- Descriptive Research Designs: Types, Examples & Methods

One of the components of research is getting enough information about the research problem—the what, how, when and where answers, which is why descriptive research is an important type of research. It is very useful when conducting research whose aim is to identify characteristics, frequencies, trends, correlations, and categories.
This research method takes a problem with little to no relevant information and gives it a befitting description using qualitative and quantitative research method s. Descriptive research aims to accurately describe a research problem.
In the subsequent sections, we will be explaining what descriptive research means, its types, examples, and data collection methods.

What is Descriptive Research?
Descriptive research is a type of research that describes a population, situation, or phenomenon that is being studied. It focuses on answering the how, what, when, and where questions If a research problem, rather than the why.
This is mainly because it is important to have a proper understanding of what a research problem is about before investigating why it exists in the first place.
For example, an investor considering an investment in the ever-changing Amsterdam housing market needs to understand what the current state of the market is, how it changes (increasing or decreasing), and when it changes (time of the year) before asking for the why. This is where descriptive research comes in.
What Are The Types of Descriptive Research?
Descriptive research is classified into different types according to the kind of approach that is used in conducting descriptive research. The different types of descriptive research are highlighted below:
- Descriptive-survey
Descriptive survey research uses surveys to gather data about varying subjects. This data aims to know the extent to which different conditions can be obtained among these subjects.
For example, a researcher wants to determine the qualification of employed professionals in Maryland. He uses a survey as his research instrument , and each item on the survey related to qualifications is subjected to a Yes/No answer.
This way, the researcher can describe the qualifications possessed by the employed demographics of this community.
- Descriptive-normative survey
This is an extension of the descriptive survey, with the addition being the normative element. In the descriptive-normative survey, the results of the study should be compared with the norm.
For example, an organization that wishes to test the skills of its employees by a team may have them take a skills test. The skills tests are the evaluation tool in this case, and the result of this test is compared with the norm of each role.
If the score of the team is one standard deviation above the mean, it is very satisfactory, if within the mean, satisfactory, and one standard deviation below the mean is unsatisfactory.
- Descriptive-status
This is a quantitative description technique that seeks to answer questions about real-life situations. For example, a researcher researching the income of the employees in a company, and the relationship with their performance.
A survey will be carried out to gather enough data about the income of the employees, then their performance will be evaluated and compared to their income. This will help determine whether a higher income means better performance and low income means lower performance or vice versa.
- Descriptive-analysis
The descriptive-analysis method of research describes a subject by further analyzing it, which in this case involves dividing it into 2 parts. For example, the HR personnel of a company that wishes to analyze the job role of each employee of the company may divide the employees into the people that work at the Headquarters in the US and those that work from Oslo, Norway office.
A questionnaire is devised to analyze the job role of employees with similar salaries and who work in similar positions.
- Descriptive classification
This method is employed in biological sciences for the classification of plants and animals. A researcher who wishes to classify the sea animals into different species will collect samples from various search stations, then classify them accordingly.
- Descriptive-comparative
In descriptive-comparative research, the researcher considers 2 variables that are not manipulated, and establish a formal procedure to conclude that one is better than the other. For example, an examination body wants to determine the better method of conducting tests between paper-based and computer-based tests.
A random sample of potential participants of the test may be asked to use the 2 different methods, and factors like failure rates, time factors, and others will be evaluated to arrive at the best method.
- Correlative Survey
Correlative surveys are used to determine whether the relationship between 2 variables is positive, negative, or neutral. That is, if 2 variables say X and Y are directly proportional, inversely proportional or are not related to each other.
Examples of Descriptive Research
There are different examples of descriptive research, that may be highlighted from its types, uses, and applications. However, we will be restricting ourselves to only 3 distinct examples in this article.
- Comparing Student Performance:
An academic institution may wish 2 compare the performance of its junior high school students in English language and Mathematics. This may be used to classify students based on 2 major groups, with one group going ahead to study while courses, while the other study courses in the Arts & Humanities field.
Students who are more proficient in mathematics will be encouraged to go into STEM and vice versa. Institutions may also use this data to identify students’ weak points and work on ways to assist them.
- Scientific Classification
During the major scientific classification of plants, animals, and periodic table elements, the characteristics and components of each subject are evaluated and used to determine how they are classified.
For example, living things may be classified into kingdom Plantae or kingdom animal is depending on their nature. Further classification may group animals into mammals, pieces, vertebrae, invertebrae, etc.
All these classifications are made a result of descriptive research which describes what they are.
- Human Behavior
When studying human behaviour based on a factor or event, the researcher observes the characteristics, behaviour, and reaction, then use it to conclude. A company willing to sell to its target market needs to first study the behaviour of the market.
This may be done by observing how its target reacts to a competitor’s product, then use it to determine their behaviour.
What are the Characteristics of Descriptive Research?
The characteristics of descriptive research can be highlighted from its definition, applications, data collection methods, and examples. Some characteristics of descriptive research are:
- Quantitativeness
Descriptive research uses a quantitative research method by collecting quantifiable information to be used for statistical analysis of the population sample. This is very common when dealing with research in the physical sciences.
- Qualitativeness
It can also be carried out using the qualitative research method, to properly describe the research problem. This is because descriptive research is more explanatory than exploratory or experimental.
- Uncontrolled variables
In descriptive research, researchers cannot control the variables like they do in experimental research.
- The basis for further research
The results of descriptive research can be further analyzed and used in other research methods. It can also inform the next line of research, including the research method that should be used.
This is because it provides basic information about the research problem, which may give birth to other questions like why a particular thing is the way it is.
Why Use Descriptive Research Design?
Descriptive research can be used to investigate the background of a research problem and get the required information needed to carry out further research. It is used in multiple ways by different organizations, and especially when getting the required information about their target audience.
- Define subject characteristics :
It is used to determine the characteristics of the subjects, including their traits, behaviour, opinion, etc. This information may be gathered with the use of surveys, which are shared with the respondents who in this case, are the research subjects.
For example, a survey evaluating the number of hours millennials in a community spends on the internet weekly, will help a service provider make informed business decisions regarding the market potential of the community.
- Measure Data Trends
It helps to measure the changes in data over some time through statistical methods. Consider the case of individuals who want to invest in stock markets, so they evaluate the changes in prices of the available stocks to make a decision investment decision.
Brokerage companies are however the ones who carry out the descriptive research process, while individuals can view the data trends and make decisions.
Descriptive research is also used to compare how different demographics respond to certain variables. For example, an organization may study how people with different income levels react to the launch of a new Apple phone.
This kind of research may take a survey that will help determine which group of individuals are purchasing the new Apple phone. Do the low-income earners also purchase the phone, or only the high-income earners do?
Further research using another technique will explain why low-income earners are purchasing the phone even though they can barely afford it. This will help inform strategies that will lure other low-income earners and increase company sales.
- Validate existing conditions
When you are not sure about the validity of an existing condition, you can use descriptive research to ascertain the underlying patterns of the research object. This is because descriptive research methods make an in-depth analysis of each variable before making conclusions.
- Conducted Overtime
Descriptive research is conducted over some time to ascertain the changes observed at each point in time. The higher the number of times it is conducted, the more authentic the conclusion will be.
What are the Disadvantages of Descriptive Research?
- Response and Non-response Bias
Respondents may either decide not to respond to questions or give incorrect responses if they feel the questions are too confidential. When researchers use observational methods, respondents may also decide to behave in a particular manner because they feel they are being watched.
- The researcher may decide to influence the result of the research due to personal opinion or bias towards a particular subject. For example, a stockbroker who also has a business of his own may try to lure investors into investing in his own company by manipulating results.
- A case-study or sample taken from a large population is not representative of the whole population.
- Limited scope:The scope of descriptive research is limited to the what of research, with no information on why thereby limiting the scope of the research.
What are the Data Collection Methods in Descriptive Research?
There are 3 main data collection methods in descriptive research, namely; observational method, case study method, and survey research.
1. Observational Method
The observational method allows researchers to collect data based on their view of the behaviour and characteristics of the respondent, with the respondents themselves not directly having an input. It is often used in market research, psychology, and some other social science research to understand human behaviour.
It is also an important aspect of physical scientific research, with it being one of the most effective methods of conducting descriptive research . This process can be said to be either quantitative or qualitative.
Quantitative observation involved the objective collection of numerical data , whose results can be analyzed using numerical and statistical methods.
Qualitative observation, on the other hand, involves the monitoring of characteristics and not the measurement of numbers. The researcher makes his observation from a distance, records it, and is used to inform conclusions.
2. Case Study Method
A case study is a sample group (an individual, a group of people, organizations, events, etc.) whose characteristics are used to describe the characteristics of a larger group in which the case study is a subgroup. The information gathered from investigating a case study may be generalized to serve the larger group.
This generalization, may, however, be risky because case studies are not sufficient to make accurate predictions about larger groups. Case studies are a poor case of generalization.
3. Survey Research
This is a very popular data collection method in research designs. In survey research, researchers create a survey or questionnaire and distribute it to respondents who give answers.
Generally, it is used to obtain quick information directly from the primary source and also conducting rigorous quantitative and qualitative research. In some cases, survey research uses a blend of both qualitative and quantitative strategies.
Survey research can be carried out both online and offline using the following methods
- Online Surveys: This is a cheap method of carrying out surveys and getting enough responses. It can be carried out using Formplus, an online survey builder. Formplus has amazing tools and features that will help increase response rates.
- Offline Surveys: This includes paper forms, mobile offline forms , and SMS-based forms.
What Are The Differences Between Descriptive and Correlational Research?
Before going into the differences between descriptive and correlation research, we need to have a proper understanding of what correlation research is about. Therefore, we will be giving a summary of the correlation research below.
Correlational research is a type of descriptive research, which is used to measure the relationship between 2 variables, with the researcher having no control over them. It aims to find whether there is; positive correlation (both variables change in the same direction), negative correlation (the variables change in the opposite direction), or zero correlation (there is no relationship between the variables).
Correlational research may be used in 2 situations;
(i) when trying to find out if there is a relationship between two variables, and
(ii) when a causal relationship is suspected between two variables, but it is impractical or unethical to conduct experimental research that manipulates one of the variables.
Below are some of the differences between correlational and descriptive research:
- Definitions :
Descriptive research aims is a type of research that provides an in-depth understanding of the study population, while correlational research is the type of research that measures the relationship between 2 variables.
- Characteristics :
Descriptive research provides descriptive data explaining what the research subject is about, while correlation research explores the relationship between data and not their description.
- Predictions :
Predictions cannot be made in descriptive research while correlation research accommodates the possibility of making predictions.
Descriptive Research vs. Causal Research
Descriptive research and causal research are both research methodologies, however, one focuses on a subject’s behaviors while the latter focuses on a relationship’s cause-and-effect. To buttress the above point, descriptive research aims to describe and document the characteristics, behaviors, or phenomena of a particular or specific population or situation.
It focuses on providing an accurate and detailed account of an already existing state of affairs between variables. Descriptive research answers the questions of “what,” “where,” “when,” and “how” without attempting to establish any causal relationships or explain any underlying factors that might have caused the behavior.
Causal research, on the other hand, seeks to determine cause-and-effect relationships between variables. It aims to point out the factors that influence or cause a particular result or behavior. Causal research involves manipulating variables, controlling conditions or a subgroup, and observing the resulting effects. The primary objective of causal research is to establish a cause-effect relationship and provide insights into why certain phenomena happen the way they do.
Descriptive Research vs. Analytical Research
Descriptive research provides a detailed and comprehensive account of a specific situation or phenomenon. It focuses on describing and summarizing data without making inferences or attempting to explain underlying factors or the cause of the factor.
It is primarily concerned with providing an accurate and objective representation of the subject of research. While analytical research goes beyond the description of the phenomena and seeks to analyze and interpret data to discover if there are patterns, relationships, or any underlying factors.
It examines the data critically, applies statistical techniques or other analytical methods, and draws conclusions based on the discovery. Analytical research also aims to explore the relationships between variables and understand the underlying mechanisms or processes involved.
Descriptive Research vs. Exploratory Research
Descriptive research is a research method that focuses on providing a detailed and accurate account of a specific situation, group, or phenomenon. This type of research describes the characteristics, behaviors, or relationships within the given context without looking for an underlying cause.
Descriptive research typically involves collecting and analyzing quantitative or qualitative data to generate descriptive statistics or narratives. Exploratory research differs from descriptive research because it aims to explore and gain firsthand insights or knowledge into a relatively unexplored or poorly understood topic.
It focuses on generating ideas, hypotheses, or theories rather than providing definitive answers. Exploratory research is often conducted at the early stages of a research project to gather preliminary information and identify key variables or factors for further investigation. It involves open-ended interviews, observations, or small-scale surveys to gather qualitative data.
Read More – Exploratory Research: What are its Method & Examples?
Descriptive Research vs. Experimental Research
Descriptive research aims to describe and document the characteristics, behaviors, or phenomena of a particular population or situation. It focuses on providing an accurate and detailed account of the existing state of affairs.
Descriptive research typically involves collecting data through surveys, observations, or existing records and analyzing the data to generate descriptive statistics or narratives. It does not involve manipulating variables or establishing cause-and-effect relationships.
Experimental research, on the other hand, involves manipulating variables and controlling conditions to investigate cause-and-effect relationships. It aims to establish causal relationships by introducing an intervention or treatment and observing the resulting effects.
Experimental research typically involves randomly assigning participants to different groups, such as control and experimental groups, and measuring the outcomes. It allows researchers to control for confounding variables and draw causal conclusions.
Related – Experimental vs Non-Experimental Research: 15 Key Differences
Descriptive Research vs. Explanatory Research
Descriptive research focuses on providing a detailed and accurate account of a specific situation, group, or phenomenon. It aims to describe the characteristics, behaviors, or relationships within the given context.
Descriptive research is primarily concerned with providing an objective representation of the subject of study without explaining underlying causes or mechanisms. Explanatory research seeks to explain the relationships between variables and uncover the underlying causes or mechanisms.
It goes beyond description and aims to understand the reasons or factors that influence a particular outcome or behavior. Explanatory research involves analyzing data, conducting statistical analyses, and developing theories or models to explain the observed relationships.
Descriptive Research vs. Inferential Research
Descriptive research focuses on describing and summarizing data without making inferences or generalizations beyond the specific sample or population being studied. It aims to provide an accurate and objective representation of the subject of study.
Descriptive research typically involves analyzing data to generate descriptive statistics, such as means, frequencies, or percentages, to describe the characteristics or behaviors observed.
Inferential research, however, involves making inferences or generalizations about a larger population based on a smaller sample.
It aims to draw conclusions about the population characteristics or relationships by analyzing the sample data. Inferential research uses statistical techniques to estimate population parameters, test hypotheses, and determine the level of confidence or significance in the findings.
Related – Inferential Statistics: Definition, Types + Examples
Conclusion
The uniqueness of descriptive research partly lies in its ability to explore both quantitative and qualitative research methods. Therefore, when conducting descriptive research, researchers have the opportunity to use a wide variety of techniques that aids the research process.
Descriptive research explores research problems in-depth, beyond the surface level thereby giving a detailed description of the research subject. That way, it can aid further research in the field, including other research methods .
It is also very useful in solving real-life problems in various fields of social science, physical science, and education.
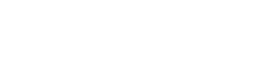
Connect to Formplus, Get Started Now - It's Free!
- descriptive research
- descriptive research method
- example of descriptive research
- types of descriptive research
- busayo.longe
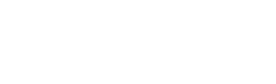
You may also like:
Extrapolation in Statistical Research: Definition, Examples, Types, Applications
In this article we’ll look at the different types and characteristics of extrapolation, plus how it contrasts to interpolation.

Type I vs Type II Errors: Causes, Examples & Prevention
This article will discuss the two different types of errors in hypothesis testing and how you can prevent them from occurring in your research
Acceptance Sampling: Meaning, Examples, When to Use
In this post, we will discuss extensively what acceptance sampling is and when it is applied.
Cross-Sectional Studies: Types, Pros, Cons & Uses
In this article, we’ll look at what cross-sectional studies are, how it applies to your research and how to use Formplus to collect...
Formplus - For Seamless Data Collection
Collect data the right way with a versatile data collection tool. try formplus and transform your work productivity today..
- Privacy Policy

Home » Descriptive Research Design – Types, Methods and Examples
Descriptive Research Design – Types, Methods and Examples
Table of Contents

Descriptive Research Design
Definition:
Descriptive research design is a type of research methodology that aims to describe or document the characteristics, behaviors, attitudes, opinions, or perceptions of a group or population being studied.
Descriptive research design does not attempt to establish cause-and-effect relationships between variables or make predictions about future outcomes. Instead, it focuses on providing a detailed and accurate representation of the data collected, which can be useful for generating hypotheses, exploring trends, and identifying patterns in the data.
Types of Descriptive Research Design
Types of Descriptive Research Design are as follows:
Cross-sectional Study
This involves collecting data at a single point in time from a sample or population to describe their characteristics or behaviors. For example, a researcher may conduct a cross-sectional study to investigate the prevalence of certain health conditions among a population, or to describe the attitudes and beliefs of a particular group.
Longitudinal Study
This involves collecting data over an extended period of time, often through repeated observations or surveys of the same group or population. Longitudinal studies can be used to track changes in attitudes, behaviors, or outcomes over time, or to investigate the effects of interventions or treatments.
This involves an in-depth examination of a single individual, group, or situation to gain a detailed understanding of its characteristics or dynamics. Case studies are often used in psychology, sociology, and business to explore complex phenomena or to generate hypotheses for further research.
Survey Research
This involves collecting data from a sample or population through standardized questionnaires or interviews. Surveys can be used to describe attitudes, opinions, behaviors, or demographic characteristics of a group, and can be conducted in person, by phone, or online.
Observational Research
This involves observing and documenting the behavior or interactions of individuals or groups in a natural or controlled setting. Observational studies can be used to describe social, cultural, or environmental phenomena, or to investigate the effects of interventions or treatments.
Correlational Research
This involves examining the relationships between two or more variables to describe their patterns or associations. Correlational studies can be used to identify potential causal relationships or to explore the strength and direction of relationships between variables.
Data Analysis Methods
Descriptive research design data analysis methods depend on the type of data collected and the research question being addressed. Here are some common methods of data analysis for descriptive research:
Descriptive Statistics
This method involves analyzing data to summarize and describe the key features of a sample or population. Descriptive statistics can include measures of central tendency (e.g., mean, median, mode) and measures of variability (e.g., range, standard deviation).
Cross-tabulation
This method involves analyzing data by creating a table that shows the frequency of two or more variables together. Cross-tabulation can help identify patterns or relationships between variables.
Content Analysis
This method involves analyzing qualitative data (e.g., text, images, audio) to identify themes, patterns, or trends. Content analysis can be used to describe the characteristics of a sample or population, or to identify factors that influence attitudes or behaviors.
Qualitative Coding
This method involves analyzing qualitative data by assigning codes to segments of data based on their meaning or content. Qualitative coding can be used to identify common themes, patterns, or categories within the data.
Visualization
This method involves creating graphs or charts to represent data visually. Visualization can help identify patterns or relationships between variables and make it easier to communicate findings to others.
Comparative Analysis
This method involves comparing data across different groups or time periods to identify similarities and differences. Comparative analysis can help describe changes in attitudes or behaviors over time or differences between subgroups within a population.
Applications of Descriptive Research Design
Descriptive research design has numerous applications in various fields. Some of the common applications of descriptive research design are:
- Market research: Descriptive research design is widely used in market research to understand consumer preferences, behavior, and attitudes. This helps companies to develop new products and services, improve marketing strategies, and increase customer satisfaction.
- Health research: Descriptive research design is used in health research to describe the prevalence and distribution of a disease or health condition in a population. This helps healthcare providers to develop prevention and treatment strategies.
- Educational research: Descriptive research design is used in educational research to describe the performance of students, schools, or educational programs. This helps educators to improve teaching methods and develop effective educational programs.
- Social science research: Descriptive research design is used in social science research to describe social phenomena such as cultural norms, values, and beliefs. This helps researchers to understand social behavior and develop effective policies.
- Public opinion research: Descriptive research design is used in public opinion research to understand the opinions and attitudes of the general public on various issues. This helps policymakers to develop effective policies that are aligned with public opinion.
- Environmental research: Descriptive research design is used in environmental research to describe the environmental conditions of a particular region or ecosystem. This helps policymakers and environmentalists to develop effective conservation and preservation strategies.
Descriptive Research Design Examples
Here are some real-time examples of descriptive research designs:
- A restaurant chain wants to understand the demographics and attitudes of its customers. They conduct a survey asking customers about their age, gender, income, frequency of visits, favorite menu items, and overall satisfaction. The survey data is analyzed using descriptive statistics and cross-tabulation to describe the characteristics of their customer base.
- A medical researcher wants to describe the prevalence and risk factors of a particular disease in a population. They conduct a cross-sectional study in which they collect data from a sample of individuals using a standardized questionnaire. The data is analyzed using descriptive statistics and cross-tabulation to identify patterns in the prevalence and risk factors of the disease.
- An education researcher wants to describe the learning outcomes of students in a particular school district. They collect test scores from a representative sample of students in the district and use descriptive statistics to calculate the mean, median, and standard deviation of the scores. They also create visualizations such as histograms and box plots to show the distribution of scores.
- A marketing team wants to understand the attitudes and behaviors of consumers towards a new product. They conduct a series of focus groups and use qualitative coding to identify common themes and patterns in the data. They also create visualizations such as word clouds to show the most frequently mentioned topics.
- An environmental scientist wants to describe the biodiversity of a particular ecosystem. They conduct an observational study in which they collect data on the species and abundance of plants and animals in the ecosystem. The data is analyzed using descriptive statistics to describe the diversity and richness of the ecosystem.
How to Conduct Descriptive Research Design
To conduct a descriptive research design, you can follow these general steps:
- Define your research question: Clearly define the research question or problem that you want to address. Your research question should be specific and focused to guide your data collection and analysis.
- Choose your research method: Select the most appropriate research method for your research question. As discussed earlier, common research methods for descriptive research include surveys, case studies, observational studies, cross-sectional studies, and longitudinal studies.
- Design your study: Plan the details of your study, including the sampling strategy, data collection methods, and data analysis plan. Determine the sample size and sampling method, decide on the data collection tools (such as questionnaires, interviews, or observations), and outline your data analysis plan.
- Collect data: Collect data from your sample or population using the data collection tools you have chosen. Ensure that you follow ethical guidelines for research and obtain informed consent from participants.
- Analyze data: Use appropriate statistical or qualitative analysis methods to analyze your data. As discussed earlier, common data analysis methods for descriptive research include descriptive statistics, cross-tabulation, content analysis, qualitative coding, visualization, and comparative analysis.
- I nterpret results: Interpret your findings in light of your research question and objectives. Identify patterns, trends, and relationships in the data, and describe the characteristics of your sample or population.
- Draw conclusions and report results: Draw conclusions based on your analysis and interpretation of the data. Report your results in a clear and concise manner, using appropriate tables, graphs, or figures to present your findings. Ensure that your report follows accepted research standards and guidelines.
When to Use Descriptive Research Design
Descriptive research design is used in situations where the researcher wants to describe a population or phenomenon in detail. It is used to gather information about the current status or condition of a group or phenomenon without making any causal inferences. Descriptive research design is useful in the following situations:
- Exploratory research: Descriptive research design is often used in exploratory research to gain an initial understanding of a phenomenon or population.
- Identifying trends: Descriptive research design can be used to identify trends or patterns in a population, such as changes in consumer behavior or attitudes over time.
- Market research: Descriptive research design is commonly used in market research to understand consumer preferences, behavior, and attitudes.
- Health research: Descriptive research design is useful in health research to describe the prevalence and distribution of a disease or health condition in a population.
- Social science research: Descriptive research design is used in social science research to describe social phenomena such as cultural norms, values, and beliefs.
- Educational research: Descriptive research design is used in educational research to describe the performance of students, schools, or educational programs.
Purpose of Descriptive Research Design
The main purpose of descriptive research design is to describe and measure the characteristics of a population or phenomenon in a systematic and objective manner. It involves collecting data that describe the current status or condition of the population or phenomenon of interest, without manipulating or altering any variables.
The purpose of descriptive research design can be summarized as follows:
- To provide an accurate description of a population or phenomenon: Descriptive research design aims to provide a comprehensive and accurate description of a population or phenomenon of interest. This can help researchers to develop a better understanding of the characteristics of the population or phenomenon.
- To identify trends and patterns: Descriptive research design can help researchers to identify trends and patterns in the data, such as changes in behavior or attitudes over time. This can be useful for making predictions and developing strategies.
- To generate hypotheses: Descriptive research design can be used to generate hypotheses or research questions that can be tested in future studies. For example, if a descriptive study finds a correlation between two variables, this could lead to the development of a hypothesis about the causal relationship between the variables.
- To establish a baseline: Descriptive research design can establish a baseline or starting point for future research. This can be useful for comparing data from different time periods or populations.
Characteristics of Descriptive Research Design
Descriptive research design has several key characteristics that distinguish it from other research designs. Some of the main characteristics of descriptive research design are:
- Objective : Descriptive research design is objective in nature, which means that it focuses on collecting factual and accurate data without any personal bias. The researcher aims to report the data objectively without any personal interpretation.
- Non-experimental: Descriptive research design is non-experimental, which means that the researcher does not manipulate any variables. The researcher simply observes and records the behavior or characteristics of the population or phenomenon of interest.
- Quantitative : Descriptive research design is quantitative in nature, which means that it involves collecting numerical data that can be analyzed using statistical techniques. This helps to provide a more precise and accurate description of the population or phenomenon.
- Cross-sectional: Descriptive research design is often cross-sectional, which means that the data is collected at a single point in time. This can be useful for understanding the current state of the population or phenomenon, but it may not provide information about changes over time.
- Large sample size: Descriptive research design typically involves a large sample size, which helps to ensure that the data is representative of the population of interest. A large sample size also helps to increase the reliability and validity of the data.
- Systematic and structured: Descriptive research design involves a systematic and structured approach to data collection, which helps to ensure that the data is accurate and reliable. This involves using standardized procedures for data collection, such as surveys, questionnaires, or observation checklists.
Advantages of Descriptive Research Design
Descriptive research design has several advantages that make it a popular choice for researchers. Some of the main advantages of descriptive research design are:
- Provides an accurate description: Descriptive research design is focused on accurately describing the characteristics of a population or phenomenon. This can help researchers to develop a better understanding of the subject of interest.
- Easy to conduct: Descriptive research design is relatively easy to conduct and requires minimal resources compared to other research designs. It can be conducted quickly and efficiently, and data can be collected through surveys, questionnaires, or observations.
- Useful for generating hypotheses: Descriptive research design can be used to generate hypotheses or research questions that can be tested in future studies. For example, if a descriptive study finds a correlation between two variables, this could lead to the development of a hypothesis about the causal relationship between the variables.
- Large sample size : Descriptive research design typically involves a large sample size, which helps to ensure that the data is representative of the population of interest. A large sample size also helps to increase the reliability and validity of the data.
- Can be used to monitor changes : Descriptive research design can be used to monitor changes over time in a population or phenomenon. This can be useful for identifying trends and patterns, and for making predictions about future behavior or attitudes.
- Can be used in a variety of fields : Descriptive research design can be used in a variety of fields, including social sciences, healthcare, business, and education.
Limitation of Descriptive Research Design
Descriptive research design also has some limitations that researchers should consider before using this design. Some of the main limitations of descriptive research design are:
- Cannot establish cause and effect: Descriptive research design cannot establish cause and effect relationships between variables. It only provides a description of the characteristics of the population or phenomenon of interest.
- Limited generalizability: The results of a descriptive study may not be generalizable to other populations or situations. This is because descriptive research design often involves a specific sample or situation, which may not be representative of the broader population.
- Potential for bias: Descriptive research design can be subject to bias, particularly if the researcher is not objective in their data collection or interpretation. This can lead to inaccurate or incomplete descriptions of the population or phenomenon of interest.
- Limited depth: Descriptive research design may provide a superficial description of the population or phenomenon of interest. It does not delve into the underlying causes or mechanisms behind the observed behavior or characteristics.
- Limited utility for theory development: Descriptive research design may not be useful for developing theories about the relationship between variables. It only provides a description of the variables themselves.
- Relies on self-report data: Descriptive research design often relies on self-report data, such as surveys or questionnaires. This type of data may be subject to biases, such as social desirability bias or recall bias.
About the author
Muhammad Hassan
Researcher, Academic Writer, Web developer
You may also like

