Thank you for visiting nature.com. You are using a browser version with limited support for CSS. To obtain the best experience, we recommend you use a more up to date browser (or turn off compatibility mode in Internet Explorer). In the meantime, to ensure continued support, we are displaying the site without styles and JavaScript.
- View all journals

Medical imaging articles from across Nature Portfolio
Medical imaging comprises different imaging modalities and processes to image human body for diagnostic and treatment purposes. It is also used to follow the course of a disease already diagnosed and/or treated.
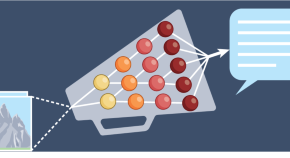
Adapting vision–language AI models to cardiology tasks
Vision–language models can be trained to read cardiac ultrasound images with implications for improving clinical workflows, but additional development and validation will be required before such models can replace humans.
- Rima Arnaout
Related Subjects
- Bone imaging
- Brain imaging
- Magnetic resonance imaging
- Molecular imaging
- Radiography
- Radionuclide imaging
- Three-dimensional imaging
- Ultrasonography
- Whole body imaging
Latest Research and Reviews
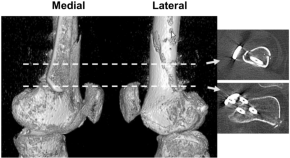
Morphological analysis of the distal femur as a surgical reference in biplane distal femoral osteotomy
- Shohei Sano
- Takehiko Matsushita
- Ryosuke Kuroda
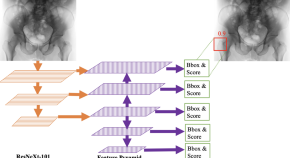
Proximal femur fracture detection on plain radiography via feature pyramid networks
- İlkay Yıldız Potter
- Diana Yeritsyan
- Ashkan Vaziri
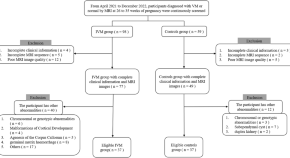
Volume development changes in the occipital lobe gyrus assessed by MRI in fetuses with isolated ventriculomegaly correlate with neurological development in infancy and early childhood
- Zhaoji Chen
- Hongsheng Liu
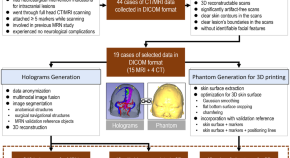
Head model dataset for mixed reality navigation in neurosurgical interventions for intracranial lesions
- Miriam H. A. Bopp
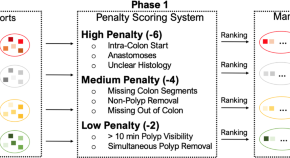
REAL-Colon: A dataset for developing real-world AI applications in colonoscopy
- Carlo Biffi
- Giulio Antonelli
- Andrea Cherubini
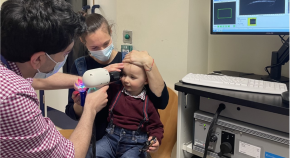
Improved feasibility of handheld optical coherence tomography in children with craniosynostosis
- Sohaib R. Rufai
- Vasiliki Panteli
- Noor ul Owase Jeelani
News and Comment
Refractive shifts in astronauts during spaceflight: mechanisms, countermeasures, and future directions for in-flight measurements.
- Kelsey Vineyard
- Andrew G. Lee
Artificial intelligence chatbot interpretation of ophthalmic multimodal imaging cases
- Andrew Mihalache
- Ryan S. Huang
- Rajeev H. Muni
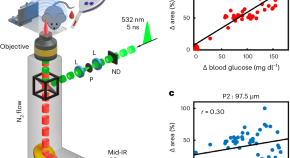
Blood glucose concentration measurement without finger pricking
A new sensor that detects optoacoustic signals generated by mid-infrared light enables measurement of glucose concentration from intracutaneous tissue rich in blood. This technology does not rely on glucose measurements in interstitial fluid or blood sampling and might yield the next generation of non-invasive glucose-sensing devices for improved diabetes management.
Enhancing diagnostic precision in liver lesion analysis using a deep learning-based system: opportunities and challenges
A recent study reported the development and validation of the Liver Artificial Intelligence Diagnosis System (LiAIDS), a fully automated system that integrates deep learning for the diagnosis of liver lesions on the basis of contrast-enhanced CT scans and clinical information. This tool improved diagnostic precision, surpassed the accuracy of junior radiologists (and equalled that of senior radiologists) and streamlined patient triage. These advances underscore the potential of artificial intelligence to enhance hepatology care, although challenges to widespread clinical implementation remain.
- Jeong Min Lee
- Jae Seok Bae
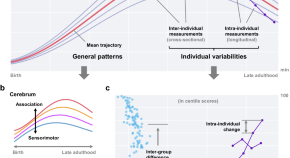
Population imaging cerebellar growth for personalized neuroscience
Growth chart studies of the human cerebellum, which is increasingly recognized as pivotal for cognitive development, are rare. Gaiser and colleagues utilized population-level neuroimaging to unveil cerebellar growth charts from childhood to adolescence, offering insights into brain development.
- Zi-Xuan Zhou
- Xi-Nian Zuo
Quick links
- Explore articles by subject
- Guide to authors
- Editorial policies

BMC Medical Imaging
Latest collections open to submissions.
Liver shape analysis using statistical parametric maps at population scale
Automated classification of liver fibrosis stages using ultrasound imaging
- Most accessed
Tumour growth rate predicts overall survival in patients with recurrent WHO grade 4 glioma
Authors: Jeffer Hann Wei Pang, Seyed Ehsan Saffari, Guan Rong Lee, Wai-Yung Yu, Choie Cheio Tchoyoson Lim, Kheng Choon Lim, Chia Ching Lee, Wee Yao Koh, Wei Tsau, David Chia, Kevin Lee Min Chua, Chee Kian Tham, Yin Yee Sharon Low, Wai Hoe Ng, Chyi Yeu David Low and Xuling Lin
A study on the application of radiomics based on cardiac MR non-enhanced cine sequence in the early diagnosis of hypertensive heart disease
Authors: Ze-Peng Ma, Shi-Wei Wang, Lin-Yan Xue, Xiao-Dan Zhang, Wei Zheng, Yong-Xia Zhao, Shuang-Rui Yuan, Gao-Yang Li, Ya-Nan Yu, Jia-Ning Wang and Tian-Le Zhang
Fog-based deep learning framework for real-time pandemic screening in smart cities from multi-site tomographies
Authors: Ibrahim Alrashdi
Real-time sports injury monitoring system based on the deep learning algorithm
Authors: Luyao Ren, Yanyan Wang and Kaiyong Li
VER-Net: a hybrid transfer learning model for lung cancer detection using CT scan images
Authors: Anindita Saha, Shahid Mohammad Ganie, Pijush Kanti Dutta Pramanik, Rakesh Kumar Yadav, Saurav Mallik and Zhongming Zhao
Most recent articles RSS
View all articles
Age and gender specific normal values of left ventricular mass, volume and function for gradient echo magnetic resonance imaging: a cross sectional study
Authors: Peter A Cain, Ragnhild Ahl, Erik Hedstrom, Martin Ugander, Ase Allansdotter-Johnsson, Peter Friberg and Hakan Arheden
Is fasting a necessary preparation for abdominal ultrasound?
Authors: Tariq Sinan, Hans Leven and Mehraj Sheikh
MRCP compared to diagnostic ERCP for diagnosis when biliary obstruction is suspected: a systematic review
Authors: Eva C Kaltenthaler, Stephen J Walters, Jim Chilcott, Anthony Blakeborough, Yolanda Bravo Vergel and Steven Thomas
Echogenic foci in thyroid nodules: diagnostic performance with combination of TIRADS and echogenic foci
Authors: Su Min Ha, Yun Jae Chung, Hye Shin Ahn, Jung Hwan Baek and Sung Bin Park
Metrics for evaluating 3D medical image segmentation: analysis, selection, and tool
Authors: Abdel Aziz Taha and Allan Hanbury
Most accessed articles RSS
Aims and scope
Become an editorial board member.
Dmitriy Gutarev from Pixabay
To learn more about the role and how to apply, please click the button below.
BMC Series Blog
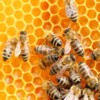
World Bee Day 2024: A hive of research from the BMC Series
20 May 2024
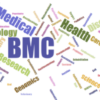
Highlights of the BMC series – April 2024
15 May 2024
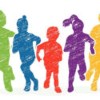
Cleft Lip and Palate Awareness Week: Highlights from the BMC Series
14 May 2024
Latest Tweets
Your browser needs to have JavaScript enabled to view this timeline
Important information
Editorial board
For authors
For editorial board members
For reviewers
- Manuscript editing services
Annual Journal Metrics
2022 Citation Impact 2.7 - 2-year Impact Factor 2.7 - 5-year Impact Factor 0.983 - SNIP (Source Normalized Impact per Paper) 0.535 - SJR (SCImago Journal Rank)
2023 Speed 34 days submission to first editorial decision for all manuscripts (Median) 177 days submission to accept (Median)
2023 Usage 951,496 downloads 161 Altmetric mentions
- More about our metrics
Peer-review Terminology
The following summary describes the peer review process for this journal:
Identity transparency: Single anonymized
Reviewer interacts with: Editor
Review information published: Review reports. Reviewer Identities reviewer opt in. Author/reviewer communication
More information is available here
- Follow us on Twitter
ISSN: 1471-2342
- General enquiries: [email protected]
Medical image data augmentation: techniques, comparisons and interpretations
- Published: 20 March 2023
- Volume 56 , pages 12561–12605, ( 2023 )
Cite this article
- Evgin Goceri 1
45 Citations
Explore all metrics
Designing deep learning based methods with medical images has always been an attractive area of research to assist clinicians in rapid examination and accurate diagnosis. Those methods need a large number of datasets including all variations in their training stages. On the other hand, medical images are always scarce due to several reasons, such as not enough patients for some diseases, patients do not want to allow their images to be used, lack of medical equipment or equipment, inability to obtain images that meet the desired criteria. This issue leads to bias in datasets, overfitting, and inaccurate results. Data augmentation is a common solution to overcome this issue and various augmentation techniques have been applied to different types of images in the literature. However, it is not clear which data augmentation technique provides more efficient results for which image type since different diseases are handled, different network architectures are used, and these architectures are trained and tested with different numbers of data sets in the literature. Therefore, in this work, the augmentation techniques used to improve performances of deep learning based diagnosis of the diseases in different organs (brain, lung, breast, and eye) from different imaging modalities (MR, CT, mammography, and fundoscopy) have been examined. Also, the most commonly used augmentation methods have been implemented, and their effectiveness in classifications with a deep network has been discussed based on quantitative performance evaluations. Experiments indicated that augmentation techniques should be chosen carefully according to image types.
Similar content being viewed by others
Neural Augmentation Using Meta-Learning for Training of Medical Images in Deep Neural Networks
Data Augmentation in Training Deep Learning Models for Medical Image Analysis
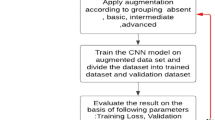
A comparative analysis of different augmentations for brain images
Avoid common mistakes on your manuscript.
1 Introduction
Medical image interpretations are mostly performed by medical professionals like clinicians and radiologists. However, the variations among different experts and complexities of medical images make it very difficult for the experts to diagnose diseases accurately all the time. Thanks to computerized techniques, the tedious image analysis task can be performed by semi-/fully-automatically, and they help the experts to make objective, rapid and accurate diagnoses. Therefore, designing deep learning based methods with medical images has always been an attractive area of research (Tsuneki 2022 ; van der Velden et al. 2022 ; Chen et al. 2022a ). Particularly, deep Convolutional Neural Network (CNN) architectures have been used for several processes (e.g., image segmentation, classification, registration, content-based image retrieval, identification of disease severity, and lesion/tumor/tissue detection, etc.) in some medical diagnosis fields like eye, breast, brain, and lung (Yu et al. 2021 ). Although CNNs can produce results with higher performance compared to the results of traditional machine learning based methods, improving their generalization abilities and developing robust models are still significant challenging issues. Because training data sets should be constructed with a large number of images including all variations (i.e., heterogeneous images) to provide the high generalization ability and robustness of those architectures. However, the number of medical images is usually limited. The data scarcity problem can be due to not enough patients for some diseases, patients do not want to allow their images to be used, lack of medical equipment or equipment, inability to obtain images that meet the desired criteria, inability to access images of patients living in different geographical regions or of different races.
Another important issue is that, even if enough images are acquired, labeling them (unlike natural image labeling which is relatively easy) is not easy, requires certain domain knowledge of medical professionals, and is time-consuming. Also, legal issues and privacy are other concerns in the labeling of medical images. Therefore, imbalanced data causing overfitting, biased and inaccurate results is a significant challenge that should be handled while developing deep network based methods.
To overcome these challenges by using balanced number of images and expanding training datasets automatically, image augmentation methods are used. Thanks to the augmentation methods, deep network architectures’ learning and generalization ability can be improved, and the desired robustness property of the networks can be provided (Sect. 2 ). However, various augmentation techniques have been applied with different types of images in the literature. It is not clear which data augmentation technique provides more efficient results for which image type since different diseases are handled, different network architectures are used, and these architectures are trained and tested with different numbers of data sets in the literature (Sect. 2 ). According to the segmentation or classification results presented in the literature, it is not possible to understand or compare the impacts or contributions of the used augmentation approaches on the results, and to decide the most appropriate augmentation method.
In the literature, researchers have presented some surveys about augmentation methods. However, they have focused on the augmentation methods applied either;
with a specific type of images like natural images (Khosla and Saini 2020 ), mammography images (Oza et al. 2022 ), Computed Tomography (CT), and Magnetic Resonance (MR) images (Chlap et al. 2021 ) acquired with different imaging techniques and have different properties.
to improve the performance of specific operations like polyp segmentation (Dorizza 2021 ) and text classification (Bayer et al. 2021 ).
with a specific technique, such as Generative Adversarial Network (GAN) (Chen et al. 2022b ), erasing and mixing (Naveed 2021 ).
In this work, a more comprehensive range of image types has been handled. Also, a more in-depth analysis has been performed through the implementation of common augmentation methods with the same data sets for objective comparisons, and evaluations based on quantitative results. According to our knowledge, there is no (i) comprehensive review that covers a greater range of medical imaging modalities and applications than articles in the literature, and (ii) any work on quantitative evaluations of the augmentation methods applied with different medical images to determine the most appropriate augmentation method.
To fill this gap in the literature, in this paper, the augmentation techniques that have been applied to improve performances of deep learning-based diagnosis of diseases in different organs (brain, lung, breast, and eye) using different imaging modalities (MR, CT, mammography, and fundoscopy) have been examined. The chosen imaging modalities are widely used in medicine for many applications such as classification of brain tumors, lung nodules, and breast lesions (Meijering 2020 ). Also, in this study, the most commonly used augmentation methods have been implemented using the same datasets. Additionally, to evaluate the effectiveness of those augmentation methods in classifications from the four types of images, a classifier model has been designed and classifications with the augmented images for each augmentation method have been performed with the same classifier model. The effectiveness of the augmentation methods in the classifications has been discussed based on quantitative results. This paper presents a novel comprehensive insight into the augmentation methods proposed for diverse medical images by considering popular medical research problems altogether.
The key features of this paper are as follows:
The techniques used for augmentation of brain MR images, lung CT images, breast mammography images, and eye fundus images have been reviewed.
The papers published between 2020 and 2022 and mainly collected by Springer, IEEE Xplore, and ELSEVIER, have been reviewed, and a systematic and comprehensive review and analyses of the augmentation methods have been carried out.
Commonly used augmentations in the literature have been implemented with four different medical image modalities.
For comparisons of the effectiveness of the augmentation methods in deep learning-based classifications, the same network architecture and expanded data sets with the augmented images from each augmentation methods have been used.
Five evaluation metrics have been used, and the results provided from each classification have been evaluated with the same evaluation metrics.
The best augmentation method for different imaging modalities showing different organs has been determined according to quantitative values.
We believe that the results and conclusions presented in this work will be helpful for researchers to choose the most appropriate augmentation technique in developing deep learning-based approaches to diagnose diseases with a limited number of image data. Also, this work can be helpful for researchers interested in developing new augmentation methods for different types of images. This manuscript has been structured as follows: A detailed review of the augmentation approaches applied with the medical images handled in this work between 2020 and 2022 is presented in the second section. The data sets and methods implemented in this work are explained in the third section. Evaluation metrics and quantitative results are given in the fourth section. Discussions are given in the fifth section, and conclusions are presented in the sixth section.
2 Related works
In this section, the methods used in the literature for augmentation of brain MR images, lung CT images, breast mammography images, and eye fundus images are presented.
2.1 Augmentation of brain MR images
In the literature, augmentation of brain MR images have been performed with various methods such as rotation, noise addition, shearing, and translation to increase number of images and improve performances of several tasks (Table 1 ). For example, augmentation has been provided by noise addition and sharpening to increase the accuracy of tumor segmentation and classification in a study (Khan et al. 2021 ). In another study, augmented images have been obtained by translation, rotation, random cropping, blurring, and noise addition to obtain high performance from the method applied for age prediction, schizophrenia diagnosis, and sex classification (Dufumier et al. 2021 ). In different studies, random scaling, rotation, and elastic deformation have been applied to increase tumor segmentation accuracy (Isensee et al. 2020 ; Fidon et al. 2020 ). Although mostly those techniques have been preferred in the literature, augmentation has also been provided by producing synthetic images. To generate synthetic images, Mixup method, which combines two randomly selected images and their labels, or modified Mixup, or GAN structures have been used (Table 1 ).
For instance, a modified Mixup is a voxel-based approach called TensorMixup, which combines two image patches using a tensor, and has been applied (Wang et al. 2022 ) to boost the accuracy of tumor segmentation. In a recent study, a GAN based augmentation has been used for cerebrovascular segmentation (Kossen et al. 2021 ).
All methods applied for augmentation of brain MR images in the publications reviewed in this work and the details in those publications are presented in Table 1 . Those augmentation methods have been used in the applications developed for different purposes. In those applications, different network architectures, datasets, and numbers of images have been used, and also their performances have been evaluated with different metrics. High performances have been obtained from those applications according to the reported results. However, the most effective and appropriate augmentation method used in them with the brain MR images is not clear due to those differences. Also, it is not possible to determine augmentation methods’ contributions to those performances according to the presented results in those publications.
2.2 Augmentation of lung CT images
In computerized methods being developed to assist medical professionals in various tasks [e.g., nodule or lung parenchyma segmentation, nodule classification, prediction of malignancy level, disease diagnosis like coronavirus (covid-19), etc.], augmentations of CT lung images are commonly provided by traditional techniques like rotation, flipping, and scaling (Table 2 ). For example, image augmentation has been provided using flipping, translation, rotation, and brightness changing to improve automated diagnosis of covid-19 by Hu et al. ( 2020 ). Similarly, a set of augmentations (Gaussian noise addition, cropping, flipping, blurring, brightness changing, shearing, and rotation) has been applied to increase the performance of covid-19 diagnosis by Alshazly et al. ( 2021 ). Also, several GAN models have been used to augment lung CT images by generating new synthetic images (Table 2 ). While doing a GAN based augmentation of lung CT scans, researchers usually tend to generate the area showing lung nodules in the whole image. Because the lung nodules are small and therefore it is more cost-effective than generating a larger whole image or background area. For instance, guided GAN structures have been implemented to produce synthetic images showing the area with lung nodules by Wang et al. ( 2021 ). Nodules’ size information and guided GANs have been used to produce images with diverse sizes of lung nodules by Nishio et al. ( 2020 ).
All methods applied for augmentation of breast mammography images in the publications reviewed in this study and the details in those publications are presented in Table 2 . Those augmentation methods have been applied in the applications developed for different purposes. In those applications, different network models, data sets and number of images have been used, and also their performances have been evaluated with different metrics. High performances have been obtained from those applications according to the reported results. However, the most effective and appropriate augmentation method used in them with the lung CT images is not clear due to those differences. Also, it is not possible to determine augmentation methods’ contributions to those performances according to the presented results in those publications.
2.3 Augmentation of breast mammography images
Generally, augmentation methods with mammography images have been applied to improve automated recognition, segmentation, and detection of breast lesions. Because failures in the detection or identification of the lesions lead to unnecessary biopsies or inaccurate diagnoses. In some works, in the literature, augmentation methods have been applied on extracted positive and negative patches instead of the whole image (Karthiga et al. 2022 ; Zeiser et al. 2020 ; Miller et al. 2022 ; Kim and Kim 2022 ; Wu et al. 2020 b). Positive patches are extracted based on radiologist marks while negative patches are extracted as random regions from the same image. Also, refinement and segmentation of the lesion regions before the application of an augmentation method is the most preferred way to provide good performance from the detection and classification of the lesions. For instance, image refinement has been provided by noise reduction and resizing steps, and then segmentation and augmentation steps have been applied (Zeiser et al. 2020 ). Similarly, noise and artifacts have been eliminated before the segmentation of the images into non-overlapping parts and augmentation (Karthiga et al. 2022 ). Augmentation of patches or whole mammography images have usually been performed by scaling, noise addition, mirroring, shearing, and rotation techniques or using several combinations of them (Table 3 ). For instance, a set of augmentation methods (namely mirroring, zooming, and resizing) has been applied to improve automated classification of images into three categories (as normal, malignant, and benign) (Zeiser et al. 2020 ). Also, those techniques have been used together with GANs to provide augmentations with new synthetic images. For instance, flipping and deep convolutional GAN has been combined for binary classifications of images (as normal and with a mass) by Alyafi et al. ( 2020 ). Only GAN based augmentations have also been used. For instance, contextual GAN, which generates new images by synthesizing lesions according to the context of surrounding tissue, has been applied to improve binary classification (as normal and malignant) by Wu et al. ( 2020 b). In another work (Shen et al. 2021 ), both contextual and deep convolutional GAN have been used to generate synthetic lesion images with margin and texture information and achieve improvement in the detection and segmentation of lesions.
All methods applied for augmentation of breast mammography images in the publications reviewed in this work and the details in those publications are presented in Table 3 . Those augmentation methods have been applied in the applications developed for different purposes. In those applications, different network architectures, datasets and number of images have been used, and also their performances have been evaluated with different metrics. High performances have been obtained from those applications according to the reported results. However, the most effective and appropriate augmentation method used in them with breast mammography images is not clear due to those differences. Also, it is not possible to determine augmentation methods’ contributions to those performances according to the presented results in those publications.
2.4 Augmentation of eye fundus images
Eye fundus images show various important features, which are symptoms of eye diseases, such as abnormal blood veins (neovascularization), red/reddish spots (hemorrhages, microaneurysms), and bright lesions (soft or hard exudates). Those features are used in deep learning based methods being developed for different purposes like glaucoma identification, classification of diabetic retinopathy (DR), and identification of DR severity. Commonly used augmentation techniques with eye fundus images to boost the performance of deep learning based approaches are rotation, shearing, flipping, and translation (Table 4 ). For example, in a study (Shyamalee and Meedeniya 2022 ), a combined method including rotation, shearing, zooming, flipping, and shifting has been applied for glaucoma identification. Another combined augmentation including shifting and blurring has been used by Tufail et al. ( 2021 ) before binary classification (as healthy and nonhealthy) and multi-class (5 classes) classification of images to differentiate different stages of DR. In a different study (Kurup et al. 2020 ), combination of rotation, translation, mirroring, and zooming has been used to achieve automated detection of malaria retinopathy. Also, pixel intensity values have been used in some augmentation techniques since fundus images are colored images. For instance, to achieve robust segmentation of retinal vessels, augmentation has been provided by a channel-wise random gamma correction (Sun et al. 2021 ). The authors have also applied a channel-wise vessel augmentation using morphological transformations. In another work (Agustin et al. 2020 ), to improve the robustness of DR detection performance, image augmentation has been performed by zooming and a contrast enhancement process known as Contrast Limited Adaptive Histogram Equalization (CLAHE). Augmentations have also been provided by generating synthetic images using GAN models. For instance, conditional GAN (Zhou et al. 2020 ) and deep convolutional GAN (Balasubramanian et al. 2020 ) based augmentations have been used to increase the performance of the methods developed for automatic classifications of DR according to its severity level. In some works, retinal features (such as lesion and vascular features) have been developed and added to new images. For instance, NeoVessel (NV)-like structures have been synthesized in a heuristic image augmentation (Ara´ujo et al. 2020 ) to improve detection of proliferative DR which is an advanced DR stage characterized by neovascularization. In this augmentation, different NV kinds (trees, wheels, brooms) have been generated depending on the expected shape and location of NVs to synthesize new images.
All methods applied for augmentation of eye fundus images in the publications reviewed in this study and the details in those publications are presented in Table 4 . Those augmentation methods have been applied in the applications developed for different purposes. In those applications, different network structures, data sets and number of images have been used, and also their performances have been evaluated with different metrics. High performances have been obtained from those applications according to the reported results. However, the most effective and appropriate augmentation method used in them with eye fundus images is not clear due to those differences. Also, it is not possible to determine augmentation methods’ contributions to those performances according to the presented results in those publications.
2.5 Commonly applied augmentation methods
2.5.1 rotation.
Rotation-based image augmentations are provided by rotating an image by concerning its original position. The rotation uses a new coordinate system and retains the same relative positions of the pixels of an image. It can be rightward or leftward across an axis within the range of [1°, 359°]. Image labels may not always be preserved if the degree of rotation increases. Therefore, the safety of this augmentation technique depends on the rotation degree. Although rotation transformation is not safe on images showing 6 and 9 in digit recognition applications, it is generally safe on medical images.
2.5.2 Flipping
Flipping is a technique generating a mirror image from an image. The pixel’s positions are inverted by concerning one of the two axes (for a two-dimensional image). Although it can be applied with vertical or/and horizontal axes, vertical flipping is rarely preferred since the bottom and top regions of an image may not always be interchangeable (Nalepa et al. 2019 ).
2.5.3 Translation
Images are translated along an axis using a translation vector. This technique preserves the relative positions between pixels. Therefore, translated images provide prevention of positional bias (Shorten and Khoshgoftaar 2019 ) and the models do not focus on properties in a single spatial location (Nalepa et al. 2019 ).
2.5.4 Scaling
Images are scaled along different axes with a scaling factor, which can be different or the same for each axis. Especially, scaling changings can be interpreted as zoom out (when the scaling factor is less than 1) or zoom in (when the scaling factor is greater than 1).

