- DOI: 10.1007/978-3-030-04960-7_2
- Corpus ID: 150238778

Research on Cyberbullying: Strengths and Limitations
- Published in Narratives in Research and… 2019
- Narratives in Research and Interventions on Cyberbullying among Young People
27 Citations
Defining cyberbullying: a multifaceted definition based on the perspectives of emerging adults.
- Highly Influenced
Friendship Quality and Gender Differences in Association With Cyberbullying Involvement and Psychological Well-Being
Moral disengagement and cyberbullying involvement: a systematic review, a comprehensive investigation of cyberbullying and cyber victimization among secondary school students, cyberbullying characteristics and prevention—what can we learn from narratives provided by adolescents and their teachers, prevalence of bullying in grassroots soccer in spain: victims, bullies, and bystanders, the psychosocial impacts of cybervictimisation and barriers to seeking social support: young people’s perspectives, governing the risks of cyber bullying in the workplace during the era of covid-19, young people's conceptualizations of the nature of cyberbullying: a systematic review and synthesis of qualitative research, personality traits and aggression as explanatory variables of cyberbullying in spanish preadolescents, 70 references, cyberbullying: an overrated phenomenon, scientific research on bullying and cyberbullying: where have we been and where are we going, issues of cross-cultural variations in cyber bullying across europe and beyond, systematic review of theoretical studies on bullying and cyberbullying: facts, knowledge, prevention, and intervention, cyberbullying: neither an epidemic nor a rarity, victims’ perceptions of traditional and cyberbullying, and the psychosocial correlates of their victimisation, following you home from school: a critical review and synthesis of research on cyberbullying victimization, bullying in the digital age: a critical review and meta-analysis of cyberbullying research among youth., cyberbullying: another main type of bullying, cyberbullying: a storm in a teacup, related papers.
Showing 1 through 3 of 0 Related Papers
Click through the PLOS taxonomy to find articles in your field.
For more information about PLOS Subject Areas, click here .
Loading metrics
Open Access
Peer-reviewed
Research Article
Positive youth development attributes and cyberbullying victimization among Chinese middle school students: A longitudinal moderated mediation model involving internet gaming disorder and depression
Contributed equally to this work with: Xiong Gan, Pinyi Wang
Roles Conceptualization, Data curation, Writing – review & editing
* E-mail: [email protected]
Affiliation Department of Psychology, College of Education and Sports Sciences, Yangtze University, Jingzhou, China

Roles Formal analysis, Writing – original draft, Writing – review & editing
Roles Data curation, Writing – review & editing
Roles Writing – original draft, Writing – review & editing
Affiliation Department of Psychology, College of Education and Sports Sciences, Yangtze University College of Technology and Engineering, Jingzhou, China
- Xiong Gan,
- Pinyi Wang,
- Guoxing Xiang,
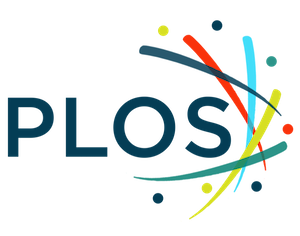
- Published: June 30, 2023
- https://doi.org/10.1371/journal.pone.0287729
- Reader Comments
As an extension of traditional bullying, cyberbullying emerges with the increasing popularity of the internet, and seriously affects the health of students. However, fewer studies have explored the potential influencing mechanisms of cyberbullying victimization from a positive psychology perspective. Therefore, based on the positive youth development theory, this study will explore the potential mediator and moderator in the relationship between positive youth development (PYD) attributes and cyberbullying victimization through a longitudinal design. 719 students ( M age = 15.95 years, SD = 0.76, 45.2% boy) participated in the study and completed self-report questionnaires on relevant variables. The result found that students’ level of PYD significantly and negatively predicted the level of cyberbullying victimization. Meanwhile, SEM analysis showed that PYD influenced individuals’ cyberbullying victimization by affecting their internet gaming disorder (IGD), while depression levels moderated the relationship between PYD and IGD. This study examines cyberbullying victimization from a positive psychology orientation, with potential prevention and intervention value.
Citation: Gan X, Wang P, Xiang G, Jin X (2023) Positive youth development attributes and cyberbullying victimization among Chinese middle school students: A longitudinal moderated mediation model involving internet gaming disorder and depression. PLoS ONE 18(6): e0287729. https://doi.org/10.1371/journal.pone.0287729
Editor: Gianpiero Greco, University of Study of Bari Aldo Moro, ITALY
Received: February 19, 2023; Accepted: June 11, 2023; Published: June 30, 2023
Copyright: © 2023 Gan et al. This is an open access article distributed under the terms of the Creative Commons Attribution License , which permits unrestricted use, distribution, and reproduction in any medium, provided the original author and source are credited.
Data Availability: All relevant data are within the manuscript and its Supporting Information files.
Funding: This research was supported by Youth project of Science and Technology Research Plan of Department of Education of Hubei Province in 2020 (Q20201306), the Social Science Fund Project of Yangtze University in 2022 (2022csz03), the Faculty Scientific Fund Project of the College of Education and Sports Sciences of Yangtze University in 2022 (2022JTB01), the key projects of education science plan of Hubei Province in 2022: Study on the influencing factors and intervention mechanism of non-suicidal self-injurious behaviors in adolescents (2022GA030), and the Major Project for Philosophy and Social Science Research of Hubei Province (2022). The funders had no role in study design, data collection and analysis, decision to publish, or preparation of the manuscript.
Competing interests: The authors have declared that no competing interests exist.
1. Introduction
According to the China Internet Network Information Center’s 50th Statistical Report, as of June 2022, the number of internet users in China had reached 1.051 billion, with 13.5% of internet users between the ages of 10 and 19 [ 1 ]. With the rising popularity of the internet, the issue of cyberbullying among adolescents is becoming an extremely incisive social problem [ 2 ]. Although the bullying problem in mainland China has improved in recent years [ 3 ], it is still a hot issue of widespread concern among relevant researchers due to its inherent complexity and its serious impact on the physical and mental health of youth. For example, it has been shown that psychological distress (e.g., anxiety) predicts adolescent victimization [ 4 ], but bullying experiences have also been shown to influence adolescent psychological distress, which in turn plays an important role in non-suicidal self-injury [ 5 ]. As an extension of traditional bullying behavior on the internet, cyberbullying is defined as an intentional, aggressive, and repetitive behavior perpetrated by a more powerful individual against someone more vulnerable through the use of technology such as the internet, social media, and cellular phones [ 6 ]. Because of the medium of the internet, cyberbullying is more spreadable than traditional bullying [ 7 , 8 ]. Relevant studies show that cyber deviance [ 9 ], especially cyberbullying [ 10 ], is very common in the social context of mainland China as well as Taiwan and Hong Kong. As a result, this behavior can cause immeasurable harm to the victims. Many studies have confirmed the negative impact of cyberbullying behavior on adolescents [ 6 ], especially on the victims [ 7 , 11 ]. For example, Chen used structural equation modeling analysis through a cross-societal probability sample survey to demonstrate a significant direct association between cyberbullying victimization and psychological distress [ 12 ]. In addition, cyberbullying victimization has been shown to significantly influence adolescents’ emotions [ 7 , 13 ] and academic performance [ 14 ]. Therefore, the increased attention to cyberbullying victimization contributes to the healthy physical and mental development of students. However, previous researchers have mostly explored the negative effects of cyberbullying victimization and have paid less attention to its influencing factors and underlying influencing mechanisms. In addition, researchers have focused more on cyberbullying victimization as a problem behavior and explored how to manage it, and less on how to create a positive environment to have a long-lasting impact on it. Therefore, in order to better understand adolescent cyberbullying victimization and provide prevention and intervention value, this study intends to investigate the effects of positive youth development attributes on adolescent cyberbullying victimization and the potential mediator (i.e. internet gaming disorder) and moderator (i.e. depression) involved.
Because cyberbullying behavior uses the internet as a medium and has only been gaining attention in recent years [ 15 , 16 ], research on this behavior is not yet as rich as that on offline bullying (e.g., school bullying). In addition, with the emerging trend of positive psychology, research on the factors influencing cyberbullying victimization can shift from previous risk factors (e.g., low socioeconomic status, introverted personality traits) [ 8 ] to protective factors (e.g., positive youth development attributes). According to D’Urso et al., being bullied is part of a broader, complex system of adolescent psychological and social development [ 17 ]. However, previous research on bullying does not usually consider such a wide range of relevant factors and rarely places bullying in a specific context. Positive youth development theory [ 18 ] indicates that by providing adolescents with some external developmental resources and enhancing adolescents’ own internal resources, adolescents can develop well and achieve effective prevention or reduction of problem behaviors. Taking a positive youth development perspective on youth cyberbullying victimization not only places the behavior in a broader context, but also emphasizes the potential for youth development rather than the "possible" deficits, marking a fundamental change in the understanding of the nature of youth development. Based on revealing the influencing factors of adolescent cyberbullying victimization, the exploration of its mediating and moderating variables will also help us better understand the influence pathways and boundary conditions of positive youth development attributes and provide new perspectives for prevention and intervention measures.
1.1. PYD attributes and cyberbullying victimization
Positive youth development (PYD) is an optimal development that strives for full, healthy, and successful development [ 14 ], which focuses on the strengths and potentials of youth. According to positive youth development theory [ 18 ], by providing youth with some external developmental resources (e.g., family support, community resources, etc.) and improving youth’s internal resources (e.g., self-efficacy, future beliefs, psychological resilience, etc.), youth can achieve positive development and achieve effective prevention or reduction of problem behaviors due to less exposure to risky environments [ 19 , 20 ]. For example, researchers have found significant direct effects of parental rejection on school victimization and school bullying through large samples [ 21 ]. School engagement has also been shown to be a significant negative predictor of victimization in school and cyberspace [ 22 ]. In addition, a good relationship with parents has been shown to be a protective factor against bullying [ 23 ]. Therefore, it is consistent with the comprehension theoretical model of problem behavior (i.e., family, peers, school, and community all have protective and risk factors that can have a direct impact on problem behavior) [ 24 ], for individuals with lower levels of PYD, problem behavior is relatively high due to the lack of contextual resources.
Previous researchers have illustrated the relationship between PYD attributes and school bullying. For example, Hui et al. [ 25 ] used the positive youth development paradigm to explore prevention programs for school bullying. In addition, D’Urso et al. [ 17 ] explored the dynamic relationship between PYD attributes and bullying through a longitudinal study design and found that PYD attributes had a significant predictive effect on subsequent bullying victimization. However, research on PYD attributes and cyberbullying victimization needs to be deepened. As one of the branches of bullying, cyberbullying and school bullying have very high similarity and commonality [ 26 ]. Thus, combining positive youth development theory and the comprehension theoretical model of problem behavior, PYD may be a significant predictor of cyberbullying victimization.
1.2. IGD as a mediator
Internet gaming disorder (IGD) is defined as an individual’s uncontrollable, excessive and compulsive play of online games that results in impaired physical, psychological and social functioning [ 27 ]. IGD is one of the serious adverse outcomes that occur in the younger generation [ 28 ]. According to recent studies, Chinese adolescents have such a high prevalence of IGD, ranging from 2.97% to 13% [ 29 , 30 ]. As consequence, IGD in adolescents has become a major concern in the relevant academic fields nowadays. On the one hand, Researchers showed that IGD causes a variety of negative effects in adolescents, including anxiety, insomnia, poor academic performance, and so on [ 31 – 34 ]. Adolescents with higher levels of IGD spend more time in online environments and are more likely to suffer from adverse environmental repercussions. A strong relationship between internet addiction and increased cyberbullying has been shown [ 35 ]. On the other hand, the positive youth development theory [ 18 ] focuses on the strengths and potentials of youth, nurturing youth as a resource. It has been shown that adolescents with different PYD assets have different levels and types of problem behaviors, such as IGD [ 36 , 37 ]. Shek demonstrates that PYD intervention is a proactive intervention strategy to address adolescent IGD [ 38 ]. According to self-determination theory [ 39 ], individuals with higher levels of PYD have higher levels of internal resources and refined internal motivation to better identify the advantages and disadvantages of networks [ 40 ]. Therefore, students with higher levels of PYD attributes can also clearly identify the negative effects of IGD as a way to regulate their internet use. For example, Yu and Shek, using Hong Kong students as their participants, found that specific PYD attributes were important protective factors for internet disorder [ 41 ]. Dou and Shek’s study not only confirmed the predictive effect of PYD attributes on internet addiction at the cross-sectional level, but also demonstrated significant effects of PYD at the longitudinal level [ 40 ].
According to developmental contextualism [ 42 ], human development is achieved through the continuous interaction between an individual and the context in which they are placed. Students with IGD spend more time in the internet than those without this disorder [ 43 ]. As a result, they may have more opportunities to be victimized by cyberbullying. Under this model, PYD not only directly influences cyberbullying victimization but may also influence cyberbullying victimization in conjunction with the internet context. And research has shown that PYD interventions influence youth bullying victimization by improving the context [ 16 , 25 ]. Therefore, we believe that PYD attributes may have an impact on cyberbullying victimization by influencing IGD.
1.3. Depression as a moderator
Although PYD attributes may affect IGD, it does not influence adolescents equally. It should be noted that adolescent development is the result of a combination of multiple factors, not only environmental factors, but also individual factors, and even the interaction of two factors. In other words, the influence of environmental factors on adolescent development is different for different individuals [ 42 ]. Depression is defined as a negative emotional experience resulting from an individual’s feeling of inability to cope with external stressors [ 44 ]. Depression as a negative emotion has become a major risk factor affecting the physical and mental health of adolescents due to its huge impact on the individual’s physiology and psychology [ 44 ]. Previous studies have shown that individuals’ negative emotions are influenced not only by their acquired environment but also by genetic factors and show relatively stable individual differences [ 45 ]. The differential susceptibility model states that some children are more sensitive to environmental factors and susceptible to adverse or favorable environmental factors due to temperament or genetics [ 46 ]. It has been shown that depression can act as a moderating variable in the influence of environmental factors on individual behavior or cognition [ 47 , 48 ].
IGD is usually the result of individuals using external means for self-regulation and experimenting with negative emotions [ 44 ]. Adolescents with higher levels of depression are more likely to seek external resources and experiences through online games because they have difficulty getting satisfaction in real life [ 49 , 50 ]. It has been demonstrated that depressive symptoms are a risk factor for IGD [ 44 , 49 – 51 ]. For example, Burleigh et al. showed that individuals with higher levels of depressed mood were more likely to exhibit IGD as a result of the type of game [ 44 ]. According to the comprehension theoretical model of problem behavior [ 24 ], IGD is the result of a dynamic interaction between risk and protective factors. Thus, depression may be a risk factor that increases the likelihood of adolescents developing problem behaviors when they are in specific developmental contexts. However, different researchers have held different views on the mechanisms by which depression affects individual behavior. Some researchers have suggested that "highly sensitive" individuals are both more likely to be overwhelmed by adverse circumstances and more likely to benefit from positive ones [ 45 ]. Other researchers have argued that for individuals with high negative emotions, simply improving their surroundings is not enough to modify their problem behavior because of their own high levels of negative emotions [ 48 ]. As middle school students are in an important period of physical and mental development, their psychological development is still at a semi-mature and semi-naive stage, and their psychological self-adjustment and recovery abilities are much lower than those of adults [ 52 ]. Therefore, we believe that individuals with high levels of depressed mood may still exhibit higher levels of IGD even if they possess high levels of PYD attributes. To our knowledge, the moderation effect of depression on the association between PYD and IGD needs to be deepened. Therefore, it is crucial to investigate how depression interacts with PYD in the formation of IGD in individuals.
1.4. The present study
To sum up, fewer studies have explored the potential influential mechanisms of cyberbullying victimization from a positive psychology perspective. Therefore, we aimed to examine the influence of PYD attributes, IGD, and depression on cyberbullying victimization and its internal mechanism in the same model, based on the above mentioned literature review and related theories. The hypothesis of the association mechanism between PYD attributes and cyberbullying victimization is as follows and the whole proposed model is depicted in Fig 1 :
- H1: PYD attributes can negatively predict cyberbullying victimization during middle school students;
- H2: IGD will mediate the relationship between PYD attributes and cyberbullying victimization;
- H3: Depression will moderate the pathway from PYD attributes to IGD.
- PPT PowerPoint slide
- PNG larger image
- TIFF original image
https://doi.org/10.1371/journal.pone.0287729.g001
Taken together, IGD and depression are expected to shed light on the relationship between PYD attributes and cyberbullying victimization. Identifying the influencing factors from cyberbullying victimization and the intrinsic dynamic influencing mechanisms have crucial implications for theory and prevention, providing new ideas for improving the physical and mental health of adolescents.
2.1. Participants and procedure
We recruited all grade 10 and grade 11 students as participants from a public school through clustering convenience sampling in Hubei Province, China. Following the voluntary principle, a total of 864 questionnaires were sent out, and 802 were successfully received, and the effective recovery was 92.8%. Due to voluntary withdrawal and transfer, 802 valid questionnaires were collected for Wave 1 (Nov. 2020), 746 valid questionnaires were collected for Wave 2 (May. 2021), but only 719 valid questionnaires remained for Wave 3 (Nov. 2021). The 719 (45.2% boy) valid middle school students tracked were between 14 and 18 years old ( M age = 15.95 years, SD = 0.76), and of these, 267 (37.1%) were grade 10 students and 452 (62.9%) were grade 11 students. Using G*Power 3.1.9.7 to calculate the sample size required for this study, it was found that 134–1289 samples were required to detect a small or medium effect size. Combined with related studies [ 53 ], our final sample size meets the requirements and can provide at least 95% statistical test power (1- β ). Independent sample t -tests were used to compare the differences in the primary variables between the effective follow-up sample and the attrition sample at wave 1. The results showed that there were no significant differences between the two groups of participants in terms of PYD, IGD, and cyberbullying victimization levels ( p > 0.05). However, the depression scores of the attrition sample were higher than those of the tracking sample ( t = -2.10, p < 0.05).
The study was approved by the Research Ethics Committee of the Psychology, College of Education and Sports Sciences, Yangtze University (No. YZU20201014). A group test was conducted in the classroom, with graduate students trained in professional psychology acting as the conductor. Prior to conducting the test, the purpose of the study was explained to teachers and students, and the principle of data confidentiality was emphasized. All participants were told that they can quit at any time if they feel uncomfortable and were required to complete all items independently throughout the process. With the informed consent of students and their parents or legal guardians involved, the questionnaire survey was conducted. The questionnaire of the students who participated voluntarily was collected after they finished, while the remainder were not required to. We require students to complete the questionnaire within 20 minutes and thank them for their cooperation after completion.
2.2. Measures
2.2.1 positive youth development..
At Wave 1, The Chinese Positive Youth Development Scale (CPYDS) developed by Shek et al. [ 54 ] was used, which contains 90 items. All items were rated on a 6-point Likert-type scale ranging from 1 (completely disagree) to 6 (completely agree). For example, "When I need help, I trust my parents to help me." The average score of all items is calculated (after reverse-coding when necessary), with higher scores indicating higher levels of PYD attributes. This scale has been widely used and has demonstrated good reliability and validity in China [ 55 , 56 ]. In the present study, Cronbach’s alphas coefficient was 0.974.
2.2.2 Depression.
At Wave 1, depression was measured with The Center for Epidemiological Studies Depression Scale (CES-D). This scale was compiled by Radloff [ 57 ] and used to measure depressive mood in the general population. In 1999, scholars translated the Chinese version of the CES-D [ 58 ], which is widely used to measure the level of depression in Chinese adolescents. The scale contains 20 items and all items were rated on a 4-point scale ranging from 0 (occasionally or not) to 3 (most of the time or continuously). For example, “I feel depressed”. Item scores were averaged to create a composite of depression, with higher scores indicating higher levels of depression. Studies have shown that this scale has good reliability and validity among Chinese adolescents [ 59 , 60 ]. In the current study, the scale’s Cronbach’s alphas coefficient was 0.765.
2.2.3 Internet gaming disorder.
At Wave 2, The questionnaire of Internet Gaming Disorder (IGD) compiled by Gentile [ 61 ] and revised by Yu et al. [ 31 ] was used, which contains 11 items. All items were rated on a 3-point Likert-type scale ranging from 1 (never) to 3 (often) to assess the frequency of each symptom in the last six months of the participant’s life. For example, “Do you need to spend more time on online games to get satisfaction?” The average score of all items is calculated, with higher scores indicating the higher tendency of IGD. This questionnaire has demonstrated good reliability and validity in samples of Chinese adolescents [ 62 ]. In the present study, the Cronbach’s alphas coefficient was 0.890.
2.2.4 Cyberbullying victimization.
At Wave 3, cyberbullying victimization was measured with 6 items complied by Lam and Li [ 15 ]. All items were rated on a 7-point scale ranging from 0 (never) to 6 (more than or equal to 6 times). The average score of all items was calculated, with higher scores indicating a higher frequency of suffering from cyberbullying. For example, “How many times did someone say mean things about you using emails, texting, short messages, on a website such as Renren, etc.?” Studies have shown that this questionnaire has good reliability and validity among Chinese adolescents [ 7 , 13 ]. The questionnaire’s Cronbach’s alphas coefficient was 0.924 in this study.
2.3. Statistical analysis
Prior research has found that adolescents’ gender and grade are related to cyberbullying [ 15 , 40 , 51 , 63 ]. Thus, we controlled for these demographic variables in our statistical analyses. The regression estimation method was used to fill in missing data. Because the data were collected through self-reporting, we conducted the Harman single factor method to test the common method biases. For descriptive statistics and correlation analysis, SPSS 26.0 was utilized. We used the SPSS macro PROCESS to examine mediation effect and moderating effect [ 64 ]. We used bootstrapping with 5000 iterations to test the statistical significance of the mediation effect. The interaction product was created by multiplying the predictor and the moderator. When an interaction effect was significant, simple slope analyses (1 SD above and below the mean of the moderator) were conducted [ 65 ].
3.1. Common method bias analysis
Some approaches, such as reverse scoring and anonymous filling, were used to control the process to decrease the influence of the common method bias. Harman single factor test was used to inspect this bias [ 66 ]. The results reveal that the eigenvalues of 23 factors are more than 1, and the variance explained by the first factor is 25.73%, which is much less than the critical value of 40%, indicating that there is no significant common method bias in this study.
3.2. Descriptive statistics and correlation analyses
Means, standard deviations, and correlations are displayed in Table 1 . As regard to covariates, boys had higher tendency of IGD ( r = -0.28, p < .001) and frequency of suffering from cyberbullying ( r = -0.09, p < .05) than girls. Students in higher grade had higher PYD level ( r = 0.11 p < .01) and lower depression level ( r = -0.10, p < .01) than lower grade. PYD are negatively correlated with cyberbullying victimization ( r = -0.16, p < .001) and IGD ( r = -0.24, p < .001), meaning that students who have higher levels of PYD attributes were less likely to suffer from cyberbullying behavior and develop IGD behavior. IGD is positively correlated with cyberbullying victimization ( r = 0.16, p < .001), indicating that students with IGD are more likely to suffer from cyberbullying.
https://doi.org/10.1371/journal.pone.0287729.t001
3.3. Mediating effects of internet gaming disorder
Model 4 of the SPSS macro program PROCESS was used to test the mediating effect of IGD. The results are shown in Table 2 . After controlling for gender and grade, PYD attributes is a significant negative predictor of cyberbullying victimization ( β = -0.18, p < .001), and H1 1 is proved. When both PYD and IGD enter the regression equation, PYD can significantly negatively predict cyberbullying victimization ( β = -0.15, p < .001), and significantly negatively predict IGD ( β = -0.14, p < .001). IGD can significantly positively predict cyberbullying victimization ( β = 0.20, p < .01). The bias correction Bootstrap method test shows that IGD has a significant mediating effect between PYD attributes and cyberbullying victimization, ab = -0.03, 95% CI [-0.063, -0.001], proving H2. As shown in Fig 2 , the mediation effect accounted for 16.67% of the total effect of PYD attributes on cyberbullying victimization.
All covariates were held constant during this analysis but are not presented for reasons of simplicity.
https://doi.org/10.1371/journal.pone.0287729.g002
https://doi.org/10.1371/journal.pone.0287729.t002
3.4. Moderating effects of depression
Model 7 of the SPSS macro program PROCESS was used to test the moderating effect of depression. The results are shown in Table 3 , PYD significantly negatively predicted IGD ( β = -0.12, p < .001), and the interaction term between PYD and depression significantly positively predicted IGD ( β = 0.10, p < .05), meaning that depression moderated the relationship between PYD attributes and IGD, proving H3. Follow-up analyses (presented in Fig 3 ) tested simple slopes of PYD at lower (1 SD below mean) and higher (1 SD above mean) levels of depression to understand the nature of the interaction. For students with a high level (-1 SD ) of depression, PYD attributes was associated with higher IGD. For students with a low level (+1 SD ) of depression, the relationship between PYD attributes and IGD was relatively stronger. Thus, higher depression seemed as a risk factor while lower depression was a protective factor in the association between PYD attributes and IGD.
https://doi.org/10.1371/journal.pone.0287729.g003
https://doi.org/10.1371/journal.pone.0287729.t003
4. Discussion
This study used a longitudinal research design to explore the factors influencing cyberbullying victimization and its potential internal mechanisms from a positive psychology perspective. The findings suggest that PYD attributes is an important factor in explaining cyberbullying victimization among middle school students, and that it influences cyberbullying victimization directly or indirectly through IGD. Moreover, the relationship between PYD attributes and IGD was also moderated by the level of individual depression.
The results of the descriptive statistical analysis revealed that the IGD levels and cyberbullying victimization levels were significantly higher in boys than in girls, which has similarities with the results of previous studies [ 67 , 68 ]. This may be because boys’ brains are more sensitive to stimuli that combine light, sound and moving images, and therefore more likely to get unique experiences from online games [ 67 ]. For cyberbullying victimization, on the one hand, boys are more likely to be victims of cyberbullying because they have higher levels of IGD and have more access to the internet. On the other hand, it may be because girls are socially recognized as a protected group and thus are also less likely to be victims of bullying [ 69 ]. In addition, the results of the study showed that students in higher grades had higher levels of PYD attributes and lower levels of depression, which is similar to previous studies, but also inconsistent [ 70 , 71 ]. Such results may be related to the small range of subjects we selected, and the fact that the PYD attributes of the students may have changed due to the influence of the entrance examination.
The findings demonstrate that the higher the level of PYD, the lower the adolescents’ cyberbullying victimization level, which is in accordance with related findings [ 16 , 17 , 25 ] and also makes extension to it. Conformity positive youth development theory [ 18 ] and the comprehension theoretical model of problem behavior [ 24 ], this study consider cyberbullying victimization in a more comprehensive system. In this sense, individuals with higher levels of PYD attributes achieve positive individual development and reduce problem behaviors due to the availability of sufficient external and internal resources. The development assets theory similarly states that problem behaviors may occur due to a lack of developmental assets, such as positive psychological resources [ 72 ]. Longitudinal findings better illustrate that cyberbullying victimization can be reduced in subsequent online environments by enhancing students’ levels of PYD attributes. These finding improved our understanding of cyberbullying victimization and lay the foundation for more research into the mediating roles of IGD and moderating roles of depression in the connection between PYD attributes and cyberbullying victimization.
Our findings suggest that PYD is a negative predictor of IGD, which in turn increases the likelihood that students will be victims of cyberbullying. This finding also suggests that IGD may be an important explanatory mechanism for the link between PYD attributes and cyberbullying victimization. The mediating effect of IGD is consistent with the results of previous studies [ 16 , 25 ]. In addition, this study builds on previous research and deepens our understanding of the mechanisms influencing of IGD. According to developmental contextualism [ 42 ], PYD not only directly influences cyberbullying victimization but may also influence cyberbullying victimization in conjunction with the internet context. Of these, the level of PYD attributes reflects both the external resources for individual growth and can demonstrate internal motivation for adolescent development. For individuals with low levels of PYD attributes, internet games provide a range of external resources [ 62 ]. At the same time, adolescents with IGD spend a disproportionate amount of time in the internet environment. Thus individuals’ PYD traits increase the likelihood of cyberbullying victimization through the internet environment they are in. However, it is worth noting that IGD plays a partial mediating role in the relationship between PYD and cyberbullying victimization, indicating that the influence of PYD on cyberbullying victimization may be mediated by other factors, and extensive research is needed.
