/2018/03/CASP-Qualitative-Checklist-Download.pdf , accessed: 2018-09-07.
Das, B, & Majumder, M. (2017). Factual open cloze question generation for assessment of learner’s knowledge. International Journal of Educational Technology in Higher Education , 14 (1), 24. https://doi.org/10.1186/s41239-017-0060-3 .
Donnelly, K. (2006). SNOMED-CT: The Advanced terminology and coding system for eHealth. Studies in health technology and informatics , 121 , 279–290.
Downs, S H, & Black, N. (1998). The feasibility of creating a checklist for the assessment of the methodological quality both of randomised and non-randomised studies of health care interventions. Journal of Epidemiology & Community Health , 52 (6), 377–384.
Fairon, C. (1999). A web-based system for automatic language skill assessment: Evaling. In: Symposium on computer mediated language assessment and evaluation in natural language processing, association for computational linguistics, pp. 62–67.
Faizan, A, & Lohmann, S. (2018). Automatic generation of multiple choice questions from slide content using linked data. In: the 8th International Conference on Web Intelligence, Mining and Semantics.
Faizan, A, Lohmann, S, Modi, V. (2017). Multiple choice question generation for slides. In: Computer Science Conference for University of Bonn Students, pp. 1–6.
Fattoh, I E, Aboutabl, A E, Haggag, M H. (2015). Semantic question generation using artificial immunity. International Journal of Modern Education and Computer Science , 7 (1), 1–8.
Flor, M, & Riordan, B. (2018). A semantic role-based approach to open-domain automatic question generation. In: the 13th Workshop on Innovative Use of NLP for Building Educational Applications, pp. 254–263.
Flórez-Vargas, O., Brass, A, Karystianis, G, Bramhall, M, Stevens, R, Cruickshank, S, Nenadic, G. (2016). Bias in the reporting of sex and age in biomedical research on mouse models. eLife 5(e13615).
Gaebel, M, Kupriyanova, V, Morais, R, Colucci, E. (2014). E-learning in European higher education institutions: Results of a mapping survey conducted in october-December 2013 . Tech. rep.: European University Association.
Gamer, M, Lemon, J, Gamer, MM, Robinson, A, Kendall’s, W. (2019). Package ’irr’. https://cran.r-project.org/web/packages/irr/irr.pdf .
Gao, Y, Wang, J, Bing, L, King, I, Lyu. MR. (2018). Difficulty controllable question generation for reading comprehension. Tech. rep.
Goldbach, IR, & Hamza-Lup, FG. (2017). Survey on e-learning implementation in Eastern-Europe spotlight on Romania. In: the Ninth International Conference on Mobile, Hybrid, and On-Line Learning.
Gupta, M, Gantayat, N, Sindhgatta, R. (2017). Intelligent math tutor: Problem-based approach to create cognizance. In: the 4th ACM Conference on Learning@ Scale, ACM, pp. 241–244.
Han, S, Olonisakin, T F, Pribis, J P, Zupetic, J, Yoon, J H, Holleran, K M, Jeong, K, Shaikh, N, Rubio, D M, Lee, J S. (2017). A checklist is associated with increased quality of reporting preclinical biomedical research: a systematic review. PloS One , 12 (9), e0183591.
Hansen, J D, & Dexter, L. (1997). Quality multiple-choice test questions: Item-writing guidelines and an analysis of auditing testbanks. Journal of Education for Business , 73 (2), 94–97. https://doi.org/10.1080/08832329709601623 .
Heilman, M. (2011). Automatic factual question generation from text . PhD thesis: Carnegie Mellon University.
Heilman, M, & Smith, NA. (2009). Ranking automatically generated questions as a shared task. In: The 2nd Workshop on Question Generation, pp. 30–37.
Heilman, M, & Smith, NA. (2010a). Good question! statistical ranking for question generation. In: Human language technologies: The 2010 annual conference of the north american chapter of the association for computational linguistics, association for computational linguistics, pp. 609–617.
Heilman, M, & Smith, NA. (2010b). Rating computer-generated questions with mechanical turk. In: the NAACL HLT 2010 workshop on creating speech and language data with amazon’s mechanical turk, association for computational linguistics, pp. 35–40.
Hill, J, & Simha, R. (2016). Automatic generation of context-based fill-in-the-blank exercises using co-occurrence likelihoods and Google n-grams. In: the 11th workshop on innovative use of NLP for building educational applications, pp. 23–30.
Hingorjo, M R, & Jaleel, F. (2012). Analysis of one-best MCQs: the difficulty index, discrimination index and distractor efficiency. The Journal of the Pakistan Medical Association (JPMA) , 62 (2), 142–147.
Huang, Y, & He, L. (2016). Automatic generation of short answer questions for reading comprehension assessment. Natural Language Engineering , 22 (3), 457–489. https://doi.org/10.1017/S1351324915000455 .
Article MathSciNet Google Scholar
Huang, Y T, & Mostow, J. (2015). Evaluating human and automated generation of distractors for diagnostic multiple-choice cloze questions to assess children’s reading comprehension. In Conati, C., Heffernan, N., Mitrovic, A., Verdejo, M.F. (Eds.) Artificial intelligence in education (pp. 155–164). Cham: Springer International Publishing.
Huang, Y T, Tseng, Y M, Sun, Y S, Chen, MC. (2014). TEDQuiz: automatic quiz generation for TED talks video clips to assess listening comprehension. In 2014 IEEE 14Th international conference on advanced learning technologies (pp. 350–354). ICALT: IEEE.
Jiang, S, & Lee, J. (2017). Distractor generation for Chinese fill-in-the-blank items. In: the 12th workshop on innovative use of NLP for building educational applications, pp. 143–148.
Jouault, C, & Seta, K. (2014). Content-dependent question generation for history learning in semantic open learning space. In: The international conference on intelligent tutoring systems, Springer, pp. 300–305.
Jouault, C, Seta, K, Hayashi, Y. (2015a). A method for generating history questions using LOD and its evaluation. SIG-ALST of The Japanese Society for Artificial Intelligence , B5 (1), 28–33.
Jouault, C, Seta, K, Hayashi, Y. (2015b). Quality of LOD based semantically generated questions. In Conati, C., Heffernan, N., Mitrovic, A, Verdejo, M.F. (Eds.) Artificial intelligence in education (pp. 662–665). Cham: Springer International Publishing.