2.5.5 Shearing
This transformation slides one edge of an image along the vertical or horizontal axis, creating a parallelogram. A vertical direction shear slides an edge along the vertical axis, while a horizontal direction shear slides an edge along the horizontal axis. The amount of the shear is controlled by a shear angle.
2.5.6 Augmentation using intensities
This augmentation is provided by modification of contrast or brightness, blurring, intensity normalization, histogram equalization, sharpening, and addition of noise. If the preferred type of noise is Gaussian, intensity values are modified by sampling a Gaussian distribution randomly. If it is salt-and-pepper type of noise, pixel values are set randomly to white and black. Uniform noise addition is performed by modification of pixel values using randomly sampling a uniform distribution.
2.5.7 Random cropping and random erasing
Augmentation by random cropping is applied by taking a small region from an original image and resizing it to match the dimensions of the original image. Therefore, this augmentation can also be called scaling or zooming. Augmentation by random erasing is performed by randomly eliminating image regions.
2.5.8 Color modification
Image augmentation by color modification is performed in several ways. For example, an RGB-colored image is stored as arrays, which correspond to 3 color channels representing levels of red, green, and blue intensity values. Because of this, the color of the image can be changed without losing its spatial properties. This operation is called color space shifting, and it is possible to perform the operation in any number of spaces because the original color channels are combined in different ratios in every space. Therefore, a way to augment an RGB-colored image by color modification is to put the pixel values in the color channels of the image into a histogram and then manipulate them using filters to generate new images by changing the color space characteristics. Another way is to take 3 shifts (integer numbers) from 3 RGB filters and add each shift into a channel in the original image. Also, image augmentation can be provided by updating values of hue, saturation, and value components, isolating a channel (such as a blue, red, or green color channel), and converting color spaces into one another to augment images. However, converting a color image to its grayscale version should be performed carefully because this conversion reduces performance by up 3% (Chatfield et al. 2014 ).
2.5.9 GAN based augmentation
GANs are generative architectures constructed with a discriminator to separate synthetic and true images and a generator to generate synthetic realistic images. The main challenging issues of GAN based image augmentations are generating images with high quality (i.e., high-resolution, clear) and maintaining training stability. To overcome those issues, several GAN variants were developed such as conditional, deep convolutional, and cycle GAN. Conditional GAN adds parameters (e.g., class labels) to the input of the generator to control generated images and allows conditional image generation from the generator. Deep convolutional GAN uses deep convolution networks in the GAN structure. Cycle GAN is a conditional GAN and translates images from one domain to another (i.e., image-to-image translation), which modifies input images into novel synthetic images that meet specific conditions. Wasserstein GAN is the structure that is constructed with the loss function designed with the earth-mover distance to increase the stability of the model. Style GAN and progressively trained GAN add coarse to fine details and learn features from training images to synthesize new images.
3 Materials and methods
3.1 materials.
In this work, publicly available brain MR, lung CT, breast mammography, and eye fundus images have been used.
Brain MR images have been provided from Multimodal Brain Tumor Image Segmentation Challenge (BRaTS) (Bakas et al. 2018 ). The data for each patient includes four diverse models of MR images which are Fluid-Attenuated Inversion Recovery (FLAIR) images, T2-weighted MR images, T1-weighted MR images with Contrast Enhancement (T1-CE), and T1-weighted MR images. Different modal images provide different information about tumors. In this work, FLAIR images have been used. Example FLAIR images showing High-Grade Glioma (HGG) (Fig. 1 a, b) and Low-Grade Glioma (Fig. 1 c, d) (LGG) are presented in Fig. 1 . The BRaTS database contains 285 images including HGG (210 images) and LGG (75 images) cases.
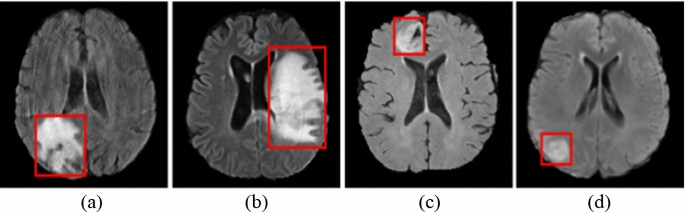
Brain MR scans showing HGG ( a , b ) and LGG ( c , d ) (Bakas et al. 2018 )
Lung images have been provided from Lung Image Database Consortium and Image Database Resource Initiative (LIDC-IDRI) database (Armato et al. 2011 ; McNitt-Gray et al. 2007 ; Wang et al. 2015 ) by the LUNA16 challenge (Setio et al. 2017 ). In the database, there are 1018 CT scans which belong to 1010 people. Those scans were annotated by four experienced radiologists. The nodules that were marked by those radiologists were divided into three groups which are “non-nodule ≥ 3 millimeters”, “nodule < 3 millimeters”, and “nodule ≥ 3 millimeters” (Armato et al. 2011 ). The images having a slice thickness greater than 3 mm were removed due to their usefulness according to the suggestions (Manos et al. 2014 ; Naidich et al. 2013 ). Also, the images having missing slices or not consistent slice spacing were discarded. Therefore, there are a total of 888 images extracted from 1018 cases. Example images with nodules (Fig. 2 a) and without nodules (Fig. 2 b) have been presented in Fig. 2 .
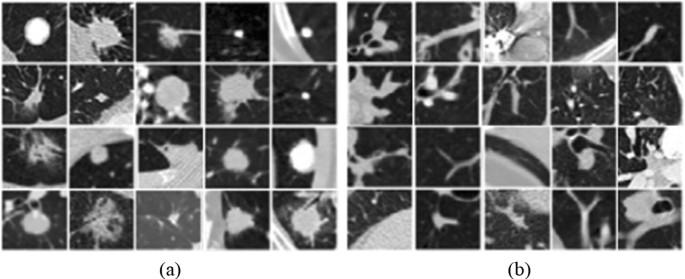
Example images showing nodules ( a ) and non-nodule regions ( b ) (Armato et al. 2011 )
Breast mammography images have been provided from the INbreast database (Moreira et al. 2012 ). It contains 410 images, of which only 107 images show breast masses, and the total number of mass lesions is 115 since an image may contain more than one lesion. The number of benign and malignant masses is 52 and 63, respectively. Example mammography images without any mass (Fig. 3 a), with a benign mass (Fig. 3 b), and malignant mass (Fig. 3 c) lesions have been presented in Fig. 3 .
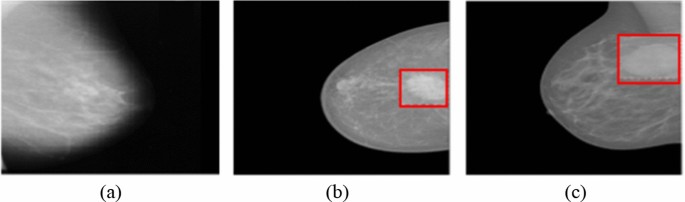
Mammography images without mass ( a ), a benign mass ( b ), and a malignant mass ( c ) (Moreira et al. 2012 )
Eye fundus images have been provided from the Messidor database (Decencière et al. 2014 ) including 1200 images categorized into three classes (0-risk, 1-risk, and 2-risk) according to the diabetic macular edema risk level. The number of images in the categories is 974, 75, and 151, respectively. Example images showing macular edema with 0-risk (Fig. 4 a), 1-risk (Fig. 4 b), and 2-risk (Fig. 4 c) have been presented in Fig. 4 .
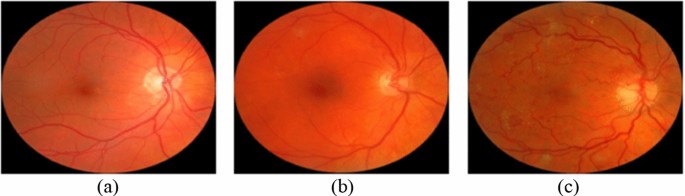
Eye fundus images showing macular edema with 0-risk ( a ), 1-risk ( b ), and 2-risk ( c ) (Decencière et al. 2014 )
3.2 Implemented augmentation methods
In this work, commonly used augmentation methods based on transformations and intensity modifications have been implemented to see and compare their effects on the performance of deep learning based classifications from MR, CT, and mammography images. Also, a method based on a color modification to augment colored eye fundus images has been applied. Those methods are explained below.
1st Method In this method, a shearing angle, which is selected randomly within the range [− 15°, 15°], is used and a shearing operation is applied within the x and y-axis. This operation is repeated 10 times with 10 different shearing angles. By this method, 10 images are generated from an original image.
2nd Method In this method, translation operation is applied on the x and y-axis. This operation is repeated 10 times by using different values sampled randomly within the range [− 15, 15]. By this method, 10 images are generated from an original image.
3rd Method Rotation angles are selected randomly within the range [− 25°, 25°] and clockwise rotations are applied using 10 different angle values. 10 images are generated from an original image by this method.
4th Method In this method, noise addition is applied by I = I + (rand(size( I )) − FV ) × N , where the term FV refers to a fixed value, N refers to noise and I refers to an input image. The noise to be added is generated using a Gaussian distribution. The variance of the distribution is computed from the input images while the mean value is set to zero. In this study, three different fixed values (0.3, 0.4, and 0.5) are used and 3 images are generated from an input image.
5th Method In this method, noise is added to each image with 3 density values (0.01, 0.02, and 0.03) and a salt-and-pepper type noise is used. Therefore, 3 images are generated from an input image by this method.
6th Method In this method, augmentation is provided by salt-and-pepper type noise addition (5th method) followed by shearing (1st method). 30 images are generated from an input image by this method.
7th Method In this method, augmentation is provided by Gaussian noise addition (4th method) followed by rotation (3rd method). 30 images are generated from an input image by this method.
8th Method In this augmentation method, clockwise rotation and then translation operations are applied. Rotation angles are selected randomly within the range [− 25°, 25°] and translation is applied on the x and y-axis by using different values sampled randomly within the range [− 15, 15]. This successive operation is applied 10 times, and 10 images are generated from an input image by this method.
9th Method In this method, translation is applied followed by the shearing. The translation step is applied on the x and y-axis with different values sampled randomly within the range [− 15, 15]. In the shearing step, an angle is selected randomly within the range [− 15°, 15°], and shearing is applied within the x and y-axis. The subsequent operations are applied 10 times and 10 images are generated from an input image by this method.
10th Method In this method, three operations (translation, shearing, and rotation) are applied subsequently. Implementation of the translation and shearing operations are performed as explained in the 9th method. In the rotation step, a random angle is selected within the range [− 25°, 25°] clockwise rotation is applied. The successive operations are applied 10 times, and 10 images are generated from an input image by this method.
11th Method Color shifting, sharpening, and contrast changing have been combined in this method. Color shifting has been applied by taking 3 shifts (integer numbers) from 3 RGB filters and adding each shift into one of the 3 color channels in the original images. Sharpening has been changed by blurring the original images and subtracting the blurred images from the original images. A Gaussian filter, whose variance value is 1, has been used for the blurring process. Contrast changing has been applied by scaling the original images linearly between i 1 and i 2 ( i 1 < i 2 ), which will be the maximum and minimum intensities in the augmented images, and then mapping the original images’ pixels with intensity values higher than i 2 (or lower than i 1 ) to 255 (or 0). Therefore, 3 images are generated from an input image by this method.
3.3 Classification
In this work, to compare the effectiveness of the augmentation approaches in classifications, a CNN-based classifier has been used. In this step, the original images taken from the public databases (Sect. 3.1 ) have been used in construction of training and testing datasets. However, the databases have imbalanced class distributions. Therefore, to obtain unbiased classification results, an equal number of images have been taken from the databases for the classes. Those balanced numbers of images have been used with the augmentation methods and the generated images from them have been added into the data sets. 80% of the total data has been used in the training stage, the rest 20% of the data has been used in the testing stage. It should be reminded here that the goal in this study is to evaluate the augmentation techniques used with different types of images in improving the classification rather than evaluations of classifier models. Therefore, ResNet101 architecture has been chosen and implemented in this work. The reason to choose this architecture is to utilize its advantage in addressing the gradient vanishing issue and high learning ability (He et al. 2016 ). Pre-training of networks by using large datasets [such as ImageNet (Russakovsky et al. 2015 )] and then fine-tuning the networks for target tasks having less training data has been commonly applied in recent years. Pre-training has provided superior results on many tasks, such as action recognition (Simonyan and Zisserman 2014 ; Carreira and Zisserman 2017 ), image segmentation (He et al. 2017 ; Long et al. 2015 ), and object detection (Ren et al. 2015 ; Girshick 2015 ; Girshick et al. 2014 ). Therefore, transfer learning from a model trained using ImageNet has been used in this work for fine-tuning of the network. Optimization has been provided by Adam, and activation has been provided by ReLU. The initial learning rate has been chosen as 0.0003, and cross-entropy loss has been computed to update the weights in the training phase. The number of epochs and mini-batch size have been set as 6 and 10, respectively.
Classification results have been evaluated by using these five measurements: specificity, accuracy, sensitivity, Matthew Correlation Coefficient (MCC), and F1-score computed by:
where FP, FN, TN, and TP refer to false positives, false negatives, true negatives, and true positives, respectively. The number of images obtained from each augmentation method for each augmentation approach is given in Table 5 .
The augmentation methods and quantitative results obtained by classifications of HGG and LGG cases from brain MR images have been presented in Table 6 .
Lung CT scans have been classified as a pulmonary nodule or a non-nodule with the same classifier. 1004 lung nodules of which 450 positive candidates provided by the LIDC-IDRI have been used. After generation more positive candidate nodules with the augmentation methods, equal number of negative and positive candidates have been used in the classifications. The augmentation methods and quantitative results of classifications have been presented in Table 7 .
The breast mammography images have been classified into three groups as malignant, benign, and normal. The augmentation methods and quantitative results of classifications have been presented in Table 8 .
Unlike from MR, CT, and mammography images, the eye fundus images are colored. Therefore, in addition to those 10 augmentation methods applied for grayscale images, the 11th method has also been used for augmentation of this images. The augmentation methods and quantitative results of the classifications into three classes according to macular edema risk level have been presented in Table 9 .
5 Discussions
To overcome the imbalance distribution and data scarcity problems in deep learning based approaches with medical images, data augmentations are commonly applied with various techniques in the literature (Sect. 2 ). In those approaches, different network architectures, different types of images, parameters, and functions have been used. Although they achieved good performances with augmented images (Tables 1 , 2 , 3 and 4 ), the results presented in the publications do not indicate on which aspects of the approaches contributed the most. Although augmentation methods are applied as pre-processing steps and therefore have significant impacts on the remaining steps and overall performance, their contributions are not clear. Because of this, it is not clear which data augmentation technique provides more efficient results for which image type. Therefore, in this study, the effects of augmentation methods have been investigated to determine the most appropriate method for automated diagnosis from different types of medical images. This has been performed by these steps: (i) The augmentation techniques used to improve the performance of deep learning based diagnosis of diseases in different organs (brain, lung, breast, and eye) have been reviewed. (ii) The most commonly used augmentation methods have been implemented with four types of images (MR, CT, mammography, and fundoscopy). (iii) To evaluate the effectiveness of those augmentation methods in classifications, a classifier model has been designed. (iv) Classifications using the augmented images for each augmentation method have been performed with the same classifier and datasets for fair comparisons of those augmentation methods. (v) The effectiveness of the augmentation methods in classifications have been discussed based on the quantitative results.
It has been observed from the experiments in this work that transformation-based augmentation methods are easy to implement with medical images. Also, their effectiveness depends on the characteristics (e.g., color, intensity, etc.) of the images, and whether all significant visual features according to medical conditions exist in the training sets. If most of the images in the training sets have similar features, then there is a risk of constructing a classifier model with overfitting and less generalization capability. In general, the pros and cons of the widely used traditional augmentation approaches are presented in Table 10 .
Generating synthetic images by GAN based methods can increase diversity. However, GANs have their own challenging problems. A significant problem is vanishing gradient and mode collapsing (Yi et al. 2019 ). Since the collapse of the mode restricts the capacity of GAN architecture to be varied, this interconnection is detrimental for augmentations of medical images (Zhang 2021 ). Another significant problem is that it is hard to acquire satisfactory training results if the training procedure does not assure the symmetry and alignment of both generator and discriminator networks. Artifacts can be added while synthesizing images, and therefore the quality of the produced images can be very low. Also, the trained model can be unpredictable since GANs are complex structures and managing the coordination of the generator and discriminator is difficult. Furthermore, they share similar flaws with neural networks (such as, poor interpretability). Additionally, they require strong computer resources, a long processing time, and hamper the quality of the generated images in case of running on computers having low computational power with constrained resources.
Quality and diversity are two critical criteria in the evaluation of synthetically generated images from GANs. Quality criterion indicates the level of similarity between the synthetic and real images. In other words, it shows how representative synthetic images are of that class. A synthetic image’s quality is characterized by a low level of distortions, fuzziness, noise (Thung and Raveendran 2009 ), and its feature distribution matching with the class label (Zhou et al. 2020 ; Costa et al. 2017 ; Yu et al. 2019 ).
Diversity criteria indicates the level of dissimilarity of the synthetic and real images. In other words, it shows how uniform or wide the feature distribution of the synthetic image is (Shmelkov et al. 2018 ). When the training datasets are expanded with the images lacking diversity, the datasets can provide only limited coverage of the target domain and cause low classification performance due to incorrect classifications of the images containing features that belong to the less represented regions. When the training datasets are expanded by adding synthetic images with low quality, the classifier cannot learn the features representing different classes which leads to low classification performance. Therefore, if synthetic images are used in the training sets, they should be sufficiently diverse to represent features of each class and have high quality.
The diversity and quality of the new images produced by GANs are evaluated manually by a physician or quantitatively by using similarity evaluation measurements, which are generally FID, structural similarity index, and peak signal-to-noise ratio (Borji 2019 ; Xu et al. 2018 ). The validity of these measurements for medical image data sets is still under investigation and there is no accepted consensus. Manual evaluations are subjective as well as time-consuming (Xu et al. 2018 ) since they are based on the domain knowledge of the physician. Also, there is no commonly accepted metric for evaluating the diversity and quality of synthesized images.
About Brain MR Image Augmentation techniques The methods used for brain MR image augmentation have some limitations or drawbacks. For instance, in a study (Isensee et al. 2020 ), the augmentation approach uses elastic deformations, which add shape variations. However, the deformations can bring lots of damage and noise when the deformation field is varied seriously. Also, the generated images seem not to be realistic and natural. It has been shown in the literature that widely used elastic deformations produce unrealistic brain MR images (Mok and Chung 2018 ). If the simulated tumors are not in realistic locations, then the classifier model can focus on the lesion’s appearance features and be invariant to contextual information. In another study (Kossen et al. 2021 ), although the generated images yielded a high dice score in the transfer learning approach, performance only slightly improved when training the network with real images and additional augmented images according to the results presented by the authors. This might be because of the less blurry and noisy appearance of the images produced by the used augmentation. The discriminator observed results of the generator network at intermediate levels in the augmentation with multi-scale gradient GAN (Deepak and Ameer 2020 ). Because the proposed GAN structure included a single discriminator and generator with multiple connections between them. Although augmentation with TensorMixup improved the diversity of the dataset (Wang et al. 2022 ), its performance should be further verified with more datasets including medical images with complicated patterns as samples generated with this augmentation may not satisfy the clinical characterizations of the images. Li et al. ( 2020 ) has observed that an unseen tumor label cannot be provided with the augmentation method and therefore the virtual semantic labels’ diversity is limited.
It has been observed that usage of the augmented images obtained by the combination of rotation with shearing, and translation provides higher performance than the other augmentation techniques in the classifications of HGG and LGG cases from FLAIR types of brain images. On the other hand, augmentation with the combination of shearing and salt-and-pepper noise addition is the least efficient approach for augmentation in improving classification performance (Table 6 ).
About Lung CT Image Augmentation techniques Further studies on augmentation techniques for lung CT images are still needed since current methods still suffer from some issues. For instance, in a study (Onishi et al. 2020 ), although performance in the classification of isolated nodules and nodules having pleural tails increased, it did not increase in the classification of nodules connected to blood vessels or pleural. The reason might be due to the heavy usage of isolated nodule images (rather than the images with the nodules adjacent to other wide tissues like the pleural) for the training of the GAN. Also, the Wasserstein GAN structure leads to gradient vanishing problems due to small weight clipping and a long time to connect because of huge clipping. In another study (Nishio et al. 2020 ), the quality of the generated 3-dimensional CT images that show nodules is not low. On the other hand, in some of those images, the lung parenchyma surrounding the nodules does not seem natural. For instance, the CT values around the lung parenchyma in the generated images are relatively higher than the CT values around the lung parenchyma in the real images. Also, lung vessels, chest walls, and bronchi in some of those generated images are not regular. The radiologists easily distinguish those generated images according to the irregular and unnatural structures. The augmentation method in a different work (Nishio et al. 2020 ) generate only lung nodules’ 3-dimensional CT images. However, there exist various radiological findings (e.g., ground glass, consolidation, and cavity) and it is not clear whether those findings are generated or not. Also, the application can classify nodules only according to their sizes rather than other properties (e.g., absence or presence of spicules, margin characteristics, etc.). Therefore, further evaluation should be performed to see whether nodule classification performance can be increased with the new lung nodules for other classifications, such as malignant or benign cases. A constant coefficient was used in the loss function to synthesize lung nodules by Wang et al. ( 2021 ). It affects the training performance and should be chosen carefully. Therefore, the performance of the augmentation method should be evaluated with increased a number of images. Although the method proposed by Toda et al. ( 2021 ) has the potential to generate images, spicula-like features are obscure in the generated images and it does not include the distribution of true images. Also, the generation of some features contained in the images (e.g., cavities around or inside the tumor, prominent pleural tail signs, non-circular shapes) are difficult with the proposed augmentation. Therefore, images with those features tend to be misclassified. In a study, augmentation with elastic deformations, which add shape variations, brings noise and damage when the deformation field is varied seriously (Müller et al. 2021 ). In a different approach, as it is identified by the authors, the predicted tumor regions are prone to imperfections (Farheen et al. 2022 ).
It has been observed that usage of the augmented images obtained by the combination of translation and shearing techniques in the classification of images with nodules and without nodules provides the highest performance than the other augmentation methods. On the other hand, augmentation only by shearing is the least efficient approach (Table 7 ).
About breast Mammography Image Augmentation techniques Augmentation of breast mammography images is one of the significant and fundamental directions that need to be focused on for further investigations and future research efforts. Although scaling, translation, rotation, and flipping are widely used, they are not suitable enough for augmentation since the additional information provided by them is not sufficient to make variations in the images, and so the diversity of the resulting dataset is limited. For instance, in a study performed by Zeiser et al. ( 2020 ), although the classifier classifies pixels having high intensity values as masses as it is desired, the network ends up producing FPs in dense breasts. Therefore, the augmentation technique applied as a pre-processing step should be modified to expand the datasets more efficiently and to increase the generalization skill of the proposed classifier. Besides, the performance of the applications should be tested with not only virtual images but also real-world images. Although the results of the applications that use generated images from a GAN based augmentation are promising (Table 3 ), their performances should be evaluated with increased numbers and variations of images. Since augmentations by synthesizing existing mammography images are difficult because of the variations of masses in terms of shape and texture as well as the presence of diverse and intricate breast tissues around the masses. The common problems in those GAN based augmentations are mode collapsing and saddle point optimization (Yadav et al. 2017 ). In the optimization problem, there exists almost no guarantee of equilibrium between the training of the discriminator and generator functions, causing one network to inevitably become stronger than the other network, which is generally the discriminator function. In the collapsing mode problem, the generator part focuses on limited data distribution modes and causes the generation of images with limited diversity.
Experiments in this study indicated that the combined technique consisting of translation, shearing, and rotation is the most appropriate approach for the augmentation of breast mammography images in order to improve the classification of the images as normal, benign, and malignant. On the other hand, the combined technique consisting of salt and pepper noise addition and shearing is the least appropriate approach (Table 8 ).
About Eye Fundus Image Augmentation techniques Further research on the augmentation techniques for fundus images is still needed to improve the reliability and robustness of computer-assisted applications. Because the tone qualities of fundus images are affected by the properties of fundus cameras (Tyler et al. 2009 ) and the images used in the literature have been acquired from different types of fundus cameras. Therefore, the presented applications fitting well to images obtained from a fundus camera may not generalize images from other kinds of fundus camera systems. Also, images can be affected by pathological alterations. Because of this reason, the applications should be robust on those alterations, particularly on the pathological changes that do not exist in the images used in the training steps. Therefore, although the results of the applications in the current literature indicated high performances (Table 4 ), their robustness should be evaluated using other data sets with increased numbers and variations of images and taken from different types of fundus cameras. Also, the contributions of the applied augmentation techniques to the presented performances are not clear. In a study performed by Zhou et al. ( 2020 ), although the GAN structure is able to synthesize high-quality images in most cases, the lesion and structural masks used as inputs are not real ground truth images. Therefore, the generator’s performance depends on the quality of those masks. Also, the applied GAN architecture fails to synthesize some lesions, such as microaneurysms. In another study performed by Ju et al. ( 2021 ), the GAN based augmentation might lead to biased results because of matching the generated images to the distribution of the target domain.
