Thank you for visiting nature.com. You are using a browser version with limited support for CSS. To obtain the best experience, we recommend you use a more up to date browser (or turn off compatibility mode in Internet Explorer). In the meantime, to ensure continued support, we are displaying the site without styles and JavaScript.
- View all journals
- Explore content
- About the journal
- Publish with us
- Sign up for alerts
- Open access
- Published: 22 May 2020

Assessing the Big Five personality traits using real-life static facial images
- Alexander Kachur ORCID: orcid.org/0000-0003-1165-2672 1 ,
- Evgeny Osin ORCID: orcid.org/0000-0003-3330-5647 2 ,
- Denis Davydov ORCID: orcid.org/0000-0003-3747-7403 3 ,
- Konstantin Shutilov 4 &
- Alexey Novokshonov 4
Scientific Reports volume 10 , Article number: 8487 ( 2020 ) Cite this article
129k Accesses
47 Citations
349 Altmetric
Metrics details
- Computer science
- Human behaviour
There is ample evidence that morphological and social cues in a human face provide signals of human personality and behaviour. Previous studies have discovered associations between the features of artificial composite facial images and attributions of personality traits by human experts. We present new findings demonstrating the statistically significant prediction of a wider set of personality features (all the Big Five personality traits) for both men and women using real-life static facial images. Volunteer participants (N = 12,447) provided their face photographs (31,367 images) and completed a self-report measure of the Big Five traits. We trained a cascade of artificial neural networks (ANNs) on a large labelled dataset to predict self-reported Big Five scores. The highest correlations between observed and predicted personality scores were found for conscientiousness (0.360 for men and 0.335 for women) and the mean effect size was 0.243, exceeding the results obtained in prior studies using ‘selfies’. The findings strongly support the possibility of predicting multidimensional personality profiles from static facial images using ANNs trained on large labelled datasets. Future research could investigate the relative contribution of morphological features of the face and other characteristics of facial images to predicting personality.
Similar content being viewed by others
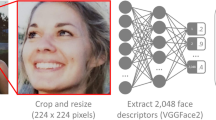
Facial recognition technology can expose political orientation from naturalistic facial images
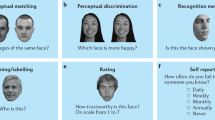
Individual differences and the multidimensional nature of face perception
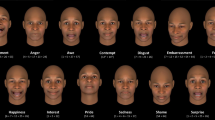
Professional actors demonstrate variability, not stereotypical expressions, when portraying emotional states in photographs
Introduction.
A growing number of studies have linked facial images to personality. It has been established that humans are able to perceive certain personality traits from each other’s faces with some degree of accuracy 1 , 2 , 3 , 4 . In addition to emotional expressions and other nonverbal behaviours conveying information about one’s psychological processes through the face, research has found that valid inferences about personality characteristics can even be made based on static images of the face with a neutral expression 5 , 6 , 7 . These findings suggest that people may use signals from each other’s faces to adjust the ways they communicate, depending on the emotional reactions and perceived personality of the interlocutor. Such signals must be fairly informative and sufficiently repetitive for recipients to take advantage of the information being conveyed 8 .
Studies focusing on the objective characteristics of human faces have found some associations between facial morphology and personality features. For instance, facial symmetry predicts extraversion 9 . Another widely studied indicator is the facial width to height ratio (fWHR), which has been linked to various traits, such as achievement striving 10 , deception 11 , dominance 12 , aggressiveness 13 , 14 , 15 , 16 , and risk-taking 17 . The fWHR can be detected with high reliability irrespective of facial hair. The accuracy of fWHR-based judgements suggests that the human perceptual system may have evolved to be sensitive to static facial features, such as the relative face width 18 .
There are several theoretical reasons to expect associations between facial images and personality. First, genetic background contributes to both face and personality. Genetic correlates of craniofacial characteristics have been discovered both in clinical contexts 19 , 20 and in non-clinical populations 21 . In addition to shaping the face, genes also play a role in the development of various personality traits, such as risky behaviour 22 , 23 , 24 , and the contribution of genes to some traits exceeds the contribution of environmental factors 25 . For the Big Five traits, heritability coefficients reflecting the proportion of variance that can be attributed to genetic factors typically lie in the 0.30–0.60 range 26 , 27 . From an evolutionary perspective, these associations can be expected to have emerged by means of sexual selection. Recent studies have argued that some static facial features, such as the supraorbital region, may have evolved as a means of social communication 28 and that facial attractiveness signalling valuable personality characteristics is associated with mating success 29 .
Second, there is some evidence showing that pre- and postnatal hormones affect both facial shape and personality. For instance, the face is a visible indicator of the levels of sex hormones, such as testosterone and oestrogen, which affect the formation of skull bones and the fWHR 30 , 31 , 32 . Given that prenatal and postnatal sex hormone levels do influence behaviour, facial features may correlate with hormonally driven personality characteristics, such as aggressiveness 33 , competitiveness, and dominance, at least for men 34 , 35 . Thus, in addition to genes, the associations of facial features with behavioural tendencies may also be explained by androgens and potentially other hormones affecting both face and behaviour.
Third, the perception of one’s facial features by oneself and by others influences one’s subsequent behaviour and personality 36 . Just as the perceived ‘cleverness’ of an individual may lead to higher educational attainment 37 , prejudice associated with the shape of one’s face may lead to the development of maladaptive personality characteristics (i.e., the ‘Quasimodo complex’ 38 ). The associations between appearance and personality over the lifespan have been explored in longitudinal observational studies, providing evidence of ‘self-fulfilling prophecy’-type and ‘self-defeating prophecy’-type effects 39 .
Fourth and finally, some personality traits are associated with habitual patterns of emotionally expressive behaviour. Habitual emotional expressions may shape the static features of the face, leading to the formation of wrinkles and/or the development of facial muscles.
Existing studies have revealed the links between objective facial picture cues and general personality traits based on the Five-Factor Model or the Big Five (BF) model of personality 40 . However, a quick glance at the sizes of the effects found in these studies (summarized in Table 1 ) reveals much controversy. The results appear to be inconsistent across studies and hardly replicable 41 . These inconsistencies may result from the use of small samples of stimulus faces, as well as from the vast differences in methodologies. Stronger effect sizes are typically found in studies using composite facial images derived from groups of individuals with high and low scores on each of the Big Five dimensions 6 , 7 , 8 . Naturally, the task of identifying traits using artificial images comprised of contrasting pairs with all other individual features eliminated or held constant appears to be relatively easy. This is in contrast to realistic situations, where faces of individuals reflect a full range of continuous personality characteristics embedded in a variety of individual facial features.
Studies relying on photographic images of individual faces, either artificially manipulated 2 , 42 or realistic, tend to yield more modest effects. It appears that studies using realistic photographs made in controlled conditions (neutral expression, looking straight at the camera, consistent posture, lighting, and distance to the camera, no glasses, no jewellery, no make-up, etc.) produce stronger effects than studies using ‘selfies’ 25 . Unfortunately, differences in the methodologies make it hard to hypothesize whether the diversity of these findings is explained by variance in image quality, image background, or the prediction models used.
Research into the links between facial picture cues and personality traits faces several challenges. First, the number of specific facial features is very large, and some of them are hard to quantify. Second, the effects of isolated facial features are generally weak and only become statistically noticeable in large samples. Third, the associations between objective facial features and personality traits might be interactive and nonlinear. Finally, studies using real-life photographs confront an additional challenge in that the very characteristics of the images (e.g., the angle of the head, facial expression, makeup, hairstyle, facial hair style, etc.) are based on the subjects’ choices, which are potentially influenced by personality; after all, one of the principal reasons why people make and share their photographs is to signal to others what kind of person they are. The task of isolating the contribution of each variable out of the multitude of these individual variables appears to be hardly feasible. Instead, recent studies in the field have tended to rely on a holistic approach, investigating the subjective perception of personality based on integral facial images.
The holistic approach aims to mimic the mechanisms of human perception of the face and the ways in which people make judgements about each other’s personality. This approach is supported by studies of human face perception, showing that faces are perceived and encoded in a holistic manner by the human brain 43 , 44 , 45 , 46 . Put differently, when people identify others, they consider individual facial features (such as a person’s eyes, nose, and mouth) in concert as a single entity rather than as independent pieces of information 47 , 48 , 49 , 50 . Similar to facial identification, personality judgements involve the extraction of invariant facial markers associated with relatively stable characteristics of an individual’s behaviour. Existing evidence suggests that various social judgements might be based on a common visual representational system involving the holistic processing of visual information 51 , 52 . Thus, even though the associations between isolated facial features and personality characteristics sought by ancient physiognomists have emerged to be weak, contradictory or even non-existent, the holistic approach to understanding the face-personality links appears to be more promising.
An additional challenge faced by studies seeking to reveal the face-personality links is constituted by the inconsistency of the evaluations of personality traits by human raters. As a result, a fairly large number of human raters is required to obtain reliable estimates of personality traits for each photograph. In contrast, recent attempts at using machine learning algorithms have suggested that artificial intelligence may outperform individual human raters. For instance, S. Hu and colleagues 40 used the composite partial least squares component approach to analyse dense 3D facial images obtained in controlled conditions and found significant associations with personality traits (stronger for men than for women).
A similar approach can be implemented using advanced machine learning algorithms, such as artificial neural networks (ANNs), which can extract and process significant features in a holistic manner. The recent applications of ANNs to the analysis of human faces, body postures, and behaviours with the purpose of inferring apparent personality traits 53 , 54 indicate that this approach leads to a higher accuracy of prediction compared to individual human raters. The main difficulty of the ANN approach is the need for large labelled training datasets that are difficult to obtain in laboratory settings. However, ANNs do not require high-quality photographs taken in controlled conditions and can potentially be trained using real-life photographs provided that the dataset is large enough. The interpretation of findings in such studies needs to acknowledge that a real-life photograph, especially one chosen by a study participant, can be viewed as a holistic behavioural act, which may potentially contain other cues to the subjects’ personality in addition to static facial features (e.g., lighting, hairstyle, head angle, picture quality, etc.).
The purpose of the current study was to investigate the associations of facial picture cues with self-reported Big Five personality traits by training a cascade of ANNs to predict personality traits from static facial images. The general hypothesis is that a real-life photograph contains cues about personality that can be extracted using machine learning. Due to the vast diversity of findings concerning the prediction accuracy of different traits across previous studies, we did not set a priori hypotheses about differences in prediction accuracy across traits.
Prediction accuracy
We used data from the test dataset containing predicted scores for 3,137 images associated with 1,245 individuals. To determine whether the variance in the predicted scores was associated with differences across images or across individuals, we calculated the intraclass correlation coefficients (ICCs) presented in Table 2 . The between-individual proportion of variance in the predicted scores ranged from 79 to 88% for different traits, indicating a general consistency of predicted scores for different photographs of the same individual. We derived the individual scores used in all subsequent analyses as the simple averages of the predicted scores for all images provided by each participant.
The correlation coefficients between the self-report test scores and the scores predicted by the ANN ranged from 0.14 to 0.36. The associations were strongest for conscientiousness and weakest for openness. Extraversion and neuroticism were significantly better predicted for women than for men (based on the z test). We also compared the prediction accuracy within each gender using Steiger’s test for dependent sample correlation coefficients. For men, conscientiousness was predicted more accurately than the other four traits (the differences among the latter were not statistically significant). For women, conscientiousness was predicted more accurately, and openness was predicted less accurately compared to the three other traits.
The mean absolute error (MAE) of prediction ranged between 0.89 and 1.04 standard deviations. We did not find any associations between the number of photographs and prediction error.
Trait intercorrelations
The structure of the correlations between the scales was generally similar for the observed test scores and the predicted values, but some coefficients differed significantly (based on the z test) (see Table 3 ). Most notably, predicted openness was more strongly associated with conscientiousness (negatively) and extraversion (positively), whereas its association with agreeableness was negative rather than positive. The associations of predicted agreeableness with conscientiousness and neuroticism were stronger than those between the respective observed scores. In women, predicted neuroticism demonstrated a stronger inverse association with conscientiousness and a stronger positive association with openness. In men, predicted neuroticism was less strongly associated with extraversion than its observed counterpart.
To illustrate the findings, we created composite images using Abrosoft FantaMorph 5 by averaging the uploaded images across contrast groups of 100 individuals with the highest and the lowest test scores on each trait. The resulting morphed images in which individual features are eliminated are presented in Fig. 1 .
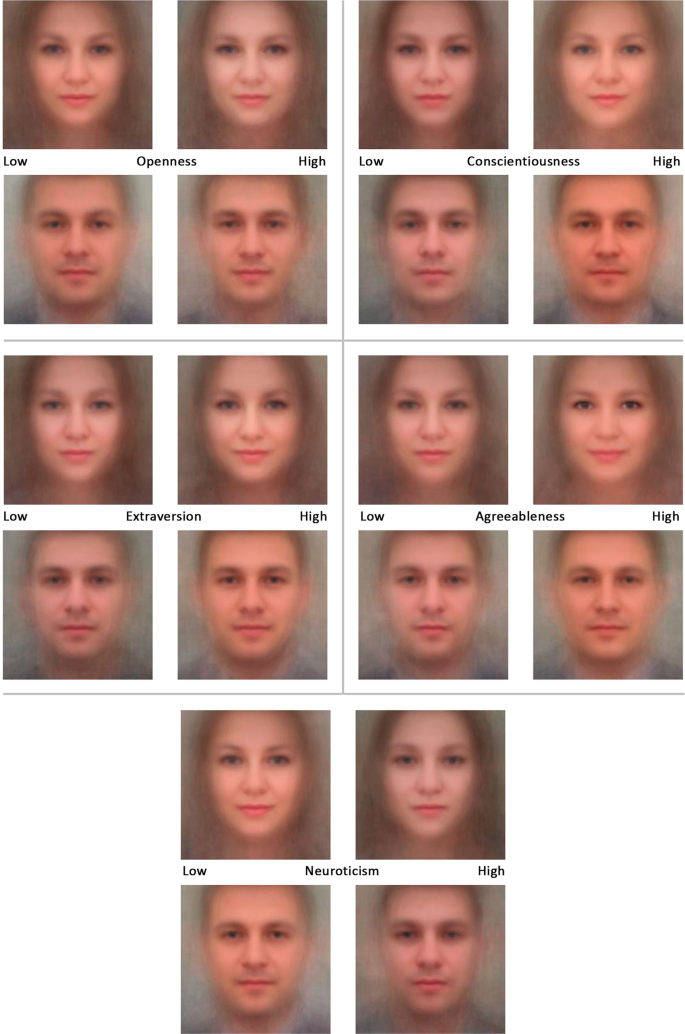
Composite facial images morphed across contrast groups of 100 individuals for each Big Five trait.
This study presents new evidence confirming that human personality is related to individual facial appearance. We expected that machine learning (in our case, artificial neural networks) could reveal multidimensional personality profiles based on static morphological facial features. We circumvented the reliability limitations of human raters by developing a neural network and training it on a large dataset labelled with self-reported Big Five traits.
We expected that personality traits would be reflected in the whole facial image rather than in its isolated features. Based on this expectation, we developed a novel two-tier machine learning algorithm to encode the invariant facial features as a vector in a 128-dimensional space that was used to predict the BF traits by means of a multilayer perceptron. Although studies using real-life photographs do not require strict experimental conditions, we had to undertake a series of additional organizational and technological steps to ensure consistent facial image characteristics and quality.
Our results demonstrate that real-life photographs taken in uncontrolled conditions can be used to predict personality traits using complex computer vision algorithms. This finding is in contrast to previous studies that mostly relied on high-quality facial images taken in controlled settings. The accuracy of prediction that we obtained exceeds that in the findings of prior studies that used realistic individual photographs taken in uncontrolled conditions (e.g., selfies 55 ). The advantage of our methodology is that it is relatively simple (e.g., it does not rely on 3D scanners or 3D facial landmark maps) and can be easily implemented using a desktop computer with a stock graphics accelerator.
In the present study, conscientiousness emerged to be more easily recognizable than the other four traits, which is consistent with some of the existing findings 7 , 40 . The weaker effects for extraversion and neuroticism found in our sample may be because these traits are associated with positive and negative emotional experiences, whereas we only aimed to use images with neutral or close to neutral emotional expressions. Finally, this appears to be the first study to achieve a significant prediction of openness to experience. Predictions of personality based on female faces appeared to be more reliable than those for male faces in our sample, in contrast to some previous studies 40 .
The BF factors are known to be non-orthogonal, and we paid attention to their intercorrelations in our study 56 , 57 . Various models have attempted to explain the BF using higher-order dimensions, such as stability and plasticity 58 or a single general factor of personality (GFP) 59 . We discovered that the intercorrelations of predicted factors tend to be stronger than the intercorrelations of self-report questionnaire scales used to train the model. This finding suggests a potential biological basis of GFP. However, the stronger intercorrelations of the predicted scores can be explained by consistent differences in picture quality (just as the correlations between the self-report scales can be explained by social desirability effects and other varieties of response bias 60 ). Clearly, additional research is needed to understand the context of this finding.
We believe that the present study, which did not involve any subjective human raters, constitutes solid evidence that all the Big Five traits are associated with facial cues that can be extracted using machine learning algorithms. However, despite having taken reasonable organizational and technical steps to exclude the potential confounds and focus on static facial features, we are still unable to claim that morphological features of the face explain all the personality-related image variance captured by the ANNs. Rather, we propose to see facial photographs taken by subjects themselves as complex behavioural acts that can be evaluated holistically and that may contain various other subtle personality cues in addition to static facial features.
The correlations reported above with a mean r = 0.243 can be viewed as modest; indeed, facial image-based personality assessment can hardly replace traditional personality measures. However, this effect size indicates that an ANN can make a correct guess about the relative standing of two randomly chosen individuals on a personality dimension in 58% of cases (as opposed to the 50% expected by chance) 61 . The effect sizes we observed are comparable with the meta-analytic estimates of correlations between self-reported and observer ratings of personality traits: the associations range from 0.30 to 0.49 when one’s personality is rated by close relatives or colleagues, but only from −0.01 to 0.29 when rated by strangers 62 . Thus, an artificial neural network relying on static facial images outperforms an average human rater who meets the target in person without any prior acquaintance. Given that partner personality and match between two personalities predict friendship formation 63 , long-term relationship satisfaction 64 , and the outcomes of dyadic interaction in unstructured settings 65 , the aid of artificial intelligence in making partner choices could help individuals to achieve more satisfying interaction outcomes.
There are a vast number of potential applications to be explored. The recognition of personality from real-life photos can be applied in a wide range of scenarios, complementing the traditional approaches to personality assessment in settings where speed is more important than accuracy. Applications may include suggesting best-fitting products or services to customers, proposing to individuals a best match in dyadic interaction settings (such as business negotiations, online teaching, etc.) or personalizing the human-computer interaction. Given that the practical value of any selection method is proportional to the number of decisions made and the size and variability of the pool of potential choices 66 , we believe that the applied potential of this technology can be easily revealed at a large scale, given its speed and low cost. Because the reliability and validity of self-report personality measures is not perfect, prediction could be further improved by supplementing these measures with peer ratings and objective behavioural indicators of personality traits.
The fact that conscientiousness was predicted better than the other traits for both men and women emerges as an interesting finding. From an evolutionary perspective, one would expect the traits most relevant for cooperation (conscientiousness and agreeableness) and social interaction (certain facets of extraversion and neuroticism, such as sociability, dominance, or hostility) to be reflected more readily in the human face. The results are generally in line with this idea, but they need to be replicated and extended by incorporating trait facets in future studies to provide support for this hypothesis.
Finally, although we tried to control the potential sources of confounds and errors by instructing the participants and by screening the photographs (based on angles, facial expressions, makeup, etc.), the present study is not without limitations. First, the real-life photographs we used could still carry a variety of subtle cues, such as makeup, angle, light facial expressions, and information related to all the other choices people make when they take and share their own photographs. These additional cues could say something about their personality, and the effects of all these variables are inseparable from those of static facial features, making it hard to draw any fundamental conclusions from the findings. However, studies using real-life photographs may have higher ecological validity compared to laboratory studies; our results are more likely to generalize to real-life situations where users of various services are asked to share self-pictures of their choice.
Another limitation pertains to a geographically bounded sample of individuals; our participants were mostly Caucasian and represented one cultural and age group (Russian-speaking adults). Future studies could replicate the effects using populations representing a more diverse variety of ethnic, cultural, and age groups. Studies relying on other sources of personality data (e.g., peer ratings or expert ratings), as well as wider sets of personality traits, could complement and extend the present findings.
Sample and procedure
The study was carried out in the Russian language. The participants were anonymous volunteers recruited through social network advertisements. They did not receive any financial remuneration but were provided with a free report on their Big Five personality traits. The data were collected online using a dedicated research website and a mobile application. The participants provided their informed consent, completed the questionnaires, reported their age and gender and were asked to upload their photographs. They were instructed to take or upload several photographs of their face looking directly at the camera with enough lighting, a neutral facial expression and no other people in the picture and without makeup.
Our goal was to obtain an out-of-sample validation dataset of 616 respondents of each gender to achieve 80% power for a minimum effect we considered to be of practical significance ( r = 0.10 at p < 0.05), requiring a total of 6,160 participants of each gender in the combined dataset comprising the training and validation datasets. However, we aimed to gather more data because we expected that some online respondents might provide low-quality or non-genuine photographs and/or invalid questionnaire responses.
The initial sample included 25,202 participants who completed the questionnaire and uploaded a total of 77,346 photographs. The final combined dataset comprised 12,447 valid questionnaires and 31,367 associated photographs after the data screening procedures (below). The participants ranged in age from 18 to 60 (59.4% women, M = 27.61, SD = 12.73, and 40.6% men, M = 32.60, SD = 11.85). The dataset was split randomly into a training dataset (90%) and a test dataset (10%) used to validate the prediction model. The validation dataset included the responses of 505 men who provided 1224 facial images and 740 women who provided 1913 images. Due to the sexually dimorphic nature of facial features and certain personality traits (particularly extraversion 1 , 67 , 68 ), all the predictive models were trained and validated separately for male and female faces.
Ethical approval
The research was carried out in accordance with the Declaration of Helsinki. The study protocol was approved by the Research Ethics Committee of the Open University for the Humanities and Economics. We obtained the participants’ informed consent to use their data and photographs for research purposes and to publish generalized findings. The morphed group average images presented in the paper do not allow the identification of individuals. No information or images that could lead to the identification of study participants have been published.
Data screening
We excluded incomplete questionnaires (N = 3,035) and used indices of response consistency to screen out random responders 69 . To detect systematic careless responses, we used the modal response category count, maximum longstring (maximum number of identical responses given in sequence by participant), and inter-item standard deviation for each questionnaire. At this stage, we screened out the answers of individuals with zero standard deviations (N = 329) and a maximum longstring above 10 (N = 1,416). To detect random responses, we calculated the following person-fit indices: the person-total response profile correlation, the consistency of response profiles for the first and the second half of the questionnaire, the consistency of response profiles obtained based on equivalent groups of items, the number of polytomous Guttman errors, and the intraclass correlation of item responses within facets.
Next, we conducted a simulation by generating random sets of integers in the 1–5 range based on a normal distribution (µ = 3, σ = 1) and on the uniform distribution and calculating the same person-fit indices. For each distribution, we generated a training dataset and a test dataset, each comprised of 1,000 simulated responses and 1,000 real responses drawn randomly from the sample. Next, we ran a logistic regression model using simulated vs real responses as the outcome variable and chose an optimal cutoff point to minimize the misclassification error (using the R package optcutoff). The sensitivity value was 0.991 for the uniform distribution and 0.960 for the normal distribution, and the specificity values were 0.923 and 0.980, respectively. Finally, we applied the trained model to the full dataset and identified observations predicted as likely to be simulated based on either distribution (N = 1,618). The remaining sample of responses (N = 18,804) was used in the subsequent analyses.
Big Five measure
We used a modified Russian version of the 5PFQ questionnaire 70 , which is a 75-item measure of the Big Five model, with 15 items per trait grouped into five three-item facets. To confirm the structural validity of the questionnaire, we tested an exploratory structural equation (ESEM) model with target rotation in Mplus 8.2. The items were treated as ordered categorical variables using the WLSMV estimator, and facet variance was modelled by introducing correlated uniqueness values for the items comprising each facet.
The theoretical model showed a good fit to the data (χ 2 = 147854.68, df = 2335, p < 0.001; CFI = 0.931; RMSEA = 0.040 [90% CI: 0.040, 0.041]; SRMR = 0.024). All the items showed statistically significant loadings on their theoretically expected scales (λ ranged from 0.14 to 0.87, M = 0.51, SD = 0.17), and the absolute cross-loadings were reasonably low (M = 0.11, SD = 0.11). The distributions of the resulting scales were approximately normal (with skewness and kurtosis values within the [−1; 1] range). To assess the reliability of the scales, we calculated two internal consistency indices, namely, robust omega (using the R package coefficientalpha) and algebraic greatest lower bound (GLB) reliability (using the R package psych) 71 (see Table 4 ).
Image screening and pre-processing
The images (photographs and video frames) were subjected to a three-step screening procedure aimed at removing fake and low-quality images. First, images with no human faces or with more than one human face were detected by our computer vision (CV) algorithms and automatically removed. Second, celebrity images were identified and removed by means of a dedicated neural network trained on a celebrity photo dataset (CelebFaces Attributes Dataset (CelebA), N > 200,000) 72 that was additionally enriched with pictures of Russian celebrities. The model showed a 98.4% detection accuracy. Third, we performed a manual moderation of the remaining images to remove images with partially covered faces, those that were evidently photoshopped or any other fake images not detected by CV.
The images retained for subsequent processing were converted to single-channel 8-bit greyscale format using the OpenCV framework (opencv.org). Head position (pitch, yaw, roll) was measured using our own dedicated neural network (multilayer perceptron) trained on a sample of 8 000 images labelled by our team. The mean absolute error achieved on the test sample of 800 images was 2.78° for roll, 1.67° for pitch, and 2.34° for yaw. We used the head position data to retain the images with yaw and roll within the −30° to 30° range and pitch within the −15° to 15° range.
Next, we assessed emotional neutrality using the Microsoft Cognitive Services API on the Azure platform (score range: 0 to 1) and used 0.50 as a threshold criterion to remove emotionally expressive images. Finally, we applied the face and eye detection, alignment, resize, and crop functions available within the Dlib (dlib.net) open-source toolkit to arrive at a set of standardized 224 × 224 pixel images with eye pupils aligned to a standard position with an accuracy of 1 px. Images with low resolution that contained less than 60 pixels between the eyes, were excluded in the process.
The final photoset comprised 41,835 images. After the screened questionnaire responses and images were joined, we obtained a set of 12,447 valid Big Five questionnaires associated with 31,367 validated images (an average of 2.59 images per person for women and 2.42 for men).
Neural network architecture
First, we developed a computer vision neural network (NNCV) aiming to determine the invariant features of static facial images that distinguish one face from another but remain constant across different images of the same person. We aimed to choose a neural network architecture with a good feature space and resource-efficient learning, considering the limited hardware available to our research team. We chose a residual network architecture based on ResNet 73 (see Fig. 2 ).
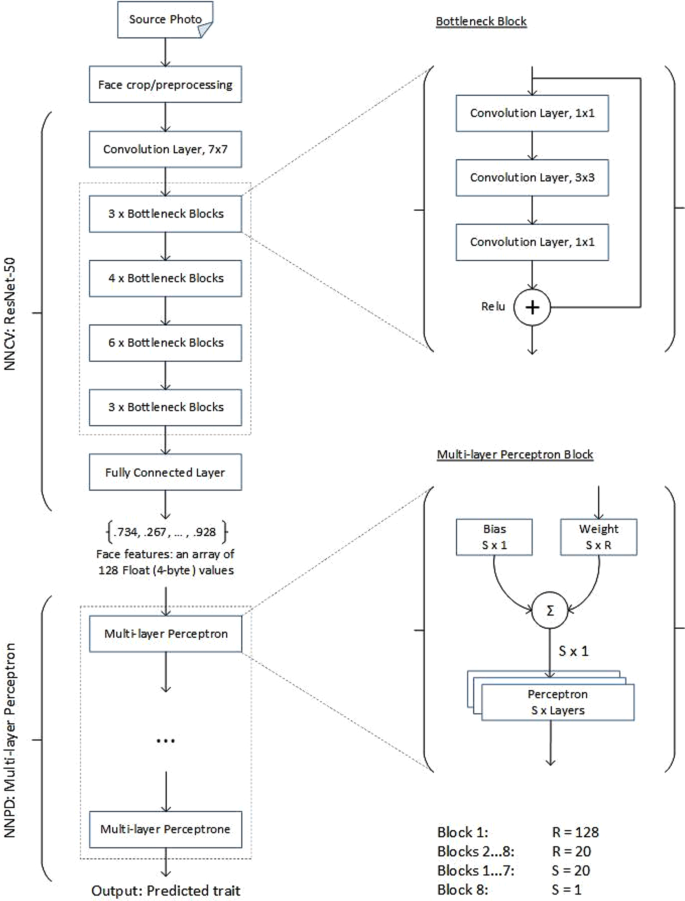
Layer architecture of the computer vision neural network (NNCV) and the personality diagnostics neural network (NNPD).
This type of neural network was originally developed for image classification. We dropped the final layer from the original architecture and obtained a NNCV that takes a static monochrome image (224 × 224 pixels in size) and generates a vector of 128 32-bit dimensions describing unique facial features in the source image. As a measure of success, we calculated the Euclidean distance between the vectors generated from different images.
Using Internet search engines, we collected a training dataset of approximately 2 million openly available unlabelled real-life photos taken in uncontrolled conditions stratified by race, age and gender (using search engine queries such as ‘face photo’, ‘face pictures’, etc.). The training was conducted on a server equipped with four NVidia Titan accelerators. The trained neural network was validated on a dataset of 40,000 images belonging to 800 people, which was an out-of-sample part of the original dataset. The Euclidean distance threshold for the vectors belonging to the same person was 0.40 after the training was complete.
Finally, we trained a personality diagnostics neural network (NNPD), which was implemented as a multilayer perceptron (see Fig. 2 ). For that purpose, we used a training dataset (90% of the final sample) containing the questionnaire scores of 11,202 respondents and a total of 28,230 associated photographs. The NNPD takes the vector of the invariants obtained from NNCV as an input and predicts the Big Five personality traits as the output. The network was trained using the same hardware, and the training process took 9 days. The whole process was performed for male and female faces separately.
Data availability
The set of photographs is not made available because we did not solicit the consent of the study participants to publish the individual photographs. The test dataset with the observed and predicted Big Five scores is available from the openICPSR repository: https://doi.org/10.3886/E109082V1 .
Kramer, R. S. S., King, J. E. & Ward, R. Identifying personality from the static, nonexpressive face in humans and chimpanzees: Evidence of a shared system for signaling personality. Evol. Hum. Behav . https://doi.org/10.1016/j.evolhumbehav.2010.10.005 (2011).
Walker, M. & Vetter, T. Changing the personality of a face: Perceived big two and big five personality factors modeled in real photographs. J. Pers. Soc. Psychol. 110 , 609–624 (2016).
Article Google Scholar
Naumann, L. P., Vazire, S., Rentfrow, P. J. & Gosling, S. D. Personality Judgments Based on Physical Appearance. Personal. Soc. Psychol. Bull. 35 , 1661–1671 (2009).
Borkenau, P., Brecke, S., Möttig, C. & Paelecke, M. Extraversion is accurately perceived after a 50-ms exposure to a face. J. Res. Pers. 43 , 703–706 (2009).
Shevlin, M., Walker, S., Davies, M. N. O., Banyard, P. & Lewis, C. A. Can you judge a book by its cover? Evidence of self-stranger agreement on personality at zero acquaintance. Pers. Individ. Dif . https://doi.org/10.1016/S0191-8869(02)00356-2 (2003).
Penton-Voak, I. S., Pound, N., Little, A. C. & Perrett, D. I. Personality Judgments from Natural and Composite Facial Images: More Evidence For A “Kernel Of Truth” In Social Perception. Soc. Cogn. 24 , 607–640 (2006).
Little, A. C. & Perrett, D. I. Using composite images to assess accuracy in personality attribution to faces. Br. J. Psychol. 98 , 111–126 (2007).
Kramer, R. S. S. & Ward, R. Internal Facial Features are Signals of Personality and Health. Q. J. Exp. Psychol. 63 , 2273–2287 (2010).
Pound, N., Penton-Voak, I. S. & Brown, W. M. Facial symmetry is positively associated with self-reported extraversion. Pers. Individ. Dif. 43 , 1572–1582 (2007).
Lewis, G. J., Lefevre, C. E. & Bates, T. Facial width-to-height ratio predicts achievement drive in US presidents. Pers. Individ. Dif. 52 , 855–857 (2012).
Haselhuhn, M. P. & Wong, E. M. Bad to the bone: facial structure predicts unethical behaviour. Proc. R. Soc. B Biol. Sci. 279 , 571 LP–576 (2012).
Valentine, K. A., Li, N. P., Penke, L. & Perrett, D. I. Judging a Man by the Width of His Face: The Role of Facial Ratios and Dominance in Mate Choice at Speed-Dating Events. Psychol. Sci . 25 , (2014).
Carre, J. M. & McCormick, C. M. In your face: facial metrics predict aggressive behaviour in the laboratory and in varsity and professional hockey players. Proc. R. Soc. B Biol. Sci. 275 , 2651–2656 (2008).
Carré, J. M., McCormick, C. M. & Mondloch, C. J. Facial structure is a reliable cue of aggressive behavior: Research report. Psychol. Sci . https://doi.org/10.1111/j.1467-9280.2009.02423.x (2009).
Haselhuhn, M. P., Ormiston, M. E. & Wong, E. M. Men’s Facial Width-to-Height Ratio Predicts Aggression: A Meta-Analysis. PLoS One 10 , e0122637 (2015).
Lefevre, C. E., Etchells, P. J., Howell, E. C., Clark, A. P. & Penton-Voak, I. S. Facial width-to-height ratio predicts self-reported dominance and aggression in males and females, but a measure of masculinity does not. Biol. Lett . 10 , (2014).
Welker, K. M., Goetz, S. M. M. & Carré, J. M. Perceived and experimentally manipulated status moderates the relationship between facial structure and risk-taking. Evol. Hum. Behav . https://doi.org/10.1016/j.evolhumbehav.2015.03.006 (2015).
Geniole, S. N. & McCormick, C. M. Facing our ancestors: judgements of aggression are consistent and related to the facial width-to-height ratio in men irrespective of beards. Evol. Hum. Behav. 36 , 279–285 (2015).
Valentine, M. et al . Computer-Aided Recognition of Facial Attributes for Fetal Alcohol Spectrum Disorders. Pediatrics 140 , (2017).
Ferry, Q. et al . Diagnostically relevant facial gestalt information from ordinary photos. Elife 1–22 https://doi.org/10.7554/eLife.02020.001 (2014).
Claes, P. et al . Modeling 3D Facial Shape from DNA. PLoS Genet. 10 , e1004224 (2014).
Carpenter, J. P., Garcia, J. R. & Lum, J. K. Dopamine receptor genes predict risk preferences, time preferences, and related economic choices. J. Risk Uncertain. 42 , 233–261 (2011).
Dreber, A. et al . The 7R polymorphism in the dopamine receptor D4 gene (<em>DRD4</em>) is associated with financial risk taking in men. Evol. Hum. Behav. 30 , 85–92 (2009).
Bouchard, T. J. et al . Sources of human psychological differences: the Minnesota Study of Twins Reared Apart. Science (80-.). 250 , 223 LP–228 (1990).
Article ADS Google Scholar
Livesley, W. J., Jang, K. L. & Vernon, P. A. Phenotypic and genetic structure of traits delineating personality disorder. Arch. Gen. Psychiatry https://doi.org/10.1001/archpsyc.55.10.941 (1998).
Bouchard, T. J. & Loehlin, J. C. Genes, evolution, and personality. Behavior Genetics https://doi.org/10.1023/A:1012294324713 (2001).
Vukasović, T. & Bratko, D. Heritability of personality: A meta-analysis of behavior genetic studies. Psychol. Bull. 141 , 769–785 (2015).
Godinho, R. M., Spikins, P. & O’Higgins, P. Supraorbital morphology and social dynamics in human evolution. Nat. Ecol. Evol . https://doi.org/10.1038/s41559-018-0528-0 (2018).
Rhodes, G., Simmons, L. W. & Peters, M. Attractiveness and sexual behavior: Does attractiveness enhance mating success? Evol. Hum. Behav . https://doi.org/10.1016/j.evolhumbehav.2004.08.014 (2005).
Lefevre, C. E., Lewis, G. J., Perrett, D. I. & Penke, L. Telling facial metrics: Facial width is associated with testosterone levels in men. Evol. Hum. Behav. 34 , 273–279 (2013).
Whitehouse, A. J. O. et al . Prenatal testosterone exposure is related to sexually dimorphic facial morphology in adulthood. Proceedings. Biol. Sci. 282 , 20151351 (2015).
Penton-Voak, I. S. & Chen, J. Y. High salivary testosterone is linked to masculine male facial appearance in humans. Evol. Hum. Behav . https://doi.org/10.1016/j.evolhumbehav.2004.04.003 (2004).
Carré, J. M. & Archer, J. Testosterone and human behavior: the role of individual and contextual variables. Curr. Opin. Psychol. 19 , 149–153 (2018).
Swaddle, J. P. & Reierson, G. W. Testosterone increases perceived dominance but not attractiveness in human males. Proc. R. Soc. B Biol. Sci . https://doi.org/10.1098/rspb.2002.2165 (2002).
Eisenegger, C., Kumsta, R., Naef, M., Gromoll, J. & Heinrichs, M. Testosterone and androgen receptor gene polymorphism are associated with confidence and competitiveness in men. Horm. Behav. 92 , 93–102 (2017).
Article CAS Google Scholar
Kaplan, H. B. Social Psychology of Self-Referent Behavior . https://doi.org/10.1007/978-1-4899-2233-5 . (Springer US, 1986).
Rosenthal, R. & Jacobson, L. Pygmalion in the classroom. Urban Rev . https://doi.org/10.1007/BF02322211 (1968).
Masters, F. W. & Greaves, D. C. The Quasimodo complex. Br. J. Plast. Surg . 204–210 (1967).
Zebrowitz, L. A., Collins, M. A. & Dutta, R. The Relationship between Appearance and Personality Across the Life Span. Personal. Soc. Psychol. Bull. 24 , 736–749 (1998).
Hu, S. et al . Signatures of personality on dense 3D facial images. Sci. Rep. 7 , 73 (2017).
Kosinski, M. Facial Width-to-Height Ratio Does Not Predict Self-Reported Behavioral Tendencies. Psychol. Sci. 28 , 1675–1682 (2017).
Walker, M., Schönborn, S., Greifeneder, R. & Vetter, T. The basel face database: A validated set of photographs reflecting systematic differences in big two and big five personality dimensions. PLoS One 13 , (2018).
Goffaux, V. & Rossion, B. Faces are ‘spatial’ - Holistic face perception is supported by low spatial frequencies. J. Exp. Psychol. Hum. Percept. Perform . https://doi.org/10.1037/0096-1523.32.4.1023 (2006).
Schiltz, C. & Rossion, B. Faces are represented holistically in the human occipito-temporal cortex. Neuroimage https://doi.org/10.1016/j.neuroimage.2006.05.037 (2006).
Van Belle, G., De Graef, P., Verfaillie, K., Busigny, T. & Rossion, B. Whole not hole: Expert face recognition requires holistic perception. Neuropsychologia https://doi.org/10.1016/j.neuropsychologia.2010.04.034 (2010).
Quadflieg, S., Todorov, A., Laguesse, R. & Rossion, B. Normal face-based judgements of social characteristics despite severely impaired holistic face processing. Vis. cogn. 20 , 865–882 (2012).
McKone, E. Isolating the Special Component of Face Recognition: Peripheral Identification and a Mooney Face. J. Exp. Psychol. Learn. Mem. Cogn . https://doi.org/10.1037/0278-7393.30.1.181 (2004).
Sergent, J. An investigation into component and configural processes underlying face perception. Br. J. Psychol . https://doi.org/10.1111/j.2044-8295.1984.tb01895.x (1984).
Tanaka, J. W. & Farah, M. J. Parts and Wholes in Face Recognition. Q. J. Exp. Psychol. Sect. A https://doi.org/10.1080/14640749308401045 (1993).
Young, A. W., Hellawell, D. & Hay, D. C. Configurational information in face perception. Perception https://doi.org/10.1068/p160747n (2013).
Calder, A. J. & Young, A. W. Understanding the recognition of facial identity and facial expression. Nature Reviews Neuroscience https://doi.org/10.1038/nrn1724 (2005).
Todorov, A., Loehr, V. & Oosterhof, N. N. The obligatory nature of holistic processing of faces in social judgments. Perception https://doi.org/10.1068/p6501 (2010).
Junior, J. C. S. J. et al . First Impressions: A Survey on Computer Vision-Based Apparent Personality Trait Analysis. (2018).
Wang, Y. & Kosinski, M. Deep neural networks are more accurate than humans at detecting sexual orientation from facial images. J. Pers. Soc. Psychol. 114 , 246–257 (2018).
Qiu, L., Lu, J., Yang, S., Qu, W. & Zhu, T. What does your selfie say about you? Comput. Human Behav. 52 , 443–449 (2015).
Digman, J. M. Higher order factors of the Big Five. J.Pers.Soc.Psychol . https://doi.org/10.1037/0022-3514.73.6.1246 (1997).
Musek, J. A general factor of personality: Evidence for the Big One in the five-factor model. J. Res. Pers . https://doi.org/10.1016/j.jrp.2007.02.003 (2007).
DeYoung, C. G. Higher-order factors of the Big Five in a multi-informant sample. J. Pers. Soc. Psychol. 91 , 1138–1151 (2006).
Rushton, J. P. & Irwing, P. A General Factor of Personality (GFP) from two meta-analyses of the Big Five: Digman (1997) and Mount, Barrick, Scullen, and Rounds (2005). Pers. Individ. Dif. 45 , 679–683 (2008).
Wood, D., Gardner, M. H. & Harms, P. D. How functionalist and process approaches to behavior can explain trait covariation. Psychol. Rev. 122 , 84–111 (2015).
Dunlap, W. P. Generalizing the Common Language Effect Size indicator to bivariate normal correlations. Psych. Bull. 116 , 509–511 (1994).
Connolly, J. J., Kavanagh, E. J. & Viswesvaran, C. The convergent validity between self and observer ratings of personality: A meta-analytic review. Int. J. of Selection and Assessment. 15 , 110–117 (2007).
Harris, K. & Vazire, S. On friendship development and the Big Five personality traits. Soc. and Pers. Psychol. Compass. 10 , 647–667 (2016).
Weidmann, R., Schönbrodt, F. D., Ledermann, T. & Grob, A. Concurrent and longitudinal dyadic polynomial regression analyses of Big Five traits and relationship satisfaction: Does similarity matter? J. Res. in Personality. 70 , 6–15 (2017).
Cuperman, R. & Ickes, W. Big Five predictors of behavior and perceptions in initial dyadic interactions: Personality similarity helps extraverts and introverts, but hurts “disagreeables”. J. of Pers. and Soc. Psychol. 97 , 667–684 (2009).
Schmidt, F. L. & Hunter, J. E. The validity and utility of selection methods in personnel psychology: Practical and theoretical implications of 85 years of research findings. Psychol. Bull. 124 , 262–274 (1998).
Brown, M. & Sacco, D. F. Unrestricted sociosexuality predicts preferences for extraverted male faces. Pers. Individ. Dif. 108 , 123–127 (2017).
Lukaszewski, A. W. & Roney, J. R. The origins of extraversion: joint effects of facultative calibration and genetic polymorphism. Pers. Soc. Psychol. Bull. 37 , 409–21 (2011).
Curran, P. G. Methods for the detection of carelessly invalid responses in survey data. J. Exp. Soc. Psychol. 66 , 4–19 (2016).
Khromov, A. B. The five-factor questionnaire of personality [Pjatifaktornyj oprosnik lichnosti]. In Rus. (Kurgan State University, 2000).
Trizano-Hermosilla, I. & Alvarado, J. M. Best alternatives to Cronbach’s alpha reliability in realistic conditions: Congeneric and asymmetrical measurements. Front. Psychol . https://doi.org/10.3389/fpsyg.2016.00769 (2016).
Liu, Z., Luo, P., Wang, X. & Tang, X. Deep Learning Face Attributes in the Wild. in 2015 IEEE International Conference on Computer Vision (ICCV) 3730–3738 https://doi.org/10.1109/ICCV.2015.425 (IEEE, 2015).
He, K., Zhang, X., Ren, S. & Sun, J. Deep Residual Learning for Image Recognition. in 2016 IEEE Conference on Computer Vision and Pattern Recognition (CVPR) 770–778 https://doi.org/10.1109/CVPR.2016.90 (IEEE, 2016).
Download references
Acknowledgements
We appreciate the assistance of Oleg Poznyakov, who organized the data collection, and we are grateful to the anonymous peer reviewers for their detailed and insightful feedback.
Contributions
A.K., E.O., D.D. and A.N. designed the study. K.S. and A.K. designed the ML algorithms and trained the ANN. A.N. contributed to the data collection. A.K., K.S. and D.D. contributed to data pre-processing. E.O., D.D. and A.K. analysed the data, contributed to the main body of the manuscript, and revised the text. A.K. prepared Figs. 1 and 2. All the authors contributed to the final version of the manuscript.
Corresponding authors
Correspondence to Alexander Kachur or Evgeny Osin .
Ethics declarations
Competing interests.
A.K., K.S. and A.N. were employed by the company that provided the datasets for the research. E.O. and D.D. declare no competing interests.
Additional information
Publisher’s note Springer Nature remains neutral with regard to jurisdictional claims in published maps and institutional affiliations.
Supplementary information
Supplementary information., rights and permissions.
Open Access This article is licensed under a Creative Commons Attribution 4.0 International License, which permits use, sharing, adaptation, distribution and reproduction in any medium or format, as long as you give appropriate credit to the original author(s) and the source, provide a link to the Creative Commons license, and indicate if changes were made. The images or other third party material in this article are included in the article’s Creative Commons license, unless indicated otherwise in a credit line to the material. If material is not included in the article’s Creative Commons license and your intended use is not permitted by statutory regulation or exceeds the permitted use, you will need to obtain permission directly from the copyright holder. To view a copy of this license, visit http://creativecommons.org/licenses/by/4.0/ .
Reprints and permissions
About this article
Cite this article.
Kachur, A., Osin, E., Davydov, D. et al. Assessing the Big Five personality traits using real-life static facial images. Sci Rep 10 , 8487 (2020). https://doi.org/10.1038/s41598-020-65358-6
Download citation
Received : 12 April 2019
Accepted : 28 April 2020
Published : 22 May 2020
DOI : https://doi.org/10.1038/s41598-020-65358-6
Share this article
Anyone you share the following link with will be able to read this content:
Sorry, a shareable link is not currently available for this article.
Provided by the Springer Nature SharedIt content-sharing initiative
This article is cited by
Personality of organizational social media accounts and its relationship with characteristics of their photos: analyses of startups’ instagram photos.
- Yunhwan Kim
BMC Psychology (2024)
Seeing the darkness: identifying the Dark Triad from emotionally neutral faces
- Danielle Haroun
- Yaarit Amram
- Joseph Glicksohn
Current Psychology (2024)
A Richer Vocabulary of Chinese Personality Traits: Leveraging Word Embedding Technology for Mining Personality Descriptors
- Yigang Ding
- Feijun Zheng
Journal of Psycholinguistic Research (2024)
Unravelling the many facets of human cooperation in an experimental study
- Victoria V. Rostovtseva
- Mikael Puurtinen
- Franz J. Weissing
Scientific Reports (2023)
Using deep learning to predict ideology from facial photographs: expressions, beauty, and extra-facial information
- Stig Hebbelstrup Rye Rasmussen
- Steven G. Ludeke
- Robert Klemmensen
By submitting a comment you agree to abide by our Terms and Community Guidelines . If you find something abusive or that does not comply with our terms or guidelines please flag it as inappropriate.
Quick links
- Explore articles by subject
- Guide to authors
- Editorial policies
Sign up for the Nature Briefing: AI and Robotics newsletter — what matters in AI and robotics research, free to your inbox weekly.