Focus Groups – Steps, Examples and Guide

Qualitative Research Methods

Experimental Design – Types, Methods, Guide

Questionnaire – Definition, Types, and Examples

Explanatory Research – Types, Methods, Guide

Case Study – Methods, Examples and Guide
Have a language expert improve your writing
Run a free plagiarism check in 10 minutes, generate accurate citations for free.
- Knowledge Base
Methodology
- Descriptive Research | Definition, Types, Methods & Examples
Descriptive Research | Definition, Types, Methods & Examples
Published on May 15, 2019 by Shona McCombes . Revised on June 22, 2023.
Descriptive research aims to accurately and systematically describe a population, situation or phenomenon. It can answer what , where , when and how questions , but not why questions.
A descriptive research design can use a wide variety of research methods to investigate one or more variables . Unlike in experimental research , the researcher does not control or manipulate any of the variables, but only observes and measures them.
Table of contents
When to use a descriptive research design, descriptive research methods, other interesting articles.
Descriptive research is an appropriate choice when the research aim is to identify characteristics, frequencies, trends, and categories.
It is useful when not much is known yet about the topic or problem. Before you can research why something happens, you need to understand how, when and where it happens.
Descriptive research question examples
- How has the Amsterdam housing market changed over the past 20 years?
- Do customers of company X prefer product X or product Y?
- What are the main genetic, behavioural and morphological differences between European wildcats and domestic cats?
- What are the most popular online news sources among under-18s?
- How prevalent is disease A in population B?
Here's why students love Scribbr's proofreading services
Discover proofreading & editing
Descriptive research is usually defined as a type of quantitative research , though qualitative research can also be used for descriptive purposes. The research design should be carefully developed to ensure that the results are valid and reliable .
Survey research allows you to gather large volumes of data that can be analyzed for frequencies, averages and patterns. Common uses of surveys include:
- Describing the demographics of a country or region
- Gauging public opinion on political and social topics
- Evaluating satisfaction with a company’s products or an organization’s services
Observations
Observations allow you to gather data on behaviours and phenomena without having to rely on the honesty and accuracy of respondents. This method is often used by psychological, social and market researchers to understand how people act in real-life situations.
Observation of physical entities and phenomena is also an important part of research in the natural sciences. Before you can develop testable hypotheses , models or theories, it’s necessary to observe and systematically describe the subject under investigation.
Case studies
A case study can be used to describe the characteristics of a specific subject (such as a person, group, event or organization). Instead of gathering a large volume of data to identify patterns across time or location, case studies gather detailed data to identify the characteristics of a narrowly defined subject.
Rather than aiming to describe generalizable facts, case studies often focus on unusual or interesting cases that challenge assumptions, add complexity, or reveal something new about a research problem .
If you want to know more about statistics , methodology , or research bias , make sure to check out some of our other articles with explanations and examples.
- Normal distribution
- Degrees of freedom
- Null hypothesis
- Discourse analysis
- Control groups
- Mixed methods research
- Non-probability sampling
- Quantitative research
- Ecological validity
Research bias
- Rosenthal effect
- Implicit bias
- Cognitive bias
- Selection bias
- Negativity bias
- Status quo bias
Cite this Scribbr article
If you want to cite this source, you can copy and paste the citation or click the “Cite this Scribbr article” button to automatically add the citation to our free Citation Generator.
McCombes, S. (2023, June 22). Descriptive Research | Definition, Types, Methods & Examples. Scribbr. Retrieved July 2, 2024, from https://www.scribbr.com/methodology/descriptive-research/
Is this article helpful?
Shona McCombes
Other students also liked, what is quantitative research | definition, uses & methods, correlational research | when & how to use, descriptive statistics | definitions, types, examples, "i thought ai proofreading was useless but..".
I've been using Scribbr for years now and I know it's a service that won't disappoint. It does a good job spotting mistakes”
- What is descriptive research?
Last updated
5 February 2023
Reviewed by
Cathy Heath
Short on time? Get an AI generated summary of this article instead
Descriptive research is a common investigatory model used by researchers in various fields, including social sciences, linguistics, and academia.
Read on to understand the characteristics of descriptive research and explore its underlying techniques, processes, and procedures.
Analyze your descriptive research
Dovetail streamlines analysis to help you uncover and share actionable insights
Descriptive research is an exploratory research method. It enables researchers to precisely and methodically describe a population, circumstance, or phenomenon.
As the name suggests, descriptive research describes the characteristics of the group, situation, or phenomenon being studied without manipulating variables or testing hypotheses . This can be reported using surveys , observational studies, and case studies. You can use both quantitative and qualitative methods to compile the data.
Besides making observations and then comparing and analyzing them, descriptive studies often develop knowledge concepts and provide solutions to critical issues. It always aims to answer how the event occurred, when it occurred, where it occurred, and what the problem or phenomenon is.
- Characteristics of descriptive research
The following are some of the characteristics of descriptive research:
Quantitativeness
Descriptive research can be quantitative as it gathers quantifiable data to statistically analyze a population sample. These numbers can show patterns, connections, and trends over time and can be discovered using surveys, polls, and experiments.
Qualitativeness
Descriptive research can also be qualitative. It gives meaning and context to the numbers supplied by quantitative descriptive research .
Researchers can use tools like interviews, focus groups, and ethnographic studies to illustrate why things are what they are and help characterize the research problem. This is because it’s more explanatory than exploratory or experimental research.
Uncontrolled variables
Descriptive research differs from experimental research in that researchers cannot manipulate the variables. They are recognized, scrutinized, and quantified instead. This is one of its most prominent features.
Cross-sectional studies
Descriptive research is a cross-sectional study because it examines several areas of the same group. It involves obtaining data on multiple variables at the personal level during a certain period. It’s helpful when trying to understand a larger community’s habits or preferences.
Carried out in a natural environment
Descriptive studies are usually carried out in the participants’ everyday environment, which allows researchers to avoid influencing responders by collecting data in a natural setting. You can use online surveys or survey questions to collect data or observe.
Basis for further research
You can further dissect descriptive research’s outcomes and use them for different types of investigation. The outcomes also serve as a foundation for subsequent investigations and can guide future studies. For example, you can use the data obtained in descriptive research to help determine future research designs.
- Descriptive research methods
There are three basic approaches for gathering data in descriptive research: observational, case study, and survey.
You can use surveys to gather data in descriptive research. This involves gathering information from many people using a questionnaire and interview .
Surveys remain the dominant research tool for descriptive research design. Researchers can conduct various investigations and collect multiple types of data (quantitative and qualitative) using surveys with diverse designs.
You can conduct surveys over the phone, online, or in person. Your survey might be a brief interview or conversation with a set of prepared questions intended to obtain quick information from the primary source.
Observation
This descriptive research method involves observing and gathering data on a population or phenomena without manipulating variables. It is employed in psychology, market research , and other social science studies to track and understand human behavior.
Observation is an essential component of descriptive research. It entails gathering data and analyzing it to see whether there is a relationship between the two variables in the study. This strategy usually allows for both qualitative and quantitative data analysis.
Case studies
A case study can outline a specific topic’s traits. The topic might be a person, group, event, or organization.
It involves using a subset of a larger group as a sample to characterize the features of that larger group.
You can generalize knowledge gained from studying a case study to benefit a broader audience.
This approach entails carefully examining a particular group, person, or event over time. You can learn something new about the study topic by using a small group to better understand the dynamics of the entire group.
- Types of descriptive research
There are several types of descriptive study. The most well-known include cross-sectional studies, census surveys, sample surveys, case reports, and comparison studies.
Case reports and case series
In the healthcare and medical fields, a case report is used to explain a patient’s circumstances when suffering from an uncommon illness or displaying certain symptoms. Case reports and case series are both collections of related cases. They have aided the advancement of medical knowledge on countless occasions.
The normative component is an addition to the descriptive survey. In the descriptive–normative survey, you compare the study’s results to the norm.
Descriptive survey
This descriptive type of research employs surveys to collect information on various topics. This data aims to determine the degree to which certain conditions may be attained.
You can extrapolate or generalize the information you obtain from sample surveys to the larger group being researched.
Correlative survey
Correlative surveys help establish if there is a positive, negative, or neutral connection between two variables.
Performing census surveys involves gathering relevant data on several aspects of a given population. These units include individuals, families, organizations, objects, characteristics, and properties.
During descriptive research, you gather different degrees of interest over time from a specific population. Cross-sectional studies provide a glimpse of a phenomenon’s prevalence and features in a population. There are no ethical challenges with them and they are quite simple and inexpensive to carry out.
Comparative studies
These surveys compare the two subjects’ conditions or characteristics. The subjects may include research variables, organizations, plans, and people.
Comparison points, assumption of similarities, and criteria of comparison are three important variables that affect how well and accurately comparative studies are conducted.
For instance, descriptive research can help determine how many CEOs hold a bachelor’s degree and what proportion of low-income households receive government help.
- Pros and cons
The primary advantage of descriptive research designs is that researchers can create a reliable and beneficial database for additional study. To conduct any inquiry, you need access to reliable information sources that can give you a firm understanding of a situation.
Quantitative studies are time- and resource-intensive, so knowing the hypotheses viable for testing is crucial. The basic overview of descriptive research provides helpful hints as to which variables are worth quantitatively examining. This is why it’s employed as a precursor to quantitative research designs.
Some experts view this research as untrustworthy and unscientific. However, there is no way to assess the findings because you don’t manipulate any variables statistically.
Cause-and-effect correlations also can’t be established through descriptive investigations. Additionally, observational study findings cannot be replicated, which prevents a review of the findings and their replication.
The absence of statistical and in-depth analysis and the rather superficial character of the investigative procedure are drawbacks of this research approach.
- Descriptive research examples and applications
Several descriptive research examples are emphasized based on their types, purposes, and applications. Research questions often begin with “What is …” These studies help find solutions to practical issues in social science, physical science, and education.
Here are some examples and applications of descriptive research:
Determining consumer perception and behavior
Organizations use descriptive research designs to determine how various demographic groups react to a certain product or service.
For example, a business looking to sell to its target market should research the market’s behavior first. When researching human behavior in response to a cause or event, the researcher pays attention to the traits, actions, and responses before drawing a conclusion.
Scientific classification
Scientific descriptive research enables the classification of organisms and their traits and constituents.
Measuring data trends
A descriptive study design’s statistical capabilities allow researchers to track data trends over time. It’s frequently used to determine the study target’s current circumstances and underlying patterns.
Conduct comparison
Organizations can use a descriptive research approach to learn how various demographics react to a certain product or service. For example, you can study how the target market responds to a competitor’s product and use that information to infer their behavior.
- Bottom line
A descriptive research design is suitable for exploring certain topics and serving as a prelude to larger quantitative investigations. It provides a comprehensive understanding of the “what” of the group or thing you’re investigating.
This research type acts as the cornerstone of other research methodologies . It is distinctive because it can use quantitative and qualitative research approaches at the same time.
What is descriptive research design?
Descriptive research design aims to systematically obtain information to describe a phenomenon, situation, or population. More specifically, it helps answer the what, when, where, and how questions regarding the research problem rather than the why.
How does descriptive research compare to qualitative research?
Despite certain parallels, descriptive research concentrates on describing phenomena, while qualitative research aims to understand people better.
How do you analyze descriptive research data?
Data analysis involves using various methodologies, enabling the researcher to evaluate and provide results regarding validity and reliability.
Should you be using a customer insights hub?
Do you want to discover previous research faster?
Do you share your research findings with others?
Do you analyze research data?
Start for free today, add your research, and get to key insights faster
Editor’s picks
Last updated: 18 April 2023
Last updated: 27 February 2023
Last updated: 6 February 2023
Last updated: 6 October 2023
Last updated: 5 February 2023
Last updated: 16 April 2023
Last updated: 7 March 2023
Last updated: 9 March 2023
Last updated: 12 December 2023
Last updated: 11 March 2024
Last updated: 6 March 2024
Last updated: 5 March 2024
Last updated: 13 May 2024
Latest articles
Related topics, .css-je19u9{-webkit-align-items:flex-end;-webkit-box-align:flex-end;-ms-flex-align:flex-end;align-items:flex-end;display:-webkit-box;display:-webkit-flex;display:-ms-flexbox;display:flex;-webkit-flex-direction:row;-ms-flex-direction:row;flex-direction:row;-webkit-box-flex-wrap:wrap;-webkit-flex-wrap:wrap;-ms-flex-wrap:wrap;flex-wrap:wrap;-webkit-box-pack:center;-ms-flex-pack:center;-webkit-justify-content:center;justify-content:center;row-gap:0;text-align:center;max-width:671px;}@media (max-width: 1079px){.css-je19u9{max-width:400px;}.css-je19u9>span{white-space:pre;}}@media (max-width: 799px){.css-je19u9{max-width:400px;}.css-je19u9>span{white-space:pre;}} decide what to .css-1kiodld{max-height:56px;display:-webkit-box;display:-webkit-flex;display:-ms-flexbox;display:flex;-webkit-align-items:center;-webkit-box-align:center;-ms-flex-align:center;align-items:center;}@media (max-width: 1079px){.css-1kiodld{display:none;}} build next, decide what to build next.

Users report unexpectedly high data usage, especially during streaming sessions.

Users find it hard to navigate from the home page to relevant playlists in the app.

It would be great to have a sleep timer feature, especially for bedtime listening.

I need better filters to find the songs or artists I’m looking for.
Log in or sign up
Get started for free
Just one more step to your free trial.
.surveysparrow.com
Already using SurveySparrow? Login
By clicking on "Get Started", I agree to the Privacy Policy and Terms of Service .
This site is protected by reCAPTCHA and the Google Privacy Policy and Terms of Service apply.
Enterprise Survey Software
Enterprise Survey Software to thrive in your business ecosystem
NPS® Software
Turn customers into promoters
Offline Survey
Real-time data collection, on the move. Go internet-independent.
360 Assessment
Conduct omnidirectional employee assessments. Increase productivity, grow together.
Reputation Management
Turn your existing customers into raving promoters by monitoring online reviews.
Ticket Management
Build loyalty and advocacy by delivering personalized support experiences that matter.
Chatbot for Website
Collect feedback smartly from your website visitors with the engaging Chatbot for website.
Swift, easy, secure. Scalable for your organization.
Executive Dashboard
Customer journey map, craft beautiful surveys, share surveys, gain rich insights, recurring surveys, white label surveys, embedded surveys, conversational forms, mobile-first surveys, audience management, smart surveys, video surveys, secure surveys, api, webhooks, integrations, survey themes, accept payments, custom workflows, all features, customer experience, employee experience, product experience, marketing experience, sales experience, hospitality & travel, market research, saas startup programs, wall of love, success stories, sparrowcast, nps® benchmarks, learning centre, apps & integrations, testimonials.
Our surveys come with superpowers ⚡
Blog General
Descriptive Research 101: Definition, Methods and Examples
Parvathi vijayamohan.
Last Updated:
5 June 2024
Table Of Contents
- Descriptive Research 101: The Definitive Guide
What is Descriptive Research?
Key characteristics of descriptive research.
- Descriptive Research Methods: The 3 You Need to Know!
Observation
Case studies, 7 types of descriptive research, descriptive research: examples to build your next study, tips to excel at descriptive research.
Imagine you are a detective called to a crime scene. Your job is to study the scene and report whatever you find: whether that’s the half-smoked cigarette on the table or the large “RACHE” written in blood on the wall. That, in a nutshell, is descriptive research .
Researchers often need to do descriptive research on a problem before they attempt to solve it. So in this guide, we’ll take you through:
- What is descriptive research + characteristics
- Descriptive research methods
- Types of descriptive research
- Descriptive research examples
- Tips to excel at the descriptive method
Click to jump to the section that interests you.
Definition: As its name says, descriptive research describes the characteristics of the problem, phenomenon, situation, or group under study.
So the goal of all descriptive studies is to explore the background, details, and existing patterns in the problem to fully understand it. In other words, preliminary research.
However, descriptive research can be both preliminary and conclusive . You can use the data from a descriptive study to make reports and get insights for further planning.
What descriptive research isn’t: Descriptive research finds the what/when/where of a problem, not the why/how .
Because of this, we can’t use the descriptive method to explore cause-and-effect relationships where one variable (like a person’s job role) affects another variable (like their monthly income).
- Answers the “what,” “when,” and “where” of a research problem. For this reason, it is popularly used in market research , awareness surveys , and opinion polls .
- Sets the stage for a research problem. As an early part of the research process, descriptive studies help you dive deeper into the topic.
- Opens the door for further research. You can use descriptive data as the basis for more profound research, analysis and studies.
- Qualitative and quantitative . It is possible to get a balanced mix of numerical responses and open-ended answers from the descriptive method.
- No control or interference with the variables . The researcher simply observes and reports on them. However, specific research software has filters that allow her to zoom in on one variable.
- Done in natural settings . You can get the best results from descriptive research by talking to people, surveying them, or observing them in a suitable environment. For example, suppose you are a website beta testing an app feature. In that case, descriptive research invites users to try the feature, tracking their behavior and then asking their opinions .
- Can be applied to many research methods and areas. Examples include healthcare, SaaS, psychology, political studies, education, and pop culture.
Descriptive Research Methods: The Top Three You Need to Know!
In short, survey research is a brief interview or conversation with a set of prepared questions about a topic.
So you create a questionnaire, share it, and analyze the data you collect for further action. Learn about the differences between surveys and questionnaires here .
You can access free survey templates , over 20+ question types, and pass data to 1,500+ applications with survey software, like SurveySparrow . It enables you to create surveys, share them and capture data with very little effort.
Sign up today to launch stunning surveys for free.
Please enter a valid Email ID.
14-Day Free Trial • No Credit Card Required • No Strings Attached
- Surveys can be hyper-local, regional, or global, depending on your objectives.
- Share surveys in-person, offline, via SMS, email, or QR codes – so many options!
- Easy to automate if you want to conduct many surveys over a period.
The observational method is a type of descriptive research in which you, the researcher, observe ongoing behavior.
Now, there are several (non-creepy) ways you can observe someone. In fact, observational research has three main approaches:
- Covert observation: In true spy fashion, the researcher mixes in with the group undetected or observes from a distance.
- Overt observation : The researcher identifies himself as a researcher – “The name’s Bond. J. Bond.” – and explains the purpose of the study.
- Participatory observation : The researcher participates in what he is observing to understand his topic better.
- Observation is one of the most accurate ways to get data on a subject’s behavior in a natural setting.
- You don’t need to rely on people’s willingness to share information.
- Observation is a universal method that can be applied to any area of research.
In the case study method, you do a detailed study of a specific group, person, or event over a period.
This brings us to a frequently asked question: “What’s the difference between case studies and longitudinal studies?”
A case study will go very in-depth into the subject with one-on-one interviews, observations, and archival research. They are also qualitative, though sometimes they will use numbers and stats.
An example of longitudinal research would be a study of the health of night shift employees vs. general shift employees over a decade. An example of a case study would involve in-depth interviews with Casey, an assistant director of nursing who’s handled the night shift at the hospital for ten years now.
- Due to the focus on a few people, case studies can give you a tremendous amount of information.
- Because of the time and effort involved, a case study engages both researchers and participants.
- Case studies are helpful for ethically investigating unusual, complex, or challenging subjects. An example would be a study of the habits of long-term cocaine users.
Cross-sectional research | Studies a particular group of people or their sections at a given point in time. Example: current social attitudes of Gen Z in the US |
Longitudinal research | Studies a group of people over a long period of time. Example: tracking changes in social attitudes among Gen-Zers from 2022 – 2032. |
Normative research | Compares the results of a study against the existing norms. Example: comparing a verdict in a legal case against similar cases. |
Correlational/relational research | Investigates the type of relationship and patterns between 2 variables. Example: music genres and mental states. |
Comparative research | Compares 2 or more similar people, groups or conditions based on specific traits. Example: job roles of employees in similar positions from two different companies. |
Classification research | Arranges the data into classes according to certain criteria for better analysis. Example: the classification of newly discovered insects into species. |
Archival research | Searching for and extracting information from past records. Example: Tracking US Census data over the decades. |
1. Case Study: Airbnb’s Growth Strategy
In an excellent case study, Tam Al Saad, Principal Consultant, Strategy + Growth at Webprofits, deep dives into how Airbnb attracted and retained 150 million users .
“What Airbnb offers isn’t a cheap place to sleep when you’re on holiday; it’s the opportunity to experience your destination as a local would. It’s the chance to meet the locals, experience the markets, and find non-touristy places.
Sure, you can visit the Louvre, see Buckingham Palace, and climb the Empire State Building, but you can do it as if it were your hometown while staying in a place that has character and feels like a home.” – Tam al Saad, Principal Consultant, Strategy + Growth at Webprofits
2. Observation – Better Tech Experiences for the Elderly
We often think that our elders are so hopeless with technology. But we’re not getting any younger either, and tech is changing at a hair trigger! This article by Annemieke Hendricks shares a wonderful example where researchers compare the levels of technological familiarity between age groups and how that influences usage.
“It is generally assumed that older adults have difficulty using modern electronic devices, such as mobile telephones or computers. Because this age group is growing in most countries, changing products and processes to adapt to their needs is increasingly more important. “ – Annemieke Hendricks, Marketing Communication Specialist, Noldus
3. Surveys – Decoding Sleep with SurveySparrow
SRI International (formerly Stanford Research Institute) – an independent, non-profit research center – wanted to investigate the impact of stress on an adolescent’s sleep. To get those insights, two actions were essential: tracking sleep patterns through wearable devices and sending surveys at a pre-set time – the pre-sleep period.
“With SurveySparrow’s recurring surveys feature, SRI was able to share engaging surveys with their participants exactly at the time they wanted and at the frequency they preferred.”
Read more about this project : How SRI International decoded sleep patterns with SurveySparrow
1: Answer the six Ws –
- Who should we consider?
- What information do we need?
- When should we collect the information?
- Where should we collect the information?
- Why are we obtaining the information?
- Way to collect the information
#2: Introduce and explain your methodological approach
#3: Describe your methods of data collection and/or selection.
#4: Describe your methods of analysis.
#5: Explain the reasoning behind your choices.
#6: Collect data.
#7: Analyze the data. Use software to speed up the process and reduce overthinking and human error.
#8: Report your conclusions and how you drew the results.
Growth Marketer at SurveySparrow
Fledgling growth marketer. Cloud watcher. Aunty to a naughty beagle.
You Might Also Like
How to create a survey on facebook | a complete guide, an in-depth guide to build a better work order process, top 10 fillout forms alternatives (low code/ no-code), see it to believe it..
14-Day Free Trial • Cancel Anytime • No Credit Card Required • Need a Demo?
Start your free trial today
No Credit Card Required. 14-Day Free Trial
Request a Demo
Want to learn more about SurveySparrow? We'll be in touch soon!
Scale up your descriptive research with the best survey software
Build surveys that actually work. give surveysparrow a free try today.
14-Day Free Trial • No Credit card required • 40% more completion rate
Hi there, we use cookies to offer you a better browsing experience and to analyze site traffic. By continuing to use our website, you consent to the use of these cookies. Learn More
Have a language expert improve your writing
Run a free plagiarism check in 10 minutes, automatically generate references for free.
- Knowledge Base
- Methodology
- Descriptive Research Design | Definition, Methods & Examples
Descriptive Research Design | Definition, Methods & Examples
Published on 5 May 2022 by Shona McCombes . Revised on 10 October 2022.
Descriptive research aims to accurately and systematically describe a population, situation or phenomenon. It can answer what , where , when , and how questions , but not why questions.
A descriptive research design can use a wide variety of research methods to investigate one or more variables . Unlike in experimental research , the researcher does not control or manipulate any of the variables, but only observes and measures them.
Table of contents
When to use a descriptive research design, descriptive research methods.
Descriptive research is an appropriate choice when the research aim is to identify characteristics, frequencies, trends, and categories.
It is useful when not much is known yet about the topic or problem. Before you can research why something happens, you need to understand how, when, and where it happens.
- How has the London housing market changed over the past 20 years?
- Do customers of company X prefer product Y or product Z?
- What are the main genetic, behavioural, and morphological differences between European wildcats and domestic cats?
- What are the most popular online news sources among under-18s?
- How prevalent is disease A in population B?
Prevent plagiarism, run a free check.
Descriptive research is usually defined as a type of quantitative research , though qualitative research can also be used for descriptive purposes. The research design should be carefully developed to ensure that the results are valid and reliable .
Survey research allows you to gather large volumes of data that can be analysed for frequencies, averages, and patterns. Common uses of surveys include:
- Describing the demographics of a country or region
- Gauging public opinion on political and social topics
- Evaluating satisfaction with a company’s products or an organisation’s services
Observations
Observations allow you to gather data on behaviours and phenomena without having to rely on the honesty and accuracy of respondents. This method is often used by psychological, social, and market researchers to understand how people act in real-life situations.
Observation of physical entities and phenomena is also an important part of research in the natural sciences. Before you can develop testable hypotheses , models, or theories, it’s necessary to observe and systematically describe the subject under investigation.
Case studies
A case study can be used to describe the characteristics of a specific subject (such as a person, group, event, or organisation). Instead of gathering a large volume of data to identify patterns across time or location, case studies gather detailed data to identify the characteristics of a narrowly defined subject.
Rather than aiming to describe generalisable facts, case studies often focus on unusual or interesting cases that challenge assumptions, add complexity, or reveal something new about a research problem .
Cite this Scribbr article
If you want to cite this source, you can copy and paste the citation or click the ‘Cite this Scribbr article’ button to automatically add the citation to our free Reference Generator.
McCombes, S. (2022, October 10). Descriptive Research Design | Definition, Methods & Examples. Scribbr. Retrieved 2 July 2024, from https://www.scribbr.co.uk/research-methods/descriptive-research-design/
Is this article helpful?
Shona McCombes
Other students also liked, a quick guide to experimental design | 5 steps & examples, correlational research | guide, design & examples, qualitative vs quantitative research | examples & methods.
Last updated 27/06/24: Online ordering is currently unavailable due to technical issues. We apologise for any delays responding to customers while we resolve this. For further updates please visit our website: https://www.cambridge.org/news-and-insights/technical-incident
We use cookies to distinguish you from other users and to provide you with a better experience on our websites. Close this message to accept cookies or find out how to manage your cookie settings .
Login Alert