Experiments in this study indicated that usage of the augmented images obtained by the combination of color shifting with sharpening, and contrast changing provides higher performance than the other augmentation techniques in the classifications of eye fundus images. On the other hand, augmentation with translation is the least efficient approach for augmentation to improve classification performance (Table 9 ).
6 Conclusion
Transformation-based augmentation methods are easy to implement with medical images. Also, their effectiveness depends on the characteristics (e.g., color, intensity, etc.) of the images, and whether all significant visual features according to medical conditions exist in the training sets.
GAN based augmentation methods can increase diversity. On the other hand, GANs have vanishing gradient and mode collapsing problems. Also, obtaining satisfactory training results is not easy if the training procedure does not assure the symmetry and alignment of both generator and discriminator networks. Besides, GANs are complex structures and managing the coordination of the generator and discriminator is difficult.
Combination of rotation with shearing, and translation provides higher performance than the other augmentation techniques in the classifications of HGG and LGG cases from FLAIR types of brain images. However, augmentation with the combination of shearing and salt-and-pepper noise addition is the least efficient approach for augmentation in improving classification performance.
Combination of translation and shearing techniques in the classification of lung CT images with nodules and without nodules provides the highest performance than the other augmentation methods. However, augmentation only by shearing is the least efficient approach.
Combination of translation, shearing, and rotation is the most appropriate approach for the augmentation of breast mammography images in order to improve the classification of the images as normal, benign, and malignant. On the other hand, the combined technique consisting of salt and pepper noise addition and shearing is the least appropriate approach.
Combination of color shifting with sharpening, and contrast changing provides higher performance than the other augmentation techniques in the classifications of eye fundus images. On the other hand, augmentation with translation is the least efficient approach for augmentation to improve classification performance.
As an extension of this work, the effectiveness of the augmentation methods will be evaluated in the diagnosis of diseases from positron emission tomography, ultrasonography images, other types of MR sequences (e.g., T2, T1, and proton density-weighted), and also in the classification of other types of images such as satellite or natural images. Also, GAN based augmentations will be applied, quantitative and qualitative analyses of the generated images will be performed to ensure their diversity and realness. In this study, ResNet101 has been used due to its advantage based on residual connections and efficiency in classification. Therefore, the implementation of other convolutional network models will be performed in our future works.
Agustin T, Utami E, Al Fatta H (2020) Implementation of data augmentation to improve performance cnn method for detecting diabetic retinopathy. In: 3rd International conference on information and communications technology (ICOIACT), Indonesia, Yogyakarta, pp 83–88
Alshazly H, Linse C, Barth E et al (2021) Explainable covid-19 detection using chest ct scans and deep learning. Sensors 21:1–22
Google Scholar
Aly GH, Marey M, El-Sayed SA, Tolba MF (2021) Yolo based breast masses detection and classification in full-field digital mammograms. Comput Methods Programs Biomed 200:105823
Alyafi B, Diaz O, Marti R (2020) DCGANs for realistic breast mass augmentation in X-ray mammography. IN: Medical imaging 2020: computer-aided diagnosis, International Society for Optics and Photonics, pp 1–4. https://doi.org/10.1117/12.2543506
Araújo T, Aresta G, Mendonça L et al (2020) Data augmentation for improving proliferative diabetic retinopathy detection in eye fundus images. IEEE Access 8:462–474
Armato IIISG, McLennan G, Bidaut L, McNitt-Gray MF, Meyer CR, Reeves AP, Zhao B, Aberle DR, Henschke CI, Hoffman EA, Kazerooni EA (2011) The lung image database consortium (LIDC) and image database resource initiative (IDRI): a completed reference database of lung nodules on CT scans. Med Phys 38:915–931
Asia AO, Zhu CZ, Althubiti SA, Al-Alimi D, Xiao YL, Ouyang PB, Al-Qaness MA (2020) Detection of diabetic retinopathy in retinal fundus images using cnn classification models. Electronics 11:1–20
Aswathy AL, Vinod Chandra SS (2022) Cascaded 3D UNet architecture for segmenting the COVID-19 infection from lung CT volume. Sci Rep. https://doi.org/10.1038/s41598-022-06931-z
Article Google Scholar
Ayana G, Park J, Choe SW (2022) Patchless multi-stage transfer learning for improved mammographic breast mass classification. Cancers. https://doi.org/10.3390/cancers14051280
Bakas S, Reyes M, Jakab A, Bauer S, Rempfler M, Crimi A, Shinohara RT, Berger C, Ha SM, Rozycki M, Prastawa M (2018) Identifying the best machine learning algorithms for brain tumor segmentation, progression assessment, and overall survival prediction in the brats challenge. arXiv preprint, pp 1–49. arXiv:1811.02629
Balasubramanian R, Sowmya V, Gopalakrishnan EA, Menon VK, Variyar VS, Soman KP (2020) Analysis of adversarial based augmentation for diabetic retinopathy disease grading. In: 11th International conference on computing, communication and networking technologies (ICCCNT), India, Kharagpur, pp 1–5
Barile B, Marzullo A, Stamile C, Durand-Dubief F, Sappey-Marinier D (2021) Data augmentation using generative adversarial neural networks on brain structural connectivity in multiple sclerosis. Comput Methods Programs Biomed 206:1–12
Basu A, Sheikh KH, Cuevas E, Sarkar R (2022) Covid-19 detection from CT scans using a two-stage framework. Expert Syst Appl 193:1–14
Bayer M, Kaufhold MA, Reuter C (2021) A survey on data augmentation for text classification. ACM-CSUR. https://doi.org/10.1145/3544558
Borji A (2019) Pros and cons of gan evaluation measures. Comput Vis Image Underst 179:41–65
Carreira J, Zisserman A (2017) Quo vadis, action recognition? a new model and the kinetics dataset. In: Conference on computer vision and pattern recognition, Hawaii, Honolulu, pp 6299–6308
Chaki J (2022) Two-fold brain tumor segmentation using fuzzy image enhancement and DeepBrainet2.0. Multimed Tools Appl 81:30705–30731
Chatfield K, Simonyan K, Vedaldi A, Zisserman A (2014) Return of the devil in the details: delving deep into convolutional nets, pp 1–11. arXiv preprint. https://doi.org/10.48550/arXiv.1405.3531
Chen X, Wang X, Zhang K, Fung KM, Thai TC, Moore K, Mannel RS, Liu H, Zheng B, Qiu Y (2022a) Recent advances and clinical applications of deep learning in medical image analysis. Med Image Anal. https://doi.org/10.1016/j.media.2022.102444
Chen Y, Yang X, Wei Z, Heidari AA et al (2022b) Generative adversarial networks in medical image augmentation: a review. Comput Biol Med. https://doi.org/10.1016/j.compbiomed.2022.105382
Chlap P, Min H, Vandenberg N et al (2021) A review of medical image data augmentation techniques for deep learning applications. Med Imaging Radiat Oncol 65:545–563
Costa P, Galdran A, Meyer MI, Niemeijer M, Abràmoff M, Mendonça AM, Campilho A (2017) End-to-end adversarial retinal image synthesis. IEEE Trans Med Imaging 37:781–791
Decencière E, Zhang X, Cazuguel G, Lay B, Cochener B, Trone C, Gain P, Ordonez R, Massin P, Erginay A et al (2014) Feedback on a publicly distributed image database: the messidor database. Image Anal Stereol 33:231–234
MATH Google Scholar
Deepak S, Ameer P (2020) MSG-GAN based synthesis of brain mri with meningioma for data augmentation. In: IEEE international conference on electronics, computing and communication technologies (CONECCT), India, Bangalore, pp 1–6
Desai SD, Giraddi S, Verma N, Gupta P, Ramya S (2020) Breast cancer detection using gan for limited labeled dataset. In: 12th International conference on computational intelligence and communication networks, India, Bhimtal, pp 34–39
Dodia S, Basava A, Padukudru Anand M (2022) A novel receptive field-regularized V‐net and nodule classification network for lung nodule detection. Int J Imaging Syst Technol 32:88–101. https://doi.org/10.1002/ima.22636
Dorizza A (2021) Data augmentation approaches for polyp segmentation. Dissertation, Universita Degli Studi Di Padova
Dufumier B, Gori P, Battaglia I, Victor J, Grigis A, Duchesnay E (2021) Benchmarking cnn on 3d anatomical brain mri: architectures, data augmentation and deep ensemble learning. arXiv preprint, pp 1–25. arXiv:2106.01132
Farheen F, Shamil MS, Ibtehaz N, Rahman MS (2022) Revisiting segmentation of lung tumors from CT images. Comput Biol Med 144:1–12
Fidon L, Ourselin S, Vercauteren T (2020) Generalized wasserstein dice score, distributionally robust deep learning, and ranger for brain tumor segmentation: brats 2020 challenge. in: International MICCAI brain lesion workshop, Lima, Peru, pp 200–214
Girshick R (2015) Fast R-CNN. In: IEEE international conference on computer vision, Santiago, USA, pp 1440–1448
Girshick R, Donahue J, Darrell T, Malik J (2014) Rich feature hierarchies for accurate object detection and semantic segmentation. In: IEEE conference on computer vision and pattern recognition, Columbus, USA, pp 580–587
Halder A, Datta B (2021) COVID-19 detection from lung CT-scan images using transfer learning approach. Mach Learn: Sci Technol 2:1–12
Haq AU, Li JP, Agbley BLY et al (2022) IIMFCBM: Intelligent integrated model for feature extraction and classification of brain tumors using mri clinical imaging data in IoT-Healthcare. IEEE J Biomed Health Inf 26:5004–5012
Hashemi N, Masoudnia S, Nejad A, Nazem-Zadeh MR (2022) A memory-efficient deep framework for multi-modal mri-based brain tumor segmentation. In: 2022 44th annual international conference of the IEEE Engineering in Medicine & Biology Society (EMBC), Scotland, Glasgow, UK, pp 3749–3752
He K, Zhang X, Ren S, Sun J (2016) Deep residual learning for image recognition. In: IEEE conference on computer vision and pattern recognition (CVPR), Las Vegas, USA, pp 770–778
He K, Gkioxari G, Dollár P, Girshick R (2017) Mask R-CNN. In: Proceedings of the IEEE international conference on computer vision, Venice, Italy, pp 2961–2969
Hu R, Ruan G, Xiang S, Huang M, Liang Q, Li J (2020) Automated diagnosis of covid-19 using deep learning and data augmentation on chest CT. medRxiv, pp 1–11
Humayun M, Sujatha R, Almuayqil SN, Jhanjhi NZ (2022) A transfer learning approach with a convolutional neural network for the classification of lung carcinoma. Healthcare. https://doi.org/10.3390/healthcare10061058
Isensee F, J¨ager PF, Full PM et al (2020) nnu-Net for brain tumor segmentation. In: International MICCAI brainlesion workshop. Springer, Cham, pp 118–132
Islam MR, Abdulrazak LF, Nahiduzzaman M, Goni MO, Anower MS, Ahsan M, Haider J, Kowalski M (2022) Applying supervised contrastive learning for the detection of diabetic retinopathy and its severity levels from fundus images. Comput Biol Med. https://doi.org/10.1016/j.compbiomed.2022.105602
Jha M, Gupta R, Saxena R (2022) A framework for in-vivo human brain tumor detection using image augmentation and hybrid features. Health Inf Sci Syst 10:1–12
Ju L, Wang X, Zhao X, Bonnington P, Drummond T, Ge Z (2021) Leveraging regular fundus images for training UWF fundus diagnosis models via adversarial learning and pseudo-labeling. IEEE Trans Med Imaging 40:2911–2925
Karthiga R, Narasimhan K, Amirtharajan R (2022) Diagnosis of breast cancer for modern mammography using artificial intelligence. Math Comput Simul 202:316–330
MathSciNet MATH Google Scholar
Khan AR, Khan S, Harouni M, Abbasi R, Iqbal S, Mehmood Z (2021) Brain tumor segmentation using K-means clustering and deep learning with synthetic data augmentation for classification. Microsc Res Tech 84:1389–1399
Khosla C, Saini BS (2020) Enhancing performance of deep learning models with different data augmentation techniques: a survey. In: International conference on intelligent engineering and management (ICIEM), London, UK, pp 79–85
Kim YJ, Kim KG (2022) Detection and weak segmentation of masses in gray-scale breast mammogram images using deep learning. Yonsei Med J 63:S63
Kossen T, Subramaniam P, Madai VI, Hennemuth A, Hildebrand K, Hilbert A, Sobesky J, Livne M, Galinovic I, Khalil AA, Fiebach JB (2021) Synthesizing anonymized and labeled TOF-MRA patches for brain vessel segmentation using generative adversarial networks. Comput Biol Med 131:1–9
Kurup A, Soliz P, Nemeth S, Joshi V (2020) Automated detection of malarial retinopathy using transfer learning. In: IEEE southwest symposium on image analysis and interpretation (SSIAI), Albuquerque, USA, pp 18–21
Li Q, Yu Z, Wang Y et al (2020) Tumorgan: a multi-modal data augmentation framework for brain tumor segmentation. Sensors 20:1–16
Li H, Chen D, Nailon WH, Davies ME, Laurenson DI (2021) Dual convolutional neural networks for breast mass segmentation and diagnosis in mammography. IEEE Trans Med Imaging 41:3–13
Li Z, Guo C, Nie D, Lin D, Cui T, Zhu Y, Chen C, Zhao L, Zhang X, Dongye M, Wang D (2022) Automated detection of retinal exudates and drusen in ultra-widefield fundus images based on deep learning. Eye 36:1681–1686
Lim G, Thombre P, Lee ML, Hsu W (2020) Generative data augmentation for diabetic retinopathy classification. In: IEEE 32nd international conference on tools with artificial intelligence (ICTAI), Baltimore, USA, pp 1096–1103
Lin M, Hou B, Liu L, Gordon M, Kass M, Wang F, Van Tassel SH, Peng Y (2022) Automated diagnosing primary open-angle glaucoma from fundus image by simulating human’s grading with deep learning. Sci Rep. https://doi.org/10.1038/s41598-022-17753-4
Liu Y, Kwak HS, Oh IS (2022) Cerebrovascular segmentation model based on spatial attention-guided 3D inception U-Net with multi-directional MIPs. Appl Sci. https://doi.org/10.3390/app12052288
Long J, Shelhamer E, Darrell T (2015) Fully convolutional networks for semantic segmentation. In: Proceedings of the IEEE conference on computer vision and pattern recognition, Boston, USA, pp 3431–3440
Mahmood T, Li J, Pei Y, Akhtar F, Jia Y, Khand ZH (2021) Breast mass detection and classification using deep convolutional neural networks for radiologist diagnosis assistance. In: 45th Annual computers, software, and applications conf (COMPSAC), Madrid, Spain, pp 1918–1923
Mahmood T, Li J, Pei Y, Akhtar F, Rehman MU, Wasti SH (2022) Breast lesions classifications of mammographic images using a deep convolutional neural network-based approach. PLoS ONE. https://doi.org/10.1371/journal.pone.0263126
Manos D, Seely JM, Taylor J, Borgaonkar J, Roberts HC, Mayo JR (2014) The lung reporting and data system (LU-RADS): a proposal for computed tomography screening. Can Assoc Radiol J 65:121–134
Mayya V, Kulkarni U, Surya DK, Acharya UR (2022) An empirical study of preprocessing techniques with convolutional neural networks for accurate detection of chronic ocular diseases using fundus images. Appl Intell 1:1–19
McNitt-Gray MF, Armato SG III, Meyer CR, Reeves AP, McLennan G, Pais RC, Freymann J, Brown MS, Engelmann RM, Bland PH et al (2007) The Lung Image Database Consortium (LIDC) data collection process for nodule detection and annotation. Acad Radiol 14:1464–1474
Meijering E (2020) A bird’s-eye view of deep learning in bioimage analysis. Comput Struct Biotechnol J 18:2312–2325
Miller JD, Arasu VA, Pu AX, Margolies LR, Sieh W, Shen L (2022) Self-supervised deep learning to enhance breast cancer detection on screening mammography. arXiv preprint, pp 1–11. arXiv:2203.08812
Mok TC, Chung A (2018) Learning data augmentation for brain tumor segmentation with coarse-to-fine generative adversarial networks. In: International MICCAI brain lesion workshop, Granada, Spain, pp 70–80
Moreira IC, Amaral I, Domingues I, Cardoso A, Cardoso MJ, Cardoso JS (2012) Inbreast: toward a full-field digital mammographic database. Acad Radiol 19:236–248
Müller D, Soto-Rey I, Kramer F (2021) Robust chest CT image segmentation of COVID-19 lung infection based on limited data. Inf Med Unlocked 25:1–11
Naidich DP, Bankier AA, MacMahon H, Schaefer-Prokop CM, Pistolesi M, Goo JM, Macchiarini P, Crapo JD, Herold CJ, Austin JH, Travis WD (2013) Recommendations for the management of subsolid pulmonary nodules detected at CT: a statement from the Fleischner Society. Radiology 266:304–317
Nalepa J, Marcinkiewicz M, Kawulok M (2019) Data augmentation for brain-tumor segmentation: a review. Front Comput Neurosci 13:1–18
Naveed H (2021) Survey: Image mixing and deleting for data augmentation. arXiv preprint, pp 1–15. arXiv:2106.07085
Nayan AA, Mozumder AN, Haque M, Sifat FH, Mahmud KR, Azad AK, Kibria MG (2022) A deep learning approach for brain tumor detection using magnetic resonance imaging. arXiv preprint, pp 1–9. arXiv:2210.13882
Neelima G, Chigurukota DR, Maram B, Girirajan B (2022) Optimal DeepMRSeg based tumor segmentation with GAN for brain tumor classification. Biomed Signal Process Control. https://doi.org/10.1016/j.bspc.2022.103537
Nishio M, Muramatsu C, Noguchi S, Nakai H, Fujimoto K, Sakamoto R, Fujita H (2020) Attribute-guided image generation of three-dimensional computed tomography images of lung nodules using a generative adversarial network. Comput Biol Med. https://doi.org/10.1016/j.compbiomed.2020.104032
Onishi Y, Teramoto A, Tsujimoto M, Tsukamoto T, Saito K, Toyama H, Imaizumi K, Fujita H (2020) Multiplanar analysis for pulmonary nodule classification in CT images using deep convolutional neural network and generative adversarial networks. Int J Comput Assist Radiol Surg 15:173–178
Oza P, Sharma P, Patel S, Adedoyin F, Bruno A (2022) Image augmentation techniques for mammogram analysis. J Imaging. https://doi.org/10.3390/jimaging8050141
Padalia D, Vora K, Mehta D, Mehendale N (2022) EEF-Net: an enhanced efficientnet for breast tumor classification in mammograms. SSRN 4220435. https://doi.org/10.2139/ssrn.4220435
Quintana-Quintana OJ, De León-Cuevas A, Gonzalez-Gutierrez A, Gorrostieta-Hurtado E, Tovar-Arriaga S (2022) Dual U-Net-based conditional generative adversarial network for blood vessel segmentation with reduced cerebral MR training volumes. Micromachines. https://doi.org/10.3390/mi13060823
Ramya J, Rajakumar MP, Maheswari BU (2022) Deep cnn with hybrid binary local search and particle swarm optimizer for exudates classification from fundus images. J Digit Imaging 35:56–67
Razali NF, Isa IS, Sulaiman SN, Karim NK, Osman MK (2021) High-level features in deeper deep learning layers for breast cancer classification. In: 11th IEEE international conference on control system, computing and engineering (ICCSCE), Penang, Malaysia, pp 170–175
Ren S, He K, Girshick R, Sun J (2015) Faster R-CNN: towards real-time object detection with region proposal networks. Adv Neural Inf Process Syst 28:1–9
Russakovsky O, Deng J, Su H, Krause J, Satheesh S, Ma S, Huang Z, Karpathy A, Khosla A, Bernstein M, Berg AC (2015) Imagenet large scale visual recognition challenge. Int J Comput Vis 115:211–252
MathSciNet Google Scholar
Sabani A, Landsmann A, Hejduk P, Schmidt C, Marcon M, Borkowski K, Rossi C, Ciritsis A, Boss A (2022) BI-RADS-based classification of mammographic soft tissue opacities using a deep convolutional neural network. Diagnostics. https://doi.org/10.3390/diagnostics12071564
Salama WM, Aly MH (2021) Deep learning in mammography images segmentation and classification: automated CNN approach. Int Alexandria Eng J 60:4701–4709. https://doi.org/10.1016/j.aej.2021.03.048
Setio AA, Traverso A, De Bel T, Berens MS, Van Den Bogaard C, Cerello P, Chen H, Dou Q, Fantacci ME, Geurts B, van der Gugten R (2017) Validation, comparison, and combination of algorithms for automatic detection of pulmonary nodules in computed tomography images: the luna16 challenge. Med Image Anal 42:1–3
Shmelkov K, Schmid C, Alahari K (2018) How good is my GAN? In: Proceedings of the European conference on computer vision (ECCV), Germany, Munich, pp 213–229
Shen T, Hao K, Gou C, Wang FY (2021) Mass image synthesis in mammogram with contextual information based on GANS. Comput Methods Programs Biomed. https://doi.org/10.1016/j.cmpb.2021.106019
Shi H, Lu J, Zhou Q (2020) A novel data augmentation method using style-based GAN for robust pulmonary nodule segmentation. In: IEEE Chinese control and decision conference (CCDC), Hefei, China, pp 2486–2491
Shorten C, Khoshgoftaar TM (2019) A survey on image data augmentation for deep learning. J Big Data 6:1–48
Shyamalee T, Meedeniya (2022) D CNN based fundus images classification for glaucoma identification. In: 2nd International conference on advanced research in computing (ICARC), Belihuloya, Sri Lanka, pp 200–205
Simonyan K, Zisserman A (2014) Two-stream convolutional networks for action recognition in videos. Adv Neural Inf Process Syst 27:1–9
Singh LK, Garg H, Khanna M (2022) Deep learning system applicability for rapid glaucoma prediction from fundus images across various data sets. Evol Syst 13:807–836
Soulami KB, Kaabouch N, Saidi MN (2022) Breast cancer: classification of suspicious regions in digital mammograms based on capsule network. Biomed Signal Process Control. https://doi.org/10.1016/j.bspc.2022.103696
Srinivas C, KS NP, Zakariah M, Alothaibi YA, Shaukat K, Partibane B, Awal H (2022) Deep transfer learning approaches in performance analysis of brain tumor classification using MRI images. J Healthc Eng. https://doi.org/10.1155/2022/3264367
Sun Y, Yuan P, Sun Y (2020) MM-GAN: 3D MRI data augmentation for medical image segmentation via generative adversarial networks. In: IEEE international conference on knowledge graph (ICKG), Nanjing, China, pp 227–234
Sun X, Fang H, Yang Y et al (2021) Robust retinal vessel segmentation from a data augmentation perspective. In: International workshop on ophthalmic medical image analysis, pp 189–198
Tandon R, Agrawal S, Raghuwanshi R, Rathore NP, Prasad L, Jain V (2022a) Automatic lung carcinoma identification and classification in CT images using CNN deep learning model. In: Augmented intelligence in healthcare: a pragmatic and integrated analysis. Springer, Singapore. https://doi.org/10.1007/978-981-19-1076-0_9
Tandon R, Agrawal S, Chang A, Band SS (2022b) VCNet: Hybrid deep learning model for detection and classification of lung carcinoma using chest radiographs. Front Public Health. https://doi.org/10.3389/fpubh.2022.894920
Tang N, Zhang R, Wei Z, Chen X, Li G, Song Q, Yi D, Wu Y (2021) Improving the performance of lung nodule classification by fusing structured and unstructured data. Inf Fusion 88:161–174
Thung KH, Raveendran P (2009) A survey of image quality measures. In: IEEE international conference for technical postgraduates (TECHPOS), Kuala Lumpur, Malaysia, pp 1–4
Toda R, Teramoto A, Tsujimoto M, Toyama H, Imaizumi K, Saito K, Fujita H (2021) Synthetic ct image generation of shape-controlled lung cancer using semi-conditional InfoGAN and its applicability for type classification. Int J Comput Assist Radiol Surg 16:241–251
Tsuneki M (2022) Deep learning models in medical image analysis. J Oral Biosci. https://doi.org/10.1016/j.job.2022.03.003
Tufail AB, Ullah I, Khan WU, Asif M, Ahmad I, Ma YK, Khan R, Ali M (2021) Diagnosis of diabetic retinopathy through retinal fundus images and 3D convolutional neural networks with limited number of samples. Wirel Commun Mob Comput 2021:1–15
Tyler ME, Hubbard LD, Boydston K, Pugliese AJ (2009) Characteristics of digital fundus camera systems affecting tonal resolution in color retinal images. J Ophthalmic Photogr 31:1–9
Ueda D, Yamamoto A, Onoda N, Takashima T, Noda S, Kashiwagi S, Morisaki T, Fukumoto S, Shiba M, Morimura M et al (2022) Development and validation of a deep learning model for detection of breast cancers in mammography from multi-institutional datasets. PLoS ONE 17:1–15
van der Velden BH, Kuijf HJ, Gilhuijs KG, Viergever MA (2022) Explainable artificial intelligence (XAI) in deep learning-based medical image analysis. Med Image Anal. https://doi.org/10.1016/j.media.2022.102470
Wang W, Luo J, Yang X, Lin H (2015) Data analysis of the lung imaging database consortium and image database resource initiative. Acad Radiol 22:488–495
Wang Q, Zhang X, Zhang W, Gao M, Huang S, Wang J, Zhang J, Yang D, Liu C (2021) Realistic lung nodule synthesis with multi-target co-guided adversarial mechanism. IEEE Trans Med Imaging 40:2343–2353
Wang Y, Ji Y, Xiao H (2022) A Data Augmentation Method for Fully Automatic Brain Tumor Segmentation. arXiv preprint, pp 1–15. arXiv:2202.06344
Woan SL, Lai KW, Chuah JH, Hasikin K, Khalil A, Qian P, Xia K, Jiang Y, Zhang Y, Dhanalakshmi S (2022) Multiclass convolution neural network for classification of COVID-19 CT images. Comput Intell Neurosci. https://doi.org/10.1155/2022/9167707
Wu W, Lu Y, Mane R, Society et al (2020a) EMBC, Canada, Montreal, pp 1516–1519
Wu E, Wu K, Lotter W (2020b) Synthesizing lesions using contextual gans improves breast cancer classification on mammograms. arXiv preprint, pp 1–10. arXiv:2006.00086
Xie L, Chen Z, Sheng X et al (2022) Semi-supervised region-connectivity-based cerebrovascular segmentation for time-of-flight magnetic resonance angiography image. Comput Biol Med. https://doi.org/10.1016/j.compbiomed.2022.105972
Xu Q, Huang G, Yuan Y, Guo C, Sun Y, Wu F, Weinberger K (2018) An empirical study on evaluation metrics of generative adversarial networks. arXiv preprint, pp 1–14. arXiv:1806.07755
Yadav JK, Yadav S (2022) Computer-aided diagnosis of cataract severity using retinal fundus images and deep learning. Comput Intell 38:1450–1473
Yadav A, Shah S, Xu Z, Jacobs D, Goldstein T (2017) Stabilizing adversarial nets with prediction methods. arXiv preprint, pp 1–21. arXiv:1705.07364
Yi X, Walia E, Babyn P (2019) Generative adversarial network in medical imaging: a review. Med Image Anal. https://doi.org/10.1016/j.media.2019.101552
Yu Z, Xiang Q, Meng J, Kou C, Ren Q, Lu Y (2019) Retinal image synthesis from multiple-landmarks input with generative adversarial networks. Biomed Eng Online. https://doi.org/10.1186/s12938-019-0682-x
Yu H, Yang LT, Zhang Q, Armstrong D, Deen MJ (2021) Convolutional neural networks for medical image analysis: state-of-the-art, comparisons, improvement and perspectives. Neurocomputing 444:92–110. https://doi.org/10.1016/j.neucom.2020.04.157
Yuan Y (2020) Automatic brain tumor segmentation with scale attention network. In: International MICCAI brain lesion workshop. Lecture notes in computer science. https://doi.org/10.1007/978-3-030-72084-1_26
Zahoor S, Shoaib U, Lali IU (2022) Breast Cancer Mammograms classification using deep neural network and entropy-controlled Whale optimization Algorithm. Diagnostics. https://doi.org/10.3390/diagnostics12020557
Zeiser FA, da Costa CA, Zonta T et al (2020) Segmentation of masses on mammograms using data augmentation and deep learning. J Digit Imaging 33:858–868
Zhang K (2021) On mode collapse in generative adversarial networks. In: 30th International conference on artificial neural networks, Bratislava, Slovakia, pp 563–574
Zhou Y, Wang B, He X, Cui S, Shao L (2020) DR-GAN: conditional generative adversarial network for fine-grained lesion synthesis on diabetic retinopathy images. IEEE J Biomedical Health Inf 26:56–66
Download references
Author information
Authors and affiliations.
Department of Biomedical Engineering, Engineering Faculty, Akdeniz University, Antalya, Turkey
Evgin Goceri
You can also search for this author in PubMed Google Scholar
Contributions
It is a single-authored article.
Corresponding author
Correspondence to Evgin Goceri .
Ethics declarations
Competing interests.
The authors declare no known competing financial interests or personal relationships that could have appeared to influence the work reported in this paper.
Additional information
Publisher’s note.
Springer Nature remains neutral with regard to jurisdictional claims in published maps and institutional affiliations.
Rights and permissions
Springer Nature or its licensor (e.g. a society or other partner) holds exclusive rights to this article under a publishing agreement with the author(s) or other rightsholder(s); author self-archiving of the accepted manuscript version of this article is solely governed by the terms of such publishing agreement and applicable law.
Reprints and permissions
About this article
Goceri, E. Medical image data augmentation: techniques, comparisons and interpretations. Artif Intell Rev 56 , 12561–12605 (2023). https://doi.org/10.1007/s10462-023-10453-z
Download citation
Accepted : 27 February 2023
Published : 20 March 2023
Issue Date : November 2023
DOI : https://doi.org/10.1007/s10462-023-10453-z
Share this article
Anyone you share the following link with will be able to read this content:
Sorry, a shareable link is not currently available for this article.
Provided by the Springer Nature SharedIt content-sharing initiative
- Data augmentation
- Medical images
- Find a journal
- Publish with us
- Track your research
Continual Learning in Medical Imaging from Theory to Practice: A Survey and Practical Analysis
- Areeb Qazi, Mohammad
- Rehman Hashmi, Anees Ur
- Sanjeev, Santosh
- Almakky, Ibrahim
- Saeed, Numan
- Yaqub, Mohammad
Deep Learning has shown great success in reshaping medical imaging, yet it faces numerous challenges hindering widespread application. Issues like catastrophic forgetting and distribution shifts in the continuously evolving data stream increase the gap between research and applications. Continual Learning offers promise in addressing these hurdles by enabling the sequential acquisition of new knowledge without forgetting previous learnings in neural networks. In this survey, we comprehensively review the recent literature on continual learning in the medical domain, highlight recent trends, and point out the practical issues. Specifically, we survey the continual learning studies on classification, segmentation, detection, and other tasks in the medical domain. Furthermore, we develop a taxonomy for the reviewed studies, identify the challenges, and provide insights to overcome them. We also critically discuss the current state of continual learning in medical imaging, including identifying open problems and outlining promising future directions. We hope this survey will provide researchers with a useful overview of the developments in the field and will further increase interest in the community. To keep up with the fast-paced advancements in this field, we plan to routinely update the repository with the latest relevant papers at https://github.com/BioMedIA-MBZUAI/awesome-cl-in-medical .
- Computer Science - Computer Vision and Pattern Recognition

