News alert: UC Berkeley has announced its next university librarian

Secondary menu
- Log in to your Library account
- Hours and Maps
- Connect from Off Campus
- UC Berkeley Home
Search form
Research methods--quantitative, qualitative, and more: overview.
- Quantitative Research
- Qualitative Research
- Data Science Methods (Machine Learning, AI, Big Data)
- Text Mining and Computational Text Analysis
- Evidence Synthesis/Systematic Reviews
- Get Data, Get Help!
About Research Methods
This guide provides an overview of research methods, how to choose and use them, and supports and resources at UC Berkeley.
As Patten and Newhart note in the book Understanding Research Methods , "Research methods are the building blocks of the scientific enterprise. They are the "how" for building systematic knowledge. The accumulation of knowledge through research is by its nature a collective endeavor. Each well-designed study provides evidence that may support, amend, refute, or deepen the understanding of existing knowledge...Decisions are important throughout the practice of research and are designed to help researchers collect evidence that includes the full spectrum of the phenomenon under study, to maintain logical rules, and to mitigate or account for possible sources of bias. In many ways, learning research methods is learning how to see and make these decisions."
The choice of methods varies by discipline, by the kind of phenomenon being studied and the data being used to study it, by the technology available, and more. This guide is an introduction, but if you don't see what you need here, always contact your subject librarian, and/or take a look to see if there's a library research guide that will answer your question.
Suggestions for changes and additions to this guide are welcome!
START HERE: SAGE Research Methods
Without question, the most comprehensive resource available from the library is SAGE Research Methods. HERE IS THE ONLINE GUIDE to this one-stop shopping collection, and some helpful links are below:
- SAGE Research Methods
- Little Green Books (Quantitative Methods)
- Little Blue Books (Qualitative Methods)
- Dictionaries and Encyclopedias
- Case studies of real research projects
- Sample datasets for hands-on practice
- Streaming video--see methods come to life
- Methodspace- -a community for researchers
- SAGE Research Methods Course Mapping
Library Data Services at UC Berkeley
Library Data Services Program and Digital Scholarship Services
The LDSP offers a variety of services and tools ! From this link, check out pages for each of the following topics: discovering data, managing data, collecting data, GIS data, text data mining, publishing data, digital scholarship, open science, and the Research Data Management Program.
Be sure also to check out the visual guide to where to seek assistance on campus with any research question you may have!
Library GIS Services
Other Data Services at Berkeley
D-Lab Supports Berkeley faculty, staff, and graduate students with research in data intensive social science, including a wide range of training and workshop offerings Dryad Dryad is a simple self-service tool for researchers to use in publishing their datasets. It provides tools for the effective publication of and access to research data. Geospatial Innovation Facility (GIF) Provides leadership and training across a broad array of integrated mapping technologies on campu Research Data Management A UC Berkeley guide and consulting service for research data management issues
General Research Methods Resources
Here are some general resources for assistance:
- Assistance from ICPSR (must create an account to access): Getting Help with Data , and Resources for Students
- Wiley Stats Ref for background information on statistics topics
- Survey Documentation and Analysis (SDA) . Program for easy web-based analysis of survey data.
Consultants
- D-Lab/Data Science Discovery Consultants Request help with your research project from peer consultants.
- Research data (RDM) consulting Meet with RDM consultants before designing the data security, storage, and sharing aspects of your qualitative project.
- Statistics Department Consulting Services A service in which advanced graduate students, under faculty supervision, are available to consult during specified hours in the Fall and Spring semesters.
Related Resourcex
- IRB / CPHS Qualitative research projects with human subjects often require that you go through an ethics review.
- OURS (Office of Undergraduate Research and Scholarships) OURS supports undergraduates who want to embark on research projects and assistantships. In particular, check out their "Getting Started in Research" workshops
- Sponsored Projects Sponsored projects works with researchers applying for major external grants.
- Next: Quantitative Research >>
- Last Updated: Apr 3, 2023 3:14 PM
- URL: https://guides.lib.berkeley.edu/researchmethods

Top Science News
Latest top headlines.
- Fibromyalgia
- Personalized Medicine
- Parkinson's Research
- Parkinson's
- Disorders and Syndromes
- Diseases and Conditions
- Immune System
- Crohn's Disease
- Black Holes
- Solar Flare
- Astrophysics
- Extrasolar Planets
- Computers and Internet
- Quantum Computers
- Information Technology
- Wild Animals
- Mating and Breeding
- New Species
- Ozone Holes
- Global Warming
- Oceanography
- Snow and Avalanches
- Ancient Civilizations
- Genes for Strong Muscles: Healthy Long Life
- Parkinson's: New Theory On Origins and Spread
- Physical Activity Best in the Evening
- How the Body Switches out of 'Fight' Mode
Top Physical/Tech
- Brightest Gamma-Ray Burst
- Stellar Winds of Three Sun-Like Stars Detected
- Clash of Stars Solves Stellar Mystery
- Secure Quantum Computing at Home
Top Environment
- Fences Causing Genetic Problems for Mammals
- Ozone Removes Mating Barriers Between Fly ...
- Ocean Currents: Collapse of Antarctic Ice ...
- Pacific Cities Much Older Than Previously ...
Health News
Latest health headlines.
- Breast Cancer
- Colon Cancer
- Skin Cancer
- Lung Cancer
- Medical Topics
- Schizophrenia
- Birth Defects
- Brain-Computer Interfaces
- Neural Interfaces
- Mental Health
- Mental Health Research
- Sleep Disorder Research
- Human Biology
- Healthy Aging
- Chronic Illness
- Consumer Behavior
- Racial Issues
- Computer Programming
- Diet and Weight Loss
- Eating Disorder Research
Health & Medicine
- Link Between Poor Diet and Higher Cancer Risk
- PFAS Exposure from High Seafood Diets
- Melanomas Resist Drugs by 'Breaking' Genes
- Cells Putting On a Face
Mind & Brain
- Two Key Brain Systems Are Central to Psychosis
- AI Can Help People Feel Heard
- Detecting Stress Levels During Sleep
- Degenerative Disease Prevention and Telomeres
Living Well
- Childhood Maltreatment Casts a Long Shadow
- Goals, Willpower and Trustworthiness
- Can the Bias in Algorithms Help Us See Our Own?
- Size of Salty Snack Influences Amount Eaten
Physical/Tech News
Latest physical/tech headlines.
- CRISPR Gene Editing
- Biochemistry Research
- Materials Science
- Electronics
- Spintronics Research
- Sports Science
- Nanotechnology
- Thermodynamics
- Asteroids, Comets and Meteors
- Consumerism
- Resource Shortage
- Medical Imaging
- Medical Devices
- Computer Modeling
- Mathematical Modeling
- Mathematics
- Spintronics
Matter & Energy
- Antiviral Defense With New CRISPR Tool
- 'Surprising' Activity of Semiconductor Material
- Trapped in the Middle: Billiards With Memory
- Better Radiation Detector
Space & Time
- Exoplanets True to Size
- Twinkle Twinkle Baby Star
- The Milky Way in Ancient Egyptian Mythology
- Assessing Liveability of Other Planets
Computers & Math
- Forest Products Are Being Overlooked
- AI Makes Retinal Imaging 100 Times Faster
- How to Prevent Toxic Answers from AI Chatbots
- When Light Makes Materials Magnetic
Environment News
Latest environment headlines.
- Dolphins and Whales
- Ocean Policy
- Marine Biology
- Behavioral Science
- Evolutionary Biology
- Developmental Biology
- Food and Agriculture
- Agriculture and Food
- Energy and the Environment
- Renewable Energy
- Earthquakes
- Energy and Resources
- Coral Reefs
- Genetically Modified
- K-12 Education
- STEM Education
- Archaeology
- Anthropology
Plants & Animals
- Where Have All the Right Whales Gone?
- Bonobos Less Peaceful Than Previously Thought
- How Seaweed Became Multicellular
- Microbial Food to Help Feed the World
Earth & Climate
- Africa's Iconic Flamingos Threatened
- Geothermal Energy, Rock and Microquakes
- Corrosion and Clean Energy Future
- Tropical Coral-Infecting Parasites Head North
Fossils & Ruins
- Geobiology: New Placozoan Habitat Discovered
- 3D Mouth of an Ancient Jawless Fish
- Evolving Attitudes of Gen X Toward Evolution
- Early Medieval Money Mystery Solved
Society/Education News
Latest society/education headlines.
- Land Management
- Children's Health
- Health Policy
- Infant's Health
- Child Development
- Infant and Preschool Learning
- Language Acquisition
- Child Psychology
- Sustainability
- Environmental Awareness
- Environmental Policies
- Intelligence
- Social Psychology
- Relationships
- Energy Issues
- Educational Psychology
- Gender Difference
Science & Society
- Reconciling Biodiversity and Agriculture
- Food Security in Developed Countries
- Mixed Diets Balance Nutrition and Carbon
- Parental Leave Policy and Premature Babies
Education & Learning
- Talk to Your Baby: It Matters
- Exercise Habits in Youth, Good Health Later
- Universities Connected to Local Sustainability?
- Early Detection of Language Disorders Key
Business & Industry
- Active Workstations and Cognitive Performance
- Suppressing Boredom at Work Hurts Productivity
- Pairing Crypto Mining With Green Hydrogen
- Feeling Apathetic? There May Be Hope
Trending Topics
Strange & offbeat, about this site.
ScienceDaily features breaking news about the latest discoveries in science, health, the environment, technology, and more -- from leading universities, scientific journals, and research organizations.
Visitors can browse more than 500 individual topics, grouped into 12 main sections (listed under the top navigational menu), covering: the medical sciences and health; physical sciences and technology; biological sciences and the environment; and social sciences, business and education. Headlines and summaries of relevant news stories are provided on each topic page.
Stories are posted daily, selected from press materials provided by hundreds of sources from around the world. Links to sources and relevant journal citations (where available) are included at the end of each post.
For more information about ScienceDaily, please consult the links listed at the bottom of each page.
- USC Libraries
- Research Guides
Organizing Your Social Sciences Research Paper
- Theoretical Framework
- Purpose of Guide
- Design Flaws to Avoid
- Independent and Dependent Variables
- Glossary of Research Terms
- Reading Research Effectively
- Narrowing a Topic Idea
- Broadening a Topic Idea
- Extending the Timeliness of a Topic Idea
- Academic Writing Style
- Applying Critical Thinking
- Choosing a Title
- Making an Outline
- Paragraph Development
- Research Process Video Series
- Executive Summary
- The C.A.R.S. Model
- Background Information
- The Research Problem/Question
- Citation Tracking
- Content Alert Services
- Evaluating Sources
- Primary Sources
- Secondary Sources
- Tiertiary Sources
- Scholarly vs. Popular Publications
- Qualitative Methods
- Quantitative Methods
- Insiderness
- Using Non-Textual Elements
- Limitations of the Study
- Common Grammar Mistakes
- Writing Concisely
- Avoiding Plagiarism
- Footnotes or Endnotes?
- Further Readings
- Generative AI and Writing
- USC Libraries Tutorials and Other Guides
- Bibliography
Theories are formulated to explain, predict, and understand phenomena and, in many cases, to challenge and extend existing knowledge within the limits of critical bounded assumptions or predictions of behavior. The theoretical framework is the structure that can hold or support a theory of a research study. The theoretical framework encompasses not just the theory, but the narrative explanation about how the researcher engages in using the theory and its underlying assumptions to investigate the research problem. It is the structure of your paper that summarizes concepts, ideas, and theories derived from prior research studies and which was synthesized in order to form a conceptual basis for your analysis and interpretation of meaning found within your research.
Abend, Gabriel. "The Meaning of Theory." Sociological Theory 26 (June 2008): 173–199; Kivunja, Charles. "Distinguishing between Theory, Theoretical Framework, and Conceptual Framework: A Systematic Review of Lessons from the Field." International Journal of Higher Education 7 (December 2018): 44-53; Swanson, Richard A. Theory Building in Applied Disciplines . San Francisco, CA: Berrett-Koehler Publishers 2013; Varpio, Lara, Elise Paradis, Sebastian Uijtdehaage, and Meredith Young. "The Distinctions between Theory, Theoretical Framework, and Conceptual Framework." Academic Medicine 95 (July 2020): 989-994.
Importance of Theory and a Theoretical Framework
Theories can be unfamiliar to the beginning researcher because they are rarely applied in high school social studies curriculum and, as a result, can come across as unfamiliar and imprecise when first introduced as part of a writing assignment. However, in their most simplified form, a theory is simply a set of assumptions or predictions about something you think will happen based on existing evidence and that can be tested to see if those outcomes turn out to be true. Of course, it is slightly more deliberate than that, therefore, summarized from Kivunja (2018, p. 46), here are the essential characteristics of a theory.
- It is logical and coherent
- It has clear definitions of terms or variables, and has boundary conditions [i.e., it is not an open-ended statement]
- It has a domain where it applies
- It has clearly described relationships among variables
- It describes, explains, and makes specific predictions
- It comprises of concepts, themes, principles, and constructs
- It must have been based on empirical data [i.e., it is not a guess]
- It must have made claims that are subject to testing, been tested and verified
- It must be clear and concise
- Its assertions or predictions must be different and better than those in existing theories
- Its predictions must be general enough to be applicable to and understood within multiple contexts
- Its assertions or predictions are relevant, and if applied as predicted, will result in the predicted outcome
- The assertions and predictions are not immutable, but subject to revision and improvement as researchers use the theory to make sense of phenomena
- Its concepts and principles explain what is going on and why
- Its concepts and principles are substantive enough to enable us to predict a future
Given these characteristics, a theory can best be understood as the foundation from which you investigate assumptions or predictions derived from previous studies about the research problem, but in a way that leads to new knowledge and understanding as well as, in some cases, discovering how to improve the relevance of the theory itself or to argue that the theory is outdated and a new theory needs to be formulated based on new evidence.
A theoretical framework consists of concepts and, together with their definitions and reference to relevant scholarly literature, existing theory that is used for your particular study. The theoretical framework must demonstrate an understanding of theories and concepts that are relevant to the topic of your research paper and that relate to the broader areas of knowledge being considered.
The theoretical framework is most often not something readily found within the literature . You must review course readings and pertinent research studies for theories and analytic models that are relevant to the research problem you are investigating. The selection of a theory should depend on its appropriateness, ease of application, and explanatory power.
The theoretical framework strengthens the study in the following ways :
- An explicit statement of theoretical assumptions permits the reader to evaluate them critically.
- The theoretical framework connects the researcher to existing knowledge. Guided by a relevant theory, you are given a basis for your hypotheses and choice of research methods.
- Articulating the theoretical assumptions of a research study forces you to address questions of why and how. It permits you to intellectually transition from simply describing a phenomenon you have observed to generalizing about various aspects of that phenomenon.
- Having a theory helps you identify the limits to those generalizations. A theoretical framework specifies which key variables influence a phenomenon of interest and highlights the need to examine how those key variables might differ and under what circumstances.
- The theoretical framework adds context around the theory itself based on how scholars had previously tested the theory in relation their overall research design [i.e., purpose of the study, methods of collecting data or information, methods of analysis, the time frame in which information is collected, study setting, and the methodological strategy used to conduct the research].
By virtue of its applicative nature, good theory in the social sciences is of value precisely because it fulfills one primary purpose: to explain the meaning, nature, and challenges associated with a phenomenon, often experienced but unexplained in the world in which we live, so that we may use that knowledge and understanding to act in more informed and effective ways.
The Conceptual Framework. College of Education. Alabama State University; Corvellec, Hervé, ed. What is Theory?: Answers from the Social and Cultural Sciences . Stockholm: Copenhagen Business School Press, 2013; Asher, Herbert B. Theory-Building and Data Analysis in the Social Sciences . Knoxville, TN: University of Tennessee Press, 1984; Drafting an Argument. Writing@CSU. Colorado State University; Kivunja, Charles. "Distinguishing between Theory, Theoretical Framework, and Conceptual Framework: A Systematic Review of Lessons from the Field." International Journal of Higher Education 7 (2018): 44-53; Omodan, Bunmi Isaiah. "A Model for Selecting Theoretical Framework through Epistemology of Research Paradigms." African Journal of Inter/Multidisciplinary Studies 4 (2022): 275-285; Ravitch, Sharon M. and Matthew Riggan. Reason and Rigor: How Conceptual Frameworks Guide Research . Second edition. Los Angeles, CA: SAGE, 2017; Trochim, William M.K. Philosophy of Research. Research Methods Knowledge Base. 2006; Jarvis, Peter. The Practitioner-Researcher. Developing Theory from Practice . San Francisco, CA: Jossey-Bass, 1999.
Strategies for Developing the Theoretical Framework
I. Developing the Framework
Here are some strategies to develop of an effective theoretical framework:
- Examine your thesis title and research problem . The research problem anchors your entire study and forms the basis from which you construct your theoretical framework.
- Brainstorm about what you consider to be the key variables in your research . Answer the question, "What factors contribute to the presumed effect?"
- Review related literature to find how scholars have addressed your research problem. Identify the assumptions from which the author(s) addressed the problem.
- List the constructs and variables that might be relevant to your study. Group these variables into independent and dependent categories.
- Review key social science theories that are introduced to you in your course readings and choose the theory that can best explain the relationships between the key variables in your study [note the Writing Tip on this page].
- Discuss the assumptions or propositions of this theory and point out their relevance to your research.
A theoretical framework is used to limit the scope of the relevant data by focusing on specific variables and defining the specific viewpoint [framework] that the researcher will take in analyzing and interpreting the data to be gathered. It also facilitates the understanding of concepts and variables according to given definitions and builds new knowledge by validating or challenging theoretical assumptions.
II. Purpose
Think of theories as the conceptual basis for understanding, analyzing, and designing ways to investigate relationships within social systems. To that end, the following roles served by a theory can help guide the development of your framework.
- Means by which new research data can be interpreted and coded for future use,
- Response to new problems that have no previously identified solutions strategy,
- Means for identifying and defining research problems,
- Means for prescribing or evaluating solutions to research problems,
- Ways of discerning certain facts among the accumulated knowledge that are important and which facts are not,
- Means of giving old data new interpretations and new meaning,
- Means by which to identify important new issues and prescribe the most critical research questions that need to be answered to maximize understanding of the issue,
- Means of providing members of a professional discipline with a common language and a frame of reference for defining the boundaries of their profession, and
- Means to guide and inform research so that it can, in turn, guide research efforts and improve professional practice.
Adapted from: Torraco, R. J. “Theory-Building Research Methods.” In Swanson R. A. and E. F. Holton III , editors. Human Resource Development Handbook: Linking Research and Practice . (San Francisco, CA: Berrett-Koehler, 1997): pp. 114-137; Jacard, James and Jacob Jacoby. Theory Construction and Model-Building Skills: A Practical Guide for Social Scientists . New York: Guilford, 2010; Ravitch, Sharon M. and Matthew Riggan. Reason and Rigor: How Conceptual Frameworks Guide Research . Second edition. Los Angeles, CA: SAGE, 2017; Sutton, Robert I. and Barry M. Staw. “What Theory is Not.” Administrative Science Quarterly 40 (September 1995): 371-384.
Structure and Writing Style
The theoretical framework may be rooted in a specific theory , in which case, your work is expected to test the validity of that existing theory in relation to specific events, issues, or phenomena. Many social science research papers fit into this rubric. For example, Peripheral Realism Theory, which categorizes perceived differences among nation-states as those that give orders, those that obey, and those that rebel, could be used as a means for understanding conflicted relationships among countries in Africa. A test of this theory could be the following: Does Peripheral Realism Theory help explain intra-state actions, such as, the disputed split between southern and northern Sudan that led to the creation of two nations?
However, you may not always be asked by your professor to test a specific theory in your paper, but to develop your own framework from which your analysis of the research problem is derived . Based upon the above example, it is perhaps easiest to understand the nature and function of a theoretical framework if it is viewed as an answer to two basic questions:
- What is the research problem/question? [e.g., "How should the individual and the state relate during periods of conflict?"]
- Why is your approach a feasible solution? [i.e., justify the application of your choice of a particular theory and explain why alternative constructs were rejected. I could choose instead to test Instrumentalist or Circumstantialists models developed among ethnic conflict theorists that rely upon socio-economic-political factors to explain individual-state relations and to apply this theoretical model to periods of war between nations].
The answers to these questions come from a thorough review of the literature and your course readings [summarized and analyzed in the next section of your paper] and the gaps in the research that emerge from the review process. With this in mind, a complete theoretical framework will likely not emerge until after you have completed a thorough review of the literature .
Just as a research problem in your paper requires contextualization and background information, a theory requires a framework for understanding its application to the topic being investigated. When writing and revising this part of your research paper, keep in mind the following:
- Clearly describe the framework, concepts, models, or specific theories that underpin your study . This includes noting who the key theorists are in the field who have conducted research on the problem you are investigating and, when necessary, the historical context that supports the formulation of that theory. This latter element is particularly important if the theory is relatively unknown or it is borrowed from another discipline.
- Position your theoretical framework within a broader context of related frameworks, concepts, models, or theories . As noted in the example above, there will likely be several concepts, theories, or models that can be used to help develop a framework for understanding the research problem. Therefore, note why the theory you've chosen is the appropriate one.
- The present tense is used when writing about theory. Although the past tense can be used to describe the history of a theory or the role of key theorists, the construction of your theoretical framework is happening now.
- You should make your theoretical assumptions as explicit as possible . Later, your discussion of methodology should be linked back to this theoretical framework.
- Don’t just take what the theory says as a given! Reality is never accurately represented in such a simplistic way; if you imply that it can be, you fundamentally distort a reader's ability to understand the findings that emerge. Given this, always note the limitations of the theoretical framework you've chosen [i.e., what parts of the research problem require further investigation because the theory inadequately explains a certain phenomena].
The Conceptual Framework. College of Education. Alabama State University; Conceptual Framework: What Do You Think is Going On? College of Engineering. University of Michigan; Drafting an Argument. Writing@CSU. Colorado State University; Lynham, Susan A. “The General Method of Theory-Building Research in Applied Disciplines.” Advances in Developing Human Resources 4 (August 2002): 221-241; Tavallaei, Mehdi and Mansor Abu Talib. "A General Perspective on the Role of Theory in Qualitative Research." Journal of International Social Research 3 (Spring 2010); Ravitch, Sharon M. and Matthew Riggan. Reason and Rigor: How Conceptual Frameworks Guide Research . Second edition. Los Angeles, CA: SAGE, 2017; Reyes, Victoria. Demystifying the Journal Article. Inside Higher Education; Trochim, William M.K. Philosophy of Research. Research Methods Knowledge Base. 2006; Weick, Karl E. “The Work of Theorizing.” In Theorizing in Social Science: The Context of Discovery . Richard Swedberg, editor. (Stanford, CA: Stanford University Press, 2014), pp. 177-194.
Writing Tip
Borrowing Theoretical Constructs from Other Disciplines
An increasingly important trend in the social and behavioral sciences is to think about and attempt to understand research problems from an interdisciplinary perspective. One way to do this is to not rely exclusively on the theories developed within your particular discipline, but to think about how an issue might be informed by theories developed in other disciplines. For example, if you are a political science student studying the rhetorical strategies used by female incumbents in state legislature campaigns, theories about the use of language could be derived, not only from political science, but linguistics, communication studies, philosophy, psychology, and, in this particular case, feminist studies. Building theoretical frameworks based on the postulates and hypotheses developed in other disciplinary contexts can be both enlightening and an effective way to be more engaged in the research topic.
CohenMiller, A. S. and P. Elizabeth Pate. "A Model for Developing Interdisciplinary Research Theoretical Frameworks." The Qualitative Researcher 24 (2019): 1211-1226; Frodeman, Robert. The Oxford Handbook of Interdisciplinarity . New York: Oxford University Press, 2010.
Another Writing Tip
Don't Undertheorize!
Do not leave the theory hanging out there in the introduction never to be mentioned again. Undertheorizing weakens your paper. The theoretical framework you describe should guide your study throughout the paper. Be sure to always connect theory to the review of pertinent literature and to explain in the discussion part of your paper how the theoretical framework you chose supports analysis of the research problem or, if appropriate, how the theoretical framework was found to be inadequate in explaining the phenomenon you were investigating. In that case, don't be afraid to propose your own theory based on your findings.
Yet Another Writing Tip
What's a Theory? What's a Hypothesis?
The terms theory and hypothesis are often used interchangeably in newspapers and popular magazines and in non-academic settings. However, the difference between theory and hypothesis in scholarly research is important, particularly when using an experimental design. A theory is a well-established principle that has been developed to explain some aspect of the natural world. Theories arise from repeated observation and testing and incorporates facts, laws, predictions, and tested assumptions that are widely accepted [e.g., rational choice theory; grounded theory; critical race theory].
A hypothesis is a specific, testable prediction about what you expect to happen in your study. For example, an experiment designed to look at the relationship between study habits and test anxiety might have a hypothesis that states, "We predict that students with better study habits will suffer less test anxiety." Unless your study is exploratory in nature, your hypothesis should always explain what you expect to happen during the course of your research.
The key distinctions are:
- A theory predicts events in a broad, general context; a hypothesis makes a specific prediction about a specified set of circumstances.
- A theory has been extensively tested and is generally accepted among a set of scholars; a hypothesis is a speculative guess that has yet to be tested.
Cherry, Kendra. Introduction to Research Methods: Theory and Hypothesis. About.com Psychology; Gezae, Michael et al. Welcome Presentation on Hypothesis. Slideshare presentation.
Still Yet Another Writing Tip
Be Prepared to Challenge the Validity of an Existing Theory
Theories are meant to be tested and their underlying assumptions challenged; they are not rigid or intransigent, but are meant to set forth general principles for explaining phenomena or predicting outcomes. Given this, testing theoretical assumptions is an important way that knowledge in any discipline develops and grows. If you're asked to apply an existing theory to a research problem, the analysis will likely include the expectation by your professor that you should offer modifications to the theory based on your research findings.
Indications that theoretical assumptions may need to be modified can include the following:
- Your findings suggest that the theory does not explain or account for current conditions or circumstances or the passage of time,
- The study reveals a finding that is incompatible with what the theory attempts to explain or predict, or
- Your analysis reveals that the theory overly generalizes behaviors or actions without taking into consideration specific factors revealed from your analysis [e.g., factors related to culture, nationality, history, gender, ethnicity, age, geographic location, legal norms or customs , religion, social class, socioeconomic status, etc.].
Philipsen, Kristian. "Theory Building: Using Abductive Search Strategies." In Collaborative Research Design: Working with Business for Meaningful Findings . Per Vagn Freytag and Louise Young, editors. (Singapore: Springer Nature, 2018), pp. 45-71; Shepherd, Dean A. and Roy Suddaby. "Theory Building: A Review and Integration." Journal of Management 43 (2017): 59-86.
- << Previous: The Research Problem/Question
- Next: 5. The Literature Review >>
- Last Updated: Apr 11, 2024 1:27 PM
- URL: https://libguides.usc.edu/writingguide
- Share full article
Advertisement
Supported by
What Researchers Discovered When They Sent 80,000 Fake Résumés to U.S. Jobs
Some companies discriminated against Black applicants much more than others, and H.R. practices made a big difference.