Jouault, C, Seta, K, Hayashi, Y. (2016a). Content-dependent question generation using LOD for history learning in open learning space. New Generation Computing , 34 (4), 367–394. https://doi.org/10.1007/s00354-016-0404-x .
Jouault, C, Seta, K, Yuki, H, et al. (2016b). Can LOD based question generation support work in a learning environment for history learning?. SIG-ALST , 5 (03), 37–41.
Jouault, C, Seta, K, Hayashi, Y. (2017). SOLS: An LOD based semantically enhanced open learning space supporting self-directed learning of history. IEICE Transactions on Information and Systems , 100 (10), 2556–2566.
Kaur, A, & Singh, S. (2017). Automatic question generation system for Punjabi. In: The international conference on recent innovations in science, Agriculture, Engineering and Management.
Kaur, J, & Bathla, A K. (2015). A review on automatic question generation system from a given Hindi text. International Journal of Research in Computer Applications and Robotics (IJRCAR) , 3 (6), 87–92.
Khodeir, N A, Elazhary, H, Wanas, N. (2018). Generating story problems via controlled parameters in a web-based intelligent tutoring system. The International Journal of Information and Learning Technology , 35 (3), 199–216.
Killawala, A, Khokhlov, I, Reznik, L. (2018). Computational intelligence framework for automatic quiz question generation. In: 2018 IEEE International Conference on Fuzzy Systems (FUZZ-IEEE), pp. 1–8. https://doi.org/10.1109/FUZZ-IEEE.2018.8491624 .
Kitchenham, B, & Charters, S. (2007). Guidelines for performing systematic literature reviews in software engineering . Tech. rep.: Keele University and University of Durham.
Kovacs, L, & Szeman, G. (2013). Complexity-based generation of multi-choice tests in AQG systems, IEEE, CogInfoCom. https://doi.org/10.1109/CogInfoCom.2013.6719278 .
Kumar, G, Banchs, R, D’Haro, LF. (2015a). Revup: Automatic gap-fill question generation from educational texts. In: the 10th workshop on innovative use of NLP for building educational applications, pp. 154–161.
Kumar, G, Banchs, R, D’Haro, LF. (2015b). Automatic fill-the-blank question generator for student self-assessment. In: IEEE Frontiers in Education Conference (FIE), pp. 1–3. https://doi.org/10.1109/FIE.2015.7344291 .
Kumar, V, Boorla, K, Meena, Y, Ramakrishnan, G, Li, Y F. (2018). Automating reading comprehension by generating question and answer pairs. In Phung, D., Tseng, V.S., Webb, G.I., Ho, B., Ganji, M., Rashidi, L. (Eds.) Advances in knowledge discovery and data mining (pp. 335–348). Cham: Springer International Publishing.. https://doi.org/10.1007/978-3-319-93040-4_27
Kurdi, G, Parsia, B, Sattler, U. (2017). An experimental evaluation of automatically generated multiple choice questions from ontologies. In Dragoni, M., Poveda-Villalón, M., Jimenez-Ruiz, E. (Eds.) OWL: Experiences And directions – reasoner evaluation (pp. 24–39). Cham: Springer International Publishing.. https://doi.org/10.1007/978-3-319-54627-8_3
Kurdi, G, Leo, J, Matentzoglu, N, Parsia, B, Forege, S, Donato, G, Dowling, W. (2019). A comparative study of methods for a priori prediction of MCQ difficulty. the Semantic Web journal, In press.
Kusuma, S F, & Alhamri, R Z. (2018). Generating Indonesian question automatically based on Bloom’s taxonomy using template based method. KINETIK: Game Technology, Information System, Computer Network, Computing, Electronics, and Control , 3 (2), 145–152.
Kwankajornkiet, C, Suchato, A, Punyabukkana, P. (2016). Automatic multiple-choice question generation from Thai text. In: the 13th International Joint Conference on Computer Science and Software Engineering (JCSSE), pp. 1–6. https://doi.org/10.1109/JCSSE.2016.7748891 .
Le, N T, Kojiri, T, Pinkwart, N. (2014). Automatic question generation for educational applications – the state of art. In van Do, T., Thi, H.A.L, Nguyen, N.T. (Eds.) Advanced computational methods for knowledge engineering (pp. 325–338). Cham: Springer International Publishing.
Lee, CH, Chen, TY, Chen, LP, Yang, PC, Tsai, RTH. (2018). Automatic question generation from children’s stories for companion chatbot. In: 2018 IEEE International Conference on Information Reuse and Integration (IRI), pp. 491–494. https://doi.org/10.1109/IRI.2018.00078 .
Leo, J, Kurdi, G, Matentzoglu, N, Parsia, B, Forege, S, Donato, G, Dowling, W. (2019). Ontology-based generation of medical, multi-term MCQs. International Journal of Artificial Intelligence, in Education. https://doi.org/10.1007/s40593-018-00172-w .
Liang, C, Yang, X, Wham, D, Pursel, B, Passonneau, R, Giles, CL. (2017). Distractor generation with generative adversarial nets for automatically creating fill-in-the-blank questions. In: the Knowledge Capture Conference, p. 33. https://doi.org/10.1145/3148011.3154463 .
Liang, C, Yang, X, Dave, N, Wham, D, Pursel, B, Giles, CL. (2018). Distractor generation for multiple choice questions using learning to rank. In: the 13th Workshop on Innovative Use of NLP for Building Educational Applications, pp. 284–290. https://doi.org/10.18653/v1/W18-0533 .
Lim, C S, Tang, K N, Kor, L K. (2012). Drill and practice in learning (and Beyond), Springer US, pp. 1040–1042. https://doi.org/10.1007/978-1-4419-1428-6_706 .
Lin, C, Liu, D, Pang, W, Apeh, E. (2015). Automatically predicting quiz difficulty level using similarity measures. In: the 8th International Conference on Knowledge Capture, ACM.
Lin, CY. (2004). ROUGE: A package for automatic evaluation of summaries. In: the Workshop on Text Summarization Branches Out.
Liu, M, & Calvo, RA. (2012). Using information extraction to generate trigger questions for academic writing support. In: the International Conference on Intelligent Tutoring Systems, Springer, pp. 358–367. https://doi.org/10.1007/978-3-642-30950-2_47 .