As expected, depression moderated the pathway from PYD to IGD. To be specific, individuals with higher levels depression were more likely to develop IGD than those with lower levels of depression. That is, depression is a risk factor for IGD. However, this result is not entirely consistent with previous studies [ 73 – 75 ]. The researchers concluded that it was internet gaming or even problematic internet gaming behavior that significantly predicted an individual’s psychological quality of life [ 76 ], distress (e.g., depression) [ 77 ]. However, there are other researchers who support the reverse path. They suggest that psychological distress (e.g., depression) is an antecedent of problematic use of internet-related activities [ 78 ] and can significantly predict problematic gaming [ 79 ]. Chang et al. demonstrated that internet gaming may be a coping strategy for individuals with schizophrenia through their study of participants diagnosed with schizophrenia [ 80 ]. Kakul and Javed, based on clinical model, also showed that individuals with high IGD levels may subscribe to a unique set of maladaptive beliefs that underlie persistent and excessive engagement in internet gaming activities. Therefore, we believe that students with higher levels of depression are more inclined to experience negative emotions and fulfill desires that are difficult to satisfy in real life through the internet environment [ 59 , 60 ]. So reducing students’ depression levels is of great importance to fully utilize PYD resources. At the same time, we should also note that although individuals with higher levels of depression have higher levels of IGD at the same level of PYD, their PYD attributes are relatively more weakly associated with IGD. That is, the favorable impact of the PYD attributes shrinks significantly when individual risk is high. According to this result, improving PYD attributes most benefited individuals with lower levels of depression, while lowering depression levels most benefited individuals with higher levels of PYD. In addition, future research needs to explore additional risk factors and protective factors to better leverage the strengths of adolescents and reduce cyberbullying victimization.
5. Contributions and limitations
There are some important contributions of this study. First, this study examines the impact of PYD attributes on cyberbullying victimization from a positive psychology perspective, and the underlying mechanisms. This finding clarifies the importance of PYD attributes in students’ deviant behaviors, rather than continuing to understand their behavioral changes in terms of managing problem behaviors. At the same time, exploring adolescent cyberbullying victimization from the perspective of intrinsic mechanisms rather than impact effects not only provides empirical support for positive youth development theory, but also offers new perspectives on the prevention and intervention of adolescent problem behaviors. Therefore, in the subsequent educational process, we should focus on creating an environment conducive to the positive development of students and enhancing their own abilities so that they can be free from cyberbullying victimization. Second, the mediating role of IGD suggests that intervention programs can focus on reducing students’ time spent online and further improving mental health. In addition, the moderating effect of depression suggests that by reducing depression levels in adolescents, students can reduce the occurrence of IGD in specific settings, thereby reducing cyberbullying victimization. Although PYD attributes are protective factors for IGD, the effect of this protective factor is significantly diminished when individuals have high levels of depression. Therefore, for reducing individual depression levels can significantly improve the IGD levels of individuals with high PYD.
Despite the above-mentioned contributions, some limitations of this study should be mentioned. First, this study examined the dynamic relationships between variables using a longitudinal research design, but little attention was paid to the interactions between variables. It is expected that future studies will examine the interactions between variables and use more advanced statistical methods (e.g., cross-lagged panel models) for analysis. Second, we used the self-reporting method to collect data, which may be affected by social desirability. Future research should use multiple methods and multiple informants to increase the validity of the findings. Third, this study found that the relationship between PYD attributes and cyberbullying victimization was partially mediated, suggesting that a further understanding of this relationship requires information on other mediators. Fourth, research has identified the complex nature of the bullying experience, in that some bullies are also victims. Whether cyberbullying shares this characteristic and the mechanisms underlying such group behavior is also something we need to explore in the future. Finally, the current study was conducted with a sample of Chinese middle school students, and again, the measurement instrument has only been shown to have good reliability in the Chinese cultural context (e.g., the questionnaire of Internet Gaming Disorder revised by Yu et al. [ 31 ]). Future studies should include cross-cultural samples of adolescents, using international scales with good psychometric properties (e.g., the nine-item Internet Gaming Disorder Scale-Short Form [ 81 – 84 ]), in order to test the generalizability of our findings.
6. Conclusion
This study explores the mediating role of IGD and moderating role of depression in PYD attributes and cyberbullying victimization, and draws the following conclusions: PYD attributes could negatively predict cyberbullying victimization; The relationship between PYD attributes and cyberbullying victimization is mediated by IGD; The relationship between PYD attributes and cyberbullying victimization is moderated by depression.
Supporting information
S1 dataset. dataset used for analyses in present study..
https://doi.org/10.1371/journal.pone.0287729.s001
Acknowledgments
The authors thank all the leaders and teachers of the two schools who helped and coordinated this testing process, as well as the adolescent students who volunteered to participate in this study.
- View Article
- Google Scholar
- PubMed/NCBI
- 58. Wang XD, Wang XL, Ma H. Handbook of mental health rating scale (Updated version). Beijing: Chinese Mental Health Journal; 1999. https://dx.doi.org/10.1177/014662167700100306 .
- 64. Hayes AF. Introduction to mediation, moderation, and conditional process analysis: A regression-based approach. New York: The Guiford Press; 2013. https://dx.doi.org/10.1111/jedm.12050 .
- SpringerLink
- SpringerNature APIs
- SpringerMaterials
Citation Details

Research on Cyberbullying: Strengths and Limitations
Narratives in Research and Interventions on Cyberbullying among Young People, 2019
ISBN : 978-3-030-04959-1
22 Items cite this Chapter
(cyber)bullying in schools – when bullying stretches across con/fflating spaces.
Tabea Bork-Hüffer, Belinda Mahlknecht and Katja Kaufmann
Journal: Children's Geographies , 2021 , Volume 19, Number 2 , Page 241
DOI: 10.1080/14733285.2020.1784850
Prevalence, Inequalities, and Impact of Bullying in Adolescence: Insights from the #BeeWell Study
Emma Thornton, Margarita Panayiotou and Neil Humphrey
Journal: International Journal of Bullying Prevention , 2024
DOI: 10.1007/s42380-024-00244-7
Psychosocial Difficulties and Help-Seeking Behaviors in Singapore Adolescents Involved in Cyberbullying
Say How Ong, Yi Ren Tan, Jerrine Z.N. Khong, John M. Elliott, Andre Sourander and Daniel S.S. Fung
Journal: Cyberpsychology, Behavior, and Social Networking , 2021 , Volume 24, Number 11 , Page 737
DOI: 10.1089/cyber.2020.0630
Workplace cyberbullying and creativity: examining the roles of psychological distress and psychological capital
Masood Nawaz Kalyar, Munazza Saeed, Aydin Usta and Imran Shafique
Journal: Management Research Review , 2021 , Volume 44, Number 4 , Page 607
DOI: 10.1108/MRR-03-2020-0130
Examining the Factors Affecting Parental Supervision in Cyberbullying Prevention: Demographics, Parental Mediation, and Digital Parenting Awareness
Aykut Durak, Hatice Yildiz Durak, Mustafa Saritepeci and Bülent Dilmaç
Journal: Families in Society: The Journal of Contemporary Social Services , 2024
DOI: 10.1177/10443894231225793
A comprehensive investigation of cyberbullying and cyber victimization among secondary school students
Hasan Güllü, Engin Karahan and Ahmet Oğuz Akçay
Journal: Education and Information Technologies , 2023 , Volume 28, Number 10 , Page 12633
DOI: 10.1007/s10639-023-11687-8
Prevalence of Bullying in Grassroots Soccer in Spain: Victims, Bullies, and Bystanders
Xènia Ríos, Carles Ventura, Teresa Lleixà, Maria Prat and Gonzalo Flores
Journal: Physical Culture and Sport. Studies and Research , 2022 , Volume 94, Number 1 , Page 11
DOI: 10.2478/pcssr-2022-0002
Handbook of Research on Bullying in Media and Beyond
Aftab Hossain
Series: Advances in Social Networking and Online Communities , Year: 2022 , Page 175
DOI: 10.4018/978-1-6684-5426-8.ch011
Sustainable Smart Cities and Territories
Niloufar Shoeibi, Nastaran Shoeibi, Vicente Julian, Sascha Ossowski, Angelica González Arrieta and Pablo Chamoso
Series: Lecture Notes in Networks and Systems , Year: 2022 , Volume 253 , Page 289
DOI: 10.1007/978-3-030-78901-5_25
The severity of bullying messages predicts increased levels of targets’ depression and general anxiety as a function of targets’ inferences of a bully’s goals
V. Skye Wingate and Nicholas A. Palomares
Journal: Journal of Social and Personal Relationships , 2021 , Volume 38, Number 4 , Page 1194
DOI: 10.1177/0265407520983439
citation rank
Citations per year.
2019 | 2024 |
citing journals
- Frontiers in Psychology 2 (%)
- Child Indicators Research 1 (%)
- Children's Geographies 1 (%)
- Cyberpsychology, Behavior, and Social N ... 1 (%)
- Education and Information Technologies 1 (%)
- Erdkunde 1 (%)
- European Journal of Developmental Psych ... 1 (%)
- Families in Society: The Journal of Con ... 1 (%)
- International Journal of Bullying Preve ... 1 (%)
- Journal of Business Ethics 1 (%)
- Journal of Social and Personal Relation ... 1 (%)
- Jurnal Studi Komunikasi (Indonesian Jou ... 1 (%)
- Management Research Review 1 (%)
- Physical Culture and Sport. Studies and ... 1 (%)
- Psichologija 1 (%)
- Research Papers in Education 1 (%)
- Sex Roles 1 (%)
citing books
- Asian Perspectives on Workplace Bullyin ... 1 (%)
- Handbook of Research on Bullying in Med ... 1 (%)
- Sustainable Smart Cities and Territorie ... 1 (%)
- The Wiley Blackwell Handbook of Bullyin ... 1 (%)

© 2024 Springer. Part of Springer Nature . Manage Cookies
Warning: The NCBI web site requires JavaScript to function. more...

An official website of the United States government
The .gov means it's official. Federal government websites often end in .gov or .mil. Before sharing sensitive information, make sure you're on a federal government site.
The site is secure. The https:// ensures that you are connecting to the official website and that any information you provide is encrypted and transmitted securely.
- Publications
- Account settings
- Browse Titles
NCBI Bookshelf. A service of the National Library of Medicine, National Institutes of Health.
Committee on the Biological and Psychosocial Effects of Peer Victimization: Lessons for Bullying Prevention; Board on Children, Youth, and Families; Committee on Law and Justice; Division of Behavioral and Social Sciences and Education; Health and Medicine Division; National Academies of Sciences, Engineering, and Medicine; Rivara F, Le Menestrel S, editors. Preventing Bullying Through Science, Policy, and Practice. Washington (DC): National Academies Press (US); 2016 Sep 14.

Preventing Bullying Through Science, Policy, and Practice.
- Hardcopy Version at National Academies Press
2 The Scope of the Problem
Although attention to bullying has increased markedly among researchers, policy makers, and the media since the late 1990s, bullying and cyberbullying research is underdeveloped and uneven. Despite a growing literature on bullying in the United States, a reliable estimate for the number of children who are bullied in the United States today still eludes the field ( Kowalski et al., 2012 ; Olweus, 2013 ). Estimates of bullying prevalence vary greatly, and there is little consensus on the value and accuracy of existing estimates.
This chapter describes the current state of research focused on estimating rates of bullying and cyberbullying in the United States and based on the findings from four major, federally funded, nationally representative samples. The committee considers overall trends in these prevalence estimates, as well as areas of inconsistencies and potential reasons for these discrepancies across the particular studies. The committee also draws upon other large-scale studies to provide insight into various demographic factors—such as gender, age, and ethnicity—as potential risk or protective factors for youth involvement in bullying. Although perceptions and interpretations of communications may be different in digital communities, the committee decided to address cyberbullying within a shared bullying framework rather than treating cyberbullying and traditional bullying as separate entities because there are shared risk factors, shared negative consequences, and interventions that work on both cyberbullying and traditional bullying. However, there are differences between these behaviors that have been noted in previous research, such as different power differentials, different perceptions of communication, and questions of how best to approach the issue of repetition in an online context. These differences suggest that although the Centers for Disease Control and Prevention (CDC) definition, developed in the context of traditional bullying, may not apply in a blanket fashion to cyberbullying, these two forms are not separate species. This chapter offers insights into the complexities and limitations of current estimates and underscores the challenges faced by policy makers, practitioners, advocates, and researchers. 1 Although exact estimates are challenging to identify and require more comprehensive measurement of bullying that addresses the current prevalence research limitations, it is clear that a sizable portion of youth is exposed to bullying.
Perspectives from the Field
“[Bullying is] emotionally, or mentally, or physically putting down someone and it happens everywhere, it never stops.”
—Young adult in a focus group discussing bullying (See Appendix B for additional highlights from interviews.)
- NATIONALLY REPRESENTATIVE STUDIES OF BULLYING IN THE UNITED STATES
Several national surveys provide insight into the prevalence of bullying and cyberbullying in the United States. In this section, the committee focuses specifically on the School Crime Supplement (SCS) of the National Crime Victimization Survey (NCVS), the National School-Based Youth Risk Behavior Survey (YRBS), the Health Behaviour in School-Aged Children (HBSC) survey, and the National Survey of Children's Exposure to Violence (NatSCEV) because their samples of youth are nationally representative and epidemiologically defined. The committee notes that there are a number of methodological differences in the samples and measurement across the four studies. The prevalence of bullying behavior at school ranged from 17.9 percent to 30.9 percent, whereas the prevalence of cyberbullying ranged from 6.9 percent to 14.8 percent of youth ( Centers for Disease Control and Prevention, 2014b ; Finkelhor et al., 2015 ; Iannotti, 2013 ; U.S. Department of Education, 2015 ; see Table 2-1 for a summary of these nationally representative surveys and Appendix C for detailed results from these surveys). The discussion below considers in greater detail the strengths and weaknesses of the methods employed by each of these surveys, in an effort to elucidate factors that may contribute to the variation in reported prevalence rates.
Comparison of Current National Data Sources on Bullying for School-Aged Children and Adolescents.
School Crime Supplement of the National Crime Victimization Survey
The SCS is a national survey of 4,942 students ages 12 through 18 in U.S. public and private elementary, middle, and high schools as well as home-schooled youth ( U.S. Department of Education, 2015 ). Created as a supplement to the NCVS and co-designed by the Department of Education, National Center for Education Statistics, and Bureau of Justice Statistics, the SCS survey collects information about victimization, crime, and safety at school ( U.S. Department of Education, 2015 ). The survey was designed to assist policy makers as well as academic researchers and practitioners at the federal, state, and local levels so they can make informed decisions concerning crime in schools. NCVS crime data come from surveys administered by field representatives to a representative sample of households in the United States throughout the year in person and over the phone ( U.S. Department of Education, 2015 ). 2 In 2015, the SCS administration tested two different ways of asking about bullying to better align with the CDC definition of bullying.
The SCS asked students a number of key questions about their experiences with and perceptions of crime and violence that occurred inside their school, on school grounds, on a school bus, or on the way to or from school. 3 Additional questions not included in the NCVS were added to the SCS, such as students' self-reports of being bullied and perceived rejection at school. This survey's approach to bullying and cyberbullying is far more intensive than the other national surveys; however, it is limited by its focus exclusively on reports of being bullied (being a target of bullying behavior), with no information on perpetration. Additional information is also available regarding differences in rates of being bullied and cyberbullied by student characteristics such as gender, race and ethnicity, school and grade level, school enrollment, geographic region, eligibility for reduced-price lunch, household income, and student-teacher ratio. Other characteristics of the events assessed include whether or not an adult was notified of the bullying incident, injury, frequency of bullying, form of bullying, and location of the bullying ( U.S. Department of Education, 2015 ). The SCS data showed that in 2013, 21.5 percent of students ages 12-18 were bullied on school property and 6.9 percent of students were cyberbullied anywhere ( U.S. Department of Education, 2015 ; see Appendix C , Tables C-1 through C-3 ). 4
Although the SCS provides the most recent and in-depth assessment of bullying and cyberbullying prevalence in the United States, it has several major limitations. The questions about being bullied or cyberbullied are only included in the SCS, a supplement to the NCVS; therefore, its sample size is only a fraction of that of the larger NCVS. 5 The SCS and NCVS data, similar to the other national datasets, are voluntary self-report surveys. These surveys focused on students ages 12-18 and on their experience being bullied; data are not available from younger children and from children who have bullied others or children who have witnessed bullying instances. The survey also fails to address rates of bullying among various subpopulations of youth, such as groups differentiated by their sexual orientation or gender identity, by weight status, or by religious minorities.
School-Based Youth Risk Behavior Survey
The YRBS is one component of the Youth Risk Behavior Surveillance System (YRBSS), an epidemiological surveillance system developed by the CDC to monitor the prevalence of youth behaviors that most influence health ( Centers for Disease Control and Prevention, 2014b ). The YRBS is conducted biennially and focuses on priority health-risk behavior established during youth (grades 9-12) that result in the most significant mortality, morbidity, disability, and social problems during both youth and adulthood. 6 State and local education and health agencies are permitted to supplement the national survey to meet their individual needs.
National YRBS
Bullying and cyberbullying estimates include responses by student characteristics, such as gender, race and ethnicity, grade level, and urbanicity of the school. 7 , 8 The data showed that 19.6 percent of children ages 14-18 were bullied on school property and 14.8 percent of children ages 14-18 were electronically bullied ( Centers for Disease Control and Prevention, 2014b ; see Appendix C , Table C-4 ). The data captured by the national YRBS reflect self-report surveys from students enrolled in grades 9-12 at public or private schools. As with the other nationally representative samples, it does not identify many subpopulations that are at increased risk for bullying such as lesbian, gay, bisexual, and transgender (LGBT) youth and overweight children. The YRBS gathers information from adolescents approximately ages 14-17; but it offers no nationally representative information on younger children ( Centers for Disease Control and Prevention, 2014b ). The survey gathers information on Hispanic, black, and white students but does not identify other races and ethnicities.
State and Local YRBS
The YRBSS is the only surveillance system designed to monitor a wide range of priority health risk behavior among representative samples of high school students at the state and local levels as well as the national level ( Centers for Disease Control and Prevention, 2014b ). 9 There is a smaller sample of middle school youth that is included in various state YRBS results, but national-level estimates are not available. The 2014 CDC report includes state- and local-level surveys conducted by 42 states and 21 large urban school districts. Of the 42 states that conducted their own YRBS survey, 26 asked questions about bullying and cyberbullying. 10 The state-specific results for bullying prevalence ranged from a high of 26.3 percent in Montana to a low of 15.7 percent in Florida ( Centers for Disease Control and Prevention, 2014b ). Whereas this state-level high is relatively similar to the prevalence of 19.6 percent reported by the national YRBS, the state-level low is less than a third of the national prevalence. For cyberbullying, the state results ranged from a high of 20.6 percent in Maine to a low of 11.9 percent in Mississippi. The national YRBS cyberbullying prevalence of 14.8 percent is about in the middle of these extremes ( Centers for Disease Control and Prevention, 2014b ).
At this time, the available state and local data are highly variable due to major limitations caused by self-reports, variable definitions of bullying, and the limited age range of students, making it difficult to gauge differences in bullying prevalence among states and in comparison to national estimates.
The Health Behaviour in School-Aged Children Survey
The HBSC survey is an international study that generally addresses youth well-being, health behavior, and their social context ( Iannotti, 2013 ). This research is conducted in collaboration with the World Health Organization Regional Office for Europe, and the survey is administered every 4 years in 43 countries and regions across Europe and North America. The HBSC survey collects data on a wide range of health behaviors, health indicators, and factors that may influence them. These factors are primarily characteristics of the children themselves, such as their psychological attributes and personal circumstances, and characteristics of their perceived social environment, including their family relationships, peer-group associations, school climate, and perceived socioeconomic status ( Iannotti, 2013 ).
The most recent survey focused solely on the United States was conducted in the 2009-2010 school year. The 2009-2010 HBSC survey included questions about nutrition; physical activity; violence; bullying; relationships with family and friends; perceptions of school as a supportive environment; and use of alcohol, tobacco, marijuana, and other drugs ( Iannotti, 2013 ). 11 , 12 Regarding bullying and cyberbullying, the HBSC asked questions only about the frequency with which children were bullied in the “past couple of months,” with follow-up questions about the frequency of a certain type of bullying a student experienced (called names or teased, left out of things, kicked or pushed, etc.). The survey found that 30.9 percent of children ages 10-16 were bullied at school and 14.8 percent of children ages 10-16 were bullied using a computer or e-mail ( Iannotti, 2013 ; see Appendix C , Tables C-6 and C-7 ). 13 The survey is the only nationally representative survey that asked students how often they bullied another student and the type of bullying they carried out. It found that 31.8 percent of students bullied others and 14.0 percent of students cyberbullied other children ( Iannotti, 2013 ). It is the only national survey that asked students to report on the reason they thought they were bullied (e.g., how often were you bullied for your race/color?; how often were you bullied for your religion?). (For additional detail, see Appendix C , Tables C-6 and C-7 ). Nevertheless, like the other surveys reviewed here, the HBSC survey is limited by the nature of self-reported and voluntary data from minors, as well as by its decision to limit questions only to frequency of incidents.
National Survey of Children's Exposure to Violence
The National Survey of Children's Exposure to Violence II (NatSCEV II) was designed to obtain up-to-date incidence and prevalence estimates for a wide range of childhood victimizations ( Finkelhor et al., 2015 ). The first such assessment, the National Survey of Children's Exposure to Violence I (NatSCEV I), was conducted in 2008. This updated assessment, conducted in 2011, asked students to report on 54 forms of offenses against them. The offenses include sexual assault, child maltreatment, conventional crime, Internet victimization, peer and sibling victimization, witnessing victimization, and indirect victimization ( Finkelhor et al., 2015 ). 14 While this survey asked questions regarding bullying-type incidents, many of the questions referred to the offenses as “assault” rather than bullying, which typically includes a wider scope of victimization. It addressed these offenses by age and gender of the child who was bullied. NatSCEV II found that 17.9 percent of children ages 1 month to age 17 had experienced an assault by a nonsibling peer, 1.8 percent of children had experienced a bias assault, and 6.0 percent experienced Internet/cell phone harassment ( Finkelhor et al., 2015 ; see Appendix C , Table C-5 ). It is not clear whether Internet or cell phone harassment meets the CDC definition of bullying.
Trends over Time
Although attention to bullying and cyberbullying has increased, the extent to which rates of bullying have changed in recent years is unclear ( Figures 2-1 and 2-2 ) ( Kowalski et al., 2012 ; Limber, 2014 ). As illustrated in Figure 2-1 , data from the SCS-NCVS indicate a sharp reduction in the percentage of 12-18 year olds who reported being bullied at school—from 27.8 percent to 21.5 percent in just 2 years ( U.S. Department of Education, 2015 ).
Trends in bullying over time as reported by national surveys. NOTES: HBSC = Health Behaviour in School-Aged Children; NatSCEV = National Survey of Children's Exposure to Violence, NCVS = National Crime Victimization Survey; SCS = School Crime Supplement (more...)
Trends in cyberbullying over time as reported by national surveys. NOTES: HBSC = Health Behaviour in School-Aged Children; NatSCEV = National Survey of Children's Exposure to Violence, NCVS = National Crime Victimization Survey; SCS = School Crime Supplement (more...)
While the YRBS and NatSCEV mirror this decline, neither found so large a change ( Finkelhor et al., 2015 ; Centers for Disease Control and Prevention, 2014b ; see Figure 2-1 ). Findings from the HBSC survey show an increase in bullying among 11-, 13-, and 15-year-old youth in the United States of about 1 percentage point between 2006 and 2010 ( Iannotti, 2013 ). As illustrated in Figure 2-2 , the trend in cyberbullying over time is even less clear. According to the SCS-NCVS data, the percentage of students ages 12-18 who were cyberbullied doubled between 2001 and 2007 but declined by 2 percentage points between 2011 and 2013 ( U.S. Department of Education, 2015 ). 15 While the HBSC survey and the YRBS also showed a decline in the percentage of students who have been cyberbullied, the NatSCEV showed an increase in the percentage of students who experienced Internet and/or cell phone harassment (see Figure 2-2 ).
Because the available national trend data are limited in the range of years for which data are available and because findings vary somewhat among the major national samples, it is difficult to gauge the extent to which bullying may have increased or decreased in recent years. Additional data points will be necessary to determine national trends in the prevalence rates for children and youth who are bullied.
- EXISTING ESTIMATES OF BULLYING IN THE UNITED STATES BY SUBPOPULATION
In an effort to understand the nature and extent of bullying in the United States, some studies have examined specific subpopulations or subsets of children involved in bullying incidents. Because the major national surveys that include bullying do not uniformly or fully address the bullying experience of subpopulations of interest, 16 in this section the committee also draws upon findings from meta-analyses and independent large-scale research. Although these studies are limited by inconsistent definitions, survey data based on self-reports, differing age ranges, and a lack of questions seeking responses from children who have bullied or have witnessed bullying incidents, they do provide valuable insight into particular risk factors or protective factors for involvement in bullying, insights that are generally not available from the surveys of nationally representative samples. The committee expands on risk and protective factors in Chapter 3 .
Prevalence of Bullying by Age
A majority of bullying research has shown that children's experiences with bullying vary significantly according to their age. Decreases with age in rates of being bullied were reported in the SCS.
As reported by Limber (2014) , a meta-analysis by Cook and colleagues (2010) found that the likelihood of both being bullied and perpetrating bullying behavior peaked in the early adolescent years (ages 12-14) before decreasing slightly in later adolescence ( Limber, 2014 ). Decreases with increasing grade level in rates of being bullied were also reported in the SCS-NCVS.
For example, whereas 27.8 percent of sixth graders reported being bullied at school in 2013, 23.0 percent of ninth graders and 14.1 percent of twelfth graders said they had been bullied ( U.S. Department of Education, 2015 ; see Figure 2-3 ). Although these data suggest that the overall chances of being bullied are particularly likely in middle childhood, children are more or less likely to be involved in specific forms of bullying at different ages, depending on their verbal, cognitive, and social development ( Limber, 2014 ).
Prevalence of bullying and cyberbullying among students, ages 12-18, by grade level, as reported by the 2013 School Crime Supplement of the National Crime Victimization Survey. SOURCE: Data from U.S. Department of Education (2015).
Reports of being bullied through an electronic context appear to peak later than reports of being bullied by a more traditional context; the SCS, for example, reported a peak for cyberbullying in tenth grade ( U.S. Department of Education, 2015 ). According to a 2015 overview of teen's social media and technology use, the Pew Research Center found that 68 percent of teens ages 13-14 had access to a smartphone and 84 percent had access to a desktop or laptop computer, whereas 76 percent of teens ages 15-17 had access to a smartphone and 90 percent had access to a desktop or laptop computer ( Lenhart et al., 2015 ). Today's youth are often referred to as “digital natives” due to their upbringing immersed in technological tools including smartphones and social media, while adults are often referred to as “digital immigrants.” This report found that approximately three-fourths of teens ages 13-17 reported access to a cell phone and 94 percent of teens reported going online daily, including 24 percent who said they go online “almost constantly” ( Lenhart et al., 2015 ). Owning a mobile phone allows for ongoing access to the Internet, including social media and other communication tools that may foster opportunities for bullying. Approximately one-quarter of teens surveyed described themselves as “constantly connected” to the Internet ( Lenhart et al., 2015 ). Among teens 13-17 years old, most reported using several forms of social media including Facebook, Instagram, Snapchat, and Twitter (see Figure 2-4 ). A previous study found that older adolescents viewed Facebook as a powerful source of influence through four major processes: connection to others, comparison with peers, building an online identity, and an immersive multimedia experience ( Moreno et al., 2013 ).