By Claire Cain Miller and Josh Katz
A group of economists recently performed an experiment on around 100 of the largest companies in the country, applying for jobs using made-up résumés with equivalent qualifications but different personal characteristics. They changed applicants’ names to suggest that they were white or Black, and male or female — Latisha or Amy, Lamar or Adam.
On Monday, they released the names of the companies . On average, they found, employers contacted the presumed white applicants 9.5 percent more often than the presumed Black applicants.
Yet this practice varied significantly by firm and industry. One-fifth of the companies — many of them retailers or car dealers — were responsible for nearly half of the gap in callbacks to white and Black applicants.
Two companies favored white applicants over Black applicants significantly more than others. They were AutoNation, a used car retailer, which contacted presumed white applicants 43 percent more often, and Genuine Parts Company, which sells auto parts including under the NAPA brand, and called presumed white candidates 33 percent more often.
In a statement, Heather Ross, a spokeswoman for Genuine Parts, said, “We are always evaluating our practices to ensure inclusivity and break down barriers, and we will continue to do so.” AutoNation did not respond to a request for comment.
Companies With the Largest and Smallest Racial Contact Gaps
Of the 97 companies in the experiment, two stood out as contacting presumed white job applicants significantly more often than presumed Black ones. At 14 companies, there was little or no difference in how often they called back the presumed white or Black applicants.
Source: Patrick Kline, Evan K. Rose and Christopher R. Walters
Known as an audit study , the experiment was the largest of its kind in the United States: The researchers sent 80,000 résumés to 10,000 jobs from 2019 to 2021. The results demonstrate how entrenched employment discrimination is in parts of the U.S. labor market — and the extent to which Black workers start behind in certain industries.
“I am not in the least bit surprised,” said Daiquiri Steele, an assistant professor at the University of Alabama School of Law who previously worked for the Department of Labor on employment discrimination. “If you’re having trouble breaking in, the biggest issue is the ripple effect it has. It affects your wages and the economy of your community going forward.”
Some companies showed no difference in how they treated applications from people assumed to be white or Black. Their human resources practices — and one policy in particular (more on that later) — offer guidance for how companies can avoid biased decisions in the hiring process.
A lack of racial bias was more common in certain industries: food stores, including Kroger; food products, including Mondelez; freight and transport, including FedEx and Ryder; and wholesale, including Sysco and McLane Company.
“We want to bring people’s attention not only to the fact that racism is real, sexism is real, some are discriminating, but also that it’s possible to do better, and there’s something to be learned from those that have been doing a good job,” said Patrick Kline, an economist at the University of California, Berkeley, who conducted the study with Evan K. Rose at the University of Chicago and Christopher R. Walters at Berkeley.
The researchers first published details of their experiment in 2021, but without naming the companies. The new paper, which is set to run in the American Economic Review, names the companies and explains the methodology developed to group them by their performance, while accounting for statistical noise.
Sample Résumés From the Experiment
Fictitious résumés sent to large U.S. companies revealed a preference, on average, for candidates whose names suggested that they were white.