Liu, M, Calvo, RA, Aditomo, A, Pizzato, LA. (2012a). Using Wikipedia and conceptual graph structures to generate questions for academic writing support. IEEE Transactions on Learning Technologies , 5 (3), 251–263. https://doi.org/10.1109/TLT.2012.5 .
Liu, M, Calvo, RA, Rus, V. (2012b). G-Asks: An intelligent automatic question generation system for academic writing support. Dialogue & Discourse , 3 (2), 101–124. https://doi.org/10.5087/dad.2012.205 .
Liu, M, Calvo, R A, Rus, V. (2014). Automatic generation and ranking of questions for critical review. Journal of Educational Technology & Society , 17 (2), 333–346.
Liu, M, Rus, V, Liu, L. (2017). Automatic Chinese factual question generation. IEEE Transactions on Learning Technologies , 10 (2), 194–204. https://doi.org/10.1109/TLT.2016.2565477 .
Liu, M, Rus, V, Liu, L. (2018). Automatic Chinese multiple choice question generation using mixed similarity strategy. IEEE Transactions on Learning Technologies , 11 (2), 193–202. https://doi.org/10.1109/TLT.2017.2679009 .
Lopetegui, MA, Lara, BA, Yen, PY, Çatalyürek, Ü.V., Payne, PR. (2015). A novel multiple choice question generation strategy: alternative uses for controlled vocabulary thesauri in biomedical-sciences education. In: the AMIA annual symposium, american medical informatics association, pp. 861–869.
Majumder, M, & Saha, SK. (2015). A system for generating multiple choice questions: With a novel approach for sentence selection. In: the 2nd workshop on natural language processing techniques for educational applications, pp. 64–72.
Marrese-Taylor, E, Nakajima, A, Matsuo, Y, Yuichi, O. (2018). Learning to automatically generate fill-in-the-blank quizzes. In: the 5th workshop on natural language processing techniques for educational applications. https://doi.org/10.18653/v1/W18-3722 .
Mazidi, K. (2018). Automatic question generation from passages. In Gelbukh, A. (Ed.) Computational linguistics and intelligent text processing (pp. 655–665). Cham: Springer International Publishing.
Mazidi, K, & Nielsen, RD. (2014). Linguistic considerations in automatic question generation. In: the 52nd annual meeting of the association for computational linguistics, pp. 321–326.
Mazidi, K, & Nielsen, R D. (2015). Leveraging multiple views of text for automatic question generation. In Conati, C., Heffernan, N., Mitrovic, A., Verdejo, M.F. (Eds.) Artificial intelligence in education (pp. 257–266). Cham: Springer International Publishing.
Mazidi, K, & Tarau, P. (2016a). Automatic question generation: From NLU to NLG Micarelli, A., Stamper, J., Panourgia K. (Eds.), Springer International Publishing, Cham. https://doi.org/10.1007/978-3-319-39583-8_3 .
Mazidi, K, & Tarau, P. (2016b). Infusing NLU into automatic question generation. In: the 9th International Natural Language Generation conference, pp. 51–60.
Miller, G A, Beckwith, R, Fellbaum, C, Gross, D, Miller, K J. (1990). Introduction to WordNet: An on-line lexical database. International Journal of Lexicography , 3 (4), 235–244.
Mitkov, R, & Ha, L A. (2003). Computer-aided generation of multiple-choice tests. In The HLT-NAACL 03 workshop on building educational applications using natural language processing, association for computational linguistics, pp. 17–22 .
Mitkov, R, Le An, H, Karamanis, N. (2006). A computer-aided environment for generating multiple-choice test items. Natural language engineering , 12 (2), 177–194. https://doi.org/10.1017/S1351324906004177 .
Montenegro, C S, Engle, V G, Acuba, M G J, Ferrenal, A M A. (2012). Automated question generator for Tagalog informational texts using case markers. In TENCON 2012-2012 IEEE region 10 conference, IEEE, pp. 1–5 . https://doi.org/10.1109/TENCON.2012.6412273 .
Mostow, J, & Chen, W. (2009). Generating instruction automatically for the reading strategy of self-questioning. In: the 14th international conference artificial intelligence in education, pp. 465–472.
Mostow, J, Beck, J, Bey, J, Cuneo, A, Sison, J, Tobin, B, Valeri, J. (2004). Using automated questions to assess reading comprehension, vocabulary, and effects of tutorial interventions. Technology Instruction Cognition and Learning , 2 , 97–134.
Mostow, J, Yt, Huang, Jang, H, Weinstein, A, Valeri, J, Gates, D. (2017). Developing, evaluating, and refining an automatic generator of diagnostic multiple choice cloze questions to assess children’s comprehension while reading. Natural Language Engineering , 23 (2), 245–294. https://doi.org/10.1017/S1351324916000024 .
Niraula, NB, & Rus, V. (2015). Judging the quality of automatically generated gap-fill question using active learning. In: the 10th workshop on innovative use of NLP for building educational applications, pp. 196–206.
Odilinye, L, Popowich, F, Zhang, E, Nesbit, J, Winne, PH. (2015). Aligning automatically generated questions to instructor goals and learner behaviour. In: the IEEE 9th international conference on semantic computing (ICS), pp. 216–223. https://doi.org/10.1109/ICOSC.2015.7050809 .
Olney, A M, Pavlik, P I, Maass, J K. (2017). Improving reading comprehension with automatically generated cloze item practice. In André, E., Baker, R., Hu, X., Rodrigo, M.M.T., du Boulay, B. (Eds.) Artificial intelligence in education . https://doi.org/10.1007/978-3-319-61425-0_22 (pp. 262–273). Cham: Springer International Publishing.
Papasalouros, A, & Chatzigiannakou, M. (2018). Semantic web and question generation: An overview of the state of the art. In: The international conference e-learning, pp. 189–192.
Papineni, K, Roukos, S, Ward, T, Zhu, WJ. (2002). BLEU: a method for automatic evaluation of machine translation. In: the 40th annual meeting on association for computational linguistics, Association for computational linguistics, pp. 311–318.
Park, J, Cho, H, Sg, Lee. (2018). Automatic generation of multiple-choice fill-in-the-blank question using document embedding. In Penstein Rosé, C., Martínez-Maldonado, R., Hoppe, H.U., Luckin, R., Mavrikis, M., Porayska-Pomsta, K., McLaren, B., du Boulay, B. (Eds.) Artificial intelligence in education (pp. 261–265). Cham: Springer International Publishing.