Applications of Personality Assessment to the Workplace: A Review
- Published: September 1999
- Volume 13 , pages 291–322, ( 1999 )
Cite this article
- Leonard D. Goodstein 1 &
- Richard I. Lanyon 2
4266 Accesses
58 Citations
7 Altmetric
Explore all metrics
Our review of the relevant research literature in the recent past strongly supports the view that personality assessment has high utility in the workplace. We review the evidence that personality assessment measures, especially those based upon the Big Five factors of personality, can effectively predict job performance and thus can be used for personnel selection. The validity of integrity testing in predicting counterproductivity on the job has been demonstrated both for overt measures of integrity and, to a lesser degree, for more general (subtle) measures of personality. We also found good evidence of validity for measures of ( supervisory ) management and ( transformational ) leadership , a complex field which includes a number of multidimensional instruments each built around its own theory. Finally, we review the empirical literature that supports the use of assessment centers and the use of personality assessment in training and development.
This is a preview of subscription content, log in via an institution to check access.
Access this article
Price includes VAT (Russian Federation)
Instant access to the full article PDF.
Rent this article via DeepDyve
Institutional subscriptions
Similar content being viewed by others
Improving Selection: Advances in the Belgian Defence Forces
The Role of Personality in Workplace Bullying Research
Association of Personnel Test Publishers (1991). Model guidelines for pre-employment integrity testing programs . Washington, D. C.: Author.
Google Scholar
Barrick, M. R., & Mount, M. K. (1991). The Big Five personality dimensions and job performance: A meta-analysis. Personnel Psychology , 44 , 1–26.
Barrick, M. R., & Mount, M. K. (1993). Autonomy as a moderator of the relationships between the Big Five personality dimensions and job performance. Journal of Applied Psychology , 78 , 111–118.
Barrick, M. R., & Mount, M. K. (1996). Effects of impression management and self-deception on the predictive validity of personality constructs. Journal of Applied Psychology , 81 , 261–272.
Barrick, M. R., Mount, M. K., & Strauss, J. P. (1993). Conscientiousness and performance of sales representatives: Test of the mediating effects of goal setting. Journal of Applied Psychology , 78 , 715–722.
Bass, B. M. (1985). Leadership beyond expectations . New York: Free Press.
Bass, B. M. (1990). Bass and Stogdill's handbook of leadership (3rd ed.). New York: Free Press.
Bass, B. M., & Avolio, B. J. (1990). Transformational leadership development: Manual for the Multifactor leadership questionnaire . Palo Alto, CA: Consulting Psychologists Press.
Bentz, V. J. (1990). Contextual issues in predicting high-level leadership performance. In K. E. Clark & M. B. Clark (Eds.), Measures of leadership . West Orange, NJ: Leadership Library of America.
Bray, D. W., & Byham, W. C. (1991). Assessment centers and their derivatives. Journal of Continuing Higher Education , 39 , 8–11.
Bray, D. W., & Howard, A. (1983). Personality and the assessment center method. In C. D. Spielberger and J. N. Butcher (Eds.), Advances in personality assessment , Vol. 3. Hillsdale, NJ: Erlbaum.
Burke, W. W. (1994). Leadership Assessment Inventory (rev. ed.). Pelham, NY: W. Warner Burke and Associates.
Burns, J. M. (1978). Leadership . New York: Harper & Brothers.
Campbell, D.P. (1991). Manual for the Campbell Leadership Index . Minneapolis: National Computer Systems.
Campbell, D. T., & Fiske, D. W. (1959). Convergent and discriminant validation by the multitrait-multimethod matrix. Psychological Bulletin , 56 , 81–105.
Cascio, W. F. (1995). Whither industrial and organizational psychology in a changing world of work. American Psychologist , 50 , 928–939.
Cattell, R. B. (1945). The principal trait clusters for describing personality. Psychological Bulletin , 42 , 129–169.
Clark, K. E., & Clark, M. B. (Eds.) (1990). Measures of leadership . West Orange, NJ: Leadership Library of America.
Conoley, J. C., & Kramer, J. J. (Eds.) (1989). Tenth mental measurements yearbook . Lincoln, NE: Buros Institute of Mental Measurements, University of Nebraska.
Costa, P., & McCrae, R. R. (1992a). Four ways five factors are basic. Personality and Individual Differences , 13 , 653–665.
Costa, P., & McCrae, R. (1992b). NEO PI-R professional manual . Odessa, FL: Psychological Assessment Resources.
Cunningham, M. R., Wong, D. T., & Barbee, A. P. (1994). Self-presentation dynamics on overt integrity tests: Experimental studies of the Reid Report. Journal of Applied Psychology , 79 , 643–658.
De Rand, B., & Van Heck, G. L. (1994). The fifth of the big five. [Special issue]. European Journal of Psychology , 8 (4).
Digman, J. M. (1990). Personality structure: The emergence of the five-factor model. Annual Review of Psychology , 41 , 417–440.
Eysenck, H. J. (1947). Dimensions of personality . London: Routledge & Kegan Paul.
Fiedler, F. E. (1967). A theory of leadership effectiveness . New York: McGraw-Hill.
Fielder, F. E. (1986). The contribution of cognitive resources to leadership performance. Journal of Applied Social Psychology , 16 , 532–548.
Fiske, D. W. (1949). Consistency of the factorial structures of personality ratings from different sources. Journal of Personality and Social Psychology , 44 , 329–344.
Fleenor, J. W. (1996). Constructs and development assessment centers: Further troubling empirical findings. Journal of Business and Psychology , 10 , 319–335.
Fromm, E. (1947). Man for himself . New York: Holt, Rinehart, and Winston.
Gaugler, B. B., Rosenthal, D. B., Thornton, G. C., & Bentson, C. (1987). Meta-analysis of assessment center validity. Journal of Applied Psychology , 72 , 493–511.
Gaugler, B. B., & Thornton, G. C. (1989). Number of assessment center dimensions as a determinant of assessor accuracy. Journal of Applied Psychology , 74 , 611–618.
Goldberg, L. R. (1981). Language and individual differences: The search for universals in personality lexicons. In L. Wheeler (Ed.), Review of personality and social psychology , Vol 2 , Beverly Hills, CA: Sage Publications.
Goldberg, L. R. (1982). From Ace to Zombie: Some explorations in the language of personality. In C. D. Spielberger & J. N. Butcher (Eds.). Advances in. personality assessment , Vol. 1 . Hillsdale, NJ: Erlbaum.
Goldberg, L. R. (1992). The development of markers for the Big Five factor structure. Psychological Assessment , 4, 26–42.
Goldberg, L. R. (1993). The structure of phenotypic personality traits. American Psychologist , 48, 26–34.
Goldberg, L. R., Grenier, J. R., Guion, R. M., Sechrest, L. B., & Wing, H. (1991). Questionnaires used in the prediction of trustworthiness in pre-employment selection decisions: An APA task force report . Washington, D.C.: American Psychological Association.
Goodstein, L. D., & Schrader, W. J. (1963). An empirically-derived managerial key for the California Psychological Inventory. Journal of Applied Psychology , 47 , 42–45.
Gough, H. G. (1972). Manual for the Personnel Reaction Blank , Palo Alto, CA: Consulting Psychologists Press.
Gough, H. G. (1984). A managerial potential scale for the California Psychological Inventory. Journal of Applied Psychology , 69 , 233–240.
Gough, H. G. (1990). Testing for leadership with the California Psychological Inventory. In K. E. Clark and M. B. Clark (Eds.), Measures of leadership . West Orange, NJ: Leadership Library of America.
Guastello, S. J., & Rieke, M. L. (1991). A review and critique of honesty testing research. Behavioral Sciences and the Law , 9 , 501–523.
Guilford, J. P. (1948). The principal trait clusters for describing personality. Psychological Bulletin , 42 , 129–169.
Guion, R. M., & Gottier, R. F. (1965). The validity of personality measures in personnel selection. Personnel Psychology , 18 , 135–164.
Hersey, P., & Blanchard, K. H. (1988). Management of organizational behavior (5th ed.). Englewood Cliffs, NJ: Prentice-Hall.
Hogan, R., Curphy, G. J., & Hogan, J. (1994). What we know about leadership: Effectiveness and personality. American Psychologist , 49 , 493–504.
Hogan, R., Hogan, J. & Roberts, B. W. (1996). Personality measurement and employment decisions: Questions and answers. American Psychologist , 51, 469–577.
Hollinger, R., & Clark, J. (1983). Theft by employees . Lexington, MA: Lexington Books.
Hough, L. H., Eaton, N. K., Dunnette, M. D., Kamp, J. D., & McCloy, R. A. (1990). Criterion-related validities of personality constructs and the effects of response distortions on those validities. Journal of Applied Psychology , 75 , 581–595.
Howard, A., and Bray, D. W. (1988). Managerial lives in transition: Advancing age and changing times . New York: Guilford.
John, O. P., Goldberg, L. R., & Angleitner, A. (1984). Better than the alphabet: Taxonomies of personality-descriptive terms in English, Dutch, and German. In H. C. J. Bonarius, G. L. M. Van Heck, and N. G. Smid (Eds.), Personality psychology in Europe: Theoretical and empirical developments , Vol. 1 . Lisse: Swets & Zeitlinger.
Jones, J. W. (1991). Personnel selection and corporate financial performance . Paper presented at the 1991 Society for Human Resource Management Conference, Cincinnati, Ohio.
Jones, J. W., & Terris, W. (1991). Integrity testing for personnel selection: An overview. Forensic Reports , 4 , 117–140.
Joyce, L., Thayer, P., & Pond, S. (1994). Managerial functions: An alternative to traditional assessment center dimensions. Personnel Psychology , 47 , 109–121.
Kouzes, J. M., & Posner, B. Z. (1987). The leadership challenge: How to get extraordinary things done in organizations . San Francisco: Jossey-Bass.
Kouzes, J. M., & Posner, B. Z. (1988). The leadership practices inventory . San Diego, CA: Pfeiffer & Co.
Lang, V. R., & Krug, S. E. (1983). Perspectives on the executive personality: A manual for the Executive Profile Survey . Champaign, IL: Institute for Personality and Ability Testing.
Lanyon, R. I., & Goodstein, L. D. (1997). Personality assessment , (3rd. ed.) New York: Wiley.
London House Press (1980). Employee Attitude Inventory . Park Ridge, IL: Author.
Lykken, D. T. (1981). A tremor in the blood . New York: McGraw-Hill.
Matarazzo, J. D. (1995). Psychological testing and assessment in the 21st Century. American Psychologist , 47 , 1007–1018.
McCrae, R. R. (1996). Social consequences of experiential openness. Psychological Bulletin , 120 ,323–337.
McCrae, R. R., & Costa, P. T., Jr. (1987). Validation of the five-factor model of personality across instruments and observers. Journal of Personality and Social Psychology , 52 , 81–90.
McCrae, R. R., & Costa, P. T., Jr. (1990). Personality and adulthood: Emerging lives, enduring dispositions . New York: Guilford.
McCrae, R. R., & Costa, P. T., Jr. (1994). The stability of personality: Observations and evaluations. Current Directions in Psychological Science , 3 , 173–175.
McDaniel, M. A., & Jones, J. W. (1988). A meta-analysis of the Employee Attitude Inventory theft scales. Journal of Business and Psychology , 2 , 327–345.
Morris, C. (1956). Varieties of human values . Chicago: University of Chicago Press.
Mount, M. K., Barrick, M. R., & Strauss, J. P. (1994). Validity of observer ratings of Big Five personality factors. Journal of Applied Psychology , 79 , 272–280.
Murphy, K. R. (1993). Honesty in the workplace . Pacific Grove, CA: Brooks/Cole.
Norman, W. T. (1963). Toward an adequate taxonomy of personality attributes: Replicated factor structure in peer nomination personality ratings. Journal of Abnormal and Social Psychology , 66 , 547–583.
O'Bannon, A. M., Goldinger, L. A., & Appleby, G. S. (1989). Honesty and integrity testing: A practical guide . Atlanta, GA. Applied Information Resources.
Ones, D. S., Schmidt, F. L., Viswesvaran, C., and Lykken, D. T. (1996). Controversies about integrity testing: Two view points. Journal of Business and Psychology , 10 , 487–501.
Ones, D. S., Viswesvaran, C., & Schmidt, F. L. (1993). Comprehensive meta-analysis of integrity test validities: Findings and implications for personnel selection and theories of job performance. Journal of Applied Psychology , 78 , 679–703.
Ones, D. S., Viswesaran, C., & Schmidt, F. L. (1995). Integrity tests: Overlooked facts, resolved issues, and remaining questions. American Psychologist , 50 , 456–457.
Posner, B. Z., & Kouzes, J. M. (1992). Psychometric properties of the Leadership Practices Inventory . San Diego, CA: Pfeiffer & Co.
Reed, H. (1982). The Stanton Survey: Description and validation manual . Chicago: Stanton Corporation.
Reid Psychological Systems (1951). Reid Report . Chicago: Reid Psychological Systems.
Reisman, D. (1950). The lonely crowd . New Haven: Yale University Press.
Ryan, A. M., & Sackett, P. R. (1987). Pre-employment honesty testing: Fakability, reactions to test takers, and company image. Journal of Business and Psychology , 1 , 248–256.
Ryan, A. M., Daum, D., Bauman, T., Grisez, M., Mattimore, K., Naloda, T., and McCormick, S. (1995). Direct, indirect, and controlled observations and rating accuracy. Journal of Applied Psychology , 80 , 664–670.
Sackett, P. R., Burris, L. R., & Callahan, C. (1989). Integrity testing for personnel selection: An update. Personnel Psychology , 42 , 491–529.
Sackett, P. R., & Harris, M. M. (1985). Honesty testing for personnel selection: A review and critique. In H. J. Bernadin & D. A. Bownes (Eds.), Personnel assessment in organizations . New York: Praeger.
Sashkin, M. (1996). The visionary leader: Leadership behavior questionnaire trainer's guide . Amherst, MA: HRD Press.
Sackett, P. R., & Dreher, G. (1982). Constructs and assessment center dimensions: Some troubling empirical findings. Journal of Applied Psychology , 67 , 401–410.
Sackett, P. R., & Harris, M. M. (1984). Honesty testing for personnel selection: A review and critique. Personnel Psychology , 37, 221–245.
Sashkin, M., & Burke, W. W. (1990). Understanding and assessing organizational leadership. In K. E. Clark & M. B. Clark (Eds.), Measures of leadership . West Orange, NJ: Leadership Library of America.
Schmitt, N. Gooding, R. Z., Noe, R. A., & Kirsch, M. (1984). Meta-analyses of validity studies published between 1964 and 1982 and the investigation of study characteristics. Personnel Psychology , 37 , 407–421.
Science Research Associates (1983). Personal Outlook Inventory . Park Ridge, IL: Author.
Slora, K. (1989). An empirical approach to determining employee deviance base rates. Journal of Business and Psychology , 4 , 199–219.
Sparks, C. P. (1990). Testing for management potential. In K. E. Clark & M. B. Clark (Eds.), Measures of leadership . West Orange, NJ: Library of America.
Stogdill, R. M. (1963). Manual for the Leader Behavior Description Questionnaire. Form XII . Columbus, OH: Ohio State University, Bureau of Business Research.
Tett, R. P., Jackson, D. N., & Rothstein, M. (1991). Personality measures as predictors of job performance. Personnel Psychology, 44 703–742.
Thornton, G. C. (1992). Assessment centers in human resource management . Reading, MA: Addison-Wesley.
Thurstone, L. L. (1934). The vectors of the mind. Psychological Review , 41 , 1–32.
Tupes, E. C., & Christal, R. E. (1958). Stability of personality trait rating factors obtained under diverse conditions. USAF WADC Technical Note , No. 58–61.
U. S. Congress, Office of Technology Assessment (1990). The use of integrity tests for pre-employment screening (OTA-SET-442). Washington, DC: US Government Printing Office.
Van Eron, A. M., & Burke, W. W. (1992). The transformational/transactional leadership model: A study of critical components. In K. E. Clark & M. B. Clark (Eds.), Impact of leadership . Greensboro, NC: Center for Creative Leadership.
Wiggins, J. S., & Pincus, A. L. (1992). Personality: Structure and assessment. Annual Review of Psychology , 43 , 473–504.
Yukl, G. (1993). Leadership in organizations (3rd ed.). Englewood Cliffs, NJ: Prentice-Hall.
Yukl, G., & Van Fleet, D. D. (1991). Theory and research on leadership in organizations. In M. D. Dunnette & L. M. Hough (Eds.). Handbook of industrial and organizational psychology, Vol. 2 (2nd ed.). Palo Alto, CA: Consulting Psychologists Press.
Download references
Author information
Authors and affiliations.
Washington, D.C.
Leonard D. Goodstein
Arizona State University, USA
Richard I. Lanyon
You can also search for this author in PubMed Google Scholar
Rights and permissions
Reprints and permissions
About this article
Goodstein, L.D., Lanyon, R.I. Applications of Personality Assessment to the Workplace: A Review. Journal of Business and Psychology 13 , 291–322 (1999). https://doi.org/10.1023/A:1022941331649
Download citation
Issue Date : September 1999
DOI : https://doi.org/10.1023/A:1022941331649
Share this article
Anyone you share the following link with will be able to read this content:
Sorry, a shareable link is not currently available for this article.
Provided by the Springer Nature SharedIt content-sharing initiative
- Social Psychology
- Social Issue
- Good Evidence
- Research Literature
- Recent Past
- Find a journal
- Publish with us
- Track your research
- Research article
- Open access
- Published: 05 May 2020
Personality traits, emotional intelligence and decision-making styles in Lebanese universities medical students
- Radwan El Othman 1 ,
- Rola El Othman 2 ,
- Rabih Hallit 1 , 3 , 4 na1 ,
- Sahar Obeid 5 , 6 , 7 na1 &
- Souheil Hallit 1 , 5 , 7 na1
BMC Psychology volume 8 , Article number: 46 ( 2020 ) Cite this article
58k Accesses
33 Citations
16 Altmetric
Metrics details
This study aims to assess the impact of personality traits on emotional intelligence (EI) and decision-making among medical students in Lebanese Universities and to evaluate the potential mediating role-played by emotional intelligence between personality traits and decision-making styles in this population.
This cross-sectional study was conducted between June and December 2019 on 296 general medicine students.
Higher extroversion was associated with lower rational decision-making style, whereas higher agreeableness and conscientiousness were significantly associated with a higher rational decision-making style. More extroversion and openness to experience were significantly associated with a higher intuitive style, whereas higher agreeableness and conscientiousness were significantly associated with lower intuitive style. More agreeableness and conscientiousness were significantly associated with a higher dependent decision-making style, whereas more openness to experience was significantly associated with less dependent decision-making style. More agreeableness, conscientiousness, and neuroticism were significantly associated with less spontaneous decision-making style. None of the personality traits was significantly associated with the avoidant decision-making style. Emotional intelligence seemed to fully mediate the association between conscientiousness and intuitive decision-making style by 38% and partially mediate the association between extroversion and openness to experience with intuitive decision-making style by 49.82 and 57.93% respectively.
Our study suggests an association between personality traits and decision-making styles. The results suggest that EI showed a significant positive effect on intuitive decision-making style and a negative effect on avoidant and dependent decision-making styles. Additionally, our study underlined the role of emotional intelligence as a mediator factor between personality traits (namely conscientiousness, openness, and extroversion) and decision-making styles.
Peer Review reports
Decision-making is a central part of daily interactions; it was defined by Scott and Bruce in 1995 as «the learned habitual response pattern exhibited by an individual when confronted with a decision situation. It is not a personality trait, but a habit-based propensity to react in a certain way in a specific decision context» [ 1 ]. Understanding how people make decisions within the moral domain is of great importance theoretically and practically. Its theoretical value is related to the importance of understanding the moral mind to further deepen our knowledge on how the mind works, thus understanding the role of moral considerations in our cognitive life. Practically, this understanding is important because we are highly influenced by the moral decisions of people around us [ 2 ]. According to Scott and Bruce (1995), there are five distinct decision-making styles (dependent, avoidant, spontaneous, rational, intuitive) [ 1 ] and each individuals’ decision-making style has traits from these different styles with one dominant style [ 3 ].
The dependent decision-making style can be regarded as requiring support, advice, and guidance from others when making decisions. Avoidant style is characterized by its tendency to procrastinate and postpone decisions if possible. On the other hand, spontaneous decision-making style is hallmarked by making snap and impulsive decisions as a way to quickly bypass the decision-making process. In other words, spontaneous decision-makers are characterized by the feeling of immediacy favoring to bypass the decision-making process rapidly without employing much effort in considering their options analytically or relying on their instinct. Rational decision-making style is characterized by the use of a structured rational approach to analyze information and options to make decision [ 1 ]. In contrast, intuitive style is highly dependent upon premonitions, instinct, and feelings when it comes to making decisions driving focus toward the flow of information rather than systematic procession and analysis of information, thus relying on hunches and gut feelings. Several studies have evaluated the factors that would influence an individual’s intuition and judgment. Rand et al. (2016) discussed the social heuristics theory and showed that women and not men tend to internalize altruism _ the selfless concern for the well-being of others_ in their intuition and thus in their intuitive decision-making process [ 4 ]. Additionally, intuitive behavior honesty is influenced by the degree of social relationships with individuals affected by the outcome of our decision: when dishonesty harms abstract others, intuition promotion causes more dishonesty. On the contrary, when dishonesty harms concrete others, intuition promotion has no significant effect on dishonesty. Hence, the intuitive appeal of pro-sociality may cancel out the intuitive selfish appeal of dishonesty [ 5 ]. Moreover, the decision-making process and styles have been largely evaluated in previous literature. Greene et al. (2008) and Rand (2016) showed that utilitarian moral judgments aiming to minimize cost and maximize benefits across concerned individuals are driven by controlled cognitive process (i.e. rational); whereas, deontological moral judgments _where rights and duties supersede utilitarian considerations_ are dictated by an automatic emotional response (e.g. spontaneous decision-making) [ 6 , 7 ]. Trémolière et al. (2012) found that mortality salience makes people less utilitarian [ 8 ].
Another valuable element influencing our relationships and career success [ 9 ] is emotional intelligence (EI) a cardinal factor to positive patient experience in the medical field [ 10 ]. EI was defined by Goleman as «the capacity of recognizing our feelings and those of others, for motivating ourselves, and for managing emotions both in us and in our relationships» [ 11 ]. Hence, an important part of our success in life nowadays is dependent on our ability to develop and preserve social relationships, depict ourselves positively, and control the way people descry us rather than our cognitive abilities and traditional intelligence measured by IQ tests [ 12 ]. In other words, emotional intelligence is a subtype of social intelligence involving observation and analyses of emotions to guide thoughts and actions. Communication is a pillar of modern medicine; thus, emotional intelligence should be a cornerstone in the education and evaluation of medical students’ communication and interpersonal skills.
An important predictor of EI is personality [ 13 ] defined as individual differences in characteristic patterns of thinking, feeling and behaving [ 14 ]. An important property of personality traits is being stable across time [ 15 ] and situations [ 16 ], which makes it characteristic of each individual. One of the most widely used assessment tools for personality traits is the Five-Factor model referring to «extroversion, openness to experience, agreeableness, conscientiousness, neuroticism». In fact, personality traits have an important impact on individuals’ life, students’ academic performance [ 17 ] and decision-making [ 18 ].
Extroversion is characterized by higher levels of self-confidence, positive emotions, enthusiasm, energy, excitement seeking, and social interactions. Openness to experience individuals are creative, imaginative, intellectually curious, impulsive, and original, open to new experiences and ideas [ 19 ]. Agreeableness is characterized by cooperation, morality, sympathy, low self-confidence, high levels of trust in others, and tend to be happy and satisfied because of their close interrelationships [ 19 ]. Conscientiousness is characterized by competence, hard work, self-discipline, organization, strive for achievement and goal orientation [ 20 ] with a high level of deliberation making conscientious individuals capable of analyzing the pros and cons of a given situation [ 21 ]. Neuroticism is characterized by anxiety, anger, insecurity, impulsiveness, self-consciousness,and vulnerability [ 20 ]. High neurotic individuals have higher levels of negative affect, are easily irritated, and more likely to turn to inappropriate coping responses, such as interpersonal hostility [ 22 ].
Multiple studies have evaluated the impact of personality traits on decision-making styles. Narooi and Karazee (2015) studied personality traits, attitude to life, and decision-making styles among university students in Iran [ 23 ]. They deduced the presence of a strong relationship between personality traits and decision-making styles [ 23 ]. Riaz and Batool (2012) evaluated the relationship between personality traits and decision-making among a group of university students (Fig. 1 ). They concluded that «15.4 to 28.1% variance in decision-making styles is related to personality traits» [ 24 ]. Similarly, Bajwa et al. (2016) studied the relationship between personality traits and decision-making among students. They concluded that conscientiousness personality trait is associated with rational decision-making style [ 25 ]. Bayram and Aydemir (2017) studied the relationship between personality traits and decision-making styles among a group of university students in Turkey [ 26 ]. Their work yielded to multiple conclusion namely a significant association between rational and intuitive decision-making styles and extroversion, openness to experience, conscientiousness, and agreeableness personality traits [ 26 ]. The dependent decision-making style had a positive relation with both neuroticism and agreeableness. The spontaneous style had a positive relation with neuroticism and significant negative relation with agreeableness and conscientiousness. Extroversion personality traits had a positive effect on spontaneous style. Agreeableness personality had a positive effect on the intuitive and dependent decision-making style. Conscientiousness personality had a negative effect on avoidant and spontaneous decision-making style and a positive effect on rational style. Neuroticism trait had a positive effect on intuitive, dependent and spontaneous decision-making style. Openness to experience personality traits had a positive effect on rational style [ 26 ].
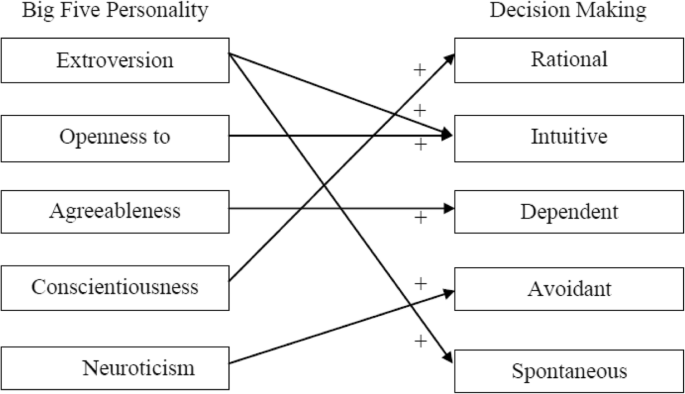
Schematic representation of the effect of the big five personality types on decision-making styles [ 24 ]
Furthermore, several studies have evaluated the relationship between personality traits and emotional intelligence. Dawda and Hart (2000) found a significant relationship between emotional intelligence and all Big Five personality traits [ 27 ]. Day and al. (2005) found a high correlation between emotional intelligence and extroversion and conscientiousness personality traits [ 28 ]. A study realized by Avsec and al. (2009) revealed that emotional intelligence is a predictor of the Big Five personality traits [ 29 ]. Alghamdi and al. (2017) investigated the predictive role of EI on personality traits among university advisors in Saudi Arabia. They found that extroversion, agreeableness, and openness to experience emerged as significant predictors of EI. The study also concluded that conscientiousness and neuroticism have no impact on EI [ 13 ].
Nonetheless, decision-making is highly influenced by emotion making it an emotional process. The degree of emotional involvement in a decision may influence our choices [ 30 ] especially that emotions serve as a motivational process for decision-making [ 31 ]. For instance, patients suffering from bilateral lesions of the ventromedial prefrontal cortex (interfering with normal processing of emotional signals) develop severe impairments in personal and social decision-making despite normal cognitive capabilities (intelligence and creativity); highlighting the guidance role played by emotions in the decision-making process [ 32 ]. Furthermore, EI affects attention, memory, and cognitive intelligence [ 33 , 34 ] with higher levels of EI indicating a more efficient decision-making [ 33 ]. In one study, Khan and al. concluded that EI had a significant positive effect on rational and intuitive decision-making styles and negative effect on dependent and spontaneous decision-making styles among a group of university students in Pakistan [ 35 ].
This study aims to assess the impact of personality traits on both emotional intelligence and decision-making among medical students in Lebanese Universities and to test the potential mediating role played by emotional intelligence between personality and decision-making styles in this yet unstudied population to our knowledge. The goal of the present research is to evaluate the usefulness of implementing such tools in the selection process of future physicians. It also aimed at assessing the need for developing targeted measures, aiming to ameliorate the psychosocial profile of Lebanese medical students, in order to have a positive impact on patients experience and on medical students’ career success.
Study design
This cross-sectional study was conducted between June and December 2019. A total of 296 participants were recruited from all the 7 faculties of medicine in Lebanon. Data collection was done through filling an anonymous online or paper-based self-administered English questionnaire upon the participant choice. All participants were aware of the purpose of the study, the quality of data collected and gave prior informed consent. Participation in this study was voluntary and no incentive was given to the participants. All participants were General medicine students registered as full-time students in one of the 7 national schools of medicine aged 18 years and above regardless of their nationality. The questionnaire was only available in English since the 7 faculties of medicine in Lebanon require a minimum level of good English knowledge in their admission criteria. A pilot test was conducted on 15 students to check the clarity of the questionnaire. To note that these 15 questionnaires related data was not entered in the final database. The methodology used in similar to the one used in a previous paper [ 36 ]
Questionnaire and variables
The questionnaire assessed demographic and health characteristics of participants, including age, gender, region, university, current year in medical education, academic performance (assessed using the current cumulative GPA), parental highest level of education, and health questions regarding the personal history of somatic, and psychiatric illnesses.
The personality traits were evaluated using the Big Five Personality Test, a commonly used test in clinical psychology. Since its creation by John, Donahue, and Kentle (1991) [ 37 ], the five factor model was widely used in different countries including Lebanon [ 38 ]; it describes personality in terms of five board factors: extroversion, openness to experience, agreeableness, conscientiousness and neuroticism according to an individual’s response to a set of 50 questions on a 5-point Likert scale: 1 (disagree) to 5 (agree). A score for each personality trait is calculated in order to determine the major trait(s) in an individual personality (i.e. the trait with the highest score). The Cronbach’s alpha values were as follows: total scale (0.885), extroversion (0.880), openness to experience (0.718), agreeableness (0.668), conscientiousness (0.640), and neuroticism (0.761).
Emotional intelligence was assessed using the Quick Emotional Intelligence Self-Assessment scale [ 38 ]. The scale is divided into four domains: «emotional alertness, emotional control, social-emotional awareness, and relationship management». Each domain is composed of 10 questions, with answers measured on a 5-point Likert scale: 0 (never) to 4 (always). Higher scores indicate higher emotional intelligence [ 38 ] (α Cronbach = 0.950).
The decision-making style was assessed using the Scott and Bruce General Decision-Making Style Inventory commonly used worldwide since its creation in 1995 for this purpose [ 1 ]. The inventory consists of 25 questions answered according to a 5-point Likert scale: 1 (strongly disagree) to 5 (strongly agree) intended to evaluate the importance of each decision-making style among the 5 styles proposed by Scott and Bruce: dependent, avoidant, spontaneous, rational and intuitive. The score for each decision-making style is computed in order to determine the major style for each responder (α Cronbach total scale = 0.744; α Cronbach dependent style = 0.925; α Cronbach avoidant style = 0.927; α Cronbach spontaneous style = 0.935; α Cronbach rational style = 0.933; α Cronbach intuitive style = 0.919).
Sample size calculation
The Epi info program (Centers for Disease Control and Prevention (CDC), Epi Info™) was employed for the calculation of the minimal sample size needed for our study, with an acceptable margin of error of 5% and an expected variance of decision-making styles that is related to personality types estimated by 15.4 to 28.1% [ 24 ] for 5531 general medicine student in Lebanon [ 39 ]. The result showed that 294 participants are needed.
Statistical analysis
Statistical Package for Social Science (SPSS) version 23 was used for the statistical analysis. The Student t-test and ANOVA test were used to assess the association between each continuous independent variable (decision-making style scores) and dichotomous and categorical variables respectively. The Pearson correlation test was used to evaluate the association between two continuous variables. Reliability of all scales and subscales was assessed using Cronbach’s alpha.
Mediation analysis
The PROCESS SPSS Macro version 3.4, model four [ 40 ] was used to calculate five pathways (Fig. 2 ). Pathway A determined the regression coefficient for the effect of each personality trait on emotional intelligence, Pathway B examined the association between EI and each decision-making style, independent of the personality trait, and Pathway C′ estimated the total and direct effect of each personality trait on each decision-making style respectively. Pathway AB calculated the indirect intervention effects. To test the significance of the indirect effect, the macro generated bias-corrected bootstrapped 95% confidence intervals (CI) [ 40 ]. A significant mediation was determined if the CI around the indirect effect did not include zero [ 40 ]. The covariates that were included in the mediation model were those that showed significant associations with each decision-making style in the bivariate analysis.
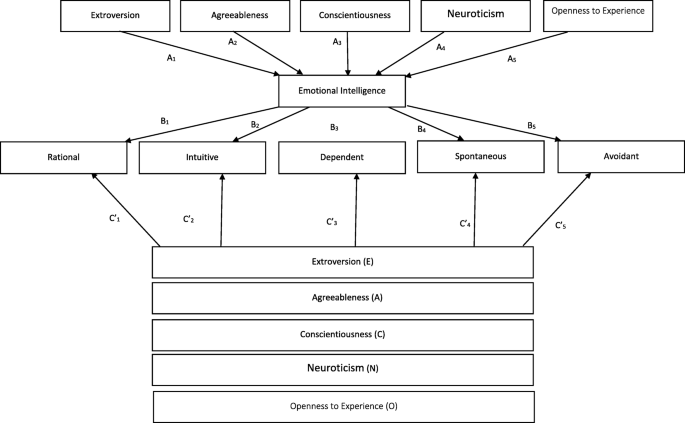
Summary of the pathways followed during the mediation analysis
Sociodemographic and other characteristics of the participants
The mean age of the participants was 22.41 ± 2.20 years, with 166 (56.1%) females. The mean scores of the scales used were as follows: emotional intelligence (108.27 ± 24.90), decision-making: rationale style (13.07 ± 3.17), intuitive style (16.04 ± 3.94), dependent style (15.53 ± 4.26), spontaneous style (13.52 ± 4.22), avoidant style (12.44 ± 4.11), personality trait: extroversion (21.18 ± 8.96), agreeableness (28.01 ± 7.48), conscientiousness (25.20 ± 7.06), neuroticism (19.29 ± 8.94) and openness (27.36 ± 7.81). Other characteristics of the participants are summarized in Table 1 .
Bivariate analysis
Males vs females, having chronic pain compared to not, originating from South Lebanon compared to other governorates, having an intermediate income compared to other categories, those whose mothers had a primary/complementary education level and those whose fathers had an undergraduate diploma vs all other categories had higher mean rationale style scores. Those fathers, who had a postgraduate diploma, had a higher mean intuitive style scores compared to all other education levels. Those who have chronic pain compared to not and living in South Lebanon compared to other governorates had higher dependent style scores. Those who have chronic pain compared to not, those who take medications for a mental illness whose mothers had a primary/complementary education level vs all other categories and those whose fathers had a postgraduate diploma vs all other categories had higher spontaneous style scores (Table 2 ).
Higher agreeableness and conscientiousness scores were significantly associated with higher rational style scores, whereas higher extroversion and neuroticism scores were significantly associated with lower rational style scores. Higher extroversion, openness and emotional intelligence scores were significantly associated with higher intuitive scores, whereas higher agreeableness, conscientiousness and neuroticism scores were significantly associated with lower intuitive style scores. Higher agreeableness and conscientiousness were associated with higher dependent style scores, whereas higher openness and emotional intelligence scores were significantly associated with lower dependent styles scores. Higher agreeableness, conscientiousness, neuroticism, and emotional intelligence scores were significantly associated with lower spontaneous style scores. Finally, higher extroversion, neuroticism and emotional intelligence scores were significantly associated with lower avoidant style scores (Table 3 ).
Post hoc analysis: rationale style: governorate (Beirut vs Mount Lebanon p = 0.022; Beirut vs South p < 0.001; Mount Lebanon vs South p = 0.004; South vs North p = 0.001; South vs Bekaa p = 0.047); monthly income (intermediate vs high p = 0.024); mother’s educational level (high school vs undergraduate diploma p = 0.048); father’s education level (undergraduate vs graduate diploma p = 0.01).
Intuitive style: father’s education level (high school vs postgraduate diploma p = 0.046).
Dependent style: governorate (Beirut vs Mount Lebanon p = 0.006; Beirut vs South p = 0.003);
Avoidant style: mother’s educational level (high school vs undergraduate diploma p = 0.008; undergraduate vs graduate diploma p = 0.004; undergraduate vs postgraduate diploma p = 0.001).
Mediation analysis was run to check if emotional intelligence would have a mediating role between each personality trait and each decision-making style, after adjusting overall covariates that showed a p < 0.05 with each decision-making style in the bivariate analysis.
Rational decision-making style (Table 4 , model 1)
Higher extroversion was significantly associated with higher EI, b = 0.91, 95% BCa CI [0.60, 1.23], t = 5.71, p < 0.001 (R2 = 0.31). Higher extroversion was significantly associated with lower rational decision-making even with EI in the model, b = − 0.06, 95% BCa CI [− 0.11, − 0.02], t = − 2.81, p = 0.003; EI was not significantly associated with rational decision-making, b = 0.02, 95% BCa CI [− 0.0003, 0.03], t = 1.93, p = 0.054 (R2 = 0.29). When EI was not in the model, higher extroversion was significantly associated with lower rational decision-making, b = − 0.05, 95% BCa CI [− 0.09, − 0.01], t = − 2.43, p = 0.015 (R2 = 0.28). The mediating effect of EI was 21.22%.
Higher agreeableness was not significantly associated with EI, b = − 0.05, 95% BCa CI [− 0.40, 0.31], t = − 0.26, p = 0.798 (R2 = 0.31). Higher agreeableness was significantly associated with higher rational decision-making style even with EI in the model, b = 0.07, 95% BCa CI [0.02, 0.11], t = 2.89, p = 0.004; EI was not significantly associated with the rational decision-making, b = 0.01, 95% BCa CI [− 0.0003, 0.03], t = 1.92, p = 0.055 (R2 = 0.29). When EI was not in the model, higher agreeableness was significantly associated with higher rational decision-making, b = 0.07, 95% BCa CI [0.02, 0.11], t = 2.86, p = 0.004 (R2 = 0.28). The mediating effect of EI was 0.10%.
Higher conscientiousness was significantly associated with higher EI, b = 1.40, 95% BCa CI [1.04, 1.76], t = 7.62, p < 0.001 (R2 = 0.31). Higher conscientiousness was significantly associated with the rational decision-making style even with EI in the model, b = 0.09, 95% BCa CI [0.04, 0.14], t = 3.55, p < 0.001; EI was not significantly associated with the rational decision-making, b = 0.01, 95% BCa CI [− 0.0003, 0.03], t = 1.93, p = 0.055 (R2 = 0.29). When EI was not in the model, conscientiousness was significantly associated with the rational decision-making style, b = 0.11, 95% BCa CI [0.07, 0.16], t = 4.76, p < 0.001 (R2 = 0.28). The mediating effect of EI was 22.47%.
Higher neuroticism was significantly associated with lower EI, b = − 0.50, 95% BCa CI [− 0.80, − 0.20], t = − 3.26, p = 0.001 (R2 = 0.31). Neuroticism was not significantly associated with rational decision-making style with EI in the model, b = − 0.09, 95% BCa CI [− 0.05, 0.03], t = − 0.43, p = 0.668; EI was not significantly associated with rational decision-making, b = 0.01, 95% BCa CI [− 0.0003, 0.03], t = 1.93, p = 0.055 (R2 = 0.29). When EI was not in the model, neuroticism was not significantly associated with the rational decision-making style, b = − 0.02, 95% BCa CI [− 0.06, 0.02], t = − 0.81, p = 0.418 (R2 = 0.28).
No calculations were done for the openness to experience personality traits since it was not significantly associated with the rational decision-making style in the bivariate analysis.
Intuitive decision-making style (Table 4 , model 2)
Higher extroversion was significantly associated with higher EI, b = 0.86, 95% BCa CI [0.59, 1.13], t = 6.28, p < 0.001 (R2 = 0.41). Higher extroversion was significantly associated with higher intuitive decision-making even with EI in the model, b = 0.05, 95% BCa CI [0.002, 0.11], t = 2.03, p = 0.043; EI was significantly associated with intuitive decision-making style, b = 0.03, 95% BCa CI [0.01, 0.05], t = 2.91, p = 0.003 (R2 = 0.21). When EI was not in the model, higher extroversion was significantly associated with higher intuitive decision-making, b = 0.08, 95% BCa CI [0.03, 0.13], t = 3.21, p = 0.001 (R2 = 0.18). The mediating effect of EI was 49.82%.
Higher agreeableness was significantly associated with EI, b = − 0.33, 95% BCa CI [− 0.65, − 0.02], t = − 2.06, p = 0.039 (R2 = 0.41). Higher agreeableness was significantly associated with lower intuitive decision-making style even with EI in the model, b = − 0.15, 95% BCa CI [− 0.21, − 0.10], t = − 5.16, p < 0.001; higher EI was significantly associated with higher intuitive decision-making, b = 0.03, 95% BCa CI [0.01, 0.05], t = 2.91, p = 0.004 (R2 = 0.21). When EI was not in the model, higher agreeableness was significantly associated with lower intuitive decision-making, b = − 0.17, 95% BCa CI [− 0.22, − 0.11], t = − 5.48, p < 0.001 (R2 = 0.18). The mediating effect of EI was 6.80%.
Higher conscientiousness was significantly associated with higher EI, b = 1.18, 95% BCa CI [0.85, 1.51], t = 7.06, p < 0.001 (R2 = 0.41). Higher conscientiousness was significantly associated with lower intuitive decision-making style even with EI in the model, b = − 0.10, 95% BCa CI [− 0.16, − 0.03], t = − 2.95, p = 0.003; higher EI was also significantly associated with higher intuitive decision-making, b = 0.03, 95% BCa CI [0.01, 0.05], t = 2.91, p = 0.004 (R2 = 0.21). When EI was not in the model, conscientiousness was not significantly associated with the intuitive decision-making style, b = − 0.06, 95% BCa CI [− 0.12, 0.0004], t = − 1.95, p = 0.051 (R2 = 0.18). The mediating effect of EI was 38%.
Higher openness to experience was significantly associated with higher EI, b = 1.44, 95% BCa CI [1.13, 1.75], t = 9.11, p < 0.001 (R2 = 0.41). Higher openness to experience was significantly associated with higher intuitive decision-making style with EI in the model, b = 0.08, 95% BCa CI [0.01, 0.14], t = 2.38, p = 0.017; higher EI was also significantly associated with intuitive decision-making style, b = 0.03, 95% BCa CI [0.01, 0.05], t = 2.91, p = 0.004 (R2 = 0.21). When EI was not in the model, higher openness to experience was significantly associated with intuitive decision-making style, b = 0.12, 95% BCa CI [0.06, 0.18], t = 4.22, p < 0.001 (R2 = 0.18). The mediating effect of EI was 57.93%.
No calculations were done for neuroticism personality trait since it was not significantly associated with the intuitive decision-making style in the bivariate analysis.
Dependent decision-making style (Table 4 , model 3)
Agreeableness was not significantly associated with EI, b = − 0.15, 95% BCa CI [− 0.49, 0.17], t = − 0.94, p = 0.345 (R2 = 0.32). Higher agreeableness was significantly associated with higher dependent decision-making style even with EI in the model, b = 0.29, 95% BCa CI [0.23, 0.34], t = 10.51, p < 0.001; higher EI was significantly associated with lower dependent decision-making, b = − 0.04, 95% BCa CI [− 0.06, − 0.02], t = − 4.50, p < 0.001 (R2 = 0.40). When EI was not in the model, higher agreeableness was significantly associated with higher dependent decision-making, b = 0.29, 95% BCa CI [0.24, 0.35], t = 10.44, p < 0.001 (R2 = 0.18). The mediating effect of EI was 2.38%.
Higher conscientiousness was significantly associated with higher EI, b = 1.04, 95% BCa CI [0.69, 1.38], t = 5.93, p < 0.001 (R2 = 0.32). Higher conscientiousness was significantly associated with higher dependent decision-making style even with EI in the model, b = 0.15, 95% BCa CI [0.09, 0.20], t = 4.88, p < 0.001; higher EI was also significantly associated with lower dependent decision-making, b = − 0.04, 95% BCa CI [− 0.06, − 0.02], t = − 4.50, p < 0.001 (R2 = 0.40). When EI was not in the model, higher conscientiousness was significantly associated with a higher dependent decision-making style, b = 0.10, 95% BCa CI [0.04, 0.16], t = 3.49, p < 0.001 (R2 = 0.36). The mediating effect of EI was 30.25%.
Higher openness to experience was significantly associated with higher EI, b = 1.37, 95% BCa CI [1.05, 1.69], t = 8.41, p < 0.001 (R2 = 0.32). Higher openness to experience was significantly associated with lower dependent decision-making style even with EI in the model, b = − 0.13, 95% BCa CI [− 0.19, − 0.08], t = − 4.55, p < 0.001; higher EI was also significantly associated with dependent decision-making style, b = − 0.04, 95% BCa CI [− 0.19, − 0.08], t = − 4.50, p < 0.001 (R2 = 0.40). When EI was not in the model, higher openness to experience was significantly associated with lower dependent decision-making style, b = − 0.19, 95% BCa CI [− 0.24, − 0.14], t = − 7.06, p < 0.001 (R2 = 0.36). The mediating effect of EI was 43.69%.
No calculations were done for neuroticism and extroversion personality traits since they were not significantly associated with the dependent decision-making style in the bivariate analysis.
Spontaneous decision-making style (Table 4 , model 4)
Agreeableness was not significantly associated with EI, b = 0.17, 95% BCa CI [− 0.19, 0.53], t = 0.91, p = 0.364 (R2 = 0.17). Higher agreeableness was significantly associated with lower spontaneous decision-making style even with EI in the model, b = − 0.10, 95% BCa CI [− 0.16, − 0.03], t = − 3.07, p = 0.002; EI was not significantly associated with spontaneous decision-making, b = − 0.01, 95% BCa CI [− 0.03, 0.01], t = − 0.71, p = 0.476 (R2 = 0.15). When EI was not in the model, higher agreeableness was significantly associated with lower spontaneous decision-making, b = − 0.10, 95% BCa CI [− 0.16, − 0.04], t = − 3.11, p = 0.002 (R2 = 0.15). The mediating effect of EI was 1.25%.
Higher conscientiousness was significantly associated with higher EI, b = 1.26, 95% BCa CI [0.88, 1.64], t = 6.56, p < 0.001 (R2 = 0.17). Higher conscientiousness was significantly associated with lower spontaneous decision-making style even with EI in the model, b = − 0.16, 95% BCa CI [− 0.23, − 0.09], t = − 4.51, p < 0.001; EI was not significantly associated with spontaneous decision-making style, b = − 0.01, 95% BCa CI [− 0.03, 0.01], t = − 0.71, p = 0.476 (R2 = 0.15). When EI was not in the model, higher conscientiousness was significantly associated with lower spontaneous decision-making style, b = − 0.17, 95% BCa CI [− 0.23, − 0.10], t = − 5.11, p < 0.001 (R2 = 0.15). The mediating effect of EI was 5.64%.
Neuroticism was not significantly associated with EI, b = − 0.22, 95% BCa CI [− 0.53, 0.08], t = − 1.43, p = 0.153 (R2 = 0.17). Higher neuroticism was significantly associated with lower spontaneous decision-making style even with EI in the model, b = − 0.11, 95% BCa CI [− 0.16, − 0.06], t = − 4.05, p < 0.001; EI was not significantly associated with spontaneous decision-making style, b = − 0.01, 95% BCa CI [− 0.03, 0.01], t = − 0.71, p = 0.476 (R2 = 0.15). When EI was not in the model, higher neuroticism was significantly associated with lower spontaneous decision-making style, b = − 0.11, 95% BCa CI [− 0.16, − 0.05], t = − 4.01, p < 0.001 (R2 = 0.15). The mediating effect of EI was 1.49%.
No calculations were done for openness to experience and extroversion personality traits since they were not significantly associated with the spontaneous decision-making style in the bivariate analysis .
Avoidant decision-making style (Table 4 , model 5)
Higher extroversion was significantly associated with higher EI, b = 0.88, 95% BCa CI [0.54, 1.21], t = 5.18, p < 0.001 (R2 = 0.15). Extroversion was not significantly associated with avoidant decision-making style even with EI in the model, b = − 0.01, 95% BCa CI [− 0.06, 0.05], t = − 0.27, p = 0.790; higher EI was significantly associated with avoidant decision-making style, b = − 0.04, 95% BCa CI [− 0.06, 0.03], t = − 4.79, p < 0.001 (R2 = 0.25). When EI was not in the model, extroversion was not significantly associated with avoidant decision-making style, b = − 0.05, 95% BCa CI [− 0.1, 0.08], t = − 1.69, p = 0.092 (R2 = 0.19).
Higher neuroticism was significantly associated with lower EI, b = − 0.59, 95% BCa CI [− 0.91, − 0.27], t = − 3.60, p < 0.001 (R2 = 0.15). Neuroticism was not significantly associated with avoidant decision-making style even with EI in the model, b = − 0.03, 95% BCa CI [− 0.09, 0.02], t = − 1.34, p = 0.182; higher EI was significantly associated with lower avoidant decision-making style, b = − 0.04, 95% BCa CI [− 0.06, − 0.03], t = − 4.79, p < 0.001 (R2 = 0.25). When EI was not in the model, neuroticism was not significantly associated with avoidant decision-making style, b = − 0.09, 95% BCa CI [− 0.06, 0.04], t = − 0.33, p = 0.739 (R2 = 0.19).
No calculations were done for openness to experience, agreeableness, and conscientiousness personality traits since they were not significantly associated with the avoidant decision-making style in the bivariate analysis.
This study examined the relationship between personality traits and decision-making styles, and the mediation role of emotional intelligence in a sample of general medicine students from different medical schools in Lebanon.
Agreeableness is characterized by cooperation, morality, sympathy, low self-confidence, high levels of trust in others and agreeable individuals tend to be happy and satisfied because of their close interrelationships [ 19 , 20 ]. Likewise, dependent decision-making style is characterized by extreme dependence on others when it comes to making decisions [ 1 ]. Our study confirmed this relationship similarly to Wood (2012) [ 41 ] and Bayram and Aydemir (2017) [ 26 ] findings of a positive relationship between dependent decision-making style and agreeableness personality trait and a negative correlation between this same personality trait and spontaneous decision-making style. In fact, this negative correlation can be explained by the reliance and trust accorded by agreeable individuals to their surroundings, making them highly influenced by others opinions when it comes to making a decision; hence, avoiding making rapid and snap decisions on the spur of the moment (i.e. spontaneous decision-making style); in order to explore the point of view of their surrounding before deciding on their own.
Conscientiousness is characterized by competence, hard work, self-discipline, organization, strive for achievement, and goal orientation [ 20 ]. Besides, conscientious individuals have a high level of deliberation making them capable of analyzing the pros and cons of a given situation [ 21 ]. Similarly, rational decision-makers strive for achievements by searching for information and logically evaluating alternatives before making decisions; making them high achievement-oriented [ 20 , 42 ]. This positive relationship between rational decision-making style and conscientiousness was established by Nygren and White (2005) [ 43 ] and Bajwa et al. (2016) [ 25 ]; thus, solidifying our current findings. Furthermore, we found that conscientiousness was positively associated with dependent decision-making; this relationship was not described in previous literature to our knowledge and remained statistically significant after adding EI to the analysis model. This relationship may be explained by the fact that conscientious individuals tend to take into consideration the opinions of their surrounding in their efforts to analyze the pros and cons of a situation. Further investigations in similar populations should be conducted in order to confirm this association. Moreover, we found a positive relationship between conscientiousness and intuitive decision-making that lost significance when EI was removed from the model. Thus, solidifying evidence of the mediating role played by EI between personality trait and decision-making style with an estimated mediation effect of 38%.
Extroversion is characterized by higher levels of self-confidence, positive emotions, enthusiasm, energy, excitement seeking, and social interactions. Similarly, intuitive decision-making is highly influenced by emotions and instinct. The positive relationship between extroversion and intuitive decision-making style was supported by Wood (2012) [ 41 ], Riaz et al. (2012) [ 24 ] and Narooi and Karazee (2015) [ 23 ] findings and by our present study.
Neuroticism is characterized by anxiety, anger, self-consciousness, and vulnerability [ 20 ]. High neurotic individuals have higher levels of negative affect, depression, are easily irritated, and more likely to turn to inappropriate coping responses, such as interpersonal hostility [ 22 ]. Our study results showed a negative relationship between neuroticism and spontaneous decision-making style.
Openness to experience individuals are creative, imaginative, intellectually curious, impulsive and original, open to new experiences and ideas [ 19 , 20 ]. One important characteristic of intuitive decision-making style is tolerance for ambiguity and the ability to picture the problem and its potential solution [ 44 ]. The positive relationship between openness to experience and intuitive decision-making style was established by Riaz and Batool (2012) [ 24 ] and came in concordance with our study findings. Additionally, our results suggest that openness personality trait is negatively associated with dependent decision-making style similar to previous findings [ 23 ]. Openness to experience individuals are impulsive and continuously seek intellectual pursuits and new experiences; hence, they tend to depend to a lesser extent on others’ opinions when making decisions since they consider the decision-making process a way to uncover new experiences and opportunities.
Our study results showed that EI had a significant positive effect on intuitive decision-making style. Intuition can be regarded as an interplay between cognitive and affective processes highly influenced by tactic knowledge [ 45 ]; hence, intuitive decision-making style is the result of personal and environmental awareness [ 46 , 47 , 48 ] in which individuals rely on the overall context without much concentration on details. In other words, they depend on premonitions, instinct, and predications of possibilities focusing on designing the overall plan [ 49 ] and take responsibility for their decisions [ 46 ]. Our study finding supports the results of Khan and al. (2016) who concluded that EI and intuitive decision-making had a positive relationship [ 35 ]. On the other hand, our study showed a negative relationship between EI and avoidant and dependent decision-making styles. Avoidant decision-making style is defined as a continuous attempt to avoid decision-making when possible [ 1 ] since they find it difficult to act upon their intentions and lack personal and environmental awareness [ 50 ]. Similarly to our findings, Khan and al. (2016) found that avoidant style is negatively influenced by EI [ 35 ]. The dependent decision-making style can be regarded as requiring support, advice, and guidance from others when making decisions. In other words, it can be described as an avoidance of responsibility and adherence to cultural norms; thus, dependent decision-makers tend to be less influenced by their EI in the decision-making process. Our conclusion supports Avsec’s (2012) findings [ 51 ] on the negative relationship between EI and dependent decision-making style.
Practical implications
The present study helps in determining which sort of decision is made by which type of people. This study also represents a valuable contribution to the Lebanese medical society in order to implement such variables in the selection methods of future physicians thus recruiting individuals with positively evaluated decision-making styles and higher levels of emotional intelligence; implying better communication skills and positively impacting patients’ experience. Also, the present study may serve as a valuable tool for the medical school administration to develop targeted measures to improve students’ interpersonal skills.
Limitations
Even though the current study is an important tool in order to understand the complex relationship between personality traits, decision-making styles and emotional intelligence among medical students; however, it still carries some limitations. This study is a descriptive cross-sectional study thus having a lower internal validity in comparison with experimental studies. The Scott and Bruce General Decision-Making Style Inventory has been widely used internationally for assessing decision-making styles since 1995 but has not been previously validated in the Lebanese population. In addition, the questionnaire was only available in English taking into consideration the mandatory good English knowledge in all the Lebanese medical schools; however, translation, and cross-language validation should be conducted in other categories of Lebanese population. Furthermore, self-reported measures were employed in the present research where participants self-reported themselves on personality types, decision-making styles and emotional intelligence. Although, all used scales are intended to be self-administered; however, this caries risk of common method variance; hence, cross-ratings may be employed in the future researches in order to limit this variance.
The results suggest that EI showed a significant positive effect on intuitive decision-making style and a negative effect on avoidant and dependent decision-making styles. In addition, our study showed a positive relationship between agreeableness and dependent decision-making style and a negative correlation with spontaneous decision-making style. Furthermore, conscientiousness had a positive relationship with rational and dependent decision-making style and extroversion showed a positive relationship with intuitive decision-making style. Neuroticism had a negative relationship with spontaneous style and openness to experience showed a positive relationship with intuitive decision-making style and a negative relationship with dependent style. Additionally, our study underlined the role of emotional intelligence as a mediation factor between personality traits and decision-making styles namely openness to experience, extroversion, and conscientiousness personality traits with intuitive decision-making style. Personality traits are universal [ 20 ]; beginning in adulthood and remaining stable with time [ 52 ]. Comparably, decision-making styles are stable across situations [ 1 ]. The present findings further solidify a previously established relationship between personality traits and decision-making and describes the effect of emotional intelligence on this relationship.
Availability of data and materials
All data generated or analyzed during this study are not publicly available to maintain the privacy of the individuals’ identities. The dataset supporting the conclusions is available upon request to the corresponding author.
Scott SG, Bruce RA. Decision-making style: the development and assessment of a new measure. Educ Psychol Meas. 1995;55(5):818–31.
Article Google Scholar
Capraro V, Everett JA, Earp BD. Priming intuition disfavors instrumental harm but not impartial beneficence. J Exp Soc Psychol. 2019;83:142–9.
Allwood CM, Salo I. Decision-making styles and stress. Int J Stress Manag. 2012;19(1):34.
Rand DG, Brescoll VL, Everett JA, Capraro V, Barcelo H. Social heuristics and social roles: intuition favors altruism for women but not for men. J Exp Psychol Gen. 2016;145(4):389–96.
Article PubMed Google Scholar
Kobis NC, Verschuere B, Bereby-Meyer Y, Rand D, Shalvi S. Intuitive honesty versus dishonesty: meta-analytic evidence. Perspect Psychol Sci. 2019;14(5):778–96.
Greene JD, Morelli SA, Lowenberg K, Nystrom LE, Cohen JD. Cognitive load selectively interferes with utilitarian moral judgment. Cognition. 2008;107(3):1144–54.
Rand DG. Cooperation, fast and slow: meta-analytic evidence for a theory of social heuristics and self-interested deliberation. Psychol Sci. 2016;27(9):1192–206.
Tremoliere B, Neys WD, Bonnefon JF. Mortality salience and morality: thinking about death makes people less utilitarian. Cognition. 2012;124(3):379–84.
Brown C, George-Curran R, Smith ML. The role of emotional intelligence in the career commitment and decision-making process. J Career Assess. 2003;11(4):379–92.
Clancy C. The importance of emotional intelligence. Nursing Management (2014+). 2014;21(8):15.
Goleman D. Working with emotional intelligence. New York: Bantam; 1998.
Google Scholar
Cotruş A, Stanciu C, Bulborea AA. EQ vs. IQ which is Most important in the success or failure of a student? Procedia Soc Behav Sci. 2012;46:5211–3.
Alghamdi NG, Aslam M, Khan K. Personality traits as predictor of emotional intelligence among the university teachers as advisors. Educ Res Int. 2017;2017:1–6 https://doi.org/10.1155/2017/9282565 .
Kazdin AE. Encyclopedia of psychology, vol. Vol 8. Washington, DC: American Psychological Association; 2000.
Bleidorn W, Klimstra TA, Denissen JJ, Rentfrow PJ, Potter J, Gosling SD. Personality maturation around the world: a cross-cultural examination of social-investment theory. Psychol Sci. 2013;24(12):2530–40.
Borkenau P, Riemann R, Spinath FM, Angleitner A. Genetic and environmental influences on person× situation profiles. J Pers. 2006;74(5):1451–80.
Hazrati-Viari A, Rad AT, Torabi SS. The effect of personality traits on academic performance: the mediating role of academic motivation. Procedia Soc Behav Sci. 2012;32:367–71.
Proceedings of the international conference on multiple academic disciplines. HanoiISBN: 978–1–943579-61-7 Hai Phong - . . Paper ID: VM714. 18–19, August: Vietnam (MAD17Vietnam Conference); 2017.
McCrae RR, John OP. An introduction to the five-factor model and its applications. J Pers. 1992;60(2):175–215.
McCrae R, Costa P Jr. Personality in adulthood. In: O envelhecimento. Uma abordagem psicológica . New York: Guilford; 1990. p. 2.
Johnson JA. Units of analysis for the description and explanation of personality. In R. Hogan, J. Johnson, & S. Briggs (Eds.) Handbook of personality psychology : Elsevier; 1997. p. 73–93.
Camps J, Stouten J, Euwema M. The relation between supervisors’ big five personality traits and employees’ experiences of abusive supervision. Front Psychol. 2016;7:112.
Article PubMed PubMed Central Google Scholar
Narooi ZS, Karazee F. Investigating the relationship among personality traits, decision-making styles, and attitude to life (Zahedan branch of Islamic Azad University as case study in Iran). Mediterr J Soc Sci. 2015;6(6 S6):311.
Riaz MN, Riaz MA, Batool N. Personality types as predictors of decision making styles. J Behav Sci. 2012;22(2):100–14.
Bajwa RS, Batool I, Asma M, Ali H, Ajmal A. Personality traits and decision making styles among university students (Pakistan). Pakistan J Life Soc Sci. 2016;14(1):38–41.
Bayram N, Aydemir M. Decision-making styles and personality traits. Int J Recent Adv Organizational Behaviour Decision Sci. 2017;3:905–15.
Dawda D, Hart SD. Assessing emotional intelligence: reliability and validity of the Bar-on emotional quotient inventory (EQ-i) in university students. Personal Individ Differ. 2000;28(4):797–812.
Day AL, Therrien DL, Carroll SA. Predicting psychological health: assessing the incremental validity of emotional intelligence beyond personality, type a behaviour, and daily hassles. Eur J Personality. 2005;19(6):519–36.
Avsec A, Takšić V, Mohorić T. The relationship of trait emotional intelligence with the big five in Croatian and Slovene university student samples. Psihološka obzorja/Horizons Psychol. 2009;18(3):99–110.
Spicer DP, Sadler-Smith E. An examination of the general decision making style questionnaire in two UK samples. J Manag Psychol. 2005;20(2):137–49.
Zeelenberg M, Pieters R. Feeling is for doing: a pragmatic approach to the study of emotions in decision-makingManuscript under review; 2005.
Bechara A, Damasio AR. The somatic marker hypothesis: a neural theory of economic decision. Games Econ Behavior. 2005;52(2):336–72.
Kustubayeva A, Bagayeva E. Emotional intelligence and decision making. RUDN J Psychol Pedagogics. 2011;S5:104–9.
Jaušovec N, Jaušovec K. Sex differences in brain activity related to general and emotional intelligence. Brain Cogn. 2005;59(3):277–86.
Khan E, Riaz M, Batool N, Riaz M. Emotional intelligence as a predictor of decision making styles among university students. J Appl Environ Biol Sci. 2016;6(4S):93–9.
Sarkis A. Hallit S. Hajj A. Lebanese students’ motivation in medical school: does it change throughout the years? A cross-sectional study. BMC Med Educ 20, 94 (2020). https://doi.org/10.1186/s12909-020-02011-w .
John OP, Donahue EM, Kentle RL. The big five inventory—versions 4a and 54. Berkeley: University of California, Berkeley, Institute of Personality; 1991.
Mohapel P. The quick emotional intelligence self-assessment: San Diego City College MESA Program; 2015.
Soueid M, Ghanem S, Hariri Z, Yamout N, Nehme R. Analysis of Lebanon's education sector: BankMed Market & Economic Research Divisionupdated Nov 10; cited 2015 Apr 6; 2014. Available from: http://www.bankmed.com.lb/BOMedia/subservices/categories/News/20150515170635891.pdf . Accessed 17 Jan 2020.
Hayes AF. Introduction to Mediation, Moderation, and Conditional Process Analysis: A Regression‐Based Approach. New York, NY: The Guilford Press.
Thomas E. Nygren, Rebecca J. White; 2005. Relating Decision Making Styles to Predicting Selfefficacy and a Generalized Expectationof Success and Failure. Article in Human Factors andErgonomics Society Annual Meeting Proceedings 49(3):432–34.
Baiocco R, Laghi F, D'Alessio M. Decision-making style among adolescents: relationship with sensation seeking and locus of control. J Adolesc. 2009;32(4):963–76.
Nygren TE, White RJ. Relating decision making styles to predicting selfefficacy and a generalized expectation of success and failure. Paper presented at: Proceedings of the Human Factors and Ergonomics Society Annual Meeting. 2005.
Bergstrand B. Situating the estimate: naturalistic decision-making as an alternative to analytical decision making in the Canadian forcesunpublished article. n. pag. http://www.cfcsc.dnd.ca/irc/nh/nh9798/0021.html ; 2001. p. 24.
Sinclair M, Ashkanasy NM. Intuition: myth or a decision-making tool? Manag Learn. 2005;36(3):353–70.
Harren VA. A model of career decision making for college students. J Vocat Behav. 1979;14(2):119–33.
Hablemitoglu S, Yildirim F. The relationship between perception of risk and decision making styles of Turkish university students: a descriptive study of individual differences. World Appl Sci J. 2008;4(2):214–24.
Singh R, Greenhaus JH. The relation between career decision-making strategies and person–job fit: a study of job changers. J Vocat Behav. 2004;64(1):198–221.
Miller CC, Ireland RD. Intuition in strategic decision making: friend or foe in the fast-paced 21st century? Acad Manag Perspect. 2005;19(1):19–30.
Phillips SD, Pazienza NJ, Ferrin HH. Decision-making styles and problem-solving appraisal. J Couns Psychol. 1984;31(4):497.
Avsec A. Do emotionally intelligent individuals use more adaptive decision-making styles? Stud Psychol. 2012;54(3):209.
Soldz S, Vaillant GE. The big five personality traits and the life course: a 45-year longitudinal study. J Res Pers. 1999;33(2):208–32.
Download references
Acknowledgements
We would like to thank all students who agreed to participate in this study.
Author information
Rabih Hallit, Sahar Obeid and Souheil Hallit are last co-authors.
Authors and Affiliations
Faculty of Medicine and Medical Sciences, Holy Spirit University of Kaslik (USEK), Jounieh, Lebanon
Radwan El Othman, Rabih Hallit & Souheil Hallit
Department of Pediatrics, Bahman Hospital, Beirut, Lebanon
Rola El Othman
Department of Infectious Disease, Bellevue Medical Center, Mansourieh, Lebanon
Rabih Hallit
Department of Infectious Disease, Notre Dame des Secours University Hospital Center, Byblos, Lebanon
Research and Psychology departments, Psychiatric Hospital of the Cross, P.O. Box 60096, Jal Eddib, Lebanon
Sahar Obeid & Souheil Hallit
Faculty of Arts and Sciences, Holy Spirit University of Kaslik (USEK), Jounieh, Lebanon
Sahar Obeid
INSPECT-LB: Institut National de Santé Publique, Epidémiologie Clinique et Toxicologie – Liban, Beirut, Lebanon
You can also search for this author in PubMed Google Scholar
Contributions
REO and REO were responsible for the data collection and entry and drafted the manuscript. SH and SO designed the study; SH carried out the analysis and interpreted the results; RH assisted in drafting and reviewing the manuscript; All authors reviewed the final manuscript and gave their consent; SO, SH and RH were the project supervisors.
Corresponding authors
Correspondence to Sahar Obeid or Souheil Hallit .
Ethics declarations
Ethics approval and consent to participate.
The Holy Spirit University of Kaslik – School of Medicine and Medical Sciences ethics committee approved the study protocol. A written consent was obtained from each student.
Consent for publication
Not applicable.
Competing interests
The authors have nothing to disclose.
Additional information
Publisher’s note.
Springer Nature remains neutral with regard to jurisdictional claims in published maps and institutional affiliations.
Rights and permissions
Open Access This article is licensed under a Creative Commons Attribution 4.0 International License, which permits use, sharing, adaptation, distribution and reproduction in any medium or format, as long as you give appropriate credit to the original author(s) and the source, provide a link to the Creative Commons licence, and indicate if changes were made. The images or other third party material in this article are included in the article's Creative Commons licence, unless indicated otherwise in a credit line to the material. If material is not included in the article's Creative Commons licence and your intended use is not permitted by statutory regulation or exceeds the permitted use, you will need to obtain permission directly from the copyright holder. To view a copy of this licence, visit http://creativecommons.org/licenses/by/4.0/ . The Creative Commons Public Domain Dedication waiver ( http://creativecommons.org/publicdomain/zero/1.0/ ) applies to the data made available in this article, unless otherwise stated in a credit line to the data.
Reprints and permissions
About this article
Cite this article.
El Othman, R., El Othman, R., Hallit, R. et al. Personality traits, emotional intelligence and decision-making styles in Lebanese universities medical students. BMC Psychol 8 , 46 (2020). https://doi.org/10.1186/s40359-020-00406-4
Download citation
Received : 27 January 2020
Accepted : 12 April 2020
Published : 05 May 2020
DOI : https://doi.org/10.1186/s40359-020-00406-4
Share this article
Anyone you share the following link with will be able to read this content:
Sorry, a shareable link is not currently available for this article.
Provided by the Springer Nature SharedIt content-sharing initiative
- Personality traits
- Decision-making
- Decision-making style
- Emotional intelligence
- Medical students
BMC Psychology
ISSN: 2050-7283
- General enquiries: [email protected]
BRIEF RESEARCH REPORT article
How good is the myers-briggs type indicator for predicting leadership-related behaviors.
- 1 Colegio de Estudios Superiores de Administración, Bogotá, Colombia
- 2 Tecnológico de Monterrey, Monterrey, Mexico
The Myers-Briggs Type Indicator (MBTI) is a popular tool used by psychologists working as managers' coaches in organizational contexts. Despite its popularity, few studies provide empirical evidence on the role of the MBTI as a predictor of managers' leadership-related behaviors. This article is written based on research that answers the question of how good the MBTI is to prove leadership behavior. It does so by comparing goodness-of-fit indexes of two confirmatory factor analysis models and two structural models on the personality-leadership relationship, following standards of reproducible research principles. We sampled 529 participants who were graduate and undergraduate students enrolled in business administration programs from Colombian universities. Results show conclusive evidence of the psychometric measurement of both MBTI and leadership practices, even though the relationship between MBTI and the leadership practices inventory proved to be weak.
1. Introduction
The Myers-Briggs Type Indicator (MBTI) is well-known in psychology and related fields as a self-report questionnaire. Its development relied on Jung's seminal ideas on psychological types as a framework to describe human personality ( Jung, 1923 ). Nowadays, MBTI is a tool that provides a variety of practical purposes. Credit scores prediction ( Ertemel and Çaylak, 2021 ), analysis of construction workers' safety behavior ( Ma et al., 2021 ), validation of artificial intelligence techniques ( Sahono et al., 2020 ; Genina et al., 2021 ), or prediction of judging-perceiving behaviors in online social forum ( Choong and Varathan, 2021 ) are just a few recent examples of such purposes.
In business and managerial contexts, the reputation of MBTI probably emerged in the last decade of the last century ( Schweiger, 1985 ; Furnham and Stringfield, 1993 ; Zumbo and Taylor, 1993 ; Ramsoomair, 1994 ; Mani, 1995 ) when its utility as a professional tool was summarized by Gardner and Martinko (1996) . Among the many usages of MBTI, one of the most relevant is its value as a data collection tool to understand leaders' differences, let alone its value in promoting self-awareness of their behaviors and boosting team learning and development in organizational settings ( Costello, 1993 ; Pestana and Codina, 2019 , 2020 ; Penzias, 2020 ). Even though the MBTI is useful for teaching leadership skills ( Shope et al., 2000 ), we are not aware of previous endeavors focusing on assessing its strengths as a leadership predictor. To the best of our knowledge, previous works have focused on literature reviews ( Gardner and Martinko, 1996 ; Brown and Reilly, 2009 ) while the study of Bess and Harvey (2002) emerged as one of the few statistical studies that analyzed the bimodal empirical distributions that result from using this tool as a data collection technique. The aim of the current article is to analyze the strength of the relationship between personality, as described by the MBTI, and leadership performance. The research was conducted by resuming the conceptual ideas that pinpoint the link between leadership-related behaviors with the MBTI scores that describe personality dichotomies. To do this, we sampled 529 participants who were graduate and undergraduate students enrolled in business administration programs from Colombian universities. Apart from continuing this research orientation, the contribution of this article is visible in terms of principles of open science, as it incorporates reproducible research standards that facilitate the audit of findings and maximizes the chances of reproducibility in further studies ( Hardwicke et al., 2022 ).
1.1. Personality dichotomies-MBTI
The use of MBTI as a tool for identifying personality characteristics in university students is not novel and the work of Zavyalova et al. (2021) with Russian students illustrates it. Nonetheless, we are not aware of similar efforts in Latin-American countries. As per Choong and Varathan (2021) there are two approaches to personality, the trait-based approach and the type-based approach. The so-called Big Five , created by Srivastava et al. (2003) , is probably the best-known measurement instrument that relied on the trait-based approach that posed the idea of five large dimensions of personality, hence its name in its original English language version. In contrast, the MBTI is the best-known measurement tool that relied on the type-based approach.
The MBTI is a forced-choice instrument designed to determine how people see the world and how people make decisions ( Myers and Mccaulley, 1985 ; Myers, 1993 , 2016 , 2019 ; King and Mason, 2020 ). It was created by Katherine and Isabel Briggs following Carl Jung's personality theory ( Myers and Mccaulley, 1985 ; Myers, 1993 ; King and Mason, 2020 ). The MBTI consists of four opposite dichotomies ( Myers and Myers, 2010 ), Extraversion-Introversion , that refers to where to focus attention and energy, introverts focus their energy inside of them and they are interested in the world of thoughts and reflections while extroverts focus their attention and energy outward, and they are interested in the world of people and things; Intuition-Sensing , that refers to what kind of information people like and trust, sensitives prefer to take information using their five senses while intuitive people go beyond what is real or concrete and focus on meaning, associations, and relationships; Feeling-Thinking , that refers to the way people make decisions, feelers make their decisions with a person-centered, values-based process while thinkers make their decisions based on impersonal, objective logic, and finally Judgment-Perceiving , that relates to the way we orient ourselves to the external world, people who prefer judgment want the external world to be organized and orderly while people who prefer perceiving, seek to experience the world, not organize it ( Choong and Varathan, 2021 ). When combining the four dichotomies, extroversion-introversion, intuition-sensing, feeling-thinking, judgment-perceiving, it results in sixteen types of personalities (i.e., ISTJ, ISTP, ISFJ, ISFP, INTJ, INTP, INFJ, INFP, ESTJ, ESTP, ESFJ, ESFP, ENTJ, ENTP, ENFJ, and ENFP) ( King and Mason, 2020 ). The MBTI has received criticism in basically one way from trait theorists: people can not be classified dichotomously ( King and Mason, 2020 ). Despite of this criticism, MBTI researchers have shown different studies with acceptable indexes of reliability and validity ( Furnham and Stringfield, 1993 ; King and Mason, 2020 ) and this has paved the way to its continuing use in research across the world as shown by Garland and Village (2021) .
1.2. Leadership practices inventory
Academics have traditionally studied leadership from two perspectives, one that focuses on positional leadership within an organization's hierarchy and one that views leadership as a process of social influence that occurs naturally in a social system ( Helland and Winston, 2005 ). Within these perspectives, four approaches to leadership theories have been determined: traits, behaviorist, contingency, and transformational ( Helland and Winston, 2005 ). According to Helland and Winston (2005) , all of these approaches have examined the traits and behaviors of leaders, how they employ power and influence, and how they adapt their behavior to particular situations. According to Van Maurik (2001) , none of these four approaches is mutually exclusive or is determined for a certain period of time only.
Bass and Riggio (2006) refer to the Leadership Practices Inventory (LPI) as a measurement instrument for transformational leadership taught in leadership development programs.
The seminal conception, empirical development, and psychometric validation of the LPI were the resulting work of Posner and Kouzes (1988) who based this approach from Burns (1978) . The original design of LPI relied on systematic feedback and interviews from participants that were surveyed with a set of behaviorally-based statements under Burn's ideas of Transformational leadership as contrasted with Transactional leadership . As per Burns (1978) , transformational leadership is a process in which leaders and followers help each other to reach a higher level of morale and motivation, whereas transactional leadership has more to do with the use of rewards and punishments to shape and promote followers' compliance. Existent meta-analyzes focusing on comparing both leadership styles have shown that transformational leadership seems to have stronger correlation with teams' productivity ( Lowe et al., 1996 ), commitment, role clarity, and well-being as compared with transactional leadership ( Tafvelin, 2013 ). More recent studies revealed that transformational leadership and the behavior of sharing knowledge proved to be uncorrelated from supervisors' perspective, whereas they are correlated from collaborators' perspective ( Durán and Castañeda, 2015 ).
The LPI evaluates five leadership behaviors that are considered exemplary behaviors of leaders ( Zárate-Torres and Matviuk, 2012 ; Kouzes and Posner, 2019 ). These behaviors are: 1) Challenging the process: This practice refers to questioning the status quo, seeking innovation, finding opportunities, taking risks, and learning from experience. 2) Inspire a shared vision: This practice refers to how often the leader shares or describes the vision to his followers if he involves his followers informing that vision and the passion with which he shares that vision. 3) Enabling others to act: This practice refers to empowering followers, fostering collaboration, and delegation. 4) Modeling the way: This practice refers to the example that the leader gives and the clarity in his values and his knowledge of himself and the consistency between what he says and what he does and how he lives and manifests his values. 5) Encouraging the heart: This last practice refers to the recognition made by the leader both public and private of the individual and group achievements.
1.3. The impact of personality on leadership
The personality-leadership relationship is perhaps one of the most relevant relationships in the contemporary literature of management ( Luong et al., 2021 ; Spark and O'Connor, 2021 ; Harvey and Green, 2022 ; Perret and Powers, 2022 ). According to Bass et al. (1990) , some definitions conceptualize leadership from a personality perspective that suggests that leadership is a combination of special traits or characteristics possessed by an individual, and they empower a person to influence others to achieve a goal. Nonetheless, in the work of Bass et al. (1990) , there is no single piece of empirical evidence that shows the direct impact that personality has on influencing others to achieve a common goal. As per Waite and McKinney (2015) , when one person recognizes his/her personality preferences using a self-assessment tool, he or she has a critical input that facilitates his/her leadership development. Thus, it becomes highly relevant to quantify the link between personality and leadership, for the following reasons.
According to Arévalo-Avecillas et al. ( 2019 ) the United States of America has produced most of the empirical studies tackling the relationship between personality and leadership, and although Norway, Australia, Singapore, and Canada have shown a few other set of studies on the correlation between these two constructs, no similar efforts are evident from developing countries ( Arévalo-Avecillas et al., 2019 ). In a recent study, Zárate-Torres et al. ( 2022 ) reported the scarcity of Latin-American studies focusing on the relationship between personality, gender, and leadership. Likewise, Chacón-Henao et al. ( 2022 ) reported that in emerging Latin-American countries such as Colombia, leadership studies have focused mainly on particular attributes and styles of individual leaders ( Hincapié-Montoya et al., 2018 ; Reyes Bastidas and Briano-Turrent, 2018 ; Gaviria-Rivera and López Zapata, 2019 ; Rojero-Jiménez et al., 2019 ). We regard these recent studies as evidence that shows how leadership studies in Colombia and Latin America countries deserve additional local efforts to unveil how leadership and personality proved to be linked when using tools such as the MBTI and the LPI.
When partitioning leadership into their behavioral practices and personality into their dichotomies, one can identify some patterns of interest. For example, Spark and O'Connor ( 2021 ) has provided evidence about the relationship between state extraversion and emergent leadership, as extraverts are more likely than introverts to emerge as leaders ( Colbert et al., 2012 ). In practical terms, this means that introverts face more difficulties in growing professionally and reaching leadership roles. This argument is also probed by the study of Luong et al. (2021) where they compared extraverts and introverts and the challenges they faced, suggesting that personality matters when leading. Another token that illustrates this point, is the study of Garland and Village (2021) who used MBTI to classify people in leadership positions and found that intuitive leaders are more visionaries that sensitive leaders (e.g., sensitives have clear guidelines on expectations roles and responsibilities, while intuitives find opportunities to participate in designing the future Myers and Mccaulley (1985) ). Likewise, Zhang et al. (2021) suggested that in order to enhance team effectiveness, managers should balance team members in terms of judgers and perceivers. For example, when all members are perceivers, they will face problems with deadlines definitions; in comparison, when all members are judgers they will find difficulties how to handle unexpected contingencies. Until this point, it should be evident that the personality-leadership link deserves scrutiny in its own right. A note of consideration is worth mentioning here. In real-world scenarios, a manager is more concerned with what works than with ultimate theoretical developments. Although such a vision is certainly distant from an orthodox academic perspective, its soundness in team performance is of highly relevance. For example, when a manager detects that his/her subordinates are more sensing (e.g., they follow the norms and procedures) than intuitive (i.e., they look for alternative ways to introduce innovations in the organization, regardless these innovations exist in current norms and procedures), the ideal leader understands how to work as a team with his/her followers.
To evidence the above, in the literature, several studies show that MBTI dichotomies relate to leaders' behaviors in general ( Connor et al., 2014 ; Uusi-Kakkuri and Brandt, 2015 ), transformational leaders' behavior in particular ( Brown and Reilly, 2009 ), and leadership practices as captured by LPI ( Zárate-Torres et al., 2022 ). All these behaviors have important implications for effective leadership. In their MBTI manual (chapter 13) Myers and Mccaulley (1985) , pinpoint several practical uses of this tool, such as improving communications in firms, conflict resolutions, enhancement of problem-solving and decision-making, planning, implementing, and managing organizational change, and improving teams' productivity.
2. Materials and methods
Our methodological approach is similar to previous efforts that explored the relationship between personality and leadership qualities ( Zhang et al., 2021 ), although it focuses on a different framework to address other goals. In the current article, we sampled 529 participants who were graduate and undergraduate students enrolled in business administration programs from Colombian universities. After data cleaning and preprocessing, we obtained a total of 464 valid observations of which 50.64 % were from men, and 48.06 % from women. 7.11 % of the participants were students in their last semester of their undergraduate program, while 91.6 % were graduate students. 15.94 % of the participants were between 15 and 21 years old, 47.41% aged between 26 and 35 years old, 24.56% were between 36 and 45 years old, and 10.77% were older than 46. Data collection relied on valid and already translated questionnaires in their Spanish version. Also, participants took the instruments at different times and days during class hours. Questionnaire administration was conducted by the principal investigator, who was always present in the classroom to answer any questions from participants and prevent confounding factors that could emerge during questionnaire administration and lead to problems of uncontrolled statistical variance ( Rodríguez-Ardura and Meseguer-Artola, 2020 ).
As compared with previous endeavors that explored leadership practices in relation to emotional intelligence from Colombian samples ( Zárate-Torres and Matviuk, 2012 ), we are unaware of specific studies tackling leadership practices in relation to personality dichotomies in Colombia. The focus on Colombia is highly relevant as compared with samples from other countries, because Colombia joined the organiczation for Economic Co-operation and Development, OECD, in April 28, 2020 ( Sakiru et al., 2022 ). Indeed, the so-called soft skills (e.g., leadership, teamwork, creativeness) are of paramount importance, as suggested by previous OECD reports ( OECD, 2016 ) because these skills allow the future generation of Colombian businessmen to compete in a more globalized context where Colombia is expected to leapfrog its current dynamic capacities. As individuals need to be trained in how to compete, higher education executive programs are the standard societal mechanism that paves the way for the next generation of managers to succeed ( Núñez et al., 2012 ). In our view, teaching leadership as a soft skill provides an incomplete landscape for students if the leadership-personality link is not clearly defined.
2.1. Data collection procedure
All participants were told about the purpose of this research and provided their consent agreement to participate in the study. We documented supplemental material following reproducible research standards to increase data transparency and results reproducibility ( Peikert and Brandmaier, 2021 ; Peer et al., 2022 ). We used MBTI and the Leadership Practices Inventory (LPI) as data collection techniques. The MBTI consists of 93 items and normally takes between 20 and 40 min to complete ( https://www.humanmetrics.com/personality/test ). The score of the questionnaire provides participants with their 4-letter personality type. The first type (P1) is Extraversion-Introversion , the second type (P2) is Intuition-Sensing , the third type (P3) is Feeling-Thinking , and the fourth type (P4) is Judgment-Perceiving . The LPI consist of 30 questions ( https://cpb-us-w2.wpmucdn.com/u.osu.edu/dist/5/60574/files/2018/05/LPI-plus-scoring-guide-203il6w.pdf ). These questions are distributed in the following five conceptual dimensions. The first dimension (L1) refers to challenging the process . The second dimension (L2) is inspiring a shared vision . The third dimension (L3) is enabling others to act . The fourth dimension (L4) is modeling the way , and the fifth dimension (L5) is encouraging the heart . Participants' responses were then tabulated in a standard data set that we used as computational input for statistical analyzes.
2.2. Statistical analyzes
The scrutiny followed in this article relies on the combination of bivariate correlation analyzes with the help of the R package psych ( Revelle, 2022 ) and conventional confirmatory factor analyzes proposed by Bollen (1989) and implemented with the help of the lavaan R package ( Rosseel, 2012 ). A note of consideration is worth mentioning here. It is widely accepted that for psychometric validation purposes, a standard approach is to rely on an exploratory factor analysis that reveals that the empirical structure is consistent with the theoretical structure and then apply a confirmatory factor analysis as an ultimate empirical test. This standard approach is only valid when the psychometric structure of the questionnaires or tests is unknown. When the psychometric structure of these tests is already known in advance, there is no need to rely on a preliminary exploratory factor analysis. In Bollen's terms “ in confirmatory factor analysis a model is constructed in advance, the number of latent variables is set by the analyst ” (p. 228).
We used the software Ωnyx ( von Oertzen et al., 2015 ) to specify and identify structural equation models on the personality-leadership relationship. The syntaxes produced by Ωnyx were then exported and statistically tested in RStudio. We also used the R package semTable ( Johnson and Kite, 2020 ) to facilitate the reporting of findings via L A T E Xreproducible documentation ( Gandrud, 2018 ).
We initialize the analysis by describing the univariate distribution of every single conceptual dimension for both the personality self-report of the Myers-Briggs Type Indicator (i.e., P1, P2, P3, and P4) and the Leadership Practices Inventory and its five dimensions (i.e., L1, L2, L3, L4, and L5). We observed that dichotomies assessing personality follow a Bernoulli distribution, while the practices assessing leadership follow a Binomial distribution. As these distributions are discrete and their parametric properties deviate from those of a Gaussian distribution, we evaluated their bivariate statistical behavior through the Spearman Correlation Matrix Plot as depicted in Figure 1 . As expected, all correlations among leadership practices proved to be statistically significant (0.50 ≥ ρ ≤ 0.73, p < 0.01). Although correlations between dichotomies assessing personality were also statistically significant, their magnitude proved to be lower (−0.2 ≥ ρ ≤ 0.18, p < 0.01) and one item (i.e., P1) was not correlated with the rest.
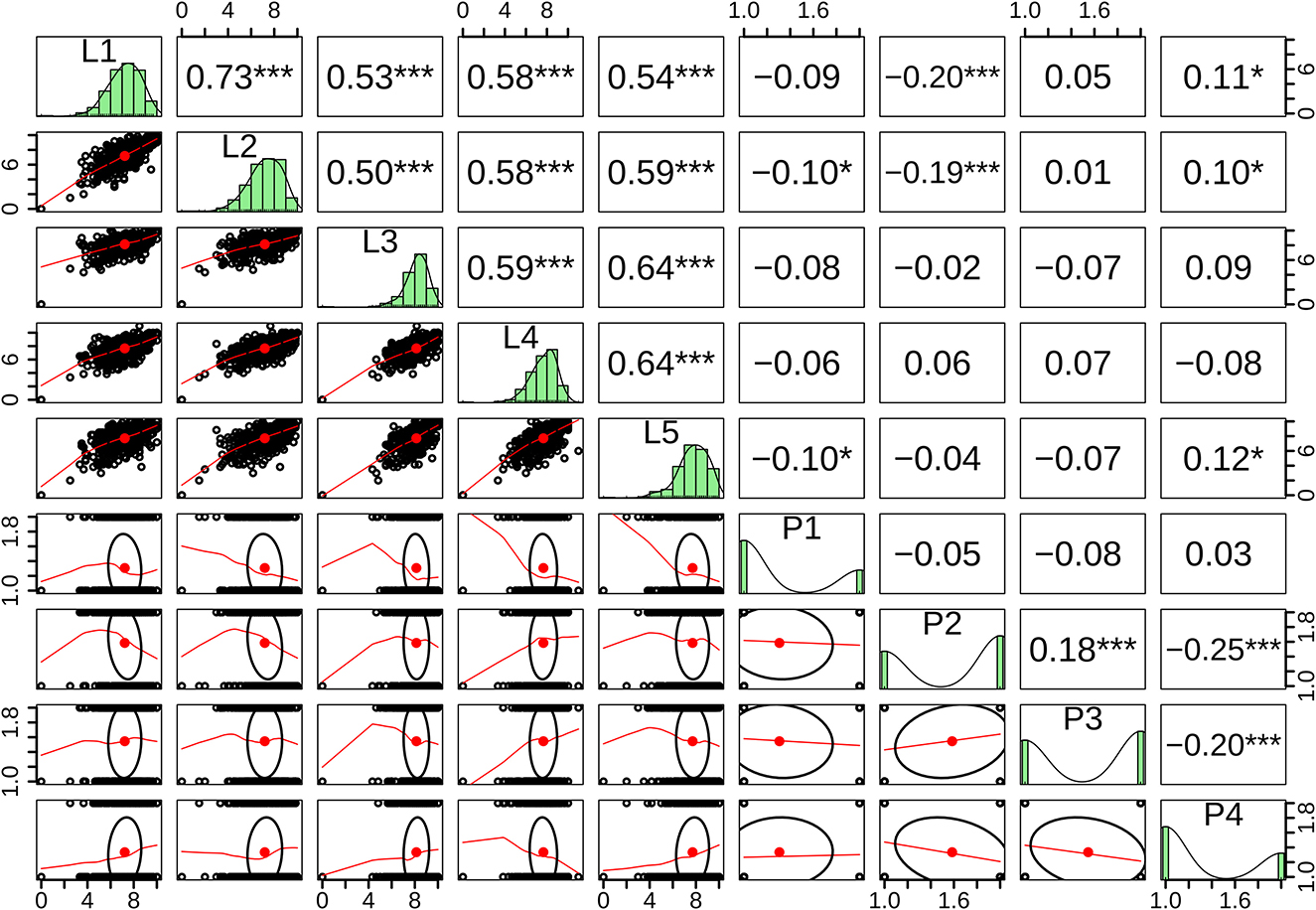
Figure 1 . Spearman correlation matrix plot for the five items of leadership and four items of personality. * the correlation is significant at 0.1; ** the correlation is significant at 0.05; *** the correlation is significant at or below 0.001.
The correlations above reveal interesting findings. Leadership practices share intermediate to high correlations among them. Nonetheless, MBTI dichotomies show low correlations among them. Above and beyond these patterns, it is worth mentioning how the leadership practices of challenging the process and inspiring a shared vision correlate with the MBTI dichotomy Intuition-Sensation . When people score high in these two leadership practices, they tend to fall as intuitive persons. In other words, intuitive persons tend to be more creative and this creativity allows them to challenge the processes around them. The Extraversion-Introversion dichotomy shows a low correlation with inspiring a shared vision and encouraging the heart . Particularly, leaders with higher scores on these two practices tend to be extroverted. We also noticed that the dichotomy Judgement-Perception shows a low positive correlation with two leadership practices: challenge the process and inspiring a shared vision , and a low negative correlation with encouraging the heart . This particular pattern of associations suggests that leaders with higher scores in the first two leadership practice tend to be perceivers while leaders with higher scores in encouraging the heart tend to be of judgement type. In order to test our main hypothesis that personality is a statistically significant predictor of leadership, we now proceed with reporting resulting confirmatory factor analyzes.
3.1. Confirmatory factor analyzes
Our first confirmatory factor analyzes (CFA) relate to the personality self-report questionnaire (see Figure 2A ). In Table 1 we present two statistical parameter estimations. In model 1, parameters were estimated through the Full Information Maximum Likelihood (FIML) estimation method, which can be regarded as a more restricted approach as it assumes that the observed indicators follow a continuous and multivariate normal distribution. In model 2, parameters were estimated through Diagonally Weighted Least Squares method (DWLS), which can be regarded as a less restricted estimation method ( Li, 2016 ).
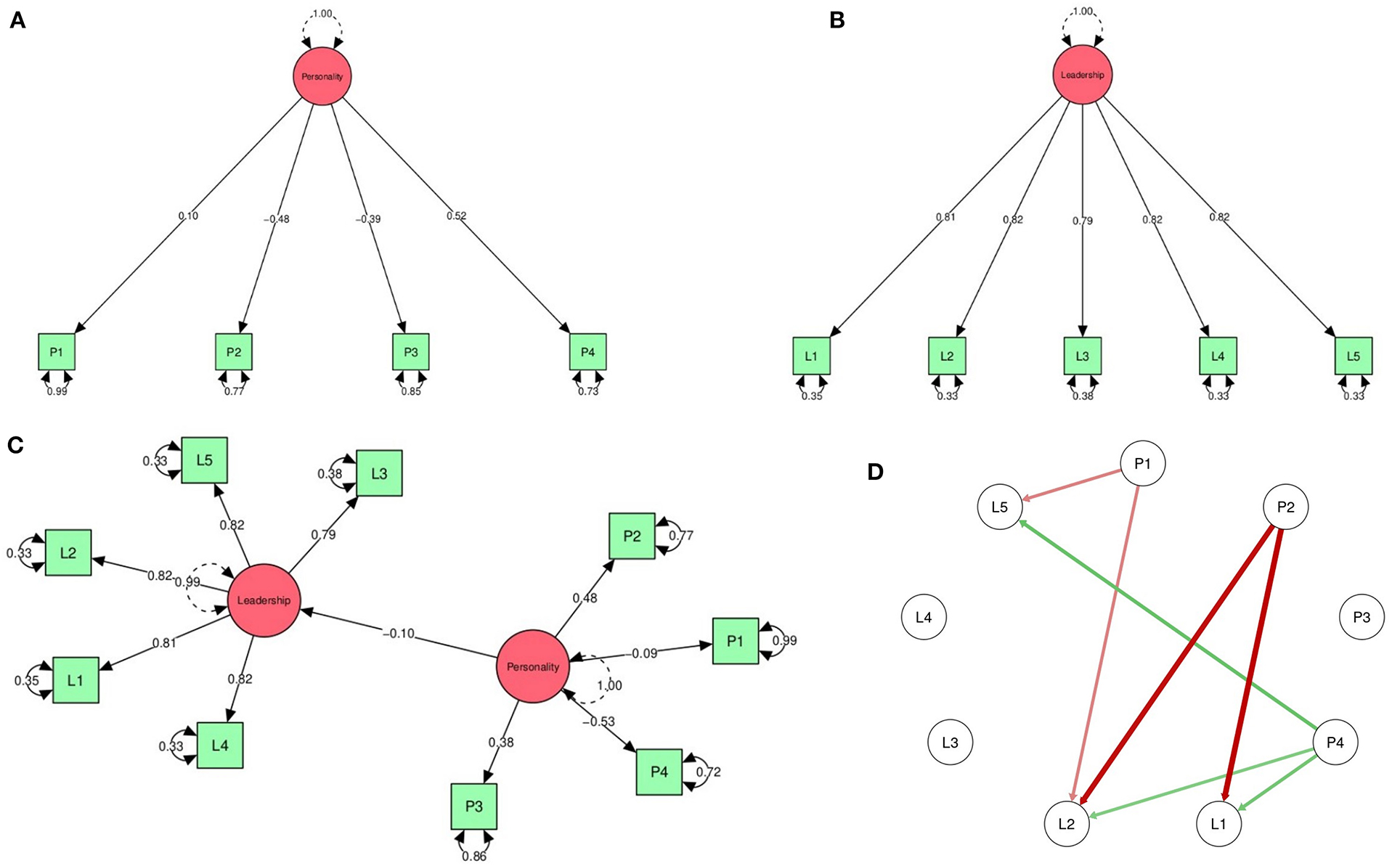
Figure 2 . Models of (A) confirmatory Factor analysis for personality, (B) confirmatory factor analysis for leadership, (C) structural personality-leadership model, and (D) network visualization.
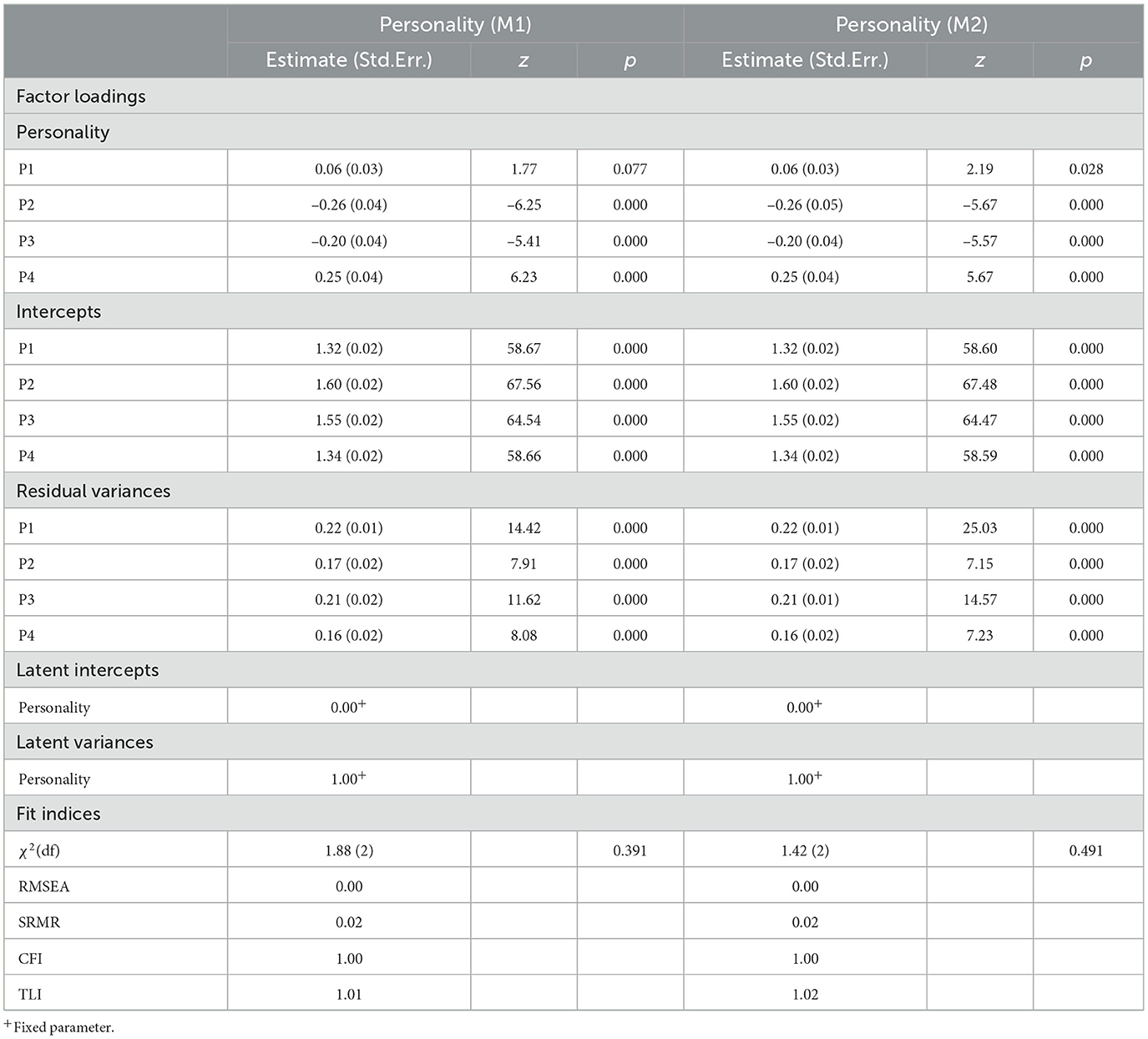
Table 1 . Statistical estimated parameters for personality CFA.
By considering traditional suggestions of cutoff criteria for fit indexes for structural equation models ( Hu and Bentler, 1999 ), the results of Table 1 proved to be satisfactory for both personality model estimations. Our second set of CFA results relate to the leadership practices inventory (see Figure 2B ). In Table 2 , we proceeded as before. Model 1 shows statistical parameters estimated through the full information maximum likelihood estimation method, and model 2 reports the same parameters estimated through diagonally weighted least squares. Here, the results of the CFA differ depending on the estimation method. Indeed, results from model 1 are far from being conclusive because at least two of its goodness-of-fit indexes suggest that data does not confirm the proposed theoretical structure [i.e., χ ( d f ) 2 = 74.07, p = 0.000 and RMSEA = 0.18]. Nonetheless, all goodness-of-fit indexes resulting from model 2 suggest that data confirms the proposed measurement model for leadership.
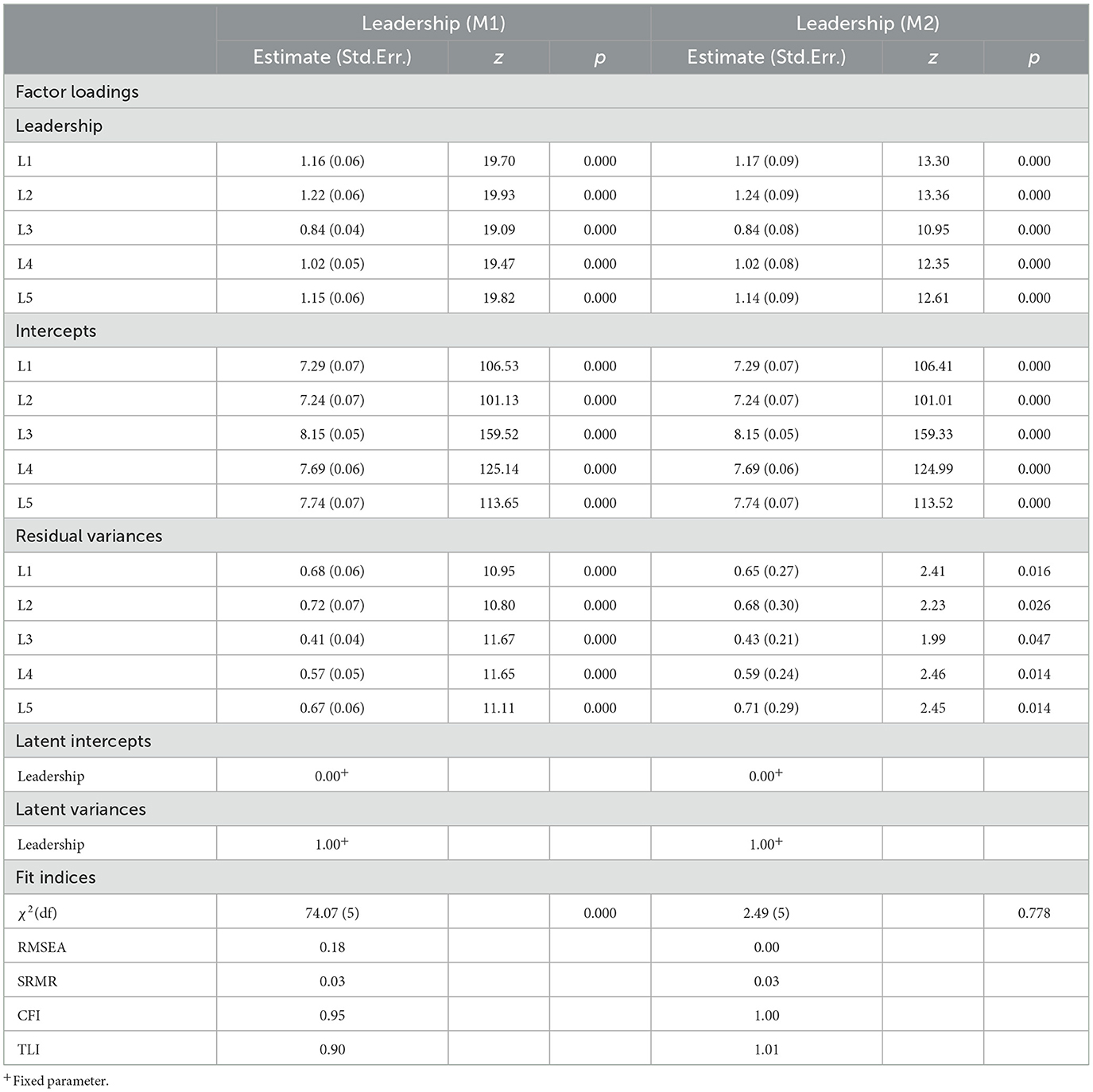
Table 2 . Statistical estimated parameters for leadership CFA.
3.2. Personality-leadership structural analyzes
After confirming the psychometric structure of our two constructs, we now switch the attention to their structural relationship (as depicted in Figure 2C and summarized in Table 3 ). As expected, Tables 1 , 2 show that all factor loadings remained statistically significant under both parametric estimation methods. Nonetheless, the personality-leadership structural relationship proved to be weak either under the FIML estimation method (Est = –0.10, standard error = 0.07, p < 0.01) or with the DWLS estimation method (Est = –0.11, standard error = 0.03, p < 0.01), as in either case the effect size that the personality has on leadership practices is about just one percent of explained variance (R 2 = 0.01). By depicting all conceptual dimensions from the leadership practices inventory and all personality dichotomies as nodes of a network, we revealed with red and green arrows all resulting relevant connections (as captured by their positive and negative correlations that proved to be statistically significant) between these two theoretical-related constructs (as illustrated in Figure 2D ).
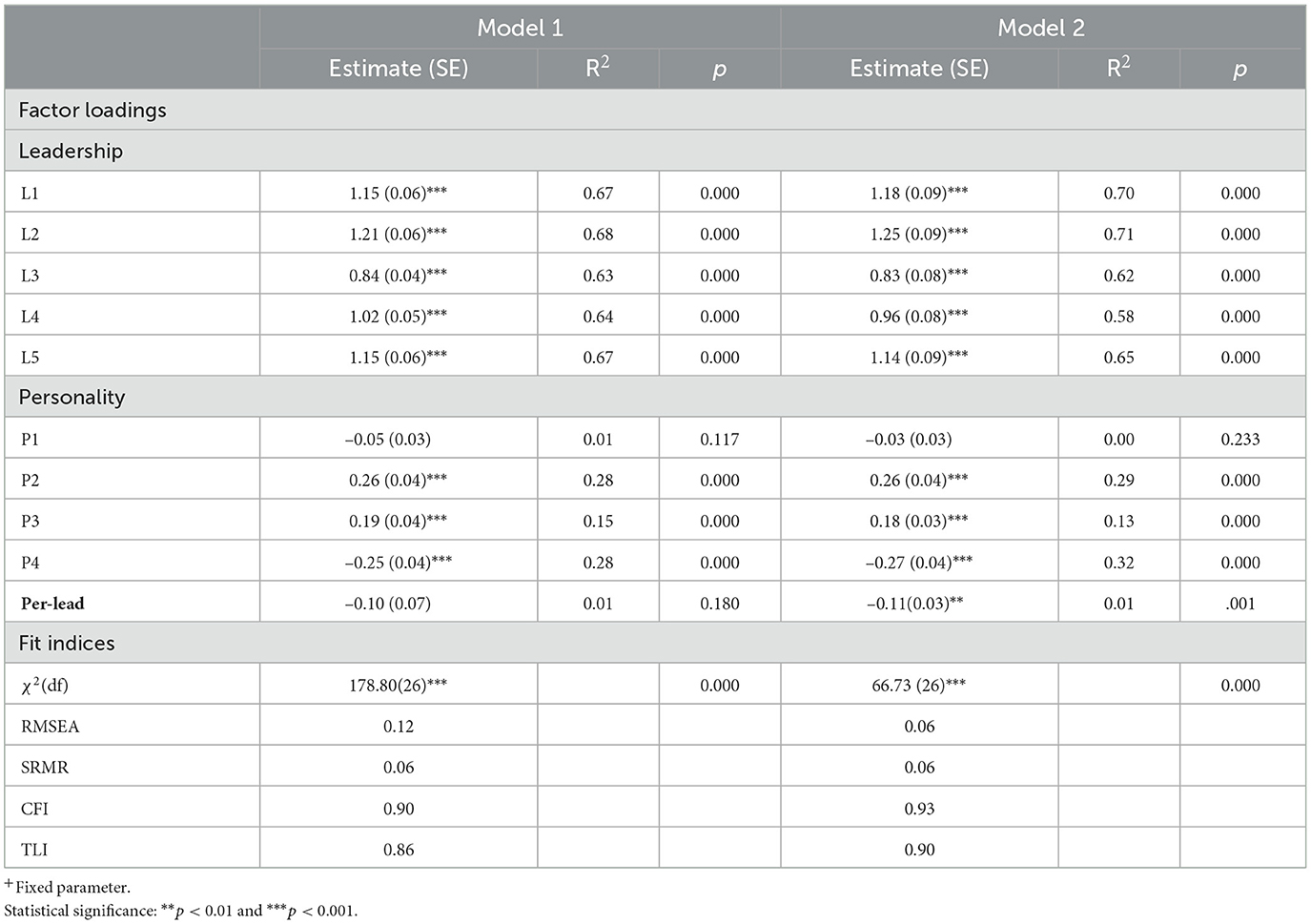
Table 3 . Statistical estimated parameters for the personality-leadership structural model.
4. Discussion
As noted throughout this article, the link between personality and leadership has important implications for organizations and is considered crucial in the management literature ( Bass et al., 1990 ). In this writing, we researched this relationship among hundreds of graduate and undergraduate students in Colombia. We provided fresh evidence of this relationship by assessing the association between the MBTI as a proxy for personality and the LPI as a proxy for leadership-related behaviors. We concluded that the relationship between these two constructs proves to be a weak one according to the standards used in the classic descriptions of summaries of research on psychological theories ( Meehl, 1990 ).
The findings of the present study confirm what other authors have said, that there is a relationship between the different personality traits displayed by individuals and their propensity to manifest or develop leadership related behaviors ( Bono and Judge, 2004 ; D'Alessio, 2008 ). By combining the four personality dichotomies from the perspective of the psychological types that inspired the MBTI ( Gardner and Martinko, 1996 ) with the five leadership practices from the transformational leadership theory ( Posner and Kouzes, 1988 ), we mapped and evaluated 20 possible theoretical relationships between these two constructs. Only seven out of these 20 potential theoretical relationships proved to be statistically significant, and based on these pairwise relationships, we paved the way to unveil unexplored insights for theory-building purposes.
We noticed that the first personality dichotomy (P1: Extraversion-Introversion ) only revealed a relevant connection with the second (L2: inspiring a shared vision ) and fifth leadership practice (L5: encouraging the heart ). This first relationship means extroverts inspire a shared vision more than introverts do. For the practice of encouraging the heart, extravert types are the ones that tend to motivate their followers more compared to introverts. These findings prove what Colbert et al. (2012) mentioned, that extravert types are more likely than introverts to emerge as leaders. In practical terms, this means that introverts face more difficulties in growing professionally and reaching leadership roles as they need help understanding and dealing whit this issue. A practical implication in this regard might be evident for introverts. They should learn how to express themselves without fearing vulnerable in front of others.
Likewise, the second dichotomy (P2: Intuition-Sensing ) revealed a relevant connection with the first (L1: challenge the process ) and second (L2: inspiring a shared vision ) leadership practices, which means that Intuitives tend to challenge the processes more than sensitives do. For the second practice, intuitive types are the ones that inspire a shared vision more than sensitives. These findings are aligned with the study of Garland and Village (2021) , who used the MBTI to classify people in leadership positions and found that intuitive leaders are more visionaries than sensitive type leaders, which relates with Myers and Mccaulley (1985) that mentioned that sensitives have clear guidelines on expected roles and responsibilities. In contrast, intuitive types find opportunities to participate in designing the future; that is, accepting the uncertainty in changing times.
Finally, the fourth personality dichotomy (P4: Judgment-Perceiving ) proved to be the most relevant personality type as it associates with encouraging the heart, inspiring a shared vision , and challenging the process . This final relationship means that perceivers are more likely to motivate their followers, describe a possible path for the future, and question the status quo than judgment types do. For example, when facing transitions, perceivers tend to facilitate required changes while judgers might avoid them as they opt for maintaining traditions.
These relevant and significant connections might be the psychological mechanisms psychologists, and other management practitioners use to promote self-awareness of their behaviors and boost team learning and development in organizational settings ( Costello, 1993 ; Penzias, 2020 ). In other words, these findings have a relevant significance to organizations. Theoretically and practically, any person can be a leader, but they must be aware of their personality to identify their strengths and weaknesses and strategically work on them to improve their leadership practices.
It is also interesting to note those connections that were not relevant, as they have to do with the third personality type (P3: Thinking-Feeling ) with the third (L3: enabling others to act ) and fourth leadership dimension (L4: modeling the way ). The lack of relevant relationships between these conceptual nodes might be fruitful in advancing our knowledge of the different mechanisms by which personality types affect leadership practices, which is promising for teaching leadership skills ( Shope et al., 2000 ). Suppose the way we make decisions (i.e., Thinking vs. Feeling) is an essential element that drives the willingness to become a transformational leader. In that case, this should facilitate leaders to interact with followers as a model that shows commitment to ideals and long-term goals, though this might not necessarily be an instantaneous process. In any case, the set of relationships that we examined in this article deserves other comments. To the best of our knowledge, previous works have focused on literature reviews ( Gardner and Martinko, 1996 ; Brown and Reilly, 2009 ) and the study of Bess and Harvey (2002) is an exception in terms of describing the statistical behavior of the bimodal empirical distributions that result from using this tool as a data collection technique. In this article, we resumed the conceptual ideas that pinpoint the link between leadership-related behaviors and the MBTI scores that describe personality dichotomies ( Waite and McKinney, 2015 ).
The current research is not free of limitations. Following Meehl's (1990) classic description of summaries of research on psychological theories, the fact of obtaining an effect size of 0.01 between MBTI and LPI should be interpreted as a weak connection that deserves systematic scrutiny from a longitudinal approach; that is, further research should unveil whether the reported effect size here remains statistically stable in other samples that might include university students from Colombia or any other Latin American country. Other limitations refer to the impossibility of assuming any causal relationship between personality and leadership, as the design of this work was not experimental. In this regard, we encourage future researchers to think about possible ways to design more controlled observations to infer causality from these two concepts.
For future studies, we suggest including other variables in the analysis. For example, supervisor experience (i.e., supervising and directing others) might be revealing as it provides another angle to the personality-leadership relationship. We are keenly aware that those with a longer supervisor experience might know how to regulate their behavior when they have to disagree with colleagues and/or persuade peers, supervisors, and collaborators.
Data availability statement
The datasets presented in the study can be found in online repositories. This data can be found here: https://github.com/jcorrean/MBTI-LEADERSHIP .
Ethics statement
The studies involving human participants were reviewed and approved by Colegio de Estudios Superiores-Ethical Committee. The patients/participants provided their written informed consent to participate in this study.
Author contributions
RZ-T conceived the study, collected the data, organized the database, wrote the manuscript, and wrote sections of the manuscript. JC cured the data, analyzed the data, and wrote the results along with the supplemental material. RZ-T and JC revised final version of the manuscript read and approved the submitted version.
Acknowledgments
We thank Colegio de Estudios Superiores de Administración in Bogotá Colombia, for providing the tools and facilities to write this article.
Conflict of interest
The authors declare that the research was conducted in the absence of any commercial or financial relationships that could be construed as a potential conflict of interest.
Publisher's note
All claims expressed in this article are solely those of the authors and do not necessarily represent those of their affiliated organizations, or those of the publisher, the editors and the reviewers. Any product that may be evaluated in this article, or claim that may be made by its manufacturer, is not guaranteed or endorsed by the publisher.
Arévalo-Avecillas, D., Padilla-Lozano, C., Pino, R., and Cevallos, H. (2019). Los dominios de la personalidad y su relación con el estilo de liderazgo transformacional. CIT Inform. Tecnol . 30, 237–248. doi: 10.4067/S0718-07642019000300237
PubMed Abstract | CrossRef Full Text | Google Scholar
Bass, B., and Riggio, R. (2006). Transformational Leadership . New York, NY: Taylor & Francis.
Google Scholar
Bass, B., Stogdill, R., and Press, F. (1990). Bass & Stogdill's Handbook of Leadership: Theory, Research, and Managerial Applications . New York, NY: Free Press.
Bess, T., and Harvey, R. (2002). Bimodal score distributions and the Myers-Briggs type indicator: fact or artifact? J. Pers. Assess . 78, 176–186. doi: 10.1207/S15327752JPA7801_11
Bollen, K. A. (1989). Structural Equations With Latent Variables . New York, NY: Wiley and Sons.
Bono, J. E., and Judge, T. A. (2004). Personality and transformational and transactional leadership: a meta-analysis. J. Appl. Psychol . 89, 901–910. doi: 10.1037/0021-9010.89.5.901
Brown, F., and Reilly, M. (2009). The myers-briggs type indicator and transformational leadership. J. Manag. Dev . 28, 916–932. doi: 10.1108/02621710911000677
Burns, J. (1978). Leadership . New York, NY: Harper and Row.
Chacón-Henao, J., López-Zapata, E., and Arias-Pérez, J. (2022). Liderazgo compartido en equipos directivos y desempeño organizacional: el rol mediador del capital social. Estud. Gerenc . 2022, 32–44. doi: 10.18046/j.estger.2022.162.4524
CrossRef Full Text | Google Scholar
Choong, E., and Varathan, K. (2021). Predicting judging-perceiving of Myers-Briggs Type Indicator (MBTI) in online social forum. PeerJ . 9, e11382. doi: 10.7717/peerj.11382
Colbert, A. E., Judge, T. A., Choi, D., and Wang, G. (2012). Assessing the trait theory of leadership using self and observer ratings of personality: the mediating role of contributions to group success. Leadership Q . 23, 670–685. doi: 10.1016/j.leaqua.2012.03.004
Connor, B., Bardol, D., and Fenster, M. (2014). Jungian personality types, gender, and preferred leadership style. Int. J. Educ. Organ. Leadership 20, 1–12. doi: 10.18848/2329-1656/CGP/v20i02/48477
Costello, K. (1993). The Myers-Briggs Type Indicator– a management tool. Nurs. Manag . 24, 46–51. doi: 10.1097/00006247-199305000-00010
D'Alessio, F. (2008). The influence of personality domains and working experience in Peruvian managers' leadership styles: an initial study. J. Cent. Cathedra 1, 13+.
Durán, M., and Castañeda, D. (2015). Relación entre liderazgo transformacional y transaccional con la conducta de compartir conocimiento en dos empresas de servicios. Acta Colombiana de Psicología 18, 135–147. doi: 10.14718/ACP.2015.18.1.13
Ertemel, A., and Çaylak, G. (2021). The effect of personality traits on credit score using Myers-Briggs Type Indicator (MBTI) personality types. Eur. Stud. Bus. Econ . 19, 185–199. doi: 10.1007/978-3-030-77438-7_11
Furnham, A., and Stringfield, P. (1993). Personality and occupational behavior: Myers-Briggs Type Indicator correlates of managerial practices in two cultures. Hum. Relations 46, 827–848. doi: 10.1177/001872679304600703
Gandrud, C. (2018). Reproducible Research With R and RStudio . New York, NY: Chapman and Hall/CRC.
Gardner, W., and Martinko, M. (1996). Using the Myers-Briggs type indicator to study managers: a literature review and research agenda. J. Manag . 22, 45–83. doi: 10.1177/014920639602200103
Garland, G., and Village, A. (2021). Psychological type profiles and temperaments of ministers in the Baptist Union of Great Britain (BUGB). Ment. Health Relig. Cult . 2021, 1–15. doi: 10.1080/13674676.2021.1908974
Gaviria-Rivera, J., and López Zapata, E. (2019). Transformational leadership, organizational climate and job satisfaction in work teams. Eur Res. Stud. J . 22, 68–82. doi: 10.35808/ersj/1457
Genina, A., Gawich, M., and Hegazy, A. (2021). An approach for sentiment analysis and personality prediction using Myers Briggs Type Indicator. Adv. Intell. Syst. Comput . 1261, 179–186. doi: 10.1007/978-3-030-58669-0_16
Hardwicke, T., Thibault, R., Kosie, J., Wallach, J., Kidwell, M., and Ioannidis, J. (2022). Estimating the prevalence of transparency and reproducibility-related research practices in psychology (2014–2017). Perspect. Psychol. Sci . 17, 239–251. doi: 10.1177/1745691620979806
Harvey, J.-F., and Green, J.P. (2022). Constructive feedback: When leader agreeableness stifles team reflexivity. Pers. Individual Diff. 194, 111624. doi: 10.1016/j.paid.2022.111624
Helland, M. R., and Winston, B. E. (2005). Towards a deeper understanding of hope and leadership. J. Leadership Organ. Stud . 12, 42–54. doi: 10.1177/107179190501200204
Hincapié-Montoya, S. M., Zuluaga-Correa, Y. C., and López-Zapata, E. (2018). Transformational leadership and continuous improvement in work teams of colombian smes. Rev. Venez. Gerenc. 23, 649–662.
Hu, L.-T., and Bentler, P. M. (1999). Cutoff criteria for fit indexes in covariance structure analysis: Conventional criteria versus new alternatives. Struct. Equ. Model . 6, 1–55. doi: 10.1080/10705519909540118
Johnson, P., and Kite, B. (2020). semTable: Structural Equation Modeling Tables . R package version 1.8.
Jung, C. G. (1923). Theory of the types. The Portable Jung, HG Baynes, trans . New York, NY: Penguin Books 1971.
King, S., and Mason, B (2020). “Myers Briggs type indicator,” in Wiley-Blackwell Encyclopedia of Personality and Individual Differences, Vol. II , eds B. J. Carducci, C. Nave, J. S. Mio, and R. E. Riggio (New York, NY: Wiley Blackwell).
Kouzes, T. K., and Posner, B. Z. (2019). Influence of managers' mindset on leadership behavior. Leadership Organ. Dev. J . 40, 829–844. doi: 10.1108/LODJ-03-2019-0142
Li, C.-H. (2016). The performance of ml, dwls, and uls estimation with robust corrections in structural equation models with ordinal variables. Psychol. Methods 21, 369. doi: 10.1037/met0000093
Lowe, K. B., Kroeck, K. G., and Sivasubramaniam, N. (1996). Effectiveness correlates of transformational and transactional leadership: a meta-analytic review of the mlq literature. Leadership Q . 7, 385–425. doi: 10.1016/S1048-9843(96)90027-2
Luong, V., Shields, C., Petrie, A., and Neumann, K. (2021). Does personality matter? perceptions and experiences of introverts and extraverts as general surgeons. Teach. Learn. Med. 34, 255–265. doi: 10.1080/10401334.2021.1922284
Ma, L., Guo, H., and Fang, Y. (2021). Analysis of Construction workers' safety behavior based on Myers-Briggs Type indicator personality test in a bridge construction project. J. Construct. Eng. Manag . 147, 1958. doi: 10.1061/(ASCE)CO.1943-7862.0001958
Mani, B. (1995). Progress on the journey to total quality management: using the myers-briggs type indicator and the adjective check list in management development. Public Pers. Manag . 24, 365–398. doi: 10.1177/009102609502400308
Meehl, P. E. (1990). Why summaries of research on psychological theories are often uninterpretable. Psychol. Rep . 66, 195–244. doi: 10.2466/pr0.1990.66.1.195
Myers, B. (1993). Introduction to Type. A guide to understanding your results on the Myers-Briggs Type Indicator . Palo Alto, CA: Consulting Psychologists Press.
Myers, B., and Mccaulley, I. (1985). Manual, A Guide to the Development and Use of the Myers-Briggs Type Indicator . Palo Alto, CA: Consulting Psychologists Press.
Myers, I. B., and Myers, P. B. (2010). Gifts Differing: Understanding Personality Type . Mountain View, CA: Nicholas Brealey.
Myers, S. (2016). Myers-briggs typology and jungian individuation. J. Anal. Psychol . 61, 289–308. doi: 10.1111/1468-5922.12233
Myers, S. (2019). Myers-Briggs Typology and Jungian Individuation . New York, NY: Overcoming One-Sidedness in Self and Society.
Núñez, J. A. L., El Homrani, M., and Martín, R. L. (2012). El liderazgo en los estudiantes universitarios: una fructífera línea de investigación. Educar 48, 91–119. doi: 10.5565/rev/educar.37
OECD (2016). Perspectivas económicas de América Latina 2017 . Paris: OECD Publishing.
Peer, L., Biniossek, C., Betz, D., and Christian, T.-M. (2022). Reproducible research publication workflow: A canonical workflow framework and fair digital object approach to quality research output. Data Intell . 2022, 133. doi: 10.1162/dint_a_00133
Peikert, A., and Brandmaier, A. M. (2021). A reproducible data analysis workflow with R markdown, git, make, and docker. Quant. Comput. Methods Behav. Sci . 1, 3763. doi: 10.5964/qcmb.3763
Penzias, A. (2020). Team learning and development using the Myers-Briggs type indicator ® and the clifton strengthsfinder ® . J. Radiol. Nurs . 39, 80–81. doi: 10.1016/j.jradnu.2019.12.013
CrossRef Full Text
Perret, C., and Powers, S (2022). An investigation of the role of leadership in consensus decision-making. J. Theor. Biol. 543, 111094. doi: 10.1016/j.jtbi.2022.111094
Pestana, J. V., and Codina, N. (2019). Being conscious of one's own heroism: an empirical approach to analyzing the leadership potential of future CEOs. Front. Psychol. 9, 2787. doi: 10.3389/fpsyg.2018.02787
Pestana, J. V., and Codina, N. (2020). Collective and individual sources of women's creativity: Heroism and psychological types involved in enhancing the talent of emerging leaders. Sustainability 12, 4414. doi: 10.3390/su12114414
Posner, B., and Kouzes, J. (1988). Development and validation of the leadership practices inventory. Educ. Psychol. Meas . 48, 483–496. doi: 10.1177/0013164488482024
Ramsoomair, F. (1994). Relating theoretical concepts to life in the classroom: applying the Myers-Briggs type indicator. J. Manag. Educ . 18, 111–116. doi: 10.1177/105256299401800110
Revelle, W. (2022). psych: Procedures for Psychological, Psychometric, and Personality Research . Northwestern University, Evanston, Illinois. R package version 2.2.5.
Reyes Bastidas, C., and Briano-Turrent, G. D. C. (2018). Las mujeres en posiciones de liderazgo y la sustentabilidad empresarial: evidencia en empresas cotizadas de colombia y chile. Estud. Gerenc . 34, 385–398. doi: 10.18046/j.estger.2018.149.2877
Rodríguez-Ardura, I., and Meseguer-Artola, A. (2020). Editorial: how to prevent, detect and control common method variance in electronic commerce research. J. Theor. Appl. Electron. Comm. Res . 15, i-v. doi: 10.4067/S0718-18762020000200101
Rojero-Jiménez, R., Gómez-Romero, J., and Quintero-Robles, L. (2019). El liderazgo transformacional y su influencia en los atributos de los seguidores en las mipymes mexicanas. Estud. Gerenc . 35, 3192. doi: 10.18046/j.estger.2019.151.3192
Rosseel, Y. (2012). lavaan: an R Package for structural equation modeling. J. Stat. Softw . 48, 1–36. doi: 10.18637/jss.v048.i02
Sahono, M., Sidiastahta, F., Shidik, G., Fanani, A., Muljono Nuraisha, S., and Lutfina, E. (2020). “Extrovert and introvert classification based on Myers-Briggs Type Indicator(MBTI) using support vector machine (SVM),” in 2020 International Seminar on Application for Technology of Information and Communication (iSemantic) (Semarang: IEEE), 572–577.
Sakiru, S., Gil-Alana, L., and Gonzalez-Blanch, M. (2022). Persistence of economic complexity in OECD countries. Physica A 603, 127860. doi: 10.1016/j.physa.2022.127860
Schweiger, D. (1985). Measuring managerial cognitive styles: on the logical validity of the Myers-Briggs Type Indicator. J. Bus. Res . 13, 315–328. doi: 10.1016/0148-2963(85)90004-9
Shope, T., Frohna, J., and Frohna, A. (2000). Using the Myers-Briggs Type Indicator (MBTI) in the teaching of leadership skills. Med. Educ . 34, 956. doi: 10.1046/j.1365-2923.2000.0784l.x
Spark, A., and O'Connor, P. (2021). State extraversion and emergent leadership: Do introverts emerge as leaders when they act like extraverts? Leadership Q. 32, 101474. doi: 10.1016/j.leaqua.2020.101474
Srivastava, S., John, O. P., Gosling, S. D., and Potter, J. (2003). Development of personality in early and middle adulthood: Set like plaster or persistent change? J. Pers. Soc. Psychol . 84, 1041. doi: 10.1037/0022-3514.84.5.1041
Tafvelin, S. (2013). The transformational leadership process: Antecedents, mechanisms, and outcomes in the social services . (Ph.D. thesis). Umeåuniversitet.
Uusi-Kakkuri, P., and Brandt, T. (2015). Preferred leadership behaviours by different personalities. Int. J. Bus. Global . 15, 461–474. doi: 10.1504/IJBG.2015.072518
Van Maurik, J. (2001). Writers on Leadership. Penguin Business . New York, NY: Penguin Books Limited.
von Oertzen, T., Brandmaier, A. M., and Tsang, S. (2015). Structural equation modeling with Ωnyx. Struct. Equ. Model . 22, 148–161. doi: 10.1080/10705511.2014.935842
Waite, R., and McKinney, N. (2015). Knowing your preference: The nexus of personality and leadership. Creat. Nurs . 21, 172–178. doi: 10.1891/1078-4535.21.3.172
Zárate-Torres, R., and Matviuk, S. (2012). Inteligencia emocional y prácticas de liderazgo en las organizaciones colombianas. Cuadernos de Administración 28, 91–104. doi: 10.25100/cdea.v28i47.76
Zárate-Torres, R., Rey-Sarmiento, F., Prada, R., and Acosta-Prado, J. (2022). Leadership style according to gender: differences based on the personality of men and women [estilo de liderazgo según el género: diferencias basadas en la personalidad de hombres y mujeres]. Revista Venezolana de Gerencia 27, 167–185. doi: 10.52080/rvgluz.27.7.12
Zavyalova, E., Posokhova, S., Lisovskaya, A., and Sokolov, D. (2021). Who studies management in Russia: Personality traits of students of master of business administration programs. Organizatsionnaya Psikhologiya 11, 162–187.
Zhang, W., Yang, A., Huang, L., Leung, D., and Lau, N. (2021). Correlation between the composition of personalities and project success in project-based learning among design students. Int. J. Technol. Design Educ . 32, 2873–2895. doi: 10.1007/s10798-021-09716-z
Zumbo, B., and Taylor, S. (1993). The construct validity of the extraversion subscales of the Myers-Briggs type indicator. Can. J. Behav. Sci . 25, 590–604. doi: 10.1037/h007884
Keywords: leadership, leadership practices inventory, LPI, MBTI, personality, reproducible research
Citation: Zárate-Torres R and Correa JC (2023) How good is the Myers-Briggs Type Indicator for predicting leadership-related behaviors? Front. Psychol. 14:940961. doi: 10.3389/fpsyg.2023.940961
Received: 10 May 2022; Accepted: 06 February 2023; Published: 02 March 2023.
Reviewed by:
Copyright © 2023 Zárate-Torres and Correa. This is an open-access article distributed under the terms of the Creative Commons Attribution License (CC BY) . The use, distribution or reproduction in other forums is permitted, provided the original author(s) and the copyright owner(s) are credited and that the original publication in this journal is cited, in accordance with accepted academic practice. No use, distribution or reproduction is permitted which does not comply with these terms.
*Correspondence: Rodrigo Zárate-Torres, rodrigo.zarate@cesa.edu.co
Disclaimer: All claims expressed in this article are solely those of the authors and do not necessarily represent those of their affiliated organizations, or those of the publisher, the editors and the reviewers. Any product that may be evaluated in this article or claim that may be made by its manufacturer is not guaranteed or endorsed by the publisher.
Personality Assessments: 10 Best Inventories, Tests, & Methods