- > The Research Imagination
- > COMPARATIVE RESEARCH METHODS
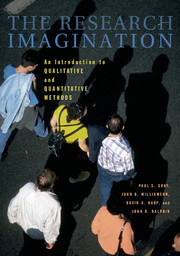
Book contents
- Frontmatter
- 1 RESEARCH PROCESS
- 2 THEORY AND METHOD
- 3 RESEARCH DESIGN
- 4 MEASUREMENT
- 5 ETHICAL AND POLITICAL ISSUES
- 7 SURVEY RESEARCH
- 8 INTENSIVE INTERVIEWING
- 9 OBSERVATIONAL FIELD RESEARCH
- 10 FEMINIST METHODS
- 11 HISTORICAL ANALYSIS
- 12 EXPERIMENTAL RESEARCH
- 13 CONTENT ANALYSIS
- 14 AGGREGATE DATA ANALYSIS
- 15 COMPARATIVE RESEARCH METHODS
- 16 EVALUATION RESEARCH
- 17 INDEXES AND SCALES
- 18 BASIC STATISTICAL ANALYSIS
- 19 MULTIVARIATE ANALYSIS AND STATISTICAL SIGNIFICANCE
- EPILOGUE: THE VALUE AND LIMITS OF SOCIAL SCIENCE KNOWLEDGE
- Appendix A A Precoded Questionnaire
- Appendix B Excerpt from a Codebook
- Author Index
- Subject Index
15 - COMPARATIVE RESEARCH METHODS
Published online by Cambridge University Press: 05 June 2012
INTRODUCTION
In contrast to the chapters on survey research, experimentation, or content analysis that described a distinct set of skills, in this chapter, a variety of comparative research techniques are discussed. What makes a study comparative is not the particular techniques employed but the theoretical orientation and the sources of data. All the tools of the social scientist, including historical analysis, fieldwork, surveys, and aggregate data analysis, can be used to achieve the goals of comparative research. So, there is plenty of room for the research imagination in the choice of data collection strategies. There is a wide divide between quantitative and qualitative approaches in comparative work. Most studies are either exclusively qualitative (e.g., individual case studies of a small number of countries) or exclusively quantitative, most often using many cases and a cross-national focus (Ragin, 1991:7). Ideally, increasing numbers of studies in the future will use both traditions, as the skills, tools, and quality of data in comparative research continue to improve.
In almost all social research, we look at how social processes vary and are experienced in different settings to develop our knowledge of the causes and effects of human behavior. This holds true if we are trying to explain the behavior of nations or individuals. So, it may then seem redundant to include a chapter in this book specifically dedicated to comparative research methods when all the other methods discussed are ultimately comparative.
Access options
Save book to kindle.
To save this book to your Kindle, first ensure [email protected] is added to your Approved Personal Document E-mail List under your Personal Document Settings on the Manage Your Content and Devices page of your Amazon account. Then enter the ‘name’ part of your Kindle email address below. Find out more about saving to your Kindle .
Note you can select to save to either the @free.kindle.com or @kindle.com variations. ‘@free.kindle.com’ emails are free but can only be saved to your device when it is connected to wi-fi. ‘@kindle.com’ emails can be delivered even when you are not connected to wi-fi, but note that service fees apply.
Find out more about the Kindle Personal Document Service .
- COMPARATIVE RESEARCH METHODS
- Paul S. Gray , Boston College, Massachusetts , John B. Williamson , Boston College, Massachusetts , David A. Karp , Boston College, Massachusetts , John R. Dalphin
- Book: The Research Imagination
- Online publication: 05 June 2012
- Chapter DOI: https://doi.org/10.1017/CBO9780511819391.016
Save book to Dropbox
To save content items to your account, please confirm that you agree to abide by our usage policies. If this is the first time you use this feature, you will be asked to authorise Cambridge Core to connect with your account. Find out more about saving content to Dropbox .
Save book to Google Drive
To save content items to your account, please confirm that you agree to abide by our usage policies. If this is the first time you use this feature, you will be asked to authorise Cambridge Core to connect with your account. Find out more about saving content to Google Drive .

18 Descriptive Research Examples
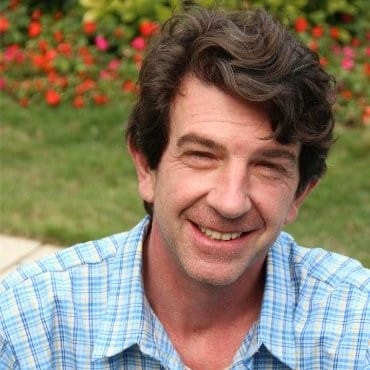
Dave Cornell (PhD)
Dr. Cornell has worked in education for more than 20 years. His work has involved designing teacher certification for Trinity College in London and in-service training for state governments in the United States. He has trained kindergarten teachers in 8 countries and helped businessmen and women open baby centers and kindergartens in 3 countries.
Learn about our Editorial Process
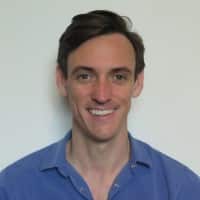
Chris Drew (PhD)
This article was peer-reviewed and edited by Chris Drew (PhD). The review process on Helpful Professor involves having a PhD level expert fact check, edit, and contribute to articles. Reviewers ensure all content reflects expert academic consensus and is backed up with reference to academic studies. Dr. Drew has published over 20 academic articles in scholarly journals. He is the former editor of the Journal of Learning Development in Higher Education and holds a PhD in Education from ACU.

Descriptive research involves gathering data to provide a detailed account or depiction of a phenomenon without manipulating variables or conducting experiments.
A scholarly definition is:
“Descriptive research is defined as a research approach that describes the characteristics of the population, sample or phenomenon studied. This method focuses more on the “what” rather than the “why” of the research subject.” (Matanda, 2022, p. 63)
The key feature of descriptive research is that it merely describes phenomena and does not attempt to manipulate variables nor determine cause and effect .
To determine cause and effect , a researcher would need to use an alternate methodology, such as experimental research design .
Common approaches to descriptive research include:
- Cross-sectional research : A cross-sectional study gathers data on a population at a specific time to get descriptive data that could include categories (e.g. age or income brackets) to get a better understanding of the makeup of a population.
- Longitudinal research : Longitudinal studies return to a population to collect data at several different points in time, allowing for description of changes in categories over time. However, as it’s descriptive, it cannot infer cause and effect (Erickson, 2017).
Methods that could be used include:
- Surveys: For example, sending out a census survey to be completed at the exact same date and time by everyone in a population.
- Case Study : For example, an in-depth description of a specific person or group of people to gain in-depth qualitative information that can describe a phenomenon but cannot be generalized to other cases.
- Observational Method : For example, a researcher taking field notes in an ethnographic study. (Siedlecki, 2020)

Descriptive Research Examples
1. Understanding Autism Spectrum Disorder (Psychology): Researchers analyze various behavior patterns, cognitive skills, and social interaction abilities specific to children with Autism Spectrum Disorder to comprehensively describe the disorder’s symptom spectrum. This detailed description classifies it as descriptive research, rather than analytical or experimental, as it merely records what is observed without altering any variables or trying to establish causality.
2. Consumer Purchase Decision Process in E-commerce Marketplaces (Marketing): By documenting and describing all the factors that influence consumer decisions on online marketplaces, researchers don’t attempt to predict future behavior or establish causes—just describe observed behavior—making it descriptive research.
3. Impacts of Climate Change on Agricultural Practices (Environmental Studies): Descriptive research is seen as scientists outline how climate changes influence various agricultural practices by observing and then meticulously categorizing the impacts on crop variability, farming seasons, and pest infestations without manipulating any variables in real-time.
4. Work Environment and Employee Performance (Human Resources Management): A study of this nature, describing the correlation between various workplace elements and employee performance, falls under descriptive research as it merely narrates the observed patterns without altering any conditions or testing hypotheses.
5. Factors Influencing Student Performance (Education): Researchers describe various factors affecting students’ academic performance, such as studying techniques, parental involvement, and peer influence. The study is categorized as descriptive research because its principal aim is to depict facts as they stand without trying to infer causal relationships.
6. Technological Advances in Healthcare (Healthcare): This research describes and categorizes different technological advances (such as telemedicine, AI-enabled tools, digital collaboration) in healthcare without testing or modifying any parameters, making it an example of descriptive research.
7. Urbanization and Biodiversity Loss (Ecology): By describing the impact of rapid urban expansion on biodiversity loss, this study serves as a descriptive research example. It observes the ongoing situation without manipulating it, offering a comprehensive depiction of the existing scenario rather than investigating the cause-effect relationship.
8. Architectural Styles across Centuries (Art History): A study documenting and describing various architectural styles throughout centuries essentially represents descriptive research. It aims to narrate and categorize facts without exploring the underlying reasons or predicting future trends.
9. Media Usage Patterns among Teenagers (Sociology): When researchers document and describe the media consumption habits among teenagers, they are performing a descriptive research study. Their main intention is to observe and report the prevailing trends rather than establish causes or predict future behaviors.
10. Dietary Habits and Lifestyle Diseases (Nutrition Science): By describing the dietary patterns of different population groups and correlating them with the prevalence of lifestyle diseases, researchers perform descriptive research. They merely describe observed connections without altering any diet plans or lifestyles.
11. Shifts in Global Energy Consumption (Environmental Economics): When researchers describe the global patterns of energy consumption and how they’ve shifted over the years, they conduct descriptive research. The focus is on recording and portraying the current state without attempting to infer causes or predict the future.
12. Literacy and Employment Rates in Rural Areas (Sociology): A study aims at describing the literacy rates in rural areas and correlating it with employment levels. It falls under descriptive research because it maps the scenario without manipulating parameters or proving a hypothesis.
13. Women Representation in Tech Industry (Gender Studies): A detailed description of the presence and roles of women across various sectors of the tech industry is a typical case of descriptive research. It merely observes and records the status quo without establishing causality or making predictions.
14. Impact of Urban Green Spaces on Mental Health (Environmental Psychology): When researchers document and describe the influence of green urban spaces on residents’ mental health, they are undertaking descriptive research. They seek purely to understand the current state rather than exploring cause-effect relationships.
15. Trends in Smartphone usage among Elderly (Gerontology): Research describing how the elderly population utilizes smartphones, including popular features and challenges encountered, serves as descriptive research. Researcher’s aim is merely to capture what is happening without manipulating variables or posing predictions.
16. Shifts in Voter Preferences (Political Science): A study describing the shift in voter preferences during a particular electoral cycle is descriptive research. It simply records the preferences revealed without drawing causal inferences or suggesting future voting patterns.
17. Understanding Trust in Autonomous Vehicles (Transportation Psychology): This comprises research describing public attitudes and trust levels when it comes to autonomous vehicles. By merely depicting observed sentiments, without engineering any situations or offering predictions, it’s considered descriptive research.
18. The Impact of Social Media on Body Image (Psychology): Descriptive research to outline the experiences and perceptions of individuals relating to body image in the era of social media. Observing these elements without altering any variables qualifies it as descriptive research.
Descriptive vs Experimental Research
Descriptive research merely observes, records, and presents the actual state of affairs without manipulating any variables, while experimental research involves deliberately changing one or more variables to determine their effect on a particular outcome.
De Vaus (2001) succinctly explains that descriptive studies find out what is going on , but experimental research finds out why it’s going on /
Simple definitions are below:
- Descriptive research is primarily about describing the characteristics or behaviors in a population, often through surveys or observational methods. It provides rich detail about a specific phenomenon but does not allow for conclusive causal statements; however, it can offer essential leads or ideas for further experimental research (Ivey, 2016).
- Experimental research , often conducted in controlled environments, aims to establish causal relationships by manipulating one or more independent variables and observing the effects on dependent variables (Devi, 2017; Mukherjee, 2019).
Experimental designs often involve a control group and random assignment . While it can provide compelling evidence for cause and effect, its artificial setting might not perfectly mirror real-worldly conditions, potentially affecting the generalizability of its findings.
These two types of research are complementary, with descriptive studies often leading to hypotheses that are then tested experimentally (Devi, 2017; Zhao et al., 2021).
Parameter | Descriptive Research | Experimental Research |
---|---|---|
To describe and explore phenomena without influencing variables (Monsen & Van Horn, 2007). | To investigate cause-and-effect relationships by manipulating variables. | |
Observational and non-intrusive. | Manipulative and controlled. | |
Typically not aimed at testing a hypothesis. | Generally tests a hypothesis (Mukherjee, 2019). | |
No variables are manipulated (Erickson, 2017). | Involves manipulation of one or more variables (independent variables). | |
No control over variables and environment. | Strict control over variables and environment. | |
Does not establish causal relationships. | Aims to establish causal relationships. | |
Not focused on predicting outcomes. | Often seeks to predict outcomes based on variable manipulation (Zhao et al., 2021). | |
Uses surveys, observations, and case studies (Ivey, 2016). | Employs controlled experiments often with experimental and control groups. | |
Typically fewer ethical concerns due to non-interference. | Potential ethical considerations due to manipulation and intervention (Devi, 2017). |
Benefits and Limitations of Descriptive Research
Descriptive research offers several benefits: it allows researchers to gather a vast amount of data and present a complete picture of the situation or phenomenon under study, even within large groups or over long time periods.
It’s also flexible in terms of the variety of methods used, such as surveys, observations, and case studies, and it can be instrumental in identifying patterns or trends and generating hypotheses (Erickson, 2017).
However, it also has its limitations.
The primary drawback is that it can’t establish cause-effect relationships, as no variables are manipulated. This lack of control over variables also opens up possibilities for bias, as researchers might inadvertently influence responses during data collection (De Vaus, 2001).
Additionally, the findings of descriptive research are often not generalizable since they are heavily reliant on the chosen sample’s characteristics.
Provides a comprehensive and detailed profile of the subject or issue through rich data, offering a thorough understanding (Gresham, 2016). | Cannot or external factors, potentially influencing the accuracy and reliability of the data. |
Helps to identify patterns, trends, and variables for subsequent experimental or correlational research – Krishnaswamy et al. (2009) call it “fact finding” research, setting the groundwork for future experimental studies. | Cannot establish causal relationships due to its observational nature, limiting the explanatory power. |
See More Types of Research Design Here
De Vaus, D. A. (2001). Research Design in Social Research . SAGE Publications.
Devi, P. S. (2017). Research Methodology: A Handbook for Beginners . Notion Press.
Erickson, G. S. (2017). Descriptive research design. In New Methods of Market Research and Analysis (pp. 51-77). Edward Elgar Publishing.
Gresham, B. B. (2016). Concepts of Evidence-based Practice for the Physical Therapist Assistant . F.A. Davis Company.
Ivey, J. (2016). Is descriptive research worth doing?. Pediatric nursing , 42 (4), 189. ( Source )
Krishnaswamy, K. N., Sivakumar, A. I., & Mathirajan, M. (2009). Management Research Methodology: Integration of Principles, Methods and Techniques . Pearson Education.
Matanda, E. (2022). Research Methods and Statistics for Cross-Cutting Research: Handbook for Multidisciplinary Research . Langaa RPCIG.
Monsen, E. R., & Van Horn, L. (2007). Research: Successful Approaches . American Dietetic Association.
Mukherjee, S. P. (2019). A Guide to Research Methodology: An Overview of Research Problems, Tasks and Methods . CRC Press.
Siedlecki, S. L. (2020). Understanding descriptive research designs and methods. Clinical Nurse Specialist , 34 (1), 8-12. ( Source )
Zhao, P., Ross, K., Li, P., & Dennis, B. (2021). Making Sense of Social Research Methodology: A Student and Practitioner Centered Approach . SAGE Publications.
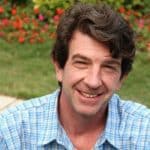
- Dave Cornell (PhD) https://helpfulprofessor.com/author/dave-cornell-phd/ 23 Achieved Status Examples
- Dave Cornell (PhD) https://helpfulprofessor.com/author/dave-cornell-phd/ 25 Defense Mechanisms Examples
- Dave Cornell (PhD) https://helpfulprofessor.com/author/dave-cornell-phd/ 15 Theory of Planned Behavior Examples
- Dave Cornell (PhD) https://helpfulprofessor.com/author/dave-cornell-phd/ 18 Adaptive Behavior Examples
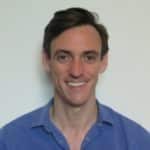
- Chris Drew (PhD) https://helpfulprofessor.com/author/chris-drew-phd-2/ 23 Achieved Status Examples
- Chris Drew (PhD) https://helpfulprofessor.com/author/chris-drew-phd-2/ 15 Ableism Examples
- Chris Drew (PhD) https://helpfulprofessor.com/author/chris-drew-phd-2/ 25 Defense Mechanisms Examples
- Chris Drew (PhD) https://helpfulprofessor.com/author/chris-drew-phd-2/ 15 Theory of Planned Behavior Examples
1 thought on “18 Descriptive Research Examples”
Very nice, educative article. I appreciate the efforts.
Leave a Comment Cancel Reply
Your email address will not be published. Required fields are marked *
Educational resources and simple solutions for your research journey
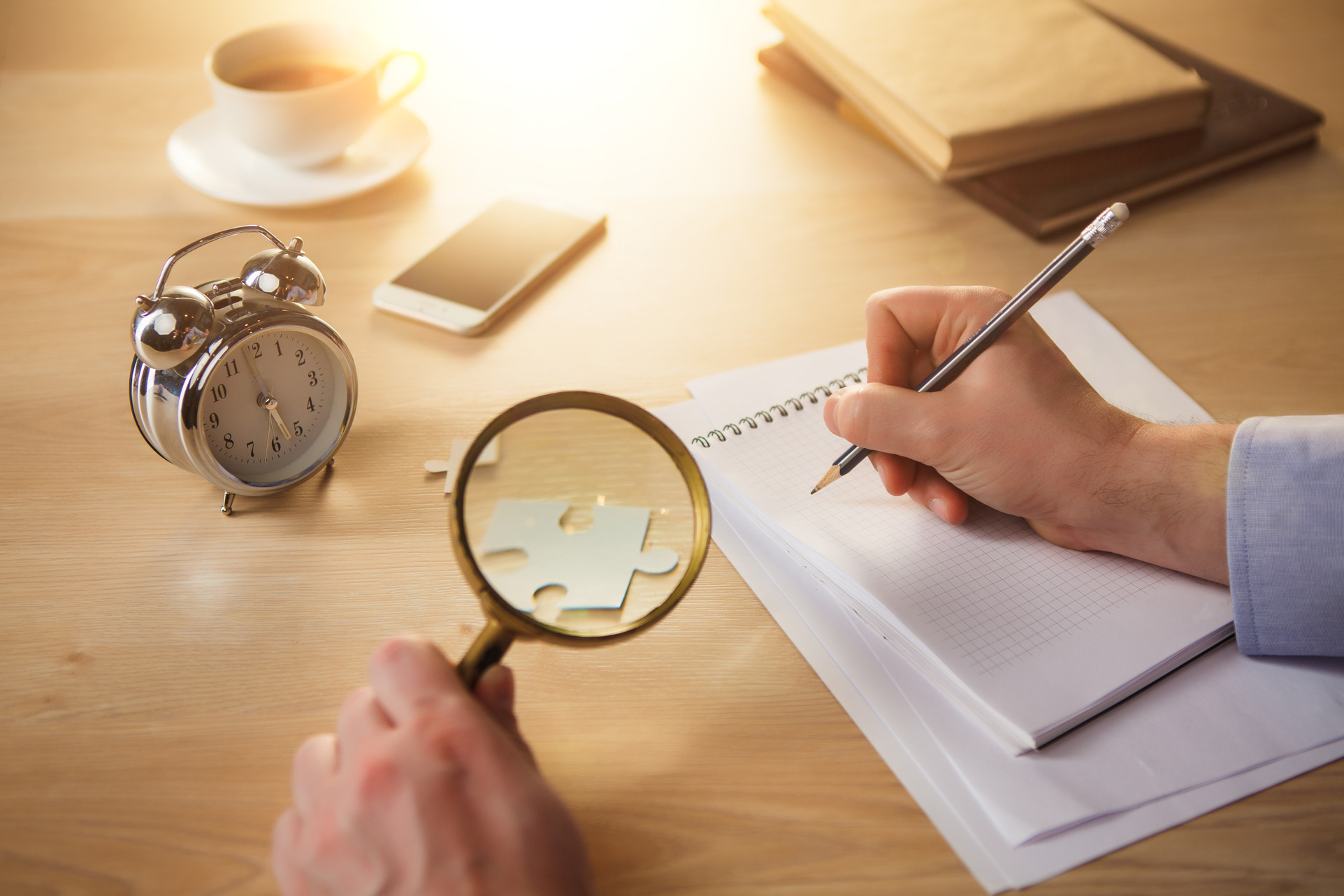
What is Descriptive Research? Definition, Methods, Types and Examples
Descriptive research is a methodological approach that seeks to depict the characteristics of a phenomenon or subject under investigation. In scientific inquiry, it serves as a foundational tool for researchers aiming to observe, record, and analyze the intricate details of a particular topic. This method provides a rich and detailed account that aids in understanding, categorizing, and interpreting the subject matter.
Descriptive research design is widely employed across diverse fields, and its primary objective is to systematically observe and document all variables and conditions influencing the phenomenon.
After this descriptive research definition, let’s look at this example. Consider a researcher working on climate change adaptation, who wants to understand water management trends in an arid village in a specific study area. She must conduct a demographic survey of the region, gather population data, and then conduct descriptive research on this demographic segment. The study will then uncover details on “what are the water management practices and trends in village X.” Note, however, that it will not cover any investigative information about “why” the patterns exist.
Table of Contents
What is descriptive research?
If you’ve been wondering “What is descriptive research,” we’ve got you covered in this post! In a nutshell, descriptive research is an exploratory research method that helps a researcher describe a population, circumstance, or phenomenon. It can help answer what , where , when and how questions, but not why questions. In other words, it does not involve changing the study variables and does not seek to establish cause-and-effect relationships.
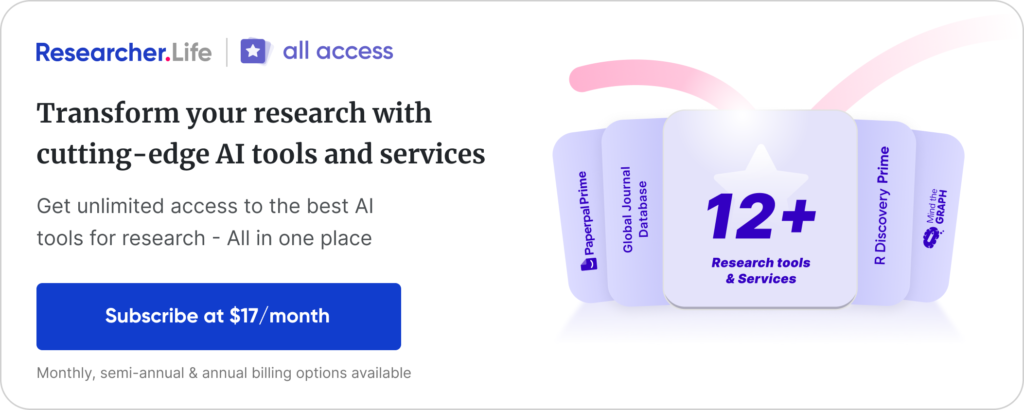
Importance of descriptive research
Now, let’s delve into the importance of descriptive research. This research method acts as the cornerstone for various academic and applied disciplines. Its primary significance lies in its ability to provide a comprehensive overview of a phenomenon, enabling researchers to gain a nuanced understanding of the variables at play. This method aids in forming hypotheses, generating insights, and laying the groundwork for further in-depth investigations. The following points further illustrate its importance:
Provides insights into a population or phenomenon: Descriptive research furnishes a comprehensive overview of the characteristics and behaviors of a specific population or phenomenon, thereby guiding and shaping the research project.
Offers baseline data: The data acquired through this type of research acts as a reference for subsequent investigations, laying the groundwork for further studies.
Allows validation of sampling methods: Descriptive research validates sampling methods, aiding in the selection of the most effective approach for the study.
Helps reduce time and costs: It is cost-effective and time-efficient, making this an economical means of gathering information about a specific population or phenomenon.
Ensures replicability: Descriptive research is easily replicable, ensuring a reliable way to collect and compare information from various sources.
When to use descriptive research design?
Determining when to use descriptive research depends on the nature of the research question. Before diving into the reasons behind an occurrence, understanding the how, when, and where aspects is essential. Descriptive research design is a suitable option when the research objective is to discern characteristics, frequencies, trends, and categories without manipulating variables. It is therefore often employed in the initial stages of a study before progressing to more complex research designs. To put it in another way, descriptive research precedes the hypotheses of explanatory research. It is particularly valuable when there is limited existing knowledge about the subject.
Some examples are as follows, highlighting that these questions would arise before a clear outline of the research plan is established:
- In the last two decades, what changes have occurred in patterns of urban gardening in Mumbai?
- What are the differences in climate change perceptions of farmers in coastal versus inland villages in the Philippines?
Characteristics of descriptive research
Coming to the characteristics of descriptive research, this approach is characterized by its focus on observing and documenting the features of a subject. Specific characteristics are as below.
- Quantitative nature: Some descriptive research types involve quantitative research methods to gather quantifiable information for statistical analysis of the population sample.
- Qualitative nature: Some descriptive research examples include those using the qualitative research method to describe or explain the research problem.
- Observational nature: This approach is non-invasive and observational because the study variables remain untouched. Researchers merely observe and report, without introducing interventions that could impact the subject(s).
- Cross-sectional nature: In descriptive research, different sections belonging to the same group are studied, providing a “snapshot” of sorts.
- Springboard for further research: The data collected are further studied and analyzed using different research techniques. This approach helps guide the suitable research methods to be employed.
Types of descriptive research
There are various descriptive research types, each suited to different research objectives. Take a look at the different types below.
- Surveys: This involves collecting data through questionnaires or interviews to gather qualitative and quantitative data.
- Observational studies: This involves observing and collecting data on a particular population or phenomenon without influencing the study variables or manipulating the conditions. These may be further divided into cohort studies, case studies, and cross-sectional studies:
- Cohort studies: Also known as longitudinal studies, these studies involve the collection of data over an extended period, allowing researchers to track changes and trends.
- Case studies: These deal with a single individual, group, or event, which might be rare or unusual.
- Cross-sectional studies : A researcher collects data at a single point in time, in order to obtain a snapshot of a specific moment.
- Focus groups: In this approach, a small group of people are brought together to discuss a topic. The researcher moderates and records the group discussion. This can also be considered a “participatory” observational method.
- Descriptive classification: Relevant to the biological sciences, this type of approach may be used to classify living organisms.
Descriptive research methods
Several descriptive research methods can be employed, and these are more or less similar to the types of approaches mentioned above.
- Surveys: This method involves the collection of data through questionnaires or interviews. Surveys may be done online or offline, and the target subjects might be hyper-local, regional, or global.
- Observational studies: These entail the direct observation of subjects in their natural environment. These include case studies, dealing with a single case or individual, as well as cross-sectional and longitudinal studies, for a glimpse into a population or changes in trends over time, respectively. Participatory observational studies such as focus group discussions may also fall under this method.
Researchers must carefully consider descriptive research methods, types, and examples to harness their full potential in contributing to scientific knowledge.
Examples of descriptive research
Now, let’s consider some descriptive research examples.
- In social sciences, an example could be a study analyzing the demographics of a specific community to understand its socio-economic characteristics.
- In business, a market research survey aiming to describe consumer preferences would be a descriptive study.
- In ecology, a researcher might undertake a survey of all the types of monocots naturally occurring in a region and classify them up to species level.
These examples showcase the versatility of descriptive research across diverse fields.
Advantages of descriptive research
There are several advantages to this approach, which every researcher must be aware of. These are as follows:
- Owing to the numerous descriptive research methods and types, primary data can be obtained in diverse ways and be used for developing a research hypothesis .
- It is a versatile research method and allows flexibility.
- Detailed and comprehensive information can be obtained because the data collected can be qualitative or quantitative.
- It is carried out in the natural environment, which greatly minimizes certain types of bias and ethical concerns.
- It is an inexpensive and efficient approach, even with large sample sizes
Disadvantages of descriptive research
On the other hand, this design has some drawbacks as well:
- It is limited in its scope as it does not determine cause-and-effect relationships.
- The approach does not generate new information and simply depends on existing data.
- Study variables are not manipulated or controlled, and this limits the conclusions to be drawn.
- Descriptive research findings may not be generalizable to other populations.
- Finally, it offers a preliminary understanding rather than an in-depth understanding.
To reiterate, the advantages of descriptive research lie in its ability to provide a comprehensive overview, aid hypothesis generation, and serve as a preliminary step in the research process. However, its limitations include a potential lack of depth, inability to establish cause-and-effect relationships, and susceptibility to bias.
Frequently asked questions
When should researchers conduct descriptive research.
Descriptive research is most appropriate when researchers aim to portray and understand the characteristics of a phenomenon without manipulating variables. It is particularly valuable in the early stages of a study.
What is the difference between descriptive and exploratory research?
Descriptive research focuses on providing a detailed depiction of a phenomenon, while exploratory research aims to explore and generate insights into an issue where little is known.
What is the difference between descriptive and experimental research?
Descriptive research observes and documents without manipulating variables, whereas experimental research involves intentional interventions to establish cause-and-effect relationships.
Is descriptive research only for social sciences?
No, various descriptive research types may be applicable to all fields of study, including social science, humanities, physical science, and biological science.
How important is descriptive research?
The importance of descriptive research lies in its ability to provide a glimpse of the current state of a phenomenon, offering valuable insights and establishing a basic understanding. Further, the advantages of descriptive research include its capacity to offer a straightforward depiction of a situation or phenomenon, facilitate the identification of patterns or trends, and serve as a useful starting point for more in-depth investigations. Additionally, descriptive research can contribute to the development of hypotheses and guide the formulation of research questions for subsequent studies.
Researcher.Life is a subscription-based platform that unifies top AI tools and services designed to speed up, simplify, and streamline a researcher’s journey, from reading to writing, submission, promotion and more. Based on over 20 years of experience in academia, Researcher.Life empowers researchers to put their best research forward and move closer to success.
Try for free or sign up for the Researcher.Life All Access Pack , a one-of-a-kind subscription that unlocks full access to an AI academic writing assistant, literature reading app, journal finder, scientific illustration tool, and exclusive discounts on professional services from Editage. Find the best AI tools a researcher needs, all in one place – Get All Access now for prices starting at just $17 a month !
Related Posts