Facebook, Instagram, and Snapchat top social media platforms for teens (n = 1,060 teens ages, 13-17). SOURCE: Adapted from Lenhart (2015, p. 2)
This increasing access to and use of technologies with age may help explain rising rates of cyberbullying as adolescents age. An older study of 10-17 year olds found an “online harassment” prevalence of approximately 9 percent ( Wolak et al., 2007 ). However, a more recent study, which focused on middle school adolescents, found a lower prevalence of cyberbullying: 5 percent reported being a perpetrator of cyberbullying, and 6.6 percent reported being a target of cyberbullying ( Rice et al., 2015 ).
Smith and colleagues (2008) found rates of cyberbullying to be lower than rates of traditional bullying, but appreciable, and reported higher cyberbullying prevalence outside of school than inside. It is possible that reported cyberbullying rates are lower than traditional bullying rates because much of technology use occurs outside of school and current approaches to measuring bullying are designed mostly to assess rates of traditional bullying in school ( Smith et al., 2008 ). Previous work has suggested that increased Internet use is associated with increased risk for cyberbullying ( Juvonen and Gross, 2008 ).
Although research has suggested that the prevalence of bullying among older adolescents is lower than that of younger adolescents, researchers have proposed that cyberbullying among older students may represent a continuation of behaviors from previous grades but with a focus on technological tools for more subtle bullying techniques ( Cowie et al., 2013 ).
Prevalence of Bullying by Gender
Research has confirmed that there are gender differences in the frequency with which children and youth are involved in bullying. A recent meta-analysis found that although boys and girls experienced relatively similar rates of being bullied, boys were more likely to bully others, or to bully others and be bullied, than girls were ( Cook et al., 2010 ; Limber, 2014 ). Research has suggested that there are gender differences in the frequency with which children and youth are involved in bullying. The SCS, YRBS, and NatSCEV found that rates for self-reports of being bullied range from 19.5 to 22.8 percent for boys and from 12.8 to 23.7 percent for girls ( Centers for Disease Control and Prevention, 2014b ; Finkelhor et al., 2015 ; U.S. Department of Education, 2015 ). All three of these national surveys found that girls were more likely to report being bullied than were boys (see Figure 2-5 for SCS data).
Prevalence of being bullied among 12-18 year olds by gender, as reported by the 2013 School Crime Supplement of the National Crime Victimization Survey. SOURCE: Data from U.S. Department of Education (2015).
Research has suggested similarities and differences, beyond just overall frequency, in how often boys and girls experience different forms of bullying ( Felix and Green, 2010 ). As noted in Chapter 1 , there are two modes of bullying (direct and indirect) as well as different types of bullying (physical, verbal, relational, and damage to property). As illustrated in Figure 2-6 , being made fun of or called names and being the subject of rumors are the two most common forms of bullying experienced by children and youth, and both are much more frequently experienced than physical bullying ( Iannotti, 2013 ; Limber, 2014 ; U.S. Department of Education, 2015 ). For example, the 2013 SCS found that 13.2 percent of youth ages 12-18 reported being the subject of rumors and 13.6 percent said they had been made fun of, called names, or insulted, compared with 6.0 percent who reported being pushed, shoved, tripped, or spit on ( U.S. Department of Education, 2015 ; see Figure 2-6 ). Notions of gendered forms of bullying are common because physical aggression has been regularly associated with boys, whereas relational aggression has been considered to be the domain of girls ( Oppliger, 2013 ). For example, studies have shown that indirect aggression is normative for both genders, while boys are more strongly represented in physical and verbal aggression (see review by Card et. al., 2008). As for differences in different forms of cyberbullying, according to the 2013 SCS, girls experienced a higher prevalence of being bullied in nearly all types, except for receiving unwanted contact while playing online games and facing purposeful exclusion from an online community ( Limber, 2014 ; U.S. Department of Education, 2015 ; see Figure 2-7 ). However, because there is not yet a common definition of cyberbullying, there is no agreement on what forms of online harassment fall under the umbrella term of “cyberbullying.”
Prevalence of different types of bullying among students, ages 12-18, bullied in a school year, as reported by the 2013 School Crime Supplement of the National Crime Victimization Survey. SOURCE: Data from U.S. Department of Education (2015).
Prevalence of different types of cyberbullying among students, ages 12-18, bullied in a school year, as reported by the 2013 School Crime Supplement of the National Crime Victimization Survey. SOURCE: Data from U.S. Department of Education (2015).
Limber and colleagues (2013) observed that age trends for self-reports of bullying others varied for boys and girls. Among boys, bullying others increased from grades 3 through 12, but among girls, rates of bullying others peaked in eighth grade ( Limber et al., 2013 ). Among older adolescents and college students, cyberbullying may be more common than traditional bullying. Prevalence rates of cyberbullying among young adults and college students have been estimated to be around 10-15 percent ( Kraft and Wang, 2010 ; Schenk and Fremouw, 2012 ; Wensley and Campbell, 2012 ).
Prevalence of Bullying by Race and Ethnicity
There has been only limited research on the roles that race and ethnicity may play in bullying ( Larochette et al., 2010 ; Peskin et al., 2006 ; Spriggs et al., 2007 ). 17 Data from the SCS indicate that the percentage of students who reported being bullied at school in 2013 was highest for white students (23.7%) and lowest for Asian students (9.2%), with rates for black students (20.3%) and Hispanic students (19.2%) falling between (see Figure 2-8 ; data from U.S. Department of Education, 2015 ). Data from the national YRBS were highest for white students (21.8%), next highest for Hispanic students (17.8%), and lowest for black students (12.7%) ( Centers for Disease Control and Prevention, 2014b ). The YRBS data did not include any other ethnicities/races.
Prevalence of being bullied and cyberbullied among students, ages 12-18, by race/ethnicity, as reported by the 2013 School Crime Supplement of the National Crime Victimization Survey. SOURCE: Data from U.S. Department of Education (2015).
It is challenging to interpret the percentages of children and youth who are bullied across different racial and ethnic groups, due to the limited information currently available on racial and ethnic differences in definitions of bullying and on whether and how bullying may vary according to the racial/ethnic diversity and density of schools and communities. See Chapter 3 for a discussion of contextual factors, including the school and community contexts, and their modulation of the relations between individual characteristics and prevalence of involvement in and consequences of bullying by race/ethnicity.
- DISPARITIES IN BULLYING PREVALENCE IN THE UNITED STATES AMONG VULNERABLE GROUPS
In addition to exploring standard demographic differences in bullying (i.e., gender, age, race/ethnicity), researchers have identified specific populations that are at increased risk for being bullied. This section reviews the research on groups for which there is consistent epidemiologic evidence of disparities in being the target of bullying, including LGBT youth, overweight/obese youth, and youth with disabilities. The committee also identified groups for which the evidence of increased risk is not currently consistent and which therefore warrant greater research attention ( U.S. Government Accountability Office, 2012 ). In this chapter, we report descriptive data on prevalence rates; see Chapter 3 for a discussion of factors that contribute to these disparities in rates of bullying (e.g., stigma) as well as research evidence on specific forms of bullying (e.g., bias-based bullying) that are more likely to occur among some of the groups covered in this section.
Differences in Bullying by Sexual Orientation and Gender Identity
LGBT youth, youth questioning their sexuality, and youth who do not conform to gender stereotypes frequently face bullying by their peers ( Eisenberg and Aalsma, 2005 ; Espelage et al., 2008 ; Garofalo et al., 1998 ; Rivers, 2001 ; Russell et al., 2014 ). The prevalence of bullying of lesbian, gay, and bisexual (LGB) males and females ranges from 25.6 percent to 43.6 percent ( Berlan et al., 2010 ).
Most research on bullying related to sexual orientation and gender identity comes from nonprobability samples. For example, the 2003 Massachusetts Youth Risk Behavior Survey found that 42.0 percent of sexual-minority youth reported being bullied in the 12 months prior to survey administration ( Hanlon, 2004 ). Similarly, the cross-sectional analysis of the 2001 questionnaire from the Growing Up Today study, a national longitudinal study involving 7,559 youths (ages 14-22) who were children of nurses participating in the Nurses' Health study found that the prevalence of bullying victimization was lowest in heterosexual female respondents (15.9%) and highest in gay male respondents (43.6%) ( Berlan et al., 2010 ). Girls identifying as “mostly heterosexual” and “mostly bisexual” were at increased risk for perpetrating bullying compared to heterosexual girls, while boys identifying as gay were less likely to perpetrate bullying than were heterosexual boys ( Berlan et al., 2010 ).
A growing body of research has aimed to assess the experiences of transgender youth specifically. The existing quantitative research suggests that most transgender youth experience regular bullying and harassment at school ( Grant et al., 2011 ; Kosciw et al., 2012 ; McGuire et al., 2010 ). For instance, in a sample of 5,542 adolescents sampled online, 82 percent of the transgender or gender nonconforming youth reported any bullying experience in the past 12 months, compared to 57 percent among cisgender boys and girls ( Reisner et al., 2015 ). 18
Measures of sexual orientation—including sexual attraction, sexual behavior, and sexual identity—have been recently incorporated into large surveillance systems, such as some state and local versions of the YRBSS, which have provided population-based estimates of bullying among LGB youth. Two of CDC's large surveillance systems—School Health Profiles and the School Health Policies and Practices studies—assess school health policies and practices relevant to LGB students including the prohibition of harassment and bullying ( Centers for Disease Control and Prevention, 2014a ). The results from these sources provide a means to assess sexual-orientation differences in bullying perpetration and victimization among youth by location within the United States ( Centers for Disease Control and Prevention, 2014a ). 19 Recent analyses by Olsen and colleagues (2014) were conducted by creating two datasets: one that combined 2009-2011 YRBS data from 10 states (Connecticut, Delaware, Hawaii, Illinois, Maine, Massachusetts, North Dakota, Rhode Island, Vermont, and Wisconsin) and the other that combined YRBS data from 10 school districts (Boston, Chicago, District of Columbia, Houston, Los Angeles, Milwaukee, New York City, San Diego, San Francisco, and Seattle). Adjusted prevalence rates for being bullied on school property were lowest for both heterosexual boys and girls (18.3% and 19.9%, respectively, based on the state dataset; 11.4% and 11.8%, respectively, based on the district dataset) and highest among gay boys (43.1% and 25.7%, respectively, based on the state and district datasets) and bisexual boys (35.2% and 33.2%, respectively, based on the state and district datasets) ( Olsen et al., 2014 ). Rates of being bullied on school property were intermediate for the lesbian girls (29.5% in the state dataset, and 14.0% in the district dataset) and bisexual girls (35.3% in the state dataset, and 18.8% in the district dataset).
Given the absence of measures of gender identity disaggregated from sex in these large state and local datasets, population-based estimates of the prevalence of bullying among transgender youth are not currently available. However, recent research has conducted cognitive testing to determine the most reliable and valid way of assessing gender identity among both adults ( GenIUSS Group, 2013 ) and youth (e.g., Conron et al., 2008 ). Further, population-based datasets have very recently begun to include measures of gender identity among youth (e.g., the 2013-2014 California Healthy Kids Survey), which will enable researchers to examine gender identity–related disparities in bullying using representative samples of youth.
Using data from the first wave (1994-1995 school year) of the National Longitudinal Study of Adolescent Health, which included 10,587 youth between 13 and 18, Russell and colleagues (2002) examined differences in experiencing, witnessing, and perpetrating violence, depending on the respondent's self-reported category of romantic attraction (same-sex, both-sex, or other-sex), a measure of sexual orientation. Youth who reported same-sex or both-sex attraction were more likely to experience and perpetrate the most dangerous forms of violence (e.g., pulling a gun or knife on someone, shooting or stabbing someone) and to witness violence ( Russell et al., 2002 ). These findings were not disaggregated by sex or gender identity.
Differences in Bullying Among Youth with Disabilities
Much of the existing data suggests that students with disabilities are overrepresented within the bullying dynamic ( McLaughlin et al., 2010 ; Rose, 2015 ; Rose et al., 2010 ), whether as children who have bullied ( Rose et al., 2009 ), children who have been bullied ( Blake et al., 2012 ; Son et al., 2012 ), or children who have both bullied and have been bullied ( Farmer et al., 2012 ). 20 Specifically, national prevalence data suggest that students with disabilities, as a whole, are up to 1.5 times more likely to be bullied than youth without disabilities ( Blake et al., 2012 ); this disproportionate bullying begins in preschool ( Son et al., 2012 ) and continues through adolescence ( Blake et al., 2012 ; Rose, 2015 ).
However, variability exists in reported prevalence rates of involvement for various subgroups of youth with disabilities. For example, Rose and colleagues (2015) conducted a prevalence study of a large sample of youth with and without disabilities in middle and high school ( n = 14,508) and determined that 35.3 percent of students with emotional and behavioral disorders, 33.9 percent of students with autism spectrum disorders, 24.3 percent of students with intellectual disabilities, 20.8 percent of students with another health impairment, and 19.0 percent of students with specific learning disabilities experienced high levels of victimization. In addition, 15.3 percent of youth with emotional and behavioral disorders, 19.4 percent of youth with autism spectrum disorders, 24.1 percent of youth with intellectual disabilities, 16.9 percent of youth with other health impairment, and 14.4 percent of youth with specific learning disabilities perpetrated bullying behavior. These estimates are in contrast to 14.5 percent of youth without disabilities who experienced high rates of being bullied and 13.5 percent who engaged in high rates of perpetration. The authors of this study acknowledge that the study has a number of limitations—mainly self-report, cross-sectional data, and data that were examined at the group level.
This literature on bullying and disabilities has several inconsistencies, which stem from differences in three basic factors: (1) measurement and definition, (2) disability identification, and (3) comparative groups. For instance, separating subclasses of youth with specific typographies of learning disabilities proves difficult, resulting in the general assessment of a combined class of specific learning disabilities ( Rose, 2015 ). This confounding factor leads to conflicting measures of bullying involvement, with some studies suggesting that rates of bullying perpetration are relatively comparable among youth with and without disabilities ( Rose et al., 2015 ), while others found that students with specific learning disabilities were almost six times more likely to engage in bully perpetration than their peers without disabilities ( Twyman et al., 2010 ). These conflicting results suggest further assessment or disaggregation of subgroups of youth with specific learning disabilities may be necessary to better understand bullying involvement among this subpopulation of youth.
Differences in Bullying by Weight Status
Weight status, specifically being overweight or obese, can be a factor in bullying among children and youth ( Puhl and Latner, 2007 ). The CDC defines childhood overweight as a body mass index (BMI) at or above the 85th percentile and below the 95th percentile of a CDC-defined reference population of the same age and sex. It defines childhood obesity as a BMI at or above the 95th percentile of this reference population for the same age and sex ( Centers for Disease Control and Prevention, 2015b ).
In 2012, 31.8 percent of U.S. children and youth 6 to 19 years of age were overweight or obese, using the CDC weight status categories. Eighteen percent of children 6 to 11 and 21 percent of youth 12 to 19 years of age were obese ( Centers for Disease Control and Prevention, 2015a ). Although the 2012 National Health and Nutrition Examination Survey (NHANES) data showed a decrease in obesity rates for children 2 to 5 years of age, the obesity rates for 2-19-year olds between 2003-2004 and 2011-2012 remained unchanged at 31.8 percent ( Ogden et al., 2014 ). Thus, weight-based bullying can affect a substantial number of youth.
In 2007, Puhl and Latner reviewed the growing literature on social marginalization and stigmatization of obesity in children and adolescents, paying attention to the nature and extent of weight bias toward overweight youth and the primary sources of stigma in their lives, including peers. 21 The researchers found that existing studies on weight stigma suggest that experiences with various forms of bullying is a common experience for overweight and obese youth; however, determining specific prevalence rates of bias is difficult because various assessment methods are used across the literature ( Puhl and Latner, 2007 ). For example, Neumark-Sztainer and colleagues (2002) examined the prevalence of weight-based teasing among middle and high school students ( n = 4,746) and found that 63 percent of girls at or above the 95th percentile for BMI and 58 percent of boys at or above the 95th percentile for BMI experienced “weight-based teasing.” However, in a recent longitudinal study of weight-based teasing ( n = 8,210), Griffiths and colleagues (2006) found that 34 percent of girls at or above the 95th percentile for BMI and 36 percent of boys at or above the 95th percentile for BMI reported being victims of “weight-based teasing and various forms of bullying” ( Griffiths et al., 2006 ). Griffiths and colleagues (2006) found that obese boys and girls were more likely to be victims of overt bullying one year later.
Janssen and colleagues (2004) found that among 5,749 children, ages 11-16, girls with a higher BMI were more likely to be targets of bullying behavior than their average-weight peers. They found that the likelihood of these girls being targeted in verbal, physical, and relational bullying incidents only increased as BMI rose. Among boys, however, the researchers found no significant associations between BMI and physical victimization. When they looked at the older portion of the sample, they found that among 15-16-year-old boys and girls, BMI was positively associated with being the perpetrator of bullying behavior compared with BMI among average-weight children ( Puhl and Latner, 2007 ). In this sample of 15 and 16 year olds, girls still faced an increased likelihood of both being bullied and being a perpetrator of bullying ( Puhl and Latner, 2007 ).
In their review of the literature on peer victimization and pediatric obesity, Gray and colleagues (2009) summarized evidence since 1960 on stigmatization, marginalization, and peer victimization of obese children. They concluded that obesity in children and youth places them at risk for harmful physical, emotional, and psychosocial effects of bullying and similar types of peer mistreatment. They also noted that “over time, a cyclical relationship may emerge between obese individuals and victimization such that children who are victimized are less likely to be active, which in turn leads to increased weight gain and a greater likelihood of experiencing weight-based victimization” ( Gray et al., 2009 , p. 722).
In summary, although numerous studies indicate that overweight and obese youth are at increased risk of being bullied, it can be difficult to attribute weight-based bullying to a single physical attribute, given that being overweight or obese often co-exists with other factors (see also the subsection below on “Youth with Intersectional Identities”). Additional research is needed to identify the relative importance of weight as a reason for being bullied or being a perpetrator of bullying among children and youth.
Other Disparity Groups Requiring More Research
Although most research on groups that are at disproportionate risk for bullying has focused on LGBT youth, overweight/obese youth, or youth with disabilities, some recent research has begun to identify other groups that may be at heightened risk. 22 Because this research is in its early stages, the evidence is not yet compelling on whether these groups do experience disparities in perpetrating or being targeted by bullying behavior. Consequently, the committee highlights the following groups as warranting further study to establish their risk status.
Socioeconomic Status
The literature on socioeconomic status and bullying contains conflicting results. Higher socioeconomic status has been associated with higher levels of perpetration ( Barboza et al., 2009 ; Shetgiri et al., 2012 ) but so has lower socioeconomic status ( Christie-Mizell et al., 2011 ; Garner and Hinton, 2010 ; Glew et al., 2005 ; Jansen et al., 2011 , 2012 ; Nordhagen et al., 2005 ; Pereira et al., 2004 ; Schwartz et al., 1997 ). Other studies found that socioeconomic status was not associated with perpetration ( Flouri and Buchanan, 2003 ; Zimmerman et al., 2005 ).
The evidence for an association between socioeconomic status and being bullied is similarly inconsistent. Specifically, some studies found that neither economic deprivation ( Wilson et al., 2012 ), family income ( Garner and Hinton, 2010 ), nor general socioeconomic status ( Magklara et al., 2012 ) predicted greater risk of being targeted by bullying behavior. Other studies found that insufficient parental income ( Lemstra et al., 2012 ) and low social class ( Pereira et al., 2004 ) predicted increased rates of being the target in bullying incidents. These conflicting results may be due in part to different measures and conceptualizations of socioeconomic status. In addition, other environmental or social–ecological factors that are often not included in evaluative models may account for the differences in these findings. For example, Barboza and colleagues (2009) argued that perpetration emerges as a function of social climate deficits, where social supports may mediate perpetration regardless of demographic characteristics, including socioeconomic status. Thus, further research is warranted on the mediating and moderating variables in the association between socioeconomic status and either bullying perpetration or being targeted for bullying. (See Chapter 3 for a more detailed discussion of moderation.)
Immigration Status
The results to date from research on the association between immigration status and bullying involvement are inconsistent. For example, Lim and Hoot (2015) investigated the bullying involvement of third and sixth grade students who were immigrants, refugees, or native born. The majority of these students who were refugees or immigrants came from Burma, Burundi, Iraq, Somalia, Thailand, and Yemen. The refugees and immigrants did not report higher levels of being bullied than the native-born American students. However, qualitative data suggested that youth with refugee status responded as “nonpassive victims,” meaning they would try to defend themselves when physically attacked, whereas immigrants and native-born youth who were bullied responded to bullying more passively. The inconsistencies in the results may be associated with age of the respondents, total sample size, nationality of the immigrants/refugees, or other environmental or social–ecological factors ( Hong et al., 2014 ), all of which require greater attention in future studies.
Minority Religious Affiliations
Few studies have specifically investigated the bullying involvement of youth from minority religious groups. However, evidence from other areas of violence suggests that youth from religious minorities may experience higher rates of being bullied than those who identify as Christians. For instance, the percentage of hate crimes in the United States that are grounded in religious affiliation has increased from 10 percent in 2004 to 28 percent in 2012 ( Wilson, 2014 ). Since schools are reflective of society as a whole, and bullying involvement is grounded in a social–ecological context that includes community and societal factors ( Hong and Espelage, 2012 ), this targeting of religious minorities may carry over into the school environment. However, this hypothesis requires empirical documentation.
Youth with Intersectional Identities
As noted in the earlier discussion of weight status as a factor in bullying, “intersectionality” refers to individuals with multiple stigmatized statuses (e.g., black lesbian youth). The majority of studies on bullying perpetration and targeting have examined identity groups in isolation, but there is increasing acknowledgement that multiple intersecting identities can exacerbate or attenuate health outcomes (e.g., Bowleg, 2008 ; McCall, 2005 ). An exception is the study by Garnett and colleagues (2014) , which analyzed the intersectionality of weight-related bullying with bullying for other reasons. Among 965 Boston youth sampled in the 2006 Boston Youth Survey, participants had been discriminated against or bullied (or assaulted) for any of four attributes (race or ethnicity, immigration status, perceived sexual orientation, and weight). Participants who were bullied for their race and weight had higher rates of being targeted for bullying behavior, compared with students who had two or more of the other characteristics ( Garnett et al., 2014 ). As discussed earlier, the extent to which intersecting identities affect the prevalence of bullying perpetration and targeting remains largely unknown and therefore represents an important area for future study.
Children and adolescents have mostly stated that the differences in their physical appearance contribute to the possibility of their being bullied ( Lunde et al., 2007 ). There is concern that students with characteristics, such as obesity, disabilities, food allergies, and gender issues could put them directly in the path of being more likely to be bullied ( Schuster and Bogart, 2013 ). These categories may intersect at the micro level of individual experience to reflect multiple interlocking systems of privilege and oppression at the macro, social-structural level ( Bowleg, 2012 ).
Is bullying more prevalent in urban schools than in suburban or rural schools? Because large-city urban schools are often located in inner-city areas of concentrated poverty and exposure to violence, theories of social disorganization suggest that bullying might be more common in such contexts ( Bradshaw et al., 2009 ). However, there is not much research in support of this hypothesis. Rural students have self-reported at least as much bullying in their schools as did urban youth ( Dulmus et al., 2004 ; Stockdale et al., 2002 ). Moreover, data from large national studies in the United States indicate that students in rural schools report somewhat more bullying than those in urban and suburban schools ( Nansel et al., 2001 ; Robers et al., 2013 ). In particular Robers and colleagues (2013) found, using 2011 National Center for Education Statistics data, that 25 percent of students in urban schools reported some bullying, compared with 29 percent in suburban schools and 30 percent in rural schools. One reason that has been suggested for this difference is that smaller rural schools, some of which have fewer school transitions (e.g., lacking a separate middle school between elementary and high school grades), may typically consolidate social reputations and provide fewer opportunities for targeted youth to redefine how they are perceived by peers ( Farmer et al., 2011 ).
What may differ by urbanicity of schools are the reasons for targeting certain individuals in a pattern of bullying behavior. For example, Goldweber and colleagues (2013) documented that urban African American youth were more likely to report race-based bullying by peers than were rural or suburban youth. As noted above in the section on “Prevalence of Bullying by Race and Ethnicity,” the connection between experiences of peer bullying and racial discrimination merits further study.
- ISSUES IN DEVELOPING ESTIMATES OF BULLYING IN THE UNITED STATES
Current efforts to estimate prevalence of bullying and cyberbullying behavior are characterized by disagreement and confusion. This chapter has pointed out the major challenges associated with generating accurate and reliable estimates of bullying and cyberbullying rates in the United States. The issues to be addressed are summarized here in terms of definitional issues and issues of measurement and sampling.
Definitional Issues
As attention to bullying behavior has grown in recent years, concerns have been raised that efforts to characterize bullying vary considerably and that a lack of a consistent definition “hinders our ability to understand the true magnitude, scope, and impact of bullying and track trends over time” ( Gladden et al., 2014 , p. 1). One such approach to measuring bullying includes providing an explicit definition or explanation of what is meant by bullying to study participants. In contrast, some approaches simply use the word “bullying” but do not define it, whereas others list specific behaviors that constitute bullying without using the term “bullying” ( Gladden et al., 2014 ; Sawyer et al., 2008 ). Even if the definition is provided, researchers must assume that respondents (who are often children) fully understand the broad and difficult concept of bullying—including its elements of hostile intent, repetition, and power imbalance and its various forms—when answering. However, research has shown that this level of comprehension might not be uniformly present for children of all age groups and cultures ( Monks and Smith, 2006 ; Smith et al., 2002 ; Strohmeier and Toda, 2008 ; Vaillancourt et al., 2008 ). For instance, 8-year-old children consider fewer negative behavior options to be bullying than do 14-year-old adolescents ( Smith et al., 2002 ). Furthermore, children hold very different definitions of bullying from those held by researchers. Bullying may also be understood and defined differently in different languages and cultures ( Arora, 1996 ). Smith and colleagues (2002) showed that terms used in different cultures differed remarkably in their meanings. For example, some terms captured verbal aggression, while others were connected instead with physically aggressive acts or with social exclusion. These definitional issues are also relevant to cyberbullying, as there is no uniform definition used across studies.
There is still a lot of variability when it comes to defining bullying: Parents, children, and schools or medical professionals can mean a wide range of different things when they use the term “bullying.” Bullying varies in different developmental stages, and we should acknowledge that it is not always obvious. Even so, bullying can be characterized as the kind of behavior that would actually be considered harassment if the people involved were over age 18. However you look at it, a standardized definition would help us more precisely target bullying behavior and consequences while avoiding misunderstandings.
—Summary of themes from service providers/community-based providers focus group (See Appendix B for additional highlights from interviews.)

Measurement and Sampling Issues
Measuring bullying and cyberbullying is very difficult. The variability in prevalence rates reflects a number of measurement and sampling issues. First, studies reporting prevalence rates of bullying problems may rely on different data sources, such as peer versus teacher nominations or ratings, observations by researchers, or self-report questionnaires. Particularly with children, the self-report strategy poses a unique problem in regard to possible underreporting or overreporting ( Solberg, 2003 ). Some children who bully other students will choose not to respond honestly on the relevant questionnaire items for fear of retribution from adults. To date, a majority of information is gathered via self-reports, which have limitations; however, the committee does not believe that official reports are necessarily a better or more reliable source of information. The committee also acknowledges that for studies examining the prevalence of bullying by a certain demographic category, such as obesity or sexual orientation, it is not possible to say who is the “most bullied” by comparing students with one set of demographic characteristics with other students with different demographic characteristics.