Patra, R, & Saha, SK. (2018a). Automatic generation of named entity distractors of multiple choice questions using web information Pattnaik, P.K., Rautaray, SS, Das, H, Nayak, J (Eds.), Springer, Berlin.
Patra, R, & Saha, SK. (2018b). A hybrid approach for automatic generation of named entity distractors for multiple choice questions. Education and Information Technologies pp. 1–21.
Polozov, O, O’Rourke, E, Smith, A M, Zettlemoyer, L, Gulwani, S, Popovic, Z. (2015). Personalized mathematical word problem generation. In The 24th international joint conference on artificial intelligence (IJCAI 2015), pp. 381–388 .
Qayyum, A, & Zawacki-Richter, O. (2018). Distance education in Australia, Europe and the americas, Springer, Berlin.
Rajpurkar, P, Zhang, J, Lopyrev, K, Liang, P. (2016). Squad: 100,000+ questions for machine comprehension of text. In: the 2016 conference on empirical methods in natural language processing, pp. 2383–2392.
Rakangor, S, & Ghodasara, Y R. (2015). Literature review of automatic question generation systems. International Journal of Scientific and Research Publications , 5 (1), 2250–3153.
Reisch, J S, Tyson, J E, Mize, S G. (1989). Aid to the evaluation of therapeutic studies. Pediatrics , 84 (5), 815–827.
Rocha, OR, & Zucker, CF. (2018). Automatic generation of quizzes from DBpedia according to educational standards. In: the 3rd educational knowledge management workshop (EKM).
Rus, V, Wyse, B, Piwek, P, Lintean, M, Stoyanchev, S, Moldovan, C. (2012). A detailed account of the first question generation shared task evaluation challenge. Dialogue & Discourse , 3 (2), 177–204.
Rush, B R, Rankin, D C, White, B J. (2016). The impact of item-writing flaws and item complexity on examination item difficulty and discrimination value. BMC Medical Education , 16 (1), 250. https://doi.org/10.1186/s12909-016-0773-3 .
Santhanavijayan, A, Balasundaram, S, Narayanan, S H, Kumar, S V, Prasad, V V. (2017). Automatic generation of multiple choice questions for e-assessment. International Journal of Signal and Imaging Systems Engineering , 10 (1-2), 54–62.
Sarin, Y, Khurana, M, Natu, M, Thomas, A G, Singh, T. (1998). Item analysis of published MCQs. Indian Pediatrics , 35 , 1103–1104.
Satria, AY, & Tokunaga, T. (2017a). Automatic generation of english reference question by utilising nonrestrictive relative clause. In: the 9th international conference on computer supported education, pp. 379–386. https://doi.org/10.5220/0006320203790386 .
Satria, AY, & Tokunaga, T. (2017b). Evaluation of automatically generated pronoun reference questions. In: the 12th workshop on innovative use of NLP for building educational applications, pp. 76–85.
Serban, IV, García-Durán, A., Gulcehre, C, Ahn, S, Chandar, S, Courville, A, Bengio, Y. (2016). Generating factoid questions with recurrent neural networks: The 30M factoid question-answer corpus. ACL.
Seyler, D, Yahya, M, Berberich, K. (2017). Knowledge questions from knowledge graphs. In: The ACM SIGIR international conference on theory of information retrieval, pp. 11–18.
Shah, R, Shah, D, Kurup, L. (2017). Automatic question generation for intelligent tutoring systems. In: the 2nd international conference on communication systems, computing and it applications (CSCITA), pp. 127–132. https://doi.org/10.1109/CSCITA.2017.8066538 .
Shenoy, V, Aparanji, U, Sripradha, K, Kumar, V. (2016). Generating DFA construction problems automatically. In: The international conference on learning and teaching in computing and engineering (LATICE), pp. 32–37. https://doi.org/10.1109/LaTiCE.2016.8 .
Shirude, A, Totala, S, Nikhar, S, Attar, V, Ramanand, J. (2015). Automated question generation tool for structured data. In: International conference on advances in computing, communications and informatics (ICACCI), pp. 1546–1551. https://doi.org/10.1109/ICACCI.2015.7275833 .
Singhal, R, & Henz, M. (2014). Automated generation of region based geometric questions.
Singhal, R, Henz, M, Goyal, S. (2015a). A framework for automated generation of questions across formal domains. In: the 17th international conference on artificial intelligence in education, pp. 776–780.
Singhal, R, Henz, M, Goyal, S. (2015b). A framework for automated generation of questions based on first-order logic Conati, C., Heffernan, N., Mitrovic, A., Verdejo, M.F. (Eds.), Springer International Publishing, Cham.
Singhal, R, Goyal, R, Henz, M. (2016). User-defined difficulty levels for automated question generation. In: the IEEE 28th international conference on tools with artificial intelligence (ICTAI), pp. 828–835. https://doi.org/10.1109/ICTAI.2016.0129 .
Song, L, & Zhao, L. (2016a). Domain-specific question generation from a knowledge base. Tech. rep.
Song, L, & Zhao, L. (2016b). Question generation from a knowledge base with web exploration. Tech. rep.
Soonklang, T, & Muangon, W. (2017). Automatic question generation system for English exercise for secondary students. In: the 25th international conference on computers in education.
Stasaski, K, & Hearst, MA. (2017). Multiple choice question generation utilizing an ontology. In: the 12th workshop on innovative use of NLP for building educational applications, pp. 303–312.
Susanti, Y, Iida, R, Tokunaga, T. (2015). Automatic generation of English vocabulary tests. In: the 7th international conference on computer supported education, pp. 77–87.
Susanti, Y, Nishikawa, H, Tokunaga, T, Hiroyuki, O. (2016). Item difficulty analysis of English vocabulary questions. In The 8th international conference on computer supported education (CSEDU 2016), pp. 267–274 .
Susanti, Y, Tokunaga, T, Nishikawa, H, Obari, H. (2017a). Controlling item difficulty for automatic vocabulary question generation. Research and Practice in Technology Enhanced Learning , 12 (1), 25. https://doi.org/10.1186/s41039-017-0065-5 .
Susanti, Y, Tokunaga, T, Nishikawa, H, Obari, H. (2017b). Evaluation of automatically generated English vocabulary questions. Research and Practice in Technology Enhanced Learning 12(1). https://doi.org/10.1186/s41039-017-0051-y .