An official website of the United States government
The .gov means it’s official. Federal government websites often end in .gov or .mil. Before sharing sensitive information, make sure you’re on a federal government site.
The site is secure. The https:// ensures that you are connecting to the official website and that any information you provide is encrypted and transmitted securely.
- Publications
- Account settings
Preview improvements coming to the PMC website in October 2024. Learn More or Try it out now .
- Advanced Search
- Journal List
- Biomed Res Int

Modern Diagnostic Imaging Technique Applications and Risk Factors in the Medical Field: A Review
Shah hussain.
1 Department of Biochemistry, Faculty of Biological Sciences, Quaid-i-Azam University Islamabad, Pakistan
Iqra Mubeen
Niamat ullah.
2 Department of Pharmacy, Faculty of Biological Sciences, University of Malakand, Pakistan
Syed Shahab Ud Din Shah
Bakhtawar abduljalil khan.
3 Women's Wellness and Research Center, Hamad Medical Corporation, Doha, Qatar
Muhammad Zahoor
4 Department of Biochemistry, University of Malakand, Chakdara, Dir Lower, KPK, Pakistan
5 Department of Pharmacognosy, College of Pharmacy, King Saud University, Riyadh, Saudi Arabia
Farhat Ali Khan
6 Department of Pharmacy, Shaheed Benazir Bhutto University, Sheringal, Dir Upper, KPK, Pakistan
Mujeeb A. Sultan
7 Department of Pharmacy, Faculty of Medical Sciences, Aljanad University for Science and Technology, Taiz, Yemen
Associated Data
This is a review article. All data are taken from published research papers and available online.
Medical imaging is the process of visual representation of different tissues and organs of the human body to monitor the normal and abnormal anatomy and physiology of the body. There are many medical imaging techniques used for this purpose such as X-ray, computed tomography (CT), positron emission tomography (PET), magnetic resonance imaging (MRI), single-photon emission computed tomography (SPECT), digital mammography, and diagnostic sonography. These advanced medical imaging techniques have many applications in the diagnosis of myocardial diseases, cancer of different tissues, neurological disorders, congenital heart disease, abdominal illnesses, complex bone fractures, and other serious medical conditions. There are benefits as well as some risks to every imaging technique. There are some steps for minimizing the radiation exposure risks from imaging techniques. Advance medical imaging modalities such as PET/CT hybrid, three-dimensional ultrasound computed tomography (3D USCT), and simultaneous PET/MRI give high resolution, better reliability, and safety to diagnose, treat, and manage complex patient abnormalities. These techniques ensure the production of new accurate imaging tools with improving resolution, sensitivity, and specificity. In the future, with mounting innovations and advancements in technology systems, the medical diagnostic field will become a field of regular measurement of various complex diseases and will provide healthcare solutions.
1. Introduction
Medical imaging is the process of visual representation of the structure and function of different tissues and organs of the human body for clinical purposes and medical science for detailed study of normal and abnormal anatomy and physiology of the body. Medical imaging techniques are used to show internal structures under the skin and bones, as well as to diagnose abnormalities and treat diseases [ 1 ]. Medical imaging has changed into healthcare science. It is an important part of biological imaging and includes radiology which uses the imaging technologies like X-ray radiography, X-ray computed tomography (CT), endoscopy, magnetic resonance imaging (MRI), magnetic resonance spectroscopy (MRS), positron emission tomography (PET), thermography, medical photography, electrical source imaging (ESI), digital mammography, tactile imaging, magnetic source imaging (MSI), medical optical imaging, single-photon emission computed tomography (SPECT), and ultrasonic and electrical impedance tomography (EIT) [ 2 ].
Imaging technologies play a vital role in the diagnosis of abnormalities and therapy, the refined process of visual representation which contributes to medical personnel access to awareness about their patient's situation [ 3 , 4 ]. Electroencephalography (EEG), magnetoencephalography (MEG), and electrocardiography (ECG) are recording and measurement techniques that are not responsible to produce images, but these represent the data as a parameter graph vs. time or maps which shows the susceptible information with less accuracy. Therefore, these technologies can be said to form medical imaging on a limited scale. Worldwide, up until 2010, approximately 5 billion medical imaging techniques studies have been shown [ 5 ].
In the United States, approximately 50% of total ionizing radiation exposure is composed of radiation exposure from medical imaging [ 6 ]. Medical imaging technologies are used to measure illnesses, manage, treat, and prevent. Nowadays, imaging techniques have become a necessary tool to diagnose almost all major types of medical abnormalities and illnesses, such as trauma disease, many types of cancer diseases, cardiovascular diseases, neurological disorders, and many other medical conditions. Medical imaging techniques are used by highly trained technicians like medical specialists, from oncologists to internists [ 1 ].
Medical imaging technologies are mostly used for medical diagnoses. Medical diagnosis is the process of identification of patient disease and its symptoms. The medical diagnosis gives the information about the disease or condition needed for treatment that is collected from patient history and physical checkups or surveys. Due to no specificity of the many signs and symptoms of a disorder, its diagnosis becomes a challenging phase in medical science. For example, the case of erythema (redness of the skin) gives a sign of many diseases. Thus, there is a need for different diagnostic procedures the determination the causes of different diseases and their cure or prevention [ 7 ].
Historically, the first medical diagnosis composed by humans was dependent upon the observation of ancient doctors with their eyes, ears, and sometimes examination of human specimens. For example, the oldest methods were used to test on body fluids like urine and saliva (before 400 B.C). In ancient Egypt and Mesopotamia, doctors were able to measure the problem of the digestive tract, blood circulation, heartbeat, spleen, liver menstrual problems, etc. But unfortunately, medicine for curing diseases was only for wealthy and royal people.
At around 300 B.C., the use of the mind and senses as diagnostic tools was promoted by Hippocrates. He got a reputation as the “Father of Medicine.” Hippocrates supported a diagnostic protocol by testing the patient urine, observing the skin color, and listening to the lungs and other outward appearances. The link between disease and heredity also had been recorded by them [ 8 ]. In the Islamic world, Abu al-Qasim al-Zahrawi (Arabic physician) provided the first report on a hereditary genetic disease referred as hemophilia. In this report, he wrote about a family of Andalusia, whose males died due to hemophilia [ 9 ]. In the Middle Ages, many different techniques were used by physicians to detect the causes of imbalance function of the body. Uroscopy was the most common method of diagnosis. The patient's urine was collected in a special type of flask known as “Matula.” Urine was checked on the basis of color, smell, density, and presence of precipitate [ 10 ]. The viscosity and color of the blood were also examined by physicians to detect chronic or acute diseases [ 11 ]. The pulse rate, power, and tempo of a patient's artery were observed by physicians through a technique known as palpation [ 12 ]. In Middle Ages, physicians were also used to combining the study of medicine and zodiac signs [ 13 ].
In the 19 th century, X-rays and microscopes were the diagnostic tools that helped to diagnose and treat illnesses. At the beginning of the 19 th century, medical doctors diagnosed diseases by the examination of symptoms and signs. By the 1850s, many diagnostic tools such as ophthalmoscopes, stethoscopes, and laryngoscopes lead to evoke the medical doctors with the sensory power to develop other novel methods and techniques for diagnosing different illnesses. And in this way, a series of diagnostic tools including chemical tests, bacteriological tests, microscopic tests, X-ray tests, and many other medical tests were generated [ 8 ].
Medical imaging techniques are developed after the discovery of X-rays. In November 1895, Wilhelm Conrad Roentgen discovered X-ray. He got the Nobel Prize in 1901 for his discovery. Radiologists gave names to X-ray basis as “X-rays” or “plane film” used for diagnosing bone fractures and chest abnormalities. Fluoroscopy was developed due to a more powerful beam of X-ray for diagnosing the patient abnormalities. In 1920s, radiologists started giving information about various diseases like cancer of the esophagus, ulcers, and stomach. Fluoroscopy is now converted into computed tomography (CT).
Today CT scan is commonly used to diagnose many diseases. The mammography technique also uses an X-ray beam, to generate high-resolution breast images, monitoring breast cancer. In the 1940s, the X-ray tomography technique was developed, looking for a desired part of the tissue. In this technique, the whole process was accomplished by rotating the tube of X-ray focus on part of the tissue. Today, tomography is replaced with advanced imaging techniques such as CT scanning or computerized axial tomography (CAT) scanning. X-ray is also a source of a technique known as “angiography,” which is used to obtain images of blood vessels. In 1950s, diagnostic imaging tests along with nuclear medicine were started. Radioactive compounds are used as X-ray sources rather than X-ray tubes. Radioactive compounds produce gamma rays. They are joined with other complexes that are an essential part of the disease analysis to study a certain illness. For an instant, technetium 99m is combined with methylene diphosphonate, which is absorbed by bone tumors. In this way, breast or lung cancer spread to other body parts such as bone can be detected from this type of nuclear bone scan technique [ 14 ].
2. Advance Modalities in Medical Imaging
Many advanced techniques are developed and can be explained with their principle of work, application in medical labs, and development in imaging techniques. Computed tomography (CT), positron emission tomography (PET), magnetic resonance imaging (MRI), single-photon emission computed tomography (SPECT), digital mammography, and sonography are included in advanced medical imaging techniques. These are all mentioned below to understand their advantages and applications in the diagnosis, management, and treatment of different diseases such as cardiovascular disease, cancer, neurological illnesses, and trauma. These techniques are readily used by clinicians because through images, they can easily choose how to manage diseases.
3. Computed Tomography (CT)
In the 1969s, Hounsfield invented the first CT-scanner prototype [ 15 ]. Computed tomography is also known as X-ray CT. A CT scan is used by radiologists, biologists, archaeologists, and many other scientists to generate cross-sectional images of different scanned objects. A modern CT scanner system is shown in Figure 1 . In the medical field, technicians use CT scanners, machines to produce the images that lead to diagnosing the abnormalities and other therapeutic measurements. In this technology, X-rays are produced from different angles that are eventually processed by computers to create tomographic images. This computer-based technology has been greatly improved, developing reconstructed images with high revolution [ 16 ]. In the pharmaceutical industry, it has been used to study and improve the medicine manufacturing process to generate good quality products [ 17 ].