What is a Case Study in Research? Definition, Methods, and Examples

Take Top AI Tools for Researchers for a Spin with the Editage All Access 7-Day Pass!

On Evaluating Curricular Effectiveness: Judging the Quality of K-12 Mathematics Evaluations (2004)
Chapter: 5 comparative studies, 5 comparative studies.
It is deceptively simple to imagine that a curriculum’s effectiveness could be easily determined by a single well-designed study. Such a study would randomly assign students to two treatment groups, one using the experimental materials and the other using a widely established comparative program. The students would be taught the entire curriculum, and a test administered at the end of instruction would provide unequivocal results that would permit one to identify the more effective treatment.
The truth is that conducting definitive comparative studies is not simple, and many factors make such an approach difficult. Student placement and curricular choice are decisions that involve multiple groups of decision makers, accrue over time, and are subject to day-to-day conditions of instability, including student mobility, parent preference, teacher assignment, administrator and school board decisions, and the impact of standardized testing. This complex set of institutional policies, school contexts, and individual personalities makes comparative studies, even quasi-experimental approaches, challenging, and thus demands an honest and feasible assessment of what can be expected of evaluation studies (Usiskin, 1997; Kilpatrick, 2002; Schoenfeld, 2002; Shafer, in press).
Comparative evaluation study is an evolving methodology, and our purpose in conducting this review was to evaluate and learn from the efforts undertaken so far and advise on future efforts. We stipulated the use of comparative studies as follows:
A comparative study was defined as a study in which two (or more) curricular treatments were investigated over a substantial period of time (at least one semester, and more typically an entire school year) and a comparison of various curricular outcomes was examined using statistical tests. A statistical test was required to ensure the robustness of the results relative to the study’s design.
We read and reviewed a set of 95 comparative studies. In this report we describe that database, analyze its results, and draw conclusions about the quality of the evaluation database both as a whole and separated into evaluations supported by the National Science Foundation and commercially generated evaluations. In addition to describing and analyzing this database, we also provide advice to those who might wish to fund or conduct future comparative evaluations of mathematics curricular effectiveness. We have concluded that the process of conducting such evaluations is in its adolescence and could benefit from careful synthesis and advice in order to increase its rigor, feasibility, and credibility. In addition, we took an interdisciplinary approach to the task, noting that various committee members brought different expertise and priorities to the consideration of what constitutes the most essential qualities of rigorous and valid experimental or quasi-experimental design in evaluation. This interdisciplinary approach has led to some interesting observations and innovations in our methodology of evaluation study review.
This chapter is organized as follows:
Study counts disaggregated by program and program type.
Seven critical decision points and identification of at least minimally methodologically adequate studies.
Definition and illustration of each decision point.
A summary of results by student achievement in relation to program types (NSF-supported, University of Chicago School Mathematics Project (UCSMP), and commercially generated) in relation to their reported outcome measures.
A list of alternative hypotheses on effectiveness.
Filters based on the critical decision points.
An analysis of results by subpopulations.
An analysis of results by content strand.
An analysis of interactions among content, equity, and grade levels.
Discussion and summary statements.
In this report, we describe our methodology for review and synthesis so that others might scrutinize our approach and offer criticism on the basis of
our methodology and its connection to the results stated and conclusions drawn. In the spirit of scientific, fair, and open investigation, we welcome others to undertake similar or contrasting approaches and compare and discuss the results. Our work was limited by the short timeline set by the funding agencies resulting from the urgency of the task. Although we made multiple efforts to collect comparative studies, we apologize to any curriculum evaluators if comparative studies were unintentionally omitted from our database.
Of these 95 comparative studies, 65 were studies of NSF-supported curricula, 27 were studies of commercially generated materials, and 3 included two curricula each from one of these two categories. To avoid the problem of double coding, two studies, White et al. (1995) and Zahrt (2001), were coded within studies of NSF-supported curricula because more of the classes studied used the NSF-supported curriculum. These studies were not used in later analyses because they did not meet the requirements for the at least minimally methodologically adequate studies, as described below. The other, Peters (1992), compared two commercially generated curricula, and was coded in that category under the primary program of focus. Therefore, of the 95 comparative studies, 67 studies were coded as NSF-supported curricula and 28 were coded as commercially generated materials.
The 11 evaluation studies of the UCSMP secondary program that we reviewed, not including White et al. and Zahrt as previously mentioned, benefit from the maturity of the program, while demonstrating an orientation to both establishing effectiveness and improving a product line. For these reasons, at times we will present the summary of UCSMP’s data separately.
The Saxon materials also present a somewhat different profile from the other commercially generated materials because many of the evaluations of these materials were conducted in the 1980s and the materials were originally developed with a rather atypical program theory. Saxon (1981) designed its algebra materials to combine distributed practice with incremental development. We selected the Saxon materials as a middle grades commercially generated program, and limited its review to middle school studies from 1989 onward when the first National Council of Teachers of Mathematics (NCTM) Standards (NCTM, 1989) were released. This eliminated concerns that the materials or the conditions of educational practice have been altered during the intervening time period. The Saxon materials explicitly do not draw from the NCTM Standards nor did they receive support from the NSF; thus they truly represent a commercial venture. As a result, we categorized the Saxon studies within the group of studies of commercial materials.
At times in this report, we describe characteristics of the database by
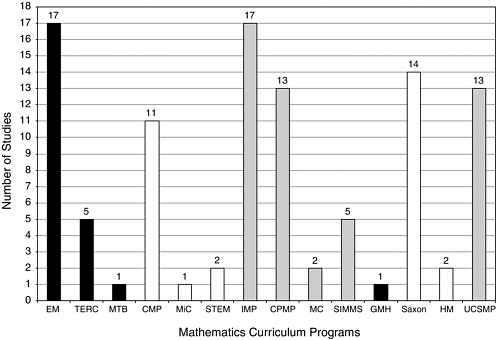
FIGURE 5-1 The distribution of comparative studies across programs. Programs are coded by grade band: black bars = elementary, white bars = middle grades, and gray bars = secondary. In this figure, there are six studies that involved two programs and one study that involved three programs.
NOTE: Five programs (MathScape, MMAP, MMOW/ARISE, Addison-Wesley, and Harcourt) are not shown above since no comparative studies were reviewed.
particular curricular program evaluations, in which case all 19 programs are listed separately. At other times, when we seek to inform ourselves on policy-related issues of funding and evaluating curricular materials, we use the NSF-supported, commercially generated, and UCSMP distinctions. We remind the reader of the artificial aspects of this distinction because at the present time, 18 of the 19 curricula are published commercially. In order to track the question of historical inception and policy implications, a distinction is drawn between the three categories. Figure 5-1 shows the distribution of comparative studies across the 14 programs.
The first result the committee wishes to report is the uneven distribution of studies across the curricula programs. There were 67 coded studies of the NSF curricula, 11 studies of UCSMP, and 17 studies of the commercial publishers. The 14 evaluation studies conducted on the Saxon materials compose the bulk of these 17-non-UCSMP and non-NSF-supported curricular evaluation studies. As these results suggest, we know more about the
evaluations of the NSF-supported curricula and UCSMP than about the evaluations of the commercial programs. We suggest that three factors account for this uneven distribution of studies. First, evaluations have been funded by the NSF both as a part of the original call, and as follow-up to the work in the case of three supplemental awards to two of the curricula programs. Second, most NSF-supported programs and UCSMP were developed at university sites where there is access to the resources of graduate students and research staff. Finally, there was some reported reluctance on the part of commercial companies to release studies that could affect perceptions of competitive advantage. As Figure 5-1 shows, there were quite a few comparative studies of Everyday Mathematics (EM), Connected Mathematics Project (CMP), Contemporary Mathematics in Context (Core-Plus Mathematics Project [CPMP]), Interactive Mathematics Program (IMP), UCSMP, and Saxon.
In the programs with many studies, we note that a significant number of studies were generated by a core set of authors. In some cases, the evaluation reports follow a relatively uniform structure applied to single schools, generating multiple studies or following cohorts over years. Others use a standardized evaluation approach to evaluate sequential courses. Any reports duplicating exactly the same sample, outcome measures, or forms of analysis were eliminated. For example, one study of Mathematics Trailblazers (Carter et al., 2002) reanalyzed the data from the larger ARC Implementation Center study (Sconiers et al., 2002), so it was not included separately. Synthesis studies referencing a variety of evaluation reports are summarized in Chapter 6 , but relevant individual studies that were referenced in them were sought out and included in this comparative review.
Other less formal comparative studies are conducted regularly at the school or district level, but such studies were not included in this review unless we could obtain formal reports of their results, and the studies met the criteria outlined for inclusion in our database. In our conclusions, we address the issue of how to collect such data more systematically at the district or state level in order to subject the data to the standards of scholarly peer review and make it more systematically and fairly a part of the national database on curricular effectiveness.
A standard for evaluation of any social program requires that an impact assessment is warranted only if two conditions are met: (1) the curricular program is clearly specified, and (2) the intervention is well implemented. Absent this assurance, one must have a means of ensuring or measuring treatment integrity in order to make causal inferences. Rossi et al. (1999, p. 238) warned that:
two prerequisites [must exist] for assessing the impact of an intervention. First, the program’s objectives must be sufficiently well articulated to make
it possible to specify credible measures of the expected outcomes, or the evaluator must be able to establish such a set of measurable outcomes. Second, the intervention should be sufficiently well implemented that there is no question that its critical elements have been delivered to appropriate targets. It would be a waste of time, effort, and resources to attempt to estimate the impact of a program that lacks measurable outcomes or that has not been properly implemented. An important implication of this last consideration is that interventions should be evaluated for impact only when they have been in place long enough to have ironed out implementation problems.
These same conditions apply to evaluation of mathematics curricula. The comparative studies in this report varied in the quality of documentation of these two conditions; however, all addressed them to some degree or another. Initially by reviewing the studies, we were able to identify one general design template, which consisted of seven critical decision points and determined that it could be used to develop a framework for conducting our meta-analysis. The seven critical decision points we identified initially were:
Choice of type of design: experimental or quasi-experimental;
For those studies that do not use random assignment: what methods of establishing comparability of groups were built into the design—this includes student characteristics, teacher characteristics, and the extent to which professional development was involved as part of the definition of a curriculum;
Definition of the appropriate unit of analysis (students, classes, teachers, schools, or districts);
Inclusion of an examination of implementation components;
Definition of the outcome measures and disaggregated results by program;
The choice of statistical tests, including statistical significance levels and effect size; and
Recognition of limitations to generalizability resulting from design choices.
These are critical decisions that affect the quality of an evaluation. We further identified a subset of these evaluation studies that met a set of minimum conditions that we termed at least minimally methodologically adequate studies. Such studies are those with the greatest likelihood of shedding light on the effectiveness of these programs. To be classified as at least minimally methodologically adequate, and therefore to be considered for further analysis, each evaluation study was required to:
Include quantifiably measurable outcomes such as test scores, responses to specified cognitive tasks of mathematical reasoning, performance evaluations, grades, and subsequent course taking; and
Provide adequate information to judge the comparability of samples. In addition, a study must have included at least one of the following additional design elements:
A report of implementation fidelity or professional development activity;
Results disaggregated by content strands or by performance by student subgroups; and/or
Multiple outcome measures or precise theoretical analysis of a measured construct, such as number sense, proof, or proportional reasoning.
Using this rubric, the committee identified a subset of 63 comparative studies to classify as at least minimally methodologically adequate and to analyze in depth to inform the conduct of future evaluations. There are those who would argue that any threat to the validity of a study discredits the findings, thus claiming that until we know everything, we know nothing. Others would claim that from the myriad of studies, examining patterns of effects and patterns of variation, one can learn a great deal, perhaps tentatively, about programs and their possible effects. More importantly, we can learn about methodologies and how to concentrate and focus to increase the likelihood of learning more quickly. As Lipsey (1997, p. 22) wrote:
In the long run, our most useful and informative contribution to program managers and policy makers and even to the evaluation profession itself may be the consolidation of our piecemeal knowledge into broader pictures of the program and policy spaces at issue, rather than individual studies of particular programs.
We do not wish to imply that we devalue studies of student affect or conceptions of mathematics, but decided that unless these indicators were connected to direct indicators of student learning, we would eliminate them from further study. As a result of this sorting, we eliminated 19 studies of NSF-supported curricula and 13 studies of commercially generated curricula. Of these, 4 were eliminated for their sole focus on affect or conceptions, 3 were eliminated for their comparative focus on outcomes other than achievement, such as teacher-related variables, and 19 were eliminated for their failure to meet the minimum additional characteristics specified in the criteria above. In addition, six others were excluded from the studies of commercial materials because they were not conducted within the grade-
level band specified by the committee for the selection of that program. From this point onward, all references can be assumed to refer to at least minimally methodologically adequate unless a study is referenced for illustration, in which case we label it with “EX” to indicate that it is excluded in the summary analyses. Studies labeled “EX” are occasionally referenced because they can provide useful information on certain aspects of curricular evaluation, but not on the overall effectiveness.
The at least minimally methodologically adequate studies reported on a variety of grade levels. Figure 5-2 shows the different grade levels of the studies. At times, the choice of grade levels was dictated by the years in which high-stakes tests were given. Most of the studies reported on multiple grade levels, as shown in Figure 5-2 .
Using the seven critical design elements of at least minimally methodologically adequate studies as a design template, we describe the overall database and discuss the array of choices on critical decision points with examples. Following that, we report on the results on the at least minimally methodologically adequate studies by program type. To do so, the results of each study were coded as either statistically significant or not. Those studies
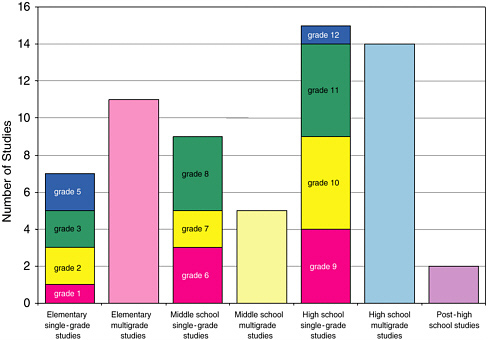
FIGURE 5-2 Single-grade studies by grade and multigrade studies by grade band.
that contained statistically significant results were assigned a percentage of outcomes that are positive (in favor of the treatment curriculum) based on the number of statistically significant comparisons reported relative to the total number of comparisons reported, and a percentage of outcomes that are negative (in favor of the comparative curriculum). The remaining were coded as the percentage of outcomes that are non significant. Then, using seven critical decision points as filters, we identified and examined more closely sets of studies that exhibited the strongest designs, and would therefore be most likely to increase our confidence in the validity of the evaluation. In this last section, we consider alternative hypotheses that could explain the results.
The committee emphasizes that we did not directly evaluate the materials. We present no analysis of results aggregated across studies by naming individual curricular programs because we did not consider the magnitude or rigor of the database for individual programs substantial enough to do so. Nevertheless, there are studies that provide compelling data concerning the effectiveness of the program in a particular context. Furthermore, we do report on individual studies and their results to highlight issues of approach and methodology and to remain within our primary charge, which was to evaluate the evaluations, we do not summarize results of the individual programs.
DESCRIPTION OF COMPARATIVE STUDIES DATABASE ON CRITICAL DECISION POINTS
An experimental or quasi-experimental design.
We separated the studies into experimental and quasiexperimental, and found that 100 percent of the studies were quasiexperimental (Campbell and Stanley, 1966; Cook and Campbell, 1979; and Rossi et al., 1999). 1 Within the quasi-experimental studies, we identified three subcategories of comparative study. In the first case, we identified a study as cross-curricular comparative if it compared the results of curriculum A with curriculum B. A few studies in this category also compared two samples within the curriculum to each other and specified different conditions such as high and low implementation quality.
A second category of a quasi-experimental study involved comparisons that could shed light on effectiveness involving time series studies. These studies compared the performance of a sample of students in a curriculum
| One study, by Peters (1992), used random assignment to two classrooms, but was classified as quasi-experimental with its sample size and use of qualitative methods. |
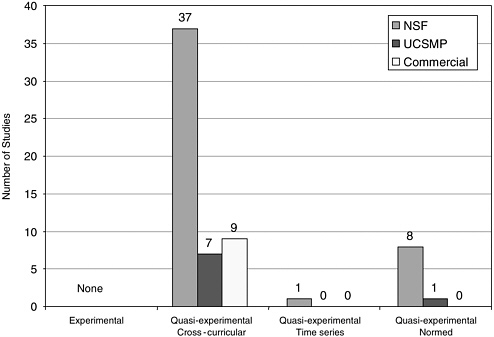
FIGURE 5-3 The number of comparative studies in each category.
under investigation across time, such as in a longitudinal study of the same students over time. A third category of comparative study involved a comparison to some form of externally normed results, such as populations taking state, national, or international tests or prior research assessment from a published study or studies. We categorized these studies and divided them into NSF, UCSMP, and commercial and labeled them by the categories above ( Figure 5-3 ).
In nearly all studies in the comparative group, the titles of experimental curricula were explicitly identified. The only exception to this was the ARC Implementation Center study (Sconiers et al., 2002), where three NSF-supported elementary curricula were examined, but in the results, their effects were pooled. In contrast, in the majority of the cases, the comparison curriculum is referred to simply as “traditional.” In only 22 cases were comparisons made between two identified curricula. Many others surveyed the array of curricula at comparison schools and reported on the most frequently used, but did not identify a single curriculum. This design strategy is used often because other factors were used in selecting comparison groups, and the additional requirement of a single identified curriculum in
these sites would often make it difficult to match. Studies were categorized into specified (including a single or multiple identified curricula) and nonspecified curricula. In the 63 studies, the central group was compared to an NSF-supported curriculum (1), an unnamed traditional curriculum (41), a named traditional curriculum (19), and one of the six commercial curricula (2). To our knowledge, any systematic impact of such a decision on results has not been studied, but we express concern that when a specified curriculum is compared to an unspecified content which is a set of many informal curriculum, the comparison may favor the coherency and consistency of the single curricula, and we consider this possibility subsequently under alternative hypotheses. We believe that a quality study should at least report the array of curricula that comprise the comparative group and include a measure of the frequency of use of each, but a well-defined alternative is more desirable.
If a study was both longitudinal and comparative, then it was coded as comparative. When studies only examined performances of a group over time, such as in some longitudinal studies, it was coded as quasi-experimental normed. In longitudinal studies, the problems created by student mobility were evident. In one study, Carroll (2001), a five-year longitudinal study of Everyday Mathematics, the sample size began with 500 students, 24 classrooms, and 11 schools. By 2nd grade, the longitudinal sample was 343. By 3rd grade, the number of classes increased to 29 while the number of original students decreased to 236 students. At the completion of the study, approximately 170 of the original students were still in the sample. This high rate of attrition from the study suggests that mobility is a major challenge in curricular evaluation, and that the effects of curricular change on mobile students needs to be studied as a potential threat to the validity of the comparison. It is also a challenge in curriculum implementation because students coming into a program do not experience its cumulative, developmental effect.
Longitudinal studies also have unique challenges associated with outcome measures, a study by Romberg et al. (in press) (EX) discussed one approach to this problem. In this study, an external assessment system and a problem-solving assessment system were used. In the External Assessment System, items from the National Assessment of Educational Progress (NAEP) and Third International Mathematics and Science Survey (TIMSS) were balanced across four strands (number, geometry, algebra, probability and statistics), and 20 items of moderate difficulty, called anchor items, were repeated on each grade-specific assessment (p. 8). Because the analyses of the results are currently under way, the evaluators could not provide us with final results of this study, so it is coded as EX.
However, such longitudinal studies can provide substantial evidence of the effects of a curricular program because they may be more sensitive to an
TABLE 5-1 Scores in Percentage Correct by Everyday Mathematics Students and Various Comparison Groups Over a Five-Year Longitudinal Study
| Sample Size | 1st Grade | 2nd Grade | 3rd Grade | 4th Grade | 5th Grade |
EM | n=170-503 | 58 | 62 | 61 | 71 | 75 |
Traditional U.S. | n=976 | 43 | 53.5 |
|
| 44 |
Japanese | n=750 | 64 | 71 |
|
| 80 |
Chinese | n=1,037 | 52 |
|
|
| 76 |
NAEP Sample | n=18,033 |
|
| 44 | 44 |
|
NOTE: 1st grade: 44 items; 2nd grade: 24 items; 3rd grade: 22 items; 4th grade: 29 items; and 5th grade: 33 items. SOURCE: Adapted from Carroll (2001). |
accumulation of modest effects and/or can reveal whether the rates of learning change over time within curricular change.
The longitudinal study by Carroll (2001) showed that the effects of curricula may often accrue over time, but measurements of achievement present challenges to drawing such conclusions as the content and grade level change. A variety of measures were used over time to demonstrate growth in relation to comparison groups. The author chose a set of measures used previously in studies involving two Asian samples and an American sample to provide a contrast to the students in EM over time. For 3rd and 4th grades, where the data from the comparison group were not available, the authors selected items from the NAEP to bridge the gap. Table 5-1 summarizes the scores of the different comparative groups over five years. Scores are reported as the mean percentage correct for a series of tests on number computation, number concepts and applications, geometry, measurement, and data analysis.
It is difficult to compare performances on different tests over different groups over time against a single longitudinal group from EM, and it is not possible to determine whether the students’ performance is increasing or whether the changes in the tests at each grade level are producing the results; thus the results from longitudinal studies lacking a control group or use of sophisticated methodological analysis may be suspect and should be interpreted with caution.
In the Hirsch and Schoen (2002) study, based on a sample of 1,457 students, scores on Ability to Do Quantitative Thinking (ITED-Q) a subset of the Iowa Tests of Education Development, students in Core-Plus showed increasing performance over national norms over the three-year time period. The authors describe the content of the ITED-Q test and point out
that “although very little symbolic algebra is required, the ITED-Q is quite demanding for the full range of high school students” (p. 3). They further point out that “[t]his 3-year pattern is consistent, on average, in rural, urban, and suburban schools, for males and females, for various minority groups, and for students for whom English was not their first language” (p. 4). In this case, one sees that studies over time are important as results over shorter periods may mask cumulative effects of consistent and coherent treatments and such studies could also show increases that do not persist when subject to longer trajectories. One approach to longitudinal studies was used by Webb and Dowling in their studies of the Interactive Mathematics Program (Webb and Dowling, 1995a, 1995b, 1995c). These researchers conducted transcript analyses as a means to examine student persistence and success in subsequent course taking.
The third category of quasi-experimental comparative studies measured student outcomes on a particular curricular program and simply compared them to performance on national tests or international tests. When these tests were of good quality and were representative of a genuine sample of a relevant population, such as NAEP reports or TIMSS results, the reports often provided one a reasonable indicator of the effects of the program if combined with a careful description of the sample. Also, sometimes the national tests or state tests used were norm-referenced tests producing national percentiles or grade-level equivalents. The normed studies were considered of weaker quality in establishing effectiveness, but were still considered valid as examples of comparing samples to populations.
For Studies That Do Not Use Random Assignment: What Methods of Establishing Comparability Across Groups Were Built into the Design
The most fundamental question in an evaluation study is whether the treatment has had an effect on the chosen criterion variable. In our context, the treatment is the curriculum materials, and in some cases, related professional development, and the outcome of interest is academic learning. To establish if there is a treatment effect, one must logically rule out as many other explanations as possible for the differences in the outcome variable. There is a long tradition on how this is best done, and the principle from a design point of view is to assure that there are no differences between the treatment conditions (especially in these evaluations, often there are only the new curriculum materials to be evaluated and a control group) either at the outset of the study or during the conduct of the study.
To ensure the first condition, the ideal procedure is the random assignment of the appropriate units to the treatment conditions. The second condition requires that the treatment is administered reliably during the length of the study, and is assured through the careful observation and
control of the situation. Without randomization, there are a host of possible confounding variables that could differ among the treatment conditions and that are related themselves to the outcome variables. Put another way, the treatment effect is a parameter that the study is set up to estimate. Statistically, an estimate that is unbiased is desired. The goal is that its expected value over repeated samplings is equal to the true value of the parameter. Without randomization at the onset of a study, there is no way to assure this property of unbiasness. The variables that differ across treatment conditions and are related to the outcomes are confounding variables, which bias the estimation process.
Only one study we reviewed, Peters (1992), used randomization in the assignment of students to treatments, but that occurred because the study was limited to one teacher teaching two sections and included substantial qualitative methods, so we coded it as quasi-experimental. Others report partially assigning teachers randomly to treatment conditions (Thompson, et al., 2001; Thompson et al., 2003). Two primary reasons seem to account for a lack of use of pure experimental design. To justify the conduct and expense of a randomized field trial, the program must be described adequately and there must be relative assurance that its implementation has occurred over the duration of the experiment (Peterson et al., 1999). Additionally, one must be sure that the outcome measures are appropriate for the range of performances in the groups and valid relative to the curricula under investigation. Seldom can such conditions be assured for all students and teachers and over the duration of a year or more.
A second reason is that random assignment of classrooms to curricular treatment groups typically is not permitted or encouraged under normal school conditions. As one evaluator wrote, “Building or district administrators typically identified teachers who would be in the study and in only a few cases was random assignment of teachers to UCSMP Algebra or comparison classes possible. School scheduling and teacher preference were more important factors to administrators and at the risk of losing potential sites, we did not insist on randomization” (Mathison et al., 1989, p. 11).