Tamura, Y, Takase, Y, Hayashi, Y, Nakano, Y I. (2015). Generating quizzes for history learning based on Wikipedia articles. In Zaphiris, P., & Ioannou, A. (Eds.) Learning and collaboration technologies (pp. 337–346). Cham: Springer International Publishing.
Tarrant, M, Knierim, A, Hayes, S K, Ware, J. (2006). The frequency of item writing flaws in multiple-choice questions used in high stakes nursing assessments. Nurse Education in Practice , 6 (6), 354–363. https://doi.org/10.1016/j.nepr.2006.07.002 .
Tarrant, M, Ware, J, Mohammed, A M. (2009). An assessment of functioning and non-functioning distractors in multiple-choice questions: a descriptive analysis. BMC Medical Education , 9 (1), 40. https://doi.org/10.1186/1472-6920-9-40 .
Thalheimer, W. (2003). The learning benefits of questions. Tech. rep., Work Learning Research. http://www.learningadvantage.co.za/pdfs/questionmark/LearningBenefitsOfQuestions.pdf .
Thomas, A, Stopera, T, Frank-Bolton, P, Simha, R. (2019). Stochastic tree-based generation of program-tracing practice questions. In: the 50th ACM technical symposium on computer science education, ACM, pp. 91–97.
Vie, J J, Popineau, F, Bruillard, É., Bourda, Y. (2017). A review of recent advances in adaptive assessment, Springer, Berlin.
Viera, A J, Garrett, J M, et al. (2005). Understanding interobserver agreement: the kappa statistic. Family Medicine , 37 (5), 360–363.
Vinu, EV, & Kumar, PS. (2015a). Improving large-scale assessment tests by ontology based approach. In: the 28th international florida artificial intelligence research society conference, pp. 457– 462.
Vinu, EV, & Kumar, PS. (2015b). A novel approach to generate MCQs from domain ontology: Considering DL semantics and open-world assumption. Web Semantics: Science, Services and Agents on the World Wide Web , 34 , 40–54. https://doi.org/10.1016/j.websem.2015.05.005 .
Vinu, EV, & Kumar, PS. (2017a). Automated generation of assessment tests from domain ontologies. Semantic Web Journal , 8 (6), 1023–1047. https://doi.org/10.3233/SW-170252 .
Vinu, EV, & Kumar, PS. (2017b). Difficulty-level modeling of ontology-based factual questions. Semantic Web Journal In press.
Vinu, E V, Alsubait, T, Kumar, PS. (2016). Modeling of item-difficulty for ontology-based MCQs. Tech. rep.
Wang, K, & Su, Z. (2016). Dimensionally guided synthesis of mathematical word problems. In: the 25th International Joint Conference on Artificial Intelligence (IJCAI), pp. 2661–2668.
Wang, K, Li, T, Han, J, Lei, Y. (2012). Algorithms for automatic generation of logical questions on mobile devices. IERI Procedia , 2 , 258–263. https://doi.org/10.1016/j.ieri.2012.06.085 .
Wang, Z, Lan, AS, Nie, W, Waters, AE, Grimaldi, PJ, Baraniuk, RG. (2018). QG-net: a data-driven question generation model for educational content. In: the 5th Annual ACM Conference on Learning at Scale, pp. 15–25.
Ware, J, & Vik, T. (2009). Quality assurance of item writing: During the introduction of multiple choice questions in medicine for high stakes examinations. Medical Teacher , 31 (3), 238–243. https://doi.org/10.1080/01421590802155597 .
Webb, N L. (1997). Criteria for alignment of expectations and assessments in mathematics and science education . Tech. rep.: National Institute for Science Education.
Welbl, J, Liu, NF, Gardner, M. (2017). Crowdsourcing multiple choice science questions. In: the 3rd workshop on noisy user-generated text, pp. 94–106.
Wita, R, Oly, S, Choomok, S, Treeratsakulchai, T, Wita, S. (2018). A semantic graph-based Japanese vocabulary learning game. In Hancke, G., Spaniol, M., Osathanunkul, K., Unankard, S., Klamma, R. (Eds.) Advances in web-based learning – ICWL , (Vol. 2018 pp. 140–145). Cham: Springer International Publishing.. https://doi.org/10.1007/978-3-319-96565-9_14
Yaneva, V, & et al. (2018). Automatic distractor suggestion for multiple-choice tests using concept embeddings and information retrieval. In: the 13th workshop on innovative use of NLP for building educational applications, pp. 389–398.
Yao, X, Bouma, G, Zhang, Y. (2012). Semantics-based question generation and implementation. Dialogue & Discourse , 3 (2), 11–42.
Zavala, L, & Mendoza, B. (2018). On the use of semantic-based AIG to automatically generate programming exercises. In: the 49th ACM technical symposium on computer science education, ACM, pp. 14–19.
Zhang, J, & Takuma, J. (2015). A Kanji learning system based on automatic question sentence generation. In: 2015 international conference on asian language processing (IALP), pp. 144–147. https://doi.org/10.1109/IALP.2015.7451552 .
Zhang, L. (2015). Biology question generation from a semantic network . PhD thesis: Arizona State University.
Zhang, L, & VanLehn, K. (2016). How do machine-generated questions compare to human-generated questions?. Research and Practice in Technology Enhanced Learning, 11(7). https://doi.org/10.1186/s41039-016-0031-7 .
Zhang, T, Quan, P, et al. (2018). Domain specific automatic Chinese multiple-type question generation. In 2018 IEEE International Conference on Bioinformatics and Biomedicine (BIBM), IEEE, pp. 1967–1971 . https://doi.org/10.1109/BIBM.2018.8621162 .
Download references
Author information
Authors and affiliations.
Department of Computer Science, The University of Manchester, Manchester, UK
Ghader Kurdi, Jared Leo, Bijan Parsia & Uli Sattler
Umm Al-Qura University, Mecca, Saudi Arabia
Salam Al-Emari
You can also search for this author in PubMed Google Scholar
Corresponding author
Correspondence to Ghader Kurdi .
Additional information
Publisher’s note.
Springer Nature remains neutral with regard to jurisdictional claims in published maps and institutional affiliations.
Excluded Studies
Publication venues, active research groups, summary of included studies, quality assessment, rights and permissions.
Open Access This article is distributed under the terms of the Creative Commons Attribution 4.0 International License (http://creativecommons.org/licenses/by/4.0/), which permits unrestricted use, distribution, and reproduction in any medium, provided you give appropriate credit to the original author(s) and the source, provide a link to the Creative Commons license, and indicate if changes were made.