CT scanner.
Positron emission tomography (PET) and single-photon emission computed tomography (SPECT) are the types of CT scan. An X-ray generator is used to generate the X-rays that rotate nearby the object to be scanned. X-rays are detected by an X-ray detector located on the opposite side of the source of X-rays. A sonogram is obtained, which is a visual representation of raw form data. This scanned data is processed in the form of tomographic reconstruction that leads to generating a series of cross-sectional photos. CT scan is performed by special individuals called radiology technologists or radiographers. Over the last two decades, CT is used largely in many clinical labs in different countries [ 18 ]. According to an estimated study, almost a 72 million scans were achieved in the US in 2007 and 80 million scans in 2015 [ 19 ].
CT is an effective technique for monitoring various types of cancers such as cancer of the bladder, kidneys, skeleton, neck, and head and for diagnosing infection [ 20 – 22 ]. CT also identifies distant metastases to the lungs, skeleton, liver, and brain. CT has made a high impact on the brain and lungs [ 23 , 24 ]. CT scan is the best method than other techniques in detection as well as recording modifications in tumor mass during treatment [ 25 ]. It may show a bloated belly with enlarged lymph nodes in patients with bronchus carcinoma. In this way, CT scan help in performing before surgery [ 26 ]. Another major application of CT scans is the detection of heart diseases like myocardial disease, congenital heart disease, and coronary artery bypass grafts [ 27 ]. Gastroenterologists mostly use computed tomography for the analysis of the liver or pancreas of patients. Tumors of size 1.5-2.0 cm in diameter can be detected by CT scan. Furthermore, biliary obstruction caused by lesions can also be monitored by this technology [ 28 ]. One of the rewarding roles of technology is to study suspected intra-abdominal abnormalities with 95% accuracy, and treatment decisions can be easily made [ 29 ].
A big drawback of computed tomography is that large masses within the gastrointestinal tract may not be visible during the abdominal investigation. There is also no finding of some of the mucosal abnormalities by it. CT scan is highly useful to manage abdominal disorders such as carcinoma of the stomach, esophagus, and rectum more accurately as compared to other modalities [ 30 , 31 ] The middle column of the spine can be visualized by using the computed tomography technique during dislocation type of fractures in many thoracolumbar fractures. CT also detects lesions and provides nonsurgical management of some disorders, for example, unstable burst damages [ 31 ].
All spinal injuries are unstable and known as translational injuries. Before surgery of such patients, complete information about the site of ligament discontinuity of respective vertebrae is provided by computed tomography. It also gives the prediction of whether Harrington-rod stabilization is possible or not. CT scan can provide detailed evidence of distraction injuries and fractures. For example, flexion-distraction injury between the 11 th and 12 th thoracic vertebrae and spinal injury between the 2 nd and 3 rd lumbar vertebrae have been scanned by computed tomography scan as shown in Figures Figures2 2 and and3, 3 , respectively [ 32 ].