To assign names, the researchers started with a prior list that had been assembled using Massachusetts birth certificates from 1974 to 1979. They then supplemented this list with names found in a database of speeding tickets issued in North Carolina between 2006 and 2018, classifying a name as “distinctive” if more than 90 percent of people with that name were of a particular race.
The study includes 97 firms. The jobs the researchers applied to were entry level, not requiring a college degree or substantial work experience. In addition to race and gender, the researchers tested other characteristics protected by law , like age and sexual orientation.
They sent up to 1,000 applications to each company, applying for as many as 125 jobs per company in locations nationwide, to try to uncover patterns in companies’ operations versus isolated instances. Then they tracked whether the employer contacted the applicant within 30 days.
A bias against Black names
Companies requiring lots of interaction with customers, like sales and retail, particularly in the auto sector, were most likely to show a preference for applicants presumed to be white. This was true even when applying for positions at those firms that didn’t involve customer interaction, suggesting that discriminatory practices were baked in to corporate culture or H.R. practices, the researchers said.
Still, there were exceptions — some of the companies exhibiting the least bias were retailers, like Lowe’s and Target.
The study may underestimate the rate of discrimination against Black applicants in the labor market as a whole because it tested large companies, which tend to discriminate less, said Lincoln Quillian, a sociologist at Northwestern who analyzes audit studies. It did not include names intended to represent Latino or Asian American applicants, but other research suggests that they are also contacted less than white applicants, though they face less discrimination than Black applicants.
The experiment ended in 2021, and some of the companies involved might have changed their practices since. Still, a review of all available audit studies found that discrimination against Black applicants had not changed in three decades. After the Black Lives Matter protests in 2020, such discrimination was found to have disappeared among certain employers, but the researchers behind that study said the effect was most likely short-lived.
Gender, age and L.G.B.T.Q. status
On average, companies did not treat male and female applicants differently. This aligns with other research showing that gender discrimination against women is rare in entry-level jobs, and starts later in careers.
However, when companies did favor men (especially in manufacturing) or women (mostly at apparel stores), the biases were much larger than for race. Builders FirstSource contacted presumed male applicants more than twice as often as female ones. Ascena, which owns brands like Ann Taylor, contacted women 66 percent more than men.
Neither company responded to requests for comment.
The consequences of being female differed by race. The differences were small, but being female was a slight benefit for white applicants, and a slight penalty for Black applicants.
The researchers also tested several other characteristics protected by law, with a smaller number of résumés. They found there was a small penalty for being over 40.
Overall, they found no penalty for using nonbinary pronouns. Being gay, as indicated by including membership in an L.G.B.T.Q. club on the résumé, resulted in a slight penalty for white applicants, but benefited Black applicants — although the effect was small, when this was on their résumés, the racial penalty disappeared.
Under the Civil Rights Act of 1964, discrimination is illegal even if it’s unintentional . Yet in the real world, it is difficult for job applicants to know why they did not hear back from a company.
“These practices are particularly challenging to address because applicants often do not know whether they are being discriminated against in the hiring process,” Brandalyn Bickner, a spokeswoman for the Equal Employment Opportunity Commission, said in a statement. (It has seen the data and spoken with the researchers, though it could not use an academic study as the basis for an investigation, she said.)
What companies can do to reduce discrimination
Several common measures — like employing a chief diversity officer, offering diversity training or having a diverse board — were not correlated with decreased discrimination in entry-level hiring, the researchers found.
But one thing strongly predicted less discrimination: a centralized H.R. operation.
The researchers recorded the voice mail messages that the fake applicants received. When a company’s calls came from fewer individual phone numbers, suggesting that they were originating from a central office, there tended to be less bias . When they came from individual hiring managers at local stores or warehouses, there was more. These messages often sounded frantic and informal, asking if an applicant could start the next day, for example.
“That’s when implicit biases kick in,” Professor Kline said. A more formalized hiring process helps overcome this, he said: “Just thinking about things, which steps to take, having to run something by someone for approval, can be quite important in mitigating bias.”
At Sysco, a wholesale restaurant food distributor, which showed no racial bias in the study, a centralized recruitment team reviews résumés and decides whom to call. “Consistency in how we review candidates, with a focus on the requirements of the position, is key,” said Ron Phillips, Sysco’s chief human resources officer. “It lessens the opportunity for personal viewpoints to rise in the process.”
Another important factor is diversity among the people hiring, said Paula Hubbard, the chief human resources officer at McLane Company. It procures, stores and delivers products for large chains like Walmart, and showed no racial bias in the study. Around 40 percent of the company’s recruiters are people of color, and 60 percent are women.
Diversifying the pool of people who apply also helps, H.R. officials said. McLane goes to events for women in trucking and puts up billboards in Spanish.
So does hiring based on skills, versus degrees . While McLane used to require a college degree for many roles, it changed that practice after determining that specific skills mattered more for warehousing or driving jobs. “We now do that for all our jobs: Is there truly a degree required?” Ms. Hubbard said. “Why? Does it make sense? Is experience enough?”
Hilton, another company that showed no racial bias in the study, also stopped requiring degrees for many jobs, in 2018.
Another factor associated with less bias in hiring, the new study found, was more regulatory scrutiny — like at federal contractors, or companies with more Labor Department citations.
Finally, more profitable companies were less biased, in line with a long-held economics theory by the Nobel Prize winner Gary Becker that discrimination is bad for business. Economists said that could be because the more profitable companies benefit from a more diverse set of employees. Or it could be an indication that they had more efficient business processes, in H.R. and elsewhere.
Claire Cain Miller writes about gender, families and the future of work for The Upshot. She joined The Times in 2008 and was part of a team that won a Pulitzer Prize in 2018 for public service for reporting on workplace sexual harassment issues. More about Claire Cain Miller
Josh Katz is a graphics editor for The Upshot, where he covers a range of topics involving politics, policy and culture. He is the author of “Speaking American: How Y’all, Youse, and You Guys Talk,” a visual exploration of American regional dialects. More about Josh Katz
From The Upshot: What the Data Says
Analysis that explains politics, policy and everyday life..
Employment Discrimination: Researchers sent 80,000 fake résumés to some of the largest companies in the United States. They found that some discriminated against Black applicants much more than others .
Pandemic School Closures: A variety of data about children’s academic outcomes and about the spread of Covid-19 has accumulated since the start of the pandemic. Here is what we learned from it .
Affirmative Action: The Supreme Court effectively ended race-based preferences in admissions. But will selective schools still be able to achieve diverse student bodies? Here is how they might try .
N.Y.C. Neighborhoods: We asked New Yorkers to map their neighborhoods and to tell us what they call them . The result, while imperfect, is an extremely detailed map of the city .
Dialect Quiz: What does the way you speak say about where you’re from? Answer these questions to find out .
- Português Br
- Journalist Pass
Study documents safety, improvements from stem cell therapy after spinal cord injury
Susan Barber Lindquist
Share this:
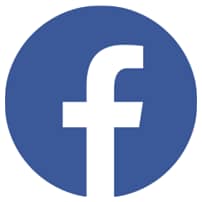
ROCHESTER, Minn. — A Mayo Clinic study shows stem cells derived from patients' own fat are safe and may improve sensation and movement after traumatic spinal cord injuries . The findings from the phase 1 clinical trial appear in Nature Communications . The results of this early research offer insights on the potential of cell therapy for people living with spinal cord injuries and paralysis for whom options to improve function are extremely limited.
In the study of 10 adults, the research team noted seven participants demonstrated improvements based on the American Spinal Injury Association (ASIA) Impairment Scale. Improvements included increased sensation when tested with pinprick and light touch, increased strength in muscle motor groups, and recovery of voluntary anal contraction, which aids in bowel function. The scale has five levels, ranging from complete loss of function to normal function. The seven participants who improved each moved up at least one level on the ASIA scale. Three patients in the study had no response, meaning they did not improve but did not get worse.
"This study documents the safety and potential benefit of stem cells and regenerative medicine," says Mohamad Bydon, M.D. , a Mayo Clinic neurosurgeon and first author of the study. "Spinal cord injury is a complex condition. Future research may show whether stem cells in combination with other therapies could be part of a new paradigm of treatment to improve outcomes for patients."
No serious adverse events were reported after stem cell treatment. The most commonly reported side effects were headache and musculoskeletal pain that resolved with over-the-counter treatment.
In addition to evaluating safety, this phase 1 clinical trial had a secondary outcome of assessing changes in motor and sensory function. The authors note that motor and sensory results are to be interpreted with caution given limits of phase 1 trials. Additional research is underway among a larger group of participants to further assess risks and benefits.
The full data on the 10 patients follows a 2019 case report that highlighted the experience of the first study participant who demonstrated significant improvement in motor and sensory function.
Watch: Dr. Mohamad Bydon discusses improvements in research study
Journalists: Broadcast-quality sound bites are available in the downloads at the end of the post. Please courtesy: "Mayo Clinic News Network." Name super/CG: Mohamad Bydon, M.D./Neurosurgery/Mayo Clinic.
Stem cells' mechanism of action not fully understood
In the multidisciplinary clinical trial, participants had spinal cord injuries from motor vehicle accidents, falls and other causes. Six had neck injuries; four had back injuries. Participants ranged in age from 18 to 65.
Participants' stem cells were collected by taking a small amount of fat from a 1- to 2-inch incision in the abdomen or thigh. Over four weeks, the cells were expanded in the laboratory to 100 million cells and then injected into the patients' lumbar spine in the lower back. Over two years, each study participant was evaluated at Mayo Clinic 10 times.
Although it is understood that stem cells move toward areas of inflammation — in this case the location of the spinal cord injury — the cells' mechanism of interacting with the spinal cord is not fully understood, Dr. Bydon says. As part of the study, researchers analyzed changes in participants' MRIs and cerebrospinal fluid as well as in responses to pain, pressure and other sensation. The investigators are looking for clues to identify injury processes at a cellular level and avenues for potential regeneration and healing.
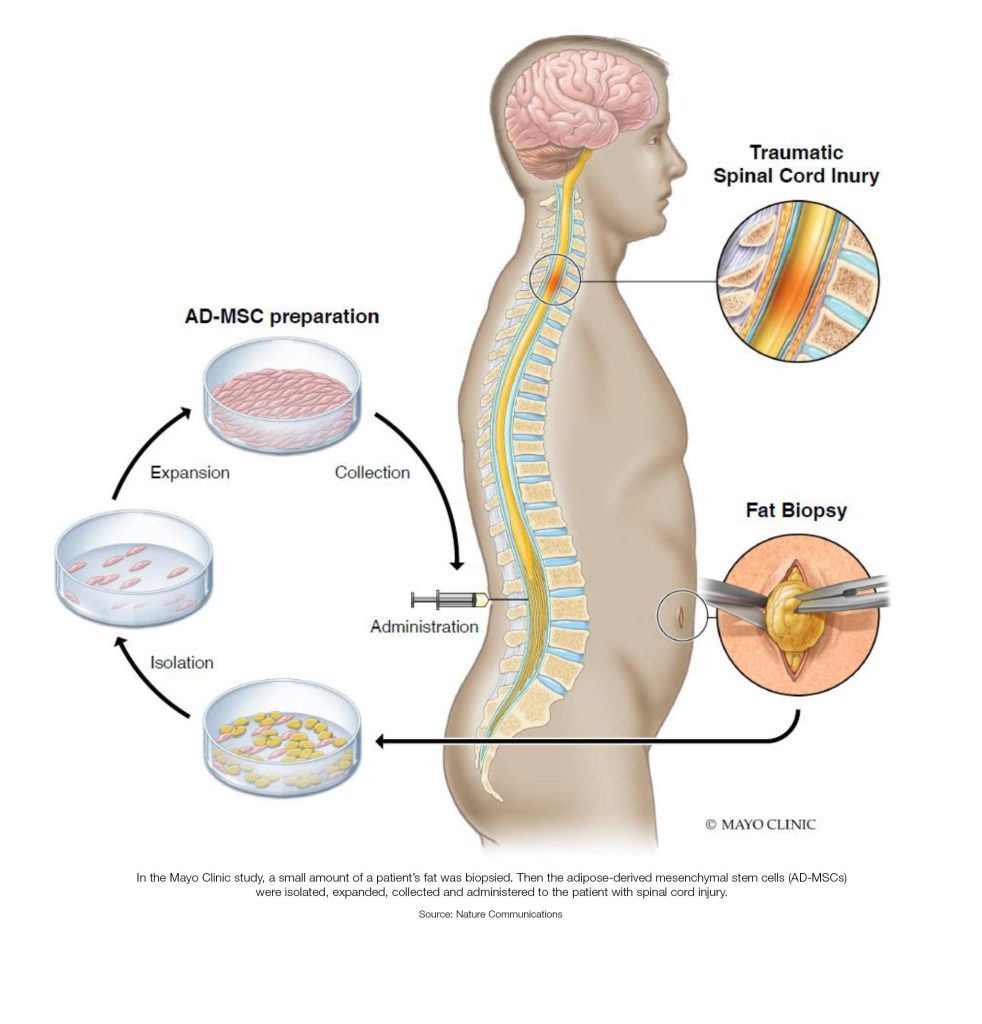
The spinal cord has limited ability to repair its cells or make new ones. Patients typically experience most of their recovery in the first six to 12 months after injuries occur. Improvement generally stops 12 to 24 months after injury. In the study, one patient with a cervical spine injury of the neck received stem cells 22 months after injury and improved one level on the ASIA scale after treatment.
Two of three patients with complete injuries of the thoracic spine — meaning they had no feeling or movement below their injury between the base of the neck and mid-back — moved up two ASIA levels after treatment. Each regained some sensation and some control of movement below the level of injury. Based on researchers' understanding of traumatic thoracic spinal cord injury, only 5% of people with a complete injury would be expected to regain any feeling or movement.
"In spinal cord injury, even a mild improvement can make a significant difference in that patient's quality of life," Dr. Bydon says.
Research continues into stem cells for spinal cord injuries
Stem cells are used mainly in research in the U.S., and fat-derived stem cell treatment for spinal cord injury is considered experimental by the Food and Drug Administration.
Between 250,000 and 500,000 people worldwide suffer a spinal cord injury each year, according to the World Health Organization .
An important next step is assessing the effectiveness of stem cell therapies and subsets of patients who would most benefit, Dr. Bydon says. Research is continuing with a larger, controlled trial that randomly assigns patients to receive either the stem cell treatment or a placebo without stem cells.
"For years, treatment of spinal cord injury has been limited to supportive care, more specifically stabilization surgery and physical therapy," Dr. Bydon says. "Many historical textbooks state that this condition does not improve. In recent years, we have seen findings from the medical and scientific community that challenge prior assumptions. This research is a step forward toward the ultimate goal of improving treatments for patients."
Dr. Bydon is the Charles B. and Ann L. Johnson Professor of Neurosurgery. This research was made possible with support from Leonard A. Lauder, C and A Johnson Family Foundation, The Park Foundation, Sanger Family Foundation, Eileen R.B. and Steve D. Scheel, Schultz Family Foundation, and other generous Mayo Clinic benefactors. The research is funded in part by a Mayo Clinic Transform the Practice grant.
Review the study for a complete list of authors and funding.
About Mayo Clinic Mayo Clinic is a nonprofit organization committed to innovation in clinical practice, education and research, and providing compassion, expertise and answers to everyone who needs healing. Visit the Mayo Clinic News Network for additional Mayo Clinic news.
Media contact:
- Susan Barber Lindquist, Mayo Clinic Communications, [email protected]
- Personal journey shapes unique perspective Mayo Clinic scientists pioneer immunotherapy technique for autoimmune diseases
Related Articles
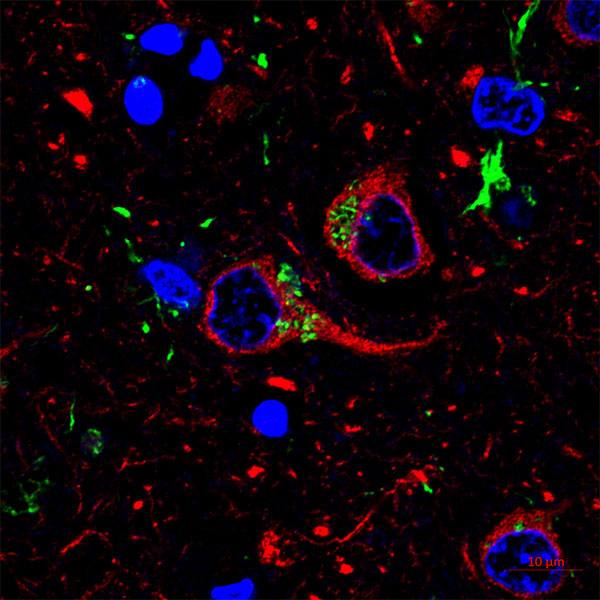
AACR Annual Meeting News: Read the latest session previews and recaps from the official news website.
Select "Patients / Caregivers / Public" or "Researchers / Professionals" to filter your results. To further refine your search, toggle appropriate sections on or off.
Home > About the AACR > Newsroom > News Release > Accelerated Aging May Increase the Risk of Early-onset Cancers in Younger Generations
- News Releases
- Media Advisories
- Media Contacts
- AACR Fast Facts
- Apply for Embargoed Access
- Media Coverage
- AACR Cancer Progress Report 2023
- AACR Annual Meeting 2023
- AACR Cancer Disparities Progress Report 2022
- AACR Report on COVID-19 and Cancer 2022
- The AACR June L. Biedler Prize for Cancer Journalism
- San Antonio Breast Cancer Symposium 2023
- Press and PIO Registration
- AACR Annual Meeting
- Embargo Policy
- Media Resources for the AACR Annual Meeting 2024
- Press Program 2024
- Peer-reviewed Journals
- National Cancer Research Month
- Resources for Cancer Center PIOs and Communications Staff
- AACR Stories Index
- AACR Blog: Cancer Research Catalyst
- Cancer Today Magazine
Accelerated Aging May Increase the Risk of Early-onset Cancers in Younger Generations
SAN DIEGO – Accelerated aging was more common in recent birth cohorts and was associated with increased incidence of early-onset solid tumors, according to research presented at the American Association for Cancer Research (AACR) Annual Meeting 2024 , held April 5-10.
“Multiple cancer types are becoming increasingly common among younger adults in the United States and globally,” said Ruiyi Tian, MPH , a graduate student in the lab of Yin Cao, ScD, MPH at Washington University School of Medicine in St. Louis. “Understanding the factors driving this increase will be key to improve the prevention or early detection of cancers in younger and future generations.”
Tian and colleagues hypothesized that increased biological age, indicative of accelerated aging, may contribute to the development of early-onset cancers, often defined as cancers diagnosed in adults younger than 55 years. In contrast to chronological age—which measures how long a person has been alive—biological age refers to the condition of a person’s body and physiological processes and is considered modifiable, Tian explained.
“Unlike chronological age, biological age may be influenced by factors such as diet, physical activity, mental health, and environmental stressors,” she added. “Accumulating evidence suggests that the younger generations may be aging more swiftly than anticipated, likely due to earlier exposure to various risk factors and environmental insults. However, the impact of accelerated aging on early-onset cancer development remains unclear.”
To examine the association between biological age and cancer risk in younger individuals, Tian and colleagues examined data of 148,724 individuals housed in the U.K. Biobank database. They calculated each participant’s biological age using nine biomarkers found in blood: albumin, alkaline phosphatase, creatinine, C-reactive protein, glucose, mean corpuscular volume, red cell distribution width, white blood cell count, and lymphocyte proportion. Individuals whose biological age was higher than their chronological age were defined as having accelerated aging.
Tian and colleagues first evaluated accelerated aging across birth cohorts and found that individuals born in or after 1965 had a 17% higher likelihood of accelerated aging than those born between 1950 and 1954. They then evaluated the association between accelerated aging and the risk of early-onset cancers. They found that each standard deviation increase in accelerated aging was associated with a 42% increased risk of early-onset lung cancer, a 22% increased risk of early-onset gastrointestinal cancer, and a 36% increased risk of early-onset uterine cancer. Accelerated aging did not significantly impact the risk of late-onset lung cancer (defined here as cancer diagnosed after age 55), but it was associated with a 16% and 23% increased risk of late-onset gastrointestinal and uterine cancers, respectively.
“By examining the relationship between accelerating aging and the risk of early-onset cancers, we provide a fresh perspective on the shared etiology of early-onset cancers,” Tian said. “If validated, our findings suggest that interventions to slow biological aging could be a new avenue for cancer prevention, and screening efforts tailored to younger individuals with signs of accelerated aging could help detect cancers early.”
Future research from Tian and colleagues will aim to uncover the mechanisms driving accelerated aging and early-onset cancers to develop precision cancer prevention strategies.
A limitation of the study is that all participants were from the United Kingdom, which may limit the generalizability of the findings to populations with different genetic backgrounds, lifestyles, and environmental exposures. Tian noted that validation in diverse populations is needed.
The study was supported by the National Institutes of Health. Tian declares no conflicts of interest.
- Healthcare Professionals Membership
- What Is Cancer Research
- Various Types of Cancer
No sign of greenhouse gases increases slowing in 2023
- April 5, 2024
Levels of the three most important human-caused greenhouse gases – carbon dioxide (CO 2 ), methane and nitrous oxide – continued their steady climb during 2023, according to NOAA scientists.
While the rise in the three heat-trapping gases recorded in the air samples collected by NOAA’s Global Monitoring Laboratory (GML) in 2023 was not quite as high as the record jumps observed in recent years, they were in line with the steep increases observed during the past decade.
“NOAA’s long-term air sampling program is essential for tracking causes of climate change and for supporting the U.S. efforts to establish an integrated national greenhouse gas measuring, monitoring and information system,” said GML Director Vanda Grubišić. “As these numbers show, we still have a lot of work to do to make meaningful progress in reducing the amount of greenhouse gases accumulating in the atmosphere.”
The global surface concentration of CO 2 , averaged across all 12 months of 2023, was 419.3 parts per million (ppm), an increase of 2.8 ppm during the year. This was the 12th consecutive year CO 2 increased by more than 2 ppm, extending the highest sustained rate of CO 2 increases during the 65-year monitoring record. Three consecutive years of CO 2 growth of 2 ppm or more had not been seen in NOAA’s monitoring records prior to 2014. Atmospheric CO 2 is now more than 50% higher than pre-industrial levels.
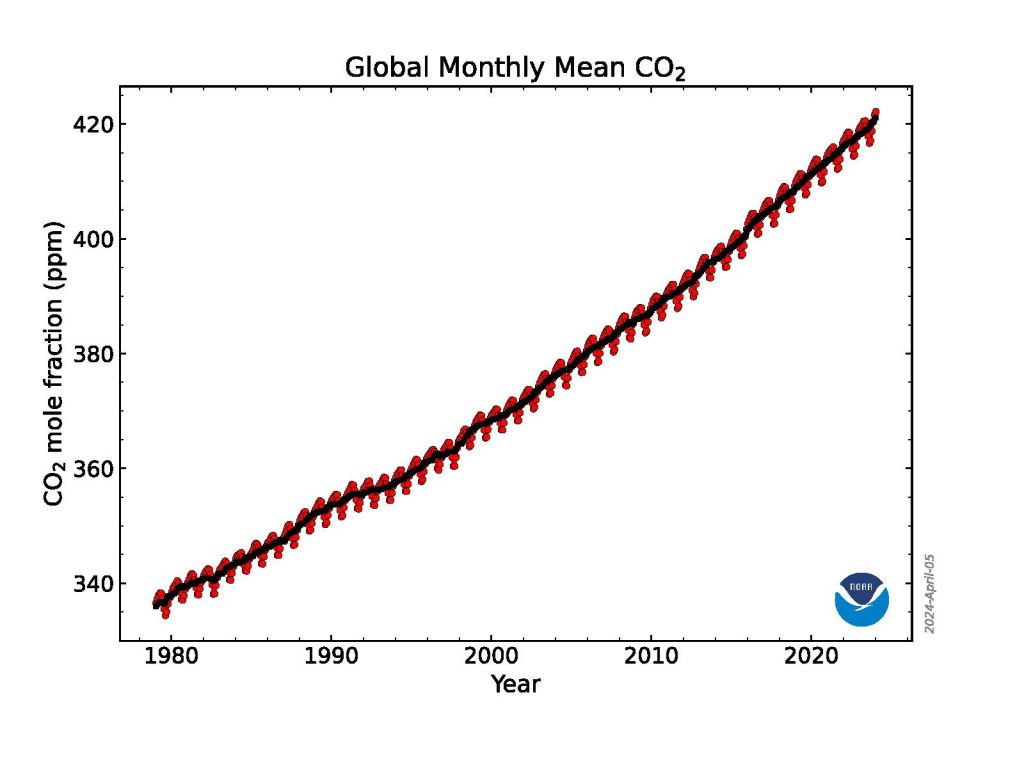
This graph shows the globally averaged monthly mean carbon dioxide abundance measured at the Global Monitoring Laboratory’s global network of air sampling sites since 1980. Data are still preliminary, pending recalibrations of reference gases and other quality control checks. Credit: NOAA GML
“The 2023 increase is the third-largest in the past decade, likely a result of an ongoing increase of fossil fuel CO 2 emissions, coupled with increased fire emissions possibly as a result of the transition from La Nina to El Nino,” said Xin Lan, a CIRES scientist who leads GML’s effort to synthesize data from the NOAA Global Greenhouse Gas Reference Network for tracking global greenhouse gas trends .
Atmospheric methane, less abundant than CO 2 but more potent at trapping heat in the atmosphere, rose to an average of 1922.6 parts per billion (ppb). The 2023 methane increase over 2022 was 10.9 ppb, lower than the record growth rates seen in 2020 (15.2 ppb), 2021(18 ppb) and 2022 (13.2 ppb), but still the 5th highest since renewed methane growth started in 2007. Methane levels in the atmosphere are now more than 160% higher than their pre-industrial level.
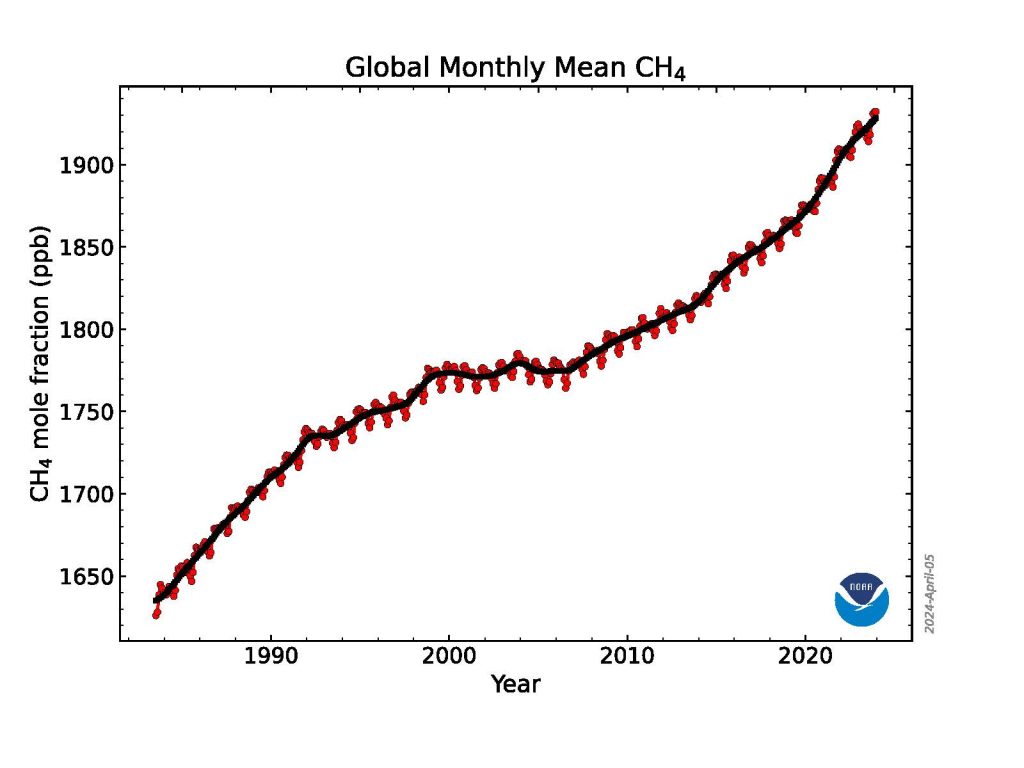
This graph shows globally-averaged, monthly mean atmospheric methane abundance determined from marine surface sites for the full NOAA time-series starting in 1983. Values for the last year are preliminary, pending recalibrations of standard gases and other quality control steps. Credit: NOAA GM
In 2023, levels of nitrous oxide, the third-most significant human-caused greenhouse gas, climbed by 1 ppb to 336.7 ppb. The two years of highest growth since 2000 occurred in 2020 (1.3 ppb) and 2021 (1.3 ppb). Increases in atmospheric nitrous oxide during recent decades are mainly from use of nitrogen fertilizer and manure from the expansion and intensification of agriculture. Nitrous oxide concentrations are 25% higher than the pre-industrial level of 270 ppb.
Taking the pulse of the planet one sample at a time NOAA’s Global Monitoring Laboratory collected more than 15,000 air samples from monitoring stations around the world in 2023 and analyzed them in its state-of-the-art laboratory in Boulder,
Colorado. Each spring, NOAA scientists release preliminary calculations of the global average levels of these three primary long-lived greenhouse gases observed during the previous year to track their abundance, determine emissions and sinks, and understand carbon cycle feedbacks.
Measurements are obtained from air samples collected from sites in NOAA’s Global Greenhouse Gas Reference Network , which includes about 53 cooperative sampling sites around the world, 20 tall tower sites, and routine aircraft operation sites from North America.
Carbon dioxide emissions remain the biggest problem
By far the most important contributor to climate change is CO 2 , which is primarily emitted by burning of fossil fuels. Human-caused CO 2 pollution increased from 10.9 billion tons per year in the 1960s – which is when the measurements at the Mauna Loa Observatory in Hawaii began – to about 36.8 billion tons per year in 2023. This sets a new record, according to the Global Carbon Project , which uses NOAA’s Global Greenhouse Gas Reference Network measurements to define the net impact of global carbon emissions and sinks.
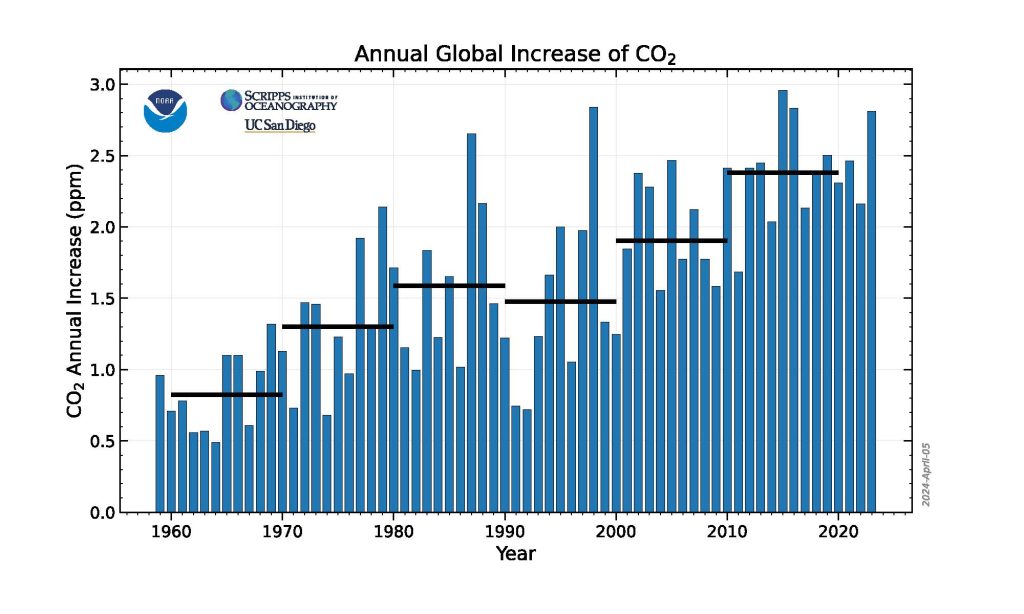
The amount of CO 2 in the atmosphere today is comparable to where it was around 4.3 million years ago during the mid- Pliocene epoch , when sea level was about 75 feet higher than today, the average temperature was 7 degrees Fahrenheit higher than in pre-industrial times, and large forests occupied areas of the Arctic that are now tundra.
About half of the CO 2 emissions from fossil fuels to date have been absorbed at the Earth’s surface, divided roughly equally between oceans and land ecosystems, including grasslands and forests. The CO 2 absorbed by the world’s oceans contributes to ocean acidification, which is causing a fundamental change in the chemistry of the ocean, with impacts to marine life and the people who depend on them. The oceans have also absorbed an estimated 90% of the excess heat trapped in the atmosphere by greenhouse gases.
Research continues to point to microbial sources for rising methane
NOAA’s measurements show that atmospheric methane increased rapidly during the 1980s, nearly stabilized in the late-1990s and early 2000s, then resumed a rapid rise in 2007.
A 2022 study by NOAA and NASA scientists and additional NOAA research in 2023 suggests that more than 85% of the increase from 2006 to 2021 was due to increased microbial emissions generated by livestock, agriculture, human and agricultural waste, wetlands and other aquatic sources. The rest of the increase was attributed to increased fossil fuel emissions.
“In addition to the record high methane growth in 2020-2022, we also observed sharp changes in the isotope composition of the methane that indicates an even more dominant role of microbial emission increase,” said Lan. The exact causes of the recent increase in methane are not yet fully known.
NOAA scientists are investigating the possibility that climate change is causing wetlands to give off increasing methane emissions in a feedback loop.
To learn more about the Global Monitoring Laboratory’s greenhouse gas monitoring, visit: https://gml.noaa.gov/ccgg/trends/.
Media Contact: Theo Stein, [email protected] , 303-819-7409
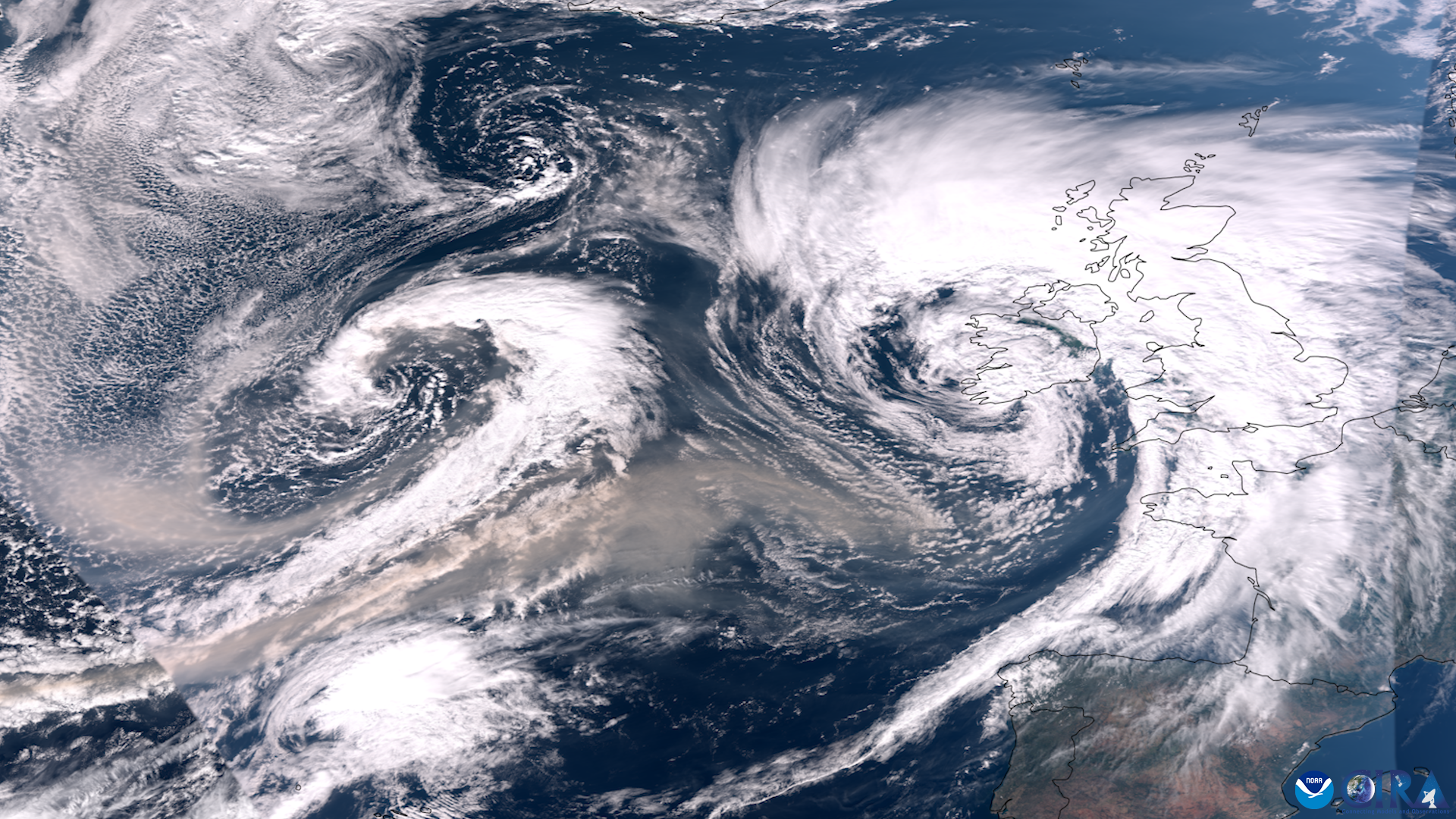