The Joint Committee on Standards for Educational Evaluation (1994, p. 165) committee of evaluations recognized the likelihood of limitations on randomization, writing:
The groups being compared are seldom formed by random assignment. Rather, they tend to be natural groupings that are likely to differ in various ways. Analytical methods may be used to adjust for these initial differences, but these methods are based upon a number of assumptions. As it is often difficult to check such assumptions, it is advisable, when time and resources permit, to use several different methods of analysis to determine whether a replicable pattern of results is obtained.
Does the dearth of pure experimentation render the results of the studies reviewed worthless? Bias is not an “either-or” proposition, but it is a quantity of varying degrees. Through careful measurement of the most salient potential confounding variables, precise theoretical description of constructs, and use of these methods of statistical analysis, it is possible to reduce the amount of bias in the estimated treatment effect. Identification of the most likely confounding variables and their measurement and subsequent adjustments can greatly reduce bias and help estimate an effect that is likely to be more reflective of the true value. The theoretical fully specified model is an alternative to randomization by including relevant variables and thus allowing the unbiased estimation of the parameter. The only problem is realizing when the model is fully specified.
We recognized that we can never have enough knowledge to assure a fully specified model, especially in the complex and unstable conditions of schools. However, a key issue in determining the degree of confidence we have in these evaluations is to examine how they have identified, measured, or controlled for such confounding variables. In the next sections, we report on the methods of the evaluators in identifying and adjusting for such potential confounding variables.
One method to eliminate confounding variables is to examine the extent to which the samples investigated are equated either by sample selection or by methods of statistical adjustments. For individual students, there is a large literature suggesting the importance of social class to achievement. In addition, prior achievement of students must be considered. In the comparative studies, investigators first identified participation of districts, schools, or classes that could provide sufficient duration of use of curricular materials (typically two years or more), availability of target classes, or adequate levels of use of program materials. Establishing comparability was a secondary concern.
These two major factors were generally used in establishing the comparability of the sample:
Student population characteristics, such as demographic characteristics of students in terms of race/ethnicity, economic levels, or location type (urban, suburban, or rural).
Performance-level characteristics such as performance on prior tests, pretest performance, percentage passing standardized tests, or related measures (e.g., problem solving, reading).
In general, four methods of comparing groups were used in the studies we examined, and they permit different degrees of confidence in their results. In the first type, a matching class, school, or district was identified.
Studies were coded as this type if specified characteristics were used to select the schools systematically. In some of these studies, the methodology was relatively complex as correlates of performance on the outcome measures were found empirically and matches were created on that basis (Schneider, 2000; Riordan and Noyce, 2001; and Sconiers et al., 2002). For example, in the Sconiers et al. study, where the total sample of more than 100,000 students was drawn from five states and three elementary curricula are reviewed (Everyday Mathematics, Math Trailblazers [MT], and Investigations [IN], a highly systematic method was developed. After defining eligibility as a “reform school,” evaluators conducted separate regression analyses for the five states at each tested grade level to identify the strongest predictors of average school mathematics score. They reported, “reading score and low-income variables … consistently accounted for the greatest percentage of total variance. These variables were given the greatest weight in the matching process. Other variables—such as percent white, school mobility rate, and percent with limited English proficiency (LEP)—accounted for little of the total variance but were typically significant. These variables were given less weight in the matching process” (Sconiers et al., 2002, p. 10). To further provide a fair and complete comparison, adjustments were made based on regression analysis of the scores to minimize bias prior to calculating the difference in scores and reporting effect sizes. In their results the evaluators report, “The combined state-grade effect sizes for math and total are virtually identical and correspond to a percentile change of about 4 percent favoring the reform students” (p. 12).
A second type of matching procedure was used in the UCSMP evaluations. For example, in an evaluation centered on geometry learning, evaluators advertised in NCTM and UCSMP publications, and set conditions for participation from schools using their program in terms of length of use and grade level. After selecting schools with heterogeneous grouping and no tracking, the researchers used a match-pair design where they selected classes from the same school on the basis of mathematics ability. They used a pretest to determine this, and because the pretest consisted of two parts, they adjusted their significance level using the Bonferroni method. 2 Pairs were discarded if the differences in means and variance were significant for all students or for those students completing all measures, or if class sizes became too variable. In the algebra study, there were 20 pairs as a result of the matching, and because they were comparing three experimental conditions—first edition, second edition, and comparison classes—in the com-
| The Bonferroni method is a simple method that allows multiple comparison statements to be made (or confidence intervals to be constructed) while still assuring that an overall confidence coefficient is maintained. |
parison study relevant to this review, their matching procedure identified 8 pairs. When possible, teachers were assigned randomly to treatment conditions. Most results are presented with the eight identified pairs and an accumulated set of means. The outcomes of this particular study are described below in a discussion of outcome measures (Thompson et al., 2003).
A third method was to measure factors such as prior performance or socio-economic status (SES) based on pretesting, and then to use analysis of covariance or multiple regression in the subsequent analysis to factor in the variance associated with these factors. These studies were coded as “control.” A number of studies of the Saxon curricula used this method. For example, Rentschler (1995) conducted a study of Saxon 76 compared to Silver Burdett with 7th graders in West Virginia. He reported that the groups differed significantly in that the control classes had 65 percent of the students on free and reduced-price lunch programs compared to 55 percent in the experimental conditions. He used scores on California Test of Basic Skills mathematics computation and mathematics concepts and applications as his pretest scores and found significant differences in favor of the experimental group. His posttest scores showed the Saxon experimental group outperformed the control group on both computation and concepts and applications. Using analysis of covariance, the computation difference in favor of the experimental group was statistically significant; however, the difference in concepts and applications was adjusted to show no significant difference at the p < .05 level.
A fourth method was noted in studies that used less rigorous methods of selection of sample and comparison of prior achievement or similar demographics. These studies were coded as “compare.” Typically, there was no explicit procedure to decide if the comparison was good enough. In some of the studies, it appeared that the comparison was not used as a means of selection, but rather as a more informal device to convince the reader of the plausibility of the equivalence of the groups. Clearly, the studies that used a more precise method of selection were more likely to produce results on which one’s confidence in the conclusions is greater.
Definition of Unit of Analysis
A major decision in forming an evaluation design is the unit of analysis. The unit of selection or randomization used to assign elements to treatment and control groups is closely linked to the unit of analysis. As noted in the National Research Council (NRC) report (1992, p. 21):
If one carries out the assignment of treatments at the level of schools, then that is the level that can be justified for causal analysis. To analyze the results at the student level is to introduce a new, nonrandomized level into
the study, and it raises the same issues as does the nonrandomized observational study…. The implications … are twofold. First, it is advisable to use randomization at the level at which units are most naturally manipulated. Second, when the unit of observation is at a “lower” level of aggregation than the unit of randomization, then for many purposes the data need to be aggregated in some appropriate fashion to provide a measure that can be analyzed at the level of assignment. Such aggregation may be as simple as a summary statistic or as complex as a context-specific model for association among lower-level observations.
In many studies, inadequate attention was paid to the fact that the unit of selection would later become the unit of analysis. The unit of analysis, for most curriculum evaluators, needs to be at least the classroom, if not the school or even the district. The units must be independently responding units because instruction is a group process. Students are not independent, the classroom—even if the teachers work together in a school on instruction—is not entirely independent, so the school is the unit. Care needed to be taken to ensure that an adequate numbers of units would be available to have sufficient statistical power to detect important differences.
A curriculum is experienced by students in a group, and this implies that individual student responses and what they learn are correlated. As a result, the appropriate unit of assignment and analysis must at least be defined at the classroom or teacher level. Other researchers (Bryk et al., 1993) suggest that the unit might be better selected at an even higher level of aggregation. The school itself provides a culture in which the curriculum is enacted as it is influenced by the policies and assignments of the principal, by the professional interactions and governance exhibited by the teachers as a group, and by the community in which the school resides. This would imply that the school might be the appropriate unit of analysis. Even further, to the extent that such decisions about curriculum are made at the district level and supported through resources and professional development at that level, the appropriate unit could arguably be the district. On a more practical level, we found that arguments can be made for a variety of decisions on the selection of units, and what is most essential is to make a clear argument for one’s choice, to use the same unit in the analysis as in the sample selection process, and to recognize the potential limits to generalization that result from one’s decisions.
We would argue in all cases that reports of how sites are selected must be explicit in the evaluation report. For example, one set of evaluation studies selected sites by advertisements in a journal distributed by the program and in NCTM journals (UCSMP) (Thompson et al., 2001; Thompson et al., 2003). The samples in their studies tended to be affluent suburban populations and predominantly white populations. Other conditions of inclusion, such as frequency of use also might have influenced this outcome,
but it is important that over a set of studies on effectiveness, all populations of students be adequately sampled. When a study is not randomized, adjustments for these confounding variables should be included. In our analysis of equity, we report on the concerns about representativeness of the overall samples and their impact on the generalizability of the results.
Implementation Components
The complexity of doing research on curricular materials introduces a number of possible confounding variables. Due to the documented complexity of curricular implementation, most comparative study evaluators attempt to monitor implementation in some fashion. A valuable outcome of a well-conducted evaluation is to determine not only if the experimental curriculum could ideally have a positive impact on learning, but whether it can survive or thrive in the conditions of schooling that are so variable across sites. It is essential to know what the treatment was, whether it occurred, and if so, to what degree of intensity, fidelity, duration, and quality. In our model in Chapter 3 , these factors were referred to as “implementation components.” Measuring implementation can be costly for large-scale comparative studies; however, many researchers have shown that variation in implementation is a key factor in determining effectiveness. In coding the comparative studies, we identified three types of components that help to document the character of the treatment: implementation fidelity, professional development treatments, and attention to teacher effects.
Implementation Fidelity
Implementation fidelity is a measure of the basic extent of use of the curricular materials. It does not address issues of instructional quality. In some studies, implementation fidelity is synonymous with “opportunity to learn.” In examining implementation fidelity, a variety of data were reported, including, most frequently, the extent of coverage of the curricular material, the consistency of the instructional approach to content in relation to the program’s theory, reports of pedagogical techniques, and the length of use of the curricula at the sample sites. Other less frequently used approaches documented the calendar of curricular coverage, requested teacher feedback by textbook chapter, conducted student surveys, and gauged homework policies, use of technology, and other particular program elements. Interviews with teachers and students, classroom surveys, and observations were the most frequently used data-gathering techniques. Classroom observations were conducted infrequently in these studies, except in cases when comparative studies were combined with case studies, typically with small numbers of schools and classes where observations
were conducted for long or frequent time periods. In our analysis, we coded only the presence or absence of one or more of these methods.
If the extent of implementation was used in interpreting the results, then we classified the study as having adjusted for implementation differences. Across all 63 at least minimally methodologically adequate studies, 44 percent reported some type of implementation fidelity measure, 3 percent reported and adjusted for it in interpreting their outcome measures, and 53 percent recorded no information on this issue. Differences among studies, by study type (NSF, UCSMP, and commercially generated), showed variation on this issue, with 46 percent of NSF reporting or adjusting for implementation, 75 percent of UCSMP, and only 11 percent of the other studies of commercial materials doing so. Of the commercial, non-UCSMP studies included, only one reported on implementation. Possibly, the evaluators for the NSF and UCSMP Secondary programs recognized more clearly that their programs demanded significant changes in practice that could affect their outcomes and could pose challenges to the teachers assigned to them.
A study by Abrams (1989) (EX) 3 on the use of Saxon algebra by ninth graders showed that concerns for implementation fidelity extend to all curricula, even those like Saxon whose methods may seem more likely to be consistent with common practice. Abrams wrote, “It was not the intent of this study to determine the effectiveness of the Saxon text when used as Saxon suggests, but rather to determine the effect of the text as it is being used in the classroom situations. However, one aspect of the research was to identify how the text is being taught, and how closely teachers adhere to its content and the recommended presentation” (p. 7). Her findings showed that for the 9 teachers and 300 students, treatment effects favoring the traditional group (using Dolciani’s Algebra I textbook, Houghton Mifflin, 1980) were found on the algebra test, the algebra knowledge/skills subtest, and the problem-solving test for this population of teachers (fixed effect). No differences were found between the groups on an algebra understanding/applications subtest, overall attitude toward mathematics, mathematical self-confidence, anxiety about mathematics, or enjoyment of mathematics. She suggests that the lack of differences might be due to the ways in which teachers supplement materials, change test conditions, emphasize
| Both studies referenced in this section did not meet the criteria for inclusion in the comparative studies, but shed direct light on comparative issues of implementation. The Abrams study was omitted because it examined a program at a grade level outside the specified grade band for that curriculum. Briars and Resnick (2000) did not provide explicit comparison scores to permit one to evaluate the level of student attainment. |
and deemphasize topics, use their own tests, vary the proportion of time spent on development and practice, use calculators and group work, and basically adapt the materials to their own interpretation and method. Many of these practices conflict directly with the recommendations of the authors of the materials.
A study by Briars and Resnick (2000) (EX) in Pittsburgh schools directly confronted issues relevant to professional development and implementation. Evaluators contrasted the performance of students of teachers with high and low implementation quality, and showed the results on two contrasting outcome measures, Iowa Test of Basic Skills (ITBS) and Balanced Assessment. Strong implementers were defined as those who used all of the EM components and provided student-centered instruction by giving students opportunities to explore mathematical ideas, solve problems, and explain their reasoning. Weak implementers were either not using EM or using it so little that the overall instruction in the classrooms was “hardly distinguishable from traditional mathematics instruction” (p. 8). Assignment was based on observations of student behavior in classes, the presence or absence of manipulatives, teacher questionnaires about the programs, and students’ knowledge of classroom routines associated with the program.
From the identification of strong- and weak-implementing teachers, strong- and weak-implementation schools were identified as those with strong- or weak-implementing teachers in 3rd and 4th grades over two consecutive years. The performance of students with 2 years of EM experience in these settings composed the comparative samples. Three pairs of strong- and weak-implementation schools with similar demographics in terms of free and reduced-price lunch (range 76 to 93 percent), student living with only one parent (range 57 to 82 percent), mobility (range 8 to 16 percent), and ethnicity (range 43 to 98 percent African American) were identified. These students’ 1st-grade ITBS scores indicated similarity in prior performance levels. Finally, evaluators predicted that if the effects were due to the curricular implementation and accompanying professional development, the effects on scores should be seen in 1998, after full implementation. Figure 5-4 shows that on the 1998 New Standards exams, placement in strong- and weak-implementation schools strongly affected students’ scores. Over three years, performance in the district on skills, concepts, and problem solving rose, confirming the evaluator’s predictions.
An article by McCaffrey et al. (2001) examining the interactions among instructional practices, curriculum, and student achievement illustrates the point that distinctions are often inadequately linked to measurement tools in their treatment of the terms traditional and reform teaching. In this study, researchers conducted an exploratory factor analysis that led them to create two scales for instructional practice: Reform Practices and Tradi-
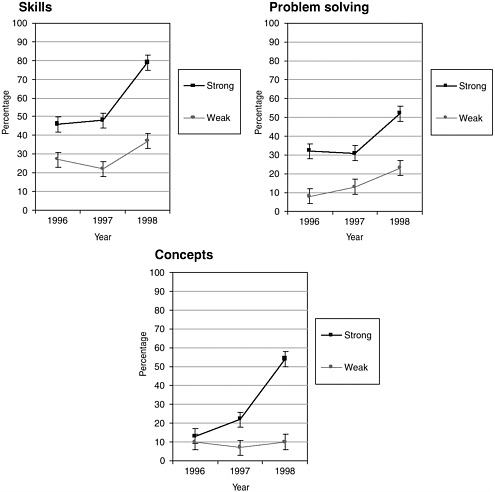
FIGURE 5-4 Percentage of students who met or exceeded the standard. Districtwide grade 4 New Standards Mathematics Reference Examination (NSMRE) performance for 1996, 1997, and 1998 by level of Everyday Mathematics implementation. Percentage of students who achieved the standard. Error bars denote the 99 percent confidence interval for each data point.
SOURCE: Re-created from Briars and Resnick (2000, pp. 19-20).
tional Practices. The reform scale measured the frequency, by means of teacher report, of teacher and student behaviors associated with reform instruction and assessment practices, such as using small-group work, explaining reasoning, representing and using data, writing reflections, or performing tasks in groups. The traditional scale focused on explanations to whole classes, the use of worksheets, practice, and short-answer assessments. There was a –0.32 correlation between scores for integrated curriculum teachers. There was a 0.27 correlation between scores for traditional
curriculum teachers. This shows that it is overly simplistic to think that reform and traditional practices are oppositional. The relationship among a variety of instructional practices is rather more complex as they interact with curriculum and various student populations.
Professional Development
Professional development and teacher effects were separated in our analysis from implementation fidelity. We recognized that professional development could be viewed by the readers of this report in two ways. As indicated in our model, professional development can be considered a program element or component or it can be viewed as part of the implementation process. When viewed as a program element, professional development resources are considered mandatory along with program materials. In relation to evaluation, proponents of considering professional development as a mandatory program element argue that curricular innovations, which involve the introduction of new topics, new types of assessment, or new ways of teaching, must make provision for adequate training, just as with the introduction of any new technology.
For others, the inclusion of professional development in the program elements without a concomitant inclusion of equal amounts of professional development relevant to a comparative treatment interjects a priori disproportionate treatments and biases the results. We hoped for an array of evaluation studies that might shed some empirical light on this dispute, and hence separated professional development from treatment fidelity, coding whether or not studies reported on the amount of professional development provided for the treatment and/or comparison groups. A study was coded as positive if it either reported on the professional development provided on the experimental group or reported the data on both treatments. Across all 63 at least minimally methodologically adequate studies, 27 percent reported some type of professional development measure, 1.5 percent reported and adjusted for it in interpreting their outcome measures, and 71.5 percent recorded no information on the issue.
A study by Collins (2002) (EX) 4 illustrates the critical and controversial role of professional development in evaluation. Collins studied the use of Connected Math over three years, in three middle schools in threat of being classified as low performing in the Massachusetts accountability system. A comparison was made between one school (School A) that engaged
| The Collins study lacked a comparison group and is coded as EX. However, it is reported as a case study. |
substantively in professional development opportunities accompanying the program and two that did not (Schools B and C). In the CMP school reports (School A) totals between 100 and 136 hours of professional development were recorded for all seven teachers in grades 6 through 8. In School B, 66 hours were reported for two teachers and in School C, 150 hours were reported for eight teachers over three years. Results showed significant differences in the subsequent performance by students at the school with higher participation in professional development (School A) and it became a districtwide top performer; the other two schools remained at risk for low performance. No controls for teacher effects were possible, but the results do suggest the centrality of professional development for successful implementation or possibly suggest that the results were due to professional development rather than curriculum materials. The fact that these two interpretations cannot be separated is a problem when professional development is given to one and not the other. The effect could be due to textbook or professional development or an interaction between the two. Research designs should be adjusted to consider these issues when different conditions of professional development are provided.
Teacher Effects
These studies make it obvious that there are potential confounding factors of teacher effects. Many evaluation studies devoted inadequate attention to the variable of teacher quality. A few studies (Goodrow, 1998; Riordan and Noyce, 2001; Thompson et al., 2001; and Thompson et al., 2003) reported on teacher characteristics such as certification, length of service, experience with curricula, or degrees completed. Those studies that matched classrooms and reported by matched results rather than aggregated results sought ways to acknowledge the large variations among teacher performance and its impact on student outcomes. We coded any effort to report on possible teacher effects as one indicator of quality. Across all 63 at least minimally methodologically adequate studies, 16 percent reported some type of teacher effect measure, 3 percent reported and adjusted for it in interpreting their outcome measures, and 81 percent recorded no information on this issue.
One can see that the potential confounding factors of teacher effects, in terms of the provision of professional development or the measure of teacher effects, are not adequately considered in most evaluation designs. Some studies mention and give a subjective judgment as to the nature of the problem, but this is descriptive at the most. Hardly any of the studies actually do anything analytical, and because these are such important potential confounding variables, this presents a serious challenge to the efficacy of these studies. Figure 5-5 shows how attention to these factors varies
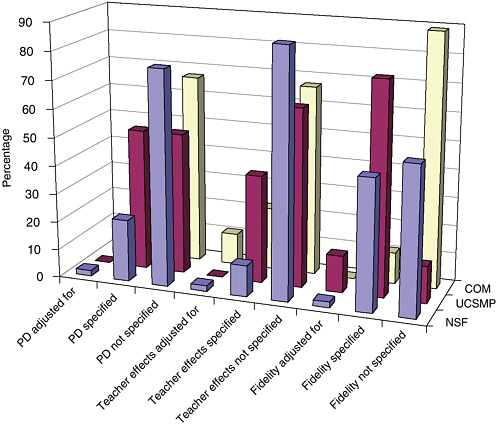
FIGURE 5-5 Treatment of implementation components by program type.
NOTE: PD = professional development.
across program categories among NSF-supported, UCSMP, and studies of commercial materials. In general, evaluations of NSF-supported studies were the most likely to measure these variables; UCSMP had the most standardized use of methods to do so across studies; and commercial material evaluators seldom reported on issues of implementation fidelity.
Identification of a Set of Outcome Measures and Forms of Disaggregation
Using the selected student outcomes identified in the program theory, one must conduct an impact assessment that refers to the design and measurement of student outcomes. In addition to selecting what outcomes should be measured within one’s program theory, one must determine how these outcomes are measured, when those measures are collected, and what
purpose they serve from the perspective of the participants. In the case of curricular evaluation, there are significant issues involved in how these measures are reported. To provide insight into the level of curricular validity, many evaluators prefer to report results by topic, content strand, or item cluster. These reports often present the level of specificity of outcome needed to inform curriculum designers, especially when efforts are made to document patterns of errors, distribution of results across multiple choices, or analyses of student methods. In these cases, whole test scores may mask essential differences in impact among curricula at the level of content topics, reporting only average performance.
On the other hand, many large-scale assessments depend on methods of test equating that rely on whole test scores and make comparative interpretations of different test administrations by content strands of questionable reliability. Furthermore, there are questions such as whether to present only gain scores effect sizes, how to link pretests and posttests, and how to determine the relative curricular sensitivity of various outcome measures.
The findings of comparative studies are reported in terms of the outcome measure(s) collected. To describe the nature of the database with regard to outcome measures and to facilitate our analyses of the studies, we classified each of the included studies on four outcome measure dimensions:
Total score reported;
Disaggregation of content strands, subtest, performance level, SES, or gender;
Outcome measure that was specific to curriculum; and
Use of multiple outcome measures.
Most studies reported a total score, but we did find studies that reported only subtest scores or only scores on an item-by-item basis. For example, in the Ben-Chaim et al. (1998) evaluation study of Connected Math, the authors were interested in students’ proportional reasoning proficiency as a result of use of this curriculum. They asked students from eight seventh-grade classes of CMP and six seventh-grade classes from the control group to solve a variety of tasks categorized as rate and density problems. The authors provide precise descriptions of the cognitive challenges in the items; however, they do not explain if the problems written up were representative of performance on a larger set of items. A special rating form was developed to code responses in three major categories (correct answer, incorrect answer, and no response), with subcategories indicating the quality of the work that accompanied the response. No reports on reliability of coding were given. Performance on standardized tests indicated that control students’ scores were slightly higher than CMP at the beginning of the
year and lower at the end. Twenty-five percent of the experimental group members were interviewed about their approaches to the problems. The CMP students outperformed the control students (53 percent versus 28 percent) overall in providing the correct answers and support work, and 27 percent of the control group gave an incorrect answer or showed incorrect thinking compared to 13 percent of the CMP group. An item-level analysis permitted the researchers to evaluate the actual strategies used by the students. They reported, for example, that 82 percent of CMP students used a “strategy focused on package price, unit price, or a combination of the two; those effective strategies were used by only 56 of 91 control students (62 percent)” (p. 264).