Distraction injury scanned by CT scan (showing damage occurrence at the 11 th and 12 th thoracic vertebrae).

An axial scan of a spinal injury by computed tomography (CT) at the 2 nd and 3 rd lumbar vertebrae.
Advanced CT bone imaging techniques include volumetric quantitative CT (QCT), high-resolution CT (CT), and micro-CT. High-resolution CT and high-resolution MR are generally used in vivo ; micro-CT and micro-MR are usually used in vitro systems. These advanced modalities are used for bone imaging to investigate bone diseases especially osteoporosis and bone cancer. In osteoporosis, disorder advanced CT bone imaging provides information about bone mineral density (BMD), bone strength, a risk factor for osteoporosis, and recovery factors after medication or bone therapy.
Dual-energy X-ray absorptiometry (DXA) and volumetric QCT are the quantitative methods used for weighing the macrostructure of suspected bone. High-resolution CT and micro-CT methods are applicable for measuring the microstructure of trabecular bone without any invasiveness or destructiveness. CT and MRI have been used to obtain bone structure. However, the CT field has been more developed as compared to other techniques, because there are more advantages of CT-based modalities; for example, QCT generates three-dimensional (3D) images in such a way that trabecular and cortical bone can be distinctly measured. vQCT technology is quicker than MRI [ 33 ].
4. Volumetric Quantitative CT (vQCT)
Initially, QCT has been used to measure trabecular BMD of the forearm and lumber midvertebrae through a particular transverse CT slice. The measurement of BMD is a static property of the advanced spiral QCT [ 34 ]. Trabecular bone in the spine and cortical bone in the hip may be indicated by this technology to estimate the fracture risk [ 35 ].
For the improvement of the 3D structure of the cortex, almost 0.5 mm isotropic spatial resolution is required, but still, almost 1.5 to 2 mm resolution is provided by QCT which is not adequate to make accurate images. This is a drawback of QCT. In general, the measurement of accurate cortical thickness for the femur is easier than the thickness in the spine, especially in aged people. Researchers have shown that women grow faster not only with small vertebrae as well as reduced bone mass but also with a slow rate of increase in cross-sectional area as compared to men [ 35 ]. QCT is a CT imaging technique that can provide information about bone density. For example, a QCT scan of the femur for the measurement of macrostructure and bone mineral density (BMD) has been shown in Figure 4(a) [ 33 ].

(a) Femora undergo vQCT to determine BMD and macrostructure. (b) Ultradistal forearm undergoes CT to measure the structure of the trabecular complex network and its texture.
5. High-Resolution CT (hrCT)
High-resolution CT is a modern CT scanner that usually requires a high radiation dose but produces high-resolution images of bone such as forearm bone submitted to CT to determine the trabecular and cortical network and texture as shown in Figure 4(b) [ 33 ]. According to many different cross-sectional studies, CT gave better imaging results in distinguishing fractured vertebral trabecular structures from nonfractured structures as compared to DXA measurements of BMD [ 36 ].
6. Micro-CT ( μ CT)
Micro-CT with 1-100 μ m spatial resolution is typically known as microscopy. Micro-CT has abilities to replace the standing techniques used in in vivo measurements in rats and mice like animals. Initially, the micro-CT technique used synchrotron radiations to obtain ultra-high-resolution applications [ 37 ].
Mostly, now, the convenient method of X-ray tube-based micro-CT is used in university-based research laboratories and special clinical centers. To make 3D structures of bone, some special software (for example, FEM) is attached with a micro-CT scanner. Finite element modeling (FEM) is a software mostly used in engineering. Its goal is to help information of 3D structures of bone for analysis of fractured bone part structures from nonfractured bone structures. Currently, structural models are generated by volumetric QCT, and computer-based programs give the element elastic properties from the bone density at the site of elements [ 38 ].
Arlot and coworkers determined the 3D microstructure of bone of postmenopausal women with osteoporotic disease; they have accomplished treatment with proper strontium ranelate therapy for 36 months [ 39 ]. Researchers investigate these 3D micro-bone structures as shown in Figure 5 [ 33 ]. Over the past two decades, considerable development occurred in imaging technologies for osteoporosis bone disease analysis. Despite the development in these technologies, there are many challenges for bone imaging, such as the sample size, spatial resolution, complexity, radiation exposure, time, and cost. Finally, there is still a requirement of high accuracy, availability, reproducibility, and proper monitoring procedure for better bone imaging [ 33 ].

Microstructure of transiliac bone biopsies is determined to undergo 3D micro-CT of two postmenopausal women who have accomplished strontium ranelate therapy for 36 months: (a) strontium ranelate therapy; (b) placebo.
CT scan for lung disease is highly used in present days. For example, a low-radiation helical chest CT scan is used to investigate lung cancer (bronchopulmonary cancer) [ 40 , 41 ]. Another lung disease is the most common type of progressive idiopathic interstitial pneumonia mostly in adults known as idiopathic pulmonary fibrosis (IPF). In IPF patients, CT-based methods include density histogram analysis, CT scan of whole lungs, and density mask technique, and other structural or texture classification methods are greatly used to examine the pulmonary function, lung disease progression, and mortality. For example, lung images of a 73-year-old male IPF patient have been taken by CT as shown in Figure 6 . These methods have the property of time efficiency, availability, and reproducibility. Still, there are many issues interrelated to computer-based CT in IPF disease analysis. But it is promising by scientists to develop advanced CT imaging techniques that must play a vital role in the future to manage lung diseases as well as other abdominal diseases [ 42 , 43 ].

Images derived using a system known as GHNC (Gaussian Histogram Normalized Correlation). Lung images of a 73-year-old male IPF patient have been taken by computed tomography (CT). Light blue and yellow color, fibrosis; dark blue color, emphysema; pink color, normal; and light green color is indicated as ground-glass opacity.
There are many possible reasons for the usage of CT scans, for example, to determine or investigate the acute stroke in the patient's head. CT is applicable to establish the diagnosis, investigate the type of stroke, respond to surgery, and finally manage the disease [ 44 ]. CT scan of the head is also responsible for the investigation of dementia disease. Accurate diagnosis is directly related to proper management of symptoms and signs of dementia. Patients with treatable lesions can also be identified by computed tomography [ 45 ]. Abdominal computed tomography is a new technology for identifying fungal infection known as disseminated fungal infection (DFI) in pediatric cancer patients. Currently, abdominal CT is greatly applicable for the diagnosis and management of DFI in cancer patients [ 46 ]. During the past few years, the usage of CT scan has become a national trend in emergency departments, especially in the US. Computed tomography plays an expanding role in diagnosing acute and chronic diseases as well as life-threatening diseases such as stroke, head injury, major trauma, heart disease, abdominal pain, pulmonary embolism, severe chest pain, and renal abnormalities [ 47 ].
7. 3D Ultrasound Computed Tomography (3D USCT)
3D USCT is a promising technology for imaging breast cancer. Simultaneous recording of reproducible reflection, speed of sound volume, fast data collection, attenuation, and high image quality production are all the main advantages of the USCT system. 3D USCT system is a full potential device used for clinical purposes. Only in 4 minutes, the full volume of breast can be picked up [ 48 ].
8. Risks of Computed Tomography
Computed tomography risks are small but if these small risk s are produced by million numbers of scans, they may drive into serious public health concerns in the future, especially from pediatric CT. The risk of cancer due to computed tomography scanning is increasing [ 49 ]. Children are of specific concern due to the sensitivity of radiation-induced cancer as compared to adults. According to a study, the risks of leukemia and brain tumors are mostly revealed after exposure to radiation from CT scans [ 50 ].
According to the authors of a recent report “long-term risks from CT scans directly would require very large-scale studies with lifelong follow-up.” The author gave this statement after the study date on CT scan exposure leading to the risk of future cancer [ 51 ]. Radiologists should be a source of discussion earlier to perform imaging technologies that contain high doses of radiation. They should also know about the risk factors of imaging technologies that can cause more adverse effects than recovery. Both families and patients should also raise the question/answers about the benefits and risks of CT scans [ 52 ].
9. Positron Emission Tomography (PET)
Positron emission tomography is a nuclear medicine functional technique that is used to display the total concentration of radioactive labeled elements in the body with clear images. It has the potential to diagnose biological processes within living bodies and is highly applicable for clinical purposes [ 53 ]. 3D images of positron-emitting radionuclides within the body are made by a computer system. In PET-CT scanners, 3D imaging is created with the help of a CT X-ray scan implemented on the patient body in the same machine and session. Positron emission tomography (PET) and nuclear magnetic resonance (NMR) both are quantitative radiological techniques that display information about biochemistry and physiology, normality, or abnormality. Nuclear magnetic resonance is not more sensitive to give high-resolution images by the distribution of substances except hydrogen. It can measure the total concentration of ATP and creatine phosphate (CP) in particular areas of the brain. Thus, both NMR and PET performed their specific function in the diagnosis. In 1953, the first PET system was established at Massachusetts General Hospital. It was followed by many other devices in a series manner such as tomographic positron camera, PET scanner, and other PET instruments [ 54 ].
10. Working Principle of PET
The PET technique detects radioactivity emission when a small concentration of radioactive tracer is intravenously injected. These tracers are frequently labeled with carbon-11, nitrogen-13, oxygen-15, and fluorine-18, as shown in Figure 7 . There is no positron emitter of hydrogen. The radioactive dose amount is the same as used in CT. 10-40 minutes is required to complete the process to perform a PET scan. The patient is fully clothed during scanning. There are specific steps in PET scan processing, explained in Figure 7 [ 55 ].

Basic principle of PET scan: (1) a positive electron (positron) is emitted by radioactive decay of radioisotope, for example, carbon-11; (2) this positron hits an electron present in the tissue to be analyzed and emits two photons having low energy; (3) scintillation crystals are present in the PET camera to absorb this emitted photon with low energy; (4) the light is produced that is converted into another signal such as electrical signals used by the computer system to produce 3D images.
Two molecular probes are mostly used to explain PET assay: 2-[F-18] fluoro-2-deoxy-D-glucose (FDG) and 3-deoxy-3-[F-18] fluorothymidine (FLT). FDG is the analog of F-18-labeled glucose, and it is used to identify diseases by changing the metabolism of glucose in heart diseases, Alzheimer's disease, and cancer. FLT is the analog of F-18 labeled thymidine and is highly used to estimate processes like cell proliferation and DNA replication by analysis of the phosphorylation process and thymidine transport. Thus, FLT and FDG are considered as best candidate probes/tracers for molecular imaging.
An early diagnosis of Alzheimer's disease can be scanned by PET technology with 93% accuracy. Huntington's disease, a hereditary disease was also detectable by PET scan. The development of PET technology provides accurate whole-body images for examining early primary and metastatic diseases. Imaging of transgenes provides information on the regulation of gene expression during cell proliferation, growth, response to environmental stimuli, the aging process, and gene therapy. Such endogenous gene expression can be monitored through the developed PET approach, which uses F-18-labeled oligodeoxynucleotides having a short single strand of almost fifteen nucleotides. In monkeys with 1-methyl-4-phenyl-1,2,3,6-tetrahydropyridine- (MPTP-) induced lesions on one side of the brain, the study of restoring dopamine production by gene therapy can be assessed by PET imaging, as shown in Figure 8 [ 56 ].