Second, research suggests that the approach to measuring bullying does affect the pattern of responses and in turn may influence the prevalence rates. For example, a study of over 24,000 elementary, middle, and high school age youth found significantly higher prevalence rates for bullying when it was assessed using a behavior-based approach (i.e., asking about the experience of specific forms and acts of bullying) than when it was measured using a definition-based approach ( Sawyer et al., 2008 ). A similar pattern occurs for cyberbullying, For example, one study used a definition that read “repeatedly [trying] to hurt you or make you feel bad by e-mailing/e-messaging you or posting a blog about you on the Internet (MySpace).” This study found the prevalence of cybervictimization to be 9 percent ( Selkie et al., 2015 ). Another study asked about “the use of the Internet, cell phones and other technologies to bully, harass, threaten or embarrass someone” and found cybervictimization prevalence to be 31 percent ( Pergolizzi et al., 2011 ).
Third, studies may differ with regard to the reference period used in measuring bullying. For example, a question may refer to a whole school year or one school term, the past couple of months, or over a lifetime. Response and rating categories may vary in both number and specificity as well. Such categories may consist of a simple yes or no dichotomy; of various applicability categories such as “does not apply at all” and “applies perfectly”; or of relatively vague frequency alternatives ranging from “seldom” to “very often” or from “not at all in the past couple of months” to “several times a week.”
Fourth, some studies use different criteria for differentiating students who have been bullied and students who have not, as well as students who have and have not bullied others. This variation in identification makes prevalence rates difficult to compare ( Solberg, 2003 ). A majority of studies do not ask questions about children who have bullied or children who have been bystanders, instead focusing on children who have been bullied. Taken together, these findings suggest that researchers need to be cautious about interpreting their findings in light of their measurement approach.
Estimates of bullying inform an evidence-based understanding about the extent of the problem and bring attention to the need to address the problem and allocate the funding to do so. Prevalence estimates provide information for policy makers, identify where education is needed, identify vulnerable populations, and help direct assistance and resources. As this chapter has explained, generating reliable estimates for the number of children who have bullied and the number who have been bullied is not an easy task. In some cases, the task is extraordinarily difficult. For example, existing research suggests disparities in rates of bullying by a variety of characteristics, including sexual orientation, disability, and obesity, mostly due to the lack of nationally representative data on these and other vulnerable groups. Bullying must be understood as a social problem characterized by numerous challenges to estimating its prevalence and the conditions associated with it. In summary, based on its review of the available evidence, the committee maintains that, despite the current imperfect estimates, bullying and cyberbullying in the United States is clearly prevalent and therefore worthy of attention.
- FINDINGS AND CONCLUSIONS
Finding 2.1: Estimates of bullying and cyberbullying prevalence reported by national surveys vary greatly, ranging from 17.9 percent to 30.9 percent of school-age children for the prevalence of bullying behavior at school and from 6.9 percent to 14.8 percent for the prevalence of cyberbullying. The prevalence of bullying among some groups of youth is even higher. For instance, the prevalence of bullying of lesbian, gay, bisexual, and transgender youth is approximately double that of heterosexual and cisgender youth. Finding 2.2: The extent to which rates of bullying and cyberbullying have changed in recent years is unclear. Finding 2.3: The four major national surveys that include bullying do not uniformly address all age groups and school levels. Finding 2.4: A majority of prevalence data collection is done through self-reports or observation. Finding 2.5: A majority of national studies do not ask questions about children who have bullied or children who have been bystanders. Finding 2.6: Many studies differ with regard to the reference period used in measuring bullying behavior (e.g., last month versus last 12 months). Finding 2.7: Studies use different definitional criteria for differentiating students who have been bullied and cyberbullied and students who have not, as well as students who bully and cyberbully and students who do not. Finding 2.8: Existing research suggests that there are disparities in rates of bullying by a variety of characteristics, including sexual orientation, disability, and obesity. However, there is a lack of nationally representative data on these and other vulnerable groups. Future research is therefore needed to generate representative estimates of bullying, including bias-based and discriminatory bullying, to accurately identify disparity groups.
Conclusions
Conclusion 2.1: Definitional and measurement inconsistencies lead to a variation in estimates of bullying prevalence, especially across disparate samples of youth. Although there is a variation in numbers, the national surveys show bullying behavior is a real problem that affects a large number of youth. Conclusion 2.2: The national datasets on the prevalence of bullying focus predominantly on the children who are bullied. Considerably less is known about perpetrators, and nothing is known about bystanders in that national data. Conclusion 2.3: Cyberbullying should be considered within the context of bullying rather than as a separate entity. The Centers for Disease Control and Prevention definition should be evaluated for its application to cyberbullying. Although cyberbullying may already be included, it is not perceived that way by the public or by the youth population. Conclusion 2.4: Different types of bullying behaviors—physical, relational, cyber—may emerge or be more salient at different stages of the developmental life course. Conclusion 2.5: The online context where cyberbullying takes place is nearly universally accessed by adolescents. Social media sites are used by the majority of teens and are an influential and immersive medium in which cyberbullying occurs.
- Arora CM. Defining bullying towards a clearer general understanding and more effective intervention strategies. School Psychology International. 1996; 17 (4):317–329.
- Barboza GE, Schiamberg LB, Oehmke J, Korzeniewski SJ, Post LA, Heraux CG. Individual characteristics and the multiple contexts of adolescent bullying: An ecological perspective. Journal of Youth and Adolescence. 2009; 38 (1):101–121. [ PubMed : 19636795 ]
- Berlan ED, Corliss HL, Field AE, Goodman E, Austin SB. Sexual orientation and bullying among adolescents in the Growing Up Today study. Journal of Adolescent Health. 2010; 46 (4):366–371. [ PMC free article : PMC2844864 ] [ PubMed : 20307826 ]
- Blake JJ, Lund EM, Zhou Q, Kwok OM, Benz MR. National prevalence rates of bully victimization among students with disabilities in the United States. School Psychology Quarterly. 2012; 27 (4):210. [ PubMed : 23294235 ]
- Bowleg L. When black + lesbian + woman ≠ black lesbian woman: The methodological challenges of qualitative and quantitative intersectionality research. Sex Roles. 2008; 59 (5-6):312–325.
- Bowleg L. The problem with the phrase women and minorities: Intersectionality—an important theoretical framework for public health. American Journal of Public Health. 2012; 102 (7):1267–1273. [ PMC free article : PMC3477987 ] [ PubMed : 22594719 ]
- Bradshaw CP, Sawyer AL, O'Brennan LM. A social disorganization perspective on bullying-related attitudes and behaviors: The influence of school context. American Journal of Community Psychology. 2009; 43 (3-4):204–220. [ PubMed : 19333749 ]
- Card NA, Stucky BD, Sawalani GM, Little TD. Direct and indirect aggression during childhood and adolescence: A meta-analytic review of gender differences, intercorrelations, and relations to maladjustment. Child Development. 2008; 79 (5):1185–1229. [ PubMed : 18826521 ]
- Centers for Disease Control and Prevention. Youth Risk Behavior Surveillance—United States, 2009. Morbidity and Mortality Weekly Report. 2010; 59 (SS-5):1–142. [ PubMed : 20520591 ]
- Centers for Disease Control and Prevention. Youth Risk Behavior Surveillance—United States, 2009. Morbidity and Mortality Weekly Report. 2012; 61 (4):1–162. [ PubMed : 22673000 ]
- Centers for Disease Control and Prevention. LGBTQ Youth Programs at a Glance. 2014a. [December 2015]. http://www .cdc.gov/lgbthealth /youth-programs.htm .
- Centers for Disease Control and Prevention. Youth Risk Behavior Surveillance—United States. Morbidity and Mortality Weekly Report. 2014b; 63 (4):1–169. [ PubMed : 24918634 ]
- Centers for Disease Control and Prevention. Childhood Obesity Facts. 2015a. [November 2015]. http://www .cdc.gov/healthyschools /obesity/facts.htm .
- Centers for Disease Control and Prevention. Defining Childhood Obesity. 2015b. [November 2015]. http://www .cdc.gov/obesity /childhood/defining.html .
- Christie-Mizell CA, Keil JM, Laske MT, Stewart J. Bullying behavior, parents' work hours, and early adolescents' perceptions of time spent with parents. Youth & Society. 2011; 43 (4):1570–1595.
- Conron KJ, Scout N, Austin SB. “Everyone has a right to, like, check their box”: Findings on a measure of gender identity from a cognitive testing study with adolescents. Journal of LGBT Health Research. 2008; 4 (1):1–9. [ PubMed : 19860014 ] [ CrossRef ]
- Cook CR, Williams KR, Guerra NG, Kim T. Handbook of Bullying in Schools: An International Perspective. Jimerson SR, Swearer SM, Espelage DL, editors. New York: Routledge; 2010. pp. 347–362. (Variability in the prevalence of bullying and victimization).
- Cowie H, Bauman S, Coyne I, Myers C, Pörhölä M, Almeida A. Cyberbullying Through the New Media: Findings from an International Network. Smither PK, Steffgen G, editors. London, UK: Psychology Press; 2013. pp. 165–177. (Cyberbullying amongst university students).
- DeVoe JF, Bauer L. Student Victimization in U.S. Schools: Results from the 2007 School Crime Supplement to the National Crime Victimization Survey. Washington, DC: U.S. Government Printing Office; 2010. (NCES 2010-319).
- DeVoe JF, Bauer L. Student Victimization in U.S. Schools: Results from the 2009 School Crime Supplement to the National Crime Victimization Survey. Washington, DC: U.S. Government Printing Office; 2011.
- Dulmus CN, Theriot MT, Sowers KM, Blackburn JA. Student reports of peer bullying victimization in a rural school. Stress, Trauma, and Crisis. 2004; 7 (1):1–16.
- Eisenberg ME, Aalsma MC. Bullying and peer victimization: Position paper of the Society for Adolescent Medicine. Journal of Adolescent Health. 2005; 36 (1):88–91. [ PubMed : 15661606 ]
- Espelage DL, Aragon SR, Birkett M, Koenig BW. Homophobic teasing, psychological outcomes, and sexual orientation among high school students: What influence do parents and schools have? School Psychology Review. 2008; 37 (2):202–216.
- Farmer TW, Hamm JV, Leung MC, Lambert K, Gravelle M. Early adolescent peer ecologies in rural communities: Bullying in schools that do and do not have a transition during the middle grades. Journal of Youth and Adolescence. 2011; 40 (9):1106–1117. [ PubMed : 21667294 ]
- Farmer TW, Petrin R, Brooks DS, Hamm JV, Lambert K, Gravelle M. Bullying involvement and the school adjustment of rural students with and without disabilities. Journal of Emotional and Behavioral Disorders. 2012; 20 (1):19–37.
- Felix ED, Green JG. Handbook of Bullying in Schools. An International Perspective. Jimerson SR, Swearer SM, Espelage DL, editors. New York: Routledge; 2010. pp. 173–185. (Popular girls and brawny boys).
- Finkelhor D, Turner H, Ormrod R, Hamby S, Kracke K. Children's Exposure to Violence: A Comprehensive Survey. U.S. Department of Justice, Office of Justice Programs, Office of Juvenile Justice and Delinquency Prevention; 2012. [June 2016]. https://www .ncjrs.gov /pdffiles1/ojjdp/grants/248444.pdf .
- Finkelhor D, Turner HA, Shattuck A, Hamby SL. Violence, Crime, and Abuse Exposure in a National Sample of Children and Youth: An Update. U.S. Department of Justice, Office of Justice Programs, Office of Juvenile Justice and Delinquency Prevention; 2015. [June 2016]. http://www .ojjdp.gov/pubs/248547.pdf .
- Flouri E, Buchanan A. The role of mother involvement and father involvement in adolescent bullying behavior. Journal of Interpersonal Violence. 2003; 18 (6):634–644.
- Garner PW, Hinton TS. Emotional display rules and emotion self-regulation: Associations with bullying and victimization in community-based after school programs. Journal of Community & Applied Social Psychology. 2010; 20 (6):480–496.
- Garnett BR, Masyn KE, Austin SB, Miller M, Williams DR, Viswanath K. The intersectionality of discrimination attributes and bullying among youth: An applied latent class analysis. Journal of Youth and Adolescence. 2014; 43 (8):1225–1239. [ PubMed : 24318776 ]
- Garofalo R, Wolf RC, Kessel S, Palfrey J, DuRant RH. The association between health risk behaviors and sexual orientation among a school-based sample of adolescents. Pediatrics. 1998; 101 (5):895–902. [ PubMed : 9565422 ]
- GenIUSS Group. Gender-Related Measures Overview. The Williams Institute; 2013. [April 2016]. http: //williamsinstitute .law.ucla.edu/wp-content /uploads/GenIUSS-Gender-relatedQuestion-Overview.pdf .
- Gladden RM, Vivolo-Kantor AM, Hamburger ME, Lumpkin CD. Bullying Surveillance among Youths: Uniform Definitions for Public Health and Recommended Data Elements, Version 1.0. Atlanta, GA: Centers for Disease Control and Prevention and U.S. Department of Education; 2014.
- Glew GM, Fan MY, Katon W, Rivara FP, Kernic MA. Bullying, psychosocial adjustment, and academic performance in elementary school. Archives of Pediatrics & Adolescent Medicine. 2005; 159 (11):1026–1031. [ PubMed : 16275791 ]
- Goldweber A, Waasdorp TE, Bradshaw CP. Examining associations between race, urbanicity, and patterns of bullying involvement. Journal of Youth and Adolescence. 2013; 42 (2):206–219. [ PubMed : 23095907 ]
- Grant JM, Mottet L, Tanis JE, Harrison J, Herman J, Keisling M. Injustice at Every Turn: A Report of the National Transgender Discrimination Survey. National Center for Transgender Equality; 2011. [October 2015]. http://www .thetaskforce .org/static_html/downloads /reports/reports/ntds_full.pdf .
- Gray WN, Kahhan NA, Janicke DM. Peer victimization and pediatric obesity: A review of the literature. Psychology in the Schools. 2009; 46 (8):720–727.
- Griffiths LJ, Wolke D, Page AS, Horwood J. Obesity and bullying: Different effects for boys and girls. Archives of Disease in Childhood. 2006; 91 (2):121–125. [ PMC free article : PMC2082670 ] [ PubMed : 16174642 ]
- Hanlon BM. 2003 Massachusetts Youth Risk Behavior Survey Results. Malden: Massachusetts Department of Education; 2004.
- Hong JS, Espelage DL. A review of research on bullying and peer victimization in school: An ecological system analysis. Aggression and Violent Behavior. 2012; 17 (4):311–322.
- Hong JS, Peguero AA, Choi S, Lanesskog D, Espelage DL, Lee NY. Social ecology of bullying and peer victimization of Latino and Asian youth in the United States: A review of the literature. Journal of School Violence. 2014; 13 (3):315–338.
- Iannotti RJ. Health Behavior in School-Aged Children (HBSC), 2005-2006. Ann Arbor, MI: Inter-university Consortium for Political and Social Research; 2012.
- Iannotti RJ. Health Behavior in School-Aged Children (HBSC), 2009-2010. Ann Arbor, MI: Inter-university Consortium for Political and Social Research; 2013.
- Jansen DE, Veenstra R, Ormel J, Verhulst FC, Reijneveld SA. Early risk factors for being a bully, victim, or bully/victim in late elementary and early secondary education. The Longitudinal Trails Study. BMC Public Health. 2011; 11 (1):1–7. [ PMC free article : PMC3128024 ] [ PubMed : 21645403 ]
- Jansen PW, Verlinden M, Dommisse-van Berkel A, Mieloo C, van der Ende J, Veenstra R, Verhulst FC, Jansen W, Tiemeier H. Prevalence of bullying and victimization among children in early elementary school: Do family and school neighbourhood socioeconomic status matter? BMC Public Health. 2012; 12 (1):1–10. [ PMC free article : PMC3575320 ] [ PubMed : 22747880 ]
- Janssen I, Craig WM, Boyce WF, Pickett W. Associations between overweight and obesity with bullying behaviors in school-aged children. Pediatrics. 2004; 113 (5):1187–1194. [ PubMed : 15121928 ]
- Juvonen J, Gross EF. Extending the school grounds?—Bullying experiences in cyberspace. Journal of School Health. 2008; 78 (9):496–505. [ PubMed : 18786042 ]
- Kosciw JG, Greytak EA, Bartkiewicz MJ, Boesen MJ, Palmer NA. The 2011 National School Climate Survey: The Experiences of Lesbian, Gay, Bisexual, and Transgender Youth in Our Nation's School. Washington, DC: Education Resources Information Center, Institute of Education Sciences, U.S. Department of Education; 2012.
- Kowalski RM, Limber SP, Agatston PW. Cyberbullying: Bullying in the Digital Age. West Sussex, UK: Wiley-Blackwell; 2012.
- Kraft E, Wang J. An exploratory study of the cyberbullying and cyberstalking experiences and factors related to victimization of students at a public liberal arts college. International Journal of Technoethics. 2010; 1 (4):74–91.
- Larochette AC, Murphy AN, Craig WM. Racial bullying and victimization in Canadian school-age children: Individual and school level effects. School Psychology International. 2010; 31 (4):389–408.
- Lemstra ME, Nielsen G, Rogers MR, Thompson AT, Moraros JS. Risk indicators and outcomes associated with bullying in youth aged 9-15 years. Canadian Journal of Public Health/Revue Canadienne de Santé Publique. 2012; 103 (1):9–13. [ PMC free article : PMC6973964 ] [ PubMed : 22338321 ]
- Lenhart A, Duggan M, Perrin A, Stepler R, Rainie H, Parker K. Teens, Social Media & Technology Overview 2015. 2015. [September 2015]. http://www .pewinternet .org/files/2015/04 /PI_TeensandTech_Update2015_0409151.pdf .
- Lim SJJ, Hoot JL. Bullying in an increasingly diverse school population: A socioecological model analysis. School Psychology International. 2015
- Limber SB. Bullying Among Children and Youth. Department of Psychology and Institute on Family and Neighborhood Life, Clemson University, SC; 2014. (Unpublished manuscript of a study commissioned by the Planning Committee on Increasing Capacity for Reducing Bullying and Its Impact on the Lifecourse of Youth Involved).
- Limber SB, Olweus D, Luxenberg H. Bullying in U.S. Schools 2012 Status Report. Center City, MN: Hazelden Foundation; 2013.
- Lunde C, Frisén A, Hwang CP. Ten-year-old girls' and boys' body composition and peer victimization experiences: Prospective associations with body satisfaction. Body Image. 2007; 4 (1):11–28. [ PubMed : 18089248 ]
- Magklara K, Skapinakis P, Gkatsa T, Bellos S, Araya R, Stylianidis S, Mavreas V. Bullying behaviour in schools, socioeconomic position, and psychiatric morbidity: A cross-sectional study in late adolescents in Greece. Child and Adolescent Psychiatry and Mental Health. 2012; 6 (8):2–13. [ PMC free article : PMC3298787 ] [ PubMed : 22325708 ]
- McCall L. The complexity of intersectionality. Signs: Journal of Women in Culture and Society. 2005; 30 (3):1771–1800.
- McGuire JK, Anderson CR, Toomey RB, Russell ST. School climate for transgender youth: A mixed-method investigation of student experiences and school responses. Journal of Youth and Adolescence. 2010; 39 (10):1175–1188. [ PubMed : 20428933 ]
- McLaughlin C, Byers R, Vaughn R. Responding to Bullying among Children with Special Educational Needs and/or Disabilities. London, UK: Anti-Bullying Alliance; 2010.
- Monks CP, Smith PK. Definitions of bullying: Age differences in understanding of the term and the role of experience. British Journal of Developmental Psychology. 2006; 24 (4):801–821.
- Moreno MA, Kota R, Schoohs S, Whitehill JM. The Facebook influence model: A concept mapping approach. Cyberpsychology, Behavior, and Social Networking. 2013; 16 (7):504–511. [ PMC free article : PMC3715786 ] [ PubMed : 23621717 ]
- Nansel TR, Overpeck M, Pilla RS, Ruan WJ, Simons-Morton B, Scheidt P. Bullying behaviors among U.S. youth: Prevalence and association with psychosocial adjustment. Journal of the American Medical Association. 2001; 285 (16):2094–2100. [ PMC free article : PMC2435211 ] [ PubMed : 11311098 ]
- Neumark-Sztainer D, Falner N, Story M, Perry C, Hannan PJ, Mulert S. Weight-teasing among adolescents: Correlations with weight status and disordered eating behaviors. International Journal of Obesity and Related Metabolic Disorders. 2002; 26 (1):123–131. [ PubMed : 11791157 ]
- Nordhagen R, Nielsen A, Stigum H, Köhler L. Parental reported bullying among Nordic children: A population-based study. Child: Care, Health and Development. 2005; 31 (6):693–701. [ PubMed : 16207227 ]
- Ogden CL, Carroll MD, Kit BK, Flegal KM. Prevalence of childhood and adult obesity in the United States, 2011-2012. Journal of the American Medical Association. 2014; 311 (8):806–814. [ PMC free article : PMC4770258 ] [ PubMed : 24570244 ]
- Olsen EOM, Kann L, Vivolo-Kantor A, Kinchen S, McManus T. School violence and bullying among sexual minority high school students, 2009-2011. Journal of Adolescent Health. 2014; 55 (3):432–438. [ PubMed : 24768163 ]
- Olweus D. School bullying: Development and some important challenges. Annual Review of Clinical Psychology. 2013; 9 :751–780. [ PubMed : 23297789 ]
- Oppliger PA. Bullies and Mean Girls in Popular Culture. Jefferson, NC: McFarland & Company; 2013.
- Pereira B, Mendonca D, Neto C, Valente L, Smith PK. Bullying in Portuguese schools. School Psychology International. 2004; 25 (2):241–254.
- Pergolizzi F, Pergolizzi J, Gan Z, Macario S, Pergolizzi JV, Ewin T, Gan TJ. Bullying in middle school: Results from a 2008 survey. International Journal of Adolescent Medicine and Health. 2011; 23 (1):11–18. [ PubMed : 21721358 ]
- Peskin M, Tortolero SR, Markham CM. Bullying and victimization among black and Hispanic adolescents. Adolescence. 2006; 41 :467, 484. [ PubMed : 17225662 ]
- Puhl RM, Latner JD. Stigma, obesity, and the health of the nation's children. Psychological Bulletin. 2007; 133 (4):557. [ PubMed : 17592956 ]
- Reisner SL, Greytak EA, Parsons JT, Ybarra ML. Gender minority social stress in adolescence: Disparities in adolescent bullying and substance use by gender identity. Journal of Sex Research. 2015; 52 (3):243–256. [ PMC free article : PMC4201643 ] [ PubMed : 24742006 ]
- Rice E, Petering R, Rhoades H, Winetrobe H, Goldbach J, Plant A, Montoya J, Kordic T. Cyberbullying perpetration and victimization among middle school students. American Journal of Public Health. 2015; 105 (3):e66–e72. [ PMC free article : PMC4330864 ] [ PubMed : 25602905 ]
- Rivers I. The bullying of sexual minorities at school: Its nature and long-term correlates. Educational and Child Psychology. 2001; 18 (1):32–46.
- Robers S, Kemp J, Truman J. Indicators of School Crime and Safety: 2012. Washington, DC: National Center for Education Statistics, U.S. Department of Education; 2013. (NCES 2013-036/NCJ 241446).
- Rose CA. Bullying and Vulnerable Populations: Exploring Predictive and Protective Factors Associated with Disproportionate Representation. Department of Special Education, University of Missouri; Columbia: 2015. (Unpublished manuscript of a study commissioned by the Committee on the Biological and Psychosocial Effects of Peer Victimization: Lessons for Bullying Prevention).
- Rose CA, Espelage DL, Monda-Amaya LE. Bullying and victimisation rates among students in general and special education: A comparative analysis. Educational Psychology. 2009; 29 (7):761–776.
- Rose CA, Monda-Amaya LE, Espelage DL. Bullying perpetration and victimization in special education: A review of the literature. Remedial and Special Education. 2010; 32 :114–130.
- Rose CA, Simpson CG, Moss A. The bullying dynamic: Prevalence of involvement among a large-scale sample of middle and high school youth with and without disabilities. Psychology in the Schools. 2015; 52 (5):515–531.
- Russell ST, Driscoll AK, Truong N. Adolescent same-sex romantic attractions and relationships: Implications for substance use and abuse. American Journal of Public Health. 2002; 92 (2):198–202. [ PMC free article : PMC1447042 ] [ PubMed : 11818291 ]
- Russell ST, Everett BG, Rosario M, Birkett M. Indicators of victimization and sexual orientation among adolescents: Analyses from Youth Risk Behavior Surveys. American Journal of Public Health. 2014; 104 (2):255–261. [ PMC free article : PMC3935664 ] [ PubMed : 24328633 ]
- Sawyer AL, Bradshaw CP, O'Brennan LM. Examining ethnic, gender, and developmental differences in the way children report being a victim of “bullying” on self-report measures. Journal of Adolescent Health. 2008; 43 :106–114. [ PubMed : 18639783 ] [ CrossRef ]
- Schenk AM, Fremouw WJ. Prevalence, psychological impact, and coping of cyberbully victims among college students. Journal of School Violence. 2012; 11 (1):21–37.
- Schuster MA, Bogart LM. Did the ugly duckling have PTSD? Bullying, its effects, and the role of pediatricians. Pediatrics. 2013; 131 (1):e288–e291. [ PMC free article : PMC3529957 ] [ PubMed : 23266921 ]
- Schwartz D, Dodge KA, Pettit GS, Bates JE. The early socialization of aggressive victims of bullying. Child Development. 1997; 68 (4):665–675. [ PubMed : 9306645 ]
- Selkie EM, Fales JL, Moreno MA. Cyberbullying prevalence among U.S. middle and high school age adolescents: A systematic review and quality assessment. Journal of Adolescent Health. 2015; 58 (2):125–133. [ PMC free article : PMC4724486 ] [ PubMed : 26576821 ]
- Shetgiri R, Lin H, Flores G. Identifying children at risk for being bullies in the United States. Academic Pediatrics. 2012; 12 (6):509–522. [ PMC free article : PMC3501605 ] [ PubMed : 22989731 ]
- Smith PK, Cowie H, Olafsson RF, Liefooghe AP, Almeida A, Araki H, del Barrio C, Costabile A, Dekleva B, Houndoumadi A. Definitions of bullying: A comparison of terms used, and age and gender differences, in a fourteen-country international comparison. Child Development. 2002; 73 (4):1119–1133. [ PubMed : 12146737 ]
- Smith PK, Mahdavi J, Carvalho M, Fisher S, Russell S, Tippett N. Cyberbullying: Its nature and impact in secondary school pupils. Journal of Child Psychology and Psychiatry. 2008; 49 (4):376–385. [ PubMed : 18363945 ]
- Solberg ME. Prevalence estimation of school bullying with the Olweus bully/victim questionnaire. Aggressive Behavior. 2003; 29 (3):239–268.
- Son E, Parish SL, Peterson NA. National prevalence of peer victimization among young children with disabilities in the United States. Children and Youth Services Review. 2012; 34 (8):1540–1545.
- Spriggs AL, Iannotti RJ, Nansel TR, Haynie DL. Adolescent bullying involvement and perceived family, peer, and school relations: Commonalities and differences across race/ethnicity. Journal of Adolescent Health. 2007; 41 (3):283–293. [ PMC free article : PMC1989108 ] [ PubMed : 17707299 ]
- Stockdale MS, Hangaduambo S, Duys D, Larson K, Sarvela PD. Rural elementary students', parents', and teachers' perceptions of bullying. American Journal of Health Behavior. 2002; 26 (4):266–277. [ PubMed : 12081359 ]
- Strohmeier D, Toda Y. Cross-National Similarities and Differences. The Extent to Which Bullying and Victimisation Are Similar, or Show Differences, Across Cultures, Especially Western and Eastern Cultures. Wurzburg, Germany: Jul, 2008. (Paper presented at the preconference “Victimisation in Children and Youth,” 20th Biennial Meeting of the International Society for the Study of Behavioural Development).