The use of item or content strand-level comparative reports had the advantage that they permitted the evaluators to assess student learning strategies specific to a curriculum’s program theory. For example, at times, evaluators wanted to gauge the effectiveness of using problems different from those on typical standardized tests. In this case, problems were drawn from familiar circumstances but carefully designed to create significant cognitive challenges, and assess how well the informal strategies approach in CMP works in comparison to traditional instruction. The disadvantages of such an approach include the use of only a small number of items and the concerns for reliability in scoring. These studies seem to represent a method of creating hybrid research models that build on the detailed analyses possible using case studies, but still reporting on samples that provide comparative data. It possibly reflects the concerns of some mathematicians and mathematics educators that the effectiveness of materials needs to be evaluated relative to very specific, research-based issues on learning and that these are often inadequately measured by multiple-choice tests. However, a decision not to report total scores led to a trade-off in the reliability and representativeness of the reported data, which must be addressed to increase the objectivity of the reports.
Second, we coded whether outcome data were disaggregated in some way. Disaggregation involved reporting data on dimensions such as content strand, subtest, test item, ethnic group, performance level, SES, and gender. We found disaggregated results particularly helpful in understanding the findings of studies that found main effects, and also in examining patterns across studies. We report the results of the studies’ disaggregation by content strand in our reports of effects. We report the results of the studies’ disaggregation by subgroup in our discussions of generalizability.
Third, we coded whether a study used an outcome measure that the evaluator reported as being sensitive to a particular treatment—this is a subcategory of what was defined in our framework as “curricular validity of measures.” In such studies, the rationale was that readily available measures such as state-mandated tests, norm-referenced standardized tests, and
college entrance examinations do not measure some of the aims of the program under study. A frequently cited instance of this was that “off the shelf” instruments do not measure well students’ ability to apply their mathematical knowledge to problems embedded in complex settings. Thus, some studies constructed a collection of tasks that assessed this ability and collected data on it (Ben-Chaim et al., 1998; Huntley et al., 2000).
Finally, we recorded whether a study used multiple outcome measures. Some studies used a variety of achievement measures and other studies reported on achievement accompanied by measures such as subsequent course taking or various types of affective measures. For example, Carroll (2001, p. 47) reported results on a norm-referenced standardized achievement test as well as a collection of tasks developed in other studies.
A study by Huntley et al. (2000) illustrates how a variety of these techniques were combined in their outcome measures. They developed three assessments. The first emphasized contextualized problem solving based on items from the American Mathematical Association of Two-Year Colleges and others; the second assessment was on context-free symbolic manipulation and a third part requiring collaborative problem solving. To link these measures to the overall evaluation, they articulated an explicit model of cognition based on how one links an applied situation to mathematical activity through processes of formulation and interpretation. Their assessment strategy permitted them to investigate algebraic reasoning as an ability to use algebraic ideas and techniques to (1) mathematize quantitative problem situations, (2) use algebraic principles and procedures to solve equations, and (3) interpret results of reasoning and calculations.
In presenting their data comparing performance on Core-Plus and traditional curriculum, they presented both main effects and comparisons on subscales. Their design of outcome measures permitted them to examine differences in performance with and without context and to conclude with statements such as “This result illustrates that CPMP students perform better than control students when setting up models and solving algebraic problems presented in meaningful contexts while having access to calculators, but CPMP students do not perform as well on formal symbol-manipulation tasks without access to context cues or calculators” (p. 349). The authors go on to present data on the relationship between knowing how to plan or interpret solutions and knowing how to carry them out. The correlations between these variables were weak but significantly different (0.26 for control groups and 0.35 for Core-Plus). The advantage of using multiple measures carefully tied to program theory is that they can permit one to test fine content distinctions that are likely to be the level of adjustments necessary to fine tune and improve curricular programs.
Another interesting approach to the use of outcome measures is found in the UCSMP studies. In many of these studies, evaluators collected infor-
TABLE 5-2 Mean Percentage Correct on the Subject Tests
Treatment Group | Geometry—Standard | Geometry—UCSMP | Advanced Algebra—UCSMP |
UCSMP | 43.1, 44.7, 50.5 | 51.2, 54.5 | 56.1, 58.8, 56.1 |
Comparison | 42.7, 45.5, 51.5 | 36.6, 40.8 | 42.0, 50.1, 50.0 |
“43.1, 44.7, 50.5” means students were correct on 43.1 percent of the total items, 44.7 percent of the fair items for UCSMP, and 50.5 percent of the items that were taught in both treatments. Too few items to report data. SOURCES: Adapted from Thompson et al. (2001); Thompson et al. (2003). |
mation from teachers’ reports and chapter reviews as to whether topics for items on the posttests were taught, calling this an “opportunity to learn” measure. The authors reported results from three types of analyses: (1) total test scores, (2) fair test scores (scores reported by program but only on items on topics taught), and (3) conservative test scores (scores on common items taught in both). Table 5-2 reports on the variations across the multiple- choice test scores for the Geometry study (Thompson et al., 2003) on a standardized test, High School Subject Tests-Geometry Form B , and the UCSMP-constructed Geometry test, and for the Advanced Algebra Study on the UCSMP-constructed Advanced Algebra test (Thompson et al., 2001). The table shows the mean scores for UCSMP classes and comparison classes. In each cell, mean percentage correct is reported first by whole test, then by fair test, and then by conservative test.
The authors explicitly compare the items from the standard Geometry test with the items from the UCSMP test and indicate overlap and difference. They constructed their own test because, in their view, the standard test was not adequately balanced among skills, properties, and real-world uses. The UCSMP test included items on transformations, representations, and applications that were lacking in the national test. Only five items were taught by all teachers; hence in the case of the UCSMP geometry test, there is no report on a conservative test. In the Advanced Algebra evaluation, only a UCSMP-constructed test was viewed as appropriate to cover the treatment of the prior material and alignment to the goals of the new course. These data sets demonstrate the challenge of selecting appropriate outcome measures, the sensitivity of the results to those decisions, and the importance of full disclosure of decision-making processes in order to permit readers to assess the implications of the choices. The methodology utilized sought to ensure that the material in the course was covered adequately by treatment teachers while finding ways to make comparisons that reflected content coverage.
Only one study reported on its outcomes using embedded assessment items employed over the course of the year. In a study of Saxon and UCSMP, Peters (1992) (EX) studied the use of these materials with two classrooms taught by the same teacher. In this small study, he randomly assigned students to treatment groups and then measured their performance on four unit tests composed of items common to both curricula and their progress on the Orleans-Hanna Algebraic Prognosis Test.
Peters’ study showed no significant difference in placement scores between Saxon and UCSMP on the posttest, but did show differences on the embedded assessment. Figure 5-6 (Peters, 1992, p. 75) shows an interesting display of the differences on a “continuum” that shows both the direction and magnitude of the differences and provides a level of concept specificity missing in many reports. This figure and a display ( Figure 5-7 ) in a study by Senk (1991, p. 18) of students’ mean scores on Curriculum A versus Curriculum B with a 10 percent range of differences marked represent two excellent means to communicate the kinds of detailed content outcome information that promises to be informative to curriculum writers, publishers, and school decision makers. In Figure 5-7 , 16 items listed by number were taken from the Second International Mathematics Study. The Functions, Statistics, and Trigonometry sample averaged 41 percent correct on these items whereas the U.S. precalculus sample averaged 38 percent. As shown in the figure, differences of 10 percent or less fall inside the banded area and greater than 10 percent fall outside, producing a display that makes it easy for readers and designers to identify the relative curricular strengths and weaknesses of topics.
While we value detailed outcome measure information, we also recognize the importance of examining curricular impact on students’ standardized test performance. Many developers, but not all, are explicit in rejecting standardized tests as adequate measures of the outcomes of their programs, claiming that these tests focus on skills and manipulations, that they are overly reliant on multiple-choice questions, and that they are often poorly aligned to new content emphases such as probability and statistics, transformations, use of contextual problems and functions, and process skills, such as problem solving, representation, or use of calculators. However, national and state tests are being revised to include more content on these topics and to draw on more advanced reasoning. Furthermore, these high-stakes tests are of major importance in school systems, determining graduation, passing standards, school ratings, and so forth. For this reason, if a curricular program demonstrated positive impact on such measures, we referred to that in Chapter 3 as establishing “curricular alignment with systemic factors.” Adequate performance on these measures is of paramount importance to the survival of reform (to large groups of parents and
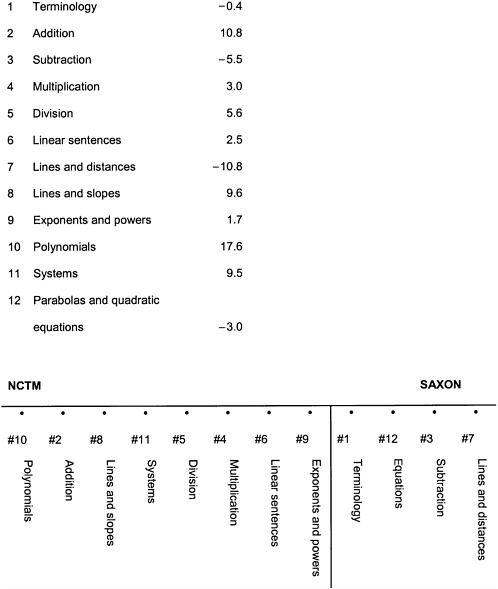
FIGURE 5-6 Continuum of criterion score averages for studied programs.
SOURCE: Peters (1992, p. 75).
school administrators). These examples demonstrate how careful attention to outcomes measures is an essential element of valid evaluation.
In Table 5-3 , we document the number of studies using a variety of types of outcome measures that we used to code the data, and also report on the types of tests used across the studies.
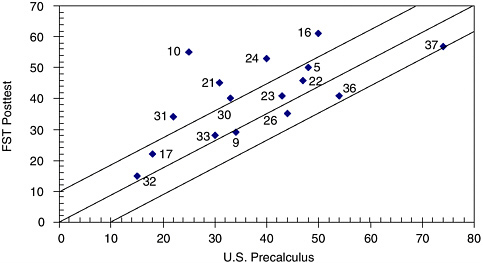
FIGURE 5-7 Achievement (percentage correct) on Second International Mathematics Study (SIMS) items by U.S. precalculus students and functions, statistics, and trigonometry (FST) students.
SOURCE: Re-created from Senk (1991, p. 18).
TABLE 5-3 Number of Studies Using a Variety of Outcome Measures by Program Type
| Total Test | Content Strands | Test Match to Program | Multiple Test | ||||
| Yes | No | Yes | No | Yes | No | Yes | No |
NSF | 43 | 3 | 28 | 18 | 26 | 20 | 21 | 25 |
Commercial | 8 | 1 | 4 | 5 | 2 | 7 | 2 | 7 |
UCSMP | 7 | 1 | 7 | 1 | 7 | 1 | 7 | 1 |
A Choice of Statistical Tests, Including Statistical Significance and Effect Size
In our first review of the studies, we coded what methods of statistical evaluation were used by different evaluators. Most common were t-tests; less frequently one found Analysis of Variance (ANOVA), Analysis of Co-
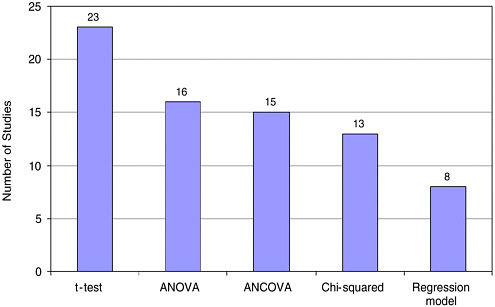
FIGURE 5-8 Statistical tests most frequently used.
variance (ANCOVA), and chi-square tests. In a few cases, results were reported using multiple regression or hierarchical linear modeling. Some used multiple tests; hence the total exceeds 63 ( Figure 5-8 ).
One of the difficult aspects of doing curriculum evaluations concerns using the appropriate unit both in terms of the unit to be randomly assigned in an experimental study and the unit to be used in statistical analysis in either an experimental or quasi-experimental study.
For our purposes, we made the decision that unless the study concerned an intact student population such as the freshman at a single university, where a student comparison was the correct unit, we believed that for statistical tests, the unit should be at least at the classroom level. Judgments were made for each study as to whether the appropriate unit was utilized. This question is an important one because statistical significance is related to sample size, and as a result, studies that inappropriately use the student as the unit of analysis could be concluding significant differences where they are not present. For example, if achievement differences between two curricula are tested in 16 classrooms with 400 students, it will always be easier to show significant differences using scores from those 400 students than using 16 classroom means.
Fifty-seven studies used students as the unit of analysis in at least one test of significance. Three of these were coded as correct because they involved whole populations. In all, 10 studies were coded as using the
TABLE 5-4 Performance on Applied Algebra Problems with Use of Calculators, Part 1
Treatment | n | M (0-100) | SD |
Control | 273 | 34.1 | 14.8 |
CPMP | 320 | 42.6 | 21.3 |
NOTE: t = -5.69, p < .001. All sites combined SOURCE: Huntley et al. (2000). Reprinted with permission. |
TABLE 5-5 Reanalysis of Algebra Performance Data
| Site Mean | Independent Samples Dependent | Difference Sample Difference | |
Site | Control | CPMP | ||
1 | 31.7 | 35.5 |
| 3.8 |
2 | 26.0 | 49.4 |
| 23.4 |
3 | 36.7 | 25.2 |
| -11.5 |
4 | 41.9 | 47.7 |
| 5.8 |
5 | 29.4 | 38.3 |
| 8.9 |
6 | 30.5 | 45.6 |
| 15.1 |
Average | 32.7 | 40.3 | 7.58 | 7.58 |
Standard deviation | 5.70 | 9.17 | 7.64 | 11.75 |
Standard error |
|
| 4.41 | 4.80 |
|
| t | 1.7 | 1.6 |
|
| p | 0.116 | 0.175 |
SOURCE: Huntley et al. (2000). |
correct unit of analysis; hence, 7 studies used teachers or classes, or schools. For some studies where multiple tests were conducted, a judgment was made as to whether the primary conclusions drawn treated the unit of analysis adequately. For example, Huntley et al. (2000) compared the performance of CPMP students with students in a traditional course on a measure of ability to formulate and use algebraic models to answer various questions about relationships among variables. The analysis used students as the unit of analysis and showed a significant difference, as shown in Table 5-4 .
To examine the robustness of this result, we reanalyzed the data using an independent sample t-test and a matched pairs t-test with class means as the unit of analysis in both tests ( Table 5-5 ). As can be seen from the analyses, in neither statistical test was the difference between groups found to be significantly different (p < .05), thus emphasizing the importance of using the correct unit in analyzing the data.
Reanalysis of student-level data using class means will not always result
TABLE 5-6 Mean Percentage Correct on Entire Multiple-Choice Posttest: Second Edition and Non-UCSMP
School | Pair | UCSMP Second Edition | |||
Code | ID | n | Mean | SD | OTL |
J | 18 | 18 | 60.8 | 9.0 | 100 |
J | 19 | 11 | 58.8 | 13.5 | 100 |
K | 20 | 22 | 63.8 | 13.0 | 94 |
K | 21 | 16 | 64.8 | 14.0 | 94 |
L | 22 | 19 | 57.6 | 16.9 | 92 |
L | 23 | 13 | 44.7 | 11.2 | 92 |
M | 24 | 29 | 58.4 | 12.7 | 92 |
M | 25 | 22 | 39.6 | 13.5 | 92 |
Overall |
| 150 | 56.1 | 15.4 |
|
NOTE: The mean is the mean percentage correct on a 36-item multiple-choice posttest. The OTL is the percentage of the items for which teachers reported their students had the opportunity to learn the needed content. Underline indicates statistically significant differences between the mean percentage correct for each pair. |
in a change in finding. Furthermore, using class means as the unit of analysis does not suggest that significant differences will not be found. For example, a study by Thompson et al. (2001) compared the performance of UCSMP students with the performance of students in a more traditional program across several measures of achievement. They found significant differences between UCSMP students and the non-UCSMP students on several measures. Table 5-6 shows results of an analysis of a multiple-choice algebraic posttest using class means as the unit of analysis. Significant differences were found in five of eight separate classroom comparisons, as shown in the table. They also found a significant difference using a matched-pairs t-test on class means.
The lesson to be learned from these reanalyses is that the choice of unit of analysis and the way the data are aggregated can impact study findings in important ways including the extent to which these findings can be generalized. Thus it is imperative that evaluators pay close attention to such considerations as the unit of analysis and the way data are aggregated in the design, implementation, and analysis of their studies.
Non-UCSMP | |||||||
n | Mean | SD | OTL | SE | t | df | p |
14 | 55.2 | 10.2 | 69 | 3.40 | 1.65 | 30 | 0.110 |
15 | 53.7 | 11.0 | 69 | 4.81 | 1.06 | 24 | 0.299 |
24 | 45.9 | 10.0 | 72 | 3.41 | 5.22 | 44 |
|
23 | 43.0 | 11.9 | 72 | 4.16 | 5.23 | 37 |
|
20 | 38.8 | 9.1 | 75 | 4.32 | 4.36 | 37 |
|
15 | 38.3 | 11.0 | 75 | 4.20 | 1.52 | 26 | 0.140 |
22 | 37.8 | 13.8 | 47 | 3.72 | 5.56 | 49 |
|
23 | 30.8 | 9.9 | 47 | 3.52 | 2.51 | 43 |
|
156 | 42.0 | 13.1 |
|
|
|
|
|
A matched-pairs t-test indicates that the differences between the two curricula are significant.
SOURCE: Thompson et al. (2001). Reprinted with permission. |
Second, effect size has become a relatively common and standard way of gauging the practical significance of the findings. Statistical significance only indicates whether the main-level differences between two curricula are large enough to not be due to chance, assuming they come from the same population. When statistical differences are found, the question remains as to whether such differences are large enough to consider. Because any innovation has its costs, the question becomes one of cost-effectiveness: Are the differences in student achievement large enough to warrant the costs of change? Quantifying the practical effect once statistical significance is established is one way to address this issue. There is a statistical literature for doing this, and for the purposes of this review, the committee simply noted whether these studies have estimated such an effect. However, the committee further noted that in conducting meta-analyses across these studies, effect size was likely to be of little value. These studies used an enormous variety of outcome measures, and even using effect size as a means to standardize units across studies is not sensible when the measures in each
study address such a variety of topics, forms of reasoning, content levels, and assessment strategies.
We note very few studies drew upon the advances in methodologies employed in modeling, which include causal modeling, hierarchical linear modeling (Bryk and Raudenbush, 1992; Bryk et al., 1993), and selection bias modeling (Heckman and Hotz, 1989). Although developing detailed specifications for these approaches is beyond the scope of this review, we wish to emphasize that these methodological advances should be considered within future evaluation designs.
Results and Limitations to Generalizability Resulting from Design Constraints
One also must consider what generalizations can be drawn from the results (Campbell and Stanley, 1966; Caporaso and Roos, 1973; and Boruch, 1997). Generalization is a matter of external validity in that it determines to what populations the study results are likely to apply. In designing an evaluation study, one must carefully consider, in the selection of units of analysis, how various characteristics of those units will affect the generalizability of the study. It is common for evaluators to conflate issues of representativeness for the purpose of generalizability (external validity) and comparativeness (the selection of or adjustment for comparative groups [internal validity]). Not all studies must be representative of the population served by mathematics curricula to be internally valid. But, to be generalizable beyond restricted communities, representativeness must be obtained by the random selection of the basic units. Clearly specifying such limitations to generalizability is critical. Furthermore, on the basis of equity considerations, one must be sure that if overall effectiveness is claimed, that the studies have been conducted and analyzed with reference of all relevant subgroups.
Thus, depending on the design of a study, its results may be limited in generalizability to other populations and circumstances. We identified four typical kinds of limitations on the generalizability of studies and coded them to determine, on the whole, how generalizable the results across studies might be.
First, there were studies whose designs were limited by the ability or performance level of the students in the samples. It was not unusual to find that when new curricula were implemented at the secondary level, schools kept in place systems of tracking that assigned the top students to traditional college-bound curriculum sequences. As a result, studies either used comparative groups who were matched demographically but less skilled than the population as a whole, in relation to prior learning, or their results compared samples of less well-prepared students to samples of students
with stronger preparations. Alternatively, some studies reported on the effects of curricula reform on gifted and talented students or on college-attending students. In these cases, the study results would also limit the generalizability of the results to similar populations. Reports using limited samples of students’ ability and prior performance levels were coded as a limitation to the generalizability of the study.
For example, Wasman (2000) conducted a study of one school (six teachers) and examined the students’ development of algebraic reasoning after one (n=100) and two years (n=73) in CMP. In this school, the top 25 percent of the students are counseled to take a more traditional algebra course, so her experimental sample, which was 61 percent white, 35 percent African American, 3 percent Asian, and 1 percent Hispanic, consisted of the lower 75 percent of the students. She reported on the student performance on the Iowa Algebraic Aptitude Test (IAAT) (1992), in the subcategories of interpreting information, translating symbols, finding relationships, and using symbols. Results for Forms 1 and 2 of the test, for the experimental and norm group, are shown in Table 5-7 for 8th graders.
In our coding of outcomes, this study was coded as showing no significant differences, although arguably its results demonstrate a positive set of
TABLE 5-7 Comparing Iowa Algebraic Aptitude Test (IAAT) Mean Scores of the Connected Mathematics Project Forms 1 and 2 to the Normative Group (8th Graders)
| Interpreting Information | Translating Symbols | Finding Relationships | Using Symbols | Total |
CMP: Form 1 | 9.35 | 8.22 | 9.90 | 8.65 | 36.12 |
7th (n=51) | (3.36) | (3.44) | (3.26) | (3.12) | (11.28) |
CMP: Form 1 | 9.76 | 8.56 | 9.41 | 8.27 | 36.00 |
8th (n=41) | (3.89) | (3.64) | (4.13) | (3.74) | (13.65) |
Norm: Form 1 | 10.03 | 9.55 | 9.14 | 8.87 | 37.59 |
(n=2,467) | (3.35) | (2.89) | (3.59) | (3.19) | (10.57) |
CMP: Form 2 | 9.41 | 7.82 | 9.29 | 7.65 | 34.16 |
7th (n=49) | (4.05) | (3.03) | (3.57) | (3.35) | (11.47) |
CMP: Form 2 | 11.28 | 8.66 | 10.94 | 9.81 | 40.69 |
8th (n=32) | (3.74) | (3.81) | (3.79) | (3.64) | (12.94) |
Norm: Form 2 | 10.63 | 8.58 | 8.67 | 9.19 | 37.07 |
(n=2,467) | (3.78) | (2.91) | (3.84) | (3.17) | (11.05) |
NOTE: Parentheses indicate standard deviation. SOURCE: Adapted from Wasman (2000). |
outcomes as the treatment group was weaker than the control group. Had the researcher used a prior achievement measure and a different statistical technique, significance might have been demonstrated, although potential teacher effects confound interpretations of results.
A second limitation to generalizability was when comparative studies resided entirely at curriculum pilot site locations, where such sites were developed as a means to conduct formative evaluations of the materials with close contact and advice from teachers. Typically, pilot sites have unusual levels of teacher support, whether it is in the form of daily technical support in the use of materials or technology or increased quantities of professional development. These sites are often selected for study because they have established cooperative agreements with the program developers and other sources of data, such as classroom observations, are already available. We coded whether the study was conducted at a pilot site to signal potential limitations in generalizability of the findings.
Third, studies were also coded as being of limited generalizability if they failed to disaggregate their data by socioeconomic class, race, gender, or some other potentially significant sources of restriction on the claims. We recorded the categories in which disaggregation occurred and compiled their frequency across the studies. Because of the need to open the pipeline to advanced study in mathematics by members of underrepresented groups, we were particularly concerned about gauging the extent to which evaluators factored such variables into their analysis of results and not just in terms of the selection of the sample.
Of the 46 included studies of NSF-supported curricula, 19 disaggregated their data by student subgroup. Nine of 17 studies of commercial materials disaggregated their data. Figure 5-9 shows the number of studies that disaggregated outcomes by race or ethnicity, SES, gender, LEP, special education status, or prior achievement. Studies using multiple categories of disaggregation were counted multiple times by program category.
The last category of restricted generalization occurred in studies of limited sample size. Although such studies may have provided more indepth observations of implementation and reports on professional development factors, the smaller numbers of classrooms and students in the study would limit the extent of generalization that could be drawn from it. Figure 5-10 shows the distribution of sizes of the samples in terms of numbers of students by study type.
Summary of Results by Student Achievement Among Program Types
We present the results of the studies as a means to further investigate their methodological implications. To this end, for each study, we counted across outcome measures the number of findings that were positive, nega-
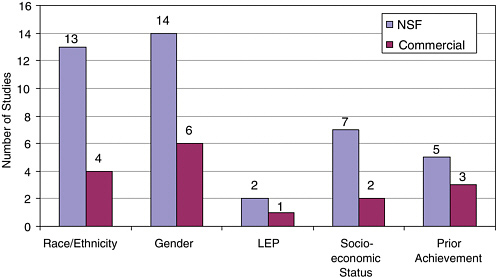
FIGURE 5-9 Disaggregation of subpopulations.
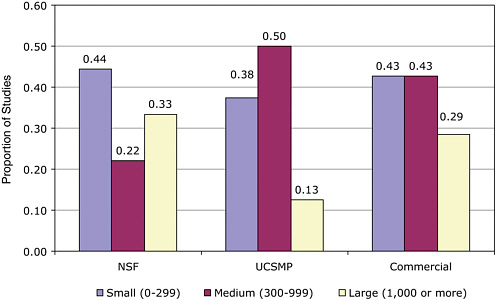
FIGURE 5-10 Proportion of studies by sample size and program.
tive, or indeterminate (no significant difference) and then calculated the proportion of each. We represented the calculation of each study as a triplet (a, b, c) where a indicates the proportion of the results that were positive and statistically significantly stronger than the comparison program, b indicates the proportion that were negative and statistically significantly weaker than the comparison program, and c indicates the proportion that showed no significant difference between the treatment and the comparative group. For studies with a single outcome measure, without disaggregation by content strand, the triplet is always composed of two zeros and a single one. For studies with multiple measures or disaggregation by content strand, the triplet is typically a set of three decimal values that sum to one. For example, a study with one outcome measure in favor of the experimental treatment would be coded (1, 0, 0), while one with multiple measures and mixed results more strongly in favor of the comparative curriculum might be listed as (.20, .50, .30). This triplet would mean that for 20 percent of the comparisons examined, the evaluators reported statistically significant positive results, for 50 percent of the comparisons the results were statistically significant in favor of the comparison group, and for 30 percent of the comparisons no significant difference were found. Overall, the mean score on these distributions was (.54, .07, .40), indicating that across all the studies, 54 percent of the comparisons favored the treatment, 7 percent favored the comparison group, and 40 percent showed no significant difference. Table 5-8 shows the comparison by curricular program types. We present the results by individual program types, because each program type relies on a similar program theory and hence could lead to patterns of results that would be lost in combining the data. If the studies of commercial materials are all grouped together to include UCSMP, their pattern of results is (.38, .11, .51). Again we emphasize that due to our call for increased methodological rigor and the use of multiple methods, this result is not sufficient to establish the curricular effectiveness of these programs as a whole with adequate certainty.
We caution readers that these results are summaries of the results presented across a set of evaluations that meet only the standard of at least
TABLE 5-8 Comparison by Curricular Program Types
Proportion of Results That Are: | NSF-Supported n=46 | UCSMP n=8 | Commercially Generated n=9 |
In favor of treatment | .591 | .491 | .285 |
In favor of comparison | .055 | .087 | .130 |
Show no significant difference | .354 | .422 | .585 |
minimally methodologically adequate . Calculations of statistical significance of each program’s results were reported by the evaluators; we have made no adjustments for weaknesses in the evaluations such as inappropriate use of units of analysis in calculating statistical significance. Evaluations that consistently used the correct unit of analysis, such as UCSMP, could have fewer reports of significant results as a consequence. Furthermore, these results are not weighted by study size. Within any study, the results pay no attention to comparative effect size or to the established credibility of an outcome measure. Similarly, these results do not take into account differences in the populations sampled, an important consideration in generalizing the results. For example, using the same set of studies as an example, UCSMP studies used volunteer samples who responded to advertisements in their newsletters, resulting in samples with disproportionately Caucasian subjects from wealthier schools compared to national samples. As a result, we would suggest that these results are useful only as baseline data for future evaluation efforts. Our purpose in calculating these results is to permit us to create filters from the critical decision points and test how the results change as one applies more rigorous standards.
Given that none of the studies adequately addressed all of the critical criteria, we do not offer these results as definitive, only suggestive—a hypothesis for further study. In effect, given the limitations of time and support, and the urgency of providing advice related to policy, we offer this filtering approach as an informal meta-analytic technique sufficient to permit us to address our primary task, namely, evaluating the quality of the evaluation studies.
This approach reflects the committee’s view that to deeply understand and improve methodology, it is necessary to scrutinize the results and to determine what inferences they provide about the conduct of future evaluations. Analogous to debates on consequential validity in testing, we argue that to strengthen methodology, one must consider what current methodologies are able (or not able) to produce across an entire series of studies. The remainder of the chapter is focused on considering in detail what claims are made by these studies, and how robust those claims are when subjected to challenge by alternative hypothesis, filtering by tests of increasing rigor, and examining results and patterns across the studies.