PET images of gene therapy in unilateral MPTP monkey as Parkinson's model: (a) image of normal dopamine production; (b) the image is representing autonomous dopamine MPTP-induced shortage (before gene therapy); (c) image of the restoration of dopamine production in the caudate and putamen (after gene therapy) [ 56 ].
The combination of PET and computed tomography (PET-CT) forms a hybrid imaging approach that is highly used to gain functional and metabolic information to measure inflammatory and infectious diseases to assess their proper treatment. PET-CT hybrid imaging technique can provide quick information during the diagnosis of a disease and its treatment response [ 57 ].
Haberkorn et al. measured FDG uptake that relates to the proliferation rate of tumor cells in the head and neck with different patterns, in two groups of patients [ 58 ]. The FDG uptake was also measured in the malignant neck and head tumors and metastases process by the use of FDG-PET. Minn and coworkers found that the uptake of FDG is related to the proliferation rate of tumor cells [ 59 ]. Jabour et al. measured the normal anatomy of the neck and head [ 60 ]. In this way, change in uptake FDG as investigated by PET provides necessary information for clinical and anticancer therapeutics [ 61 ].
In the human cerebellum, changes in local neuronal function by voluntary movement and tactile stimulation were also mapped with the help of the PET approach detection of brain blood flow. According to research, finger movement leads to the production of parasagittal and bilateral blood flow enhancement in the superior and anterior hemispheric cortex of the brain human brain cerebellum. The enhancement in midline blood flow in the posterior vermis of the human brain cerebellum is produced by saccadic eye movement. PET also allows the measurement of structural and functional relations in the cerebellum of the human brain [ 62 ]. The development of the PET brain imaging technology makes it possible to advance understanding of the anatomy of brain parts and map of neuroanatomical basis of cognitive processes and memory [ 63 ]. A pathogen SIV (simian immunodeficiency virus) causes infection in rhesus macaques (a type of monkeys) with acute viremia, and progression leads to infection in the solid tissues of lymphoid, and then, cellular degradation becomes a terminal disease, and death occurs in most cases. So, the FDG-PET imaging technique is used to take images from SIV-infected animals. In this way, infected groups can be distinguished from the uninfected control groups [ 64 ].
11. Future of PET Technology
The PET scan can be used to measure the concentration of amino acids, sugar, fatty acids, and receptor in the living body. It is a new diagnostic tool used to detect diseases such as atherosclerosis, aging, cancer, and schizophrenia, although improvement in instrumentation and modeling is still required for future purposes. Emission tomography has also been associated with a small risk of ionizing radiations [ 54 ].
12. Magnetic Resonance Imaging (MRI)
Magnetic resonance imaging (MRI) is primarily an imaging technique that is applicable for noninvasive visualization of the anatomy and physiology of the body in both disease and health conditions. An MRI-related technique known as echo-planar imaging (EPI) was developed by physicists Peter Mansfield and Paul Lauterbur in the late 1970s [ 65 ]. Magnetic fields, electric fields, and radio waves are used in an MRI scanner to produce images of organs and the structure of the body. The SI unit of magnetic flux density (magnetic field strength) is measured in tesla (T).
The most common detections by MRI are multiple sclerosis, CNS tumors, brain and spine infections, stroke, injuries in ligaments and tendons, muscle degradation, bone tumor, and occlusion of blood vessels. MRI uses nonionizing radiation, frequently preferable compared to CT. MRI also provides excellent contrast of soft tissues; for example, the white and gray matter structure of the brain can easily be distinguished through this approach. MRI employs other different techniques such as functional MRI, magnetic resonance angiography (MRA), susceptibility-weighted, diffusion-weighted (PWI), diffusion-weighted (DWI), gradient echo, and spin-echo. It provides an image of good quality without requiring repositioning of the patient [ 66 ]. There are several benefits to MRI such as it is a painless, noninvasive technique with high spatial resolution and nonionizing radiations. MRI is mostly used independently for soft tissue analysis.
13. Working Principle of MRI
An MRI machine consists of multiple components, including a slab for patients to lie on, a superconducting magnet, a protective cage, the operator's console, and computers to analyze the data and product images. During the MRI scanning process, the machine's magnet produces a strong magnetic field. Hydrogen ions align in the target body part of the patient due to a stable magnetic field. Then, bombardment of radiofrequency waves causes the alignment of lined-up hydrogen ions to move out, and then, ions return to their equilibrium state [ 67 ]. An attached computer system converts the spin echoes (signal) of hydrogen ions into the images, after several “shifting” and “working on.” A microphone is also present inside the MRI unit for communication between the patient and technologist during the imaging process. Images of only the target part of the body are created through MRI radiological analysis. The physician chooses which part of the patient's body must be analyzed by imaging, to diagnose the illness of the patient [ 68 ].
14. Applications of MRI
A major application of whole-body MRI is to investigate skeletal metastases. The MRI approach allows for visualization of the tumor because the tumor matrix contains an abundance of the proton. It is a more sensitive imaging technique than skeletal scintigraphy (bone scan) in the measurement of skeletal metastases. The whole-body MRI technique is more effective for detecting lesions in the pelvis, spine, and femur. This technique is also highly used as a primary diagnostic tool for the measurement of soft tissue diseases, whole-body fat, and polymyositis disease [ 69 ].
MRI is different from other diagnostic techniques because MRI has no risk of ionizing radiation. MRI has no side effects unlike CT and PET scans. There is no loss of image quality due to the scanning of body target parts from several angles and viewpoints [ 70 ]. Dynamic contrast-enhanced magnetic resonance (DCE-MRI) has been developed for the detection of the tumor microenvironment and its treatment. It has been supported as a useful method and improved clinical interest [ 71 ].
An advantage of using MRI to diagnose cardiovascular diseases is that examination reveals function structure perfusion, metabolism, and blood flow in the heart. A cardiovascular MRI is a source for the detection of congenital cardiac diseases, abnormalities in the thoracic aorta, and pericardium in heart patients. During the detection of myocardial tumor or right ventricular dysplasia, tissues are differentiated due to varying imaging parameters of the MRI approach. Another application of MRI, cardiovascular MRI, is applicable for determining cardiac prognosis, ischemia in a patient with heart disease, artery arteriosclerosis, and screening the myocardial viability [ 72 ].
Schizophrenia patients show mental abnormality which leads to language processing deficits and abnormal social behavior. Functional MRI has been applicable for remarks of such types of illnesses. The region of hypoactivity can be determined in the frontotemporal cortex of the patient brain. Soft neurological signs and symptoms have also been promoted. Functional MRI can detect abnormalities in the cerebrum; cerebral asymmetry images reveal changes in patients with schizophrenia compared with control as shown in Figure 9 [ 73 ].

Abnormal cerebral asymmetry in schizophrenia patients compared with control is shown by functional-MRI imaging technique.
Microfluidic LOC (lab-on-a-chip) is a device used as an emerging technology in medical laboratories. Sample (consist of suspensions of cells) and reagents react on these devices. For monitoring reaction on LOC, MRI is considered an ideal tool. MRI records the signals from the expended fluid leavings in the device. MRI combined with MRS (magnetic resonance spectroscopy) monitors fluid flow processes, chemical reaction separations, and diffusion processes in LOC. But MRI and MRS both show low sensitivity. In the future, there is a hope that MRI will be applicable for the advancement of microfluidic LOCs with powerful usage in medical diagnostic libraries [ 74 ].
Mutation in BRCA1 and BRCA2 genes leads to losing their ability to repair the damaged DNA, causing cancer, especially breast cancer. MRI diagnoses breast cancer which is due to a genetic mutation. Mostly, these hidden breast cancers are not detected by mammography. For a decade, doctors use MRI imaging tools to detect breast cancer [ 75 ]. According to previous research, 27-37% of patients have shown lesions on MRI, which are not seen through mammography. The researchers noted that mammography had a low value of positive prediction of 52.8%, as compared to MRI which is high at 72.4% [ 74 ].
Molecular MRI employed for specific and early detection of pulmonary metastatic cancer cells can improve its treatment. In research, pulmonary cancer cells are besieged by iron oxide nanoparticles having the ability to bind with ligand expressed on the cells. Then, images were taken by high-resolution hyperpolarized 3 He MRI (HP 3 He MRI). The study confirmed that HP 3 He MRI pooled with targeted superparamagnetic iron oxide nanoparticle (SPION) contrast agent detects specific and early metastatic pulmonary cancer in mice. A researcher used the LHRH-SPION agent to explore new drug procedures. For this purpose, they injected breast adenocarcinoma cells into mice and then detect pulmonary defects as cancer formation in mouse lungs with the help of LHRH-SPIONs and HP 3 He MRI results are shown in Figure 10 [ 76 ].

Breast adenocarcinoma mouse model formed for the detection of lung metastases. (a) High-resolution hyperpolarized 3 He MRI (HP 3 He MRI) images were taken from the control mouse. Screening as normal ventilation forms of lungs. (b) After injection of LHRH-SPIONs, images were produced from human breast adenocarcinoma abnormal mouse (model), showing defects in the right lobe (under circles).
Multinuclear 3D solid-state MRI allows images of tooth bone and calcium phosphate components of bone substances. It also gives information on the bone composition and texture of the bone [ 77 ]. Recent neuroimaging techniques including high-resolution MRI can investigate myeloarchitectural patterns in the cortex of the human brain. The bands of myelination have been revealed by the staining technique. Now, the same band in good quality image form can also be obtained by high-resolution MRI imaging technique. Although the advanced technology has been largely applied in the visual system, further improved methodologies are required for the investigation of another brain region. To overcome high ratio of “signal” to “noise” is a challenge for MRI machines that is produced due to the increased resolution of the image [ 78 ]. In this way, fMRI and other types of MRI have been powerfully used to change our understanding of diseases, their causes, and how to manage the conditions.
15. Simultaneous Imaging with MRI and PET
In in vivo study, imaging of small animals, for example, mouse imaging by combined MRI and PET modalities, produces constant information of different parts of the body. An experimental study reveals that the combined PET/MRI technique improves the understanding of malignant tissues and heterogeneous tumors, edema, and necrosis that are not done by MRI alone. Particular ionic 18 F is also used for PET/MRI combined imaging of small animals as models of bone metastasis, osteoporosis, and arthritis to study the complete skeletal system [ 79 ].
16. Risks of Magnetic Resonance Imaging
Magnetic resonance imaging (MRI) is highly expensive, low in sensitivity, and time-consuming for scanning and processing compared to other imaging modalities. A probe with a bulk quantity may be needed for MRI. It cannot detect abnormalities of intraluminal body parts. It gives no real-time information. And it can create a suffocating environment for some people [ 2 ].
17. Single-Photon Emission Computed Tomography (SPECT)
Single-photon emission computed tomography (SPECT) is an advanced imaging technique using gamma rays and provides three-dimensional (3D) representations of objects with high accuracy. In 1963, Kuhl and Edwards [ 80 ] gave the first report about single positron emission computed tomography. Gradually, modification with new instruments such as computer-attached systems and rotating gamma cameras leads to the development of a novel modality of single-photon emission tomography.
SPECT has become a great medical imaging technique used in research and clinical area. A dual-headed single-photon emission tomography (SPECT) system has been shown in Figure 11 [ 81 ]. It monitors the 3D information of an object by producing series of thin slices from tomographic images. These essential tomographic images can improve the ability detection of deep and very small fractures in patients [ 81 ].

Dual-headed single-photon emission computed tomography (SPECT) system.
SPECT assesses the multiple two-dimensional (2D) images from different angles by using high-energy gamma rays. Data is reconstructed and recorded, and 3D images of the target portion of the body are produced by a computer program. SPECT is greatly used in clinics and research laboratories like other tomographic modalities such as PET, MRI, and CT. SPECT and PET both use the radioactive tracer and then measure the emitted gamma rays. In the case of SPECT, emitted gamma rays by radioactive tracers are directly detected by the detector. The computer system analyzes the data from the detector and produces the true image of the area where the radioactive tracers are injected. SPECT imaging technique is less expensive than exclusively used for imaging small animals. It is sensitive to monitoring target bone metabolism, myocardial disorders, and blood flow in the cerebrum [ 82 ].
SPECT has also been designed for imaging of the brain known as neurochemical brain imaging. It has a powerful imaging technique to elaborate the neuropsychiatric diseases. It is an essential developmental technique that has great potential to monitor the pathophysiology and many other complex disorders of the brain [ 83 ].
Today, hybrid SPECT and CT are progressively employed and available in the nuclear medicine field. SPECT/CT provides exact abnormal bone turnover during inflammation, bone tumor, bone regeneration, bone infection, and trauma in complex bone joints such as the knee, hip, foot, shoulder, and hand/wrist. In most cases, CT with specificity and SPECT with high sensitivity are performed together for a complete diagnosis. SPECT/CT is also responsible for giving the proper information about therapy planning. For example, it tells us about the decision of using SPECT/CT alone or joint arthroplasty. It was observed that joint arthrography of the knee gives better results as compared to SPECT/CT alone as shown in Figure 12 [ 84 ].

(a) The SPECT/CT scan of the knee joint shows clear articular cartilage. (b) The SPECT/CT arthrography image of the knee joint showing enhance the value of SPECT/CT screening.
SPECT/CT technique has great potential for the measurement of articular cartilage, soft bodies, and synovial structures. It also gives promising results in the examination of osteochondral abnormalities [ 84 ].
18. Risks of Single-Photon Emission Computed Tomography (SPECT)
Single-photon emission computed tomography (SPECT) is expensive and requires additional care for performance with radioactive materials. Like PET, it uses ionizing radiation and creates radiation side effect for the patients [ 2 ].
19. Digital Mammography
Mammography is a technique used to screen and diagnose human breasts. Low-energy X-rays (30 kVp) are used in the mammography in performing early diagnosis and screening of human breast cancer. Ionizing radiations are used in mammograms to create images for analysis of abnormal conditions. Ultrasound is usually employed to give additional information about masses, detected by mammography. MRI, discography, and positron emission mammography (PEM) are also supporters of mammography. Mammography is more accurate for women 50 or above 50 years old as compared to younger women because old women have high breast density [ 85 ]. Today, conventional mammography has been replaced by digital mammography.
An advanced technique is used for creating 3D images of breast tissues for detailed analysis of breast cancer, known as 3D mammography. When 3D mammography is used along with usual mammography, it gives more positive results [ 86 ]. Cost-effectiveness and high radiation exposure are of high concern to 3D mammography [ 87 ].
Digital mammography is a special form of mammography employed to investigate breast tissues for breast tumor study. Digital mammography contrasts with film (conventional) mammography by using a special detector that detects the transmitted X-rays energy and converts it into an image signal by a computer system instead of a film X-ray. Digital mammography is a rapid and advanced modality that has the potential for diagnosis and proper screening of breast cancer. Such new diffusion technologies can alter the health care pattern through many mechanisms. There are different results related to breast health care for digital-screen and film mammography. Digital mammography may also change the application of diagnostic services ensuring mammograms with positive screening [ 88 ].
Digital mammography has been considered a better technique as compared to film mammography in the detection of breast cancer in premenopausal, premenopausal, and young women. A digital system has more cost (approximately 1.5 to 4 times) than a film system. Digital mammography has the advantage of diagnosis in computer-based system that generates images with easy access and better-quality transmission, recovery, and image storage. Advanced digital mammography uses an average low dose of radiation without cooperation with diagnostic accuracy [ 89 ].
A healthy breast tissue mammogram recorded by digital mammography is clear as compared to a mammogram on film as shown in Figure 13 [ 90 ]. Scientists used both digital mammography and film mammography for 42,760 women's breast X-rays. Cancer is almost equally well detected through these techniques. But digital mammography detected 28% more breast cancer in younger women or those under 50, who have dense breast tissues. Digital mammography uses a specific detector that captures the transmitted X-rays and sends the information in the form of energy that converts into an image through a computer system [ 90 ]. Digital mammography screening for breast cancer is not cost-effective, relative to conventional mammography [ 91 ].