5 science wins from the 2023 NOAA Science Report
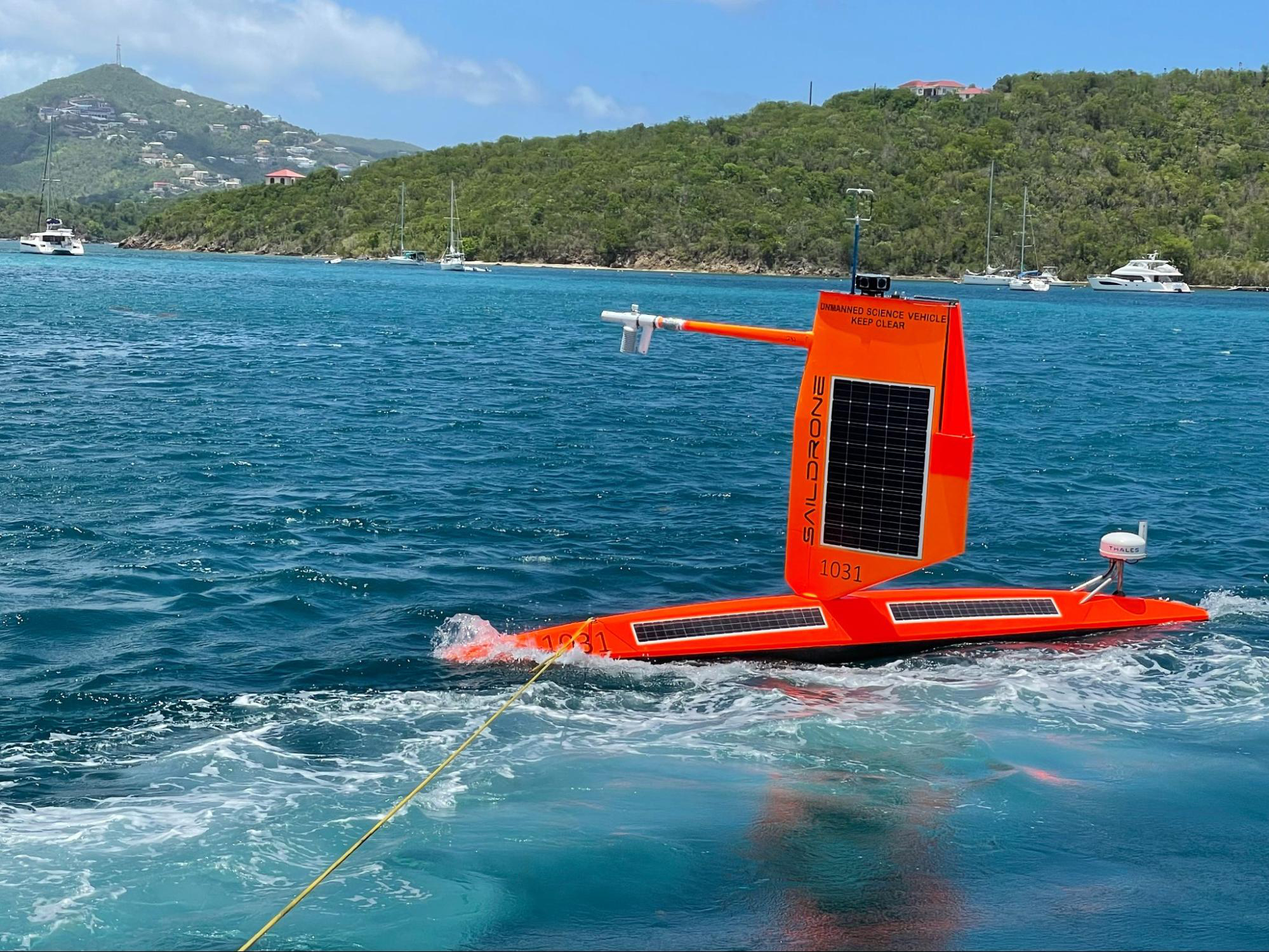
Filling A Data Gap In The Tropical Pacific To Reveal Daily Air-Sea Interactions
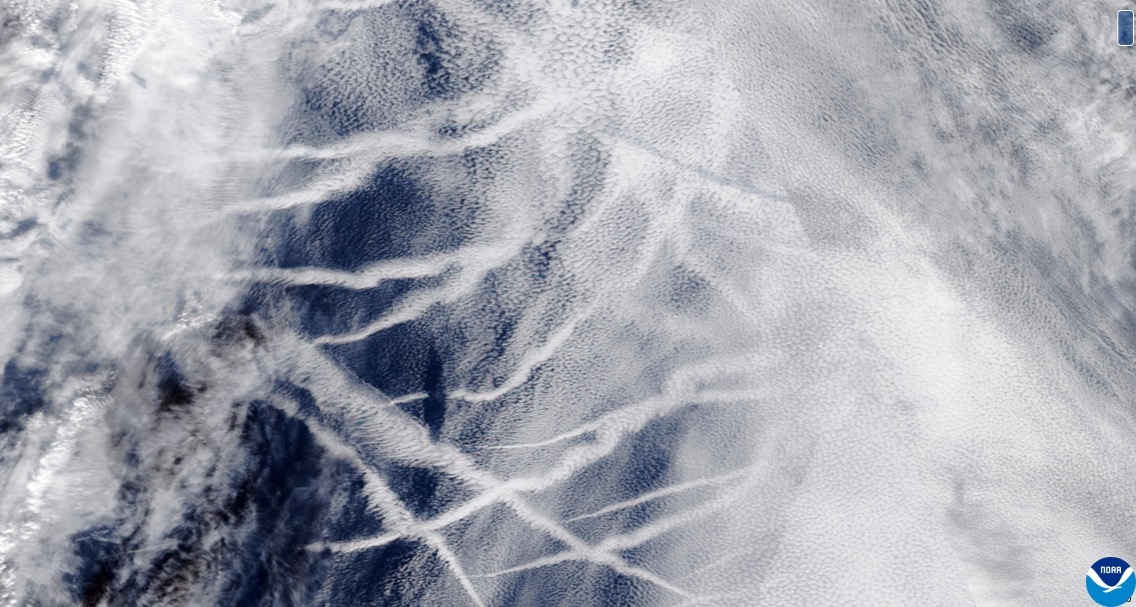
Scientists detail research to assess viability and risks of marine cloud brightening
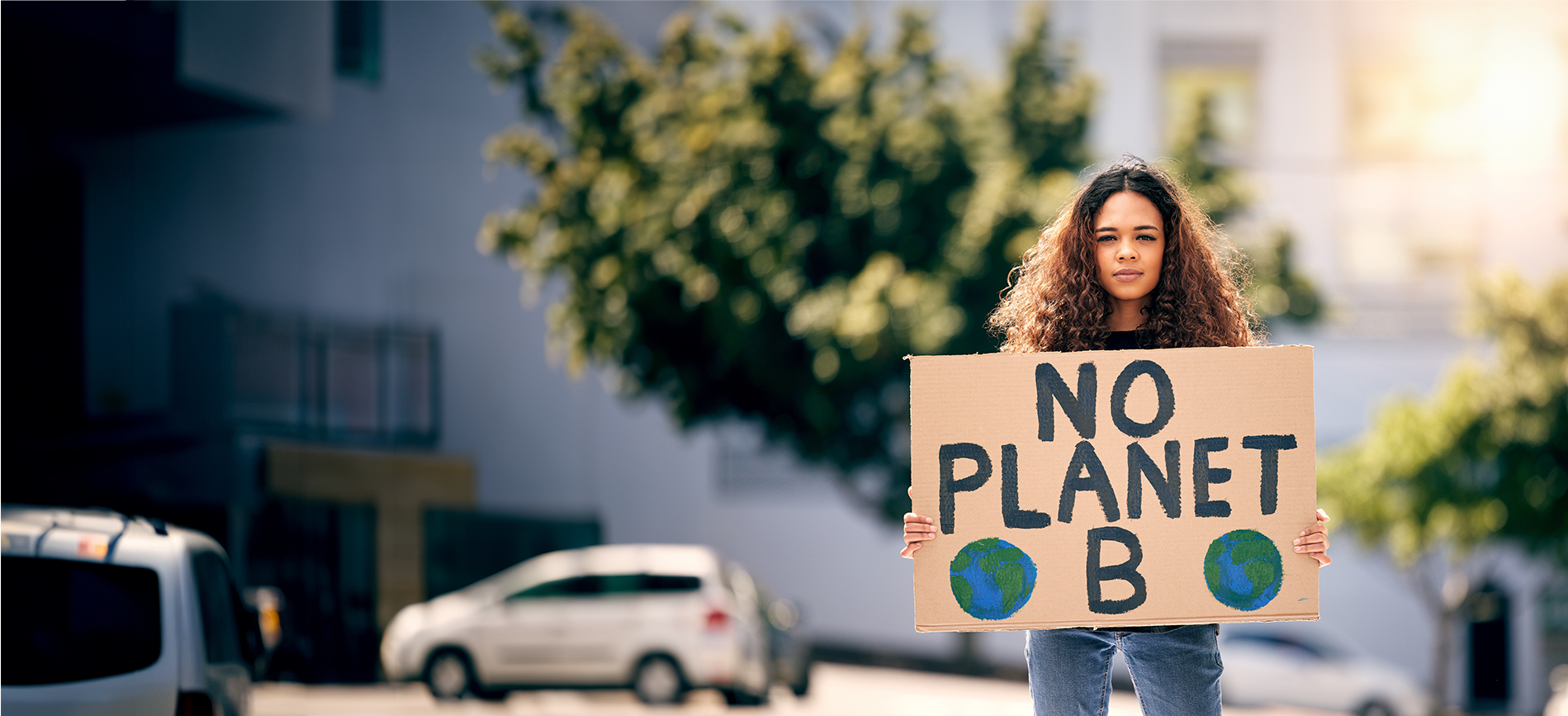
How social science helps us combat climate change
Popup call to action.
A prompt with more information on your call to action.
- U.S. Department of Health & Human Services