Perhaps they respond differently to news or react differently to your feedback. They voice different opinions and values and, as such, behave differently.
If you respond with a resounding yes, we understand the challenges you face.
As more and more organizations diversify their talent, a new challenge emerges of how to get the best out of employees and teams of all personality configurations.
In this article, we embark on a whistle-stop tour of the science of personality, focusing on personality assessments to measure clients’ and employees’ character plus the benefits of doing so, before rounding off with practical tools for those who want to bolster their professional toolkits.
Before you continue, we thought you might like to download our three Strengths Exercises for free . These detailed, science-based exercises will help your clients or employees realize their unique potential and create a life that feels energizing and authentic.
This Article Contains
What are personality assessments in psychology, 4 methods and types of personality assessments, 7 evidence-based inventories, scales, and tests.
- Helpful Tools & Questions
Fascinating Books About Personality Assessments
Resources from positivepsychology.com, a take-home message.
Personality is a tricky concept to define in concrete terms, and this is reflected both in the number of personality theories that exist and the lack of consensus among personality psychologists.
However, for this article, we can think of personality as the totality of one’s behavioral patterns and subjective experiences (Kernberg, 2016).
All individuals have a constellation of traits and experiences that make them unique yet simultaneously suggest that there are some generalizable or distinct qualities inherent in all humans.
In psychology, we are interested in understanding how traits and qualities that people possess cluster together and the extent to which these vary across and within individuals.
Now, it’s all very well and good knowing that personality exists as a concept and that your employees and clients differ in their groupings of traits and subjective experiences, but how can you apply this information to your professional work with them?
This is where measuring and assessing personality comes into play. Like most psychological concepts, researchers want to show that theoretical knowledge can be useful for working life and brought to bear in the real world.
For example, knowing a client’s or employee’s personality can be key to setting them up for success at work and pursuing and achieving work-related goals. But we first need to identify or assess personality before we can help others to reap these benefits.
Personality assessments are used for several reasons.
First, they can provide professionals with an opportunity to identify their strengths and reaffirm their sense of self. It is no coincidence that research on strengths is so popular or that strengths have such a prominent place in the working world. People like to know who they are, and they want to capitalize on the qualities and traits they possess.
Second, personality assessments can provide professionals with a social advantage by helping them to understand how they are perceived by others such as colleagues, managers, and stakeholders — the looking glass self (Cooley, 1902).
In the sections below, we will explore different personality assessments and popular evidence-based scales.