(a) A healthy breast tissue mammogram recorded by digital mammogram; (b) the same breast tissue mammogram recorded by film mammography. White spots shown in the above images are deposits of calcium which can consider the mark of cancer when they form clusters.
Now, screening MRI is highly used as an adjunct to mammography, recommended for women to ensure 20-25% or more threat of breast cancer [ 92 ]. Currently, breast cancer diagnostic programs have been recognized widely at least in 22 countries. Collective struggles lead to the formation of the Breast Cancer Screening Network (IBSN), an international program for policymaking, administration, funding, and handling of results that come from breast cancer screening of huge populations [ 93 ]. The ultrasound technology combined with mammography is used to detect elevated risk factors for breast cancer. According to research on elevated risk factors, by using ultrasound with mammography, more than 90% of cancer risk was seen in over 50% of women having dense breast tissues, and 25% of cancer risk factors were determined in women having just 26%-40% dense tissues of the breast. It is suggested that screening with ultrasound may be beneficial for those women having other risk factors and less dense breast tissues [ 94 ].
20. Medical Ultrasound
The ultrasound imaging technology was used earlier as a diagnostic tool for brain images. Today, ultrasound is a widespread imaging technology used in diagnostic laboratories and clinics. It is free from radiation exposure risk, comparatively less expensive, and highly portable as compared to other imaging techniques like MRI and CT [ 95 ]. This system is used in different fields. In the medical field, ultrasound uses sound waves of high frequency, to diagnose the organs and structure of the body. The ultrasound system is performed with high frequency. Special technicians or doctors use it to observe the kidney, heart, liver, blood vessels, and other organs of the body. The most critical component of ultrasound is a transducer. An ultrasound transducer can convert an electrical signal into sound waves and sound waves into an electrical signal. An ultrasonic image or sonogram is formed by transmitting pulses of sound waves into tissues using a special probe. Different tissues reflect these sounds to a different degree. These reflected sound waves (echoes) are detected and presented as an image with the help of the operator. In the medical field, there are many applications of ultrasound. Ultrasound scanning is a very effective and reliable technique that is greatly used for monitoring normal pregnancy, placenta previa, multiple pregnancies, and different abnormalities during pregnancy and rest [ 96 ].
An ultrasound imaging technique also known as transvaginal ultrasound (TVS) alters our understanding of the management and diagnosis of pregnancy. The TVS gives clear knowledge of early pregnancy problems. It investigates the pregnancy location as well as viability. In utero , the TVs have determined the fetal heart activity that is initial proof of pregnancy viability. Abnormal development in fetal heart rate pattern indicates the subsequent miscarriage. Less fetal heart rate especially at 6 to 8 weeks demonstrates subsequent fetal disease. Fetal heart pulsation can be seen on TVS. The routine use of TVS also leads to development in managing early pregnancy failure. Awareness of pregnant women and improvement in early pregnancy units can directly manage miscarriage. TVS is a very sensitive approach for diagnosing early miscarriage. It detects trophoblastic tissue and blood flow in the intervillous space, and the use of color Doppler images leads to estimate the level of expectant management [ 97 ].
Functional ultrasound (fUS) is highly applicable for imaging the brain and detects transient alternation of blood volume in the brain at high resolution than other brain imaging modalities. The blood volume in small vessels can be measured by functional ultrasound (fUS), which uses plane-wave illumination with a high frame rate. Functional ultrasound can detect the brain's active portion [ 98 ]. Ultrasound has major advantages as noninvasive and out-patient scanning in children to investigate neuromuscular disorders. Ultrasonography has been used for finding the normal function of muscle, muscle contraction, muscle thickness, and muscle fiber length. Real-time ultrasound is used for muscle imaging. When ultrasound applies to neuromuscular patients, different muscle disorders can be detected by a pattern of muscle echo, such as a bright spotted pattern of increase in muscle echo obtained in muscular dystrophy patients and a moderate increase in echo showed in spinal muscular atrophy [ 99 ].
Currently, early care physicians can easily understand complex patient conditions with the help of advanced 3D ultrasound algorithms. And high-speed networks are used in special health centers to enhance patient care facilities [ 100 ]. 3D ultrasound is widely used due to the reason of 2D ultrasound limitations. Clinical 3D ultrasound experience has an advantage in the diagnosis of disorders and produces a 3D image that guides invasive therapy. Further improvement in 3D imaging software, as well as hardware, will lead to routine usage of this tool [ 101 ].
High-resolution ultrasound which is also known as ultrasound biomicroscopy (UBM) has clinical applications in imaging the human eye. UBM uses 35 MHz or above frequencies to provide images of high resolution as compared to conventional ophthalmic ultrasound techniques. UBM can be used to diagnose ocular trauma and complex hypotony. It can determine eye lens displacement, iridodialysis, zonular flaw, cataract, lens subluxation, and hyphemia. Hyphemia is a condition in which blood diffuses the anterior chamber of the eye due to injury. Hyphemia scan image by Sonomed UBM is shown in Figure 14 [ 102 ].

(a) Hyphemia is due to injury; blood diffuses the anterior chamber of the eye. (b) Hyphemia scan image by Sonomed UBM.
UBM can detect several eye abnormalities, as it allows for the diagnosis of trauma, glaucoma, and foreign bodies. It can evaluate eyelids, neoplasms, normal eye anatomy, and extraocular muscles during strabismus surgery. Currently, for high-resolution diagnostic eye imaging, new advanced technologies such as pulse encoding, transducer array, and ultrasound combination with light are developed [ 102 ].
A high-frequency (40 MHz) ultrasound imaging technique has been developed for checking the programmed cell death process known as apoptosis. It is a noninvasive procedure used to monitor the apoptosis process that happens because of agents especially anticancer agents in cells of body tissue in vitro or in vivo. The procedure of detection monitored alternations in subcellular nuclear such as the condensation process after proper destruction of the cell during the programmed cell death process. The high-frequency ultrasound technique shows a high scattering rate due to these intense alternations (approximately 25-50-fold) in apoptotic cells as compared to normal tissue cells. As a result, the apoptotic tissues show greatly brighter areas as compared to normal tissue. In the future, this noninvasive imaging technique will use to check the effect of anticancer treatment and chemotherapeutic agents in laboratory model systems and then in patients [ 103 ].
Another application of ultrasound technology is the successful detection of renal masses. According to research results, 86% carcinomas and 98% renal cysts were accurately determined among 111 patients by the ultrasound imaging technique. Ultrasound is a safe, simple, and cheap diagnostic tool to diagnose complex renal masses [ 104 ]. Ultrasound screening allows imaging the cartilage for checking instability, abnormal location of the femoral head inside the acetabulum, and developmental dysplasia in newborns [ 105 ]. A powerful low-frequency ultrasound system can also be used as a noninvasively drug delivery system. Many drugs and proteins having high molecular weight can be delivered easily with excessive permeability into human skin with the help of a low-frequency ultrasound modality. For example, insulin, erythropoietin, and interferon-gamma molecules are easily and safely delivered across human skin [ 106 ].
A novel molecular imaging technique known as molecular ultrasound has been used by researchers in the molecular biology field to monitor the alternation in the expression rate of molecular markers located on intravascular targets. Contrast agents used in the advanced molecular ultrasound imaging technology are mostly micro- or nanosized particles having ligands on the surface also known as microbubbles. Specific molecular markers are targeted by these microbubbles such as selectin, vascular cell adhesion molecule 1, and integrin. In this way, these agents lead to detect specific molecular markers on intravenous targets by gathering at that specific tissue site.
Molecular ultrasound has many advantages such as low-effective cost, high resolution, portable, noninvasiveness, absence of ionizing radiation, real-time imaging potential, and high availability. It has the potential for regular investigations of different abnormalities at the molecular level, such as inflammation, tumor angiogenesis, and thrombus. In addition, improvement is still required in the field of molecular ultrasound to design the novel targeting ligand to form a more effective contrast agent. In the future, advancements in molecular ultrasound imaging technology will play a clinical role with high sensitivity and accuracy for imaging complex abnormalities at the molecular level [ 107 , 108 ].
21. Disadvantages of Medical Ultrasound
There are many useful applications of ultrasound in the medical field. But medical ultrasonography also had some side effects such as hormone change effect, breakage of chromosomes with very low frequency, chemical effects, and other health problems. It was examined that for 10-week gestation, chorionic gonadotropin hormone in humans increased after routine usage of ultrasound modality [ 109 ].
It was observed in an experiment that no chromosome damage occurred after diagnostic ultrasound exposure to human lymphocyte culture. But experimental results suggest that ultrasound can cause chromosome breakage with very low frequency [ 110 ]. Similarly, lots of experiments have been done to check fetal ultrasound safety. In vivo study demonstrates no major neurological defects, fetal growth problems, birth flaws, or childhood cancer caused by ultrasound imaging. But in vitro study demonstrates the possibility of some health problems that can occur due to diagnostic ultrasonography. For example, the effect of diagnostic ultrasound on the normal architecture of the mouse fibroblast cell with the production of fingerlike projections on the fine surface of the cell is demonstrated in Figure 15 [ 111 ].

Images of mouse fibroblast cell (a) normal smooth form and (b) abnormal cell in its rough shape. Due to the diagnostic ultrasound effect, fingerlike projections are produced on the smooth surface of the fibroblast cell.
Another disadvantage of an ultrasound system in the medical field is generating significant heat. When strong ultrasound waves migrate through the liquid environment, small cavities are produced, and these cavities expand, then collapse to each other, and generate heat. This heat-generating condition leads to create an unnecessary chemical environment [ 112 ]. Dyslexia, growth limitation, non-right handedness, and late speech-like effects are also examined after diagnostic ultrasound exposure. Continuous research is required to find the side effects of medical ultrasonography on human health [ 113 ].
22. Radiation Exposure Risk from Medical Imaging and Its Management
Radiological imaging by X-ray radiology, positron emission tomography (PET), single-photon emission computed tomography (SPECT), mammography, and computed tomography (CT) uses high-energy X-rays that leads to high radiation dose in some patients. Pregnant women and children are mostly affected by radiation exposure from radiological imaging. Possibility of cancer, genetic mutations, growth and developmental retardation in the fetus, and cardiovascular abnormalities can occur by the exposure to radiation after radiotherapy. Direct radiation exposure can cause hair loss, cataracts, skin redness, and skin damage. Radiation exposure risks can be reduced by making “National Guidelines” that aid the physician to manage their effects on patients.
Many online tools have been developed to enable physicians or technicians to record the calculation of radiation exposure from each radiological imaging technique. For this purpose, a Thermoluminescent dosimeter (TLD) is used to calculate radiation dose through different software depending on the modality being used such as Monte Carlo PENRADIO which is used for CT [ 114 ]. Magnetic resonance imaging (MRI) and ultrasound techniques are free from ionizing radiation. To minimize the risk, MRI and ultrasound can be used instead of radiological imaging techniques. Reduction in unnecessary computed tomography screening leads to the direct reduction of radiation exposure risks. Today, advanced and safe technologies are used that allow measuring signals with a low dose of radiation; e.g., low-dose computed tomography scanners permit less radiation exposure [ 115 ].
23. Advanced Machine Medical Image Analysis
4D medical imaging analysis is an advanced technology that is used in combination with different modalities such as 4D CT, 4D US, and 4D MRI. 4D CT is an excellent choice for radiation oncology, which is prone to motion artifacts. Similarly, 4D ultrasound is particularly useful in prenatal research. 4D flow MRI can help doctors diagnose and treat heart issues more precisely. For big data integration in medical imaging, researchers need to develop algorithms to store images by converting them into numerical format which will be helpful for physicians in diagnosis.
24. Artificial Intelligence in Medical Imaging
Machine learning and deep learning are branches of artificial intelligence (AI) that solves problems in medical imaging applications such computer-aided diagnosis, lesion segmentation, medical image analysis, image-guided treatment, annotation, and retrieval. AI assesses image quality, interprets image, and analyzes biomarkers, and finally, reporting is done. AI has impact on oncologic imaging. Lung cancer is one of the most prevalent and severe tumors in thoracic imaging. AI can assist in recognizing and classifying these nodules as benign or cancerous [ 116 ]. Machine learning focuses on pattern recognition. The traditional AI systems relied on preset engineering feature algorithms with specified parameters based on expert knowledge. Such features were intended to assess certain radiographic characteristics such as a tumor's 3D shape and intratumoral texture. Following that, a selection process ensures that only the most important features are used. The data is then fed into statistical machine learning models to find potential imaging-based biomarkers [ 117 ]. Deep learning algorithms learn by navigating the data space and by providing them with greater problem-solving capabilities. Convolutional neural networks (CNNs) are the most used deep learning architectural typologies in medical imaging today, even though many deep learning designs have been researched to handle diverse objectives [ 118 ].
25. Critical Analysis
Medical imaging often contains several techniques that are noninvasive to make images of different parts of the body. New imaging techniques such as computed tomography (CT), positron emission computed tomography (PET), magnetic resonance imaging (MRI), single-photon emission computed tomography (SPECT), ultrasound (US), and digital mammography reveal the internal anatomy and physiology of the body [ 2 ].
Advanced imaging technologies are used to diagnose various external as well as internal human illnesses that can also minimize diagnostic errors and produce novel and better information about the target object. There are benefits and risks to every imaging technique. Ultrasound is also employed in the medical field to look at the kidneys, heart, liver, blood vessels, and other organs of the body [ 95 ]. Computed tomography (CT) measures cancer and various abnormalities in the heart, abdomen, bone, and spinal cord with high resolution [ 33 ]. 3D ultrasound computed tomography (3D USCT) is a promising technology for the investigation of breast cancer [ 48 ]. PET is a powerful technique to visualize, characterize, and quantify the biological processes and pathological changes at the cellular and subcellular levels within a living body. The development of MRI is now employed to examine several musculoskeletal, neurologic problems, and cancer. It can be used for both soft and hard tissues [ 1 ]. Digital mammography is a rapid and computer-based modality. It is used for the diagnosis and screening of breast cancer [ 89 ].
Some imaging techniques, such as CT, PET, SPECT, and digital mammography using X-rays, lead to high ionizing radiation exposure risk in some patients. There are some management steps for minimizing the radiation exposure risks from imaging techniques [ 114 ]. The development in medical imaging techniques such as the use of PET/CT hybrid, SPECT/CT hybrid, 3D USCT, and simultaneous PET/MRI leads to an increase in our understanding of diseases and their treatment [ 84 ]. With advanced medical imaging techniques, detection of early-stage diseases is possible and then eventually aids patients to live longer and better lives. In the future, with mounting innovations and advancements in technology systems, the medical diagnostic field would become a field of regular measurement of various complex diseases and will provide healthcare solutions [ 1 ].
Acknowledgments
The authors wish to thank Research Center College of Pharmacy at King Saud University, Riyadh, Saudi Arabia, for their financial support and for providing free access to digital library and laboratory.
Abbreviations
Data availability, conflicts of interest.
The authors declare no conflicts of interest.
Authors' Contributions
All authors contributed equally.

IMAGES
VIDEO
COMMENTS
Medical imaging comprises different imaging modalities and processes to image human body for diagnostic and treatment purposes. It is also used to follow the course of a disease already diagnosed ...
This paper provides a brief review of major milestones in medical imaging from its inception to date, with a few considerations regarding future directions in this important field. ... Robust research initiatives with fMRI and diffusion tensor imaging to study brain and spine nerve fiber tracts are underway. 16, 17 Magnetic resonance ...
Abstract. This paper reviews state-of-the-art research solutions across the spectrum of medical imaging informatics, discusses clinical translation, and provides future directions for advancing clinical practice. More specifically, it summarizes advances in medical imaging acquisition technologies for different modalities, highlighting the ...
The objectives of this paper are to review the history of AI in medical imaging research, the current role, the challenges need to be resolved before AI can be adopted widely in the clinic, and the potential future. ... Issue 5), there were 16/51 papers on deep learning-based imaging research. As we know, imaging research is only one subsection ...
An official journal of the MICCAI Society Medical Image Analysis provides a forum for the dissemination of new research results in the field of medical and biological image analysis, with special emphasis on efforts related to the applications of computer vision, virtual reality and robotics to biomedical imaging problems. The journal publishes the highest quality, original papers that ...
Medical imaging for fetal diseases ... BMC Medical Imaging is an open access journal publishing original peer-reviewed research articles in the development, evaluation, ... 0.983 - SNIP (Source Normalized Impact per Paper) 0.535 - SJR (SCImago Journal Rank) 2023 Speed
Artificial intelligence (AI) is a disruptive technology that involves the use of computerised algorithms to dissect complicated data. Among the most promising clinical applications of AI is diagnostic imaging, and mounting attention is being directed at establishing and fine-tuning its performance to facilitate detection and quantification of a wide array of clinical conditions. Investigations ...
Papers relying on AI and ML report promising results in a wide range of medical ... [71], [72], [73] and it could play an important role in future research directions for medical imaging applications. Self-supervised learning can be seen as a variant of unsupervised learning, in the sense that it works with unlabelled data. ... in the medical ...
Since that point, DL research in medical imaging has increased exponentially [4]. Currently, new investigations with faster, deeper, and more efficient networks are emerging. ... the different architectures, and the most recent implementations in medical imaging. The paper is organized as follows: Section 2 introduces the basic idea behind ...
The image processing techniques were founded in the 1960s. Those techniques were used for different fields such as Space, clinical purposes, arts, and TV image improvement. In the 1970s with the ...
The survey includes research papers on various applications of CNNs in medical image understanding. The papers for the survey are queried from various journal websites. ... Medical imaging is necessary for the visualization of internal organs for the detection of abnormalities in their anatomy or functioning. Medical image capturing devices ...
Medical imaging is a place of origin of the information necessary for clinical decisions. This paper discusses the new algorithms and strategies in the area of deep learning. In this brief introduction to DLA in medical image analysis, there are two objectives. ... The potential future research for medical image analysis is the designing of ...
2.2 Deep learning. DL models enable machines to achieve the accuracy by advancements in techniques to analyze medical images. In [], the heart disease was diagnosed using the labelled chest X-Rays, cardiologist reviewed and relabelled all the data while discarding the data other than heart failure and normal images.To extract the exact features from the images, data augmentation and TL were ...
Designing deep learning based methods with medical images has always been an attractive area of research to assist clinicians in rapid examination and accurate diagnosis. Those methods need a large number of datasets including all variations in their training stages. On the other hand, medical images are always scarce due to several reasons, such as not enough patients for some diseases ...
The immense interest in Transformers has also spurred research into medical imaging applications (see Fig. 1). Being dominant in reputable top-tier medical imaging conferences and journals, it is extremely challenging for researchers and practitioners to keep up with the rate of innovation. ... Paper Organizations. The remaining sections of the ...
This paper is a tutorial review of the medical image processing and repository techniques appeared in the literature. Keywordsbio-instruments-tomography-magnetic resonance-physiologic-anatomy ...
Deep Learning has shown great success in reshaping medical imaging, yet it faces numerous challenges hindering widespread application. Issues like catastrophic forgetting and distribution shifts in the continuously evolving data stream increase the gap between research and applications. Continual Learning offers promise in addressing these hurdles by enabling the sequential acquisition of new ...
We categorized the papers based on anatomical location and modality of medical imaging. We found that most papers focus on chest or brain and on MRI (Fig. 3). This is comparable to what Litjens et al. (2017) found for deep learning methods in medical imaging in general. This trend is likely due to publicly available datasets in these organs and ...
DOI: 10.1016/j.jmir.2024.01.002 Corpus ID: 267579215; Editorial: A reflection on eighty years of the Journal of Medical Imaging and Radiation Sciences. @article{Bolderston2024EditorialAR, title={Editorial: A reflection on eighty years of the Journal of Medical Imaging and Radiation Sciences.}, author={Amanda Bolderston and Carly McCuaig and Lisa Di Prospero and John French}, journal={Journal ...
All data are taken from published research papers and available online. Abstract. Medical imaging is the process of visual representation of different tissues and organs of the human body to monitor the normal and abnormal anatomy and physiology of the body. There are many medical imaging techniques used for this purpose such as X-ray, computed ...
A presumably complete list of papers up to 2017 using deep learning techniques for brain image analysis is provided as Table 1 in Litjens at al. [41]. ... CBIR has been an active area of research in medical imaging for many years, addressing a wide range of applications, imaging modalities, ...