- Virtual Tour
- Staff Directory
- En Español
You are here
News releases.
Media Advisory
Thursday, April 11, 2024
Study reveals no causal link between neurodevelopmental disorders and acetaminophen exposure before birth
NIH-funded research in siblings finds previously reported connection is likely due to other underlying factors.
Acetaminophen exposure during pregnancy is not linked to the risk of developing autism, ADHD, or intellectual disability, according to a new study of data from more than 2 million children in Sweden. The collaborative research effort by Swedish and American investigators, which appears in JAMA , is the largest of its kind and was funded by the National Institute of Neurological Disorders and Stroke (NINDS), part of the National Institutes of Health (NIH).
Scientists compared siblings — who share genetics and other variables such as parental health, environmental exposures, and socioeconomic factors — and were able to limit the influence of other potential risk factors. This allowed them to focus specifically on, and eliminate, the risk associated with acetaminophen. The study design was unique due to the size of the population captured in the Swedish Medical Birth Register and the Swedish Prescribed Drug Register. Before siblings were considered, there appeared to be a small increase in risk for neurodevelopmental disorders in children exposed to acetaminophen, which was noted in previous studies.
Acetaminophen is commonly used as a pain reliever and fever reducer and is found in a variety of medicines available over the counter and via prescription. It is often taken during pregnancy instead of nonsteroidal anti-inflammatory drugs, known as NSAIDs, which can cause low levels of amniotic fluid, according to the Food and Drug Administration . The reasons pregnant people might take acetaminophen, including fever, or conditions such as chronic migraine, could be, and in some cases are, associated with an increased risk for later neurodevelopmental disorders following pregnancy.
One limitation of this study is that it relies on data from prescribed acetaminophen and from self-reporting from pregnant people during prenatal care. It may not capture all use or dosage in all people, particularly over-the-counter medicines. However, the number of patients included in the study sample and the ability to control for many other confounding factors support the conclusion that acetaminophen is not directly linked to an increase link of autism, ADHD, or intellectual disability.
To inform best preventative strategies, additional research is required to fully understand the genetic and non-genetic factors that increase the risk of autism, ADHD, and intellectual disability.
This study was supported by NINDS (NS107607).
Vicky Whittemore, Ph.D., program director, NINDS
Ahlqvist VH, et al. “Acetaminophen use during pregnancy and children’s risk of autism, ADHD, and intellectual disability.” JAMA. April 9, 2024. DOI: 10.1001/jama.2024.3172
NINDS is the nation’s leading funder of research on the brain and nervous system. The mission of NINDS is to seek fundamental knowledge about the brain and nervous system and to use that knowledge to reduce the burden of neurological disease.
About the National Institutes of Health (NIH): NIH, the nation's medical research agency, includes 27 Institutes and Centers and is a component of the U.S. Department of Health and Human Services. NIH is the primary federal agency conducting and supporting basic, clinical, and translational medical research, and is investigating the causes, treatments, and cures for both common and rare diseases. For more information about NIH and its programs, visit www.nih.gov .
NIH…Turning Discovery Into Health ®
Connect with Us
- More Social Media from NIH
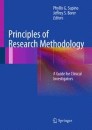
Principles of Research Methodology pp 1–14 Cite as
Overview of the Research Process
- Phyllis G. Supino EdD 3
- First Online: 01 January 2012
6256 Accesses
1 Citations
1 Altmetric
Research is a rigorous problem-solving process whose ultimate goal is the discovery of new knowledge. Research may include the description of a new phenomenon, definition of a new relationship, development of a new model, or application of an existing principle or procedure to a new context. Research is systematic, logical, empirical, reductive, replicable and transmittable, and generalizable. Research can be classified according to a variety of dimensions: basic, applied, or translational; hypothesis generating or hypothesis testing; retrospective or prospective; longitudinal or cross-sectional; observational or experimental; and quantitative or qualitative. The ultimate success of a research project is heavily dependent on adequate planning.
This is a preview of subscription content, log in via an institution .
Buying options
- Available as PDF
- Read on any device
- Instant download
- Own it forever
- Available as EPUB and PDF
- Compact, lightweight edition
- Dispatched in 3 to 5 business days
- Free shipping worldwide - see info
- Durable hardcover edition
Tax calculation will be finalised at checkout
Purchases are for personal use only
Calvert J, Martin BR (2001) Changing conceptions of basic research? Brighton, England: Background document for the Workshop on Policy Relevance and Measurement of Basic Research, Oslo, 29–30 Oct 2001. Brighton, England: SPRU.
Google Scholar
Leedy PD. Practical research. Planning and design. 6th ed. Upper Saddle River: Prentice Hall; 1997.
Tuckman BW. Conducting educational research. 3rd ed. New York: Harcourt Brace Jovanovich; 1972.
Tanenbaum SJ. Knowing and acting in medical practice. The epistemological policies of outcomes research. J Health Polit Policy Law. 1994;19:27–44.
Article PubMed CAS Google Scholar
Richardson WS. We should overcome the barriers to evidence-based clinical diagnosis! J Clin Epidemiol. 2007;60:217–27.
Article PubMed Google Scholar
MacCorquodale K, Meehl PE. On a distinction between hypothetical constructs and intervening variables. Psych Rev. 1948;55:95–107.
Article CAS Google Scholar
The National Commission for the Protection of Human Subjects of Biomedical and Behavioral Research: The Belmont Report: Ethical principles and guidelines for the protection of human subjects of research. Washington: DHEW Publication No. (OS) 78–0012, Appendix I, DHEW Publication No. (OS) 78–0013, Appendix II, DHEW Publication (OS) 780014; 1978.
Coryn CLS. The fundamental characteristics of research. J Multidisciplinary Eval. 2006;3:124–33.
Smith NL, Brandon PR. Fundamental issues in evaluation. New York: Guilford; 2008.
Committee on Criteria for Federal Support of Research and Development, National Academy of Sciences, National Academy of Engineering, Institute of Medicine, National Research Council. Allocating federal funds for science and technology. Washington, DC: The National Academies; 1995.
Busse R, Fleming I. A critical look at cardiovascular translational research. Am J Physiol Heart Circ Physiol. 1999;277:H1655–60.
CAS Google Scholar
Schuster DP, Powers WJ. Translational and experimental clinical research. Philadelphia: Lippincott, Williams & Williams; 2005.
Woolf SH. The meaning of translational research and why it matters. JAMA. 2008;299:211–21.
Robertson D, Williams GH. Clinical and translational science: principles of human research. London: Elsevier; 2009.
Goldblatt EM, Lee WH. From bench to bedside: the growing use of translational research in cancer medicine. Am J Transl Res. 2010;2:1–18.
PubMed Google Scholar
Milloy SJ. Science without sense: the risky business of public health research. In: Chapter 5, Mining for statistical associations. Cato Institute. 2009. http://www.junkscience.com/news/sws/sws-chapter5.html . Retrieved 29 Oct 2009.
Gawande A. The cancer-cluster myth. The New Yorker, 8 Feb 1999, p. 34–37.
Kerlinger F. [Chapter 2: problems and hypotheses]. In: Foundations of behavioral research 3rd edn. Orlando: Harcourt, Brace; 1986.
Ioannidis JP. Why most published research findings are false. PLoS Med. 2005;2:e124. Epub 2005 Aug 30.
Andersen B. Methodological errors in medical research. Oxford: Blackwell Scientific Publications; 1990.
DeAngelis C. An introduction to clinical research. New York: Oxford University Press; 1990.
Hennekens CH, Buring JE. Epidemiology in medicine. 1st ed. Boston: Little Brown; 1987.
Jekel JF. Epidemiology, biostatistics, and preventive medicine. 3rd ed. Philadelphia: Saunders Elsevier; 2007.
Hess DR. Retrospective studies and chart reviews. Respir Care. 2004;49:1171–4.
Wissow L, Pascoe J. Types of research models and methods (chapter four). In: An introduction to clinical research. New York: Oxford University Press; 1990.
Bacchieri A, Della Cioppa G. Fundamentals of clinical research: bridging medicine, statistics and operations. Milan: Springer; 2007.
Wood MJ, Ross-Kerr JC. Basic steps in planning nursing research. From question to proposal. 6th ed. Boston: Jones and Barlett; 2005.
DeVita VT, Lawrence TS, Rosenberg SA, Weinberg RA, DePinho RA. Cancer. Principles and practice of oncology, vol. 1. Philadelphia: Wolters Klewer/Lippincott Williams & Wilkins; 2008.
Portney LG, Watkins MP. Foundations of clinical research. Applications to practice. 2nd ed. Upper Saddle River: Prentice Hall Health; 2000.
Marks RG. Designing a research project. The basics of biomedical research methodology. Belmont: Lifetime Learning Publications: A division of Wadsworth; 1982.
Easterbrook PJ, Berlin JA, Gopalan R, Matthews DR. Publication bias in clinical research. Lancet. 1991;337:867–72.
Download references
Author information
Authors and affiliations.
Department of Medicine, College of Medicine, SUNY Downstate Medical Center, 450 Clarkson Avenue, 1199, Brooklyn, NY, 11203, USA
Phyllis G. Supino EdD
You can also search for this author in PubMed Google Scholar
Corresponding author
Correspondence to Phyllis G. Supino EdD .
Editor information
Editors and affiliations.
, Cardiovascular Medicine, SUNY Downstate Medical Center, Clarkson Avenue, box 1199 450, Brooklyn, 11203, USA
Phyllis G. Supino
, Cardiovascualr Medicine, SUNY Downstate Medical Center, Clarkson Avenue 450, Brooklyn, 11203, USA
Jeffrey S. Borer
Rights and permissions
Reprints and permissions
Copyright information
© 2012 Springer Science+Business Media, LLC
About this chapter
Cite this chapter.
Supino, P.G. (2012). Overview of the Research Process. In: Supino, P., Borer, J. (eds) Principles of Research Methodology. Springer, New York, NY. https://doi.org/10.1007/978-1-4614-3360-6_1
Download citation
DOI : https://doi.org/10.1007/978-1-4614-3360-6_1
Published : 18 April 2012
Publisher Name : Springer, New York, NY
Print ISBN : 978-1-4614-3359-0
Online ISBN : 978-1-4614-3360-6
eBook Packages : Medicine Medicine (R0)
Share this chapter
Anyone you share the following link with will be able to read this content:
Sorry, a shareable link is not currently available for this article.
Provided by the Springer Nature SharedIt content-sharing initiative
- Publish with us
Policies and ethics
- Find a journal
- Track your research
- Shinn leads new study of cash payments and peer support to reduce homelessness
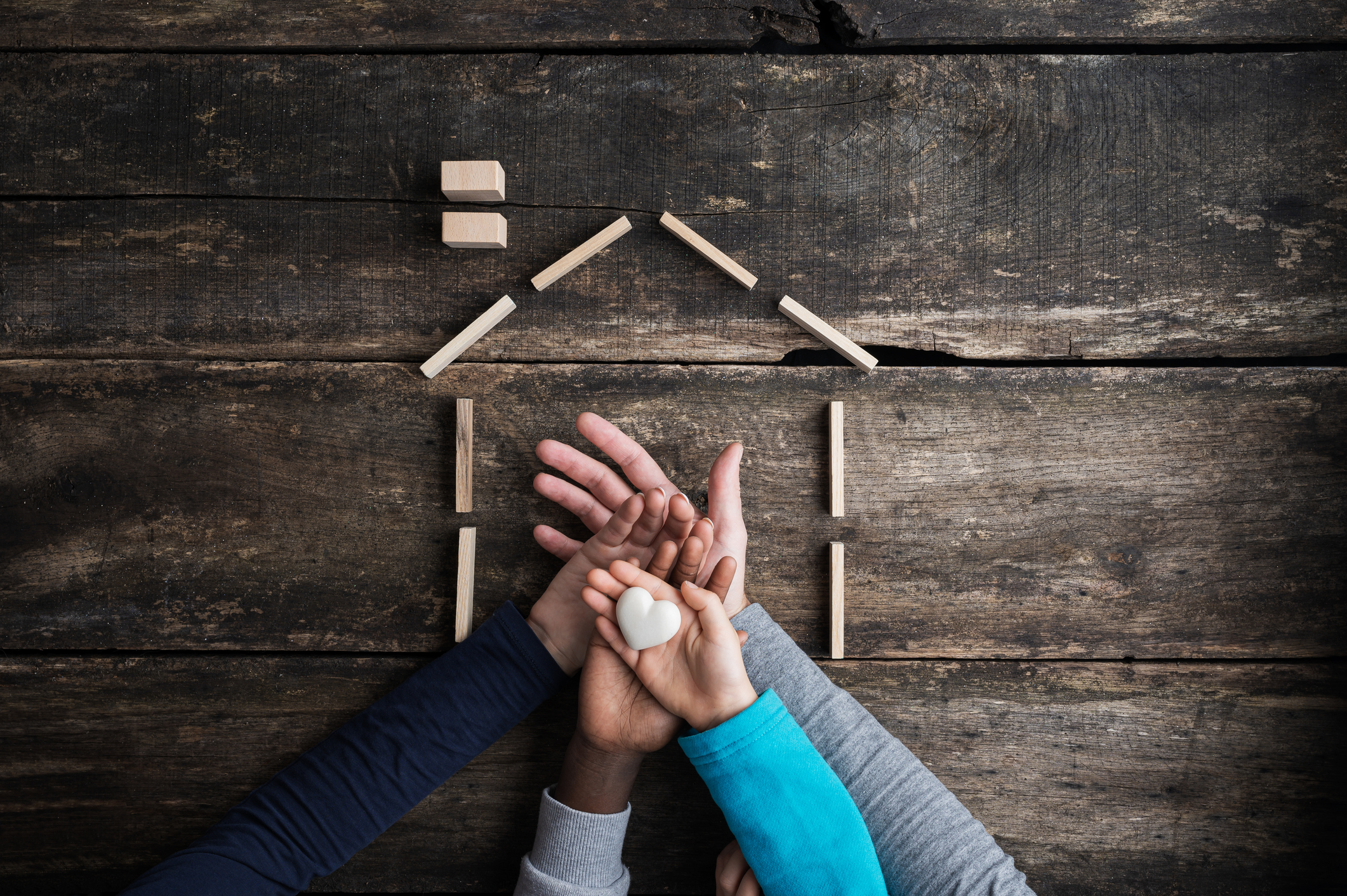
Media Inquiries
- 615-322-6397 Email
Latest Stories
- CLASS OF 2024: Emily Gaven builds team bonds, on and off the lacrosse field
- Limited Submission Opportunity: First Horizon Foundation Grants for Good
Apr 12, 2024, 4:00 PM
By Jenna Somers
Infancy is the age at which someone is most likely to live in a homeless shelter. If that sounds surprising, consider that poverty is the main reason families with young children become homeless. Given the high costs of childcare across the country, often parents leave the workforce to care for their young children. That loss of income plus the cost of housing can sometimes leave families without a home.
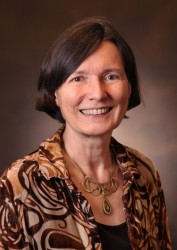
To help reduce homelessness among young families, Beth Shinn is beginning a new study to test whether unconditional direct cash payments and peer support to families who are homeless will help reduce the length of shelter stays and improve other aspects of their lives.
“This study was essentially designed by lived-expertise consultants, a team of mothers who had stayed in New York City shelters with their young children. When we asked them what would help families leave a shelter most rapidly and promote family well-being, the consultants said cash and peer support,” said Shinn, Cornelius Vanderbilt Chair and professor of human and organizational development at Vanderbilt Peabody College of education and human development .
For decades Shinn has studied how to prevent and end homelessness, including in the Family Options Study , the first large-scale analysis of housing program options to end homelessness, now in its 13 th year. Supported by the U.S. Department of Housing and Urban Development, this study found that housing vouchers were effective in stabilizing long-term housing.
The success of housing vouchers in the Family Options study informs Shinn’s rationale for testing the efficacy of unconditional direct cash payments, guided by the assumption that families, when given the funds, will know how to best spend the money to ensure they are able to leave and stay out of homeless shelters.
Shinn is collaborating with the Women in Need shelter system, the largest non-profit family shelter system in New York City. An anonymous family foundation is supporting the study with a $785,050 grant. One hundred families with a child two years old or younger at the start of the study will receive $17,000 per year (paid twice per month) and volunteer peer support for two years. Peers are women who have also gone through the shelter system as well as the numerous processes to establish housing and secure additional resources, such as opening a bank account and finding employment.
The study will include a comparison sample of 100 additional families at the WIN shelter who will only receive $600 per year without specialized peer support. Both groups will get the usual care of the shelter system. Families who could have qualified for the study but aren’t in either the active or comparison groups will make up a larger passive group.
The primary outcome of the study will be to see whether recipients of the larger cash payments have shorter stays in the homeless shelters compared to the other two groups.
“We expect not only shorter stays in homeless shelters, but based on the findings from the Family Options Study, we also expect reductions in distress, domestic violence, substance abuse, and food insecurity, as well as better outcomes for children,” Shinn said.
Beth Shinn teaches in the Community Development and Action M.Ed. program and the Community Research and Action Ph.D. program .
Keep Reading
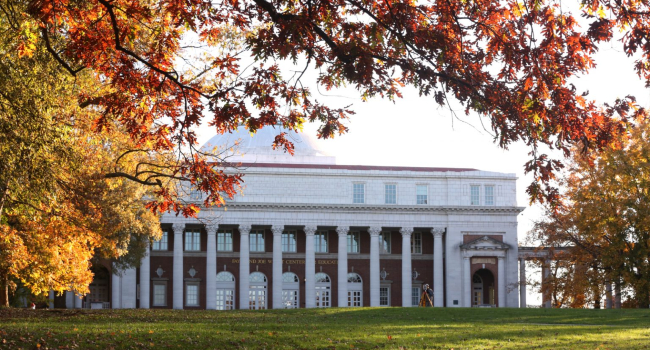
Peabody Scholars share guidance to inform school voucher policy
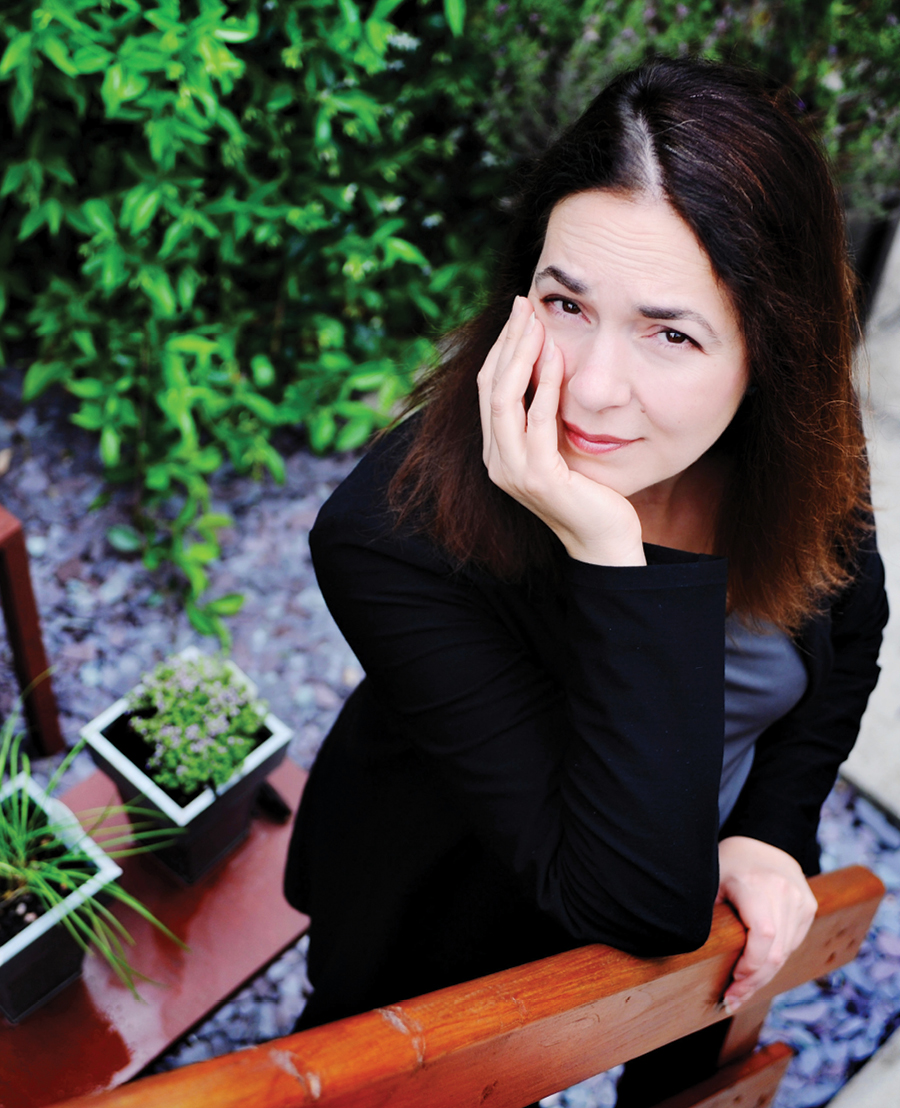
Lorrie Moore wins prestigious National Book Critics Circle Award, continues to gather accolades for new novel
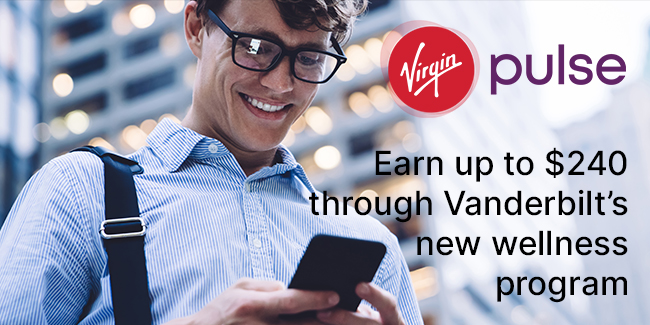
Get healthy, earn cash!
Explore story topics.
- Education and Psychology
- Human and Organizational Development
- Ideas in Action Featured
- Peabody College
- peabody-home
Read our research on: Gun Policy | International Conflict | Election 2024
Regions & Countries
Changing partisan coalitions in a politically divided nation, party identification among registered voters, 1994-2023.
Pew Research Center conducted this analysis to explore partisan identification among U.S. registered voters across major demographic groups and how voters’ partisan affiliation has shifted over time. It also explores the changing composition of voters overall and the partisan coalitions.
For this analysis, we used annual totals of data from Pew Research Center telephone surveys (1994-2018) and online surveys (2019-2023) among registered voters. All telephone survey data was adjusted to account for differences in how people respond to surveys on the telephone compared with online surveys (refer to Appendix A for details).
All online survey data is from the Center’s nationally representative American Trends Panel . The surveys were conducted in both English and Spanish. Each survey is weighted to be representative of the U.S. adult population by gender, age, education, race and ethnicity and other categories. Read more about the ATP’s methodology , as well as how Pew Research Center measures many of the demographic categories used in this report .
The contours of the 2024 political landscape are the result of long-standing patterns of partisanship, combined with the profound demographic changes that have reshaped the United States over the past three decades.
Many of the factors long associated with voters’ partisanship remain firmly in place. For decades, gender, race and ethnicity, and religious affiliation have been important dividing lines in politics. This continues to be the case today.
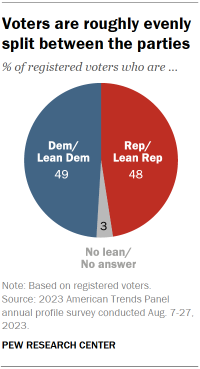
Yet there also have been profound changes – in some cases as a result of demographic change, in others because of dramatic shifts in the partisan allegiances of key groups.
The combined effects of change and continuity have left the country’s two major parties at virtual parity: About half of registered voters (49%) identify as Democrats or lean toward the Democratic Party, while 48% identify as Republicans or lean Republican.
In recent decades, neither party has had a sizable advantage, but the Democratic Party has lost the edge it maintained from 2017 to 2021. (Explore this further in Chapter 1 . )
Pew Research Center’s comprehensive analysis of party identification among registered voters – based on hundreds of thousands of interviews conducted over the past three decades – tracks the changes in the country and the parties since 1994. Among the major findings:
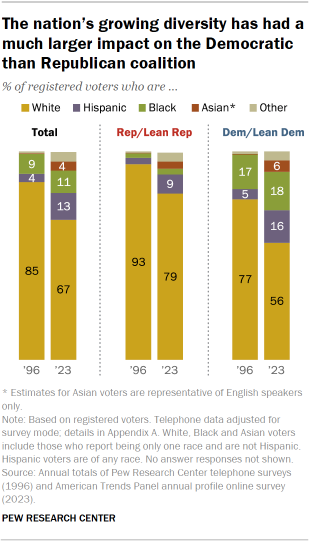
The partisan coalitions are increasingly different. Both parties are more racially and ethnically diverse than in the past. However, this has had a far greater impact on the composition of the Democratic Party than the Republican Party.
The share of voters who are Hispanic has roughly tripled since the mid-1990s; the share who are Asian has increased sixfold over the same period. Today, 44% of Democratic and Democratic-leaning voters are Hispanic, Black, Asian, another race or multiracial, compared with 20% of Republicans and Republican leaners. However, the Democratic Party’s advantages among Black and Hispanic voters, in particular, have narrowed somewhat in recent years. (Explore this further in Chapter 8 .)
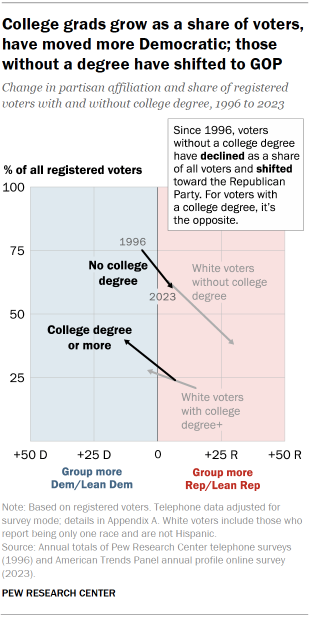
Education and partisanship: The share of voters with a four-year bachelor’s degree keeps increasing, reaching 40% in 2023. And the gap in partisanship between voters with and without a college degree continues to grow, especially among White voters. More than six-in-ten White voters who do not have a four-year degree (63%) associate with the Republican Party, which is up substantially over the past 15 years. White college graduates are closely divided; this was not the case in the 1990s and early 2000s, when they mostly aligned with the GOP. (Explore this further in Chapter 2 .)
Beyond the gender gap: By a modest margin, women voters continue to align with the Democratic Party (by 51% to 44%), while nearly the reverse is true among men (52% align with the Republican Party, 46% with the Democratic Party). The gender gap is about as wide among married men and women. The gap is wider among men and women who have never married; while both groups are majority Democratic, 37% of never-married men identify as Republicans or lean toward the GOP, compared with 24% of never-married women. (Explore this further in Chapter 3 .)
A divide between old and young: Today, each younger age cohort is somewhat more Democratic-oriented than the one before it. The youngest voters (those ages 18 to 24) align with the Democrats by nearly two-to-one (66% to 34% Republican or lean GOP); majorities of older voters (those in their mid-60s and older) identify as Republicans or lean Republican. While there have been wide age divides in American politics over the last two decades, this wasn’t always the case; in the 1990s there were only very modest age differences in partisanship. (Explore this further in Chapter 4 .)
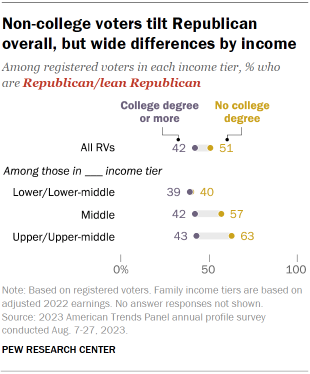
Education and family income: Voters without a college degree differ substantially by income in their party affiliation. Those with middle, upper-middle and upper family incomes tend to align with the GOP. A majority with lower and lower-middle incomes identify as Democrats or lean Democratic. There are no meaningful differences in partisanship among voters with at least a four-year bachelor’s degree; across income categories, majorities of college graduate voters align with the Democratic Party. (Explore this further in Chapter 6 .)
Rural voters move toward the GOP, while the suburbs remain divided: In 2008, when Barack Obama sought his first term as president, voters in rural counties were evenly split in their partisan loyalties. Today, Republicans hold a 25 percentage point advantage among rural residents (60% to 35%). There has been less change among voters in urban counties, who are mostly Democratic by a nearly identical margin (60% to 37%). The suburbs – perennially a political battleground – remain about evenly divided. (Explore this further in Chapter 7 . )
Growing differences among religious groups: Mirroring movement in the population overall, the share of voters who are religiously unaffiliated has grown dramatically over the past 15 years. These voters, who have long aligned with the Democratic Party, have become even more Democratic over time: Today 70% identify as Democrats or lean Democratic. In contrast, Republicans have made gains among several groups of religiously affiliated voters, particularly White Catholics and White evangelical Protestants. White evangelical Protestants now align with the Republican Party by about a 70-point margin (85% to 14%). (Explore this further in Chapter 5 .)
What this report tells us – and what it doesn’t
In most cases, the partisan allegiances of voters do not change a great deal from year to year. Yet as this study shows, the long-term shifts in party identification are substantial and say a great deal about how the country – and its political parties – have changed since the 1990s.