1. Self-report assessments
Self-reports are one of the most widely used formats for psychometric testing. They are as they sound: reports or questionnaires that a client or employee completes themselves (and often scores themselves).
Self-report measures can come in many formats. The most common are Likert scales where individuals are asked to rate numerically (from 1 to 7 for example) the extent to which they feel that each question describes their thoughts, feelings, or behaviors.
These types of assessments are popular because they are easy to distribute and complete, they are often cost effective, and they can provide helpful insights into behavior.
However, they also have downsides to be wary of, including an increase in unconscious biases such as the social desirability bias (i.e., the desire to answer “correctly”). They can also be prone to individuals not paying attention, not answering truthfully, or not fully understanding the questions asked.
Such issues can lead to an inaccurate assessment of personality. Self-reports can be completed in both personal and professional settings and can be particularly helpful in a coaching practice, for example.
However, if you are a professional working with clients in any capacity, it is advised to first try out any self-report measure before suggesting them to clients. In this way, you can gauge for yourself the usefulness and validity of the measure.
2. Behavioral observation
Another useful method of personality assessment is behavioral observation. This method entails someone observing and documenting a person’s behavior.
While this method is more resource heavy in terms of time and requires an observer (preferably one who is experienced and qualified in observing and coding the behavior), it can be useful as a complementary method employed alongside self-reports because it can provide an external corroboration of behavior.
Alternatively, behavioral observation can fail to corroborate self-report scores, raising the question of how reliably an individual has answered their self-report.
3. Interviews
Interviews are used widely from clinical settings to workplaces to determine an individual’s personality. Even a job interview is a test of behavioral patterns and experiences (i.e., personality).
During such interviews, the primary aim is to gather as much information as possible by using probing questions. Responses should be recorded, and there should be a standardized scoring system to determine the outcome of the interview (for example, whether the candidate is suitable for the role).
While interviews can elicit rich data about a client or employee, they are also subject to the unconscious biases of the interviewers and can be open to interpretation if there is no method for scoring or evaluating the interviewee.
4. Projective tests
These types of tests are unusual in that they present individuals with an abstract or vague object, task, or activity and require them to describe what they see. The idea here is that the unfiltered interpretation can provide insight into the person’s psychology and way of thinking.
A well-known example of a projective test is the Rorschach inkblot test. However, there are limitations to projective tests due to their interpretative nature and the lack of a consistent or quantifiable way of coding or scoring individuals’ responses.
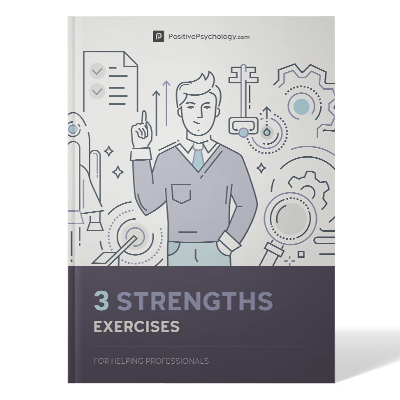
Download 3 Free Strengths Exercises (PDF)
These detailed, science-based exercises will equip you or your clients with tools to discover and harness their unique strengths.
Download 3 Free Strengths Tools Pack (PDF)
By filling out your name and email address below.
Personality assessments can be used in the workplace during recruitment to gauge whether someone would be a good fit for a job or organization and to help determine job performance, career progression, and development.
Below, we highlight a few commonly used inventories and tests for such career assessments.
1. The Hogan personality inventory (HPI)
The Hogan personality inventory (Hogan & Hogan, 2002) is a self-report personality assessment created by Robert Hogan and Joyce Hogan in the late 1970s.
It was originally based on the California Personality Inventory (Gough, 1975) and also draws upon the five-factor model of personality. The five-factor model of personality suggests there are five key dimensions of personality: openness to experience , conscientiousness, extraversion, agreeableness, and neuroticism (Digman, 1990).
The Hogan assessment comprises 206 items across seven different scales that measure and predict social behavior and social outcomes rather than traits or qualities , as do other popular personality measures.
These seven scales include:
- Sociability
- Interpersonal sensitivity
- Inquisitiveness
- Learning approach
The HPI’s primary use is within organizations to help with recruitment and the development of leaders . It is a robust scale with over 40 years of evidence to support it, and the scale itself takes roughly 15–20 minutes to complete (Hogan Assessments, n.d.).
2. DISC test
The DISC test of personality developed by Merenda and Clarke (1965) is a very popular personality self-assessment used primarily within the corporate world. It is based on the emotional and behavioral DISC theory (Marston, 1928), which measures individuals on four dimensions of behavior:
The self-report comprises 24 questions and takes roughly 10 minutes to complete. While the test is simpler and quicker to complete than other popular tests (e.g., the Myers-Briggs Type Indicator), it has been subject to criticism regarding its psychometric properties.
3. Gallup – CliftonStrengths™ Assessment
Unlike the DISC test, the CliftonStrengths™ assessment , employed by Gallup and based on the work of Marcus Buckingham and Don Clifton (2001), is a questionnaire designed specifically to help individuals identify strengths in the workplace and learn how to use them.
The assessment is a self-report Likert scale comprising 177 questions and takes roughly 30 minutes to complete. Once scored, the assessment provides individuals with 34 strength themes organized into four key domains:
- Strategic thinking
- Influencing
- Relationship building
The scale has a solid theoretical and empirical grounding, making it a popular workplace assessment around the world.
4. NEO-PI-R
The NEO-PI-R (Costa & McCrae, 2008) is a highly popular self-report personality assessment based on Allport and Odbert’s (1936) trait theory of personality.
With good reliability, this scale has amassed a large evidence base, making it an appealing inventory for many. The NEO-PI-R assesses an individual’s strengths, talents, and weaknesses and is often used by employers to identify suitable candidates for job openings.
It uses the big five factors of personality (openness, conscientiousness, extraversion, agreeableness, and neuroticism) and also includes an additional six subcategories within the big five, providing a detailed breakdown of each personality dimension.
The scale itself comprises 240 questions that describe different behaviors and takes roughly 30–40 minutes to complete. Interestingly, this inventory can be administered as a self-report or, alternatively, as an observational report, making it a favored assessment among professionals.
5. Eysenck Personality Questionnaire (EPQ)
The EPQ is a personality assessment developed by personality psychologists Hans Eysenck and Sybil Eysenck (1975).
The scale results from successive revisions and improvements of earlier scales: the Maudsley Personality Inventory (Eysenck 1959) and Eysenck Personality Inventory (Eysenck & Eysenck, 1964).
The aim of the EPQ is to measure the three dimensions of personality as espoused by Eysenck’s psychoticism–extraversion–neuroticism theory of personality The scale itself uses a Likert format and was revised and shortened in 1992 to include 48 items (Eysenck & Eysenck, 1992).
This is a generally useful scale; however, some researchers have found that there are reliability issues with the psychoticism subscale, likely because this was a later addition to the scale.
6. Minnesota Multiphasic Personality Inventory (MMPI)
The MMPI (Hathaway & McKinley, 1943) is one of the most widely used personality inventories in the world and uses a true/false format of questioning.
It was initially designed to assess mental health problems in clinical settings during the 1940s and uses 10 clinical subscales to assess different psychological conditions.
The inventory was revised in the 1980s, resulting in the MMPI-2, which comprised 567 questions, and again in 2020, resulting in the MMPI-3, which comprises a streamlined 338 questions.
While the revised MMPI-3 takes a lengthy 35–50 minutes to complete, it remains popular to this day, particularly in clinical settings, and enables the accurate capture of aspects of psychopathy and mental health disturbance. The test has good reliability but must be administered by a professional.
7. 16 Personality Factor Questionnaire (16PF)
The 16PF (Cattell et al., 1970) is another rating scale inventory used primarily in clinical settings to identify psychiatric disorders by measuring “normal” personality traits.
Cattell identified 16 primary personality traits, with five secondary or global traits underneath that map onto the big five factors of personality.
These include such traits as warmth, reasoning, and emotional stability, to name a few. The most recent version of the questionnaire (the fifth edition) comprises 185 multiple-choice questions that ask about routine behaviors on a 10-point scale and takes roughly 35–50 minutes to complete.
The scale is easy to administer and well validated but must be administered by a professional.
Helpful Tools & Questions