- Twyman KA, Saylor CF, Saia D, Macias MM, Taylor LA, Spratt E. Bullying and ostracism experiences in children with special health care needs. Journal of Developmental & Behavioral Pediatrics. 2010; 31 (1):1–8. [ PubMed : 20081430 ]
- U.S. Department of Education. Student Reports of Bullying and Cyber-Bullying: Results from the 2011 School Crime Supplement to the National Crime Victimization Survey. Washington DC: Author; 2013.
- U.S. Department of Education. Student Reports of Bullying and Cyber-Bullying: Results from the 2013 School Crime Supplement to the National Crime Victimization Survey. Washington DC: Author; 2015. [April 2015]. http://nces .ed.gov/pubs2015/2015056.pdf .
- U.S. Department of Health and Human Services; Health Resources and Services Administration. Maternal and Child Health Bureau. Health Behavior in School-Aged Children (HBSC), 2001-2002. Ann Arbor, MI: Inter-university Consortium for Political and Social Research; 2008.
- U.S. Government Accountability Office. School Bullying-Extent of Legal Protections for Vulnerable Groups Needs to Be More Fully Assessed. Washington, DC: Author; 2012.
- Vaillancourt T, McDougall P, Hymel S, Krygsman A, Miller J, Stiver K, Davis C. Bullying: Are researchers and children/youth talking about the same thing? International Journal of Behavioral Development. 2008; 32 (6):486–495.
- Wensley K, Campbell M. Heterosexual and nonheterosexual young university students' involvement in traditional and cyber forms of bullying. Cyberpsychology, Behavior, and Social Networking. 2012; 15 (12):649–654. [ PubMed : 23078337 ]
- Wilson ML, Bovet P, Viswanathan B, Suris JC. Bullying among adolescents in a sub-Saharan middle-income setting. Journal of Adolescent Health. 2012; 51 (1):96–98. [ PubMed : 22727084 ]
- Wilson MM. Hate Crime Victimization 2004-2012 Statistics. Washington, DC: U.S. Department of Justice; 2014. [November 2015]. http://www .bjs.gov/content /pub/pdf/hcv0412st.pdf .
- Wolak J, Mitchell KJ, Finkelhor D. Does online harassment constitute bullying? An exploration of online harassment by known peers and online-only contacts. Journal of Adolescent Health. 2007; 41 (6):S51–S58. [ PubMed : 18047945 ]
- World Health Organization. Health Behavior in School-Aged Children (HBSC), 1997-1998. Ann Arbor, MI: Inter-university Consortium for Political and Social Research; 2003.
- Zimmerman FJ, Glew GM, Christakis DA, Katon W. Early cognitive stimulation, emotional support, and television watching as predictors of subsequent bullying among grade-school children. Archives of Pediatrics & Adolescent Medicine. 2005; 159 (4):384–388. [ PubMed : 15809395 ]
Additional information about strategies for overcoming these limitations can be found in Chapter 7 .
Households are selected through a stratified, multistage, cluster sampling process. Households in the sample are designed to be representative of all households as well as noninstitutionalized individuals ages 12 or older.
For the SCS, being “bullied” includes students being made fun of, called names, or insulted; being the subject of rumors; being threatened with harm; being pushed, shoved, tripped, or spit on; being pressured into doing things they did not want to do; being excluded from activities on purpose; and having property destroyed on purpose. “At school” includes the school building, school property, school bus, or going to and from school. Missing data are not shown for household income.
In 1995 and 1999, “at school” was defined for respondents as in the school building, on the school grounds, or on a school bus. In 2001, the definition for “at school” was changed to mean in the school building, on school property, on a school bus, or going to and from school.
The NCVS has a nationally representative sample of about 90,000 households comprising nearly 160,000 persons, whereas the sample size of the SCS is just 4,942 students.
The YRBS uses a cluster sampling design to produce a nationally representative sample of the students in grades 9-12 of all public and private school students in the 50 states and the District of Columbia.
The 2014 YRBS does not clarify whether this includes school events held off campus or the children's journey to and from school.
Electronically bullied includes being bullied through e-mail, chat rooms, instant messaging, Websites, or texting.
Each state-based and local-school-based YRBS employs a two-stage, cluster sample design to produce representative samples of students in grades 9-12 in the survey's jurisdiction.
States and cities could modify the national YRBS questionnaire for their own surveys to meet their needs.
The student survey was administered in a regular classroom setting to participating students by a school representative (e.g., teacher, nurse, guidance counselor, etc.).
Three versions of the self-report questionnaire were administered: one for fifth and sixth graders; one for students in seventh, eighth, and ninth grade; and one for students in tenth grade. The tenth grade questionnaire contained the complete set of questions asked.
This is the highest prevalence rate for both bullying and cyberbullying reports among the four national surveys.
For NatSCEV II, data were collected by telephone interview on 4,503 children and youth ages 1 month to 17 years. If the respondent was between the ages of 10-17, the main telephone interview was conducted with the child. If the respondent was younger than age 10, the interview was conducted with the child's primary caregiver.
The statistical standard for referring to “trends” is at least three data points in the same direction. In the SCS, the decrease from 2011 to 2013 is one data point, and conclusions should not be drawn at this point in time.
The committee's Statement of Task (see Box 1-1 ) requested “a particular focus on children who are most at risk of peer victimization—i.e., those with high-risk factors in combination with few protective factors . . .” At-risk subpopulations specifically named in the Statement of Task were “children with disabilities,” poly-victims, LGBT youth, and children living in poverty . . .”
The committee expands on this topic in Chapter 3 .
Reisner and colleagues (2015, p. 1) define cisgender youth as youth “whose gender identity or expression matches one's sex assigned at birth.”
The National YRBS data available at the time of publication did not include questions about sexual identity and sex of sexual contacts, but these topics are included in the YRBS report released in June 2016.
This section is adapted from a study ( Rose, 2015 ) commissioned by the committee for this report.
In this review, weight stigma included “verbal teasing (e.g., name calling, derogatory remarks, being made fun of), physical bullying (e.g., hitting, kicking, pushing, shoving), and relational victimization (e.g., social exclusion, being ignored or avoided, the target of rumors”) ( Puhl and Latner, 2007 , p. 558).
- Cite this Page Committee on the Biological and Psychosocial Effects of Peer Victimization: Lessons for Bullying Prevention; Board on Children, Youth, and Families; Committee on Law and Justice; Division of Behavioral and Social Sciences and Education; Health and Medicine Division; National Academies of Sciences, Engineering, and Medicine; Rivara F, Le Menestrel S, editors. Preventing Bullying Through Science, Policy, and Practice. Washington (DC): National Academies Press (US); 2016 Sep 14. 2, The Scope of the Problem.
- PDF version of this title (3.8M)
In this Page
Other titles in this collection.
- The National Academies Collection: Reports funded by National Institutes of Health
Related information
- PMC PubMed Central citations
- PubMed Links to PubMed
Recent Activity
- The Scope of the Problem - Preventing Bullying Through Science, Policy, and Prac... The Scope of the Problem - Preventing Bullying Through Science, Policy, and Practice
Your browsing activity is empty.
Activity recording is turned off.
Turn recording back on
Connect with NLM
National Library of Medicine 8600 Rockville Pike Bethesda, MD 20894
Web Policies FOIA HHS Vulnerability Disclosure
Help Accessibility Careers
Current limitations in cyberbullying detection: On evaluation criteria, reproducibility, and data scarcity
- Original Paper
- Open access
- Published: 16 November 2020
- Volume 55 , pages 597–633, ( 2021 )
Cite this article
You have full access to this open access article
- Chris Emmery ORCID: orcid.org/0000-0002-2179-559X 1 , 2 ,
- Ben Verhoeven 2 ,
- Guy De Pauw 2 ,
- Gilles Jacobs 3 ,
- Cynthia Van Hee 3 ,
- Els Lefever 3 ,
- Bart Desmet 3 ,
- Véronique Hoste 3 &
- Walter Daelemans 2
11k Accesses
18 Citations
4 Altmetric
Explore all metrics
The detection of online cyberbullying has seen an increase in societal importance, popularity in research, and available open data. Nevertheless, while computational power and affordability of resources continue to increase, the access restrictions on high-quality data limit the applicability of state-of-the-art techniques. Consequently, much of the recent research uses small, heterogeneous datasets, without a thorough evaluation of applicability. In this paper, we further illustrate these issues, as we (i) evaluate many publicly available resources for this task and demonstrate difficulties with data collection. These predominantly yield small datasets that fail to capture the required complex social dynamics and impede direct comparison of progress. We (ii) conduct an extensive set of experiments that indicate a general lack of cross-domain generalization of classifiers trained on these sources, and openly provide this framework to replicate and extend our evaluation criteria. Finally, we (iii) present an effective crowdsourcing method: simulating real-life bullying scenarios in a lab setting generates plausible data that can be effectively used to enrich real data. This largely circumvents the restrictions on data that can be collected, and increases classifier performance. We believe these contributions can aid in improving the empirical practices of future research in the field.
Similar content being viewed by others
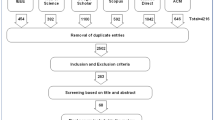
Cyberbullying detection and machine learning: a systematic literature review
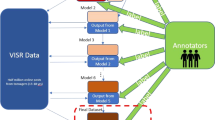
A multi-platform dataset for detecting cyberbullying in social media
Too Much Data? Opportunities and Challenges of Large Datasets and Cybercrime
Explore related subjects.
- Artificial Intelligence
Avoid common mistakes on your manuscript.
1 Introduction
Learning to accurately classify rare phenomena within large feeds of data poses challenges for numerous applications of machine learning. The volume of data required for representative instances to be included is often resource-consuming, and limited access to such instances can severely impact the reliability of predictions. These limitations are particularly prevalent in applications dealing with sensitive social phenomena such as those found in the field of forensics: e.g., predicting acts of terrorism, detecting fraud, or uncovering sexually transgressive behavior. Their events are complex and require rich representations for effective detection. Conversely, online text, images, and meta-data capturing such interactions have commercial value for the platforms they are hosted on and are often off-limits to protect users’ privacy.
An application affected by such limitations with increasing societal importance and growing interest over the last decade is that of cyberbullying detection. Not only is it sensitive, but the data is also inherently scarce in terms of public access. Most cyberbullying events are off-limits to the majority of researches, as they take place in private conversations. Fully capturing the social dynamics and complexity of these events requires much richer data than available to the research community up until now. Related to this, various issues with the operationalization of cyberbullying detection research were recently demonstrated by Rosa et al. ( 2019 ), who share much of the same concerns as we will discuss in this work. While their work focuses on methodological rigor in prior research, we will focus on the core limitations of the domain and complexity of cyberbullying detection. Through an evaluation of the current advances on the task, we illustrate how the mentioned issues affect current research, particularly cross-domain. Finally, we demonstrate crowdsourcing in an experimental setting to potentially alleviate the task’s data scarcity. First, however, we introduce the theoretical framing of cyberbullying and the task of automatically detecting such events.
1.1 Cyberbullying
Asynchrony and optional anonymity are characteristic of online communication as we know it today; it heavily relies on the ability to communicate with people who are not physically present, and stimulates interaction with people outside of one’s group of close friends through social networks (Madden et al. 2013 ). The rise of these networks brought various advantages to adolescents: studies show positive relationships between online communication and social connectedness (Bessiere et al. 2008 ; Valkenburg and Peter 2007 ), and that self-disclosure on these networks benefits the quality of existing and newly developed relationships (Steijn and Schouten 2013 ). The popularity of social networks and instant messaging among children has them connecting to the Internet from increasingly younger ages (Ólafsson et al. 2013 ), with \(95\%\) of teens Footnote 1 ages 12–17 online,of which \(80\%\) are on social media (Lenhart et al. 2011 ). For them, however, the transition from social interaction predominantly taking place on the playground to being mediated through mobile devices (Livingstone et al. 2011 ) has also moved negative communication to a platform where indirect and anonymous interaction has a window into homes.
A range of studies conducted by the Pew Research Center Footnote 2 , most notably (Lenhart et al. 2011 ), provides detailed insight into these developments. While \(78\%\) of teens report positive outcomes from their social media interactions, \(41\%\) have experienced at least some adverse outcomes, ranging from arguments, trouble with school and parents, physical fights and ending friendships. From \(19\%\) bullied in the 12 months prior to the study, \(8\%\) of all teens reported this was some form of cyberbullying. These numbers are comparable to other research (Robers et al. 2015 ; Kann et al. 2014 ) (7% for Grades 6–12, and 15% Grades 9–12 respectively). Bullying has for a while been regarded as a public health risk by numerous authorities (Xu et al. 2012 ), with depression, anxiety, low self-esteem, school absence, lower grades, and risk of self-medication as primary concerns.
The act of cyberbullying—other than being conducted online—shares the characteristics of traditional bullying: a power imbalance between the bully and victim (Sharp and Smith 2002 ), the harm is intentional, repeated over time, and has a negative psychological effect on the victim (DeHue et al. 2008 ). With the Internet as a communication platform however, some additional aspects arise: location, time, and physical presence have become an irrelevant factor in the act. Accordingly, several categories unique to this form of bullying are defined (Willard 2007 ; Beran and Li 2008 ): flaming (sending rude or vulgar messages), outing (posting private information or manipulated personal material of an individual without consent), harassment (repeatedly sending offensive messages to a single person), exclusion (from an online group), cyberstalking (terrorizing through sending explicitly threatening and intimidating messages), denigration (spreading online gossips), and impersonation . Moreover, in addition to optional anonymity hiding the critical figures behind an act of cyberbullying, it could also obfuscate the number of actors (i.e., there might only be one even though it seems there are more). Cyberbullying acts can prove challenging to remove once published; messages or images might persist through sharing and be viewable by many (as is typical for hate pages), or available to a few (in group or direct conversations). Hence, it can be argued that any form of harassment has become more accessible and intrusive. This online nature has an advantage as well: in theory, platforms record these bullying instances. Therefore, an increasing number of researches are interested in the automatic detection (and prevention) of cyberbullying.
1.2 Detection and task complexity
The task of cyberbullying detection can be broadly defined as the use of machine learning techniques to automatically classify text in messages on bullying content, or infer characteristic features based on higher-order information, such as user features or social network attributes. Bullying is most apparent in younger age groups through direct verbal outings (Vaez et al. 2004 ), and more subtle in older groups, mainly manifested in more complex social dynamics such as exclusion, sabotage, and gossip (Privitera and Campbell 2009 ). Therefore, the majority of work on the topic focuses on younger age groups, be it deliberately or given that the primary source for data is social media—which will likely result in these being highly present for some media (Duggan 2015 ). Apart from the well-established challenges that language use poses (e.g., ambiguity, sarcasmdialects, slang, neologisms), two factors in the event add further linguistic complexity, namely that of actor role and associated context . In contrast to tasks where adequate information is provided in the text of a single message alone, to completely map a cyberbullying event and pinpoint bully and victim implies some understanding of the dynamics between the involved actors and the concurrent textual interpretation of the register .
1.3 Register
Firstly, to understand the task of cyberbullying detection as a specific domain of text classification, one should consider the full scope of the register that defines it. The bullying categories discussed in Sect. 1.1 include some initial cues that can be inferred from text alone; flaming being the most obvious through simple curse word usage, slurs, or other profanity. Similarly, threatening or intimidating messages that fall under cyberstalking are clearly denoted by particular word usage. The other categories are more subtle: outing could also be done textually, in the form of a phone number, or pieces of information that are personal or sensitive in nature. Denigration would include words that are not blatantly associated with abusive acts; however, misinformation about sensitive topics might for example be paired with a victim’s name. One could further extend these cues based on the literature (as also captured in Hee et al. ( 2015 )) to include bullying event cues, such as messages that serve to defend the victim, and those in support of the bully. The linguistic task could therefore be framed (partly based on Van Hee et al. ( 2018 )) as identifying an online message context that includes aggressive or hurtful content against a victim . Several additional communicative components in these contexts further change the interpretation of these cues, however.
Secondly, there is a commonly made distinction between several actors within a cyberbullying event. A naive role allocation includes a bully B , a victim V and bystander BY , the latter of whom may or may not approve of the act of bullying. More nuanced models such as that of Xu et al. ( 2012 ) include additional roles (see Fig. 1 for a role interaction visualization), where different roles can be assigned to one person; for example, being bullied and reporting this. Most importantly, all shown roles can be present in the span of one single thread on social media, as demonstrated in Table 1 . While some roles clearly show from frequent interaction with either a positive or negative sentiment ( B , V , A ), others might not be observable through any form of conversation ( R , BY ), prove too subtle, or not distinguishable from other roles.
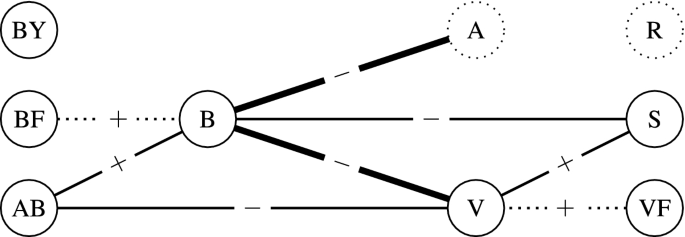
Role graph of a bullying event. Each vertex represents an actor, labeled by their role in the event: bully ( B ), victim ( V ), bystander ( BY ), reinforcer ( AB ), assistant ( BF ), defender ( S ), reporter ( S ), accuser ( A ), and friend ( VF ). Each edge indicates a stream of communication, labeled by whether this is positive ( \(+\) ) or negative (−) in nature, and its strength indicating the frequency of interaction. Dotted edges indicate nonparticipation in the event, and vertices those added by Xu et al. ( 2012 ) to account for social-media-specific roles
1.5 Context
Thirdly, the content of the messages has to be interpreted differently between these roles. While curse words can be a good indication of harassment, identification of a bully arguably requires more than these alone. Consider Table 1 : both B and A use insults (lines 7–8), the message of V (line 6) might be considered as bullying in isolation, and having already determined B , the last sentence (line 10) can generally be regarded as a threat. In conclusion, the full scope of the task is complex; it could have a temporal-sequential character, would benefit from determining actors and their interactions, and then should have some sense of severity as well (e.g. distinguish bullying from teasing).
1.6 Our contributions
Surprisingly, a significant amount of work on the task does not collect (or use) data that allows for the inference of such features (which we will further elaborate on in Sect. 3 ). To confirm this, we reproduce part of the previous cyberbullying detection research on different sources. Predictions made by current automatic methods for cyberbullying classification are demonstrated not to reflect the above-described task complexity; we show performance drops across different training domains, and give insights into content feature importance and limitations. Additionally, we report on reproducibility issues in state-of-art work when subjected to our evaluation. To facilitate future reproduction, we will provide all code open-source, including dataset readers, experimental code, and qualitative analyses. Footnote 3 Finally, we present a method to collect crowdsourced cyberbullying data in an experimental setting. It grants control over the size and richness of the data, does not invade privacy, nor rely on external parties to facilitate data access. Most importantly, we demonstrate that it successfully increases classifier performance. With this work, we provide suggestions on improving methodological rigor and hope to aid the community in a more realistic evaluation and implementation of this task of societal importance.
2 Related work
The task of detecting cyberbullying content can be roughly divided into three categories. First, research with a focus on binary classification, where it is only relevant if a message contains bullying or not. Second, more fine-grained approaches where the task is to determine either the role of actors in a bullying scenario or the content type (i.e., different categories of bullying). Both binary and fine-grained approaches predominantly focus on text-based features. Lastly, meta-data approaches that take more than just message content into account; these might include profile, network, or image information. Here, we will discuss efforts relevant to the task of cyberbullying classification within these three topics. We will predominantly focus on work conducted on openly available data, and those that report (positive) \(F_1\) -scores, to promote fair comparisons. Footnote 4 For an extensive literature review and a detailed comparison of different studies, see Rosa et al. ( 2019 ). Finally, a significant portion of our research pertains to generalizability, and therefore the field of domain adaptation. We will discuss its previous observations related text classification specifically, and their relevance to (future) research on cyberbullying detection.
2.1 Binary classification
One of the first traceable suggestions for applying text mining specifically to the task of cyberbullying detection is made by Kontostathis et al. ( 2010 ), who note that Yin et al. ( 2009 ) previously tried to classify online harassment on the caw 2.0 dataset. Footnote 5 In the latter research, Yin et al. already state that the ratio of documents with harassing content to typical documents is challengingly small. Moreover, they foresee several other critical issues with regards to the task: a lack of positive instances will make detecting characteristic features a difficult task, and human labeling of such a dataset might have to face issues of ambiguity and sarcasm that are hard to assess when messages are taken out of conversation context. Even with very sparse datasets (with less than \(1\%\) positive class instances), the harassment classifier outperforms the random baseline using tf \(\cdot \) idf, pronoun, curse word, and post similarity features.
Following up Yin et al. ( 2009 ), Reynolds et al. ( 2011 ) note that the caw 2.0 dataset is generally unfit for cyberbullying classification: in addition to lacking bullying labels (it only provides harassment labels), the conversations are predominantly between adults. Their work, along with Bayzick et al. ( 2011 ), is a first effort to create datasets for cyberbullying classification through scraping the question-answering website Formspring.me, as well as Myspace. Footnote 6 In contrast with similar research, they aim to use textual features while deliberately avoiding Bag-of-Words (BoW) features. Through a curse word dictionary and custom severity annotations, they construct several metrics for features related to these “bad” words. In their more recent paper, Kontostathis and Reynolds ( 2013 ) redid analyses on the kon_frm set, primarily focusing on the contribution curse words have in the classification of bullying messages. By forming queries from curse word dictionaries, they show that there is no one combination which retrieves all. However, by capturing them in an Essential Dimensions of Latent Semantic Indexing query vector averaged over known bullying content—classifying the top- k (by cosine similarity) as positive—they show a significant Average Precision improvement over their baseline.
More recent efforts include Bretschneider et al. ( 2014 ), who combined word normalization, Named Entity Recognition to detect person-specific references, and multiple curse word dictionaries (Noswearing.com 2016 ; Broadcasting Standards Authority 2013 ; Millwood-Hargreave 2000 ) in a rule-based pattern classifier, scoring well on Twitter data. Footnote 7 Our own work (Hee et al. 2015 ), where we collected a large dataset with posts from Ask.fm, used standard BoW features as a first test. Later, these were extended in Van Hee et al. ( 2018 ) with term lists, subjectivity lexicons, and topic model features. Recently popularized techniques of word embeddings and neural networks have been applied by Zhao et al. ( 2016 ); Zhao and Mao ( 2016 ) on xu_trec , nay_msp and sui_twi , both resulting in the highest performance for those sets. Convolutional Neural Networks (CNNs) on phonetic features were applied by Zhang et al. ( 2016 ) and Rosa et al. ( 2018 ) investigate among others the same architecture on textual features in combination with Long Short Term Memory Networks (LSTMs). Both Rosa et al. ( 2018 ) and that of Agrawal and Awekar ( 2018 ) investigate the C-LSTM (Zhou et al. 2015 ), the latter includes Synthetic Minority Over-sampling Technique (SMOTE). However, as we will show in the current research, both of these works suffer from reproducibility issues. Finally, fuzzified vectors of top- k word lists for each class were used to conduct membership likelihood-based classification by Rosa et al. ( 2018 ) on kon_frm , boosting recall over previously used methods.
2.2 Fine-grained classification
The common denominator of the previously discussed research was a focus on detecting single messages with evidence of cyberbullying per instance. As argued in Sect. 1.2 , however, there are more textual cues to infer than merely if a message might be interpreted as bullying. The work of Xu et al. ( 2012 ) proposed to expand this binary approach with more fine-grained classification tasks by looking at bullying traces ; i.e., the responses to a bullying incident. They distinguished two tasks based on keyword-retrieved ( bully ) Twitter data: Footnote 8 (1) a role labeling task, where semantic role labeling was then used to distinguish person-mention roles, and (2) the incorporation of sentiment to determine teasing , where despite high accuracy, \(48\%\) of the positive instances were misclassified.
In our work, we extended this train of thought and demonstrated the difficulty of fine-grained classification of types of bullying (curse, defamation, defense, encouragement, insult, sexual, threat), and roles (harasser, bystander assistant, bystander defender, victim) with simple BoW and sentiment features—especially in detecting types (Hee et al. 2015 ; Van Hee et al. 2015 ). Later, this was further addressed in Van Hee et al. ( 2018 ) for both Dutch and English. Evaluated against a profanity (curse word lexicon) and word n -gram baseline, a multi-feature model (as discussed in Sect. 2.1 ) achieved the lowest error rates over (almost) all labels, for both bullying type and role classification. Lastly, Tomkins et al. ( 2018 ) also adapt fine-grained knowledge about bullying events in their socio-linguistic model; in addition to performing bullying classification, they find latent text categories and roles, partly relying on social interactions on Twitter. It thereby ties in with the next category of work: leveraging meta-data from the network the data is collected from.
2.3 Meta-data features
A notable, yet less popular aspect of this task is the utilization of a graph for visualizing potential bullies and their connections. This method was first adopted by Nahar et al. ( 2013 ), who use this information in combination with a classifier trained on LDA and weighted tf \(\cdot \) idf features to detect bullies and victims on the caw_* datasets. Work that more concretely implements techniques from graph theory is that of Squicciarini et al. ( 2015 ), who used a wide range of features: network features to measure popularity (e.g., degree centrality, closeness centrality), content-based features, (length, sentiment, offensive words, second-person pronouns), and incorporated age, gender, and number of comments. They achieved the highest performance on kon_frm and bay_msp .
Work by Hosseinmardi et al. ( 2015 ) focuses on Instagram posts and incorporates platform-specific features retrieved from images and its network. They are the first to adhere to the literature more closely and define cyberagression (Kowalski et al. 2012 ) separately from cyberbullying, in that these are single negative posts rather than the repeated character of cyberbullying. They also show that certain LIWC (Linguistic Inquiry and Word Count) categories, such as death, appearance, religion, and sexuality, give a good indication of cyberbullying. While BoW features perform best, meta-data features (such as user properties and image content) in combination with textual features from the top 15 comments achieve a similar score. Cyberagression seems to be slightly easier to classify.
2.4 Domain adaptation
As the majority of the work discussed above focuses on a single corpus, a serious omission seems to be gauging how this influences model generalization. Many applications in natural language processing (NLP) are often inherently limited by expensive high-quality annotations, whereas unlabeled data is plentiful. Idiosyncrasies between source and target domains often prove detrimental to the performance of techniques relying on those annotations McClosky et al. ( 2006 ), Chan et al. ( 2006 ); Vilain et al. ( 2007 ) when applied in the wild. The field of domain adaptation identifies tasks that suffer from such limitations, and aims to overcome them either in a supervised DauméIII ( 2009 ); Finkel et al. ( 2009 ) or unsupervised Blitzer et al. ( 2007 ); Jiang and Zhai ( 2007 ); Ma et al. ( 2014 ); Schnabel and Schütze ( 2014 ) way. For text classification, sentiment analysis is arguably closest to the task of cyberbullying classification Glorot et al. ( 2011 ); Chan et al. ( 2012 ); Pan et al. ( 2010 ). In particular as imbalanced data exacerbates generalization Li et al. ( 2012 ). However, while for sentiment analysis these issues are clearly identified and actively worked on, the same cannot be said for cyberbullying detection, Footnote 9 where concrete limitations have yet to be explored. We assume to find issues similar to those in sentiment analysis in the current task, as we will further discuss in the following section.
3 Task evaluation importance and hypotheses
The domain of cyberbullying detection is in its early stages, as can be seen in Table 2 . Most datasets are quite small, and only a few have seen repeated experiments. Given the substantial societal importance of improving the methods developed so far, pinpointing shortcomings in the current state of research should assist in creating a robust framework under which to conduct future experiments—particularly concerning evaluating (domain) generalization of the classifiers. The latter of which, to our knowledge, none of the current research seems involved with. This is therefore the main focus of our work. In this section, we define three motivations for assessing this, and pose three respective hypotheses through which we will further investigate current limitations in cyberbullying detection.