Alternative Hypotheses on Effectiveness
In the spirit of scientific rigor, the committee sought to consider rival hypotheses that could explain the data. Given the weaknesses in the designs generally, often these alternative hypotheses cannot be dismissed. However, we believed that only after examining the configuration of results and
alternative hypotheses can the next generation of evaluations be better informed and better designed. We began by generating alternative hypotheses to explain the positive directionality of the results in favor of experimental groups. Alternative hypotheses included the following:
The teachers in the experimental groups tended to be self-selecting early adopters, and thus able to achieve effects not likely in regular populations.
Changes in student outcomes reflect the effects of professional development instruction, or level of classroom support (in pilot sites), and thus inflate the predictions of effectiveness of curricular programs.
Hawthorne effect (Franke and Kaul, 1978) occurs when treatments are compared to everyday practices, due to motivational factors that influence experimental participants.
The consistent difference is due to the coherence and consistency of a single curricular program when compared to multiple programs.
The significance level is only achieved by the use of the wrong unit of analysis to test for significance.
Supplemental materials or new teaching techniques produce the results and not the experimental curricula.
Significant results reflect inadequate outcome measures that focus on a restricted set of activities.
The results are due to evaluator bias because too few evaluators are independent of the program developers.
At the same time, one could argue that the results actually underestimate the performance of these materials and are conservative measures, and their alternative hypotheses also deserve consideration:
Many standardized tests are not sensitive to these curricular approaches, and by eliminating studies focusing on affect, we eliminated a key indicator of the appeal of these curricula to students.
Poor implementation or increased demands on teachers’ knowledge dampens the effects.
Often in the experimental treatment, top-performing students are missing as they are advised to take traditional sequences, rendering the samples unequal.
Materials are not well aligned with universities and colleges because tests for placement and success in early courses focus extensively on algebraic manipulation.
Program implementation has been undercut by negative publicity and the fears of parents concerning change.
There are also a number of possible hypotheses that may be affecting the results in either direction, and we list a few of these:
Examining the role of the teacher in curricular decision making is an important element in effective implementation, and design mandates of evaluation design make this impossible (and the positives and negatives or single- versus dual-track curriculum as in Lundin, 2001).
Local tests that are sensitive to the curricular effects typically are not mandatory and hence may lead to unpredictable performance by students.
Different types and extent of professional development may affect outcomes differentially.
Persistence or attrition may affect the mean scores and are often not considered in the comparative analyses.
One could also generate reasons why the curricular programs produced results showing no significance when one program or the other is actually more effective. This could include high degrees of variability in the results, samples that used the correct unit of analysis but did not obtain consistent participation across enough cases, implementation that did not show enough fidelity to the measures, or outcome measures insensitive to the results. Again, subsequent designs should be better informed by these findings to improve the likelihood that they will produce less ambiguous results and replication of studies could also give more confidence in the findings.
It is beyond the scope of this report to consider each of these alternative hypotheses separately and to seek confirmation or refutation of them. However, in the next section, we describe a set of analyses carried out by the committee that permits us to examine and consider the impact of various critical evaluation design decisions on the patterns of outcomes across sets of studies. A number of analyses shed some light on various alternative hypotheses and may inform the conduct of future evaluations.
Filtering Studies by Critical Decision Points to Increase Rigor
In examining the comparative studies, we identified seven critical decision points that we believed would directly affect the rigor and efficacy of the study design. These decision points were used to create a set of 16 filters. These are listed as the following questions:
Was there a report on comparability relative to SES?
Was there a report on comparability of samples relative to prior knowledge?
Was there a report on treatment fidelity?
Was professional development reported on?
Was the comparative curriculum specified?
Was there any attempt to report on teacher effects?
Was a total test score reported?
Was total test score(s) disaggregated by content strand?
Did the outcome measures match the curriculum?
Were multiple tests used?
Was the appropriate unit of analysis used in their statistical tests?
Did they estimate effect size for the study?
Was the generalizability of their findings limited by use of a restricted range of ability levels?
Was the generalizability of their findings limited by use of pilot sites for their study?
Was the generalizability of their findings limited by not disaggregating their results by subgroup?
Was the generalizability of their findings limited by use of small sample size?
The studies were coded to indicate if they reported having addressed these considerations. In some cases, the decision points were coded dichotomously as present or absent in the studies, and in other cases, the decision points were coded trichotomously, as description presented, absent, or statistically adjusted for in the results. For example, a study may or may not report on the comparability of the samples in terms of race, ethnicity, or socioeconomic status. If a report on SES was given, the study was coded as “present” on this decision; if a report was missing, it was coded as “absent”; and if SES status or ethnicity was used in the analysis to actually adjust outcomes, it was coded as “adjusted for.” For each coding, the table that follows reports the number of studies that met that condition, and then reports on the mean percentage of statistically significant results, and results showing no significant difference for that set of studies. A significance test is run to see if the application of the filter produces changes in the probability that are significantly different. 5
In the cases in which studies are coded into three distinct categories—present, absent, and adjusted for—a second set of filters is applied. First, the studies coded as present or adjusted for are combined and compared to those coded as absent; this is what we refer to as a weak test of the rigor of the study. Second, the studies coded as present or absent are combined and compared to those coded as adjusted for. This is what we refer to as a strong test. For dichotomous codings, there can be as few as three compari-
| The significance test used was a chi-square not corrected for discontinuity. |
sons, and for trichotomous codings, there can be nine comparisons with accompanying tests of significance. Trichotomous codes were used for adjustments for SES and prior knowledge, examining treatment fidelity, professional development, teacher effects, and reports on effect sizes. All others were dichotomous.
NSF Studies and the Filters
For example, there were 11 studies of NSF-supported curricula that simply reported on the issues of SES in creating equivalent samples for comparison, and for this subset the mean probabilities of getting positive, negative, or results showing no significant difference were (.47, .10, .43). If no report of SES was supplied (n= 21), those probabilities become (.57, .07, .37), indicating an increase in positive results and a decrease in results showing no significant difference. When an adjustment is made in outcomes based on differences in SES (n=14), the probabilities change to (.72, .00, .28), showing a higher likelihood of positive outcomes. The probabilities that result from filtering should always be compared back to the overall results of (.59, .06, .35) (see Table 5-8 ) so as to permit one to judge the effects of more rigorous methodological constraints. This suggests that a simple report on SES without adjustment is least likely to produce positive outcomes; that is, no report produces the outcomes next most likely to be positive and studies that adjusted for SES tend to have a higher proportion of their comparisons producing positive results.
The second method of applying the filter (the weak test for rigor) for the treatment of the adjustment of SES groups compares the probabilities when a report is either given or adjusted for compared to when no report is offered. The combined percentage of a positive outcome of a study in which SES is reported or adjusted for is (.61, .05, .34), while the percentage for no report remains as reported previously at (.57, .07, .37). A final filter compares the probabilities of the studies in which SES is adjusted for with those that either report it only or do not report it at all. Here we compare the percentage of (.72, .00, .28) to (.53, .08, .37) in what we call a strong test. In each case we compared the probability produced by the whole group to those of the filtered studies and conducted a test of the differences to determine if they were significant. These differences were not significant. These findings indicate that to date, with this set of studies, there is no statistically significant difference in results when one reports or adjusts for changes in SES. It appears that by adjusting for SES, one sees increases in the positive results, and this result deserves a closer examination for its implications should it prove to hold up over larger sets of studies.
We ran tests that report the impact of the filters on the number of studies, the percentage of studies, and the effects described as probabilities
for each of the three study categories, NSF-supported and commercially generated with UCSMP included. We claim that when a pattern of probabilities of results does not change after filtering, one can have more confidence in that pattern. When the pattern of results changes, there is a need for an explanatory hypothesis, and that hypothesis can shed light on experimental design. We propose that this “filtering process” constitutes a test of the robustness of the outcome measures subjected to increasing degrees of rigor by using filtering.
Results of Filtering on Evaluations of NSF-Supported Curricula
For the NSF-supported curricular programs, out of 15 filters, 5 produced a probability that differed significantly at the p<.1 level. The five filters were for treatment fidelity, specification of control group, choosing the appropriate statistical unit, generalizability for ability, and generalizability based on disaggregation by subgroup. For each filter, there were from three to nine comparisons, as we examined how the probabilities of outcomes change as tests were more stringent and across the categories of positive results, negative results, and results with no significant differences. Out of a total of 72 possible tests, only 11 produced a probability that differed significantly at the p < .1 level. With 85 percent of the comparisons showing no significant difference after filtering, we suggest the results of the studies were relatively robust in relation to these tests. At the same time, when rigor is increased for the five filters just listed, the results become generally more ambiguous and signal the need for further research with more careful designs.
Studies of Commercial Materials and the Filters
To ensure enough studies to conduct the analysis (n=17), our filtering analysis of the commercially generated studies included UCSMP (n=8). In this case, there were six filters that produced a probability that differed significantly at the p < .1 level. These were treatment fidelity, disaggregation by content, use of multiple tests, use of effect size, generalizability by ability, and generalizability by sample size. In this case, because there were no studies in some possible categories, there were a total of 57 comparisons, and 9 displayed significant differences in the probabilities after filtering at the p < .1 level. With 84 percent of the comparisons showing no significant difference after filtering, we suggest the results of the studies were relatively robust in relation to these tests. Table 5-9 shows the cases in which significant differences were recorded.
Impact of Treatment Fidelity on Probabilities
A few of these differences are worthy of comment. In the cases of both the NSF-supported and commercially generated curricula evaluation studies, studies that reported treatment fidelity differed significantly from those that did not. In the case of the studies of NSF-supported curricula, it appeared that a report or adjustment on treatment fidelity led to proportions with less positive effects and more results showing no significant differences. We hypothesize that this is partly because larger studies often do not examine actual classroom practices, but can obtain significance more easily due to large sample sizes.
In the studies of commercial materials, the presence or absence of measures of treatment fidelity worked differently. Studies reporting on or adjusting for treatment fidelity tended to have significantly higher probabilities in favor of experimental treatment, less positive effects in fewer of the comparative treatments, and more likelihood of results with no significant differences. We hypothesize, and confirm with a separate analysis, that this is because UCSMP frequently reported on treatment fidelity in their designs while study of Saxon typically did not, and the change represents the preponderance of these different curricular treatments in the studies of commercially generated materials.
Impact of Identification of Curricular Program on Probabilities
The significant differences reported under specificity of curricular comparison also merit discussion for studies of NSF-supported curricula. When the comparison group is not specified, a higher percentage of mean scores in favor of the experimental curricula is reported. In the studies of commercial materials, a failure to name specific curricular comparisons also produced a higher percentage of positive outcomes for the treatment, but the difference was not statistically significant. This suggests the possibility that when a specified curriculum is compared to an unspecified curriculum, reports of impact may be inflated. This finding may suggest that in studies of effectiveness, specifying comparative treatments would provide more rigorous tests of experimental approaches.
When studies of commercial materials disaggregate their results of content strands or use multiple measures, their reports of positive outcomes increase, the negative outcomes decrease, and in one case, the results show no significant differences. Percentage of significant difference was only recorded in one comparison within each one of these filters.
TABLE 5-9 Cases of Significant Differences
Test | Type of Comparison | Category Code | N= | Probabilities Before Filter | p= |
| |||||
Treatment fidelity | Simple compare | Specified | 21 | .51, .02, .47* | *p =.049 |
| Not specified |
| 24 | .68, .09, .23* |
|
| Adjusted for |
| 1 | .25, .00, .75 |
|
Treatment fidelity | Strong test | Adjusted for | 22 | .49*, .02, .49** | *p=.098 |
| Reported or not specified |
| 24 | .68*, .09, .23** | **p=.019 |
Control group specified | Simple compare | Specified | 8 | .33*, .00, .66** | *p=.033 |
|
| Not specified | 38 | .65*, .07, .29** | **p=.008 |
Appropriate unit of analysis | Simple compare | Correct | 5 | .30*, .40**, .30 | *p=.069 |
|
| Incorrect | 41 | .63*, .01**, .36 | **p=.000 |
Generalizability by ability | Simple compare | Limited | 5 | .22*, .41**, .37 | *p=.019 |
|
| Not limited | 41 | .64*, .01**, .35 | **p=.000 |
Generalizability by disaggregated subgroup | Simple compare | Limited | 28 | .48*, .09, .43** | *p=.013 |
| Not limited | 18 | .76*, .00, .24** | **p=.085 |
| |||||
Treatment fidelity | Simple compare | Reported | 7 | .53, .37*, .20 | *p=.032 |
|
| Not specified | 9 | .26, .67*, .11 |
|
|
| Adjusted for | 1 | .45, .00*, .55 |
|
Treatment fidelity | Weak test | Adjusted for or | 8 | .52, .33, .25* | *p=.087 |
|
| Reported versus | 9 | .26, .67, .11* |
|
|
| Not specified |
|
|
|
Outcomes disaggregated by content strand | Simple compare | Reported | 11 | .50, .37, .22* | *p=.052 |
| Not reported | 6 | .17, .77, .10* |
| |
Outcomes using multiple tests | Simple compare | Yes | 9 | .55*, .35, .19 | *p=.076 |
|
| No | 8 | .20*, .68, .20 |
|
Effect size reported | Simple compare | Yes | 3 | .72, .05, .29* | *p=.029 |
|
| No | 14 | .31, .61, .16* |
|
Generalization by ability | Simple compare | Limited | 4 | .23, .41*, .32 | *p=.004 |
|
| Not limited | 14 | .42, .53, .09 |
|
Generalization by sample size | Simple compare | Limited | 6 | .57, .23, .27* | *p=.036 |
|
| Not limited | 11 | .28, .66, .10* |
|
NOTE: In the comparisons shown, only the comparisons marked by an asterisk showed significant differences at p<.1. Probabilitie s are estimated for each significant difference. |
Impact of Units of Analysis on Probabilities 6
For the evaluations of the NSF-supported materials, a significant difference was reported on the outcomes for the studies that used the correct unit of analysis compared to those that did not. The percentage for those with the correct unit were (.30, .40, .30) compared to (.63, .01, .36) for those that used the incorrect result. These results suggest that our prediction that using the correct unit of analysis would decrease the percentage of positive outcomes is likely to be correct. It also suggests that the most serious threat to the apparent conclusions of these studies comes from selecting an incorrect unit of analysis. It causes a decrease in favorable results, making the results more ambiguous, but never reverses the direction of the effect. This is a concern that merits major attention in the conduct of further studies.
For the commercially generated studies, most of the ones coded with the correct unit of analysis were UCSMP studies. Because of the small number of studies involved, we could not break out from the overall filtering of studies of commercial materials, but report this issue to assist readers in interpreting the relative patterns of results.
Impact of Generalizability on Probabilities
Both types of studies yielded significant differences for some of the comparisons coded as restrictions to generalizability. Investigating these is important in order to understand the effects of these curricular programs on different subpopulations of students. In the case of the studies of commercially generated materials, significantly different results occurred in the categories of ability and sample size. In the studies of NSF-supported materials, the significant differences occurred in ability and disaggregation by subgroups.
In relation to generalizability, the studies of NSF-supported curricula reported significantly more positive results in favor of the treatment when they included all students. Because studies coded as “limited by ability” were restricted either by focusing only on higher achieving students or on lower achieving students, we sorted these two groups. For higher performing students (n=3), the probabilities of effects were (.11, .67, .22). For lower
| It should be noted that of the five studies in which the correct unit of analysis was used, two of these were population studies of freshmen entering college, and these reported few results in favor of the experimental treatments. However, the high proportion of these studies involving college students may skew this particular result relative to the preponderance of other studies involving K-12 students. |
performing students (n=2), the probabilities were (.39, .025, .59). The first two comparisons are significantly different at p < .05. These findings are based on only a total of five studies, but they suggest that these programs may be serving the weaker ability students more effectively than the stronger ability students, serving both less well than they serve whole heterogeneous groups. For the studies of commercial materials, there were only three studies that were restricted to limited populations. The results for those three studies were (.23, .41, .32) and for all students (n=14) were (.42, .53, .09). These studies were significantly different at p = .004. All three studies included UCSMP and one also included Saxon and was limited by serving primarily high-performing students. This means both categories of programs are showing weaker results when used with high-ability students.
Finally, the studies on NSF-supported materials were disaggregated by subgroups for 28 studies. A complete analysis of this set follows, but the studies that did not report results disaggregated by subgroup generated probabilities of results of (.48, .09, .43) whereas those that did disaggregate their results reported (.76, 0, .24). These gains in positive effects came from significant losses in reporting no significant differences. Studies of commercial materials also reported a small decrease in likelihood of negative effects for the comparison program when disaggregation by subgroup is reported offset by increases in positive results and results with no significant differences, although these comparisons were not significantly different. A further analysis of this topic follows.
Overall, these results suggest that increased rigor seems to lead in general to less strong outcomes, but never reports of completely contrary results. These results also suggest that in recommending design considerations to evaluators, there should be careful attention to having evaluators include measures of treatment fidelity, considering the impact on all students as well as one particular subgroup; using the correct unit of analysis; and using multiple tests that are also disaggregated by content strand.
Further Analyses
We conducted four further analyses: (1) an analysis of the outcome probabilities by test type; (2) content strands analysis; (3) equity analysis; and (4) an analysis of the interactions of content and equity by grade band. Careful attention to the issues of content strand, equity, and interaction is essential for the advancement of curricular evaluation. Content strand analysis provides the detail that is often lost by reporting overall scores; equity analysis can provide essential information on what subgroups are adequately served by the innovations, and analysis by content and grade level can shed light on the controversies that evolve over time.
Analysis by Test Type
Different studies used varied combinations of outcome measures. Because of the importance of outcome measures on test results, we chose to examine whether the probabilities for the studies changed significantly across different types of outcome measures (national test, local test). The most frequent use of tests across all studies was a combination of national and local tests (n=18 studies), a local test (n=16), and national tests (n=17). Other uses of test combinations were used by three studies or less. The percentages of various outcomes by test type in comparison to all studies are described in Table 5-10 .
These data ( Table 5-11 ) suggest that national tests tend to produce less positive results, and with the resulting gains falling into results showing no significant differences, suggesting that national tests demonstrate less curricular sensitivity and specificity.
TABLE 5-10 Percentage of Outcomes by Test Type
Test Type | National/Local | Local Only | National Only | All Studies |
All studies | (.48, .18, .34) n=18 | (.63, .03, .34) n=16 | (.31, .05, .64) n= 3 | (.54, .07, .40) n=63 |
NOTE: The first set of numbers in the parenthesis represent the percentage of outcomes that are positive, the second set of numbers in the parenthesis represent the percentage of outcomes that are negative, and the third set of numbers represent the percentage of outcomes that are nonsignificant. |
TABLE 5-11 Percentage of Outcomes by Test Type and Program Type
Test Type | National/Local | Local Only | National Only | All Studies |
NSF effects | (.52, .15, .34) n=14 | (.57, .03, .39) n=14 | (.44, .00, .56) n=4 | (.59, .06, .35) n=46 |
UCSMP effects | (.41, .18, .41) n=3 | *** | *** | (.49, .09, .42) n=8 |
Commercial effects | ** | ** | (.29, .08, .63) n=8 | (.29, .13, .59) n=9 |
NOTE: The first set of numbers in the parenthesis represent the percentage of outcomes that are positive, the second set of numbers represent the percentage of outcomes that are negative, and the third set of numbers represent the percentage of outcomes that are nonsignificant. |
TABLE 5-12 Number of Studies That Disaggregated by Content Strand
Program Type | Elementary | Middle | High School | Total |
NSF-supported | 14 | 6 | 9 | 29 |
Commercially generated | 0 | 4 | 5 | 9 |
Content Strand
Curricular effectiveness is not an all-or-nothing proposition. A curriculum may be effective in some topics and less effective in others. For this reason, it is useful for evaluators to include an analysis of curricular strands and to report on the performance of students on those strands. To examine this issue, we conducted an analysis of the studies that reported their results by content strand. Thirty-eight studies did this; the breakdown is shown in Table 5-12 by type of curricular program and grade band.
To examine the evaluations of these content strands, we began by listing all of the content strands reported across studies as well as the frequency of report by the number of studies at each grade band. These results are shown in Figure 5-11 , which is broken down by content strand, grade level, and program type.
Although there are numerous content strands, some of them were reported on infrequently. To allow the analysis to focus on the key results from these studies, we separated out the most frequently reported on strands, which we call the “major content strands.” We defined these as strands that were examined in at least 10 percent of the studies. The major content strands are marked with an asterisk in the Figure 5-11 . When we conduct analyses across curricular program types or grade levels, we use these to facilitate comparisons.
A second phase of our analysis was to examine the performance of students by content strand in the treatment group in comparison to the control groups. Our analysis was conducted across the major content strands at the level of NSF-supported versus commercially generated, initially by all studies and then by grade band. It appeared that such analysis permitted some patterns to emerge that might prove helpful to future evaluators in considering the overall effectiveness of each approach. To do this, we then coded the number of times any particular strand was measured across all studies that disaggregated by content strand. Then, we coded the proportion of times that this strand was reported as favoring the experimental treatment, favoring the comparative curricula, or showing no significant difference. These data are presented across the major content strands for the NSF-supported curricula ( Figure 5-12 ) and the commercially generated curricula, ( Figure 5-13 ) (except in the case of the elemen-
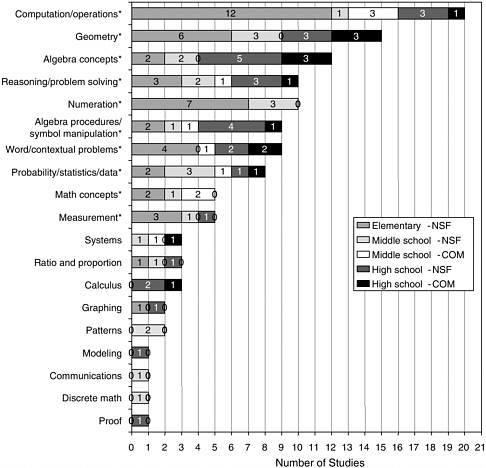
FIGURE 5-11 Study counts for all content strands.
tary curricula where no data were available) in the forms of percentages, with the frequencies listed in the bars.
The presentation of results by strands must be accompanied by the same restrictions as stated previously. These results are based on studies identified as at least minimally methodologically adequate. The quality of the outcome measures in measuring the content strands has not been examined. Their results are coded in relation to the comparison group in the study and are indicated as statistically in favor of the program, as in favor of the comparative program, or as showing no significant differences. The results are combined across studies with no weighting by study size. Their results should be viewed as a means for the identification of topics for potential future study. It is completely possible that a refinement of methodologies may affect the future patterns of results, so the results are to be viewed as tentative and suggestive.
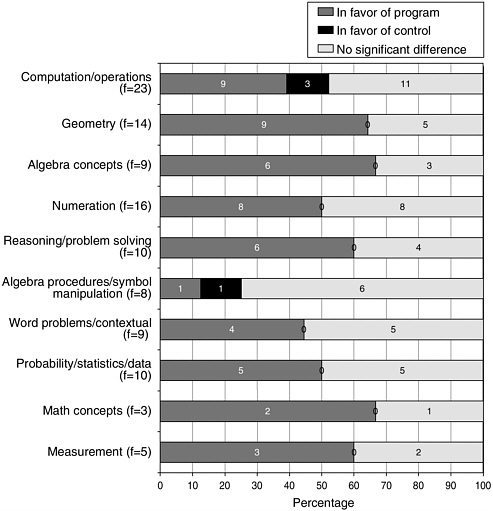
FIGURE 5-12 Major content strand result: All NSF (n=27).
According to these tentative results, future evaluations should examine whether the NSF-supported programs produce sufficient competency among students in the areas of algebraic manipulation and computation. In computation, approximately 40 percent of the results were in favor of the treatment group, no significant differences were reported in approximately 50 percent of the results, and results in favor of the comparison were revealed 10 percent of the time. Interpreting that final proportion of no significant difference is essential. Some would argue that because computation has not been emphasized, findings of no significant differences are acceptable. Others would suggest that such findings indicate weakness, because the development of the materials and accompanying professional development yielded no significant difference in key areas.
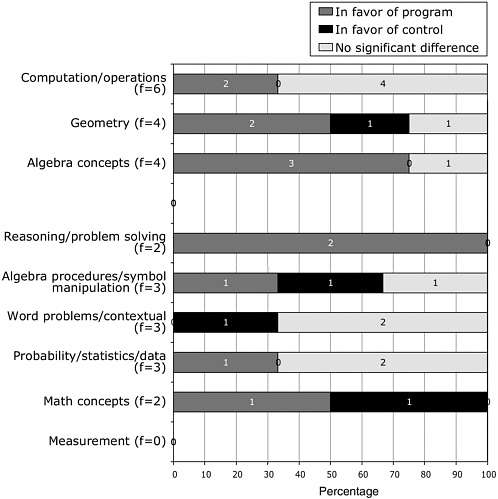
FIGURE 5-13 Major content strand result: All commercial (n=8).
From Figure 5-13 of findings from studies of commercially generated curricula, it appears that mixed results are commonly reported. Thus, in evaluations of commercial materials, lack of significant differences in computations/operations, word problems, and probability and statistics suggest that careful attention should be given to measuring these outcomes in future evaluations.
Overall, the grade band results for the NSF-supported programs—while consistent with the aggregated results—provide more detail. At the elementary level, evaluations of NSF-supported curricula (n=12) report better performance in mathematics concepts, geometry, and reasoning and problem solving, and some weaknesses in computation. No content strand analysis for commercially generated materials was possible. Evaluations
(n=6) at middle grades of NSF-supported curricula showed strength in measurement, geometry, and probability and statistics and some weaknesses in computation. In the studies of commercial materials, evaluations (n=4) reported favorable results in reasoning and problem solving and some unfavorable results in algebraic procedures, contextual problems, and mathematics concepts. Finally, at the high school level, the evaluations (n=9) by content strand for the NSF-supported curricula showed strong favorable results in algebra concepts, reasoning/problem solving, word problems, probability and statistics, and measurement. Results in favor of the control were reported in 25 percent of the algebra procedures and 33 percent of computation measures.
For the studies of commercial materials (n=4), only the geometry results favor the control group 25 percent of the time, with 50 percent having favorable results. Algebra concepts, reasoning, and probability and statistics also produced favorable results.
Equity Analysis of Comparative Studies