We share two informative videos on this topic and then move on to a short collection of questions that can be used for career development.
1. Myers-Briggs Type Indicator (MBTI)
Many of us have heard of the Myers-Briggs Type Indicator (Myers & McCaulley, 1985), and for good reason. It is one of the most popular and widely used personality assessments out there.
A mother and daughter team developed the MBTI in the 1940s during the Second World War. The MBTI comprises 93 questions that aim to measure an individual on four different dimensions of personality:
- Introversion/extraversion
- Sensing/ intuition
- Thinking/feeling
- Judging/perceiving
The test provides individuals with a type of personality out of a possible 16 combinations. Whilst this test is a favorite in workplaces, there are serious criticisms leveled at how the scale was developed and the lack of rigorous evidence to support its use.
For more information on the MBTI, you might enjoy the below videos:
We recommend that if you employ MBTI, be mindful of its scientific deficiencies and support your personality testing further by completing an additional validated scale.
10 Career development questions
- Tell me about what inspires you. What gets you out of bed in the morning?
- Tell me about your vision for your career/life.
- What aspects of your role do you love? What aspects do you struggle with?
- Tell me about a time where you used your strengths to achieve a positive outcome.
- Are there any healthy habits you want to build into your work life?
- Describe your perfect working day. What would it look like?
- Tell me about your fears.
- What do you value most about your job?
- What goals are you currently working toward?
- How would your work colleagues describe you?
If you are interested in learning more about personality and personality assessments, the following three books are an excellent place to start.
These books were chosen because they give an excellent overview of what personality is and how it can be measured. They also illuminate some issues with personality assessments. They provide a good grounding for any professional looking to implement personality assessments in the workplace.

1. Mindset: Changing the Way You Think to Fulfil Your Potential – Carol Dweck

Enter Dr. Carol Dweck and several decades of psychological research she has conducted on motivation and personality.
The main thesis of the book is to explore the idea that people can have either a fixed or growth mindset (i.e., beliefs we hold about ourselves and the world around us). Adopting a growth mindset can be a critical determinant of outcomes such as performance and academic success.
Find the book on Amazon .
2. The Personality Brokers: The Strange History of Myers-Briggs and the Birth of Personality Testing – Merve Emre

If you are interested in the dark side of psychology assessments, this is the book for you.
This book explores how the Myers-Briggs Type Indicator was developed and discusses the questionable validity of the scale despite its widespread popularity in the corporate world.
While many assessments can be helpful for self-reflecting on your own behavior, The Personality Brokers delve into the murky side of how psychological concepts can be used for monetary gains, even when evidence is lacking or disputed.
3. Psychological Types – Carl Jung

This is an excellent book from one of history’s most influential psychologists: Carl Jung.
The book focuses most on extraversion and introversion as the two key types of personality and also discusses the limitations of categorizing individuals into “types” of personality.
For those interested in the science of personality and who prefer a slightly heavier, academic read, this book is for you.
Interested in supplementing your professional life by exploring personality types? Here at PositivePsychology.com, we have several highly useful resources.
Maximizing Strengths Masterclass©
While strengths finding is a distinct and popular topic within positive psychology, we can draw parallels between strengths research and some conceptualizations of personality.
The Maximizing Strengths Masterclass© is designed to help clients reach their potential by looking at their strengths and what energizes them and helping them delve into their authentic selves. As a six-module coaching package, it includes 19 videos, a practitioner handbook, slide presentations, and much more.
Recommended Reading
For more information on personality psychology and personality assessments, check out the following related articles.
- Big Five Personality Traits: The OCEAN Model Explained
- Personality Psychology Explained: 7 Theories and Assessments
- Personality & Character Traits: The Good, the Bad, the Ugly
- Personal Strengths Defined (+ List of 92 Personal Strengths)
17 Career exercises
Designed to help people use their personality and strengths at work, this collection of 17 work and career coaching exercises is grounded in scientific evidence. The exercises help individuals and clients identify areas for career growth and development. Some of these exercises include:
- Achievement Story Chart your successes at work, take time to reflect on your achievements, and identify how to use your strengths for growth.
- Job Analysis Through a Strengths Lens Identify your strengths and opportunities to use them when encountering challenges at work.
- Job Satisfaction Wheel Complete the job satisfaction wheel, which measures your current levels of happiness at work across seven different dimensions.
- What Work Means to You Identify how meaningful your work is to you by assessing your motivational orientation toward work (i.e., whether it is something you are called to and that aligns with your sense of self).
If you’re looking for more science-based ways to help others develop their strengths, this collection contains 17 strength-finding tools for practitioners. Use them to help others better understand and harness their strengths in life-enhancing ways.
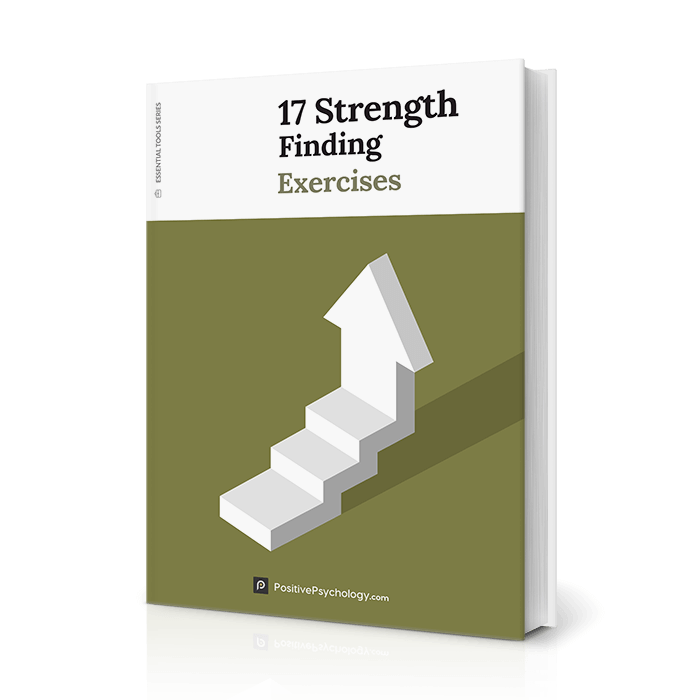
17 Exercises To Discover & Unlock Strengths
Use these 17 Strength-Finding Exercises [PDF] to help others discover and leverage their unique strengths in life, promoting enhanced performance and flourishing.
Created by Experts. 100% Science-based.
When managing people, it is always helpful to have insight into why they behave the way they do. The same applies to assisting someone on their career path. Having an understanding of the qualities that influence behavioral responses can improve relationships, parenting, how people work, and even goal setting.
But there are some caveats to be mindful of:
- When using self-reports, take the scores with a pinch of salt, particularly as we all operate with unconscious biases that can skew results.
- Remain open minded about our personality traits; if we are resigned to the idea that they are inherited at birth, fixed, and unchanging, we are unlikely to gain any real discernment into our own evolving identity.
- Labels can oftentimes be limiting. Trying to condense the myriad aspects of an individual into a neat “personality” category could backfire.
In the right hands, validated personality assessments are valuable tools for guiding clients on the right career path, ensuring a good job fit and building strong teams.
We hope you enjoyed reading this article. Don’t forget to download our three Strengths Exercises for free .
- Allport, G. W., & Odbert, H. S. (1936). Trait-names: A psycho-lexical study. Psychological Monographs , 47 (1), i–171.
- Buckingham, M., & Clifton, D. O. (2001). Now, discover your strengths . Simon and Schuster.
- Cattell, R. B., Eber, H. W., & Tatsuoka, M. M. (1970). Handbook for the Sixteen Personality Factor Questionnaire . Institute for Personality and Ability Testing.
- Cooley, C. H. (1902). Human nature and the social order . Transaction.
- Costa, P. T., Jr., & McCrae, R. R. (2008). The Revised NEO Personality Inventory (NEO-PI-R) . In G. J. Boyle, G. Matthews, & D. H. Saklofske (Eds.), The SAGE handbook of personality theory and assessment, Vol. 2. Personality measurement and testing (pp. 179–198). SAGE.
- Digman, J. M. (1990). Personality structure: Emergence of the five-factor model. Annual Review of Psychology , 41 (1), 417–440.
- Eysenck, H. J. (1959). Manual of the Maudsley Personality Inventory . University of London Press.
- Eysenck, H. J., & Eysenck, S. B. G. (1964). Manual of the Eysenck Personality Inventory . University of London Press.
- Eysenck, H. J., & Eysenck, S. B. G. (1975). Manual of the Eysenck Personality Questionnaire . Educational and Industrial Testing Service.
- Eysenck, H. J., & Eysenck, S. B. G. (1992). Manual for the Eysenck Personality Questionnaire–Revised . Educational and Industrial Testing Service.
- Gough, H. G. (1975). Manual: The California Psychological Inventory (Rev. ed.). Consulting Psychologist Press.
- Hathaway, S. R., & McKinley, J. C. (1943). The Minnesota Multiphasic Personality Inventory (Rev. ed., 2nd printing). University of Minnesota Press.
- Hogan Assessments. (n.d.). About. Retrieved May 8, 2023, from https://www.hoganassessments.com/about/.
- Hogan, R., & Hogan, J. (2002). The Hogan personality inventory. In B. de Raad & M. Perugini (Eds.), Big five assessment (pp. 329–346). Hogrefe & Huber.
- Kernberg, O. F. (2016). What is personality? Journal of Personality Disorders , 30 (2), 145–156.
- Marston, W. M. (1928). Emotions of normal people . Kegan Paul Trench Trubner and Company.
- Merenda, P. F., & Clarke, W. V. (1965). Self description and personality measurement. Journal of Clinical Psychology, 21 , 52–56.
- Myers, I. B., & McCaulley, M. H. (1985). Manual: A guide to the development and use of the Myers-Briggs Type Indicator . Palo Alto Consulting Psychologists Press.

Share this article:
Article feedback
What our readers think.

I learned so much. This article gave me more food for thought.
Let us know your thoughts Cancel reply
Your email address will not be published.
Save my name, email, and website in this browser for the next time I comment.
Related articles
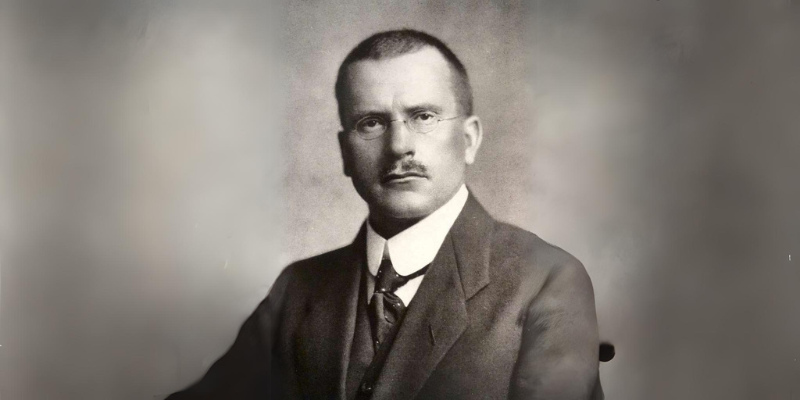
Jungian Psychology: Unraveling the Unconscious Mind
Alongside Sigmund Freud, the Swiss psychiatrist and psychoanalyst Carl Gustav Jung (1875–1961) is one of the most important innovators in the field of modern depth [...]
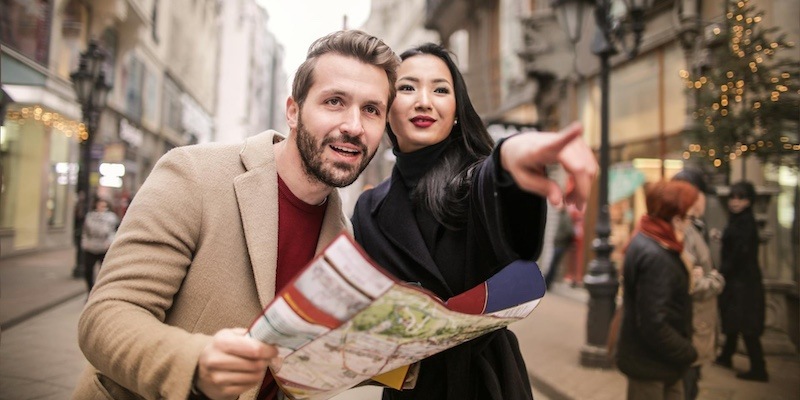
12 Jungian Archetypes: The Foundation of Personality
In the vast tapestry of human existence, woven with the threads of individual experiences and collective consciousness, lies a profound understanding of the human psyche. [...]
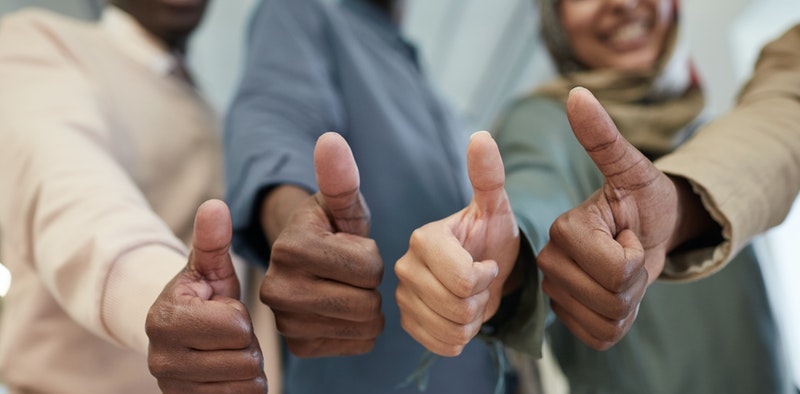
What Are the Hogan Assessments? How to Interpret the Results
A company’s performance is built on effective individuals and highly integrated teams. Working and performing well together and employee satisfaction and wellbeing are crucial to [...]
Read other articles by their category
- Body & Brain (53)
- Coaching & Application (58)
- Compassion (25)
- Counseling (51)
- Emotional Intelligence (23)
- Gratitude (18)
- Grief & Bereavement (21)
- Happiness & SWB (40)
- Meaning & Values (26)
- Meditation (20)
- Mindfulness (44)
- Motivation & Goals (46)
- Optimism & Mindset (34)
- Positive CBT (30)
- Positive Communication (22)
- Positive Education (48)
- Positive Emotions (32)
- Positive Leadership (19)
- Positive Parenting (16)
- Positive Psychology (34)
- Positive Workplace (37)
- Productivity (18)
- Relationships (45)
- Resilience & Coping (39)
- Self Awareness (21)
- Self Esteem (38)
- Strengths & Virtues (32)
- Stress & Burnout Prevention (34)
- Theory & Books (46)
- Therapy Exercises (37)
- Types of Therapy (64)
The world’s most powerful career test
Using advanced machine learning, psychometrics, and career satisfaction data, we’ve reimagined what a career test can be.
By the numbers
Discover your top career matches using careerexplorer’s cutting-edge science, rapidly-advancing platform.
Our machine learning models train on millions of data points, constantly improving the reliability and validity of our career test results.
Comprehensive model
We provide career matches based on your interests, goals, history, workplace preferences, and personality.
Intelligent enhancements
We continuously tweak our algorithms and update our datasets to provide industry-leading match accuracy.
Up-to-date information
We update our database of 1500+ career and degree profiles constantly to reflect the ever-changing world of work.
Get personalized recommendations
Learn as you go.
Each part of the career test unlocks new discoveries — insights into what makes you unique and what career paths you’ll find most fulfilling, building up to your final results.
Insights worth mentioning
Share your discoveries with friends and colleagues.
Are you a Visionary? Scholar? Builder? Share your personality archetype and career matches with your community with one simple click.
Work on your schedule
Complete the career test on your time.
Our career test is thorough to give you the most accurate results, not just a quick, generic snapshot. Take the test on your phone, at your desk, on a commute — and come back to it anytime. You can always pick up where you leave off.
How We Determine Your Matches
Four dimensions of career fit.
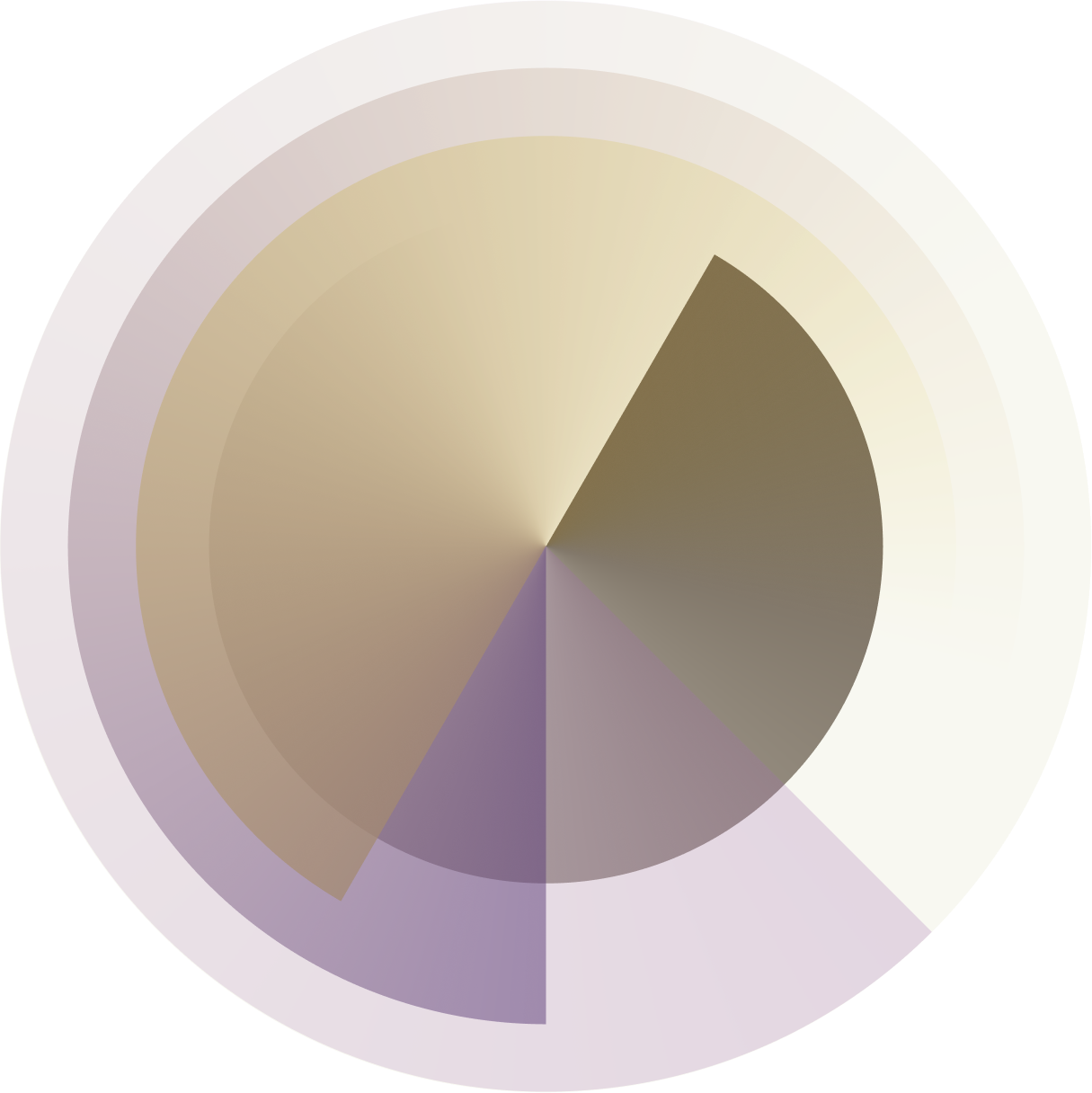
Workplace 01
Will the typical workplace of this career make me happy?
Whether you’re in an office or outside, working on a team or independently, your environment is a large contributor to your at-work happiness.
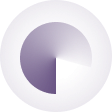
Will my past work and education help me get a job in this career?
Where you live, your experiences, and your education are important to take into consideration when choosing a career.
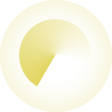
Interests 03
Will I find this career interesting?
Research shows that interest in a career or field is the number one predictor of career satisfaction.
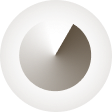
Personality 04
Will I be good at this career?
Personality fit with a career is one of the best predictors of on-the-job performance.
Whether you’re pursuing education, in the early career stages of your career, pivoting to a new path, or just looking for answers, we’re here to help you discover where you’ll find happiness in the world of work.
How our career test works, measure who you are.
We ask you a series of questions that correspond to 150+ personality traits and learn about your career interests, skills, and goals.
Calculate career attributes
We produce detailed profiles of 1,500+ careers and degrees based on labor market data, psychometric profiles, and other users’ reported happiness in them.
Compare the data
We compare these two datasets to determine your compatibility with the careers and degrees in our database.
Determine your top career matches
We surface the careers and degrees that are top matches for you, and provide insights on your unique personality.
What makes us unique
We have more data and tools to optimize our career predictions than any other career test on the planet. This means your results are accurate, thorough, and nuanced, not a quick, generic snapshot like so many other career tests.
Financial manager
Marketing manager, graphic designer, social worker, forensic scientist, psychologist, data analyst, veterinarian, robotics engineer, nutritionist, wildlife rehabilitator, dermatologist, sports writer, game tester, construction manager, questions we receive, how much does it cost.
We want to help everyone learn more about themselves and make informed career decisions, so we’ve made our entire experience free. You’ll get your career and degree matches, insights, and even reports for free.
How long will the test take?
The full assessment takes about 30 minutes to complete. The thoroughness of our career test allows us to deliver personalized, nuanced results. We believe you shouldn’t base major life decisions — like your education and career — on a quick five-minute quiz. The assessment is made up of five sections and each unlocks an additional dimension of fit.
Why should I trust my career results?
We are very good at predicting the right career matches. During the test, we ask you for your general interest in a handful of randomly selected careers, as well as how satisfied you were in any previous careers. Our models use this information to get a baseline understanding of who you are and what you're interested in, but it's also anonymously combined with all the data we have from other users on their interests, as well as their satisfaction with their previous careers and degrees. We train ever better models from this growing dataset to better identify how your interests, work and education history, and personality inform what careers you'd be a good fit in. The predictions are unique to you but validated against millions of other users.
Can I retake the career test?
Yes, you can reset your account to take the career test more than once. Many people take the test on a semi-regular basis to see how their interests and results evolve over time.
Why do my results change?
Our career matching system updates in real-time, meaning that whenever you give us a new piece of relevant information, we will automatically update all of our recommendations for you. As we gather more data and create better models, it's also possible that your results might change as we better understand what makes a career the right fit for someone.
Who developed this career test?
Our career test items were developed by a team of I/O psychologists with years of experience in the field of psychometrics. Career data comes from numerous sources, including the the US Department of Labour’s O*Net Database, US Bureau of Statistics, UK government, CareerExplorer user data, and Sokanu’s proprietary in-house content team.
The career test platform is developed and maintained by the Sokanu team.
Who is this career test designed for?
People start new careers at many different stages of life, and nothing about our assessment is age-specific. We’ve designed a career test that works for adults as well as high school and college students.
Does your test discriminate?
We don’t use race or gender when generating matches, and we work hard to make sure that our test doesn’t discriminate where relevant.
How is my personal data handled?
We firmly believe that you own your data, not us. We never sell your personal data to third parties and we use industry-standard best practices, making sure your private information is always encrypted and secure. We are also happy to delete your data upon request.
Join millions of people at all stages of their career journeys using the CareerExplorer test to find their next steps.
Your dream career is waiting.
It’s time to start exploring.