3.1 Data scarcity
Considering the complexity of the social dynamics underlying the target of classification, and the costly collection and annotation of training data, the issue of data scarcity can mostly be explained with respect to the aforementioned restrictions on data access: while on a small number of platforms most data is accessible without any internal access (commonly as a result of optional user anonymity), it can be assumed that a significant part of actual bullying takes place ‘behind closed doors’. To uncover this, one would require access to all known information within a social network (such as friends, connections, and private messages, including all meta-data). As this is unrealistic in practice, researchers rely on the small subset of publicly accessible data (predominantly text) streams. Consequently, most of the datasets used for cyberbullying detection are small and exhibit an extreme skew between positive and negative messages (as can be seen in Table 3 ). It is unlikely that these small sets accurately capture the language use on a given platform, and generalizable linguistic features of the bullying instances even less so. In line with domain adaptation research, we therefore anticipate that the samples are underpowered in terms of accurately representing the substantial language variation between platforms, both in normal language use and bullying-specific language use (Hypothesis 1).
3.2 Task definition
Furthermore, we argue that this scarcity introduces issues with adherence to the definition of the task of cyberbullying. The chances of capturing the underlying dynamics of cyberbullying (as defined in the literature) are slim with the message-level (i.e., using single documents only) approaches that the majority of work in the field has used up until now. The users in the collected sources have to be rash enough to bully in the open, and particular (curse) word usage that would explain the effectiveness of dictionary and BoW-based approaches in previous research. Hence, we also assume that the positive instances are biased; only reflecting a limited dimension of bullying (Hypothesis 2). A more realistic scenario—where characteristics such as repetitiveness and power imbalance are taken into consideration—would require looking at the interaction between persons, or even profile instances rather than single messages, which, as we argued, is not generally available. The work found in the meta-data category (Sect. 2.3 ) supports this argument with improved results using this information.
This theory regarding the definition (or operationalization) of this task is shared by Rosa et al., who pose that “ the most representative studies on automatic cyberbullying detection, published from 2011 onward, have conducted isolated online aggression classification ” (Rosa et al. 2019 , p. 341). We will mainly focus on the shared notion that this framing is limited to verbal aggression; however, our focus will empirically assess its overlap with data framed to solely contain online toxicity data (i.e., online / cyberagression) to find concrete evidence.
3.3 Domain influence
Enriching previous work with data such as network structure, interaction statistics, profile information, and time-based analyses might provide fruitful sources for classification and a correct operationalization of the task. However, they are also domain-specific, as not all social media have such a rich interaction structure. Moreover, it is arguably naive to assume that social networks such as Facebook (for which in an ideal case, all aforementioned information sources are available) will stay a dominant platform of communication. Recently, younger age groups have turned towards more direct forms of communication such as WhatsApp, Snapchat, or media-focused forms such as Instagram (Smith and Anderson 2018 ), and recently TikTok. This move implies more private and less affluent environments in which data can be accessed (resulting in even more scarcity), and that further development in the field requires a critical evaluation of the current use of the available features, and ways to improve cross-domain generalization overall. This work, therefore, does not disregard textual features; they would still need to be considered as the primary source of information, while paying particular attention to the issues mentioned here. We further try to contribute towards this goal and argue that crowdsourcing bullying content potentially decreases the influence of domain-specific language use, allows for richer representations, and alleviates data scarcity (Hypothesis 3).
For the current research, we distinguish a large variety of datasets. For those provided through the AMiCA (Automatic Monitoring in Cyberspace Applications) Footnote 10 project, the Ask.fm corpus is partially available open-source, Footnote 11 and the Crowdsourced corpus will be made available upon request. All other sources are publicly available datasets gathered from previous research Footnote 12 as discussed in Sect. 2 . Corpus statistics of all data discussed below can be found in Table 3 . The sets’ abbreviations, language ( en for English, nl for Dutch), and brief collection characteristics can be found below.
Ask.fm ( \(D_{ask}\) , \(D_{ask\_nl}\) , en , nl ) were collected from the eponymous social network by Hee et al. ( 2015 ). Ask.fm is a question answering-style network where users interact by (frequently anonymously) asking questions on other profiles, and answering questions on theirs. As such, a third party cannot react to these question-answer pairs directly. The anonymity and restrictive interactions make for a high amount of potential cyberbullying. Profiles were retrieved through profile seed list, used as a starting point for traversing to other profiles and collecting all existing question-answer pairs for those profiles—these are predominantly Dutch and English. Each message was annotated with fine-grained labels (further details can be found in Van Hee et al. ( 2015 )); however, for the current experiments these were binarized, with any form of bullying being labeled positive.
Donated ( \(D_{don\_nl}\) , nl ) contains instances of (Dutch) cyberbullying from a mixture of platforms such as Skype, Facebook, and Ask.fm. The set is quite small; however, it contains several hate pages that are valuable collections of cyberbullying directed towards one person. The data was donated for use in the AMiCA project by previously bullied teens, thus forming a reliable source of gold standard, real-life data.
Crowdsourced ( \(D_{sim\_nl}\) , nl ) originates from a crowdsourcing experiment conducted by Broeck et al. ( 2014 ), wherein 200 adolescents aged 14 to 18 partook in a role-playing experiment on an isolated SocialEngine Footnote 13 social network. Here, each respondent was given the account of a fictitious person and put in one of four roles in a group of six: a bully, a victim, two bystander-assistants, and two bystander-defenders. They were asked to read—and identify with—a character description and respond to an artificially generated initial post attributed to one of the group members. All were confronted with two initial posts containing either low- or high-perceived severity of cyberbullying.
4.2 Related work
Formspring ( \(D_{frm}\) , en ) is taken from the research by Reynolds et al. ( 2011 ) and is composed of posts from Formspring.me, a question-answering platform similar to Ask.fm. As Formspring is mostly used by teenagers and young adults, and also provides the option to interact anonymously, it is notorious for hosting large amounts of bullying content (Binns 2013 ). The data was annotated through Mechanical Turk, providing a single label by majority vote for a question-answer pair. For our experiments, the question and answer pairs were merged into one document instance.
Myspace ( \(D_{msp}\) , en ) was collected by Bayzick et al. ( 2011 ). As this was set up as an information retrieval task, the posts are labeled in batches of ten posts, and thus a single label applies to the entire batch (i.e., does it include cyberbullying). These were merged per batch as one instance and labeled accordingly. Due to this batching, the average tokens per instance are much higher than any of the other corpora.
Twitter ( \(D_{twB}\) , en ) by Bretschneider et al. ( 2014 ) was collected from the stream between 20-10-2012 and 30-12-2012, and was labeled based on a majority vote between three annotators. Excluding re-tweets, the main dataset consists of 220 positive and 5162 negative examples, which adheres to the general expected occurrence rate of 4%. Their comparably-sized test set, consisting of 194 positive and 2699 negative examples, was collected by adding a filter to the stream for messages to contain any of the words school , class , college , and campus . These sets are merged for the current experiments.
Twitter II ( \(D_{twX}\) , en ) from Xu et al. ( 2012 ) focussed on bullying traces , and was thus retrieved by keywords ( bully , bullying ), which if left unmasked generates a strong bias when utilized for classification purposes (both by word usage as well as being a mix of toxicity and victims). It does, however, allow for demonstrating the ability to detect bullying-associated topics, and (indirect) reports of bullying.
4.3 Experiment-specific
Ask.fm Context ( \(C_{ask}\) , \(C_{ask}\_nl\) , en , nl )—the Ask.fm corpus was collected on profile level, but prior experiments have focused on single message instances (Van Hee et al. 2018 ). Here, we aggregate all messages for a single profile, which is then labeled as positive when as few as a single bullying instance occurs on the profile. This aggregation shifts the task of cyberbullying message detection to victim detection on profile level, allowing for more access to context and profile-level severity (such as repeated harassment), and makes for a more balanced set (1,763 positive and 6,245 negative instances).
Formspring Context ( \(C_{frm}\) , en )—similar to the Ask.fm corpus, was collected on profile level (Reynolds et al. 2011 ). However, the set only includes 49 profiles, some of which only include a single message. Grouping on full profile level would result in very few instances; thus, we opted for creating small ‘context’ in batches of five (of the same profile). Similar to the Ask.fm approach, if one of these messages contains bullying, it is labeled positive, balancing the dataset (565 positive and 756 negative instances).
Toxicity ( \(D_{tox}\) , en )—bashed on the Detox set from Wikimedia (Thain et al. 2017 ; Wulczyn et al. 2017 ), this set offers over 300k messages Footnote 14 of Wikipedia Talk comments with Crowdflower-annotated labels for toxicity (including subtypes). Footnote 15 Noteworthy is how disjoint both the task and the platform are from the rest of the corpora used in this research. While toxicity shares many properties with bullying, the focus here is on single instances of insults directed to anonymous people, who are most likely unknown to the harasser. Given Wikipedia as a source, the article and moderation focussed comments make it topically quite different from what one would expect on social media—the fundamental overlap being curse words, which is only one of many dimensions to be captured to detect cyberbullying (as opposed to toxicity).
4.4 Preprocessing
All texts were tokenized using spaCy (Honnibal and Montani 2017 ). Footnote 16 No preprocessing was conducted for the corpus statistics in Table 3 . All models (Sect. 5 ) applied lowercasing and special character removal only; alternative preprocessing steps proved to decreased performance (see Table 6 ).
4.5 Descriptive analysis
Both Table 3 and Fig. 2 illustrate stark differences; not only across domains but more importantly, between in-domain training and test sets. Most do not exceed a Jaccard similarity coefficient over 0.20 (Fig. 2 ), implying a large part of their vocabularies do not overlap. This contrast is not necessarily problematic for classification; however, it does hamper learning a general representation for the negative class. It also clearly illustrates how even more disjoint \(D_{twX}\) (collected by trace queries) and \(D_{tox}\) are from the rest of the corpora and splits. Finally, the descriptives (Table 3 ) further show significant differences in size, message length, class balance, and type/token ratios (i.e., writing level). In conclusion, it can be assumed that the language use in both positive as negative instances will vary significantly, and that it will be challenging to model in-domain, and generalize out-of-domain.
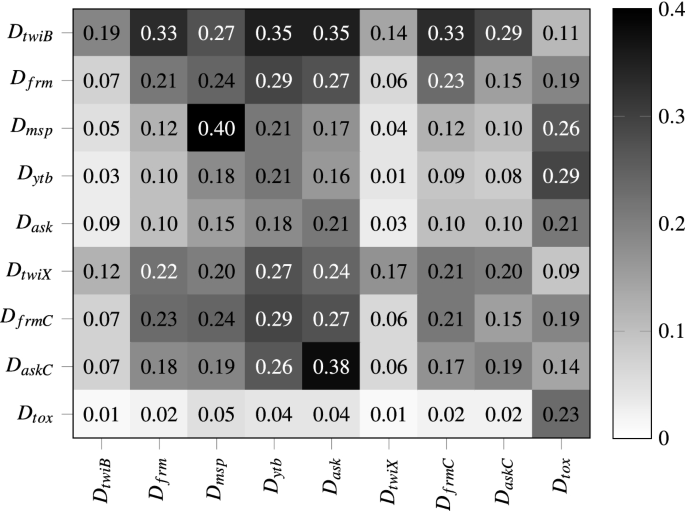
Jaccard similarity between training sets ( y -axis) and test sets ( x -axis)
5 Experimental setup
We attempt to address the following hypotheses posited in Sect. 3 :
Hypothesis 1: As researchers can only rely on scarce data of public bullying, their samples are assumed to be underpowered in terms of accurately representing the substantial language variation between platforms, both in normal language use and bullying-specific language use.
Hypothesis 2: Given knowledge from the literature, it is unlikely that single messages capture the full complexity of bullying events. Cyberbullying instances in the considered corpora are therefore expected to be largely biased, only reflecting a limited dimension of bullying.
Hypothesis 3: With control over data generation and structure, crowdsourcing bullying content potentially decreases the influence of domain-specific language use, allows for richer representations, and alleviates data scarcity.
Accordingly, we propose five main experiments. Experiments I (Hypothesis 1) and III (Hypothesis 2) deal with the problem of generalizability, whereas Experiment II (Hypothesis 1) and V (Hypothesis 3) will both propose a solution for restricted data collection. Experiment IV will reproduce a selection of state-of-the-art models for cyberbullying detection and subject them to our cross-domain evaluation, to be compared against our baselines.
5.1 Experiment I: cross-domain evaluation
In this experiment, we introduce the cross-domain evaluation framework, which will be extended in all other experiments. For this, we initially perform a many-to-many evaluation of a given model (baseline or otherwise) trained individually on all available data sources, split in train and test. In later experiments, we extend this with a one-to-many evaluation. This setup implies that (i) we fit our model on some given corpus’ training portion and evaluate prediction performance on all available corpora their test portions (many-to-many) individually. Furthermore, we (ii) fit on all corpora their train portions combined, and evaluate on all their test portions individually (one-to-many). In sum, we report on ‘small’ models trained on each corpus individually, as well as a ‘large’ one trained on them combined, for each test set individually.
For every experiment, hyper-parameter tuning was conducted through an exhaustive grid search, using nested cross-validation (with ten inner and three outer folds) on the training set to find the optimal combination of the given parameters. Any model selection steps were based on the evaluation of the outer folds. The best performing model was then refitted on the full training set (90% of the data) and applied to the test set (10%). All splits (also during cross-validation) were made in a stratified fashion, keeping the label distributions across splits similar to the whole set. Footnote 17 Henceforth, all experiments in this section can be assumed to follow this setup.
The many-to-many evaluation framework intends to test Hypothesis 1 (Sect. 3.1 ), relating to language variation and cross-domain performance of cyberbullying detection. To facilitate this, we employ an initial baseline model: Scikit-learn’s (Pedregosa et al. 2011 ) Linear Support Vector Machine (SVM) (Cortes and Vapnik 1995 ; Fan et al. 2008 ) implementation trained on binary BoW features, tuned using the grid shown in Table 3 , based on Van Hee et al. ( 2018 ). Given its use in previous research, it should form a strong candidate against which to compare. To ascertain out-of-domain performance compared to this baseline, we report test score averages across all test splits, excluding the set the model was trained on (in-domain).
Consequently, we add an evaluation criterion to that of related work: when introducing a novel approach to cyberbullying detection, it should not only perform best in-domain for the majority of available corpora, but should also achieve the highest out-of-domain performance on average to classify as a robust method. It should be noted that the selected corpora for this work are not all optimally representative for the task. The tests in our experiments should, therefore, be seen as an initial proposal to improve the task evaluation.
5.2 Experiment II: gauging domain influence
In an attempt to overcome domain restrictions on language use, and to further solidify our tests regarding Hypothesis 1, we aim to improve the performance of our baseline models through changing our representations in three distinct ways: (i) merging all available training sets (as to simulate a large, diverse corpus), (ii) by aggregating instances on user-level, and (iii) using state-of-the-art language representations over simple BoW features in all settings. We define these experiments as such:
Volume and Variety Some corpora used for training are relatively small, and can thus be assumed insufficient to represent held-out data (such as the test sets). One could argue that this can be partially mitigated through simply collecting more data or training on multiple domains. To simulate such a scenario, we merge all available cyberbullying-related training splits (creating \(D_{all}\) ), which then corresponds to the one-to-many setting of the evaluation framework. The hope is that corpora similar in size or content (the Twitter sets, Ask.fm and Formspring, YouTube and Myspace) would benefit from having more (related) data available. Additionally, training a large model on its entirety facilitates a catch-all setting for assessing the average cross-domain performance of the full task (i.e. across all test sets when trained on all available corpora). This particular evaluation will be used in Experiment IV (replication) for model comparison.
Context change Practically all corpora, save for MySpace and YouTube, have annotations based on short sentences, which is particularly noticeable in Table 3 . This one-shot (i.e., based on a single message) method of classifying cyberbullying provides minimal content (and context) to work with. It does therefore not follow the definition of cyberbullying—as previously discussed in Sect. 3.2 . As a preliminary simulation Footnote 18 of adding (richer) context, we merge the profiles of \(D_{ask}\) and (batches of) \(D_{frm}\) into single context instances (creating \(C_{ask}\) and \(C_{frm}\) , see Sect. 4 ). This allows us to compare models trained on larger contexts directly to that of single messages, and evaluate how context restrictions affect performance on the task in general, as well as cross-domain.
Improving representations Pre-trained word embeddings as language representation have been demonstrated to yield significant performance gains for a multitude of NLP-related tasks (Collobert et al. 2011 ). Given the general lack of training data—including negative instances for many corpora—word features (and weightings) trained on the available data tend to be a poor reflection of the language use on the platform itself, let alone other social media platforms. Therefore, pre-trained semantic representations provide features that in theory, should perform better in cross-domain settings. We consider two off-the-shelf embedding models per language that are suitable for the task at hand: for English, averaged 200-dimensional GloVe (Pennington et al. 2014 ) vectors trained on Twitter, Footnote 19 and DistilBERT (Sanh et al. 2019 ) sentence embeddings (Devlin et al. 2018 ). Footnote 20 For Dutch, fastText embeddings (Bojanowski et al. 2017 ) trained on Wikipedia Footnote 21 and word2vec (Mikolov et al. 2013 ) embeddings Footnote 22 (Tulkens et al. 2016 ) trained on the COrpora from the Web (COW) corpus (Schäfer and Bildhauer 2012 ) embeddings. The GLoVe, fastText , and word2vec embeddings were processed using Gensim Footnote 23 (Řehůřek et al. 2010 ).
As an additional baseline for this section, we include the Naive Bayes Support Vector Machine (NBSVM) from Wang et al. ( 2012 ), which should offer competitive performance on text classification tasks. Footnote 24 This model also served as a baseline for the Kaggle challenge related to \(D_{tox}\) . Footnote 25 NBSVM uses tf \(\cdot \) idf-weighted uni and bi-gram features as input, with a minimum document frequency of 3, and corpus prevalence of 90%. The idf values are smoothed and tf scaled sublinearly ( \(1 + \log (\) tf)). These are then weighted by their log-count ratios derived from Multinomial Naive Bayes.
Tuning of both embeddings and NB representation classifiers is done using the same grid as Table 4 , however replacing C with [1, 2, 3, 4, 5, 10, 25, 50, 100, 200, 500]. Lastly, we opted for Logistic Regression (LR), primarily as this was used in the NBSVM implementation mentioned above, as well as fastText . Moreover, we found SVM using our grid to perform marginally worse using these features, and fine-tuning DistilBERT using a fully connected layer (similar to e.g. Sun et al. ( 2019 )) to yield similar performance. The embeddings were not fine-tuned for the task. While this could potentially increase performance, it complicates direct comparison to our baselines—we leave this for Experiment IV.
5.3 Experiment III: aggression overlap
In previous research using fine-grained labels for cyberbullying classification (e.g., Van Hee et al. ( 2018 )) it was observed that cyberbullying classifiers achieve the lowest error rates on blatant cases of aggression (cursing, sexual talk, and threats), an idea that was further adopted by Rosa et al. ( 2019 ). To empirically test Hypothesis 2 (see Sect. 3.2 )—related to the bias present in the available positive instances—we adapt the idea of running a profanity baseline from this previous work. However, rather than relying on look-up lists containing profane words, we expand this idea by training a separate classifier on toxicity detection ( \(D_{tox}\) ) and seeing how well this performs on our bullying corpora (and vice-versa). For the corpora with fine-grained labels, we can further inspect and compare the bullying classes captured by this model.
We argue that high test set performance overlap of a toxicity detection model with models trained on cyberbullying detection gives strong evidence of nuanced aspects of cyberbullying not being captured by such models. Notably, in line with Rosa et al. ( 2019 ), that the current operationalization does not significantly differ from the detection of online aggression (or toxicity)—and therefore does not capture actual cyberbullying. Given enough evidence, both issues should be considered as crucial points of improvement for the further development of classifiers in this domain.
5.4 Experiment IV: replicating state-of-the-art
For this experiment, we include two architectures that achieved state-of-the-art results on cyberbullying detection. As a reference neural network model for language-based tasks, we used a Bidirectional (Schuster and Paliwal 1997 ; Baldi et al. 1999 ) Long Short-Term Memory network (Hochreiter and Schmidhuber 1997 ; Gers et al. 2002 ) (BiLSTM), partly reproducing the architecture from Agrawal and Awekar ( 2018 ). We then attempt to reproduce the Convolutional Neural Network (CNN) (Kim 2014 ) used in both Rosa et al. 2018 ) and Agrawal and Awekar ( 2018 ), and the Convolutional LSTM (C-LSTM) (Zhou et al. ( 2015 ) used in Rosa et al. ( 2018 ). As Rosa et al. ( 2018 ) do not report essential implementation details for these models (batch size, learning rate, number of epochs), there is no reliable way to reproduce their work. We will, therefore, take Agrawal and Awekar ( 2018 ) their implementation for the BiLSTM and CNN as the initial setup. Given that this work is available open-source, we run the exact architecture (including SMOTE) in our Experiment I and II evaluations. The architecture-specific details are as follows:
Reproduction We initially adopt the basic implementation Footnote 26 by Agrawal and Awekar ( 2018 ): randomly initialized embeddings with a dimension of 50 (as the paper did not find significant effects of changing the dimension, nor initialization), run for 10 epochs with a batch size of 128, dropout probability of 0.25, and a learning rate of 0.01. Further architecture details can be found in our repository. Footnote 27 We also run a variant with SMOTE on, and one from the provided notebooks directly. Footnote 28 This and following neural models were run on an NVIDIA Titan X Pascal, using Keras (Chollet et al. 2015 ) with Tensorflow (Abadi et al. 2015 ) as backend.
BiLSTM For our own version of the BiLSTM, we minimally changed the architecture from Agrawal and Awekar ( 2018 ), only tuning using a grid on batch size [32, 64, 128, 256], embedding size [50, 100, 200, 300], and learning rate [0.1, 0.01, 0.05, 0.001, 0.005]. Rather than running for ten epochs, we use a validation split (10% of the train set) and initiate early stopping when the validation loss does not go down after three epochs. Hence—and in contrast to earlier experiments—we do not run the neural models in 10-fold cross-validation, but a straightforward 2-fold train and test split where the latter is 10% of a given corpus. Again, we are predominantly interested in confirming statements made in earlier work; namely, that for this particular setting tuning of the parameters does not meaningfully affect performance.
CNN We use the same experimental setup as for the BiLSTM. The implementations of Agrawal and Awekar ( 2018 ); Rosa et al. ( 2018 ) use filter window sizes of 3, 4, and 5—max pooled at the end. Given that the same grid is used, the word embedding sizes are varied and weights trained (whereas Rosa et al. ( 2018 ) use 300-dimensional pre-trained embeddings). Therefore, for direct performance comparisons, Agrawal and Awekar ( 2018 ) their results will be used as a reference. As CNN-based architectures for text classification are often also trained on character level, we include a model variant with this input as well.
C-LSTM For this architecture, we take an open-source text classification survey implementation. Footnote 29 This uses filter windows of [10, 20, 30, 40, 50], 64-dimensional LSTM cells and a final 128 dimensional dense layer. Please refer to our repository for additional implementation details—for this and previous architectures.
5.5 Experiment V: crowdsourced data
Following up on the proposed shortcomings of the currently available corpora in Hypotheses 1 and 2, we propose the use of a crowdsourcing approach to data collection. In this experiment, we will repeat Experiment I and II with the best out-of-domain classifier from the above evaluations with three (Dutch Footnote 30 ) datasets: \(D_{ask\_nl}\) ; the Dutch part of the Ask.fm dataset used before, \(D_{sim\_nl}\) ; our synthetic, crowdsourced cyberbullying data, and lastly \(D_{don\_nl}\) ; a small donated cyberbullying test set with messages from various platforms (full overview and description of these three can be found in Sect. 4 ). The only notable difference to our setup for this experiment is that we never use \(D_{don\_nl}\) as training data. Therefore rather than \(D_{all}\) , the Ask.fm corpus is merged with the crowdsourced cyberbullying data to make up the \(D_{comb}\) set.
6 Results and discussion
We will now cover results per experiment, and to what extent these provide support for the hypotheses posed in Sect. 3 . As most of these required backward evaluation (e.g., Experiment III was tested on sets from Experiment I), the results of Experiment I-III are compressed in Table 5 . Table 7 comprises the Improving Representations part of Experiment II (under ‘word2vec’ and ‘DistilBERT’) along with the preprocessing results effect of our baselines. The results of Experiment V can be found in Table 8 . For brevity of reporting, the latter two only report on the in-domain scores, and feature the out-of-domain averages for the \(D_{all}\) models for comparison, and \(D_{tox}\) averages in Table 7 .
6.1 Experiment I
Looking at Table 5 , the upper group of rows under T1 represents the results for Experiment I. We posed in Hypothesis 1 that samples are underpowered regarding their representation of the language variation between platforms, both for bullying and normal language use. The data analysis in Sect. 4.5 showed minimal overlap between domains in vocabulary and notable variances in numerous aspects of the available corpora. Consequently, we raised doubts regarding the ability of models trained on these individual corpora to generalize to other corpora (i.e., domains).
Firstly, we consider how well our baseline performed on the in-domain test sets . For half of the corpora, it achieves the highest performance on these specific sets (i.e., the test set portion of the data the model was trained on). More importantly, this entails that for four of the other sets, models trained on other corpora perform equal or better. Particularly the effectiveness of \(D_{ask}\) was in some cases surprising; the YouTube corpus by Dadvar et al. ( 2014 ) ( \(D_{ytb}\) ), for example, contains much longer instances (see Table 2 ).
It must be noted though, that the baseline was selected from work on the Ask.fm corpus (Van Hee et al. 2018 ). This data is also one of the more diverse datasets (and largest) with exclusively short messages; therefore, one could assume a model trained on this data would work well on both longer and shorter instances. It is however also likely that particularly this baseline (binary word features) trained on this data therefore enforces the importance of more shallow features. This will be further explored in Experiments II and III.
For Experiment I, however, our goal was to assess the out-of-domain performance of these classifiers, not to maximize performance. For this, we turn to the Avg column in Table 5 . Between the top portion of the Table, the \(D_{ask}\) model performs best across all domains (achieving highest on three, as mentioned above). The second-best model is trained on the Formspring data from Reynolds et al. ( 2011 ) ( \(D_{frm}\) ), akin to Ask.fm as a domain (question-answer style, option to post anonymously). It can be observed that almost all models perform worst on the ‘bullying traces’ Twitter corpus by Xu et al. ( 2012 ), which was collected using queries. This result is relatively unsurprising, given the small vocabulary overlaps with its test set shown in Fig. 2 . We also confirm in line with Reynolds et al. ( 2011 ) that the caw data from Bayzick et al. ( 2011 ) is unfit as a bullying corpus; achieving significant positive \(F_1\) -scores with a baseline, generalizing poorly and proving difficult as a test set.
Additionally, we observe that even the best performing models yield between .1 and .2 lower \(F_1\) scores on other domains, or a \(15-30\%\) drop from the original score. To explain this, we look at how well important features generalize across test sets. As our baseline is a Linear SVM, we can directly extract all grams with positive coefficients (i.e., related to bullying). Figure 3 (right) shows the frequency of the top 5000 features with the highest coefficient values. These can be observed to follow a Zipfian-like distribution, where the important features most frequently occur in one test set ( \(25.5\%\) ) only, which quickly drops off with increasing frequency. Conversely, this implies that over \(75\%\) of the top 5,000 features seen during training do not occur in any test instance, and only \(3\%\) generalize across all sets. This coverage decreases to roughly \(60\%\) and \(4\%\) respectively for the top 10,000, providing further evidence of the strong variation in predominantly bullying-specific language use.