The steadily growing alignment between demographics and partisanship reveals an important aspect of steadily growing partisan polarization. Republicans and Democrats do not just hold different beliefs and opinions about major issues , they are much more different racially, ethnically, geographically and in educational attainment than they used to be.
Yet over this period, there have been only modest shifts in overall partisan identification. Voters remain evenly divided, even as the two parties have grown further apart. The continuing close division in partisan identification among voters is consistent with the relatively narrow margins in the popular votes in most national elections over the past three decades.
Partisan identification provides a broad portrait of voters’ affinities and loyalties. But while it is indicative of voters’ preferences, it does not perfectly predict how people intend to vote in elections, or whether they will vote. In the coming months, Pew Research Center will release reports analyzing voters’ preferences in the presidential election, their engagement with the election and the factors behind candidate support.
Next year, we will release a detailed study of the 2024 election, based on validated voters from the Center’s American Trends Panel. It will examine the demographic composition and vote choices of the 2024 electorate and will provide comparisons to the 2020 and 2016 validated voter studies.
The partisan identification study is based on annual totals from surveys conducted on the Center’s American Trends Panel from 2019 to 2023 and telephone surveys conducted from 1994 to 2018. The survey data was adjusted to account for differences in how the surveys were conducted. For more information, refer to Appendix A .
Previous Pew Research Center analyses of voters’ party identification relied on telephone survey data. This report, for the first time, combines data collected in telephone surveys with data from online surveys conducted on the Center’s nationally representative American Trends Panel.
Directly comparing answers from online and telephone surveys is complex because there are differences in how questions are asked of respondents and in how respondents answer those questions. Together these differences are known as “mode effects.”
As a result of mode effects, it was necessary to adjust telephone trends for leaned party identification in order to allow for direct comparisons over time.
In this report, telephone survey data from 1994 to 2018 is adjusted to align it with online survey responses. In 2014, Pew Research Center randomly assigned respondents to answer a survey by telephone or online. The party identification data from this survey was used to calculate an adjustment for differences between survey mode, which is applied to all telephone survey data in this report.
Please refer to Appendix A for more details.
Sign up for our Politics newsletter
Sent weekly on Wednesday
Report Materials
Table of contents, behind biden’s 2020 victory, a voter data resource: detailed demographic tables about verified voters in 2016, 2018, what the 2020 electorate looks like by party, race and ethnicity, age, education and religion, interactive map: the changing racial and ethnic makeup of the u.s. electorate, in changing u.s. electorate, race and education remain stark dividing lines, most popular.
About Pew Research Center Pew Research Center is a nonpartisan fact tank that informs the public about the issues, attitudes and trends shaping the world. It conducts public opinion polling, demographic research, media content analysis and other empirical social science research. Pew Research Center does not take policy positions. It is a subsidiary of The Pew Charitable Trusts .
Have a language expert improve your writing
Run a free plagiarism check in 10 minutes, generate accurate citations for free.
- Knowledge Base
- Starting the research process
A Beginner's Guide to Starting the Research Process
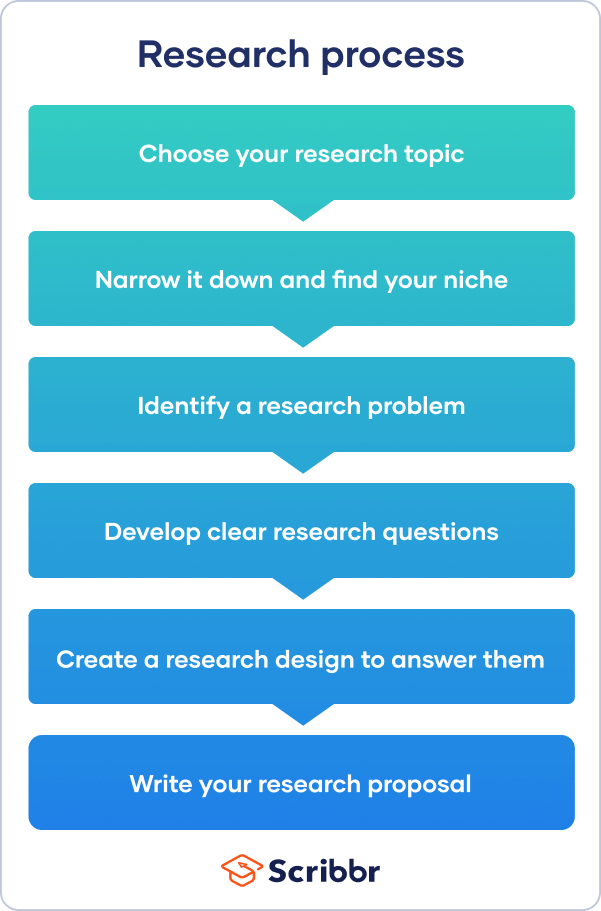
When you have to write a thesis or dissertation , it can be hard to know where to begin, but there are some clear steps you can follow.
The research process often begins with a very broad idea for a topic you’d like to know more about. You do some preliminary research to identify a problem . After refining your research questions , you can lay out the foundations of your research design , leading to a proposal that outlines your ideas and plans.
This article takes you through the first steps of the research process, helping you narrow down your ideas and build up a strong foundation for your research project.
Table of contents
Step 1: choose your topic, step 2: identify a problem, step 3: formulate research questions, step 4: create a research design, step 5: write a research proposal, other interesting articles.
First you have to come up with some ideas. Your thesis or dissertation topic can start out very broad. Think about the general area or field you’re interested in—maybe you already have specific research interests based on classes you’ve taken, or maybe you had to consider your topic when applying to graduate school and writing a statement of purpose .
Even if you already have a good sense of your topic, you’ll need to read widely to build background knowledge and begin narrowing down your ideas. Conduct an initial literature review to begin gathering relevant sources. As you read, take notes and try to identify problems, questions, debates, contradictions and gaps. Your aim is to narrow down from a broad area of interest to a specific niche.
Make sure to consider the practicalities: the requirements of your programme, the amount of time you have to complete the research, and how difficult it will be to access sources and data on the topic. Before moving onto the next stage, it’s a good idea to discuss the topic with your thesis supervisor.
>>Read more about narrowing down a research topic
Receive feedback on language, structure, and formatting
Professional editors proofread and edit your paper by focusing on:
- Academic style
- Vague sentences
- Style consistency
See an example
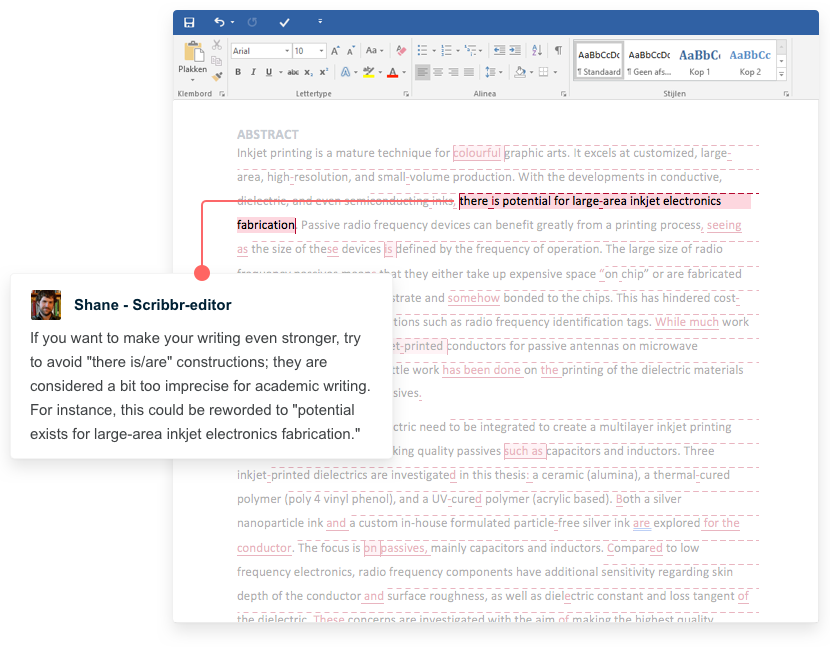
So you’ve settled on a topic and found a niche—but what exactly will your research investigate, and why does it matter? To give your project focus and purpose, you have to define a research problem .
The problem might be a practical issue—for example, a process or practice that isn’t working well, an area of concern in an organization’s performance, or a difficulty faced by a specific group of people in society.
Alternatively, you might choose to investigate a theoretical problem—for example, an underexplored phenomenon or relationship, a contradiction between different models or theories, or an unresolved debate among scholars.
To put the problem in context and set your objectives, you can write a problem statement . This describes who the problem affects, why research is needed, and how your research project will contribute to solving it.
>>Read more about defining a research problem
Next, based on the problem statement, you need to write one or more research questions . These target exactly what you want to find out. They might focus on describing, comparing, evaluating, or explaining the research problem.
A strong research question should be specific enough that you can answer it thoroughly using appropriate qualitative or quantitative research methods. It should also be complex enough to require in-depth investigation, analysis, and argument. Questions that can be answered with “yes/no” or with easily available facts are not complex enough for a thesis or dissertation.
In some types of research, at this stage you might also have to develop a conceptual framework and testable hypotheses .
>>See research question examples
The research design is a practical framework for answering your research questions. It involves making decisions about the type of data you need, the methods you’ll use to collect and analyze it, and the location and timescale of your research.
There are often many possible paths you can take to answering your questions. The decisions you make will partly be based on your priorities. For example, do you want to determine causes and effects, draw generalizable conclusions, or understand the details of a specific context?
You need to decide whether you will use primary or secondary data and qualitative or quantitative methods . You also need to determine the specific tools, procedures, and materials you’ll use to collect and analyze your data, as well as your criteria for selecting participants or sources.
>>Read more about creating a research design
Here's why students love Scribbr's proofreading services
Discover proofreading & editing
Finally, after completing these steps, you are ready to complete a research proposal . The proposal outlines the context, relevance, purpose, and plan of your research.
As well as outlining the background, problem statement, and research questions, the proposal should also include a literature review that shows how your project will fit into existing work on the topic. The research design section describes your approach and explains exactly what you will do.
You might have to get the proposal approved by your supervisor before you get started, and it will guide the process of writing your thesis or dissertation.
>>Read more about writing a research proposal
If you want to know more about the research process , methodology , research bias , or statistics , make sure to check out some of our other articles with explanations and examples.
Methodology
- Sampling methods
- Simple random sampling
- Stratified sampling
- Cluster sampling
- Likert scales
- Reproducibility
Statistics
- Null hypothesis
- Statistical power
- Probability distribution
- Effect size
- Poisson distribution
Research bias
- Optimism bias
- Cognitive bias
- Implicit bias
- Hawthorne effect
- Anchoring bias
- Explicit bias
Is this article helpful?
Other students also liked.
- Writing Strong Research Questions | Criteria & Examples
What Is a Research Design | Types, Guide & Examples
- How to Write a Research Proposal | Examples & Templates
More interesting articles
- 10 Research Question Examples to Guide Your Research Project
- How to Choose a Dissertation Topic | 8 Steps to Follow
- How to Define a Research Problem | Ideas & Examples
- How to Write a Problem Statement | Guide & Examples
- Relevance of Your Dissertation Topic | Criteria & Tips
- Research Objectives | Definition & Examples
- What Is a Fishbone Diagram? | Templates & Examples
- What Is Root Cause Analysis? | Definition & Examples
Unlimited Academic AI-Proofreading
✔ Document error-free in 5minutes ✔ Unlimited document corrections ✔ Specialized in correcting academic texts
- See us on facebook
- See us on twitter
- See us on youtube
- See us on linkedin
- See us on instagram
Two key brain systems are central to psychosis, Stanford Medicine-led study finds
When the brain has trouble filtering incoming information and predicting what’s likely to happen, psychosis can result, Stanford Medicine-led research shows.
April 11, 2024 - By Erin Digitale

People with psychosis have trouble filtering relevant information (mesh funnel) and predicting rewarding events (broken crystal ball), creating a complex inner world. Emily Moskal
Inside the brains of people with psychosis, two key systems are malfunctioning: a “filter” that directs attention toward important external events and internal thoughts, and a “predictor” composed of pathways that anticipate rewards.
Dysfunction of these systems makes it difficult to know what’s real, manifesting as hallucinations and delusions.
The findings come from a Stanford Medicine-led study , published April 11 in Molecular Psychiatry , that used brain scan data from children, teens and young adults with psychosis. The results confirm an existing theory of how breaks with reality occur.
“This work provides a good model for understanding the development and progression of schizophrenia, which is a challenging problem,” said lead author Kaustubh Supekar , PhD, clinical associate professor of psychiatry and behavioral sciences.
The findings, observed in individuals with a rare genetic disease called 22q11.2 deletion syndrome who experience psychosis as well as in those with psychosis of unknown origin, advance scientists’ understanding of the underlying brain mechanisms and theoretical frameworks related to psychosis.
During psychosis, patients experience hallucinations, such as hearing voices, and hold delusional beliefs, such as thinking that people who are not real exist. Psychosis can occur on its own and isa hallmark of certain serious mental illnesses, including bipolar disorder and schizophrenia. Schizophrenia is also characterized by social withdrawal, disorganized thinking and speech, and a reduction in energy and motivation.
It is challenging to study how schizophrenia begins in the brain. The condition usually emerges in teens or young adults, most of whom soon begin taking antipsychotic medications to ease their symptoms. When researchers analyze brain scans from people with established schizophrenia, they cannot distinguish the effects of the disease from the effects of the medications. They also do not know how schizophrenia changes the brain as the disease progresses.
To get an early view of the disease process, the Stanford Medicine team studied young people aged 6 to 39 with 22q11.2 deletion syndrome, a genetic condition with a 30% risk for psychosis, schizophrenia or both.

Kaustubh Supekar
Brain function in 22q11.2 patients who have psychosis is similar to that in people with psychosis of unknown origin, they found. And these brain patterns matched what the researchers had previously theorized was generating psychosis symptoms.
“The brain patterns we identified support our theoretical models of how cognitive control systems malfunction in psychosis,” said senior study author Vinod Menon , PhD, the Rachael L. and Walter F. Nichols, MD, Professor; a professor of psychiatry and behavioral sciences; and director of the Stanford Cognitive and Systems Neuroscience Laboratory .
Thoughts that are not linked to reality can capture the brain’s cognitive control networks, he said. “This process derails the normal functioning of cognitive control, allowing intrusive thoughts to dominate, culminating in symptoms we recognize as psychosis.”
Cerebral sorting
Normally, the brain’s cognitive filtering system — aka the salience network — works behind the scenes to selectively direct our attention to important internal thoughts and external events. With its help, we can dismiss irrational thoughts and unimportant events and focus on what’s real and meaningful to us, such as paying attention to traffic so we avoid a collision.
The ventral striatum, a small brain region, and associated brain pathways driven by dopamine, play an important role in predicting what will be rewarding or important.
For the study, the researchers assembled as much functional MRI brain-scan data as possible from young people with 22q11.2 deletion syndrome, totaling 101 individuals scanned at three different universities. (The study also included brain scans from several comparison groups without 22q11.2 deletion syndrome: 120 people with early idiopathic psychosis, 101 people with autism, 123 with attention deficit/hyperactivity disorder and 411 healthy controls.)
The genetic condition, characterized by deletion of part of the 22nd chromosome, affects 1 in every 2,000 to 4,000 people. In addition to the 30% risk of schizophrenia or psychosis, people with the syndrome can also have autism or attention deficit hyperactivity disorder, which is why these conditions were included in the comparison groups.
The researchers used a type of machine learning algorithm called a spatiotemporal deep neural network to characterize patterns of brain function in all patients with 22q11.2 deletion syndrome compared with healthy subjects. With a cohort of patients whose brains were scanned at the University of California, Los Angeles, they developed an algorithmic model that distinguished brain scans from people with 22q11.2 deletion syndrome versus those without it. The model predicted the syndrome with greater than 94% accuracy. They validated the model in additional groups of people with or without the genetic syndrome who had received brain scans at UC Davis and Pontificia Universidad Católica de Chile, showing that in these independent groups, the model sorted brain scans with 84% to 90% accuracy.
The researchers then used the model to investigate which brain features play the biggest role in psychosis. Prior studies of psychosis had not given consistent results, likely because their sample sizes were too small.