Reprints and permissions
About this article
Kurdi, G., Leo, J., Parsia, B. et al. A Systematic Review of Automatic Question Generation for Educational Purposes. Int J Artif Intell Educ 30 , 121–204 (2020). https://doi.org/10.1007/s40593-019-00186-y
Download citation
Published : 21 November 2019
Issue Date : March 2020
DOI : https://doi.org/10.1007/s40593-019-00186-y
Share this article
Anyone you share the following link with will be able to read this content:
Sorry, a shareable link is not currently available for this article.
Provided by the Springer Nature SharedIt content-sharing initiative
- Automatic question generation
- Semantic Web
- Natural language processing
- Natural language generation
- Difficulty prediction
- Find a journal
- Publish with us
- Track your research
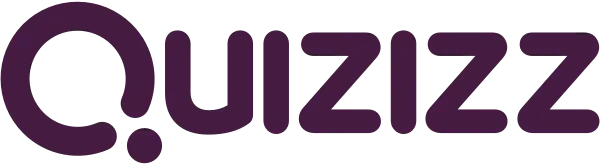
Have an account?
Suggestions for you See more
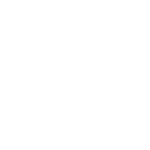
Persuasive Writing
3rd - 4th , nature of academic texts, past tense and past perfect tense, 33.8k plays, parts of a paragraph, 3rd - 5th .

Parts of a Research Paper
10 questions
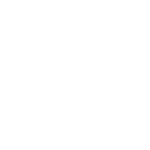
Introducing new Paper mode
No student devices needed. Know more
It is a list of works on a subject or by an author that were used or consulted to write a research paper.
Review of Related Literature
Bibliography
Definition of Terms
It is a brief summary of the researcher’s main ideas and restates the paper's main thesis, giving the reader the sense that the stated goal of the paper has been accomplished.
Introduction
Statement of the Problem
Conclusions
Recommendations
It deals with the description of the research subject, methods and tools of the study, and analysis that was used to summarize the final results of the research.
Methodology
Background of the Study
It is where the researcher introduces the overview of the topic, the main points of information, and why the subject is important.
It describes past important researches and how they specifically relate to the research thesis. It is a synthesis of the previous literature and the new idea being researched.
These are the added suggestions that the researcher wants people to follow when performing future studies.
It shows the different sections of the research paper and the page numbers on which they begin.
Table of Contents
It provides details to the reader on what and how the study will contribute and who will benefit from it.
Significance of the Study
It is a concise description of the problem or issues a research seeks to address.
It explains the extent to which the research area will be explored in the work and specifies the parameters within the study will be operating.
Scope and Limitations
Explore all questions with a free account
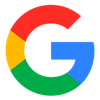
Continue with email
Continue with phone

After being insulted, writing down your feelings on paper then getting rid of it reduces anger
A research group in Japan has discovered that writing down one's reaction to a negative incident on a piece of paper and then shredding it or throwing it away reduces feelings of anger.
"We expected that our method would suppress anger to some extent," lead researcher Nobuyuki Kawai said. "However, we were amazed that anger was eliminated almost entirely."
This research is important because controlling anger at home and in the workplace can reduce negative consequences in our jobs and personal lives. Unfortunately, many anger management techniques proposed by specialists lack empirical research support. They can also be difficult to recall when angry.
The results of this study, published in Scientific Reports , are the culmination of years of previous research on the association between the written word and anger reduction. It builds on work showing how interactions with physical objects can control a person's mood.
For their project, Kawai and his graduate student Yuta Kanaya, both at the Graduate School of Informatics, Nagoya University, asked participants to write brief opinions about important social problems, such as whether smoking in public should be outlawed. They then told them that a doctoral student at Nagoya University would evaluate their writing.
However, the doctoral students doing the evaluation were plants. Regardless of what the participants wrote, the evaluators scored them low on intelligence, interest, friendliness, logic, and rationality. To really drive home the point, the doctoral students also wrote the same insulting comment: "I cannot believe an educated person would think like this. I hope this person learns something while at the university."
After handing out these negative comments, the researchers asked the participants to write their thoughts on the feedback, focusing on what triggered their emotions. Finally, one group of participants was told to either dispose of the paper they wrote in a trash can or keep it in a file on their desk. A second group was told to destroy the document in a shredder or put it in a plastic box.
The students were then asked to rate their anger after the insult and after either disposing of or keeping the paper. As expected, all participants reported a higher level of anger after receiving insulting comments. However, the anger levels of the individuals who discarded their paper in the trash can or shredded it returned to their initial state after disposing of the paper. Meanwhile, the participants who held on to a hard copy of the insult experienced only a small decrease in their overall anger.
Kawai imagines using his research to help businesspeople who find themselves in stressful situations. "This technique could be applied in the moment by writing down the source of anger as if taking a memo and then throwing it away when one feels angry in a business situation," he explained.
Along with its practical benefits, this discovery may shed light on the origins of the Japanese cultural tradition known as hakidashisara ( hakidashi refers to the purging or spitting out of something, and sara refers to a dish or plate) at the Hiyoshi shrine in Kiyosu, Aichi Prefecture, just outside of Nagoya. Hakidashisara is an annual festival where people smash small discs representing things that make them angry. Their findings may explain the feeling of relief that participants report after leaving the festival.
- Anger Management
- Social Psychology
- Disorders and Syndromes
- Educational Psychology
- Consumer Behavior
- Anger management
- Social psychology
- Cognitive dissonance
- Self-awareness
- Obsessive-compulsive disorder
- Collaboration
Story Source:
Materials provided by Nagoya University . Note: Content may be edited for style and length.
Journal Reference :
- Yuta Kanaya, Nobuyuki Kawai. Anger is eliminated with the disposal of a paper written because of provocation . Scientific Reports , 2024; 14 (1) DOI: 10.1038/s41598-024-57916-z
Cite This Page :
Explore More
- Pacific Cities Much Older Than Previously ...
- The Milky Way in Ancient Egyptian Mythology
- Physical Activity Best in the Evening
- How the Body Switches out of 'Fight' Mode
- New Drug Prevents Flu-Related Lung Damage
- 3D Mouth of an Ancient Jawless Fish
- Connecting Lab-Grown Brain Cells
- Device: Self-Healing Materials, Drug Delivery
- How We Perceive Bitter Taste
- Next-Generation Digital Displays
Trending Topics
Strange & offbeat.