- SUGGESTED TOPICS
- The Magazine
- Newsletters
- Managing Yourself
- Managing Teams
- Work-life Balance
- The Big Idea
- Data & Visuals
- Reading Lists
- Case Selections
- HBR Learning
- Topic Feeds
- Account Settings
- Email Preferences
Build a Corporate Culture That Works

There’s a widespread understanding that managing corporate culture is key to business success. Yet few companies articulate their culture in such a way that the words become an organizational reality that molds employee behavior as intended.
All too often a culture is described as a set of anodyne norms, principles, or values, which do not offer decision-makers guidance on how to make difficult choices when faced with conflicting but equally defensible courses of action.
The trick to making a desired culture come alive is to debate and articulate it using dilemmas. If you identify the tough dilemmas your employees routinely face and clearly state how they should be resolved—“In this company, when we come across this dilemma, we turn left”—then your desired culture will take root and influence the behavior of the team.
To develop a culture that works, follow six rules: Ground your culture in the dilemmas you are likely to confront, dilemma-test your values, communicate your values in colorful terms, hire people who fit, let culture drive strategy, and know when to pull back from a value statement.
Start by thinking about the dilemmas your people will face.
Idea in Brief
The problem.
There’s a widespread understanding that managing corporate culture is key to business success. Yet few companies articulate their corporate culture in such a way that the words become an organizational reality that molds employee behavior as intended.
What Usually Happens
How to fix it.
Follow six rules: Ground your culture in the dilemmas you are likely to confront, dilemma-test your values, communicate your values in colorful terms, hire people who fit, let culture drive strategy, and know when to pull back from a value.
At the beginning of my career, I worked for the health-care-software specialist HBOC. One day, a woman from human resources came into the cafeteria with a roll of tape and began sticking posters on the walls. They proclaimed in royal blue the company’s values: “Transparency, Respect, Integrity, Honesty.” The next day we received wallet-sized plastic cards with the same words and were asked to memorize them so that we could incorporate them into our actions. The following year, when management was indicted on 17 counts of conspiracy and fraud, we learned what the company’s values really were.
- EM Erin Meyer is a professor at INSEAD, where she directs the executive education program Leading Across Borders and Cultures. She is the author of The Culture Map: Breaking Through the Invisible Boundaries of Global Business (PublicAffairs, 2014) and coauthor (with Reed Hastings) of No Rules Rules: Netflix and the Culture of Reinvention (Penguin, 2020). ErinMeyerINSEAD
Partner Center
Suggestions or feedback?
MIT News | Massachusetts Institute of Technology
- Machine learning
- Social justice
- Black holes
- Classes and programs
Departments
- Aeronautics and Astronautics
- Brain and Cognitive Sciences
- Architecture
- Political Science
- Mechanical Engineering
Centers, Labs, & Programs
- Abdul Latif Jameel Poverty Action Lab (J-PAL)
- Picower Institute for Learning and Memory
- Lincoln Laboratory
- School of Architecture + Planning
- School of Engineering
- School of Humanities, Arts, and Social Sciences
- Sloan School of Management
- School of Science
- MIT Schwarzman College of Computing
A smarter way to streamline drug discovery
Press contact :.

Previous image Next image
The use of AI to streamline drug discovery is exploding. Researchers are deploying machine-learning models to help them identify molecules, among billions of options, that might have the properties they are seeking to develop new medicines.
But there are so many variables to consider — from the price of materials to the risk of something going wrong — that even when scientists use AI, weighing the costs of synthesizing the best candidates is no easy task.
The myriad challenges involved in identifying the best and most cost-efficient molecules to test is one reason new medicines take so long to develop, as well as a key driver of high prescription drug prices.
To help scientists make cost-aware choices, MIT researchers developed an algorithmic framework to automatically identify optimal molecular candidates, which minimizes synthetic cost while maximizing the likelihood candidates have desired properties. The algorithm also identifies the materials and experimental steps needed to synthesize these molecules.
Their quantitative framework, known as Synthesis Planning and Rewards-based Route Optimization Workflow (SPARROW), considers the costs of synthesizing a batch of molecules at once, since multiple candidates can often be derived from some of the same chemical compounds.
Moreover, this unified approach captures key information on molecular design, property prediction, and synthesis planning from online repositories and widely used AI tools.
Beyond helping pharmaceutical companies discover new drugs more efficiently, SPARROW could be used in applications like the invention of new agrichemicals or the discovery of specialized materials for organic electronics.
“The selection of compounds is very much an art at the moment — and at times it is a very successful art. But because we have all these other models and predictive tools that give us information on how molecules might perform and how they might be synthesized, we can and should be using that information to guide the decisions we make,” says Connor Coley, the Class of 1957 Career Development Assistant Professor in the MIT departments of Chemical Engineering and Electrical Engineering and Computer Science, and senior author of a paper on SPARROW.
Coley is joined on the paper by lead author Jenna Fromer SM ’24. The research appears today in Nature Computational Science .
Complex cost considerations
In a sense, whether a scientist should synthesize and test a certain molecule boils down to a question of the synthetic cost versus the value of the experiment. However, determining cost or value are tough problems on their own.
For instance, an experiment might require expensive materials or it could have a high risk of failure. On the value side, one might consider how useful it would be to know the properties of this molecule or whether those predictions carry a high level of uncertainty.
At the same time, pharmaceutical companies increasingly use batch synthesis to improve efficiency. Instead of testing molecules one at a time, they use combinations of chemical building blocks to test multiple candidates at once. However, this means the chemical reactions must all require the same experimental conditions. This makes estimating cost and value even more challenging.
SPARROW tackles this challenge by considering the shared intermediary compounds involved in synthesizing molecules and incorporating that information into its cost-versus-value function.
“When you think about this optimization game of designing a batch of molecules, the cost of adding on a new structure depends on the molecules you have already chosen,” Coley says.
The framework also considers things like the costs of starting materials, the number of reactions that are involved in each synthetic route, and the likelihood those reactions will be successful on the first try.
To utilize SPARROW, a scientist provides a set of molecular compounds they are thinking of testing and a definition of the properties they are hoping to find.
From there, SPARROW collects information on the molecules and their synthetic pathways and then weighs the value of each one against the cost of synthesizing a batch of candidates. It automatically selects the best subset of candidates that meet the user’s criteria and finds the most cost-effective synthetic routes for those compounds.
“It does all this optimization in one step, so it can really capture all of these competing objectives simultaneously,” Fromer says.
A versatile framework
SPARROW is unique because it can incorporate molecular structures that have been hand-designed by humans, those that exist in virtual catalogs, or never-before-seen molecules that have been invented by generative AI models.
“We have all these different sources of ideas. Part of the appeal of SPARROW is that you can take all these ideas and put them on a level playing field,” Coley adds.
The researchers evaluated SPARROW by applying it in three case studies. The case studies, based on real-world problems faced by chemists, were designed to test SPARROW’s ability to find cost-efficient synthesis plans while working with a wide range of input molecules.
They found that SPARROW effectively captured the marginal costs of batch synthesis and identified common experimental steps and intermediate chemicals. In addition, it could scale up to handle hundreds of potential molecular candidates.
“In the machine-learning-for-chemistry community, there are so many models that work well for retrosynthesis or molecular property prediction, for example, but how do we actually use them? Our framework aims to bring out the value of this prior work. By creating SPARROW, hopefully we can guide other researchers to think about compound downselection using their own cost and utility functions,” Fromer says.
In the future, the researchers want to incorporate additional complexity into SPARROW. For instance, they’d like to enable the algorithm to consider that the value of testing one compound may not always be constant. They also want to include more elements of parallel chemistry in its cost-versus-value function.
“The work by Fromer and Coley better aligns algorithmic decision making to the practical realities of chemical synthesis. When existing computational design algorithms are used, the work of determining how to best synthesize the set of designs is left to the medicinal chemist, resulting in less optimal choices and extra work for the medicinal chemist,” says Patrick Riley, senior vice president of artificial intelligence at Relay Therapeutics, who was not involved with this research. “This paper shows a principled path to include consideration of joint synthesis, which I expect to result in higher quality and more accepted algorithmic designs.”
“Identifying which compounds to synthesize in a way that carefully balances time, cost, and the potential for making progress toward goals while providing useful new information is one of the most challenging tasks for drug discovery teams. The SPARROW approach from Fromer and Coley does this in an effective and automated way, providing a useful tool for human medicinal chemistry teams and taking important steps toward fully autonomous approaches to drug discovery,” adds John Chodera, a computational chemist at Memorial Sloan Kettering Cancer Center, who was not involved with this work.
This research was supported, in part, by the DARPA Accelerated Molecular Discovery Program, the Office of Naval Research, and the National Science Foundation.
Share this news article on:
Related links.
- Coley Group
- Department of Chemical Engineering
- Department of Electrical Engineering and Computer Science
Related Topics
- Computer science and technology
- Artificial intelligence
- Drug development
- Pharmaceuticals
- Chemical engineering
- Electrical Engineering & Computer Science (eecs)
- National Science Foundation (NSF)
- Defense Advanced Research Projects Agency (DARPA)
Related Articles
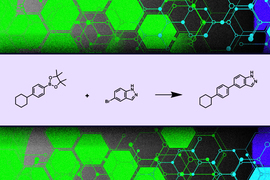
A smarter way to develop new drugs
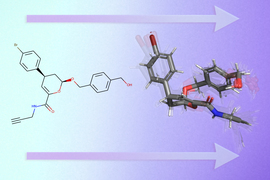
Taking some of the guesswork out of drug discovery
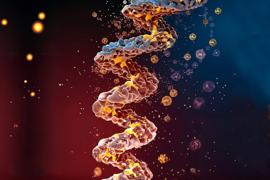
Generative AI imagines new protein structures
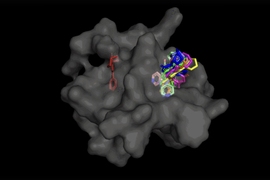
Speeding up drug discovery with diffusion generative models
Previous item Next item
More MIT News
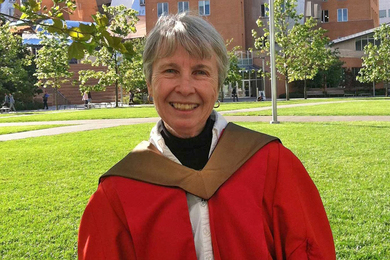
Professor Emerita Mary-Lou Pardue, pioneering cellular and molecular biologist, dies at 90
Read full story →

Helping nonexperts build advanced generative AI models

New Ragon Institute building opens in the heart of Kendall Square

Toward socially and environmentally responsible real estate
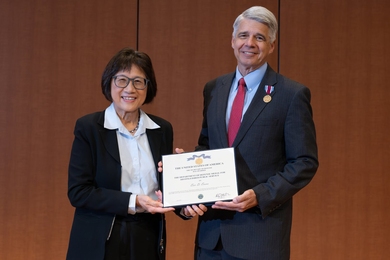
Eric Evans receives Department of Defense Medal for Distinguished Public Service

Study: Titan’s lakes may be shaped by waves
- More news on MIT News homepage →
Massachusetts Institute of Technology 77 Massachusetts Avenue, Cambridge, MA, USA
- Map (opens in new window)
- Events (opens in new window)
- People (opens in new window)
- Careers (opens in new window)
- Accessibility
- Social Media Hub
- MIT on Facebook
- MIT on YouTube
- MIT on Instagram
- Find Out Which ITR Form Is Required For Your Income Tax Filing
- Section 144 Imposed After Clash Breaks Out In Rajasthan: Cops
- 20 Students Fall Sick After Drinking Water From School Tank In Jharkhand
- Neena Gupta Shows How To Make Authentic Vada Pav, Shares Recipe
- Hindujas Made Staff Sleep In Windowless Basement At Swiss Mansion: Report
- Change Font Size A A
- Change Language हिंदी | Hindi বাঙালি | Bengali தமிழ் | Tamil
- Focus on Story
- Dark Theme Light Theme
National Exam Body Invites Applications For Biomedical Research Eligibility Test 2024
Biomedical research eligibility test 2024: the application window opened on june 19 and will close on july 9. application form corrections will be available from july 10 to 11..
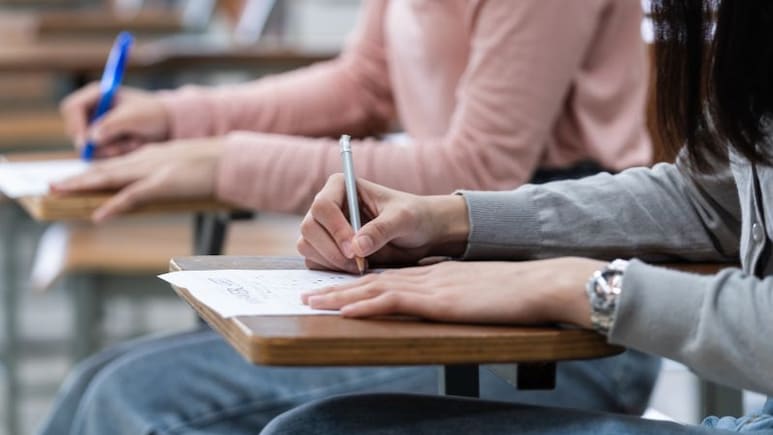
The application window opened on June 19 and will close on July 9. Application form corrections will be available from July 10 to 11. The examination will be held on July 31 in computer-based test (CBT) mode.
Exam Pattern
The test will consist of three parts, all containing objective-type, multiple-choice questions, with no break between papers. The exam will last for two hours. The question paper will have three sections (A, B, and C).
All questions are compulsory and carry one mark each. There will be a negative marking of 0.25 marks for each wrong answer. Unattempted questions will be marked zero.
Educational Qualifications
Degree Requirements:
Candidates must possess an MSc or equivalent post-graduate degree (MTech, MPharma) in fields such as life sciences, zoology, botany, biomedical sciences, microbiology, biochemistry, genetics, biotechnology, biophysics, bioinformatics, forensic sciences, environmental sciences, food and nutrition, molecular biology, biological sciences, ecology, immunology, neurosciences, veterinary sciences, nursing, biostatistics, pharmacology, pharmaceutical sciences, public health, or social work, with at least 55% marks for General/EWS/OBC candidates and 50% for SC/ST and PwBD candidates.
Final Year Candidates: Those in the final year of their MSc or equivalent degree (IV/VI Semester or final year if the semester system is not applicable) during the 2023-24 session may also apply as RA (Result Awaited).
Attestation Requirement: These candidates must submit an attestation form (Annexure-I) certified by the head of the department/institute, including signature, official seal/stamp, and address.
Provisional admission : Admission to the test will be provisional until proof of passing the Master's Degree with the required percentage is submitted.
Examination Venue Requirements:
Candidates must bring only the following items to the examination venue:
- Admit card with Self Declaration (Undertaking) downloaded from the NTA Website, printed on A4 size paper, and duly filled in.
- A simple transparent Ball Point Pen.
- An additional photograph (same as uploaded on the Online Application Form) to be pasted on the attendance sheet in the examination room/hall.
- One of the following original, valid, and non-expired photo IDs: PAN card, Driving License, Voter ID, Passport, Aadhaar Card (with photograph), E-Aadhaar with photograph, Bank Passbook with photograph.
- PwBD certificate issued by an authorized medical officer, if applicable.
- Personal transparent water bottle.
- Sugar tablets/fruits (like banana/apple/orange) if the candidate is diabetic.
- The age limit for the eligibility test is 30 years as of the last date of the application form, July 9, 2024.
About DHR-ICMR
The Indian Council of Medical Research (ICMR), New Delhi, under the Department of Health Research (DHR), Ministry of Health and Family Welfare (MoH&FW), is India's apex body for biomedical research formulation, coordination, and promotion.
Track Education News , Exam updates , Campus , Study Abroad related news live on NDTV.com
- Notifications
- Web Stories
- TV Schedule
- Big Bonus REGISTER NOW
- मध्य प्रदेश
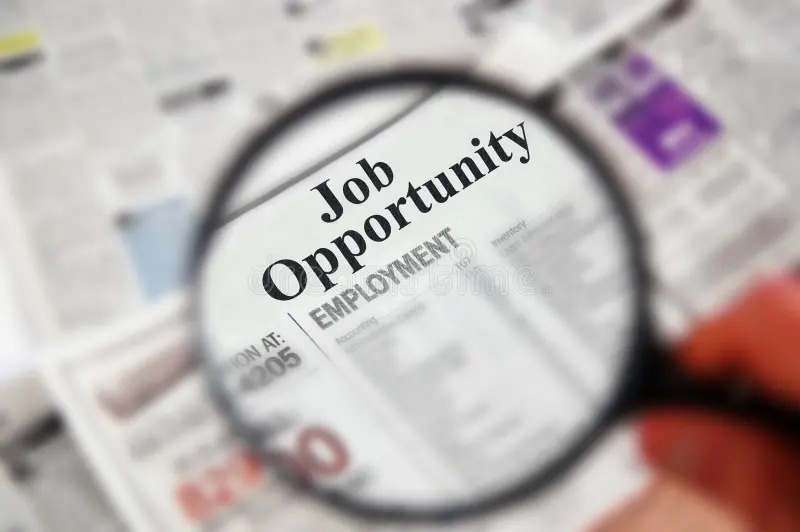
Apple Intelligence Preview

AI for the rest of us.
Coming in beta this fall *
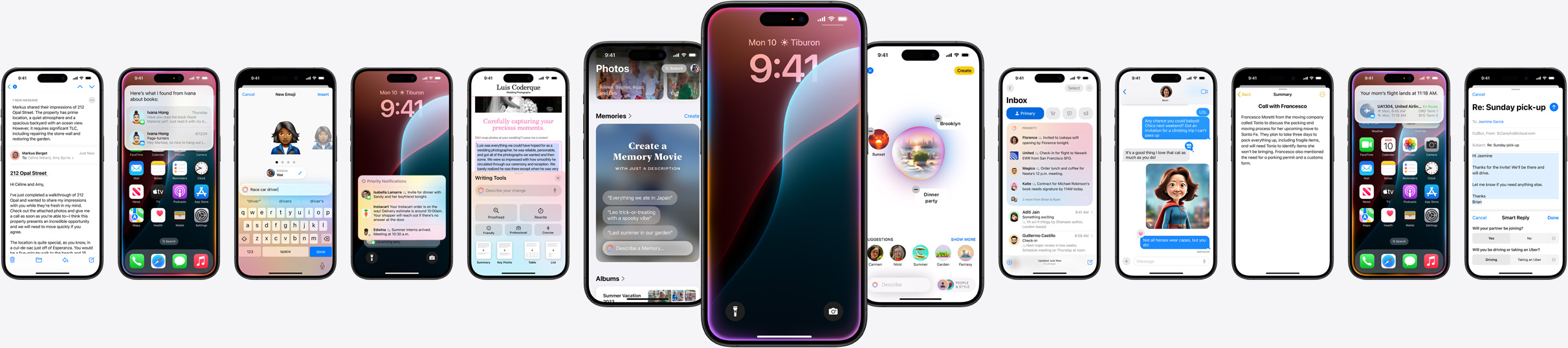
Built into your iPhone, iPad, and Mac to help you write, express yourself, and get things done effortlessly.
Draws on your personal context while setting a brand-new standard for privacy in AI.

Write with intelligent new tools. Everywhere words matter.
Apple Intelligence powers new Writing Tools, which help you find just the right words virtually everywhere you write. With enhanced language capabilities, you can summarize an entire lecture in seconds, get the short version of a long group thread, and minimize unnecessary distractions with prioritized notifications.
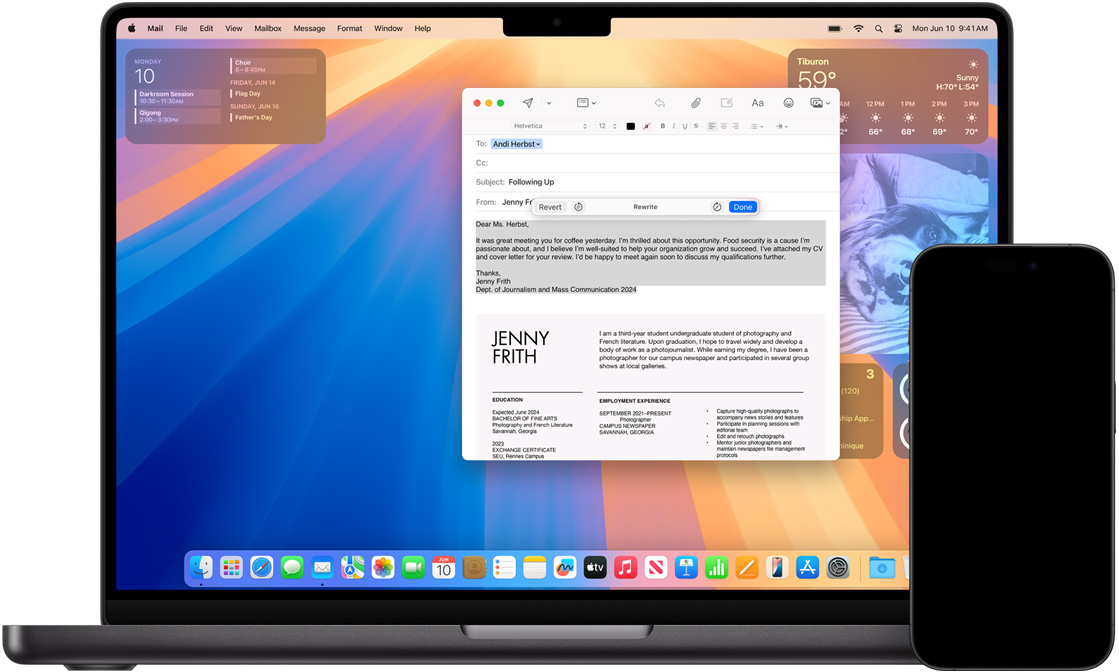
Explore new features for writing, focus, and communication.
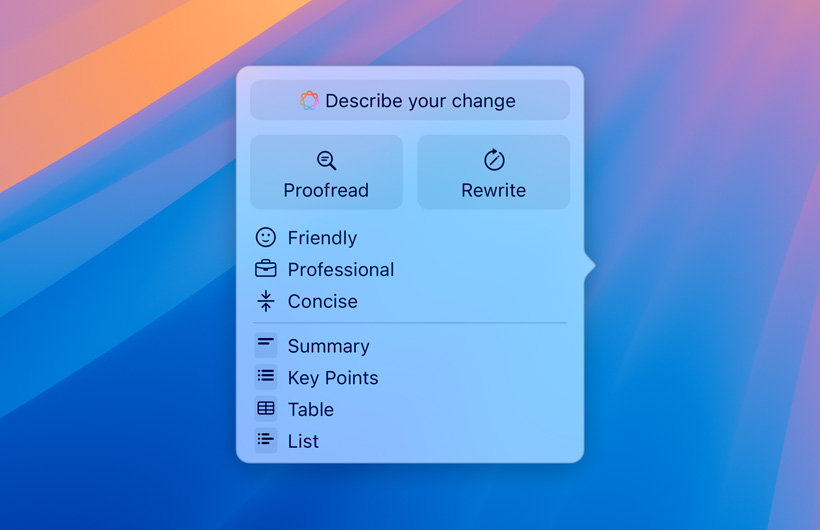
Transform how you communicate using intelligent Writing Tools that can proofread your text, rewrite different versions until the tone and wording are just right, and summarize selected text with a tap. Writing Tools are available nearly everywhere you write, including third-party apps.
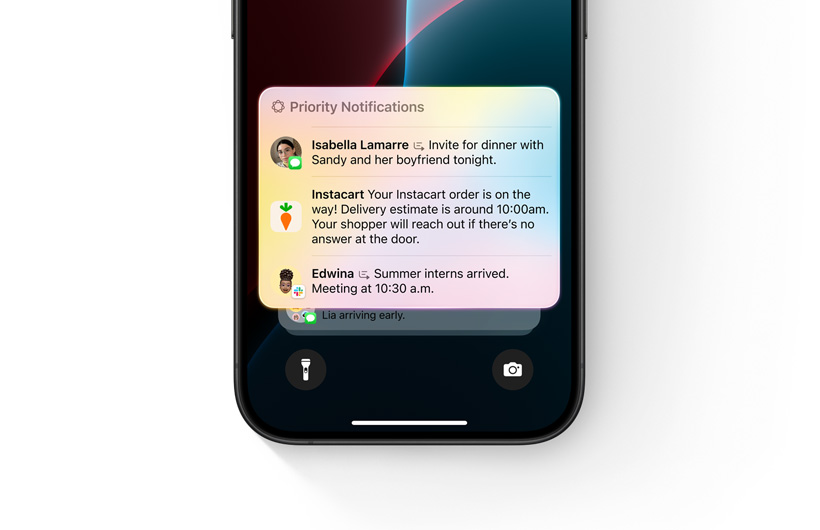
Priority notifications appear at the top of the stack, letting you know what to pay attention to at a glance. And notifications are summarized, so you can scan them faster.
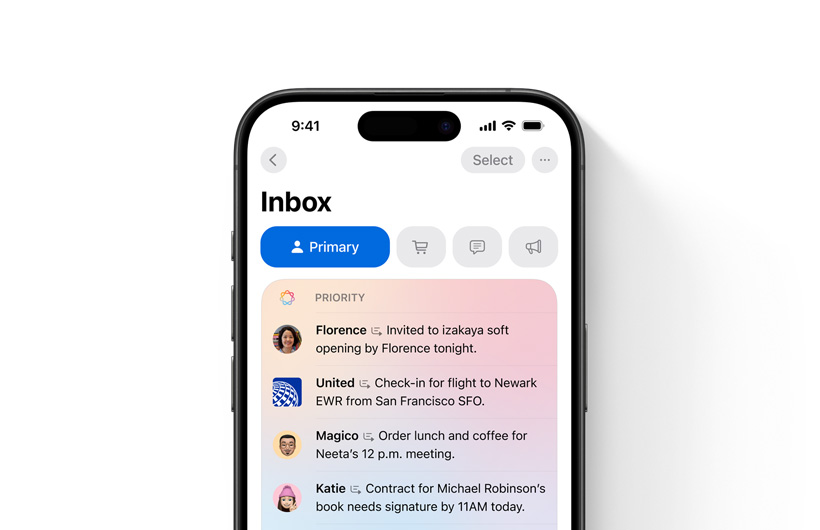
Priority messages in Mail elevate time-sensitive messages to the top of your inbox — like an invitation that has a deadline today or a check-in reminder for your flight this afternoon.
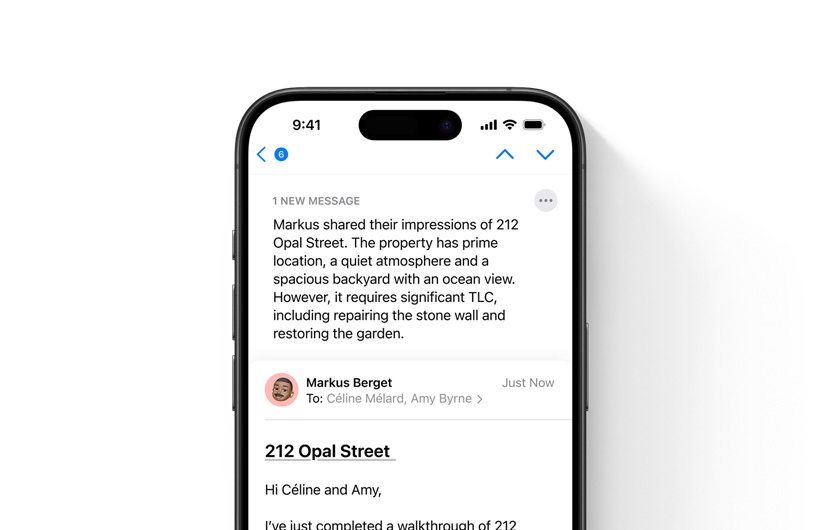
Tap to reveal a summary of a long email in the Mail app and cut to the chase. You can also view summaries of email right from your inbox.
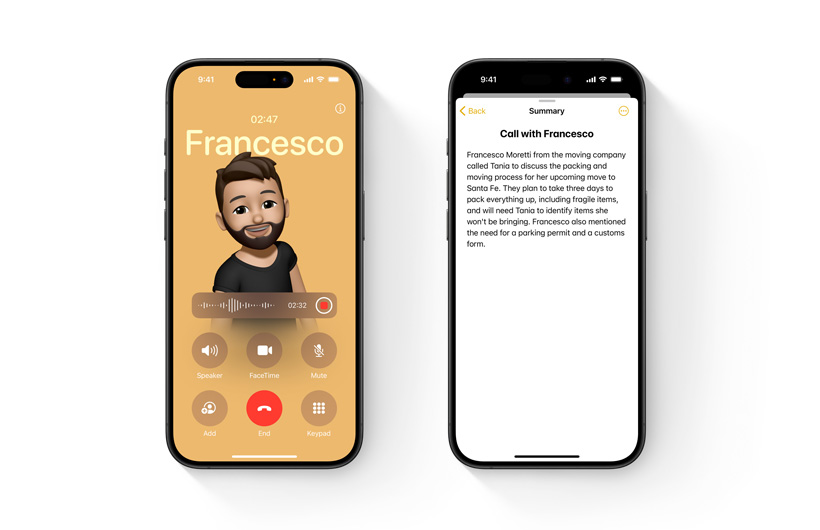
Just hit record in the Notes or Phone apps to capture audio recordings and transcripts. Apple Intelligence generates summaries of your transcripts, so you can get to the most important information at a glance.
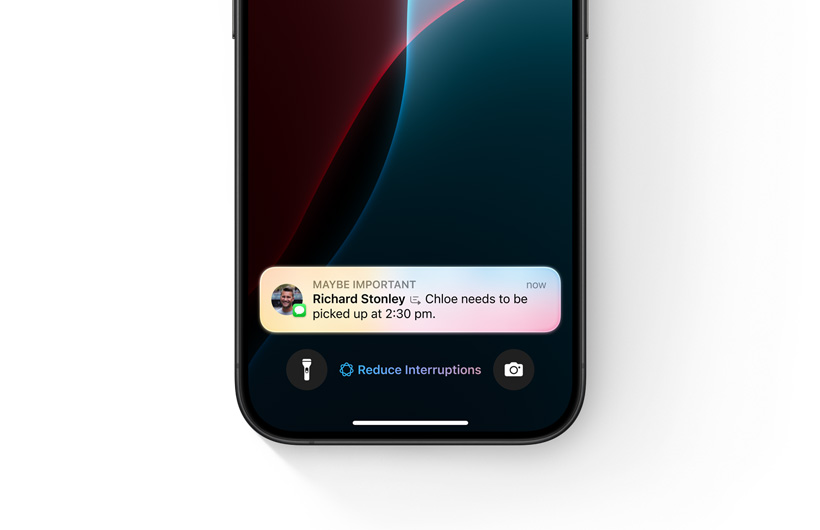
Reduce Interruptions is an all-new Focus that understands the content of your notifications and shows you the ones that might need immediate attention, like a text about picking up your child from daycare later today.
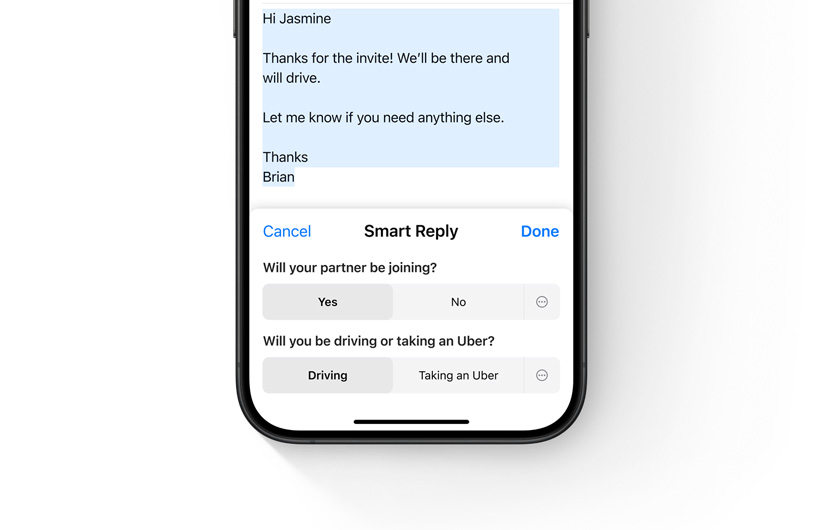
Use a Smart Reply in Mail to quickly draft an email response with all the right details. Apple Intelligence can identify questions you were asked in an email and offer relevant selections to include in your response. With a few taps you’re ready to send a reply with key questions answered.
Delightful images created just for you.
Apple Intelligence enables delightful new ways to express yourself visually. Create fun, original images and brand-new Genmoji that are truly personal to you. Turn a rough sketch into a related image that complements your notes with Image Wand. And make a custom memory movie based on the description you provide.
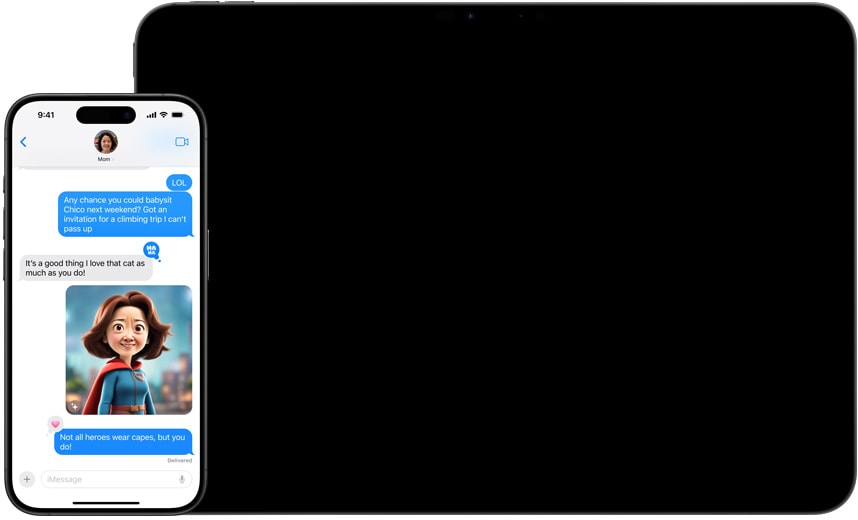
Create expressive images, unique Genmoji, and custom memory movies.
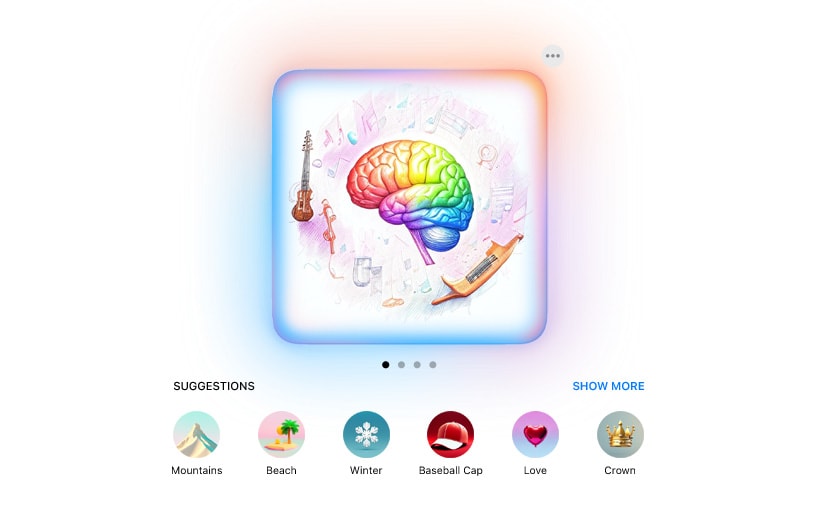
Produce fun, original images in seconds with the Image Playground experience right in your apps. Create an entirely new image based on a description, suggested concepts, and even a person from your Photos library. You can easily adjust the style and make changes to match a Messages thread, your Freeform board, or a slide in Keynote.
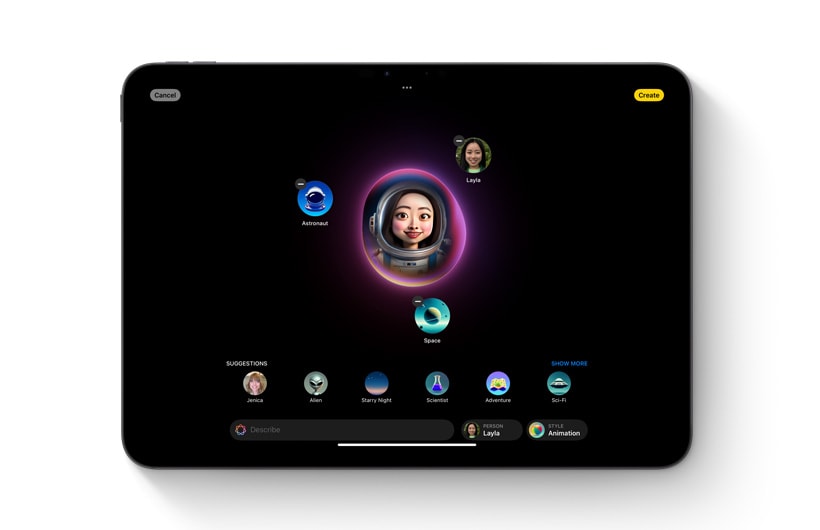
Experiment with different concepts and try out image styles like animation, illustration, and sketch in the dedicated Image Playground app . Create custom images to share with friends in other apps or on social media.
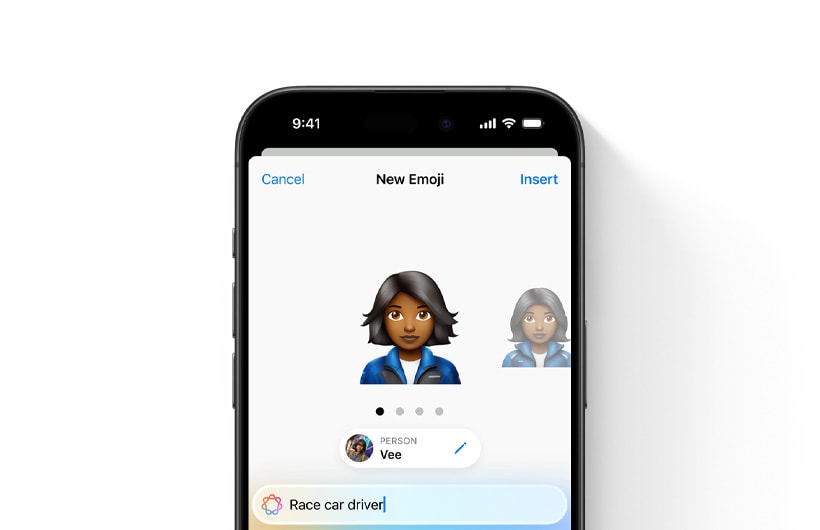
Make a brand-new Genmoji right in the keyboard to match any conversation. Provide a description to see a preview, and adjust your description until it’s perfect. You can even pick someone from your Photos library and create a Genmoji that looks like them.
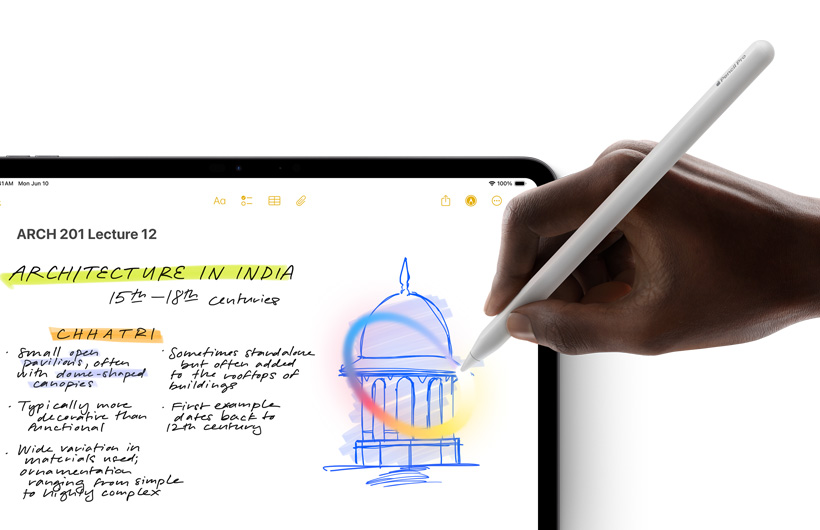
Image Wand can transform your rough sketch into a related image in the Notes app. Use your finger or Apple Pencil to draw a circle around your sketch, and Image Wand will analyze the content around it to produce a complementary visual. You can even circle an empty space, and Image Wand will use the surrounding context to create a picture.