Vinod Menon
Comparing brain scans from 22q11.2 deletion syndrome patients who had and did not have psychosis, the researchers showed that the brain areas contributing most to psychosis are the anterior insula (a key part of the salience network or “filter”) and the ventral striatum (the “reward predictor”); this was true for different cohorts of patients.
In comparing the brain features of people with 22q11.2 deletion syndrome and psychosis against people with psychosis of unknown origin, the model found significant overlap, indicating that these brain features are characteristic of psychosis in general.
A second mathematical model, trained to distinguish all subjects with 22q11.2 deletion syndrome and psychosis from those who have the genetic syndrome but without psychosis, selected brain scans from people with idiopathic psychosis with 77.5% accuracy, again supporting the idea that the brain’s filtering and predicting centers are key to psychosis.
Furthermore, this model was specific to psychosis: It could not classify people with idiopathic autism or ADHD.
“It was quite exciting to trace our steps back to our initial question — ‘What are the dysfunctional brain systems in schizophrenia?’ — and to discover similar patterns in this context,” Menon said. “At the neural level, the characteristics differentiating individuals with psychosis in 22q11.2 deletion syndrome are mirroring the pathways we’ve pinpointed in schizophrenia. This parallel reinforces our understanding of psychosis as a condition with identifiable and consistent brain signatures.” However, these brain signatures were not seen in people with the genetic syndrome but no psychosis, holding clues to future directions for research, he added.
Applications for treatment or prevention
In addition to supporting the scientists’ theory about how psychosis occurs, the findings have implications for understanding the condition — and possibly preventing it.
“One of my goals is to prevent or delay development of schizophrenia,” Supekar said. The fact that the new findings are consistent with the team’s prior research on which brain centers contribute most to schizophrenia in adults suggests there may be a way to prevent it, he said. “In schizophrenia, by the time of diagnosis, a lot of damage has already occurred in the brain, and it can be very difficult to change the course of the disease.”
“What we saw is that, early on, functional interactions among brain regions within the same brain systems are abnormal,” he added. “The abnormalities do not start when you are in your 20s; they are evident even when you are 7 or 8.”
Our discoveries underscore the importance of approaching people with psychosis with compassion.
The researchers plan to use existing treatments, such as transcranial magnetic stimulation or focused ultrasound, targeted at these brain centers in young people at risk of psychosis, such as those with 22q11.2 deletion syndrome or with two parents who have schizophrenia, to see if they prevent or delay the onset of the condition or lessen symptoms once they appear.
The results also suggest that using functional MRI to monitor brain activity at the key centers could help scientists investigate how existing antipsychotic medications are working.
Although it’s still puzzling why someone becomes untethered from reality — given how risky it seems for one’s well-being — the “how” is now understandable, Supekar said. “From a mechanistic point of view, it makes sense,” he said.
“Our discoveries underscore the importance of approaching people with psychosis with compassion,” Menon said, adding that his team hopes their work not only advances scientific understanding but also inspires a cultural shift toward empathy and support for those experiencing psychosis.
“I recently had the privilege of engaging with individuals from our department’s early psychosis treatment group,” he said. “Their message was a clear and powerful: ‘We share more similarities than differences. Like anyone, we experience our own highs and lows.’ Their words were a heartfelt appeal for greater empathy and understanding toward those living with this condition. It was a call to view psychosis through a lens of empathy and solidarity.”
Researchers contributed to the study from UCLA, Clinica Alemana Universidad del Desarrollo, Pontificia Universidad Católica de Chile, the University of Oxford and UC Davis.
The study was funded by the Stanford Maternal and Child Health Research Institute’s Uytengsu-Hamilton 22q11 Neuropsychiatry Research Program, FONDEYCT (the National Fund for Scientific and Technological Development of the government of Chile), ANID-Chile (the Chilean National Agency for Research and Development) and the U.S. National Institutes of Health (grants AG072114, MH121069, MH085953 and MH101779).
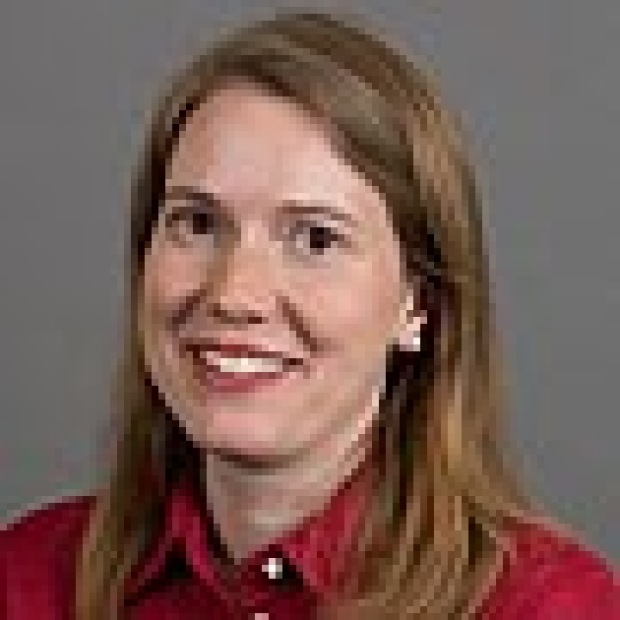
About Stanford Medicine
Stanford Medicine is an integrated academic health system comprising the Stanford School of Medicine and adult and pediatric health care delivery systems. Together, they harness the full potential of biomedicine through collaborative research, education and clinical care for patients. For more information, please visit med.stanford.edu .
Artificial intelligence
Exploring ways AI is applied to health care
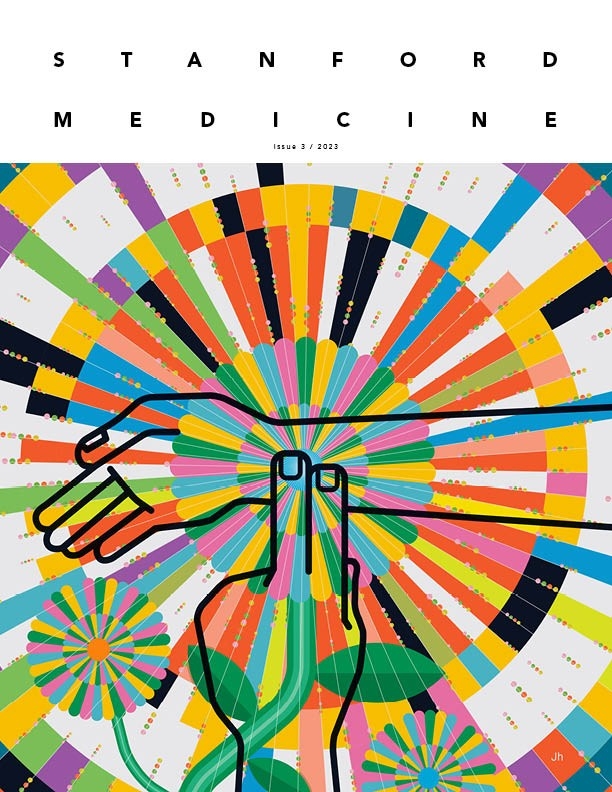

An official website of the United States government
The .gov means it's official. Federal government websites often end in .gov or .mil. Before sharing sensitive information, make sure you're on a federal government site.
The site is secure. The https:// ensures that you are connecting to the official website and that any information you provide is encrypted and transmitted securely.
- Publications
- Account settings
- Browse Titles
NCBI Bookshelf. A service of the National Library of Medicine, National Institutes of Health.
StatPearls [Internet]. Treasure Island (FL): StatPearls Publishing; 2024 Jan-.

StatPearls [Internet].
Qualitative study.
Steven Tenny ; Janelle M. Brannan ; Grace D. Brannan .
Affiliations
Last Update: September 18, 2022 .
- Introduction
Qualitative research is a type of research that explores and provides deeper insights into real-world problems. [1] Instead of collecting numerical data points or intervene or introduce treatments just like in quantitative research, qualitative research helps generate hypotheses as well as further investigate and understand quantitative data. Qualitative research gathers participants' experiences, perceptions, and behavior. It answers the hows and whys instead of how many or how much. It could be structured as a stand-alone study, purely relying on qualitative data or it could be part of mixed-methods research that combines qualitative and quantitative data. This review introduces the readers to some basic concepts, definitions, terminology, and application of qualitative research.
Qualitative research at its core, ask open-ended questions whose answers are not easily put into numbers such as ‘how’ and ‘why’. [2] Due to the open-ended nature of the research questions at hand, qualitative research design is often not linear in the same way quantitative design is. [2] One of the strengths of qualitative research is its ability to explain processes and patterns of human behavior that can be difficult to quantify. [3] Phenomena such as experiences, attitudes, and behaviors can be difficult to accurately capture quantitatively, whereas a qualitative approach allows participants themselves to explain how, why, or what they were thinking, feeling, and experiencing at a certain time or during an event of interest. Quantifying qualitative data certainly is possible, but at its core, qualitative data is looking for themes and patterns that can be difficult to quantify and it is important to ensure that the context and narrative of qualitative work are not lost by trying to quantify something that is not meant to be quantified.
However, while qualitative research is sometimes placed in opposition to quantitative research, where they are necessarily opposites and therefore ‘compete’ against each other and the philosophical paradigms associated with each, qualitative and quantitative work are not necessarily opposites nor are they incompatible. [4] While qualitative and quantitative approaches are different, they are not necessarily opposites, and they are certainly not mutually exclusive. For instance, qualitative research can help expand and deepen understanding of data or results obtained from quantitative analysis. For example, say a quantitative analysis has determined that there is a correlation between length of stay and level of patient satisfaction, but why does this correlation exist? This dual-focus scenario shows one way in which qualitative and quantitative research could be integrated together.
Examples of Qualitative Research Approaches
Ethnography
Ethnography as a research design has its origins in social and cultural anthropology, and involves the researcher being directly immersed in the participant’s environment. [2] Through this immersion, the ethnographer can use a variety of data collection techniques with the aim of being able to produce a comprehensive account of the social phenomena that occurred during the research period. [2] That is to say, the researcher’s aim with ethnography is to immerse themselves into the research population and come out of it with accounts of actions, behaviors, events, etc. through the eyes of someone involved in the population. Direct involvement of the researcher with the target population is one benefit of ethnographic research because it can then be possible to find data that is otherwise very difficult to extract and record.
Grounded Theory
Grounded Theory is the “generation of a theoretical model through the experience of observing a study population and developing a comparative analysis of their speech and behavior.” [5] As opposed to quantitative research which is deductive and tests or verifies an existing theory, grounded theory research is inductive and therefore lends itself to research that is aiming to study social interactions or experiences. [3] [2] In essence, Grounded Theory’s goal is to explain for example how and why an event occurs or how and why people might behave a certain way. Through observing the population, a researcher using the Grounded Theory approach can then develop a theory to explain the phenomena of interest.
Phenomenology
Phenomenology is defined as the “study of the meaning of phenomena or the study of the particular”. [5] At first glance, it might seem that Grounded Theory and Phenomenology are quite similar, but upon careful examination, the differences can be seen. At its core, phenomenology looks to investigate experiences from the perspective of the individual. [2] Phenomenology is essentially looking into the ‘lived experiences’ of the participants and aims to examine how and why participants behaved a certain way, from their perspective . Herein lies one of the main differences between Grounded Theory and Phenomenology. Grounded Theory aims to develop a theory for social phenomena through an examination of various data sources whereas Phenomenology focuses on describing and explaining an event or phenomena from the perspective of those who have experienced it.
Narrative Research
One of qualitative research’s strengths lies in its ability to tell a story, often from the perspective of those directly involved in it. Reporting on qualitative research involves including details and descriptions of the setting involved and quotes from participants. This detail is called ‘thick’ or ‘rich’ description and is a strength of qualitative research. Narrative research is rife with the possibilities of ‘thick’ description as this approach weaves together a sequence of events, usually from just one or two individuals, in the hopes of creating a cohesive story, or narrative. [2] While it might seem like a waste of time to focus on such a specific, individual level, understanding one or two people’s narratives for an event or phenomenon can help to inform researchers about the influences that helped shape that narrative. The tension or conflict of differing narratives can be “opportunities for innovation”. [2]
Research Paradigm
Research paradigms are the assumptions, norms, and standards that underpin different approaches to research. Essentially, research paradigms are the ‘worldview’ that inform research. [4] It is valuable for researchers, both qualitative and quantitative, to understand what paradigm they are working within because understanding the theoretical basis of research paradigms allows researchers to understand the strengths and weaknesses of the approach being used and adjust accordingly. Different paradigms have different ontology and epistemologies . Ontology is defined as the "assumptions about the nature of reality” whereas epistemology is defined as the “assumptions about the nature of knowledge” that inform the work researchers do. [2] It is important to understand the ontological and epistemological foundations of the research paradigm researchers are working within to allow for a full understanding of the approach being used and the assumptions that underpin the approach as a whole. Further, it is crucial that researchers understand their own ontological and epistemological assumptions about the world in general because their assumptions about the world will necessarily impact how they interact with research. A discussion of the research paradigm is not complete without describing positivist, postpositivist, and constructivist philosophies.
Positivist vs Postpositivist
To further understand qualitative research, we need to discuss positivist and postpositivist frameworks. Positivism is a philosophy that the scientific method can and should be applied to social as well as natural sciences. [4] Essentially, positivist thinking insists that the social sciences should use natural science methods in its research which stems from positivist ontology that there is an objective reality that exists that is fully independent of our perception of the world as individuals. Quantitative research is rooted in positivist philosophy, which can be seen in the value it places on concepts such as causality, generalizability, and replicability.
Conversely, postpositivists argue that social reality can never be one hundred percent explained but it could be approximated. [4] Indeed, qualitative researchers have been insisting that there are “fundamental limits to the extent to which the methods and procedures of the natural sciences could be applied to the social world” and therefore postpositivist philosophy is often associated with qualitative research. [4] An example of positivist versus postpositivist values in research might be that positivist philosophies value hypothesis-testing, whereas postpositivist philosophies value the ability to formulate a substantive theory.
Constructivist
Constructivism is a subcategory of postpositivism. Most researchers invested in postpositivist research are constructivist as well, meaning they think there is no objective external reality that exists but rather that reality is constructed. Constructivism is a theoretical lens that emphasizes the dynamic nature of our world. “Constructivism contends that individuals’ views are directly influenced by their experiences, and it is these individual experiences and views that shape their perspective of reality”. [6] Essentially, Constructivist thought focuses on how ‘reality’ is not a fixed certainty and experiences, interactions, and backgrounds give people a unique view of the world. Constructivism contends, unlike in positivist views, that there is not necessarily an ‘objective’ reality we all experience. This is the ‘relativist’ ontological view that reality and the world we live in are dynamic and socially constructed. Therefore, qualitative scientific knowledge can be inductive as well as deductive.” [4]
So why is it important to understand the differences in assumptions that different philosophies and approaches to research have? Fundamentally, the assumptions underpinning the research tools a researcher selects provide an overall base for the assumptions the rest of the research will have and can even change the role of the researcher themselves. [2] For example, is the researcher an ‘objective’ observer such as in positivist quantitative work? Or is the researcher an active participant in the research itself, as in postpositivist qualitative work? Understanding the philosophical base of the research undertaken allows researchers to fully understand the implications of their work and their role within the research, as well as reflect on their own positionality and bias as it pertains to the research they are conducting.
Data Sampling
The better the sample represents the intended study population, the more likely the researcher is to encompass the varying factors at play. The following are examples of participant sampling and selection: [7]
- Purposive sampling- selection based on the researcher’s rationale in terms of being the most informative.
- Criterion sampling-selection based on pre-identified factors.
- Convenience sampling- selection based on availability.
- Snowball sampling- the selection is by referral from other participants or people who know potential participants.
- Extreme case sampling- targeted selection of rare cases.
- Typical case sampling-selection based on regular or average participants.
Data Collection and Analysis
Qualitative research uses several techniques including interviews, focus groups, and observation. [1] [2] [3] Interviews may be unstructured, with open-ended questions on a topic and the interviewer adapts to the responses. Structured interviews have a predetermined number of questions that every participant is asked. It is usually one on one and is appropriate for sensitive topics or topics needing an in-depth exploration. Focus groups are often held with 8-12 target participants and are used when group dynamics and collective views on a topic are desired. Researchers can be a participant-observer to share the experiences of the subject or a non-participant or detached observer.
While quantitative research design prescribes a controlled environment for data collection, qualitative data collection may be in a central location or in the environment of the participants, depending on the study goals and design. Qualitative research could amount to a large amount of data. Data is transcribed which may then be coded manually or with the use of Computer Assisted Qualitative Data Analysis Software or CAQDAS such as ATLAS.ti or NVivo. [8] [9] [10]
After the coding process, qualitative research results could be in various formats. It could be a synthesis and interpretation presented with excerpts from the data. [11] Results also could be in the form of themes and theory or model development.
Dissemination
To standardize and facilitate the dissemination of qualitative research outcomes, the healthcare team can use two reporting standards. The Consolidated Criteria for Reporting Qualitative Research or COREQ is a 32-item checklist for interviews and focus groups. [12] The Standards for Reporting Qualitative Research (SRQR) is a checklist covering a wider range of qualitative research. [13]
Examples of Application
Many times a research question will start with qualitative research. The qualitative research will help generate the research hypothesis which can be tested with quantitative methods. After the data is collected and analyzed with quantitative methods, a set of qualitative methods can be used to dive deeper into the data for a better understanding of what the numbers truly mean and their implications. The qualitative methods can then help clarify the quantitative data and also help refine the hypothesis for future research. Furthermore, with qualitative research researchers can explore subjects that are poorly studied with quantitative methods. These include opinions, individual's actions, and social science research.
A good qualitative study design starts with a goal or objective. This should be clearly defined or stated. The target population needs to be specified. A method for obtaining information from the study population must be carefully detailed to ensure there are no omissions of part of the target population. A proper collection method should be selected which will help obtain the desired information without overly limiting the collected data because many times, the information sought is not well compartmentalized or obtained. Finally, the design should ensure adequate methods for analyzing the data. An example may help better clarify some of the various aspects of qualitative research.
A researcher wants to decrease the number of teenagers who smoke in their community. The researcher could begin by asking current teen smokers why they started smoking through structured or unstructured interviews (qualitative research). The researcher can also get together a group of current teenage smokers and conduct a focus group to help brainstorm factors that may have prevented them from starting to smoke (qualitative research).
In this example, the researcher has used qualitative research methods (interviews and focus groups) to generate a list of ideas of both why teens start to smoke as well as factors that may have prevented them from starting to smoke. Next, the researcher compiles this data. The research found that, hypothetically, peer pressure, health issues, cost, being considered “cool,” and rebellious behavior all might increase or decrease the likelihood of teens starting to smoke.
The researcher creates a survey asking teen participants to rank how important each of the above factors is in either starting smoking (for current smokers) or not smoking (for current non-smokers). This survey provides specific numbers (ranked importance of each factor) and is thus a quantitative research tool.
The researcher can use the results of the survey to focus efforts on the one or two highest-ranked factors. Let us say the researcher found that health was the major factor that keeps teens from starting to smoke, and peer pressure was the major factor that contributed to teens to start smoking. The researcher can go back to qualitative research methods to dive deeper into each of these for more information. The researcher wants to focus on how to keep teens from starting to smoke, so they focus on the peer pressure aspect.
The researcher can conduct interviews and/or focus groups (qualitative research) about what types and forms of peer pressure are commonly encountered, where the peer pressure comes from, and where smoking first starts. The researcher hypothetically finds that peer pressure often occurs after school at the local teen hangouts, mostly the local park. The researcher also hypothetically finds that peer pressure comes from older, current smokers who provide the cigarettes.
The researcher could further explore this observation made at the local teen hangouts (qualitative research) and take notes regarding who is smoking, who is not, and what observable factors are at play for peer pressure of smoking. The researcher finds a local park where many local teenagers hang out and see that a shady, overgrown area of the park is where the smokers tend to hang out. The researcher notes the smoking teenagers buy their cigarettes from a local convenience store adjacent to the park where the clerk does not check identification before selling cigarettes. These observations fall under qualitative research.
If the researcher returns to the park and counts how many individuals smoke in each region of the park, this numerical data would be quantitative research. Based on the researcher's efforts thus far, they conclude that local teen smoking and teenagers who start to smoke may decrease if there are fewer overgrown areas of the park and the local convenience store does not sell cigarettes to underage individuals.
The researcher could try to have the parks department reassess the shady areas to make them less conducive to the smokers or identify how to limit the sales of cigarettes to underage individuals by the convenience store. The researcher would then cycle back to qualitative methods of asking at-risk population their perceptions of the changes, what factors are still at play, as well as quantitative research that includes teen smoking rates in the community, the incidence of new teen smokers, among others. [14] [15]
Qualitative research functions as a standalone research design or in combination with quantitative research to enhance our understanding of the world. Qualitative research uses techniques including structured and unstructured interviews, focus groups, and participant observation to not only help generate hypotheses which can be more rigorously tested with quantitative research but also to help researchers delve deeper into the quantitative research numbers, understand what they mean, and understand what the implications are. Qualitative research provides researchers with a way to understand what is going on, especially when things are not easily categorized. [16]
- Issues of Concern
As discussed in the sections above, quantitative and qualitative work differ in many different ways, including the criteria for evaluating them. There are four well-established criteria for evaluating quantitative data: internal validity, external validity, reliability, and objectivity. The correlating concepts in qualitative research are credibility, transferability, dependability, and confirmability. [4] [11] The corresponding quantitative and qualitative concepts can be seen below, with the quantitative concept is on the left, and the qualitative concept is on the right:
- Internal validity--- Credibility
- External validity---Transferability
- Reliability---Dependability
- Objectivity---Confirmability
In conducting qualitative research, ensuring these concepts are satisfied and well thought out can mitigate potential issues from arising. For example, just as a researcher will ensure that their quantitative study is internally valid so should qualitative researchers ensure that their work has credibility.
Indicators such as triangulation and peer examination can help evaluate the credibility of qualitative work.
- Triangulation: Triangulation involves using multiple methods of data collection to increase the likelihood of getting a reliable and accurate result. In our above magic example, the result would be more reliable by also interviewing the magician, back-stage hand, and the person who "vanished." In qualitative research, triangulation can include using telephone surveys, in-person surveys, focus groups, and interviews as well as surveying an adequate cross-section of the target demographic.
- Peer examination: Results can be reviewed by a peer to ensure the data is consistent with the findings.
‘Thick’ or ‘rich’ description can be used to evaluate the transferability of qualitative research whereas using an indicator such as an audit trail might help with evaluating the dependability and confirmability.
- Thick or rich description is a detailed and thorough description of details, the setting, and quotes from participants in the research. [5] Thick descriptions will include a detailed explanation of how the study was carried out. Thick descriptions are detailed enough to allow readers to draw conclusions and interpret the data themselves, which can help with transferability and replicability.
- Audit trail: An audit trail provides a documented set of steps of how the participants were selected and the data was collected. The original records of information should also be kept (e.g., surveys, notes, recordings).
One issue of concern that qualitative researchers should take into consideration is observation bias. Here are a few examples:
- Hawthorne effect: The Hawthorne effect is the change in participant behavior when they know they are being observed. If a researcher was wanting to identify factors that contribute to employee theft and tells the employees they are going to watch them to see what factors affect employee theft, one would suspect employee behavior would change when they know they are being watched.
- Observer-expectancy effect: Some participants change their behavior or responses to satisfy the researcher's desired effect. This happens in an unconscious manner for the participant so it is important to eliminate or limit transmitting the researcher's views.
- Artificial scenario effect: Some qualitative research occurs in artificial scenarios and/or with preset goals. In such situations, the information may not be accurate because of the artificial nature of the scenario. The preset goals may limit the qualitative information obtained.
- Clinical Significance
Qualitative research by itself or combined with quantitative research helps healthcare providers understand patients and the impact and challenges of the care they deliver. Qualitative research provides an opportunity to generate and refine hypotheses and delve deeper into the data generated by quantitative research. Qualitative research does not exist as an island apart from quantitative research, but as an integral part of research methods to be used for the understanding of the world around us. [17]
- Enhancing Healthcare Team Outcomes
Qualitative research is important for all members of the health care team as all are affected by qualitative research. Qualitative research may help develop a theory or a model for health research that can be further explored by quantitative research. Much of the qualitative research data acquisition is completed by numerous team members including social works, scientists, nurses, etc. Within each area of the medical field, there is copious ongoing qualitative research including physician-patient interactions, nursing-patient interactions, patient-environment interactions, health care team function, patient information delivery, etc.
- Review Questions
- Access free multiple choice questions on this topic.
- Comment on this article.
Disclosure: Steven Tenny declares no relevant financial relationships with ineligible companies.
Disclosure: Janelle Brannan declares no relevant financial relationships with ineligible companies.
Disclosure: Grace Brannan declares no relevant financial relationships with ineligible companies.
This book is distributed under the terms of the Creative Commons Attribution-NonCommercial-NoDerivatives 4.0 International (CC BY-NC-ND 4.0) ( http://creativecommons.org/licenses/by-nc-nd/4.0/ ), which permits others to distribute the work, provided that the article is not altered or used commercially. You are not required to obtain permission to distribute this article, provided that you credit the author and journal.
- Cite this Page Tenny S, Brannan JM, Brannan GD. Qualitative Study. [Updated 2022 Sep 18]. In: StatPearls [Internet]. Treasure Island (FL): StatPearls Publishing; 2024 Jan-.
In this Page
Bulk download.
- Bulk download StatPearls data from FTP
Related information
- PMC PubMed Central citations
- PubMed Links to PubMed
Similar articles in PubMed
- Suicidal Ideation. [StatPearls. 2024] Suicidal Ideation. Harmer B, Lee S, Duong TVH, Saadabadi A. StatPearls. 2024 Jan
- Folic acid supplementation and malaria susceptibility and severity among people taking antifolate antimalarial drugs in endemic areas. [Cochrane Database Syst Rev. 2022] Folic acid supplementation and malaria susceptibility and severity among people taking antifolate antimalarial drugs in endemic areas. Crider K, Williams J, Qi YP, Gutman J, Yeung L, Mai C, Finkelstain J, Mehta S, Pons-Duran C, Menéndez C, et al. Cochrane Database Syst Rev. 2022 Feb 1; 2(2022). Epub 2022 Feb 1.
- Macromolecular crowding: chemistry and physics meet biology (Ascona, Switzerland, 10-14 June 2012). [Phys Biol. 2013] Macromolecular crowding: chemistry and physics meet biology (Ascona, Switzerland, 10-14 June 2012). Foffi G, Pastore A, Piazza F, Temussi PA. Phys Biol. 2013 Aug; 10(4):040301. Epub 2013 Aug 2.
- Review Evidence Brief: The Effectiveness Of Mandatory Computer-Based Trainings On Government Ethics, Workplace Harassment, Or Privacy And Information Security-Related Topics [ 2014] Review Evidence Brief: The Effectiveness Of Mandatory Computer-Based Trainings On Government Ethics, Workplace Harassment, Or Privacy And Information Security-Related Topics Peterson K, McCleery E. 2014 May
- Review Public sector reforms and their impact on the level of corruption: A systematic review. [Campbell Syst Rev. 2021] Review Public sector reforms and their impact on the level of corruption: A systematic review. Mugellini G, Della Bella S, Colagrossi M, Isenring GL, Killias M. Campbell Syst Rev. 2021 Jun; 17(2):e1173. Epub 2021 May 24.
Recent Activity
- Qualitative Study - StatPearls Qualitative Study - StatPearls
Your browsing activity is empty.
Activity recording is turned off.
Turn recording back on
Connect with NLM
National Library of Medicine 8600 Rockville Pike Bethesda, MD 20894
Web Policies FOIA HHS Vulnerability Disclosure
Help Accessibility Careers
Prestigious cancer research institute has retracted 7 studies amid controversy over errors
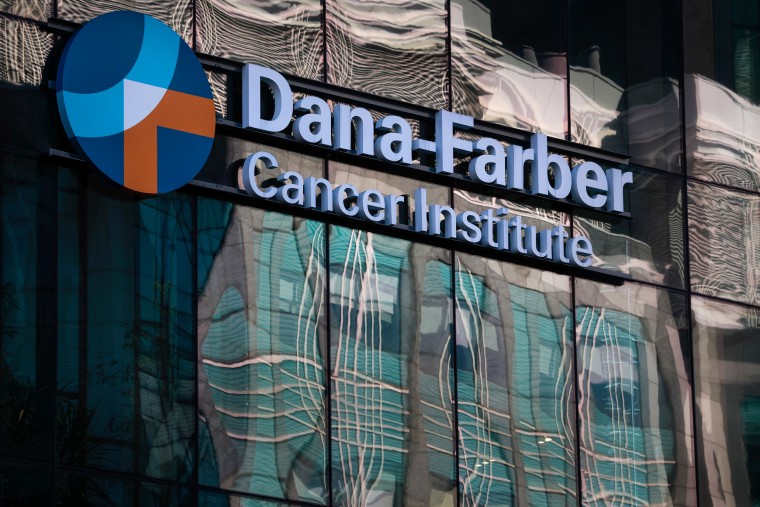
Seven studies from researchers at the prestigious Dana-Farber Cancer Institute have been retracted over the last two months after a scientist blogger alleged that images used in them had been manipulated or duplicated.
The retractions are the latest development in a monthslong controversy around research at the Boston-based institute, which is a teaching affiliate of Harvard Medical School.
The issue came to light after Sholto David, a microbiologist and volunteer science sleuth based in Wales, published a scathing post on his blog in January, alleging errors and manipulations of images across dozens of papers produced primarily by Dana-Farber researchers . The institute acknowledged errors and subsequently announced that it had requested six studies to be retracted and asked for corrections in 31 more papers. Dana-Farber also said, however, that a review process for errors had been underway before David’s post.
Now, at least one more study has been retracted than Dana-Farber initially indicated, and David said he has discovered an additional 30 studies from authors affiliated with the institute that he believes contain errors or image manipulations and therefore deserve scrutiny.
The episode has imperiled the reputation of a major cancer research institute and raised questions about one high-profile researcher there, Kenneth Anderson, who is a senior author on six of the seven retracted studies.
Anderson is a professor of medicine at Harvard Medical School and the director of the Jerome Lipper Multiple Myeloma Center at Dana-Farber. He did not respond to multiple emails or voicemails requesting comment.
The retractions and new allegations add to a larger, ongoing debate in science about how to protect scientific integrity and reduce the incentives that could lead to misconduct or unintentional mistakes in research.
The Dana-Farber Cancer Institute has moved relatively swiftly to seek retractions and corrections.
“Dana-Farber is deeply committed to a culture of accountability and integrity, and as an academic research and clinical care organization we also prioritize transparency,” Dr. Barrett Rollins, the institute’s integrity research officer, said in a statement. “However, we are bound by federal regulations that apply to all academic medical centers funded by the National Institutes of Health among other federal agencies. Therefore, we cannot share details of internal review processes and will not comment on personnel issues.”
The retracted studies were originally published in two journals: One in the Journal of Immunology and six in Cancer Research. Six of the seven focused on multiple myeloma, a form of cancer that develops in plasma cells. Retraction notices indicate that Anderson agreed to the retractions of the papers he authored.
Elisabeth Bik, a microbiologist and longtime image sleuth, reviewed several of the papers’ retraction statements and scientific images for NBC News and said the errors were serious.
“The ones I’m looking at all have duplicated elements in the photos, where the photo itself has been manipulated,” she said, adding that these elements were “signs of misconduct.”
Dr. John Chute, who directs the division of hematology and cellular therapy at Cedars-Sinai Medical Center and has contributed to studies about multiple myeloma, said the papers were produced by pioneers in the field, including Anderson.
“These are people I admire and respect,” he said. “Those were all high-impact papers, meaning they’re highly read and highly cited. By definition, they have had a broad impact on the field.”
Chute said he did not know the authors personally but had followed their work for a long time.
“Those investigators are some of the leading people in the field of myeloma research and they have paved the way in terms of understanding our biology of the disease,” he said. “The papers they publish lead to all kinds of additional work in that direction. People follow those leads and industry pays attention to that stuff and drug development follows.”
The retractions offer additional evidence for what some science sleuths have been saying for years: The more you look for errors or image manipulation, the more you might find, even at the top levels of science.
Scientific images in papers are typically used to present evidence of an experiment’s results. Commonly, they show cells or mice; other types of images show key findings like western blots — a laboratory method that identifies proteins — or bands of separated DNA molecules in gels.
Science sleuths sometimes examine these images for irregular patterns that could indicate errors, duplications or manipulations. Some artificial intelligence companies are training computers to spot these kinds of problems, as well.
Duplicated images could be a sign of sloppy lab work or data practices. Manipulated images — in which a researcher has modified an image heavily with photo editing tools — could indicate that images have been exaggerated, enhanced or altered in an unethical way that could change how other scientists interpret a study’s findings or scientific meaning.
Top scientists at big research institutions often run sprawling laboratories with lots of junior scientists. Critics of science research and publishing systems allege that a lack of opportunities for young scientists, limited oversight and pressure to publish splashy papers that can advance careers could incentivize misconduct.
These critics, along with many science sleuths, allege that errors or sloppiness are too common , that research organizations and authors often ignore concerns when they’re identified, and that the path from complaint to correction is sluggish.
“When you look at the amount of retractions and poor peer review in research today, the question is, what has happened to the quality standards we used to think existed in research?” said Nick Steneck, an emeritus professor at the University of Michigan and an expert on science integrity.
David told NBC News that he had shared some, but not all, of his concerns about additional image issues with Dana-Farber. He added that he had not identified any problems in four of the seven studies that have been retracted.
“It’s good they’ve picked up stuff that wasn’t in the list,” he said.
NBC News requested an updated tally of retractions and corrections, but Ellen Berlin, a spokeswoman for Dana-Farber, declined to provide a new list. She said that the numbers could shift and that the institute did not have control over the form, format or timing of corrections.
“Any tally we give you today might be different tomorrow and will likely be different a week from now or a month from now,” Berlin said. “The point of sharing numbers with the public weeks ago was to make clear to the public that Dana-Farber had taken swift and decisive action with regard to the articles for which a Dana-Farber faculty member was primary author.”
She added that Dana-Farber was encouraging journals to correct the scientific record as promptly as possible.
Bik said it was unusual to see a highly regarded U.S. institution have multiple papers retracted.
“I don’t think I’ve seen many of those,” she said. “In this case, there was a lot of public attention to it and it seems like they’re responding very quickly. It’s unusual, but how it should be.”
Evan Bush is a science reporter for NBC News. He can be reached at [email protected].
- Skip to main content
- Keyboard shortcuts for audio player
White-sounding names get called back for jobs more than Black ones, a new study finds
Joe Hernandez