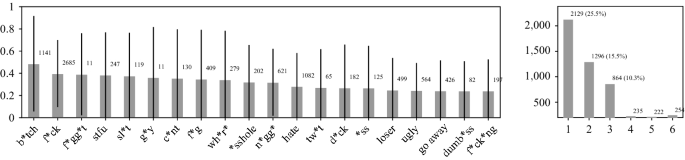
Left: Top 20 test set words with the highest average coefficient values across all classifiers (minus the model trained on \(D_{tox}\) ). Error bars represent standard deviation. Each coefficient value is only counted once per test set. The frequency of the words is listed in the annotation. Right: Test set occurrence frequencies (and percentages) of the top 5000 highest absolute feature coefficient values
Figure 3 (left) also indicates that the coefficient values are highly unstable across test sets, with most having roughly a 0.4 standard deviation. Note that these coefficient values can also flip to negative for particular sets, so for some of the features, the range goes from associated with the other class to highly associated with bullying. Given the results of Table 5 and Fig. 3 , we can conclude that our baseline model shows not to generalize out-of-domain. Given the quantitative and qualitative results reported on in this Experiment, this particular setting partly supports Hypothesis 1.
6.2 Experiment II
The results for this experiment can be predominantly found in Table 5 (middle and lower parts, and T2 in particular), and partly in Table 7 (word2vec, DistilBERT). In this experiment, we seek to further test Hypothesis 1 by employing three methods: merging all cyberbullying data to increase volume and variety , aggregating on context level for a context change , and improving representations through pre-trained word embedding features. These are all reasonably straightforward methods that can be employed in an attempt to mitigate data scarcity.
Volume and variety The results for this part are listed under \(D_{all}\) in Table 5 . For all of the following experiments, we now focus on the full results table (including that of Experiment I) and see which individual classifiers generalize best across all test sets (highlighted in gray). The Avg column shows that our ‘big’ model trained on all available corpora Footnote 31 achieves second-best performance on half of the test sets and best on the other half. More importantly, it has the highest average out-of-domain performance, without competition on any test set. These observations imply that for the baseline setting, an ensemble model of different smaller classifiers should not be preferred over the big model. Consequently, it can be concluded that collecting more data does seem to aid the task as a whole.
However, a qualitative analysis of the predictions made by this model clearly shows lingering limitations (see Table 6 ). These three randomly-picked examples give a clear indication of the focus on blatant profanity (such as d*ck , p*ss , and f*ck ). Especially combinations of words that in isolation might be associated with bullying content ( leave , touch ) tend to confuse the model. It also fails to capture more subtle threats ( skull drag ) and infrequent variations ( h* ). Both of these structural mistakes could be mitigated by providing more context that potentially includes either more toxicity or more examples of neutral content to decrease the impact of single curse words—hence, the next experiment.
Context change As for access to context scopes, we are restricted to the Ask.fm and Formspring data ( \(C_{frm}\) and \(C_{ask}\) in Table 5 ). Nevertheless, in both cases, we see a noticeable increase for in-domain performance: a positive \(F_1\) score of .579 for context scope versus .561 on Ask.fm, and .758 versus .454 on Formspring respectively. This increase implies that considering message-level detection for both individual sets should be preferred. On the other hand, however, these longer contexts do perform worse on out-of-domain sets. This can be partly explained due the fact that including more data (therefore moving the data to profile, or conversation level) shifts the task to identifying bullying conversations, or profiles of victims. While variation will be higher, chances also increase that multiple single bullying messages will be captured in a one context. This would therefore allow to learn the distinction between a profile or conversation with predominately neutral messages including a single toxic message—which might therefore be harmless, to one where there are multiple toxic messages, increasing the severity.
The change in scope clearly influences which features are deemed important. On manual inspection, averaging feature importances of all baseline models on their in-domain test sets, the top 500 most important features consist for 63% of profane words. For the models trained on Ask.fm and Formspring specifically ( \(D_{ask}\) and \(D_{frm}\) ), this is an average of 42%. Strikingly, for the models trained on context scopes ( \(C_{frm}\) and \(C_{ask}\) ), this percentage significantly reduced to 11%; many of their important bi-gram features include you , topics such as dating , boys , girls , and girlfriend occur, yet also positive words such as ( are ) beautiful —the latter of which could indicate messages from friends (defenders). This change is to an extent expected as by changing the scope, the task shifts to classifying profiles that are bullied, thus showing more diverse bullying characteristics.
These results provide evidence for extending classification to contexts to be a worthwhile platform-specific setting to pursue. However, we can conversely draw the same conclusions as Experiment I; that including direct context does not overcome the tasks general domain limitations, therefore further supporting Hypothesis 1. A plausible solution to this could be improving upon the BoW features by relying on more general representations of language, as found in word embeddings.
Improving representations The aim of this experiment was to find (out-of-the-box) representations that would improve upon the simple BoW features used in our base-line model (i.e., achieving good in-domain performance as well as out-of-domain generalization). Table 7 lists both of our considered baselines, tested under different preprocessing methods. These are subsequently compared against the two different embedding representations.
For preprocessing, several levels were used: the default for all models being (1) lowercasing only, then either (2) removal of special characters, or (3) lemmatization and more appropriate handling of special characters (e.g., splitting #word to prepend a hashtag token) were added. The corresponding results in Table 7 do not reveal an unequivocal preprocessing method for either the BoW baseline or NBSVM. While the latter achieves highest out-of-domain generalization with thorough preprocessing (‘+preproc’, .566 positive \(F_1\) ), the baseline model achieves best in-domain performance on five out of nine corpora, and an on-par out-of-domain average (.566 versus .561) with simple cleaning (‘+clean’).
According to our criterion proposed in Sect. 5.1 , a method that performs well both in- and out-of-domain should be preferred. The current consideration of preprocessing methods illustrates how this stricter evaluation criterion used in this experiment potentially yields different overall results in contrast to evaluating in-domain only, or focusing on single corpora. Conversely, we opted for simple cleaning throughout the rest of our experiment (as mentioned in Sect. 4.4 ), given its consistent performance for both baselines.
The embeddings chosen for this experiment do not seem to provide representations that yield an overall improvement for the classification performance of our Logistic Regression model. Surprisingly, however, DistilBERT does yield significant gains over our baseline for the conversation-level corpus of Ask.fm (.629 positive \(F_1\) over .579). This might imply that such representations would work well on more (balanced) data. While we did not see a significant effect on performance with shallowly fine-tuning DistilBERT, more elaborate fine-tuning would be a required point of further investigation before drawing strong conclusions.
Moreover, given that we restricted our embeddings to averaged representations on document-level for word2vec, and the sentence representation token for BERT (following common practices), numerous settings remain unexplored. While both (i.e., fine-tuning and alternate input representations) of such potential improvements would certainly merit further exploration in future work focused on optimization, this is out of scope of the current research. Similarly, embeddings trained on a similar domain would be more ideal to represent our noisy data; we settled for strictly off-the-shelf ones that at minimum included web content, and a large vocabulary.
Therefore, we conclude that no alternative (out-of-the-box) baselines seem to clearly outperform our BoW baseline. We previously eluded to the effectiveness of binary BoW representations in previous work, and argued this being a result of capturing blatant profanity. We will further test this in the next experiment.
6.3 Experiment III
Here, we investigate Hypothesis 2: the notion that positive instances across all cyberbullying corpora are biased, and only reflect a limited dimension of bullying. We have already found strong evidence for this in the previous Experiments I and II, Fig. 3 , Table 6 , and manual analyses of top features all indicated toxicity to be consistent top-ranking features. To add more empirical evidence to this, we trained models on toxicity, or cyber aggression, and tested them on bullying data (and vice-versa)—providing results on the overlap between the tasks. The results for this experiment can be found in the lower end of Table 5 , under \(D_{tox}\) and T3.
It can be noted that there is a substantial gap in performance between the cyberbullying classifiers (using \(D_{all}\) as reference) performance on the \(D_{tox}\) test set and that of the toxicity model (positive \(F_1\) score of .587 and .806 respectively). More strikingly, however, the other way around, toxicity classifiers perform second-best on the out-of-domain averages ( Avg in Table 5 ). In the context scopes ( \(C_{frm}\) and \(C_{ask}\) ) it is notably close, and for other sets relatively close, to the in-domain performance.
Cyberbullying detection should include detection of toxic content, yet also perform on more complex social phenomena, likely not found in the Wikipedia comments of the toxicity corpus. It is therefore particularly surprising that it achieves higher out-of-domain performance on cyberbullying classification than all individual models using BoW features to capture bullying content. Only when all corpora are combined, the \(D_{all}\) classifier performs better than the toxicity model. This observation combined with previous results provides significant evidence that a large part of the available cyberbullying content is not complex, and current models to only generalize to a limited extent using predominately simple aggressive features, supporting Hypothesis 3.
6.4 Experiment IV
So far, we have attempted to improve a straight-forward baseline that was trained on binary features with several different approaches. While changes in data (representations) seem to have a noticeable effect on performance (increasing the amount of messages per instance, merging all corpora), none of the experiments with different feature representations have had an impact. With the current experiment, we had hoped to leverage earlier state-of-the-art architectures by reproducing their methodology and subjecting our evaluation framework.
As can be inferred from Table 8 , our baselines outperform these neural techniques on almost all in-domain tests, as well as the out-of-domain averages. Having strictly upheld the experimental set-up from Agrawal and Awekar ( 2018 ) and as close as possible that of Rosa et al. ( 2018 ), we can conclude that—under stricter evaluation—there is sufficient evidence that these models do not provide state-of-the-art results on the task of cyberbullying. Footnote 32 Tuning these networks (at least in our set-up) does not seem to improve performance, rather decrease it. This indicates that the validation set on which early stopping is conducted is often not representative to the test set. Parameter tuning on this set is consequently sensitive to overfitting; an arguably unsurprising result given the size of the corpora.
Some further noteworthy observations can be made related to the performance of the CNN architecture, achieving quite significant leaps on word level (for \(D_{twB}\) ) and character level (for \(C_{ask}\) ). Particularly the conversation scopes ( C , with a comparitatively balanced class distribution) see much more competitive perfomance compared to the baselines. The same effect can be observed when more data is available; both averages test scores for \(D_{all}\) and \(D_{tox}\) are comparable to the baseline across almost all architectures. Additionally, the \(D_{tox}\) scores indicate that all architectures show about the same overlap on toxicity detection, although interestingly, less so for the neural models than for the baselines.
It can therefore be concluded that the current neural architectures do not provide a solution to the limitations of the task, rather, suffering more in performance. Our experiments do, however, once more illustrate that the proposed techniques of improving the representations of the corpora (by providing more data through merging all sources, and balancing by classifying batches of multiple messages, or conversations) allow the neural models to approach the baseline ballpark. As our goal here was not to completely optimize these architectures, but replication, the proposed techniques still could provide more avenues for further research. Finally, given its robust performance, we will continue to use the baseline model for the next experiment.
6.5 Experiment V
Due to the nature of its experimental set-up (which generates balanced data with simple language use, as shown in Table 2 ), the crowdsourced data proves easy to classify. Therefore, we do not report out-of-domain averages, as this set would skew them too optimistically, and be uninformative. Regardless, we are primarily interested in performance when crowdsourced data is added, or used as a replacement for real data. In contrast to the other experiments, the focus will mostly be on the Ask.fm ( \(D_{ask\_nl}\) ) and donated ( \(D_{don\_nl}\) ) scores (see Table 8 ). The scores on the Dutch part of the Ask.fm corpus are quite similar to those on the English corpus (.561 vs .598 positive \(F_1\) score), which is in line with earlier results (Van Hee et al. 2018 ). Moreover, particularly for the small amount of data, the crowdsourced corpus performs surprisingly well on \(D_{ask\_nl}\) (.516), and significantly better on the donated test data (.667 on \(D_{don\_nl}\) ). This implies that a balanced, controlled bullying set, tailored to the task specifically, does not need a significant amount of data to achieve comparable (or even better) performance, which is a promising result.
Furthermore, in the settings that utilize context representations, training on conversation scopes initially does not seem to improve detection performance in any of the configurations (save for a marginal gain on \(D_{simC\_nl}\) ). However, it does simplify the task in a meaningful way at test-time; whereas a slight gain is obtained for message-level \(D_{ask\_nl}\) (from .598 \(F_1\) -score to .608), when merging both datasets a significant performance boost can be found when training on \(D_{comb}\) and testing on \(D_{askC\_nl}\) (from .264 and .501 to .801 on the combined). Hence, it can be further concluded that enriching the existing training set with crowdsourced data yields meaningful improvements.
Based on these results, we confirm the Experiment II results hold for Dutch: more diverse, larger datasets, and increasing context sizes contributes to better performance on the task. Most importantly, there is enough evidence to support Hypothesis 3: the data generated by the crowdsourcing experiment helps detection rates for our in-the-wild test set, and its combination with externally collected data increases performance with and without additional context. These results underline the potential of this approach to collecting cyberbullying data.
6.6 Suggestions for future work
We hope our experiments have helped to shed light on, and raise further attention to multiple issues with methodological rigor pertaining the task of cyberbullying detection. It is our understanding that the disproportionate amount of work on the (oversimplified) classification task, versus the lack of focus on constructing rich, representative corpora reflecting the actual dynamics of bullying, has made critical assessment of the advances in this task difficult. We would therefore want to particularly stress the importance of simple baselines and the out-of-domain tests that we included in the evaluation criterion for this research. They would provide a fairer comparison for proposed novel classifiers, and a more unified method of evaluation. In line with this, the structural inclusion of domain adaptation techniques seems a logical next step to improve cross-domain performance, specifically those tailored to imbalanced data.
Furthermore, this should be paired with a critical view on the extent to which the full scope of the task is fulfilled. Novel research would benefit from explicitly finding evidence to support its assumptions that classifiers labeled ‘cyberbullying detection’ do more than one-shot, message-level toxicity detection. We would argue that the current framing of the majority of work on the task is still too limited to be considered theoretically-defined cyberbullying classification. In our research, we demonstrated several qualitative and quantitative methods that can facilitate such analyses. As popularity of the application of cyberbullying detection grows, this would avoid misrepresenting the conducted work, and that of in-the-wild applications in the future.
We can imagine these conclusions to be relevant for more research within the computational forensics domain: detection of online pedophilia (Bogdanova et al. 2014 ), aggression and intimidation (Escalante et al. 2017 ), terrorism and extremism (Ebrahimi et al. 2016 ; Kaati et al. 2015 ), and systematic deception Feng et al. ( 2012 )–among others. These are all examples of heavily skewed phenomena residing within more complex networks for which simplification could lead to serious misrepresentation of the task. As with cyberbullying research, a critical evaluation of multiple domains could potentially uncover problematic performance gaps.
While we demonstrated a method of collecting plausible cyberbullying with guaranteed consent, the more valuable sources of real-world data that allow for complex models of social interaction remain restricted. It is our expectation that future modeling will benefit from the construction of much larger (anonymized) corpora—as most fields dealing with language have, and we therefore hope to see future work heading this direction.
7 Conclusion
In this work, we identified several issues that affect the majority of the current research on cyberbullying detection. As it is difficult to collect accurate cyberbullying data in the wild, the field suffers from data scarcity. In an optimal scenario, rich representations capturing all required meta-data to model the complex social dynamics of what the literature defines as cyberbullying would likely prove fruitful. However, one can assume such access to remain restricted for the time being, and with current social media moving towards private communication, to not be generalizable in the first place. Thus, significant changes need to be made to the empirical practices in this field. To this end, we provided a cross-domain evaluation setup and tested several cyberbullying detection models, under a range of different representations to potentially overcome the limitations of the available data, and provide a fair, rigorous framework to facilitate direct model comparison for this task.
Additionally, we formed three hypotheses we would expect to find evidence for during these evaluations: (1) the corpora are too small and heterogeneous to represent the strong variation in language use for both bullying and neutral content across platforms accurately, (2) the positive instances are biased, predominantly capturing toxicity, and no other dimensions of bullying, and finally (3) crowdsourcing poses a resource to generate plausible cyberbullying events, and that can help expand the available data and improve the current models.
We found evidence for all three hypotheses: previous cyberbullying models generalize poorly across domains, simple BoW baselines prove difficult to improve upon, there is considerable overlap between toxicity classification and cyberbullying detection, and crowdsourced data yields well-performing cyberbullying detection models. We believe that the results of Hypotheses 1 and 2 in particular are principal hurdles that need to be tackled to advance this field of research. Furthermore, we showed that both leveraging training data from all openly available corpora, and shifting representations to include context meaningfully improves performance on the overall task. Therefore, we believe both should be considered as an evaluation point in future work. More so given that we show that these do not solve the existing limitations of the currently available corpora, and could therefore provide avenues for future research focusing on collecting (richer) data. Lastly, we show reproducibility of models that previously demonstrated state-of-the-art performance on this task to fail. We hope that the observations and contributions made in this paper can aid to improve rigor in future cyberbullying detection work.
Survey conducted in 2011 among 799 American teens. Black and Latino families were oversampled.
http://www.pewinternet.org .
Available at https://github.com/cmry/amica .
Unfortunately, numerous (recent) work on cyberbullying detection seems not to report such \(F_1\) -scores (in favor of accuracy), is limited to criticized datasets with high baseline scores (such as the caw datasets) or does not show enough methodological rigor—some are therefore not included in this overview.
Data has been made available at http://caw2.barcelonamedia.org .
Data has been made available at http://www.chatcoder.com/DataDownload .
Data has been made available at http://www.ub-web.de/research .
Data has been made available at http://research.cs.wisc.edu/bullying/data.html .
One very recent exception to the latter can be found in Cheng et al. ( 2020 ). Their work introduces a novel domain adaptation technique, and demonstrates it to increase performance on two text classification tasks, one being cyberbullying detection.
http://www.amicaproject.be .
https://osf.io/rgqw8/ .
These were collected as complete as possible. Twitter, in particular, has low recall; only an approximate of 60% of the tweets were retrieved. Such numbers are expected given the classification problem; people tend to remove harassing messages as was shown before by Xu et al. ( 2012 ).
http://www.socialengine.com .
From: https://www.kaggle.com/c/jigsaw-toxic-comment-classification-challenge .
See https://meta.wikimedia.org/wiki/Research:Detox/Data_Release for more information regarding operationalization of this dataset.
https://spacy.io ( v2.0.5 ).
Indices (similar to any other random components) were fixed by the same seed for all experiments.
Preferably, one would want to collect data on profile level by design. The corpora available were not specifically collected this way, making our set-up an approximation of such a setting.
https://nlp.stanford.edu/projects/glove/ ( v1.2 ).
https://github.com/huggingface/transformers ( 1d646ba ).
https://github.com/facebookresearch/fastText/blob/master/pretrained-vectors.md ( 2665eac ).
https://github.com/clips/dutchembeddings ( 1e3d528 ).
https://radimrehurek.com/gensim/index.html ( v3.4 ).
The implementations for these models can be found in our repository.
https://kaggle.com/jhoward/nb-svm-strong-linear-baseline/notebook .
https://github.com/sweta20/Detecting-Cyberbullying-Across-SMPs/blob/master/DNNs.ipynb .
https://github.com/cmry/amica/blob/master/neural.py .
Note this is for testing reproduction only, as it is not subjected to the same evaluation framework.
https://github.com/bicepjai/Deep-Survey-Text-Classification/ .
On account of the synthetic data being available in Dutch only. Experiment III was not repeated as there is no equivalent toxicity dataset available in this language.
This average excludes toxicity data from \(D_{tox}\) , which we found when added to substantially decrease performance on all domains, except for \(D_{twX}\) and \(C_{frm}\) . Note that it also includes scopes from the context change experiment.
Upon acquiring the results of the replication of Agrawal and Awekar ( 2018 ) (in particular failing to replicate the effect of the paper’s oversampling) we investigated the provided code and notebooks. It is our understanding that oversampling before splitting the dataset into training and test sets causes the increase in performance; we measured overlap of positive instances in these splits and found no unique test instances. Furthermore, after re-running the experiments directly from the notebooks with the oversampling conducted post-split, the effect was significantly decreased (similar to our results in Table 7 ). The authors were contacted with our observations in March 2019, and have since confirmed our results. Our analyses can be found here: https://github.com/cmry/amica/tree/master/reproduction .
Abadi, M., Agarwal, A., Barham, P., Brevdo, E., Chen, Z., Citro, C., Corrado, G.S., Davis, A., Dean, J., Devin, M., Ghemawat, S., Goodfellow, I., Harp, A., Irving, G., Isard, M., Jia, Y., Jozefowicz, R., Kaiser, L., Kudlur, M., Levenberg, J., Mané, D., Monga, R., Moore, S., Murray, D., Olah, C., Schuster, M., Shlens, J., Steiner, B., Sutskever, I., Talwar, K., Tucker, P., Vanhoucke, V., Vasudevan, V., Viégas, F., Vinyals, O., Warden, P., Wattenberg, M., Wicke, M., Yu, Y., & Zheng, X. (2015). TensorFlow: Large-scale machine learning on heterogeneous systems. http://tensorflow.org/ . Software available from tensorflow.org.
Agrawal, S., & Awekar, A. (2018). Deep learning for detecting cyberbullying across multiple social media platforms. In G. Pasi, B. Piwowarski, L. Azzopardi, & A. Hanbury (Eds.), Advances in information retrieval (pp. 141–153). Cham: Springer International Publishing.
Chapter Google Scholar
Baldi, P., Brunak, S., Frasconi, P., Soda, G., & Pollastri, G. (1999). Exploiting the past and the future in protein secondary structure prediction. Bioinformatics , 15 (11), 937–946.
Article Google Scholar
Bayzick, J., Kontostathis, A., & Edwards, L. (2011). Detecting the presence of cyberbullying using computer software. In Proceedings of the 3rd international web science conference . WebSci11; 2011.
Beran, T., & Li, Q. (2008). The relationship between cyberbullying and school bullying. The Journal of Student Wellbeing , 1 (2), 16–33.
Bessiere, K., Kiesler, S., Kraut, R., & Boneva, B. S. (2008). Effects of internet use and social resources on changes in depression. Information, Community & Society , 11 (1), 47–70.
Binns, A. (2013). Facebook’s ugly sisters: Anonymity and abuse on formspring and ask. fm. Media Education Research Journal
Blitzer, J., Dredze, M., & Pereira, F. (2007). Biographies, bollywood, boom-boxes and blenders: Domain adaptation for sentiment classification. In Proceedings of the 45th annual meeting of the association of computational linguistics (pp. 440–447).
Bogdanova, D., Rosso, P., & Solorio, T. (2014). Exploring high-level features for detecting cyberpedophilia. Computer Speech & Language , 28 (1), 108–120.
Bojanowski, P., Grave, E., Joulin, A., & Mikolov, T. (2017). Enriching word vectors with subword information. Transactions of the Association for Computational Linguistics , 5 , 135–146.
Bretschneider, U., Wöhner, T., & Peters, R. (2014). Detecting Online Harassment in Social Networks. In 35th International Conference on Information Systems Li 2007 (pp. 1–14).
Broadcasting Standards Authority: What not to swear: The acceptability of words in broadcasting. Retrieved March 03, 2016 from, http://bsa.govt.nz/images/assets/Research/Acceptability_of_Words_2013_WEB.pdf (2013).
Broeck, E., Poels, K., Vandebosch, H., & Royen, K. (2014). Online perspective-taking as an intervention tool against cyberbullying. Annual review of cybertherapy and telemedicine (pp. 113–117).
Chan, Y.S., & Ng, H.T. (2006). Estimating class priors in domain adaptation for word sense disambiguation. In Proceedings of the 21st International Conference on Computational Linguistics and the 44th annual meeting of the Association for Computational Linguistics (pp. 89–96). Association for Computational Linguistics.
Chen, M., Xu, Z., Weinberger, K., & Sha, F. (2012). Marginalized denoising autoencoders for domain adaptation. arXiv preprint arXiv:1206.4683 .
Cheng, L., Guo, R., Candan, K.S., & Liu, H. (2020). Representation learning for imbalanced cross-domain classification. In Proceedings of the 2020 SIAM international conference on data mining . (pp. 478–486). SIAM.
Cheng, L., Guo, R., Silva, Y., Hall, D., & Liu, H. (2019). Hierarchical attention networks for cyberbullying detection on the instagram social network. In Proceedings of the 2019 SIAM international conference on data mining (pp. 235–243). SIAM.
Chollet, F., et al. (2015). Keras. https://keras.io .
Collobert, R., Weston, J., Bottou, L., Karlen, M., Kavukcuoglu, K., & Kuksa, P. (2011). Natural language processing (almost) from scratch. Journal of Machine Learning Research , 12 , 2493–2537.
Google Scholar
Cortes, C., & Vapnik, V. (1995). Support-vector networks. Machine Learning , 20 (3), 273–297. https://doi.org/10.1007/BF00994018 .
Dadvar, M. (2014). Experts and machines united against cyberbullying. Ph.D. thesis, University of Twente, Netherlands. https://doi.org/10.3990/1.9789036537391 .
Dadvar, M., Trieschnigg, D., de Jong, F. (2014). Experts and machines against bullies: A hybrid approach to detect cyberbullies. In Canadian conference on artificial intelligence (pp. 275–281). Springer.
Daumé III, H. (2009). Frustratingly easy domain adaptation. arXiv preprint arXiv:0907.1815 .
DeHue, F., Bolman, C., & Völlink, T. (2008). Cyberbullying: Youngsters’ experiences and parental perception. CyberPsychology & Behavior , 11 (2), 217–223.
Devlin, J., Chang, M.W., Lee, K., & Toutanova, K. (2018). Bert: Pre-training of deep bidirectional transformers for language understanding. arXiv preprint arXiv:1810.04805 .
Dinakar, K., Reichart, R., & Lieberman, H. (2011). Modeling the detection of textual cyberbullying. the social mobile web. In 5th international AAAI conference on weblogs and social media, Barcelona, Catalonia, Spain .
Duggan, M. (2015). Mobile messaging and social media 2015 . Washington, D.C: Pew Research Center.
Ebrahimi, M., Suen, C. Y., & Ormandjieva, O. (2016). Detecting predatory conversations in social media by deep convolutional neural networks. Digital Investigation , 18 , 33–49.
Escalante, H. J., Villatoro-Tello, E., Garza, S. E., López-Monroy, A. P., Montes-y Gómez, M., & Villaseñor-Pineda, L. (2017). Early detection of deception and aggressiveness using profile-based representations. Expert Systems with Applications , 89 , 99–111.
Fan, R. E., Chang, K. W., Hsieh, C. J., Wang, X. R., & Lin, C. J. (2008). LIBLINEAR: A Library for Large Linear Classification. The Journal of Machine Learning , 9 (2008), 1871–1874. https://doi.org/10.1038/oby.2011.351 . http://dl.acm.org/citation.cfm?id=1442794 .
Feng, S., Banerjee, R., & Choi, Y. (2012). Syntactic stylometry for deception detection. In Proceedings of the 50th annual meeting of the association for computational linguistics (Volume 2: Short Papers) (pp. 171–175).
Finkel, J.R., & Manning, C.D. (2009). Hierarchical bayesian domain adaptation. In Proceedings of human language technologies: The 2009 annual conference of the North American Chapter of the Association for Computational Linguistics (pp. 602–610).
Gers, F. A., Schraudolph, N. N., & Schmidhuber, J. (2002). Learning precise timing with lstm recurrent networks. Journal of Machine Learning Research , 3 , 115–143.
Glorot, X., Bordes, A., & Bengio, Y. (2011). Domain adaptation for large-scale sentiment classification: A deep learning approach.
Hee, C.V., Lefever, E., Verhoeven, B., Mennes, J., Desmet, B., Pauw, G.D., & Daelemans, W. (2015). Detection and Fine-Grained Classification of Cyberbullying Events. In International conference recent advances in natural language processing (RANLP) (pp. 672–680).
Hochreiter, S., & Schmidhuber, J. (1997). Long short-term memory. Neural Computation , 9 (8), 1735–1780.
Honnibal, M., & Montani, I. (2017). spaCy 2: Natural language understanding with Bloom embeddings, convolutional neural networks and incremental parsing. To appear.