Create a custom memory movie of the story you want to see, right in Photos. Enter a description, and Apple Intelligence finds the best photos and videos that match. It then crafts a storyline with unique chapters based on themes it identifies and arranges your photos into a movie with its own narrative arc.
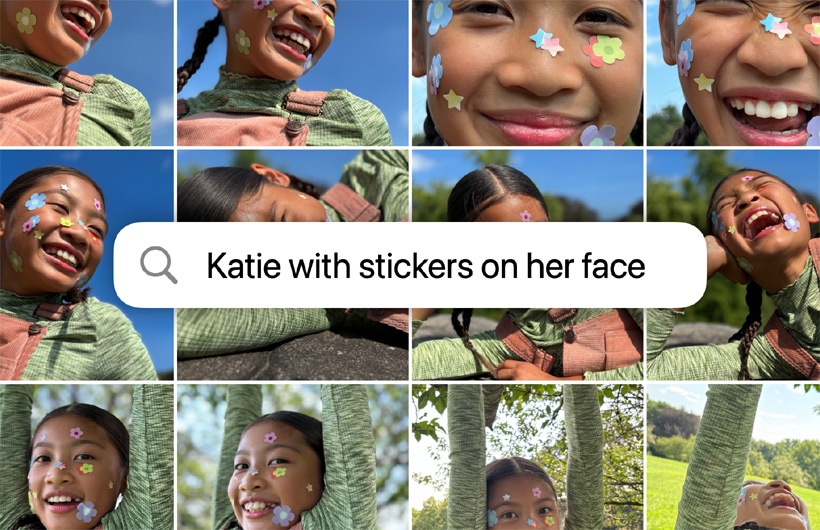
Search for photos and videos in the Photos app simply by describing what you’re looking for. Apple Intelligence can even find a particular moment in a video clip that fits your search description and take you right to it.
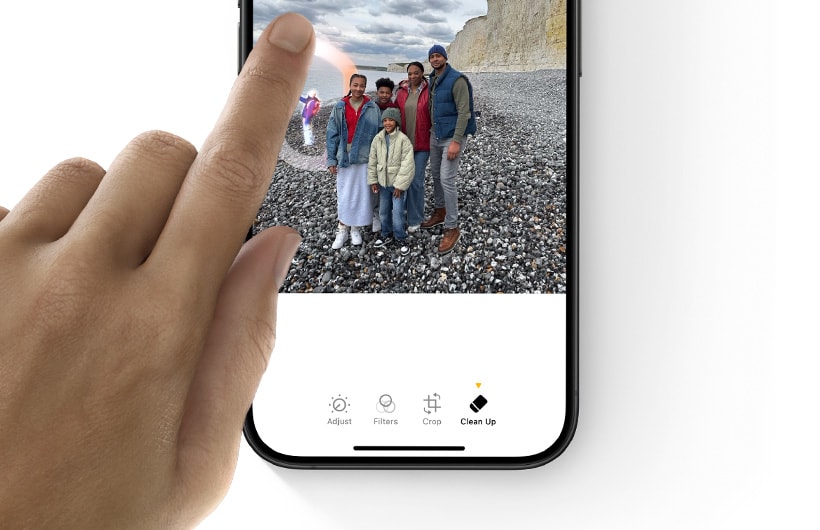
Remove distractions in your photos with the Clean Up tool in the Photos app. Apple Intelligence identifies background objects so you can remove them with a tap and perfect your shot — while staying true to the original image.
The start of a new era for Siri.
Siri draws on Apple Intelligence for all-new superpowers. With an all-new design, richer language understanding, and the ability to type to Siri whenever it’s convenient for you, communicating with Siri is more natural than ever. Equipped with awareness of your personal context, the ability to take action in and across apps, and product knowledge about your devices’ features and settings, Siri will be able to assist you like never before.
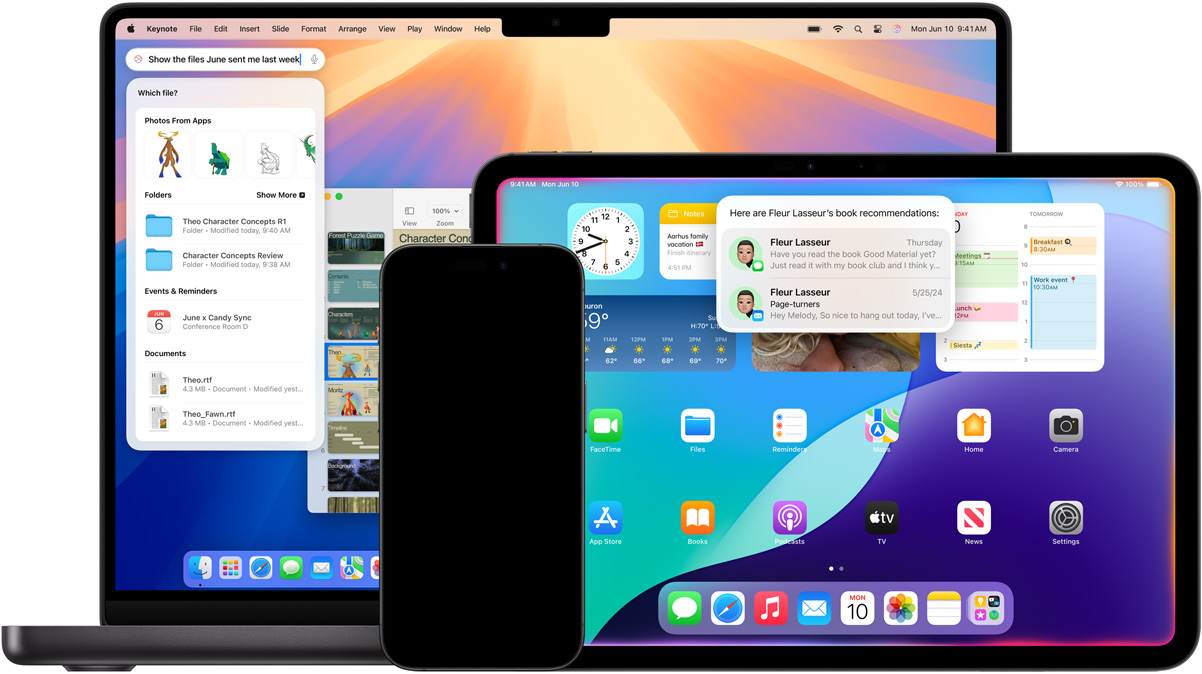
Discover an even more capable, integrated, personal Siri.
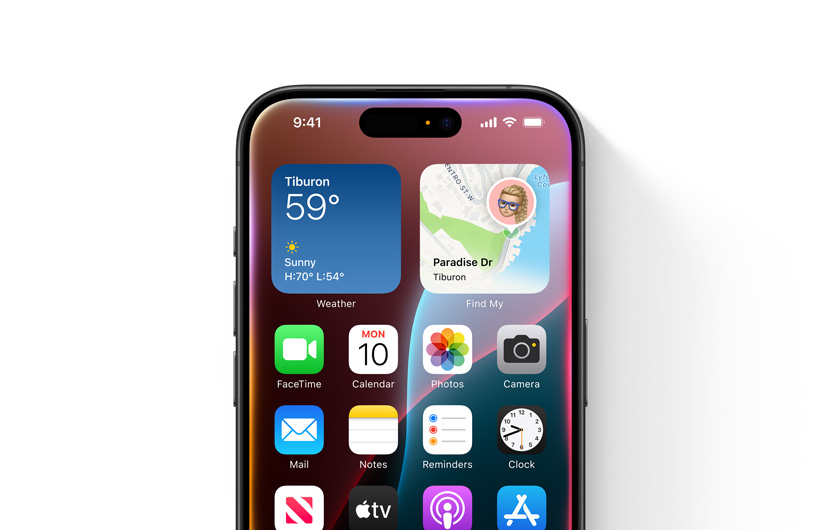
Siri has an all-new design that’s even more deeply integrated into the system experience, with an elegant, glowing light that wraps around the edge of your screen.

With a double tap on the bottom of your iPhone or iPad screen, you can type to Siri from anywhere in the system when you don’t want to speak out loud.
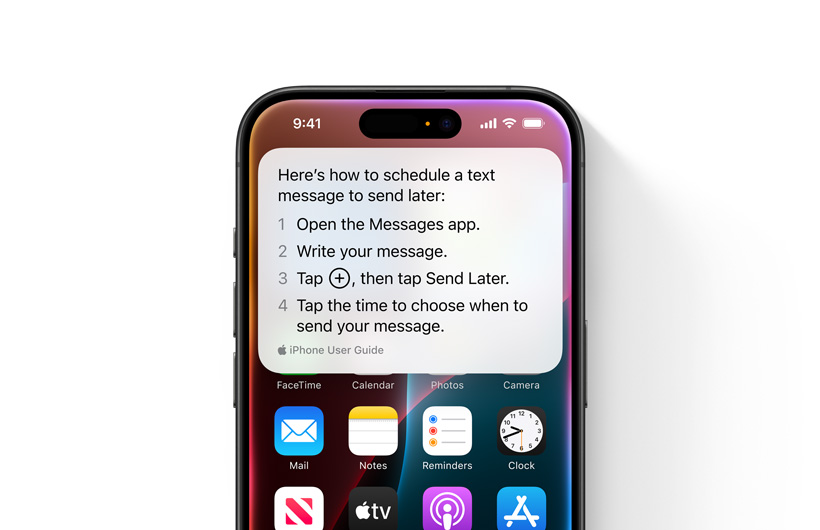
Tap into the expansive product knowledge Siri has about your devices’ features and settings. You can ask questions when you’re learning how to do something new on your iPhone, iPad, and Mac, and Siri can give you step-by-step directions in a flash.
Siri, set an alarm for — oh wait no, set a timer for 10 minutes. Actually, make that 5.
Richer language understanding and an enhanced voice make communicating with Siri even more natural. And when you refer to something you mentioned in a previous request, like the location of a calendar event you just created, and ask ”What will the weather be like there?” Siri knows what you’re talking about.
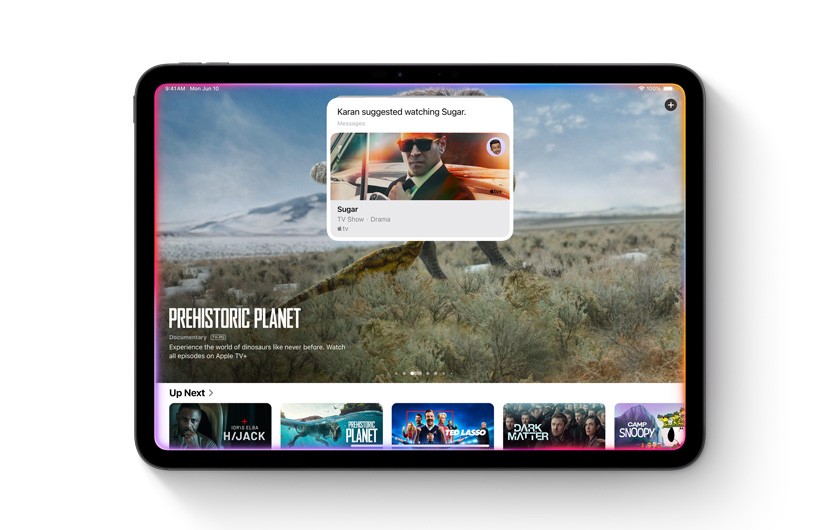
Apple Intelligence empowers Siri with onscreen awareness , so it can understand and take action with things on your screen. If a friend texts you their new address, you can say “Add this address to their contact card,” and Siri will take care of it.
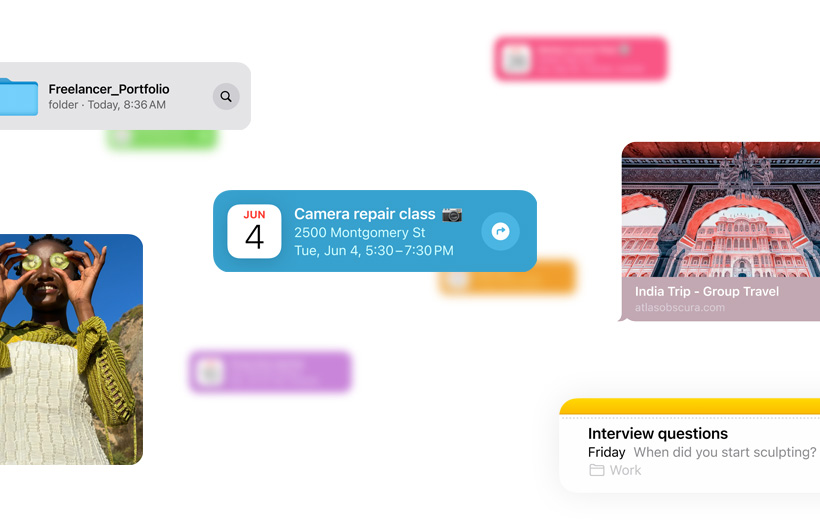
Awareness of your personal context enables Siri to help you in ways that are unique to you. Can’t remember if a friend shared that recipe with you in a note, a text, or an email? Need your passport number while booking a flight? Siri can use its knowledge of the information on your device to help find what you’re looking for, without compromising your privacy.
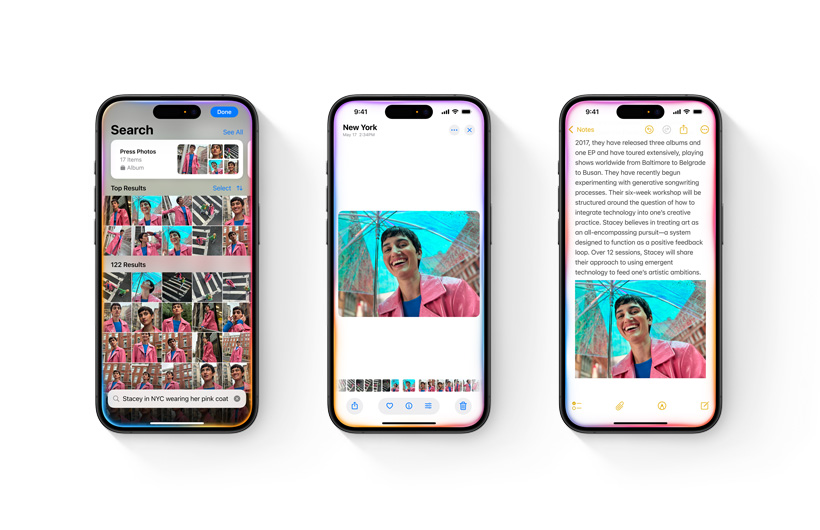
Seamlessly take action in and across apps with Siri. You can make a request like “Send the email I drafted to April and Lilly” and Siri knows which email you’re referencing and which app it’s in. And Siri can take actions across apps, so after you ask Siri to enhance a photo for you by saying “Make this photo pop,” you can ask Siri to drop it in a specific note in the Notes app — without lifting a finger.
Great powers come with great privacy.
Apple Intelligence is designed to protect your privacy at every step. It’s integrated into the core of your iPhone, iPad, and Mac through on-device processing. So it’s aware of your personal information without collecting your personal information. And with groundbreaking Private Cloud Compute, Apple Intelligence can draw on larger server-based models, running on Apple silicon, to handle more complex requests for you while protecting your privacy.
Private Cloud Compute
- Your data is never stored
- Used only for your requests
- Verifiable privacy promise
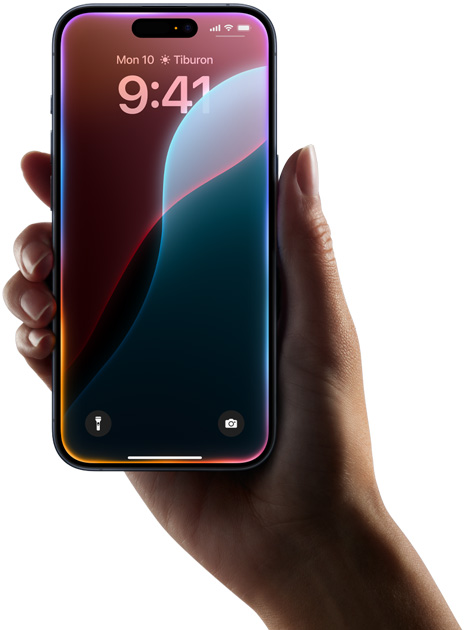
ChatGPT, seamlessly integrated.
With ChatGPT from OpenAI integrated into Siri and Writing Tools, you get even more expertise when it might be helpful for you — no need to jump between tools. Siri can tap into ChatGPT for certain requests, including questions about photos or documents. And with Compose in Writing Tools, you can create and illustrate original content from scratch.
You control when ChatGPT is used and will be asked before any of your information is shared. Anyone can access ChatGPT for free, without creating an account. ChatGPT subscribers can connect accounts to access paid features within these experiences.
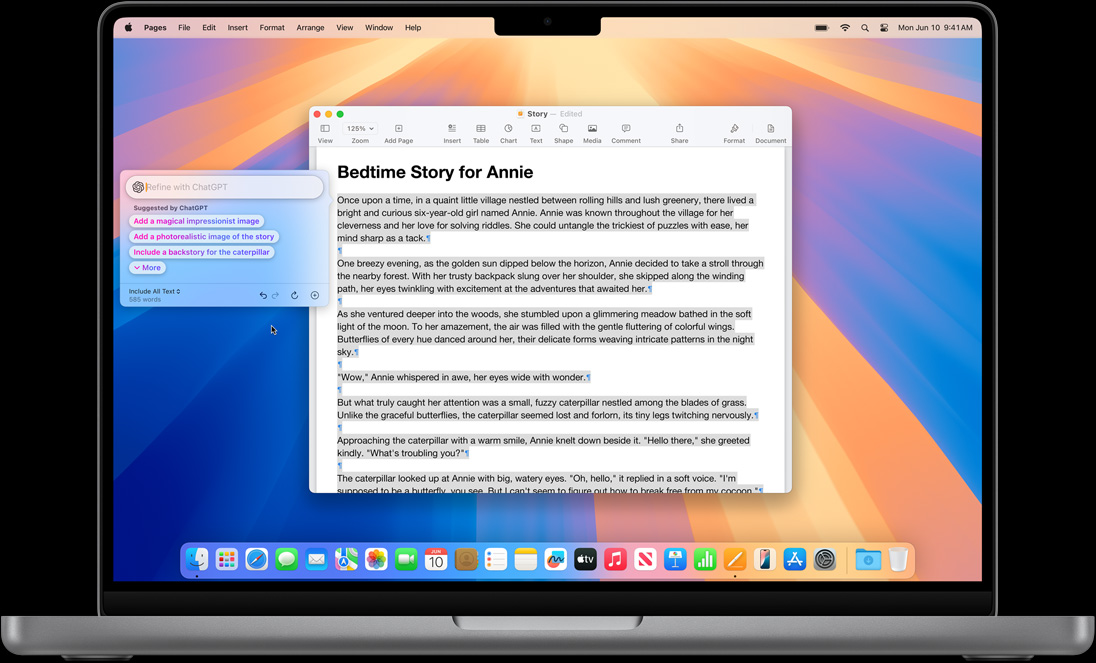
New possibilities for your favorite apps.
New App Intents, APIs, and frameworks make it incredibly easy for developers to integrate system-level features like Siri, Writing Tools, and Image Playground into your favorite apps.
Learn more about developing for Apple Intelligence
Apple Intelligence is compatible with these devices.
Apple Intelligence is free to use and will initially be available in U.S. English. Coming in beta this fall. *
- iPhone 15 Pro Max A17 Pro
- iPhone 15 Pro A17 Pro
- iPad Pro M1 and later
- iPad Air M1 and later
- MacBook Air M1 and later
- MacBook Pro M1 and later
- iMac M1 and later
- Mac mini M1 and later
- Mac Studio M1 Max and later
- Mac Pro M2 Ultra

An official website of the United States government
The .gov means it’s official. Federal government websites often end in .gov or .mil. Before sharing sensitive information, make sure you’re on a federal government site.
The site is secure. The https:// ensures that you are connecting to the official website and that any information you provide is encrypted and transmitted securely.
- Publications
- Account settings
Preview improvements coming to the PMC website in October 2024. Learn More or Try it out now .
- Advanced Search
- Journal List
- Front Behav Neurosci
Reliability and validity analysis of personality assessment model based on gait video
1 School of Electronic, Electrical and Communication Engineering, University of Chinese Academy of Sciences, Beijing, China
2 Institute of Psychology, Chinese Academy of Sciences, Beijing, China
3 School of Computer Science and Technology, University of Chinese Academy of Sciences, Beijing, China
Deyuan Chen
Tingshao zhu.
4 Department of Psychology, University of Chinese Academy of Sciences, Beijing, China
Associated Data
To protect the privacy of the participants, the original datasets in the article cannot be made public. If necessary, feature datasets of gait are available from the corresponding author on reasonable request. Requests to access the datasets should be directed to TZ, nc.ca.hcysp@uhzst .
Personality affects an individual’s academic achievements, occupational tendencies, marriage quality and physical health, so more convenient and objective personality assessment methods are needed. Gait is a natural, stable, and easy-to-observe body movement that is closely related to personality. The purpose of this paper is to propose a personality assessment model based on gait video and evaluate the reliability and validity of the multidimensional model. This study recruited 152 participants and used cameras to record their gait videos. Each participant completed a 44-item Big Five Inventory (BFI-44) assessment. We constructed diverse static and dynamic time-frequency features based on gait skeleton coordinates, interframe differences, distances between joints, angles between joints, and wavelet decomposition coefficient arrays. We established multidimensional personality trait assessment models through machine learning algorithms and evaluated the criterion validity, split-half reliability, convergent validity, and discriminant validity of these models. The results showed that the reliability and validity of the Gaussian process regression (GPR) and linear regression (LR) models were best. The mean values of their criterion validity were 0.478 and 0.508, respectively, and the mean values of their split-half reliability were all greater than 0.8. In the formed multitrait-multimethod matrix, these methods also had higher convergent and discriminative validity. The proposed approach shows that gait video can be effectively used to evaluate personality traits, providing a new idea for the formation of convenient and non-invasive personality assessment methods.
Introduction
Personality is a characteristic set of behavior, cognition and psychological state, with stability and persistence ( Corr and Matthews, 2020 ). Personality affects the behavior, mental states and subjective well-being of individuals ( Costa and McCrae, 1980 ; Baumert et al., 2017 ). Studies have shown that personality traits are even related to physical health factors such as obesity ( Jokela et al., 2013 ) and the risk of death ( Iwasa et al., 2008 ). In addition, personality is closely related to academic achievements and learning styles ( Komarraju et al., 2011 ), career choices and satisfaction ( Seibert et al., 2001 ; Kern et al., 2019 ), love quality and marriage relationships ( Holland and Roisman, 2008 ). Based on these issues, the need for a more convenient and objective user personality evaluation approach has become increasingly urgent.
Currently, many personality assessment methods are available, and questionnaires and scales are the most widely used measurement tools ( Bing et al., 2007 ; Rammstedt and John, 2007 ). However, self-reported questionnaires are not applicable in some occasions. For example, in the psychological assessment of job hunting or enrolment, the participants may have more motives for answering deceptively when interests are involved ( Ones et al., 1996 ). In addition, questionnaires are not suitable for situations where multiple measurements are required, because filling out the same questionnaire multiple times leads to practice effects.
As a natural and easily observed body movement, human gait conveys much information about emotions, cognition, intentions, and personality ( Matsumoto et al., 2015 ). Previous studies have shown that it is possible to establish a relationship between certain qualities of body motion and personality ( Koppensteiner and Grammer, 2010 ). The walking speed of a person in adulthood reflects, in part, the individual’s personality ( Stephan et al., 2018 ). Higher degrees of extroversion and conscientiousness are associated with faster initial walking speeds and lesser walking speed declines, while high neuroticism is manifested by slow walking ( Tolea et al., 2012 ; Agmon and Armon, 2016 ). Hand movements can also effectively express personality traits ( Wang et al., 2016 ). For instance, the more open a person is, the more violent their vertical arm movements and the more obvious the changes in their movement directions ( Koppensteiner and Grammer, 2010 ). In addition, thoracic and pelvic movements and the coordination of limbs are related to personality ( Satchell et al., 2017 ). For example, individuals with high neuroticism and low extroversion show decreased mobility and poor limb coordination ( LeMonda et al., 2015 ).
Although much evidence has shown that personality can be reflected by gait, a personality assessment method based on gait has not yet been fully established. Previous research has mainly focused on the statistical correlations between personality traits and gait. Sun et al. (2019) performed preliminary research explorations regarding the modeling of gait and personality traits, but they lacked a comprehensive evaluation of the model’s performance. Since personality contains many traits, it is necessary to establish a multidimensional model. Existing model evaluation methods, such as accuracy- or error-based approaches, cannot evaluate the correlations between the dimensions of the model. Therefore, we apply the reliability and validity evaluation method used for scales to a machine learning model. We use the correlations between the prediction scores of each dimension of personality obtained from models and actual scores from scales to calculate the model validity and use the correlations between the predicted scores of models based on the two halves of the input gait data to calculate the model reliability. This method has been proven feasible in the field of affective computing ( Park et al., 2015 ).
In addition, a practical method should also include convenient tools to record gait data. The need for expensive and complex facilities in previous studies, such as motion capture systems ( Mündermann et al., 2006 ; Leardini et al., 2007 ; O’Connor et al., 2007 ), smart wearable devices ( Tao et al., 2012 ), and Kinect ( Springer and Yogev Seligmann, 2016 ; Sun et al., 2018 ), made them unusable as real-life solutions. In real life, due to the popularity of cameras, we can easily obtain gait video. Many studies have shown that gait video data can be used to achieve efficient gait recognition ( Singh et al., 2018 ; Wan et al., 2018 ), providing ideas for us to use gait video to establish a personality assessment model. Because gait video is convenient, easy to obtain and non-invasive, we can carry out large-scale gait experiments.
This study uses ordinary cameras to record two-dimensional gait video, builds a multidimensional machine learning model, and explores the criterion validity, split-half reliability, convergent validity, and discriminant validity of the developed personality assessment model. The purpose is to provide a new convenient personality assessment approach.
Materials and methods
Data collection, participants.
The personalities of adult individuals tend to be stable ( Briley and Tucker-Drob, 2014 ). For meaningful evaluation, datasets should contain at least 30 participants and possibly more ( Hofmann et al., 2014 ). We recruited 152 adult participants without mental illness or physical disability, including 79 males (52%) and 73 females (48%) with an average age of approximately 23 years (SD = 1.07).
Collection process
Participants walked back and forth for 2 min in a rectangular area with a size of 6 m × 2 m according to their daily walking conditions. During this period, a camera was used to record the participants’ gait videos. The experimental setup for gait data collection is shown in Figure 1 .

Experimental setup for gait data collection. The camera was fixed on the side of a rectangular footpath with a size of 6 m × 2 m. Participants walked back and forth on the footpath.
After completing the gait collection process, the participants immediately filled out the Big Five Inventory (BFI-44) personality scale. The BFI consists of 44 items and five subscales: extraversion (8 items), agreeableness (9 items), conscientiousness (9 items), neuroticism (8 items), and openness (10 items) ( John et al., 1991 ). Each item of the BFI-44 is assessed on a five-point Likert scale, ranging from 1 (“disagree strongly”) to 5 (“agree strongly”). This study used the Chinese version of the BFI-44 scale. The range of Cronbach’s alpha was 0.698–0.807, and the test-retest reliability was between 0.694 and 0.770 ( Carciofo et al., 2016 ).
The above protocol was performed with permission from the Institutional Review Board of the Institute of Psychology, Chinese Academy of Sciences (approval number: {"type":"entrez-nucleotide","attrs":{"text":"H15010","term_id":"879830"}} H15010 ).
Data preprocessing
Key point extraction.
We used OpenPose to extract skeleton coordinates from the gait videos. OpenPose is a human posture recognition system that can detect key points of human body, hands, face, and feet ( Cao et al., 2021 ). This study used OpenPose to extract the two-dimensional coordinates of 25 key points of the body, as shown in Figure 2 .

The 25 key points of the human body in OpenPose. In the key point labels, L and R represent the left and right sides of the human skeleton, respectively. The XOY in the upper left is the original coordinate system, and the X ′ O ′ Y ′ in the middle is the new coordinate system formed after coordinate translation.
Data unification
The experimental setup in Figure 1 involved walking back and forth, so the gait video contained the participants’ front and back gaits. Related studies have shown that gait skeleton evaluation based on the front view is more accurate than that based on the back view ( Fang et al., 2019 ). The differences in the amounts of training data available for the participants can greatly affect the performance of machine learning models ( Luyckx and Daelemans, 2010 ). Therefore, we kept at least four complete gait cycles based on the front view for each participant. One gait cycle represented the process of one foot from leaving the ground until landing ( Baker and Hart, 2013 ). In this study, the gait frames of all participants were unified to 75 frames. This method has been used in many studies and has proven effective ( Sun et al., 2017 ; Zhao et al., 2019 ; Yeye et al., 2020 ).
Coordinate translation
In the XOY original coordinate system ( Figure 2 ), the coordinates of key points were greatly affected by the body shapes and positions of the participants, and their coordinate sequences changed irregularly, as shown in A and B of Figure 3 . The movement of the human center of gravity can be used to assess the stability of a person’s gait ( Iida and Yamamuro, 1987 ). The movement changes between the center of gravity and the center of the pelvis are very similar, and the movement of the pelvis during the gait is obviously related to the movements of the limbs and torso ( Whittle, 1997 ). Therefore, this study used the key points of MidHip (No. 8) as the coordinate origin to establish a new coordinate system X ′ O ′ Y ′ ( Figure 2 ). The coordinate translation formula is as follows:

Coordinate sequence of key points of gait. (A,B) Before coordinate translation, in the XOY coordinate system. (C,D) After coordinate translation, before filtering, in the X ′ O ′ Y ′ coordinate system. (E,F) After filtering. The left figures show the x-coordinate sequence of the LAnkle key point. The right figures show the y-coordinate sequence of the RHip key point.
where i = 1,2,…,24 and x i and y i represent the horizontal and vertical coordinates of point i in the XOY coordinate system, respectively. After coordinate translation, the coordinate sequence obeyed an obvious motion law, reflecting the periodicity of gait movement, as shown in C and D of Figure 3 .
Due to the interference of the video background, high-frequency noise was contained in the key point coordinates, as shown in Figure 3 . We used a template with a one-dimensional convolution kernel ([1,4,6,4,1]) to smooth the coordinate sequence, as shown in Figure 4 . The filtering formula is:

Filtering. The one-dimensional convolution kernel was [1,4,6,4,1].
where j represents the coordinate value of the jth frame before filtering, and j ′ represents the coordinate value after filtering. The time series data after filtering (E and F in Figure 3 ) were obviously smoother than the original data (C and D in Figure 3 ).
Feature engineering
Feature construction, interframe difference.
Gait is the dynamic change of the body exhibited while walking ( Minetti, 1998 ). The movement information of a gait is contained in the frame-to-frame changes shown in the corresponding gait video. We used the forward interframe difference method to focus on the key point coordinate changes between two adjacent frames. The forward difference formula is:
where f j+1 and f j represent the skeleton coordinates of the (j+1)th frame and the jth frame, respectively, and △ f j represents the difference between two adjacent frames.
Distance between joints
Gait requires coordinated movement among the joints of the body ( Murray, 1967 ; Luyckx and Daelemans, 2010 ). Therefore, gait research cannot examine the movement rules of a certain key point or joint in isolation. The distance between a pair of joints is a local motion unit composed of two joint points. We used the horizontal and vertical distances between joints to characterize the changes between two joint points. We found that the distance between joints had a corresponding meaning in terms of gait movement. For example, the distance between LWrist (No. 7) and RWrist (No. 4) represented the swing of the hands at the associated moment ( Donker et al., 2002 ; Park, 2008 ), and the distance between LAnkle (No. 14) and RAnkle (No. 11) represented the stride at that moment ( Whittle, 2014 ). We proposed 13 distances between joints, including 26 coordinate distances. See Table 1 for specific indicators.
Distances between joints.
Name | Meaning | Name | Meaning |
dist_1_0_x | Head swing | dist_10_9_x | Thigh swing |
dist_1_0_y | dist_10_9_y | (right) | |
dist_3_2_x | Upper arm | dist_11_9_x | Leg swing |
dist_3_2_y | swing (right) | dist_11_9_y | (right) |
dist_4_2_x | Arm swing | dist_13_12_x | Thigh swing |
dist_4_2_y | (right) | dist_13_12_y | (left) |
dist_6_5_x | Upper arm | dist_14_12_x | Leg swing |
dist_6_5_y | swing (left) | dist_14_12_y | (left) |
dist_7_5_x | Arm swing | dist_13_10_x | Relative swing |
dist_7_5_y | (left) | dist_13_10_y | of both knees |
dist_6_3_x | Relative swing | dist_14_11_x | Relative swing |
dist_6_3_y | of both elbows | dist_14_11_y | of both feet |
dist_7_4_x | Relative swing | ||
dist_7_4_y | of both hands |
*In the name, dist_a_b_x and dist_a_b_y, respectively, represent the x-axis distance and the y-axis distance between joints a and b, where a and b are key point (or joint) labels.
Angle between joints
The angle between joints is also an important indicator for measuring human gait movement ( Davis et al., 1991 ). The angle between joints is a local motion unit composed of multiple joints. We used the angle formed by 3 joint points to characterize the relative motion between multiple joints. For example, the angle between Nose (No. 0), Neck (No. 1), and RShoulder (No. 2) represented the tilt of the head at the associated moment, and the angle between RHip (No. 9), RKnee (No. 10), and RAnkle (No. 11) represented the bending movement of the right knee at this moment ( Seel et al., 2014 ). We proposed 10 angles between joints, as shown in Figure 5 . See Table 2 for specific indicators. It is worth noting that ∠ RNeck and ∠ LNeck both represented the tilt angle of the neck. However, when participants shrugged or slanted their shoulders, the two angles did not constitute a supplementary angle. As MidHip (No. 8) was eliminated during preprocessing, for ∠ LHip and ∠ RHip , we used angle _9_12_13 and angle _12_9_10 instead of angle _8_12_13 and angle _8_9_10, respectively.

Angle between the joints of the human body. Some unused key points have been omitted.
Angles between joints.
Name | Meaning | Symbol |
angle_0_1_2 | Neck angle (right) | ∠RNeck |
angle_0_1_5 | Neck angle (left) | ∠LNeck |
angle_1_2_3 | Shoulder angle (right) | ∠RShoulder |
angle_1_5_6 | Shoulder angle (left) | ∠LShoulder |
angle_2_3_4 | Elbow angle (right) | ∠RElbow |
angle_5_6_7 | Elbow angle (left) | ∠LElbow |
angle_12_9_10 | Hip angle (right) | ∠RHip |
angle_9_12_13 | Hip angle (left) | ∠LHip |
angle_9_10_11 | Knee angle (right) | ∠RKnee |
angle_12_13_14 | Knee angle (left) | ∠LKnee |
*In the name, angle_a_b_c represents the ∠abc composed of joints a, b, and c, where a, b, and c are key points (or joints) labels.
Wavelet transform
In frequency domain analysis, the Fourier transform is often used to observe signal spectra, but this transform is not suitable for analyzing signals whose frequencies change with time ( Oppenheim et al., 1996 ). The short-time Fourier transform developed on this basis realizes time-frequency localization by adding a moving window function, but a problem remains: the window function cannot change with the frequency ( Kwok and Jones, 2000 ). The wavelet transform overcomes the above shortcomings and can realize multiresolution analysis ( Daubechies, 1992 ). In the gait video, through observing the raw gait data, we found that there are differences in the movement amplitude and frequency of different key points. For example, RWrist (No. 4) has a larger movement amplitude than REye (No. 17), but REye has a faster movement frequency. In order to express the difference in frequency, we chose the wavelet transform and used the “haar” wavelet basis to decompose the gait skeleton coordinate sequence with 5 layers of wavelets. The source signal X is decomposed into:
where D 1 , D 2 , D 3 , D 4 , and D 5 are the high-frequency signals (or detail coefficient arrays) decomposed from the first to fifth layers, respectively, and A 5 denotes the low-frequency signal (or approximate coefficient array) decomposed from the fifth layer.
Feature extraction
For feature construction, we constructed a feature data pool, including gait skeleton coordinates, interframe differences, distances between joints, angles between joints, and frequency domain arrays of wavelet decomposition (left side of Figure 6 ). We extracted the time-frequency domain features based on the feature data pool. In Figure 6 , we used different colors to distinguish the changes of data. Different colors from left to right represented the original coordinate sequences, feature construction, feature extraction functions and extracted features, respectively.

Flow chart of feature extraction.
Time domain feature extraction
Since the gaits included both simple linear motions and complex non-linear processes ( Iqbal et al., 2015 ), we used 10 linear and non-linear functions for time domain feature extraction, as shown in Table 3 . Based on the time series data in the feature data pool, we obtained 1320-dimensional time domain features.
Time domain feature extraction functions.
Feature extraction function | Meaning |
maximum (x) | The maximum value of x. |
minimum (x) | The minimum value of x. |
mean (x) | The mean value of x. |
median (x) | The median of x. |
variance (x) | The variance of x. |
root_mean_square (x) | The root mean square of x. |
skewness (x) | The skewness of x. |
kurtosis (x) | The kurtosis of x. |
abs_energy (x) | The absolute energy of x. |
variation_coefficient (x) | The coefficient of variation of x. |
*x represents time series data.
Frequency domain feature extraction
Due to the complexity of gait, some gait patterns cannot be distinguished in the time domain, but some laws can be reflected in the frequency domain ( Orović et al., 2011 ). We calculated the absolute maximum values, mean values, variances, and absolute energy of the 6 coefficient arrays after wavelet decomposition in the feature data pool and obtained 1152-dimensional frequency domain features.
Compared with the number of participants, the dimensionality of the 2472-dimensional time-frequency features obtained after feature extraction was too high, and this easily led to serious model overfitting ( Bishop, 2006 ). We used principal component analysis (PCA) to reduce the dimensionality of the standardized features. Then we used Sequential Forward Selection (SFS) to select 40 features accordingly based on the properties of different machine learning algorithms in the modeling. SFS added features to form a feature subset in a greedy fashion. At each stage, the estimator (machine learning algorithm) chose the best feature to add based on the cross-validation score of an estimator. While selecting features, the performance of the model was continuously improved. Finally, SFS selected a feature subset containing 40 features for each model. This subset enabled the model to achieve optimal performance.
Personality assessment is a regression task. Different regression algorithms have different characteristics. Linear regression (LR) is often used as a baseline model because of its fast calculation speed, low complexity and easy interpretation. Gaussian process regression (GPR) is widely used in time series analysis, and gait videos are time series data. Random forest regression (RFR) is a typical ensemble algorithm, which can deal with errors caused by imbalanced gait data. Support vector regression (SVR) is effective in high-dimensional gait time-frequency feature space. Therefore, we selected 7 typical machine learning regression algorithms for modeling, namely GPR, LR, RFR, SVR, where the SVR algorithm contains 4 kernel functions: “linear,” “poly,” “rbf,” and “sigmoid.” The kernel function directly determines the final performance of the SVR algorithm, but the selection of an appropriate kernel function has always been an unsolved problem ( Zhou, 2021 ), so we made 4 attempts with the SVR algorithm.
As shown in Figure 7 , the modeling process included three data streams, which contained 75 frames of complete data and odd-even split-half data. We used “all frames” to train the standardization, PCA, SFS, and algorithm models and applied these models to “odd frames” and “even frames.”