A sign seeking job applicants is seen in the window of a restaurant in Miami, Florida, on May 5, 2023. Joe Raedle/Getty Images hide caption
A sign seeking job applicants is seen in the window of a restaurant in Miami, Florida, on May 5, 2023.
Twenty years ago, two economists responded to a slew of help-wanted ads in Boston and Chicago newspapers using a set of fictitious names to test for racial bias in the job market.
The watershed study found that applicants with names suggesting they were white got 50% more callbacks from employers than those whose names indicated they were Black.
Researchers at the University of California, Berkeley and the University of Chicago recently took that premise and expanded on it, filing 83,000 fake job applications for 11,000 entry-level positions at a variety of Fortune 500 companies.
Their working paper , published this month and titled "A Discrimination Report Card," found that the typical employer called back the presumably white applicants around 9% more than Black ones. That number rose to roughly 24% for the worst offenders.
The research team initially conducted its experiment in 2021, but their new paper names the 97 companies they included in the study and assigns them grades representing their level of bias, thanks to a new methodology the researchers developed.
"Putting the names out there in the public domain is to move away from a lot of the performative allyship that you see with these companies, saying, 'Oh, we value inclusivity and diversity,'" said Pat Kline, a University of California, Berkeley economics professor who worked on the study. "We're trying to create kind of an objective ground truth here."

America Reckons With Racial Injustice
From jobs to homeownership, protests put spotlight on racial economic divide.
The names that researchers tested include some used in the 2004 study as well as others culled from a database of speeding tickets in North Carolina. A name was classified as "racially distinctive" if more than 90% of people with that name shared the same race.
Applicants with names such as Brad and Greg were up against Darnell and Lamar. Amanda and Kristen competed for jobs with Ebony and Latoya.
What the researchers found was that some firms called back Black applicants considerably less, while race played little to no factor in the hiring processes at other firms.
Dorianne St Fleur, a career coach and workplace consultant, said she wasn't surprised by the findings showing fewer callbacks for presumed Black applicants at some companies.
"I know the study focused on entry-level positions. Unfortunately it doesn't stop there. I've seen it throughout the organization all the way up into the C-suite," she said.
St Fleur, who primarily coaches women of color, said many of her clients have the right credentials and experience for certain jobs but aren't being hired.
"They are sending out dozens, hundreds of resumes and receiving nothing back," she said.
What the researchers found
Much of a company's bias in hiring could be explained by its industry, the study found. Auto dealers and retailers of car parts were the least likely to call back Black applicants, with Genuine Auto Parts (which distributes NAPA products) and the used car retailer AutoNation scoring the worst on the study's "discrimination report card."
"We are always evaluating our practices to ensure inclusivity and break down barriers, and we will continue to do so," Heather Ross, vice president of strategic communications at Genuine Parts Company, said in an email.
AutoNation did not reply to a request for comment.
The companies that performed best in the analysis included Charter/Spectrum, Dr. Pepper, Kroger and Avis-Budget.

Workplace Diversity Goes Far Past Hiring. How Leaders Can Support Employees Of Color
Several patterns emerged when the researchers looked at the companies that had the lowest "contact gap" between white and Black applicants
Federal contractors and more profitable companies called back applicants from the two racial groups at more similar rates. Firms with more centralized human resources departments and policies also exhibited less racial bias, which Kline says may indicate that a standardized hiring workflow involving multiple employees could help reduce discrimination.
When it came to the sex of applicants, most companies didn't discriminate when calling back job-seekers.
Still, some firms preferred one sex over another in screening applicants. Manufacturing companies called back people with male names at higher rates, and clothing stores showing a bias toward female applicants.
What can workplaces — and workers — do
Kline said the research team hoped the public would focus as much on companies doing a bad job as those doing a good one, since they have potentially found ways to remove or limit racial bias from the hiring process.
"Even if it's true, from these insights in psychology and behavioral economics, that individuals are inevitably going to carry biases along with them, it's not automatic that those individual biases will translate into organizational biases, on average," he said.
St Fleur said there are several strategies companies can use to cut down on bias in the hiring process, including training staff and involving multiple recruiters in callback decisions.
Companies should also collect data about which candidates make it through the hiring process and consider standardizing or anonymizing that process, she added.
St Fleur also said she often tells her job-seeking clients that it's not their fault that they aren't getting called back for open positions they believe they're qualified for.
"The fact that you're not getting callbacks does not mean you suck, you're not a good worker, you don't deserve this thing," she said. "It's just the nature of the systemic forces at play, and this is what we have to deal with."
Still, she said job candidates facing bias in the hiring process can lean on their network for new opportunities, prioritize inclusive companies when applying for work and even consider switching industries or locations.

IMAGES
VIDEO
COMMENTS
A research design is a strategy for answering your research question using empirical data. Creating a research design means making decisions about: Your overall research objectives and approach. Whether you'll rely on primary research or secondary research. Your sampling methods or criteria for selecting subjects. Your data collection methods.
The study design used to answer a particular research question depends on the nature of the question and the availability of resources. In this article, which is the first part of a series on "study designs," we provide an overview of research study designs and their classification. The subsequent articles will focus on individual designs.
Created: June 15, 2016; Last Update: September 8, 2016; Next update: 2020. There are various types of scientific studies such as experiments and comparative analyses, observational studies, surveys, or interviews. The choice of study type will mainly depend on the research question being asked. When making decisions, patients and doctors need ...
INTRODUCTION. Scientific research is usually initiated by posing evidenced-based research questions which are then explicitly restated as hypotheses.1,2 The hypotheses provide directions to guide the study, solutions, explanations, and expected results.3,4 Both research questions and hypotheses are essentially formulated based on conventional theories and real-world processes, which allow the ...
Abstractspiepr Abs1. Every day people do research as they gather information to learn about something of interest. In the scientific world, however, research means something different than simply gathering information. Scientific research is characterized by its careful planning and observing, by its relentless efforts to understand and explain ...
About Research Methods. This guide provides an overview of research methods, how to choose and use them, and supports and resources at UC Berkeley. As Patten and Newhart note in the book Understanding Research Methods, "Research methods are the building blocks of the scientific enterprise. They are the "how" for building systematic knowledge.
Hereafter, I define them research styles and I briefly describe the main ones. Different styles can co-exist in a given research area and even in the same research. In particular, they are all present and relevant in Informatics. Formal research is a research that studies a formal system. This is typically the case of a large part of research ...
Breaking science news and articles on global warming, extrasolar planets, stem cells, bird flu, autism, nanotechnology, dinosaurs, evolution -- the latest discoveries ...
An overview of the REF was presented and how expert panel assessors are selected, appointed and undertake their work. Questions were posed on various scenarios, and the importance of reach and significance for each was explored. This sets the scene for Chap. 2, which will deal with the research impact case study.
The theoretical framework is the structure that can hold or support a theory of a research study. The theoretical framework encompasses not just the theory, but the narrative explanation about how the researcher engages in using the theory and its underlying assumptions to investigate the research problem. It is the structure of your paper that ...
Known as an audit study, the experiment was the largest of its kind in the United States: The researchers sent 80,000 résumés to 10,000 jobs from 2019 to 2021.The results demonstrate how ...
1. Focus on your objectives and research questions. The methodology section should clearly show why your methods suit your objectives and convince the reader that you chose the best possible approach to answering your problem statement and research questions. 2.
The objective and research questions will often integrate the terms "lived experience" verbatim. For example, the main objective of the exemplar research study is to explore PICU nurses' (whose) lived experience of environmental and quality improvement changes (what) in the context of a major hospital transformation project (context). As ...
In order to assess whether the results of a study are reliable, you first have to find out why the study was done in the first place and which questions it tried to answer. This may sound trivial, but it is crucial if you want to determine whether the study can actually provide any answers to the original research question. For instance, many ...
The study wasn't designed to answer questions about why these cancer types seemed to have the strongest ties to accelerated aging, but Ruiyi Tian, the graduate student who led the research, has ...
ROCHESTER, Minn. — A Mayo Clinic study shows stem cells derived from patients' own fat are safe and may improve sensation and movement after traumatic spinal cord injuries.The findings from the phase 1 clinical trial appear in Nature Communications.The results of this early research offer insights on the potential of cell therapy for people living with spinal cord injuries and paralysis for ...
SAN DIEGO - Accelerated aging was more common in recent birth cohorts and was associated with increased incidence of early-onset solid tumors, according to research presented at the American Association for Cancer Research (AACR) Annual Meeting 2024, held April 5-10. "Multiple cancer types are becoming increasingly common among younger adults in the United States and globally," said ...
A 2022 study by NOAA and NASA scientists and additional NOAA research in 2023 suggests that more than 85% of the increase from 2006 to 2021 was due to increased microbial emissions generated by livestock, agriculture, human and agricultural waste, wetlands and other aquatic sources. The rest of the increase was attributed to increased fossil ...
The study design was unique due to the size of the population captured in the Swedish Medical Birth Register and the Swedish Prescribed Drug Register. Before siblings were considered, there appeared to be a small increase in risk for neurodevelopmental disorders in children exposed to acetaminophen, which was noted in previous studies.
Research is a rigorous problem-solving process whose ultimate goal is the discovery of new knowledge. Research may include the description of a new phenomenon, definition of a new relationship, development of a new model, or application of an existing principle or procedure to a new context. Research is systematic, logical, empirical, reductive, replicable and transmittable, and generalizable.
Access 160+ million publications and connect with 25+ million researchers. Join for free and gain visibility by uploading your research.
The study will include a comparison sample of 100 additional families at the WIN shelter who will only receive $600 per year without specialized peer support. Both groups will get the usual care ...
Pew Research Center conducted this analysis to explore partisan identification among U.S. registered voters across major demographic groups and how voters' partisan affiliation has shifted over time. It also explores the changing composition of voters overall and the partisan coalitions. ... Yet as this study shows, the long-term shifts in ...
Step 4: Create a research design. The research design is a practical framework for answering your research questions. It involves making decisions about the type of data you need, the methods you'll use to collect and analyze it, and the location and timescale of your research. There are often many possible paths you can take to answering ...
The study was funded by the Stanford Maternal and Child Health Research Institute's Uytengsu-Hamilton 22q11 Neuropsychiatry Research Program, FONDEYCT (the National Fund for Scientific and Technological Development of the government of Chile), ANID-Chile (the Chilean National Agency for Research and Development) and the U.S. National ...
Qualitative research is a type of research that explores and provides deeper insights into real-world problems.[1] Instead of collecting numerical data points or intervene or introduce treatments just like in quantitative research, qualitative research helps generate hypotheses as well as further investigate and understand quantitative data.
The retracted studies were originally published in two journals: One in the Journal of Immunology and six in Cancer Research. Six of the seven focused on multiple myeloma, a form of cancer that ...
The research team initially conducted its experiment in 2021, but their new paper names the 97 companies they included in the study and assigns them grades representing their level of bias, thanks ...