Hosseinmardi, H., Mattson, S.A., Rafiq, R.I., Han, R., Lv, Q., Mishra, S. (2015). Prediction of cyberbullying incidents on the Instagram social network. In MobiSys, (p. 2014). arxiv:1503.03909 .
Jiang, J., & Zhai, C. (2007). A two-stage approach to domain adaptation for statistical classifiers. In Proceedings of the sixteenth ACM conference on Conference on information and knowledge management (pp. 401–410).
Kaati, L., Omer, E., Prucha, N., & Shrestha, A. (2015). Detecting multipliers of jihadism on twitter. In 2015 IEEE international conference on data mining workshop (ICDMW) (pp. 954–960). IEEE.
Kann, L., Kinchen, S., Shanklin, S. L., Flint, K. H., Kawkins, J., Harris, W. A., et al. (2014). Youth risk behavior surveillance-united states, 2013. Morbidity and Mortality Weekly Report: Surveillance Summaries , 63 (Suppl 4), 1–168.
Kim, Y. (2014). Convolutional neural networks for sentence classification. arXiv preprint arXiv:1408.5882 .
Kontostathis, A., Edwards, L., & Leatherman, A. (2010). Text mining and cybercrime. Text mining: Applications and theory. John Wiley & Sons, Ltd, Chichester, UK (pp. 149–164).
Kontostathis, A., Reynolds, K. (2013). Detecting cyberbullying: Query terms and techniques. In Proceedings of the 3rd annual ACM web science conference , WebSci 2013 (2013) (pp. 195–204).
Kowalski, R. M., Limber, S. P., & Agatston, P. W. (2012). Cyberbullying: Bullying in the digital age . John Wiley & Sons.
Lenhart, A., Madden, M., Smith, A., Purcell, K., Zickuhr, K., & Rainie, L. (2011). Teens, kindness and cruelty on social network sites: How american teens navigate the new world of. Pew Internet & American Life Project.
Li, S., Ju, S., Zhou, G., & Li, X. (2012). Active learning for imbalanced sentiment classification. In Proceedings of the 2012 Joint conference on empirical methods in natural language processing and computational natural language learning (pp. 139–148). Association for Computational Linguistics.
Livingstone, S., Haddon, L., Görzig, A., & Ólafsson, K. (2011). EU Kids Online II: Final Report 2011. Tech. rep., EU Kids Online. http://www.lse.ac.uk/collections/EUKidsOnline/ (p. 293).
Ma, J., Zhang, Y., & Zhu, J. (2014). Tagging the web: Building a robust web tagger with neural network. In Proceedings of the 52nd annual meeting of the association for computational linguistics (Vol. 1: Long Papers) (pp. 144–154).
Madden, M., Lenhart, A., Cortesi, S. (2013). Teens, social media, and privacy. Tech. rep., Pew Internet. http://www.lateledipenelope.it/public/52dff2e35b812.pdf .
McClosky, D., Charniak, E., Johnson, M.: Reranking and self-training for parser adaptation. In Proceedings of the 21st international conference on computational linguistics and the 44th annual meeting of the Association for Computational Linguistics (pp. 337–344). Association for Computational Linguistics.
Mikolov, T., Chen, K., Corrado, G., & Dean, J. (2013). Efficient estimation of word representations in vector space. arXiv preprint arXiv:1301.3781 .
Mikolov, T., Sutskever, I., Chen, K., Corrado, G.S., & Dean, J. (2013). Distributed representations of words and phrases and their compositionality. In Advances in neural information processing systems (pp. 3111–3119).
Millwood-Hargreave, A. (2000). Delete expletives? research undertaken jointly by the advertising standards authority, british broadcasting corporation, broadcasting standards commission and the independent television commission . London: ASA, BBC, BSC and ITC.
Nahar, V., Li, X., & Pang, C. (2013). An effective approach for cyberbullying detection. Communications in Information Science and Management Engineering , 3 (May), 238–247.
Noswearing.com: Bad Word List & Swear Filter. Retrieved March 03, 2016 from, http://www.noswearing.com (2016).
Ólafsson, K., Livingstone, S., & Haddon, L. (2013). Children’s Use of Online Technologies in Europe: A review of the European evidence base . EU Kids Online: Tech. Rep. May.
Pan, S.J., Ni, X., Sun, J.T., Yang, Q., & Chen, Z. (2010). Cross-domain sentiment classification via spectral feature alignment. In Proceedings of the 19th international conference on World wide web (pp. 751–760).
Pedregosa, F., Varoquaux, G., Gramfort, A., Michel, V., Thirion, B., Grisel, O., et al. (2011). Scikit-learn: Machine learning in Python. Journal of Machine Learning Research , 12 , 2825–2830.
Pennington, J., Socher, R., & Manning, C.D. (2014). Glove: Global vectors for word representation. In Empirical methods in natural language processing (EMNLP) (pp. 1532–1543). http://www.aclweb.org/anthology/D14-1162 .
Privitera, C., & Campbell, M. A. (2009). Cyberbullying: The new face of workplace bullying? CyberPsychology & Behavior , 12 (4), 395–400.
Řehůřek, R., & Sojka, P. (2010). Software framework for topic modelling with large corpora. In Proceedings of the LREC 2010 workshop on new challenges for NLP frameworks (pp. 45–50). ELRA, Valletta, Malta (2010). http://is.muni.cz/publication/884893/en .
Reynolds, K., Kontostathis, A., & Edwards, L. (2011). Using machine learning to detect cyberbullying. In 10th international conference on machine learning and applications and workshops (2011) (pp. 241–244).
Robers, S., Zhang, A., & Morgan, R.E. (2015). Indicators of school crime and safety: 2014. nces 2015-072/ncj 248036. National Center for Education Statistics.
Rosa, H., Carvalho, J.P., Calado, P., Martins, B., Ribeiro, R., & Coheur, L. (2018). Using fuzzy fingerprints for cyberbullying detection in social networks. In 2018 IEEE international conference on fuzzy systems (FUZZ-IEEE) (pp. 1–7). IEEE.
Rosa, H., Matos, D., Ribeiro, R., Coheur, L., & Carvalho, J.P. (2018). A “deeper” look at detecting cyberbullying in social networks. In 2018 international joint conference on neural networks (IJCNN) (pp. 1–8). IEEE.
Rosa, H., Pereira, N., Ribeiro, R., Ferreira, P. C., Carvalho, J. P., Oliveira, S., et al. (2019). Automatic cyberbullying detection: A systematic review. Computers in Human Behavior , 93 , 333–345.
Sanh, V., Debut, L., Chaumond, J., & Wolf, T. (2019). Distilbert, a distilled version of bert: smaller, faster, cheaper and lighter. In NeurIPS EMC 2 Workshop .
Schäfer, R., & Bildhauer, F. (2012). Building large corpora from the web using a new efficient tool chain. In LREC , (pp. 486–493).
Schnabel, T., & Schütze, H. (2014). Flors: Fast and simple domain adaptation for part-of-speech tagging. Transactions of the Association for Computational Linguistics , 2 , 15–26.
Schuster, M., & Paliwal, K. K. (1997). Bidirectional recurrent neural networks. IEEE Transactions on Signal Processing , 45 (11), 2673–2681.
Sharp, S., & Smith, P. K. (2002). School bullying: Insights and perspectives . : Routledge.
Smith, A., & Anderson, M. (2018). Social media use in 2018 . Washington, D.C.: Pew Research Center.
Squicciarini, A., Rajtmajer, S., Liu, Y., & Griffin, C. (2015). Identification and characterization of cyberbullying dynamics in an online social network. In Proceedings of the 2015 IEEE/ACM international conference on advances in social networks analysis and mining 2015 (pp. 280–285). ACM.
Steijn, W. M., & Schouten, A. P. (2013). Information sharing and relationships on social networking sites. Cyberpsychology, Behavior, and Social Networking , 16 (8), 582–587.
Sui, J. (2015). Understanding and fighting bullying with machine learning. Ph.D. thesis, The University of Wisconsin-Madison.
Sun, C., Qiu, X., Xu, Y., & Huang, X.(2019). How to fine-tune bert for text classification? In China national conference on Chinese computational linguistics (pp. 194–206). Springer.
Thain, N., Dixon, L., & Wulczyn, E. (2017). Wikipedia Talk Labels: Toxicity ,. https://doi.org/10.6084/m9.figshare.4563973.v2 . https://figshare.com/articles/Wikipedia_Talk_Labels_Toxicity/4563973 .
Tomkins, S., Getoor, L., Chen, Y., & Zhang, Y. (2018). A socio-linguistic model for cyberbullying detection. In 2018 IEEE/ACM international conference on advances in social networks analysis and mining (ASONAM) (pp. 53–60). IEEE.
Tulkens, S., Emmery, C., & Daelemans, W. (2016). Evaluating unsupervised dutch word embeddings as a linguistic resource. In: N.C.C. Chair), K. Choukri, T. Declerck, M. Grobelnik, B. Maegaard, J. Mariani, A. Moreno, J. Odijk, S. Piperidis (eds.) Proceedings of the Tenth International Conference on Language Resources and Evaluation (LREC 2016) . European Language Resources Association (ELRA), Paris, France.
Vaez, M., Ekberg, K., & LaFlamme, L. (2004). Abusive events at work among young working adults: Magnitude of the problem and its effect on self-rated health. Relations industrielles/industrial relations (pp. 569–584).
Valkenburg, P. M., & Peter, J. (2007). Preadolescents’ and adolescents’ online communication and their closeness to friends. Developmental Psychology , 43 (2), 267.
Van Hee, C., Jacobs, G., Emmery, C., Desmet, B., Lefever, E., Verhoeven, B., et al. (2018). Automatic detection of cyberbullying in social media text. PLoS ONE , 13 (10), 1–22. https://doi.org/10.1371/journal.pone.0203794 .
Van Hee, C., Verhoeven, B., Lefever, E., De Pauw, G., Daelemans, W., & Hoste, V. (2015). Guidelines for the fine-grained analysis of cyberbullying. Tech. rep., version 1.0. Technical Report LT3 15-01, LT3, Language and Translation Technology Team—Ghent University.
Vilain, M., Su, J., Lubar, S. (2007). Entity extraction is a boring solved problem—or is it? In Human language technologies 2007: The conference of the North American chapter of the Association for Computational Linguistics ; Companion Volume, Short Papers (pp. 181–184).
Wang, S., & Manning, C.D. (2012). Baselines and bigrams: Simple, good sentiment and topic classification. In Proceedings of the 50th annual meeting of the association for computational linguistics: Short papers-volume 2 (pp. 90–94). Association for Computational Linguistics.
Willard, N. E. (2007). Cyberbullying and cyberthreats: Responding to the challenge of online social aggression, threats, and distress . : Research Press.
Wulczyn, E., Thain, N., & Dixon, L. (2017). Ex machina: Personal attacks seen at scale. In Proceedings of the 26th international conference on World Wide Web (pp. 1391–1399).
Xu, J., Jun, K., Zhu, X., & Bellmore, A. (2012). Learning from Bullying Traces in Social Media. In Proceedings of the 2012 conference of the North American chapter of the Association for Computational Linguistics: Human Language Technologies (pp. 656–666). Association for Computational Linguistics.
Yin, D., Xue, Z., Hong, L., Davison, B.D., Kontostathis, A., & Edwards, L. (2009). Detection of Harassment on Web 2.0. In Proceedings of the content analysis in the WEB 2.0 (CAW2.0) Workshop at WWW2009 .
Zhang, X., Tong, J., Vishwamitra, N., Whittaker, E., Mazer, J.P., Kowalski, R., Hu, H., Luo, F., Macbeth, J., & Dillon, E. (2016). Cyberbullying detection with a pronunciation based convolutional neural network. In 2016 15th IEEE international conference on machine learning and applications (ICMLA) (pp. 740–745). IEEE.
Zhao, R., & Mao, K. (2016). Cyberbullying detection based on semantic-enhanced marginalized denoising auto-encoder. IEEE Transactions on Affective Computing , 8 (3), 328–339.
Zhao, R., Zhou, A., & Mao, K. (2016): Automatic detection of cyberbullying on social networks based on bullying features. In Proceedings of the 17th international conference on distributed computing and networking, ICDCN ’16 (pp. 43:1–43:6). ACM, New York, NY, USA. https://doi.org/10.1145/2833312.2849567 .
Zhou, C., Sun, C., Liu, Z., & Lau, F. (2015). A c-lstm neural network for text classification. arXiv preprint arXiv:1511.08630 .
Download references
Acknowledgements
We would like to thank Prodromos Ninas, Kostas Stoitsas, and Alejandra Hernández Rejón for carrying out a range of trial experiments on this task, Bram Willemsen for helpful remarks, and in particular Ákos Kádár for the many discussions throughout all stages of the current work. Finally, we want to express our gratitude to all authors providing cyberbullying data open-source. Most of all to Agrawal et al. for making their work reproducible, and thereby facilitating rigorous evaluations in this task.
Author information
Authors and affiliations.
CSAI, Tilburg University, Tilburg, The Netherlands
Chris Emmery
CLiPS, University of Antwerp, Antwerp, Belgium
Chris Emmery, Ben Verhoeven, Guy De Pauw & Walter Daelemans
LT3, Ghent University, Ghent, Belgium
Gilles Jacobs, Cynthia Van Hee, Els Lefever, Bart Desmet & Véronique Hoste
You can also search for this author in PubMed Google Scholar
Corresponding author
Correspondence to Chris Emmery .
Additional information
Publisher's note.
Springer Nature remains neutral with regard to jurisdictional claims in published maps and institutional affiliations.
The work presented in this article was carried out in the framework of the AMiCA (IWT SBO-project 120007) project, funded by the government agency for Innovation by Science and Technology (IWT).
Rights and permissions
Open Access This article is licensed under a Creative Commons Attribution 4.0 International License, which permits use, sharing, adaptation, distribution and reproduction in any medium or format, as long as you give appropriate credit to the original author(s) and the source, provide a link to the Creative Commons licence, and indicate if changes were made. The images or other third party material in this article are included in the article's Creative Commons licence, unless indicated otherwise in a credit line to the material. If material is not included in the article's Creative Commons licence and your intended use is not permitted by statutory regulation or exceeds the permitted use, you will need to obtain permission directly from the copyright holder. To view a copy of this licence, visit http://creativecommons.org/licenses/by/4.0/ .
Reprints and permissions
About this article
Emmery, C., Verhoeven, B., De Pauw, G. et al. Current limitations in cyberbullying detection: On evaluation criteria, reproducibility, and data scarcity. Lang Resources & Evaluation 55 , 597–633 (2021). https://doi.org/10.1007/s10579-020-09509-1
Download citation
Accepted : 24 September 2020
Published : 16 November 2020
Issue Date : September 2021
DOI : https://doi.org/10.1007/s10579-020-09509-1
Share this article
Anyone you share the following link with will be able to read this content:
Sorry, a shareable link is not currently available for this article.
Provided by the Springer Nature SharedIt content-sharing initiative
- Cyberbullying detection
- Cross-domain evaluation
- Reproducibility
- Crowdsourcing
- Data enrichment
- Find a journal
- Publish with us
- Track your research
- Search Menu
Sign in through your institution
- Browse content in Arts and Humanities
- Browse content in Architecture
- History of Architecture
- Browse content in Art
- History of Art
- Browse content in History
- Colonialism and Imperialism
- Historical Geography
- History by Period
- Industrial History
- Intellectual History
- Political History
- Regional and National History
- Social and Cultural History
- Urban History
- Language Teaching and Learning
- Browse content in Linguistics
- Cognitive Linguistics
- Grammar, Syntax and Morphology
- Historical and Diachronic Linguistics
- Language Variation
- Language Families
- Language Acquisition
- Language Evolution
- Lexicography
- Linguistic Theories
- Phonetics and Phonology
- Psycholinguistics
- Sociolinguistics
- Browse content in Literature
- Literary Studies (19th Century)
- Media Studies
- Browse content in Music
- Music Theory and Analysis
- Musicology and Music History
- Browse content in Philosophy
- Aesthetics and Philosophy of Art
- Epistemology
- History of Western Philosophy
- Metaphysics
- Moral Philosophy
- Philosophy of Science
- Philosophy of Language
- Philosophy of Mind
- Philosophy of Perception
- Philosophy of Mathematics and Logic
- Practical Ethics
- Social and Political Philosophy
- Browse content in Society and Culture
- Cultural Studies
- Technology and Society
- Visual Culture
- Browse content in Medicine and Health
- History of Medicine
- Browse content in Public Health and Epidemiology
- Public Health
- Browse content in Science and Mathematics
- Browse content in Biological Sciences
- Biochemistry
- Developmental Biology
- Ecology and Conservation
- Evolutionary Biology
- Genetics and Genomics
- Microbiology
- Molecular and Cell Biology
- Research Methods in Life Sciences
- Zoology and Animal Sciences
- Browse content in Computer Science
- Artificial Intelligence
- Programming Languages
- Browse content in Computing
- Computer Games
- Browse content in Earth Sciences and Geography
- Maps and Map-making
- Environmental Science
- History of Science and Technology
- Browse content in Mathematics
- Biomathematics and Statistics
- Probability and Statistics
- Browse content in Neuroscience
- Cognition and Behavioural Neuroscience
- Development of the Nervous System
- Disorders of the Nervous System
- History of Neuroscience
- Neuroscientific Techniques
- Sensory and Motor Systems
- Browse content in Physics
- History of Physics
- Browse content in Psychology
- Clinical Psychology
- Cognitive Neuroscience
- Cognitive Psychology
- Developmental Psychology
- Health Psychology
- Neuropsychology
- Social Psychology
- Browse content in Social Sciences
- Browse content in Business and Management
- Business History
- Business Strategy
- Business and Government
- Corporate Governance
- Industry Studies
- Information and Communication Technologies
- International Business
- Knowledge Management
- Organizational Theory and Behaviour
- Public and Nonprofit Management
- Browse content in Economics
- Agricultural, Environmental, and Natural Resource Economics
- Asian Economics
- Econometrics and Mathematical Economics
- Economic Systems
- Economic History
- Economic Development and Growth
- Financial Markets
- Financial Institutions and Services
- Health, Education, and Welfare
- History of Economic Thought
- International Economics
- Labour and Demographic Economics
- Macroeconomics and Monetary Economics
- Microeconomics
- Public Economics
- Welfare Economics
- Browse content in Education
- Educational Strategies and Policy
- Browse content in Environment
- Climate Change
- Social Impact of Environmental Issues (Social Science)
- Browse content in Interdisciplinary Studies
- Communication Studies
- Museums, Libraries, and Information Sciences
- Browse content in Politics
- Comparative Politics
- Conflict Politics
- Environmental Politics
- Human Rights and Politics
- International Relations
- Latin American Politics
- Political Theory
- Political Economy
- Political Institutions
- Public Policy
- Russian Politics
- Security Studies
- US Politics
- Browse content in Sociology
- Economic Sociology
- Health, Illness, and Medicine
- Social Movements and Social Change
- Sport and Leisure
- Reviews and Awards
- Journals on Oxford Academic
- Books on Oxford Academic
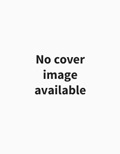
- < Previous chapter
- Next chapter >
8 Strengths and Limitations of Self-Regulation
- Published: February 2018
- Cite Icon Cite
- Permissions Icon Permissions
A more transparent self-regulatory framework is proposed here and it is argued in the chapter that operational policies need to be publicized and that mechanisms adopted by the companies need to be publicly available and regularly evaluated. Introducing regular independent evaluation of the mechanisms’ effectiveness is recommended as an essential component of effective self-regulation, and it is currently missing. Here, it is also explained why transparency is not the end-goal in and of itself. The aim behind ensuring transparency is to allow for an evaluation of the mechanisms’ effectiveness from youth perspective, which could open up space for changes in policies and mechanisms in a way that works for youth. This evaluation should nonetheless be independent –meaning that it is not executed by the companies themselves but rather by a third party whose impartial nature is established through regulatory monitoring.
Personal account
- Sign in with email/username & password
- Get email alerts
- Save searches
- Purchase content
- Activate your purchase/trial code
- Add your ORCID iD
Institutional access
Sign in with a library card.
- Sign in with username/password
- Recommend to your librarian
- Institutional account management
- Get help with access
Access to content on Oxford Academic is often provided through institutional subscriptions and purchases. If you are a member of an institution with an active account, you may be able to access content in one of the following ways:
IP based access
Typically, access is provided across an institutional network to a range of IP addresses. This authentication occurs automatically, and it is not possible to sign out of an IP authenticated account.
Choose this option to get remote access when outside your institution. Shibboleth/Open Athens technology is used to provide single sign-on between your institution’s website and Oxford Academic.
- Click Sign in through your institution.
- Select your institution from the list provided, which will take you to your institution's website to sign in.
- When on the institution site, please use the credentials provided by your institution. Do not use an Oxford Academic personal account.
- Following successful sign in, you will be returned to Oxford Academic.
If your institution is not listed or you cannot sign in to your institution’s website, please contact your librarian or administrator.
Enter your library card number to sign in. If you cannot sign in, please contact your librarian.
Society Members
Society member access to a journal is achieved in one of the following ways:
Sign in through society site
Many societies offer single sign-on between the society website and Oxford Academic. If you see ‘Sign in through society site’ in the sign in pane within a journal:
- Click Sign in through society site.
- When on the society site, please use the credentials provided by that society. Do not use an Oxford Academic personal account.
If you do not have a society account or have forgotten your username or password, please contact your society.
Sign in using a personal account
Some societies use Oxford Academic personal accounts to provide access to their members. See below.
A personal account can be used to get email alerts, save searches, purchase content, and activate subscriptions.
Some societies use Oxford Academic personal accounts to provide access to their members.
Viewing your signed in accounts
Click the account icon in the top right to:
- View your signed in personal account and access account management features.
- View the institutional accounts that are providing access.
Signed in but can't access content
Oxford Academic is home to a wide variety of products. The institutional subscription may not cover the content that you are trying to access. If you believe you should have access to that content, please contact your librarian.
For librarians and administrators, your personal account also provides access to institutional account management. Here you will find options to view and activate subscriptions, manage institutional settings and access options, access usage statistics, and more.
Our books are available by subscription or purchase to libraries and institutions.
Month: | Total Views: |
---|---|
April 2023 | 1 |
March 2024 | 1 |
- About Oxford Academic
- Publish journals with us
- University press partners
- What we publish
- New features
- Open access
- Rights and permissions
- Accessibility
- Advertising
- Media enquiries
- Oxford University Press
- Oxford Languages
- University of Oxford
Oxford University Press is a department of the University of Oxford. It furthers the University's objective of excellence in research, scholarship, and education by publishing worldwide
- Copyright © 2024 Oxford University Press
- Cookie settings
- Cookie policy
- Privacy policy
- Legal notice
This Feature Is Available To Subscribers Only
Sign In or Create an Account
This PDF is available to Subscribers Only
For full access to this pdf, sign in to an existing account, or purchase an annual subscription.

IMAGES
COMMENTS
Cyberbullying has built on a previous research tradition in school bullying, but with inputs from other disciplines. There has been a decade of a rapidly increasing number of research studies. This has been a global phenomenon, with an initial impetus from North...
Request PDF | Research on Cyberbullying: Strengths and Limitations | Cyberbullying has built on a previous research tradition in school bullying, but with inputs from other disciplines. There has ...
Research on Cyberbullying: Strengths and Limitations. Cyberbullying has built on a previous research tradition in school bullying, but with inputs from other disciplines. There has been a decade of a rapidly increasing number of research studies. This has been a global phenomenon, with an initial impetus from North America but by now an even ...
Despite a lack of scientific intervention research on cyberbullying, the review also identified several promising strategies for its prevention from the perspectives of youths, parents and schools. More research on cyberbullying is needed, especially on the issue of cross-national cyberbullying.
Cyberbullying is associated with considerable negative mental and psychosocial consequences in children and young people, making it a serious public health concern. To review the highest level of available evidence, a systematic mapping review was conducted ...
Highlights • Our study provides bibliometric overview of Cyberbullying research between 2010 and 2021 with analysis of 7045 publications. • Includes new analysis through the lens of sustainable development and the impact of COVID-19. • Science mapping of countries and journals, prolific authors, institutions, and gender-related contributions, are captured. • Top 3 SDGs are SDG16 peace ...
The articles included in this special issue of the International Journal of Bullying Prevention discuss different qualitative methods, reflect on strengths and limitations — possibilities and challenges, and suggest implications for future qualitative and mixed-methods research.
The present review will provide an up-to-date discussion of the latest in Cyberbullying (CBB) research. First, the conceptualization will be addressed…
Abstract and Figures Cyberbullying is associated with considerable negative mental and psychosocial consequences in children and young people, making it a serious public health concern. To review ...
As youth present to healthcare providers with symptoms related to cyberbullying, effective interventions are needed to guide evidence-based practice. This review supports educating the individual youth and parent on cyberbullying and teaching the youth skills in communication and social, empathy, coping with cyberbullying, and digital citizenship.
Highlights • Cyberbullying has been conceptualized as a form of traditional bullying which may be inadequate. • Theories that sufficiently explain cyberbullying perpetration are needed. • Emerging research identifies group differences in the risk for cybervictimization but current evidence is limited. • Progress is needed to address prevention and intervention strategies at the ...
2 How Has Research on Cyberbullying Developed? Research on cyberbullying has increased massively during this century. Figure 1 shows the number of articles on cyberbullying, up to 2017. It uses the Web of Sci-ence database with the following searches: cyber* and bully*; cyber* and victim*; electronic bullying; internet bullying; and online ...
As an extension of traditional bullying, cyberbullying emerges with the increasing popularity of the internet, and seriously affects the health of students. However, fewer studies have explored the potential influencing mechanisms of cyberbullying victimization from a positive psychology perspective. Therefore, based on the positive youth development theory, this study will explore the ...
Cyberbullying has become a major focus of not only youths, educators, and researchers, but also among the general population, due to high profile cases of cyberbullying victimization involving suicide and the increasing prevalence of these behaviors. The purpose of...
A comprehensive review of the literature on bullying and cyberbullying in education, covering the trends, topics, and methods of three decades of research.
Chapter. Research on Cyberbullying: Strengths and Limitations. Narratives in Research and Interventions on Cyberbullying among Young People, 2019
The objectives of this study were to systematically review, appraise, and synthesize the findings of qualitative studies of young people's conceptualizations relating to the nature of cyberbullying, with a view to informing conceptual and intervention development and the future conduct of cyberbullying research.
Abstract This study investigated the impact of cyberbullying victimization at work on well-being and strain in the workplace. This is the first study to use a longitudinal approach to research cyberbullying at work. A nationally representative sample of Finnish workers ( n = 768) took part in a five-wave survey study. Both within-person and between-person effects were analyzed using hybrid ...
Although attention to bullying has increased markedly among researchers, policy makers, and the media since the late 1990s, bullying and cyberbullying research is underdeveloped and uneven. Despite a growing literature on bullying in the United States, a reliable estimate for the number of children who are bullied in the United States today still eludes the field (Kowalski et al., 2012; Olweus ...
With this purpose, ten most cited articles on bullying and also on cyberbullying in each year published throughout the whole history of research in the field were analyzed.
The detection of online cyberbullying has seen an increase in societal importance, popularity in research, and available open data. Nevertheless, while computational power and affordability of resources continue to increase, the access restrictions on high-quality data limit the applicability of state-of-the-art techniques. Consequently, much of the recent research uses small, heterogeneous ...
Research on Cyberbullying: Strengths and Limitations. ... Narratives in Research and Interventions on Cyberbullying among Young People, 2019, p. 9-27. Publisher: Springer International Publishing ... A meta-analysis of sex differences in cyber-bullying behavior: The moderating role of age. Aggressive Behavior, 40, 474-488. ...
Cyberbullying policies of social media companies have not been a frequent object of academic study. My goal in researching and writing this book was to uncover the logic behind policy-making efforts. Now, I would like to explain what can be known about the strengths and limitations of the current self-regulatory environment as well as the self-organizational efforts of individual platforms. As ...