Prestigious cancer research institute has retracted 7 studies amid controversy over errors
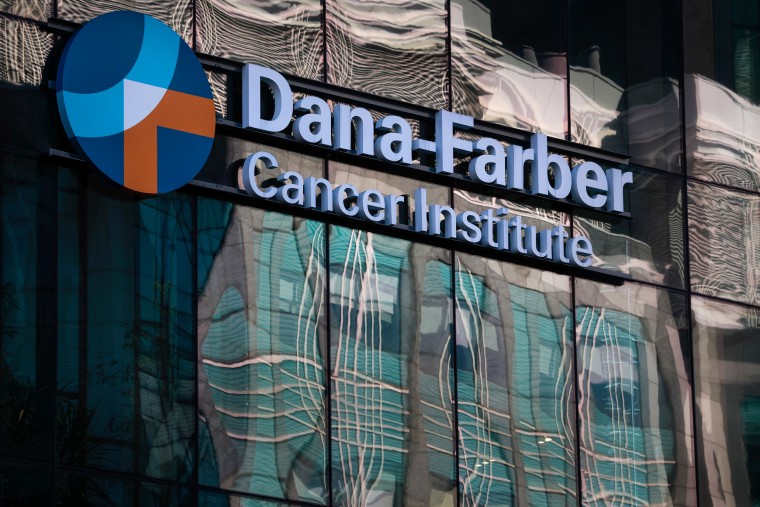
Seven studies from researchers at the prestigious Dana-Farber Cancer Institute have been retracted over the last two months after a scientist blogger alleged that images used in them had been manipulated or duplicated.
The retractions are the latest development in a monthslong controversy around research at the Boston-based institute, which is a teaching affiliate of Harvard Medical School.
The issue came to light after Sholto David, a microbiologist and volunteer science sleuth based in Wales, published a scathing post on his blog in January, alleging errors and manipulations of images across dozens of papers produced primarily by Dana-Farber researchers . The institute acknowledged errors and subsequently announced that it had requested six studies to be retracted and asked for corrections in 31 more papers. Dana-Farber also said, however, that a review process for errors had been underway before David’s post.
Now, at least one more study has been retracted than Dana-Farber initially indicated, and David said he has discovered an additional 30 studies from authors affiliated with the institute that he believes contain errors or image manipulations and therefore deserve scrutiny.
The episode has imperiled the reputation of a major cancer research institute and raised questions about one high-profile researcher there, Kenneth Anderson, who is a senior author on six of the seven retracted studies.
Anderson is a professor of medicine at Harvard Medical School and the director of the Jerome Lipper Multiple Myeloma Center at Dana-Farber. He did not respond to multiple emails or voicemails requesting comment.
The retractions and new allegations add to a larger, ongoing debate in science about how to protect scientific integrity and reduce the incentives that could lead to misconduct or unintentional mistakes in research.
The Dana-Farber Cancer Institute has moved relatively swiftly to seek retractions and corrections.
“Dana-Farber is deeply committed to a culture of accountability and integrity, and as an academic research and clinical care organization we also prioritize transparency,” Dr. Barrett Rollins, the institute’s integrity research officer, said in a statement. “However, we are bound by federal regulations that apply to all academic medical centers funded by the National Institutes of Health among other federal agencies. Therefore, we cannot share details of internal review processes and will not comment on personnel issues.”
The retracted studies were originally published in two journals: One in the Journal of Immunology and six in Cancer Research. Six of the seven focused on multiple myeloma, a form of cancer that develops in plasma cells. Retraction notices indicate that Anderson agreed to the retractions of the papers he authored.
Elisabeth Bik, a microbiologist and longtime image sleuth, reviewed several of the papers’ retraction statements and scientific images for NBC News and said the errors were serious.
“The ones I’m looking at all have duplicated elements in the photos, where the photo itself has been manipulated,” she said, adding that these elements were “signs of misconduct.”
Dr. John Chute, who directs the division of hematology and cellular therapy at Cedars-Sinai Medical Center and has contributed to studies about multiple myeloma, said the papers were produced by pioneers in the field, including Anderson.
“These are people I admire and respect,” he said. “Those were all high-impact papers, meaning they’re highly read and highly cited. By definition, they have had a broad impact on the field.”
Chute said he did not know the authors personally but had followed their work for a long time.
“Those investigators are some of the leading people in the field of myeloma research and they have paved the way in terms of understanding our biology of the disease,” he said. “The papers they publish lead to all kinds of additional work in that direction. People follow those leads and industry pays attention to that stuff and drug development follows.”
The retractions offer additional evidence for what some science sleuths have been saying for years: The more you look for errors or image manipulation, the more you might find, even at the top levels of science.
Scientific images in papers are typically used to present evidence of an experiment’s results. Commonly, they show cells or mice; other types of images show key findings like western blots — a laboratory method that identifies proteins — or bands of separated DNA molecules in gels.
Science sleuths sometimes examine these images for irregular patterns that could indicate errors, duplications or manipulations. Some artificial intelligence companies are training computers to spot these kinds of problems, as well.
Duplicated images could be a sign of sloppy lab work or data practices. Manipulated images — in which a researcher has modified an image heavily with photo editing tools — could indicate that images have been exaggerated, enhanced or altered in an unethical way that could change how other scientists interpret a study’s findings or scientific meaning.
Top scientists at big research institutions often run sprawling laboratories with lots of junior scientists. Critics of science research and publishing systems allege that a lack of opportunities for young scientists, limited oversight and pressure to publish splashy papers that can advance careers could incentivize misconduct.
These critics, along with many science sleuths, allege that errors or sloppiness are too common , that research organizations and authors often ignore concerns when they’re identified, and that the path from complaint to correction is sluggish.
“When you look at the amount of retractions and poor peer review in research today, the question is, what has happened to the quality standards we used to think existed in research?” said Nick Steneck, an emeritus professor at the University of Michigan and an expert on science integrity.
David told NBC News that he had shared some, but not all, of his concerns about additional image issues with Dana-Farber. He added that he had not identified any problems in four of the seven studies that have been retracted.
“It’s good they’ve picked up stuff that wasn’t in the list,” he said.
NBC News requested an updated tally of retractions and corrections, but Ellen Berlin, a spokeswoman for Dana-Farber, declined to provide a new list. She said that the numbers could shift and that the institute did not have control over the form, format or timing of corrections.