Modeling flowchart. The solid arrows indicate the data flows, and the dotted arrows indicate the applications of the model. “Odd frames” and “even frames” were divided from the first 74 frames among “all frames.” PCA, principal component analysis; SFS, sequential forward selection; GPR, Gaussian process regression; LR, linear regression; RFR, random forest regression. SVR-linear, SVR-poly, SVR-rbf, and SVR-sigmoid represent the SVR model using the “linear,” “poly,” “rbf,” and “sigmoid” kernel functions, respectively.
We trained and tested the model with 10 times of 10-fold cross validation and used criterion validity, odd-even split-half reliability, convergent validity, discriminant validity and root mean square error (RMSE) as the model evaluation indicators. RMSE is the most commonly used performance metric in regression tasks ( Zhou, 2021 ) and is defined as:
where Predicted n and Actual n represent the personality prediction score and personality scale score of the nth participant, respectively.
Criterion validity and split-half reliability
Using the BFI-44 scores as ground truth, criterion validity was assessed by examining the Pearson correlation coefficient between the model and scale scores for each personality trait. We chose the odd-even split-half reliability as the reliability indicator. The Pearson correlation coefficient between model prediction scores for the “odd frames” and “even frames” was used to evaluate the odd-even split-half reliability of the model. That is, the correlation coefficient between “odd predicted value” and “even predicted value” in Figure 7 was calculated to indicate reliability.
Convergent and discriminant validity
This study used a multitrait-multimethod matrix to explore the convergent and discriminant validity of the personality assessment models. The matrix was filled by Pearson correlation coefficients and included five personality traits (extraversion, agreeableness, conscientiousness, neuroticism, and openness) and two measurement methods (the BFI-44 scale and personality assessment model). Convergent validity was numerically the same as criterion validity. However, when evaluating convergent validity, the BFI-44 scores were no longer used as the calibration, but the BFI and the model were regarded as a method of measuring personality, respectively. Discriminant validity was assessed by comparing the magnitude of between-trait correlations (e.g., between extraversion and conscientiousness) within models with those within BFI-44.
Statistical analysis
To explore the relationships between the features used for modeling and the gait joint points, we mapped the optimal feature combination selected by SFS to 24 key points (MidHip was the coordinate origin and was eliminated during data preprocessing). For feature engineering, features were constructed based on the gait skeleton coordinates, so the mapping process was the inverse process of feature construction. In statistical analysis, we counted each key point with different weights according to the rules of feature construction, so as to avoid deviations in the results of statistical analysis due to different key points used in constructing features.
In Table 4 , r 1 represents the criterion validity, and r 2 represents the odd-even split-half reliability. Among the 7 algorithm models, the GPR and LR models had the best criterion validity (the mean r 1 values were 0.478 and 0.508, respectively, and their RMSE values were lower than other algorithms), and r 1 was above 0.4 for all personality traits. The other models exhibited unbalanced performance across different personality traits. In addition, except for that of the SVR-poly and RFR models, the split-half reliability of the other models was good, and the mean values of r 2 were above 0.8. In general, the GPR and LR models had the best performance with good criterion validity and split-half reliability, as shown in Table 4 . The results of the remaining models are listed in Supplementary Appendix A of the Supplementary Materials . (The appendices are in the Supplementary Materials , the same below).
Criterion validity and split-half reliability of the GPR and the LR personality assessment models.
Gaussian process regression | Linear regression | |||||
RMSE | RMSE | |||||
Extraversion | 5.416 | 0.442 | 0.834 | 5.512 | 0.427 | 0.831 |
Agreeableness | 4.690 | 0.405 | 0.840 | 4.550 | 0.487 | 0.921 |
Conscientiousness | 6.015 | 0.464 | 0.900 | 6.000 | 0.474 | 0.870 |
Neuroticism | 5.666 | 0.580 | 0.861 | 5.711 | 0.580 | 0.858 |
Openness | 5.435 | 0.500 | 0.770 | 5.305 | 0.574 | 0.802 |
RMSE, root mean squared error. r_1 represents the criterion validity, and r_2 represents the split-half reliability. All correlation coefficients are highly significant (p < 0.001).
In the multitrait-multimethod matrix (as shown in Tables 5 , ,6), 6 ), the bold numbers represent the correlations between different methods for measuring the same trait, the italic numbers represent the correlations between different traits measured by the same method, and the numbers in the rectangular area (except those in bold) represent the correlations between different methods for measuring different traits.
Convergent and discriminant validity of the GPR personality assessment model (GPR-PAM).
GPR-PAM | BFI-44 | |||||||||
E | A | C | N | O | E | A | C | N | O | |
E | ||||||||||
A | ||||||||||
C | ||||||||||
N | ||||||||||
O | ||||||||||
E | 0.082 | 0.155 | −0.241 | 0.100 | ||||||
A | 0.252 | 0.187 | −0.339 | 0.213 | ||||||
C | 0.241 | 0.209 | −0.321 | 0.276 | ||||||
N | −0.301 | –0.222 | −0.243 | −0.281 | ||||||
O | 0.257 | 0.232 | 0.279 | −0.255 |
E, extraversion; A, agreeableness; C, conscientiousness; N, neuroticism; O, openness. All correlation coefficients are highly significant (p < 0.001). Bold numbers represent the correlations between GPR-PAM and BFI-44 for measuring the same trait, the italic numbers represent the correlations between different traits measured by GPR-PAM or BFI-44.
Convergent and discriminant validity of the LR personality assessment model (LR-PAM).
LR-PAM | BFI-44 | |||||||||
E | A | C | N | O | E | A | C | N | O | |
E | ||||||||||
A | ||||||||||
C | ||||||||||
N | ||||||||||
O | ||||||||||
E | 0.118 | 0.192 | −0.240 | 0.116 | ||||||
A | 0.256 | 0.187 | −0.339 | 0.185 | ||||||
C | 0.235 | 0.200 | −0.320 | 0.288 | ||||||
N | −0.331 | –0.335 | −0.268 | −0.260 | ||||||
O | 0.227 | 0.187 | 0.268 | −0.254 |
E, extraversion; A, agreeableness; C, conscientiousness; N, neuroticism; O, openness. All correlation coefficients are highly significant (p < 0.001). Bold numbers represent the correlations between LR-PAM and BFI-44 for measuring the same trait, the italic numbers represent the correlations between different traits measured by LR-PAM or BFI-44.
Among all the models, the average convergence correlation of the optimal LR model was 0.508, and the mono-trait Pearson correlations between the assessment methods were extraversion: r = 0.427; agreeableness: r = 0.487; conscientiousness: r = 0.474; neuroticism: r = 0.580; and openness: r = 0.574 ( Table 6 ). The average convergence correlation of the GPR model ( r GPR _ BFI = 0.478, see Table 5 ) was close to that of the LR model, while that of the other models is poor ( r RFR _ BFI = 0.145, r SVRlinear _ BFI = 0.137, r SVRpoly _ BFI = 0.286, r SVRrbf _ BFI = 0.333, r SVRsigmoid _ BFI = 0.316, see Supplementary Appendix B ). In LR and GPR models, the bold numbers were significantly larger ( p < 0.001) than the values in the same column or row of the rectangular area, which showed that our models had good convergent validity.
The discriminant validity coefficients for each method were shown in italics. The average magnitudes (absolute value; the same below) of the discriminant validity coefficient of models were significantly lower than that of the BFI-44 scale ( r GPR = 0.245, r LR = 0.251, r RFR = 0.069, r SVRlinear = 0.050, r SVRpoly = 0.093, r SVRrbf = 0.164, r SVRsigmoid = 0.098, in the upper left triangle; r BFI = 0.448, in the lower right triangle; p < 0.001) in Tables 5 , ,6 6 and Supplementary Appendix B . Because italics indicated the correlation between different traits, a small correlation coefficient indicated good discriminant validity. This showed that the models were relatively better than the BFI-44 at discriminating between traits. In addition, the average magnitudes of the convergent validity coefficients of the models were significantly greater than the average magnitudes of their discriminant validity coefficients ( r Model _ BFI = r Model , p < 0.001), indicating the models had good discriminant validity. However, the RFR, SVR-linear, SVR-poly, SVR-rbf, and SVR-sigmoid models had poor convergent validity, that is, poor predictive performance, which may lead to large deviations in the above discriminant validity. In summary, the LR and GPR models had relatively good convergent and discriminant validity.
We mapped the features of the GPR and LR models with good reliability and validity to the key points of gait (B and C in Figure 8 ). A dotted line divided 50% of the key points according to their statistics. In the top 50%, the intersection of the two models contained 11 key points, namely, No. 1, No. 3, No. 4, No. 5, No. 6, No. 7, No. 9, No. 10, No. 11, No. 12, and No. 13. We converted the ladder diagram into a heatmap (A and D in Figure 8 ). The larger the statistical value was, the darker the color, which meant that the corresponding key point had a higher contribution rate to modeling. Except for those of the head and feet, the key points of other body parts presented high heat values.

Feature and key point mapping. (B,C) Statistical ladder diagrams of the GPR and LR models. The horizontal axis represents the labels of the key points, the vertical axis represents the statistical values of the key points, and the dotted line represents the 50% key point division line. (A,D) Statistical heatmaps of the GPR and LR models.
The results of this study show that personality assessment models based on gait video have good performance in terms of criterion validity, split-half reliability, convergent validity, and discriminant validity. We constructed static features (i.e., gait skeleton coordinates) and dynamic features (including interframe differences, distances between joints, and angles between joints). Interframe differences reflect the changes between different frames, and the distances and angles between joints reflect the states between the joints in the same frame. This gait feature construction method integrating static and dynamic information has been verified in many studies ( Pratheepan et al., 2009 ; Tsuji et al., 2010 ).
In machine learning, the RMSE is generally used to measure the performance of a model. We found that the mean value of r 1 was inversely proportional to the mean RMSE value for all models (see Table 4 and Supplementary Appendix A ), which shows that it is reasonable to use the criterion validity as an evaluation indicator of model performance. Cronbach’s alpha is the most commonly used reliability statistic. Usually, Cronbach’s alpha is above 0.7, which is considered acceptable reliability ( Cronbach, 1951 ). The alpha coefficient of each trait in BFI-44 is close to 0.8 ( Hongyan et al., 2015 ). For the split-half model containing 74 frames of data, it is impossible to calculate all split-half cases. So we used the odd-even split-half reliability ( VandenBos, 2007 ) approximation as the evaluation index. Except for those of the SVR-poly and RFR models, the mean r 2 values in each dimension of the other models was higher than 0.8 (see Table 4 and Supplementary Appendix A ), indicating that the reliability evaluation of the model was reasonable.
The correlations between the traits in BFI-44 (the lower right italicized areas in Tables 5 , ,6 6 and Supplementary Appendix B ) showed that extraversion, agreeableness, conscientiousness and openness had positive correlations with each other, and they had negative correlations with neuroticism. A similar pattern was found in the correlations between the dimensions of the GPR and LR models (the upper left italicized area in Tables 5 , ,6), 6 ), but no similar patterns were found in the other models. Both reliability and validity studies of the BFI-44 scale ( Carciofo et al., 2016 ) and model-based personality assessment studies ( Park et al., 2015 ) have confirmed the existence of this pattern. Therefore, it has been proven again that the GPR and LR models have good performance in many aspects.
Personality traits are closely related to body movements ( Koppensteiner and Grammer, 2010 ; Thoresen et al., 2012 ). Some studies have shown that agreeableness and pelvic motion, as well as extraversion and thoracic motion, are positively correlated, and conscientiousness and thoracic motion are negatively correlated ( Satchell et al., 2017 ). This is consistent with our results; that is, Neck (No. 1), RShoulder (No. 2) and LShoulder (No. 5) on the thorax and RHip (No. 9) and LHip (No. 12) on the pelvis all yielded high heat values ( Figure 8 ). In addition, during walking, certain differences are observed between arm swings and leg strides ( DeVita et al., 1991 ). In our results, there were differences between RWrist (No. 4) and LWrist (No. 7) on the hands and between Rankle (No. 11) and LAnkle (No. 14) on the feet ( Figure 8 ). We found that the key points of the head and feet presented low heat values, which might be due to the fact that the changes in these parts (relative changes between internal key points) were not obvious compared with the limbs and trunk, with little individual differences. In previous studies on the relationship between personality and gait ( Tolea et al., 2012 ; Wang et al., 2016 ; Satchell et al., 2017 ; Stephan et al., 2018 ), gait mainly focused on the limbs and trunk with a large range of motion. The above shows that our models learned some of the kinematic characteristics of gait. However, we have only initially explored the interpretability of personality assessment models from one perspective. Further research is needed in the future.
Our research purpose was not to find a machine learning algorithm but to explore the feasibility of predicting personality based on gait video through machine learning modeling and to provide a new idea and method for automatic personality assessment. According to a literature exploration and our knowledge, this study is the first to measure the reliability and validity of machine learning models in the field of automatic personality assessment using gait. In multidimensional studies, measuring the reliability and validity of machine learning models helps to ensure that a model can truly discover the patterns of corresponding traits; this could not be achieved by previous machine learning evaluation methods.
Our study is still in its infancy and cannot completely replace a personality scale, but it has good prospects. Our method needs only 3 s of effective gait video to efficiently and conveniently realize personality assessment. Personality traits are highly stable after adulthood ( Cobb-Clark and Schurer, 2012 ) and are closely related to health statuses ( Jokela et al., 2013 ), occupational tendencies ( Kern et al., 2019 ), and academic achievements ( Komarraju et al., 2011 ). In future job searches, enrollments and other occasions, it will be necessary to make personality assessments for the examinee. By using our method, an evaluation result can be quickly obtained without interference and used to assist the examiner in decision making.
This study exhibits some limitations. First, the educational levels of the participants in the experiment were concentrated in the postgraduate stage, and the relatively concentrated cultural level of the group may have caused their personalities to be similar. Second, deep learning algorithms may improve model performance to a certain extent, but the number of participants in our study was small. Third, we used a single camera to obtain gait videos of participants’ fronts, backs and turns while they walked back and forth. However, only the frontal gait was used in the experiment, which led to data waste. In future studies, we will expand the scope and scale of recruitment, invite participants with large individual differences, and improve our research algorithms and data collection methods.
This study moves one step forward toward a non-invasive and low-cost personality assessment solution, which will have potential value in personality-related psychological intervention and behavioral decision making. Our experiments show that Big Five personality assessment models based on gait video have good criterion validity, split-half reliability, convergent validity, and discriminant validity. Our preliminary research provides new ideas for evaluating the performance of machine learning models with multidimensional psychological characteristics and points out a possible direction for constructing convenient personality assessment methods.
Data availability statement
Ethics statement.
The studies involving human participants were reviewed and approved by the Institutional Review Board of the Institute of Psychology, Chinese Academy of Sciences (approval number: {"type":"entrez-nucleotide","attrs":{"text":"H15010","term_id":"879830"}} H15010 ). The patients/participants provided their written informed consent to participate in this study.
Author contributions
YW, BL, TZ, and DC contributed to conception and design of the study. TZ collected and provided the data and directed the writing and research process of this manuscript. YW analyzed the data and wrote the first draft of the manuscript. DC and BL proposed improvements to the research method. All authors participated in the editing and reviewing of manuscripts, contributed to the article, and approved the submitted version.
This research was funded by the Key Research Program of the Chinese Academy of Sciences (No. ZDRW-XH-2019-4) and the Fundamental Research Funds for the Central Universities (No. E0E48922X2).
Conflict of interest
The authors declare that the research was conducted in the absence of any commercial or financial relationships that could be construed as a potential conflict of interest.
Publisher’s note
All claims expressed in this article are solely those of the authors and do not necessarily represent those of their affiliated organizations, or those of the publisher, the editors and the reviewers. Any product that may be evaluated in this article, or claim that may be made by its manufacturer, is not guaranteed or endorsed by the publisher.
Supplementary material
The Supplementary Material for this article can be found online at: https://www.frontiersin.org/articles/10.3389/fnbeh.2022.901568/full#supplementary-material
- Agmon M., Armon G. A. (2016). Cross-sectional study of the association between mobility test performance and personality among older adults. BMC Geriatr. 16 : 105 . 10.1186/s12877-016-0272-8 [ PMC free article ] [ PubMed ] [ CrossRef ] [ Google Scholar ]
- Baker R., Hart H. M. (2013). Measuring Walking: A Handbook of Clinical Gait Analysis. London: Mac Keith Press. [ Google Scholar ]
- Baumert A., Schmitt M., Perugini M., Johnson W., Blum G., Borkenau P., et al. (2017). Integrating personality structure, personality process, and personality development. Eur. J. Pers. 31 503–528. 10.1002/per.2115 [ CrossRef ] [ Google Scholar ]
- Bing M. N., LeBreton J. M., Davison H. K., Migetz D. Z., James L. R. (2007). Integrating implicit and explicit social cognitions for enhanced personality assessment: a general framework for choosing measurement and statistical methods. Organ. Res. Methods 10 136–179. 10.1177/1094428106289396 [ CrossRef ] [ Google Scholar ]
- Bishop C. M. (2006). Pattern Recognition and Machine Learning. New York, NY: Springer. [ Google Scholar ]
- Briley D. A., Tucker-Drob E. M. (2014). Genetic and environmental continuity in personality development: a meta-analysis. Psychol. Bull. 140 1303–1331. 10.1037/a0037091 [ PMC free article ] [ PubMed ] [ CrossRef ] [ Google Scholar ]
- Cao Z., Hidalgo G., Simon T., Wei S. E., Sheikh Y. (2021). Openpose: realtime multi-person 2d pose estimation using part affinity fields. IEEE Trans. Pattern Anal. Mach. Intell. 43 172–186. 10.1109/tpami.2019.2929257 [ PubMed ] [ CrossRef ] [ Google Scholar ]
- Carciofo R., Yang J., Song N., Du F., Zhang K. (2016). Psychometric evaluation of Chinese-Language 44-item and 10-item big five personality inventories, including correlations with chronotype, mindfulness and mind wandering. PLoS One 11 : e0149963 . 10.1371/journal.pone.0149963 [ PMC free article ] [ PubMed ] [ CrossRef ] [ Google Scholar ]
- Cobb-Clark D. A., Schurer S. (2012). The stability of big-five personality traits. Econ. Lett. 115 11–15. 10.1016/j.econlet.2011.11.015 [ CrossRef ] [ Google Scholar ]
- Corr P. J., Matthews G. E. (2020). The Cambridge Handbook of Personality Psychology. Cambridge, MA: Cambridge University Press. [ Google Scholar ]
- Costa P. T., Jr., McCrae R. R. (1980). Influence of Extraversion and neuroticism on subjective well-being: happy and unhappy people. J. Pers. Soc. Psychol. 38 668–678. 10.1037//0022-3514.38.4.668 [ PubMed ] [ CrossRef ] [ Google Scholar ]
- Cronbach L. J. (1951). Coefficient alpha and the internal structure of tests. Psychometrika 16 297–334. 10.1007/BF02310555 [ CrossRef ] [ Google Scholar ]
- Daubechies I. (1992). Ten Lectures on Wavelets. Philadelphia, PA: Society for Industrial and Applied Mathematics. [ Google Scholar ]
- Davis R. B., Õunpuu S., Tyburski D., Gage J. R. A. (1991). Gait analysis data collection and reduction technique. Hum. Move. Sci. 10 575–587. 10.1016/0167-9457(91)90046-Z [ CrossRef ] [ Google Scholar ]
- DeVita P., Hong D., Hamill J. (1991). Effects of asymmetric load carrying on the biomechanics of walking. J. Biomech. 24 1119–1129. 10.1016/0021-9290(91)90004-7 [ PubMed ] [ CrossRef ] [ Google Scholar ]
- Donker S. F., Mulder T., Nienhuis B., Duysens J. (2002). Adaptations in arm movements for added mass to wrist or ankle during walking. Exp. Brain Res. 146 26–31. 10.1007/s00221-002-1145-2 [ PubMed ] [ CrossRef ] [ Google Scholar ]
- Fang J., Wang T., Li C., Hu X., Ngai E., Seet B. C., et al. (2019). Depression prevalence in postgraduate students and its association with gait abnormality. IEEE Access. 7 174425–174437. 10.1109/ACCESS.2019.2957179 [ CrossRef ] [ Google Scholar ]
- Hofmann M., Geiger J., Bachmann S., Schuller B., Rigoll G. (2014). The tum gait from audio, image and depth (Gaid) database: multimodal recognition of subjects and traits. J. Vis. Commun. Image Represent. 25 195–206. 10.1016/j.jvcir.2013.02.006 [ CrossRef ] [ Google Scholar ]
- Holland A. S., Roisman G. I. (2008). Big five personality traits and relationship quality: self-reported, observational, and physiological evidence. J. Soc. Pers. Relat. 25 811–829. 10.1177/0265407508096697 [ CrossRef ] [ Google Scholar ]
- Hongyan L., Jianping X., Jiyue C., Yexin F. A. (2015). Reliability meta-analysis for 44 items big five inventory: based on the reliability generalization methodology. Adv. Psychol. Sci. 23 755–765. 10.3724/sp.J.1042.2015.00755 [ CrossRef ] [ Google Scholar ]
- Iida H., Yamamuro T. (1987). Kinetic analysis of the center of gravity of the human body in normal and pathological gaits. J. Biomech. 20 987–995. 10.1016/0021-9290(87)90328-9 [ PubMed ] [ CrossRef ] [ Google Scholar ]
- Iqbal S., Zang X., Zhu Y., Saad H. M. A. A., Zhao J. (eds) (2015). “ Nonlinear time-series analysis of different human walking gaits ,” in Proceedings of the 2015 IEEE International Conference on Electro/Information Technology (EIT) , (Dekalb, IL: IEEE; ). [ Google Scholar ]
- Iwasa H., Masui Y., Gondo Y., Inagaki H., Kawaai C., Suzuki T. (2008). Personality and all-cause mortality among older adults dwelling in a japanese community: a five-year population-based prospective cohort study. Am. J. Geriatr. Psychiatry 16 399–405. 10.1097/JGP.0b013e3181662ac9 [ PubMed ] [ CrossRef ] [ Google Scholar ]
- John O. P., Donahue E. M., Kentle R. L. (1991). Big five inventory. Berkeley, CA: University of California, Berkeley, Institute of Personality and Social Research. 10.1037/t07550-000 [ CrossRef ] [ Google Scholar ]
- Jokela M., Hintsanen M., Hakulinen C., Batty G. D., Nabi H., Singh-Manoux A., et al. (2013). Association of personality with the development and persistence of obesity: a meta-analysis based on individual-participant data. Obes. Rev. 14 315–323. 10.1111/obr.12007 [ PMC free article ] [ PubMed ] [ CrossRef ] [ Google Scholar ]
- Kern M. L., McCarthy P. X., Chakrabarty D., Rizoiu M. A. (2019). Social media-predicted personality traits and values can help match people to their ideal jobs. Proc. Natl. Acad. Sci. U.S.A. 116 26459–26464. 10.1073/pnas.1917942116 [ PMC free article ] [ PubMed ] [ CrossRef ] [ Google Scholar ]
- Komarraju M., Karau S. J., Schmeck R. R., Avdic A. (2011). The big five personality traits, learning styles, and academic achievement. Pers. Individ. Dif. 51 472–477. 10.1016/j.paid.2011.04.019 [ CrossRef ] [ Google Scholar ]
- Koppensteiner M., Grammer K. (2010). Motion patterns in political speech and their influence on personality ratings. J. Res. Pers. 44 374–379. 10.1016/j.jrp.2010.04.002 [ CrossRef ] [ Google Scholar ]
- Kwok H. K., Jones D. L. (2000). Improved instantaneous frequency estimation using an adaptive short-time fourier transform. IEEE Trans. Signal Process. 48 2964–2972. 10.1109/78.869059 [ CrossRef ] [ Google Scholar ]
- Leardini A., Benedetti M. G., Berti L., Bettinelli D., Nativo R., Giannini S. (2007). Rear-foot, mid-foot and fore-foot motion during the stance phase of gait. Gait Posture 25 453–462. 10.1016/j.gaitpost.2006.05.017 [ PubMed ] [ CrossRef ] [ Google Scholar ]
- LeMonda B. C., Mahoney J. R., Verghese J., Holtzer R. (2015). The association between high neuroticism-low extraversion and dual-task performance during walking while talking in non-demented older adults. J. Int. Neuropsychol. Soc. 21 519–530. 10.1017/s1355617715000570 [ PMC free article ] [ PubMed ] [ CrossRef ] [ Google Scholar ]
- Luyckx K., Daelemans W. (2010). The effect of author set size and data size in authorship attribution. Lit Linguist. Comput. 26 35–55. 10.1093/llc/fqq013 [ CrossRef ] [ Google Scholar ]
- Matsumoto D. E., Hwang H. C., Frank M. G. (2015). Apa Handbook of Nonverbal Communication. Washington, DC: American Psychological Association. [ Google Scholar ]
- Minetti A. E. (1998). The biomechanics of skipping gaits: a third locomotion paradigm? Proc. Biol. Sci. 265 1227–1235. 10.1098/rspb.1998.0424 [ PMC free article ] [ PubMed ] [ CrossRef ] [ Google Scholar ]
- Mündermann L., Corazza S., Andriacchi T. P. (2006). The evolution of methods for the capture of human movement leading to markerless motion capture for biomechanical applications. J. Neuroeng. Rehabil. 3 : 6 . 10.1186/1743-0003-3-6 [ PMC free article ] [ PubMed ] [ CrossRef ] [ Google Scholar ]
- Murray M. P. (1967). Gait as a total pattern of movement. Am. J. Phys. Med. 46 290–333. [ PubMed ] [ Google Scholar ]
- O’Connor C. M., Thorpe S. K., O’Malley M. J., Vaughan C. L. (2007). Automatic detection of gait events using kinematic data. Gait Posture 25 469–474. 10.1016/j.gaitpost.2006.05.016 [ PubMed ] [ CrossRef ] [ Google Scholar ]
- Ones D. S., Viswesvaran C., Reiss A. D. (1996). Role of social desirability in personality testing for personnel selection: the red herring. J. Appl. Psychol. 81 : 660 . [ Google Scholar ]
- Oppenheim A. V., Willsky A. S., Nawab S. H., Hernández G. M. (1996). Signals and Systems. London: Pearson. [ Google Scholar ]
- Orović I., Stanković S., Amin M. A. (2011). New approach for classification of human gait based on time-frequency feature representations. Signal Process. 91 1448–1456. 10.1016/j.sigpro.2010.08.013 [ CrossRef ] [ Google Scholar ]
- Park G., Schwartz H. A., Eichstaedt J. C., Kern M. L., Kosinski M., Stillwell D. J., et al. (2015). Automatic personality assessment through social media Language. J. Pers. Soc. Psychol. 108 934–952. 10.1037/pspp0000020 [ PubMed ] [ CrossRef ] [ Google Scholar ]
- Park J. (2008). Synthesis of natural arm swing motion in human bipedal walking. J. Biomech. 41 1417–1426. 10.1016/j.jbiomech.2008.02.031 [ PubMed ] [ CrossRef ] [ Google Scholar ]
- Pratheepan Y., Condell J. V., Prasad G. (eds) (2009). “ The use of dynamic and static characteristics of gait for individual identification ,” in Proceedings of the 2009 13th International Machine Vision and Image Processing Conference , (Dublin: IEEE; ). [ Google Scholar ]
- Rammstedt B., John O. P. (2007). Measuring personality in one minute or less: a 10-item short version of the big five inventory in English and German. J. Res. Pers. 41 203–212. 10.1016/j.jrp.2006.02.001 [ CrossRef ] [ Google Scholar ]
- Satchell L., Morris P., Mills C., O’Reilly L., Marshman P., Akehurst L. (2017). Evidence of big five and aggressive personalities in gait biomechanics. J. Nonverbal. Behav. 41 35–44. 10.1007/s10919-016-0240-1 [ PMC free article ] [ PubMed ] [ CrossRef ] [ Google Scholar ]
- Seel T., Raisch J., Schauer T. (2014). Imu-based joint angle measurement for gait analysis. Sensors (Basel) 14 6891–6909. 10.3390/s140406891 [ PMC free article ] [ PubMed ] [ CrossRef ] [ Google Scholar ]
- Seibert S. E., Kraimer M. L., Crant J. M. (2001). What do proactive people do? A longitudinal model linking proactive personality and career success. Pers. Psychol. 54 845–874. 10.1111/j.1744-6570.2001.tb00234.x [ CrossRef ] [ Google Scholar ]
- Singh J. P., Jain S., Arora S., Singh U. P. (2018). Vision-based gait recognition: a survey. IEEE Access. 6 70497–70527. 10.1109/ACCESS.2018.2879896 [ CrossRef ] [ Google Scholar ]
- Springer S., Yogev Seligmann G. (2016). Validity of the kinect for gait assessment: a focused review. Sensors (Basel) 16 : 194 . 10.3390/s16020194 [ PMC free article ] [ PubMed ] [ CrossRef ] [ Google Scholar ]
- Stephan Y., Sutin A. R., Bovier-Lapierre G., Terracciano A. (2018). Personality and walking speed across adulthood: prospective evidence from five samples. Soc. Psychol. Pers. Sci. 9 773–780. 10.1177/1948550617725152 [ CrossRef ] [ Google Scholar ]
- Sun B., Zhang Z., Liu X., Hu B., Zhu T. (2017). Self-esteem recognition based on gait pattern using kinect. Gait Posture 58 428–432. 10.1016/j.gaitpost.2017.09.001 [ PubMed ] [ CrossRef ] [ Google Scholar ]
- Sun J., Wang Y., Li J., Wan W., Cheng D., Zhang H. (2018). View-Invariant gait recognition based on kinect skeleton feature. Multimed. Tools Appl. 77 24909–24935. 10.1007/s11042-018-5722-1 [ CrossRef ] [ Google Scholar ]
- Sun J., Wu P., Shen Y., Yang Z., Li H., Liu Y., et al. (eds) (2019). “ Relationship between personality and gait: predicting personality with gait features ,” in Proceedings of the 2018 IEEE International Conference on Bioinformatics and Biomedicine (BIBM) , (Madrid: IEEE; ). [ Google Scholar ]
- Tao W., Liu T., Zheng R., Feng H. (2012). Gait analysis using wearable sensors. Sensors (Basel) 12 2255–2283. 10.3390/s120202255 [ PMC free article ] [ PubMed ] [ CrossRef ] [ Google Scholar ]
- Thoresen J. C., Vuong Q. C., Atkinson A. P. (2012). First impressions: gait cues drive reliable trait judgements. Cognition 124 261–271. 10.1016/j.cognition.2012.05.018 [ PubMed ] [ CrossRef ] [ Google Scholar ]
- Tolea M. I., Costa P. T., Jr., Terracciano A., Ferrucci L., Faulkner K., Coday M. M., et al. (2012). Associations of openness and conscientiousness with walking speed decline: findings from the health, aging, and body composition study. J. Gerontol. B Psychol. Sci. Soc. Sci. 67 705–711. 10.1093/geronb/gbs030 [ PMC free article ] [ PubMed ] [ CrossRef ] [ Google Scholar ]
- Tsuji A., Makihara Y., Yagi Y. (eds) (2010). “ Silhouette transformation based on walking speed for gait identification ,” in Proceedings of the 2010 IEEE Computer Society Conference on Computer Vision and Pattern Recognition , (San Francisco, CA: IEEE; ). [ Google Scholar ]
- VandenBos G. R. (2007). Apa Dictionary of Psychology. Washington, DC: American Psychological Association. [ Google Scholar ]
- Wan C., Wang L., Phoha V. V. (2018). A survey on gait recognition. ACM Comput. Surv. 51 : 5 . 10.1145/3230633 [ CrossRef ] [ Google Scholar ]
- Wang Y., Tree J. E. F., Walker M., Neff M. (2016). Assessing the impact of hand motion on virtual character personality. ACM Trans. Appl. Percept. 13 : 2 . 10.1145/2874357 [ CrossRef ] [ Google Scholar ]
- Whittle M. W. (1997). Three-dimensional motion of the center of gravity of the body during walking. Hum. Move. Sci. 16 347–355. 10.1016/S0167-9457(96)00052-8 [ CrossRef ] [ Google Scholar ]
- Whittle M. W. (2014). Gait Analysis: An Introduction. Oxford: Butterworth-Heinemann. [ Google Scholar ]
- Yeye W., Deyuan C., Baobin L., Xiaoyang W., Xiaoqian L., Tingshao Z. (2020). Predicting personality based on self-introduction video. IFAC PapersOnLine 53 452–457. 10.1016/j.ifacol.2021.04.217 [ CrossRef ] [ Google Scholar ]
- Zhao N., Zhang Z., Wang Y., Wang J., Li B., Zhu T., et al. (2019). See your mental state from your walk: recognizing anxiety and depression through kinect-recorded gait data. PLoS One 14 : e0216591 . 10.1371/journal.pone.0216591 [ PMC free article ] [ PubMed ] [ CrossRef ] [ Google Scholar ]
- Zhou Z.-H. (2021). Machine Learning. Singapore: Springer. [ Google Scholar ]
Test project uses AI system to improve transit accessibility in Chattanooga
Lucas Johnson
Jun 18, 2024, 4:08 PM
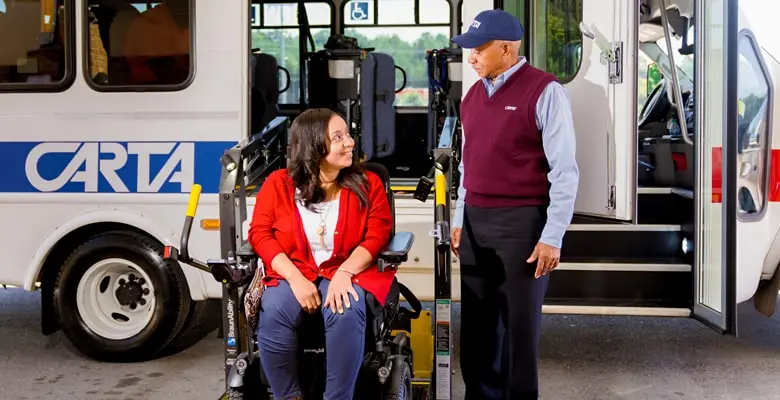
Vanderbilt researchers have developed an innovative software system incorporating artificial intelligence that aims to improve the efficiency of public transportation for individuals with special needs.
The research, led by Abhishek Dubey , associate professor of computer science and electrical and computer engineering, will be presented in a paper at the International Joint Conference on Artificial Intelligence (IJCAI) in August.
In the paper, Dubey and his team discuss their work with the Chattanooga Area Regional Transportation Authority (CARTA) that started in 2020 to improve the operation of its paratransit service, a critical component of traditional transit services that offers door-to-door assistance for people who face challenges using standard transit routes. Pick-up and drop-off times for those individuals must also be adhered to under federal regulations.
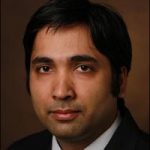
However, like other transit systems across the country, CARTA has struggled operationally because of decreasing ridership and increasing operational costs. To improve efficiency, the team developed a set of data-driven optimization modules that incorporates AI to handle online booking, day-ahead scheduling, and real-time requests received by CARTA’s paratransit fleet for routes in the Chattanooga region. Recently, the team has also started running tests with a microtransit version of the system that will be open for general public.
Results from a test of the SmartTransit system that Dubey and his team developed showed significantly fewer detour miles and a higher percentage of trips with more than one passenger, thus reducing the total number of miles the vehicles must drive, researchers said. Another area of improvement was in the generation of manifests, a sequence of pick-ups and drop-offs assigned to each vehicle.
“The CARTA operators revealed that the algorithm closely resembled the manifests generated by hand, and even more crucial, the algorithm took a minute to generate the manifests, whereas CARTA operators took two weeks to generate the manifests by hand,” said Dubey, senior research scientist at the Institute for Software Integrated Systems (ISIS) . “To the best of our knowledge, this work presents one of the first examples of using open-source algorithmic approaches for paratransit optimization.”
Co-author David Rogers , a research engineer with ISIS, said the system prioritizes the needs of dispatchers, drivers, and riders.
“We maintain continuous communication with CARTA personnel to ensure our solutions are both practical and beneficial for all stakeholders,” said Rogers.
The system is continuing to be tested, but Philip Pugliese, CARTA’s general manager of planning and grants, said its results are promising.
“The project has identified some key opportunities to improve service,” said Pugliese. “We look forward to continued development and implementation.”
Other Vanderbilt authors on the SmartTransit paper are Ayan Mukhopadhyay, Sophie Pavia , Jacob Buckelew , and Samir Gupta . Vanderbilt also collaborated with Professor Aron Laszka from Pennsylvania State University and Professor Samitha Samaranayake from Cornell University. CARTA’s Pugliese is also part of the team.
The project was funded through a grant from the National Science Foundation.
Recently, Dubey and another team developed an AI system to help improve operations of Nashville’s public transportation network that won “Best Paper” at the 15th ACM/IEEE International Conference on Cyber-Physical Systems (ICCPS) , held in Hong Kong May 13-16.
Contact: Lucas Johnson, [email protected]
Explore Story Topics
- Computer Science
- Electrical and Computer Engineering
- Home Features
- Uncategorized
- Abhishek Dubey
- artificial intelligence
- Chattanooga Area Regional Transportation Authority
- Institute for Software Integrated Systems

COMMENTS
3. Sources of Test-Score Variance. Cronbach [], following Thorndike [] (see also []), classified the sources of variance in test scores into the dimensions temporary vs. lasting, and general vs. specific individual characteristics.Lasting characteristics include personality, both lasting general ("attitudes, emotional reactions, or habits generally operating in situations like the test ...
Abstract. This review paper on the current status of the studies on personality traits, aimed at summarizing the progress achieved in the study of personality traits and examining the evidences ...
The Big Five Personality T raits. Personality traits include relatively stable patterns of cognitions, beliefs, and behaviors. The Big Five model has functioned as the powerful theoretical ...
Personality traits can be defined as broad patterns of thoughts, feelings, and behaviors (Lucas & Donnellan, 2011).Early empirical research on personality mainly focused on the structure, measurement, and consequences of traits (e.g., Digman, 1990).Stability and change in traits were less common topics, largely because traits were regarded as highly stable once people reach adulthood (McCrae ...
validity. There is limited, but growing, research on each of these methods that may offer new and improved ways of assessing personality. Test publishers and consultants report that their clients, interested in assessment, are eager to exploit the new technologies irrespective of there being good evidence of their reliability and validity.
Existing studies have revealed the links between objective facial picture cues and general personality traits based on the Five-Factor Model or the Big Five (BF) model of personality 40. However ...
the Rorschach Inkblot Test and the Draw-A-Person Test in clinical assessment and diagnosis, there is little sound empirical support for either m easure (e.g., Lilienfeld, Wood, & Garb, 2000, 2001).
1. Introduction. Research testing the differentiation of personality by intelligence hypothesis has had varied results. Following the finding of greater differentiation in cognitive ability measures for those individuals who were more intelligent (Spearman 1927; see also Deary et al. 1996; Deary and Pagliari 1991; Detterman and Daniel 1989; Lynn 1992), Brand et al. suggested that the ...
Personality traits can be defined as relatively stable patterns of thoughts, feelings and behaviours on which people differ (McCrae & Costa, Citation 1995).They are frequently used by researchers to describe and classify individuals (e.g., optimistic, ambitious, aggressive, etc.) often in order to explain and predict some external phenomena of interest (e.g., academic performance, Poropat ...
Our review of the relevant research literature in the recent past strongly supports the view that personality assessment has high utility in the workplace. We review the evidence that personality assessment measures, especially those based upon the Big Five factors of personality, can effectively predict job performance and thus can be used for personnel selection. The validity of integrity ...
Looking at current practice, selection research on personality traits has neglected two important points that might explain these findings. First, selection research usually focuses on the prediction of task performance, but personality traits have been shown to be better at predicting non-task performance (Gonzalez-Mulé et al., 2014).
About the journal. Emphasizing experimental and descriptive research, the Journal of Research in Personality presents articles that examine important issues in the field of personality and in related fields basic to the understanding of personality. The subject matter includes treatments of genetic, physiological, …. View full aims & scope.
Abstract. Personality tests are being added to large panel studies with increasing regularity, such as the Health and Retirement Study (HRS). To facilitate the inclusion and interpretation of these tests, we provide some general background on personality psychology, personality assessment, and the validity of personality tests.
The Myers-Briggs Type Indicator (MBTI®), published by CPP, Inc., is a forced-choice. instrument designed to examine preferences in how respondents see the world and make. decisions. Scores are ...
Our informants generally had a positive experience of the personality test. Previous research has documented that personality tests are often experienced as neutral (Gilliland & Steiner, Citation 2012; Steiner & Gilliland, Citation 2001) or even negative by the applicants (Hausknecht et al., Citation 2004).
The goal of the present research is to evaluate the usefulness of implementing such tools in the selection process of future physicians. ... The personality traits were evaluated using the Big Five Personality Test, a commonly used test in clinical psychology. ... HanoiISBN: 978-1-943579-61-7 Hai Phong - . . Paper ID: VM714. 18-19, August ...
The Myers-Briggs Type Indicator (MBTI) is well-known in psychology and related fields as a self-report questionnaire. Its development relied on Jung's seminal ideas on psychological types as a framework to describe human personality ( Jung, 1923 ). Nowadays, MBTI is a tool that provides a variety of practical purposes.
The DISC test of personality developed by Merenda and Clarke (1965) is a very popular personality self-assessment used primarily within the corporate world. It is based on the emotional and behavioral DISC theory (Marston, 1928), which measures individuals on four dimensions of behavior:
Personality research has had a contentious history, and there are still vestiges of doubt about the importance of personality traits. ... We thus invite new research to test and document how personality traits "work" to shape life outcomes. ... Preparation of this paper was supported by National Institute of Aging Grants AG19414 and AG20048 ...
Our career test items were developed by a team of I/O psychologists with years of experience in the field of psychometrics. Career data comes from numerous sources, including the the US Department of Labour's O*Net Database, US Bureau of Statistics, UK government, CareerExplorer user data, and Sokanu's proprietary in-house content team.
Follow six rules: Ground your culture in the dilemmas you are likely to confront, dilemma-test your values, communicate your values in colorful terms, hire people who fit, let culture drive ...
This paper suggests an archetypical personality test as a tool to uncover archetypical roles in innovative teams. ... the result of this research is an archetypical personality test with content ...
Coley is joined on the paper by lead author Jenna Fromer SM '24. The research appears today in Nature Computational Science. Complex cost considerations. In a sense, whether a scientist should synthesize and test a certain molecule boils down to a question of the synthetic cost versus the value of the experiment.
With the use of LIWC, Raje & Singh (2017) revealed that judging type personality are positively correlated to "work oriented" and "achievement focus" category, and negatively correlated to "leisure oriented". Word2Vec is a popular technique to learn word embeddings using shallow neural network that is developed by Mikolov et al. (2013).
The application window opened on June 19 and will close on July 9. Application form corrections will be available from July 10 to 11. The examination will be held on July 31 in computer-based test ...
The Big Five—Extraversion, Agreeableness, Conscientiousness, Neuroticism and Openness to Experience— are a set of five. broad, bipolar trait dimensions that constitute the most widely used ...
Transform how you communicate using intelligent Writing Tools that can proofread your text, rewrite different versions until the tone and wording are just right, and summarize selected text with a tap. Writing Tools are available nearly everywhere you write, including third-party apps.
Gait is a natural, stable, and easy-to-observe body movement that is closely related to personality. The purpose of this paper is to propose a personality assessment model based on gait video and evaluate the reliability and validity of the multidimensional model. This study recruited 152 participants and used cameras to record their gait videos.
Vanderbilt researchers have developed an innovative software system incorporating artificial intelligence that aims to improve the efficiency of public transportation for individuals with special needs. The research, led by Abhishek Dubey, associate professor of computer science and electrical and computer engineering, will be presented in a paper at the International Joint Conference on ...
This paper suggests an archetypical personality test as a tool to uncover archetypical roles in innovative teams. ... the result of this research is an archetypical personality test with content ...