“Any tally we give you today might be different tomorrow and will likely be different a week from now or a month from now,” Berlin said. “The point of sharing numbers with the public weeks ago was to make clear to the public that Dana-Farber had taken swift and decisive action with regard to the articles for which a Dana-Farber faculty member was primary author.”
She added that Dana-Farber was encouraging journals to correct the scientific record as promptly as possible.
Bik said it was unusual to see a highly regarded U.S. institution have multiple papers retracted.
“I don’t think I’ve seen many of those,” she said. “In this case, there was a lot of public attention to it and it seems like they’re responding very quickly. It’s unusual, but how it should be.”
Evan Bush is a science reporter for NBC News. He can be reached at [email protected].

IMAGES
VIDEO
COMMENTS
Have you ever prepared research papers? If you want to check how well you understand the terms, you can take this research paper quiz. With research paper quiz questions and answers, you can check your knowledge and get to learn something, which you were unable to recall during the routine. Read the questions carefully to get all the questions correct with a perfect score. All the best! And ...
This paper reports on students' engagement and motivation levels along with the learning curve during the online learning using a game-based digital quiz tool within a Human Computer Interaction ...
When choosing a question for your research paper, look for one that is: focused: not too broad nor too narrow. When looking for evidence to make your point, the information should be: relevant, reliable, recent. As possible entry points in a conversation, choose questions that are: focused, challenging, and grounded.
Quiz & Worksheet Goals. These resources will test you on: How editing and revising differ. Step where you create a general plan for writing a paper. Something you don't need to note when citing a ...
113 Great Research Paper Topics. One of the hardest parts of writing a research paper can be just finding a good topic to write about. Fortunately we've done the hard work for you and have compiled a list of 113 interesting research paper topics. They've been organized into ten categories and cover a wide range of subjects so you can easily ...
3. What is a literature review? 4. Where is a literature review typically found in written-up research? 5. What would you expect to find in a methodology chapter in a piece of empirical research? 6. Should data and discussion of data be presented as two separate chapters? Never.
Multiple Choice Quizzes. Try these quizzes to test your understanding. 1. Research analysis is the last critical step in the research process. True. False. 2. The final research report where a discussion of findings and limitations is presented is the easiest part for a researcher. True.
Choose a research paper topic. There are many ways to generate an idea for a research paper, from brainstorming with pen and paper to talking it through with a fellow student or professor.. You can try free writing, which involves taking a broad topic and writing continuously for two or three minutes to identify absolutely anything relevant that could be interesting.
Scientific Writing: Peer Review and Scientific Journals. a process for evaluating the safety of boat docks. a process by which independent scientists evaluate the technical merit of scientific research papers. a process by which a scientist's friends can give him or her advice. a method of typesetting in publishing.
1. What information does not need to be on a typical cover page? Word count. Author's name. Title. Date. 2. If you were writing a 7-10 page high school or college research paper, which of the ...
A good research question is essential to guide your research paper, dissertation, or thesis. All research questions should be: Focused on a single problem or issue. Researchable using primary and/or secondary sources. Feasible to answer within the timeframe and practical constraints. Specific enough to answer thoroughly.
The first question asks for a ready-made solution, and is not focused or researchable. The second question is a clearer comparative question, but note that it may not be practically feasible. For a smaller research project or thesis, it could be narrowed down further to focus on the effectiveness of drunk driving laws in just one or two countries.
This research paper is based on the software that was prototyped in order to increase students' interactive participation in learning. The software also intended to motivate students to be engaged in specific subject content. The students were inspired to use the activity by encompassing the gaming mode in teaching and learning. Further, excitement was created by mobile enabled game mode. The ...
This paper presents a survey of automatic question generation and assessment strategies from textual and pictorial learning resources. The purpose of this survey is to summarize the state-of-the-art techniques for generating questions and evaluating their answers automatically. ... Research & Evaluation, 1(2), 3. Google Scholar
This engaging and interactive quiz is designed to guide you through a series of thought-provoking questions, unlocking a world of exciting research possibilities along the way. From the depths of history to cutting-edge technologies, this quiz will tap into your curiosity and provide tailored suggestions to inspire your academic journey.
Research Paper Formatting Quiz. Research Paper Formatting Quiz. This quiz is designed to test your previous knowledge on a common topic that writers need to know about; writing research papers and proper research paper formatting. You will have 15 minutes to complete the quiz.
developed an online quiz system fo r self p ractice to check. your prepration before sit in real online exam.In our developed. online system it help users t o familiar with online education ...
automatically assign follow-up activities based on students' scores. assign as homework. share a link with colleagues. print as a bubble sheet. Quiz your students on Parts of research paper practice problems using our fun classroom quiz game Quizalize and personalize your teaching.
While exam-style questions are a fundamental educational tool serving a variety of purposes, manual construction of questions is a complex process that requires training, experience, and resources. This, in turn, hinders and slows down the use of educational activities (e.g. providing practice questions) and new advances (e.g. adaptive testing) that require a large pool of questions. To reduce ...
1 pt. Why is the thesis statement important to a research paper? It isn't. It will save you writing time and guide your research making your paper better. It is the first thing people will read. It explains everything. 3. Multiple Choice. 3 minutes.
The basic objective of this project is to develop an android-based system with following features, namely: (i) Questions bank, (ii) Time frame, (iii) Life lines, (iv) Data Storage, and (v ...
647. 1. Multiple Choice. It is a list of works on a subject or by an author that were used or consulted to write a research paper. 2. Multiple Choice. It is a brief summary of the researcher's main ideas and restates the paper's main thesis, giving the reader the sense that the stated goal of the paper has been accomplished. 3.
The main objective of the project MCQ Quiz Application is to manage th e details of. students, examinations, marks, courses and papers. The project is totally at administrative end. and thus only ...
A research group in Japan has discovered that writing down one's reaction to a negative incident on a piece of paper and then shredding it or throwing it away reduces feelings of anger.
April 9, 2024, 2:32 PM PDT. By Evan Bush. Seven studies from researchers at the prestigious Dana-Farber Cancer Institute have been retracted over the last two months after a scientist blogger ...
Take any one of the samples from the same area, and start your paper. Automatically your research writing skills will be developed. Cite. Yoganandan G. Nidhal Kamel Taha El-Omari. Sundus F ...