When the goal of providing a standards-based curriculum to all students was proposed, most people could recognize its merits: the replacement of dull, repetitive, largely dead-end courses with courses that would lead all students to be able, if desired and earned, to pursue careers in mathematics-reliant fields. It was clear that the NSF-supported projects, a stated goal of which was to provide standards-based courses to all students, called for curricula that would address the problem of too few students persisting in the study of mathematics. For example, as stated in the NSF Request for Proposals (RFP):
Rather than prematurely tracking students by curricular objectives, secondary school mathematics should provide for all students a common core of mainstream mathematics differentiated instructionally by level of abstraction and formalism, depth of treatment and pace (National Science Foundation, 1991, p. 1). In the elementary level solicitation, a similar statement on causes for all students was made (National Science Foundation, 1988, pp. 4-5).
Some, but not enough attention has been paid to the education of students who fall below the average of the class. On the other hand, because the above average students sometimes do not receive a demanding education, it may be incorrectly assumed they are easy to teach (National Science Foundation, 1989, p. 2).
Likewise, with increasing numbers of students in urban schools, and increased demographic diversity, the challenges of equity are equally significant for commercial publishers, who feel increasing pressures to demonstrate the effectiveness of their products in various contexts.
The problem was clearly identified: poorer performance by certain subgroups of students (minorities—non-Asian, LEP students, sometimes females) and a resulting lack of representation of such groups in mathematics-reliant fields. In addition, a secondary problem was acknowledged: Highly talented American students were not being provided adequate challenge and stimulation in comparison with their international counterparts. We relied on the concept of equity in examining the evaluation. Equity was contrasted to equality, where one assumed all students should be treated exactly the same (Secada et al., 1995). Equity was defined as providing opportunities and eliminating barriers so that the membership in a subgroup does not subject one to undue and systematically diminished possibility of success in pursuing mathematical study. Appropriate treatment therefore varies according to the needs of and obstacles facing any subgroup.
Applying the principles of equity to evaluate the progress of curricular programs is a conceptually thorny challenge. What is challenging is how to evaluate curricular programs on their progress toward equity in meeting the needs of a diverse student body. Consider how the following questions provide one with a variety of perspectives on the effectiveness of curricular reform regarding equity:
Does one expect all students to improve performance, thus raising the bar, but possibly not to decrease the gap between traditionally well-served and under-served students?
Does one focus on reducing the gap and devote less attention to overall gains, thus closing the gap but possibly not raising the bar?
Or, does one seek evidence that progress is made on both challenges—seeking progress for all students and arguably faster progress for those most at risk?
Evaluating each of the first two questions independently seems relatively straightforward. When one opts for a combination of these two, the potential for tensions between the two becomes more evident. For example, how can one differentiate between the case in which the gap is closed because talented students are being underchallenged from the case in which the gap is closed because the low-performing students improved their progress at an increased rate? Many believe that nearly all mathematics curricula in this country are insufficiently challenging and rigorous. Therefore achieving modest gains across all ability levels with evidence of accelerated progress by at-risk students may still be criticized for failure to stimulate the top performing student group adequately. Evaluating curricula with regard to this aspect therefore requires judgment and careful methodological attention.
Depending on one’s view of equity, different implications for the collection of data follow. These considerations made examination of the quality of the evaluations as they treated questions of equity challenging for the committee members. Hence we spell out our assumptions as precisely as possible:
Evaluation studies should include representative samples of student demographics, which may require particular attention to the inclusion of underrepresented minority students from lower socioeconomic groups, females, and special needs populations (LEP, learning disabled, gifted and talented students) in the samples. This may require one to solicit participation by particular schools or districts, rather than to follow the patterns of commercial implementation, which may lead to an unrepresentative sample in aggregate.
Analysis of results should always consider the impact of the program on the entire spectrum of the sample to determine whether the overall gains are distributed fairly among differing student groups, and not achieved as improvements in the mean(s) of an identifiable subpopulation(s) alone.
Analysis should examine whether any group of students is systematically less well served by curricular implementation, causing losses or weakening the rate of gains. For example, this could occur if one neglected the continued development of programs for gifted and talented students in mathematics in order to implement programs focused on improving access for underserved youth, or if one improved programs solely for one group of language learners, ignoring the needs of others, or if one’s study systematically failed to report high attrition affecting rates of participation of success or failure.
Analyses should examine whether gaps in scores between significantly disadvantaged or underperforming subgroups and advantaged subgroups are decreasing both in relation to eliminating the development of gaps in the first place and in relation to accelerating improvement for underserved youth relative to their advantaged peers at the upper grades.
In reviewing the outcomes of the studies, the committee reports first on what kinds of attention to these issues were apparent in the database, and second on what kinds of results were produced. Some of the studies used multiple methods to provide readers with information on these issues. In our report on the evaluations, we both provide descriptive information on the approaches used and summarize the results of those studies. Developing more effective methods to monitor the achievement of these objectives may need to go beyond what is reported in this study.
Among the 63 at least minimally methodologically adequate studies, 26 reported on the effects of their programs on subgroups of students. The
TABLE 5-13 Most Common Subgroups Used in the Analyses and the Number of Studies That Reported on That Variable
Identified Subgroup | Number of Studies of NSF-Supported | Number of Studies of Commercially Generated | Total |
Gender | 14 | 5 | 19 |
Race and ethnicity | 14 | 2 | 16 |
Socioeconomic status | 8 | 2 | 10 |
Achievement levels | 5 | 3 | 8 |
English as a second language (ESL) | 2 | 1 | 3 |
Total | 43 | 13 | 56 |
Achievement levels: Outcome data are reported in relation to categorizations by quartiles or by achievement level based on independent test. |
other 37 reported on the effects of the curricular intervention on means of whole groups and their standard deviations, but did not report on their data in terms of the impact on subpopulations. Of those 26 evaluations, 19 studies were on NSF-supported programs and 7 were on commercially generated materials. Table 5-13 reports the most common subgroups used in the analyses and the number of studies that reported on that variable. Because many studies used multiple categories for disaggregation (ethnicity, SES, and gender), the number of reports is more than double the number of studies. For this reason, we report the study results in terms of the “frequency of reports on a particular subgroup” and distinguish this from what we refer to as “study counts.” The advantage of this approach is that it permits reporting on studies that investigated multiple ways to disaggregate their data. The disadvantage is that in a sense, studies undertaking multiple disaggregations become overrepresented in the data set as a result. A similar distinction and approach were used in our treatment of disaggregation by content strands.
It is apparent from these data that the evaluators of NSF-supported curricula documented more equity-based outcomes, as they reported 43 of the 56 comparisons. However, the same percentage of the NSF-supported evaluations disaggregated their results by subgroup, as did commercially generated evaluations (41 percent in both cases). This is an area where evaluations of curricula could benefit greatly from standardization of ex-
pectation and methodology. Given the importance of the topic of equity, it should be standard practice to include such analyses in evaluation studies.
In summarizing these 26 studies, the first consideration was whether representative samples of students were evaluated. As we have learned from medical studies, if conclusions on effectiveness are drawn without careful attention to representativeness of the sample relative to the whole population, then the generalizations drawn from the results can be seriously flawed. In Chapter 2 we reported that across the studies, approximately 81 percent of the comparative studies and 73 percent of the case studies reported data on school location (urban, suburban, rural, or state/region), with suburban students being the largest percentage in both study types. The proportions of students studied indicated a tendency to undersample urban and rural populations and oversample suburban schools. With a high concentration of minorities and lower SES students in these areas, there are some concerns about the representativeness of the work.
A second consideration was to see whether the achievement effects of curricular interventions were achieved evenly among the various subgroups. Studies answered this question in different ways. Most commonly, evaluators reported on the performance of various subgroups in the treatment conditions as compared to those same subgroups in the comparative condition. They reported outcome scores or gains from pretest to posttest. We refer to these as “between” comparisons.
Other studies reported on the differences among subgroups within an experimental treatment, describing how well one group does in comparison with another group. Again, these reports were done in relation either to outcome measures or to gains from pretest to posttest. Often these reports contained a time element, reporting on how the internal achievement patterns changed over time as a curricular program was used. We refer to these as “within” comparisons.
Some studies reported both between and within comparisons. Others did not report findings by comparing mean scores or gains, but rather created regression equations that predicted the outcomes and examined whether demographic characteristics are related to performance. Six studies (all on NSF-supported curricula) used this approach with variables related to subpopulations. Twelve studies used ANCOVA or Multiple Analysis of Variance (MANOVA) to study disaggregation by subgroup, and two reported on comparative effect sizes. In the studies using statistical tests other than t-tests or Chi-squares, two were evaluations of commercially generated materials and the rest were of NSF-supported materials.
Of the studies that reported on gender (n=19), the NSF-supported ones (n=13) reported five cases in which the females outperformed their counterparts in the controls and one case in which the female-male gap decreased within the experimental treatments across grades. In most cases, the studies
present a mixed picture with some bright spots, with the majority showing no significant difference. One study reported significant improvements for African-American females.
In relation to race, 15 of 16 reports on African Americans showed positive effects in favor of the treatment group for NSF-supported curricula. Two studies reported decreases in the gaps between African Americans and whites or Asians. One of the two evaluations of African Americans, performance reported for the commercially generated materials, showed significant positive results, as mentioned previously.
For Hispanic students, 12 of 15 reports of the NSF-supported materials were significantly positive, with the other 3 showing no significant difference. One study reported a decrease in the gaps in favor of the experimental group. No evaluations of commercially generated materials were reported on Hispanic populations. Other reports on ethnic groups occurred too seldom to generalize.
Students from lower socioeconomic groups fared well, according to reported evaluations of NSF-supported materials (n=8), in that experimental groups outperformed control groups in all but one case. The one study of commercially generated materials that included SES as a variable reported no significant difference. For students with limited English proficiency, of the two evaluations of NSF-supported materials, one reported significantly more positive results for the experimental treatment. Likewise, one study of commercially generated materials yielded a positive result at the elementary level.
We also examined the data for ability differences and found reports by quartiles for a few evaluation studies. In these cases, the evaluations showed results across quartiles in favor of the NSF-supported materials. In one case using the same program, the lower quartiles showed the most improvement, and in the other, the gains were in the middle and upper groups for the Iowa Test of Basic Skills and evenly distributed for the informal assessment.
Summary Statements
After reviewing these studies, the committee observed that examining differences by gender, race, SES, and performance levels should be examined as a regular part of any review of effectiveness. We would recommend that all comparative studies report on both “between” and “within” comparisons so that the audience of an evaluation can simply and easily consider the level of improvement, its distribution across subgroups, and the impact of curricular implementation on any gaps in performance. Each of the major categories—gender, race/ethnicity, SES, and achievement level—contributes a significant and contrasting view of curricular impact. Further-
more, more sophisticated accounts would begin to permit, across studies, finer distinctions to emerge, such as the effect of a program on young African-American women or on first generation Asian students.
In addition, the committee encourages further study and deliberation on the use of more complex approaches to the examination of equity issues. This is particularly important due to the overlaps among these categories, where poverty can show itself as its own variable but also may be highly correlated to prior performance. Hence, the use of one variable can mask differences that should be more directly attributable to another. The committee recommends that a group of measurement and equity specialists confer on the most effective design to advance on these questions.
Finally, it is imperative that evaluation studies systematically include demographically representative student populations and distinguish evaluations that follow the commercial patterns of use from those that seek to establish effectiveness with a diverse student population. Along these lines, it is also important that studies report on the impact data on all substantial ethnic groups, including whites. Many studies, perhaps because whites were the majority population, failed to report on this ethnic group in their analyses. As we saw in one study, where Asian students were from poor homes and first generation, any subgroup can be an at-risk population in some setting, and because gains in means may not necessarily be assumed to translate to gains for all subgroups or necessarily for the majority subgroup. More complete and thorough descriptions and configurations of characteristics of the subgroups being served at any location—with careful attention to interactions—is needed in evaluations.
Interactions Among Content and Equity, by Grade Band
By examining disaggregation by content strand by grade levels, along with disaggregation by diverse subpopulations, the committee began to discover grade band patterns of performance that should be useful in the conduct of future evaluations. Examining each of these issues in isolation can mask some of the overall effects of curricular use. Two examples of such analysis are provided. The first example examines all the evaluations of NSF-supported curricula from the elementary level. The second examines the set of evaluations of NSF-supported curricula at the high school level, and cannot be carried out on evaluations of commercially generated programs because they lack disaggregation by student subgroup.
Example One
At the elementary level, the findings of the review of evaluations of data on effectiveness of NSF-supported curricula report consistent patterns of
benefits to students. Across the studies, it appears that positive results are enhanced when accompanied by adequate professional development and the use of pedagogical methods consistent with those indicated by the curricula. The benefits are most consistently evidenced in the broadening topics of geometry, measurement, probability, and statistics, and in applied problem solving and reasoning. It is important to consider whether the outcome measures in these areas demonstrate a depth of understanding. In early understanding of fractions and algebra, there is some evidence of improvement. Weaknesses are sometimes reported in the areas of computational skills, especially in the routinization of multiplication and division. These assertions are tentative due to the possible flaws in designs but quite consistent across studies, and future evaluations should seek to replicate, modify, or discredit these results.
The way to most efficiently and effectively link informal reasoning and formal algorithms and procedures is an open question. Further research is needed to determine how to most effectively link the gains and flexibility associated with student-generated reasoning to the automaticity and generalizability often associated with mastery of standard algorithms.
The data from these evaluations at the elementary level generally present credible evidence of increased success in engaging minority students and students in poverty based on reported gains that are modestly higher for these students than for the comparative groups. What is less well documented in the studies is the extent to which the curricula counteract the tendencies to see gaps emerge and result in long-term persistence in performance by gender and minority group membership as they move up the grades. However, the evaluations do indicate that these curricula can help, and almost never do harm. Finally, on the question of adequate challenge for advanced and talented students, the data are equivocal. More attention to this issue is needed.
Example Two
The data at the high school level produced the most conflicting results, and in conducting future evaluations, evaluators will need to examine this level more closely. We identify the high school as the crucible for curricular change for three reasons: (1) the transition to postsecondary education puts considerable pressure on these curricula; (2) the criteria outlined in the NSF RFP specify significant changes from traditional practice; and (3) high school freshmen arrive from a myriad of middle school curricular experiences. For the NSF-supported curricula, the RFP required that the programs provide a core curriculum “drawn from statistics/probability, algebra/functions, geometry/trigonometry, and discrete mathematics” (NSF, 1991, p. 2) and use “a full range of tools, including graphing calculators
and computers” (NSF, 1991, p. 2). The NSF RFP also specified the inclusion of “situations from the natural and social sciences and from other parts of the school curriculum as contexts for developing and using mathematics” (NSF, 1991, p. 1). It was during the fourth year that “course options should focus on special mathematical needs of individual students, accommodating not only the curricular demands of the college-bound but also specialized applications supportive of the workplace aspirations of employment-bound students” (NSF, 1991, p. 2). Because this set of requirements comprises a significant departure from conventional practice, the implementation of the high school curricula should be studied in particular detail.
We report on a Systemic Initiative for Montana Mathematics and Science (SIMMS) study by Souhrada (2001) and Brown et al. (1990), in which students were permitted to select traditional, reform, and mixed tracks. It became apparent that the students were quite aware of the choices they faced, as illustrated in the following quote:
The advantage of the traditional courses is that you learn—just math. It’s not applied. You get a lot of math. You may not know where to use it, but you learn a lot…. An advantage in SIMMS is that the kids in SIMMS tell me that they really understand the math. They understand where it comes from and where it is used.
This quote succinctly captures the tensions reported as experienced by students. It suggests that student perceptions are an important source of evidence in conducting evaluations. As we examined these curricular evaluations across the grades, we paid particular attention to the specificity of the outcome measures in relation to curricular objectives. Overall, a review of these studies would lead one to draw the following tentative summary conclusions:
There is some evidence of discontinuity in the articulation between high school and college, resulting from the organization and emphasis of the new curricula. This discontinuity can emerge in scores on college admission tests, placement tests, and first semester grades where nonreform students have shown some advantage on typical college achievement measures.
The most significant areas of disadvantage seem to be in students’ facility with algebraic manipulation, and with formalization, mathematical structure, and proof when isolated from context and denied technological supports. There is some evidence of weakness in computation and numeration, perhaps due to reliance on calculators and varied policies regarding their use at colleges (Kahan, 1999; Huntley et al., 2000).
There is also consistent evidence that the new curricula present
strengths in areas of solving applied problems, the use of technology, new areas of content development such as probability and statistics and functions-based reasoning in the use of graphs, using data in tables, and producing equations to describe situations (Huntley et al., 2000; Hirsch and Schoen, 2002).
Despite early performance on standard outcome measures at the high school level showing equivalent or better performance by reform students (Austin et al., 1997; Merlino and Wolff, 2001), the common standardized outcome measures (Preliminary Scholastic Assessment Test [PSAT] scores or national tests) are too imprecise to determine with more specificity the comparisons between the NSF-supported and comparison approaches, while program-generated measures lack evidence of external validity and objectivity. There is an urgent need for a set of measures that would provide detailed information on specific concepts and conceptual development over time and may require use as embedded as well as summative assessment tools to provide precise enough data on curricular effectiveness.
The data also report some progress in strengthening the performance of underrepresented groups in mathematics relative to their counterparts in the comparative programs (Schoen et al., 1998; Hirsch and Schoen, 2002).
This reported pattern of results should be viewed as very tentative, as there are only a few studies in each of these areas, and most do not adequately control for competing factors, such as the nature of the course received in college. Difficulties in the transition may also be the result of a lack of alignment of measures, especially as placement exams often emphasize algebraic proficiencies. These results are presented only for the purpose of stimulating further evaluation efforts. They further emphasize the need to be certain that such designs examine the level of mathematical reasoning of students, particularly in relation to their knowledge of understanding of the role of proofs and definitions and their facility with algebraic manipulation as we as carefully document the competencies taught in the curricular materials. In our framework, gauging the ease of transition to college study is an issue of examining curricular alignment with systemic factors, and needs to be considered along with those tests that demonstrate a curricular validity of measures. Furthermore, the results raising concerns about college success need replication before secure conclusions are drawn.
Also, it is important that subsequent evaluations also examine curricular effects on students’ interest in mathematics and willingness to persist in its study. Walker (1999) reported that there may be some systematic differences in these behaviors among different curricula and that interest and persistence may help students across a variety of subgroups to survive entry-level hurdles, especially if technical facility with symbol manipulation
can be improved. In the context of declines in advanced study in mathematics by American students (Hawkins, 2003), evaluation of curricular impact on students’ interest, beliefs, persistence, and success are needed.
The committee takes the position that ultimately the question of the impact of different curricula on performance at the collegiate level should be resolved by whether students are adequately prepared to pursue careers in mathematical sciences, broadly defined, and to reason quantitatively about societal and technological issues. It would be a mistake to focus evaluation efforts solely or primarily on performance on entry-level courses, which can clearly function as filters and may overly emphasize procedural competence, but do not necessarily represent what concepts and skills lead to excellence and success in the field.
These tentative patterns of findings indicate that at the high school level, it is necessary to conduct individual evaluations that examine the transition to college carefully in order to gauge the level of success in preparing students for college entry and the successful negotiation of majors. Equally, it is imperative to examine the impact of high school curricula on other possible student trajectories, such as obtaining high school diplomas, moving into worlds of work or through transitional programs leading to technical training, two-year colleges, and so on.
These two analyses of programs by grade-level band, content strand, and equity represent a methodological innovation that could strengthen the empirical database on curricula significantly and provide the level of detail really needed by curriculum designers to improve their programs. In addition, it appears that one could characterize the NSF programs (and not the commercial programs as a group) as representing a particular approach to curriculum, as discussed in Chapter 3 . It is an approach that integrates content strands; relies heavily on the use of situations, applications, and modeling; encourages the use of technology; and has a significant dose of mathematical inquiry. One could ask the question of whether this approach as a whole is “effective.” It is beyond the charge and scope of this report, but is a worthy target of investigation if one uses proper care in design, execution, and analysis. Likewise other approaches to curricular change should be investigated at the aggregate level, using careful and rigorous design.
The committee believes that a diversity of curricular approaches is a strength in an educational system that maintains local and state control of curricular decision making. While “scientifically established as effective” should be an increasingly important consideration in curricular choice, local cultural differences, needs, values, and goals will also properly influence curricular choice. A diverse set of effective curricula would be ideal. Finally, the committee emphasizes once again the importance of basing the studies on measures with established curricular validity and avoiding cor-
ruption of indicators as a result of inappropriate amounts of teaching to the test, so as to be certain that the outcomes are the product of genuine student learning.
CONCLUSIONS FROM THE COMPARATIVE STUDIES
In summary, the committee reviewed a total of 95 comparative studies. There were more NSF-supported program evaluations than commercial ones, and the commercial ones were primarily on Saxon or UCSMP materials. Of the 19 curricular programs reviewed, 23 percent of the NSF-supported and 33 percent of the commercially generated materials selected had programs with no comparative reviews. This finding is particularly disturbing in light of the legislative mandate in No Child Left Behind (U.S. Department of Education, 2001) for scientifically based curricular programs and materials to be used in the schools. It suggests that more explicit protocols for the conduct of evaluation of programs that include comparative studies need to be required and utilized.
Sixty-nine percent of NSF-supported and 61 percent of commercially generated program evaluations met basic conditions to be classified as at least minimally methodologically adequate studies for the evaluation of effectiveness. These studies were ones that met the criteria of including measures of student outcomes on mathematical achievement, reporting a method of establishing comparability among samples and reporting on implementation elements, disaggregating by content strand, or using precise, theoretical analyses of the construct or multiple measures.
Most of these studies had both strengths and weaknesses in their quasi-experimental designs. The committee reviewed the studies and found that evaluators had developed a number of features that merit inclusions in future work. At the same time, many had internal threats to validity that suggest a need for clearer guidelines for the conduct of comparative evaluations.
Many of the strengths and innovations came from the evaluators’ understanding of the program theories behind the curricula, their knowledge of the complexity of practice, and their commitment to measuring valid and significant mathematical ideas. Many of the weaknesses came from inadequate attention to experimental design, insufficient evidence of the independence of evaluators in some studies, and instability and lack of cooperation in interfacing with the conditions of everyday practice.
The committee identified 10 elements of comparative studies needed to establish a basis for determining the effectiveness of a curriculum. We recognize that not all studies will be able to implement successfully all elements, and those experimental design variations will be based largely on study size and location. The list of elements begins with the seven elements
corresponding to the seven critical decisions and adds three additional elements that emerged as a result of our review:
A better balance needs to be achieved between experimental and quasi-experimental studies. The virtual absence of large-scale experimental studies does not provide a way to determine whether the use of quasi-experimental approaches is being systematically biased in unseen ways.
If a quasi-experimental design is selected, it is necessary to establish comparability. When quasi-experimentation is used, it “pertains to studies in which the model to describe effects of secondary variables is not known but assumed” (NRC, 1992, p. 18). This will lead to weaker and potentially suspect causal claims, which should be acknowledged in the evaluation report, but may be necessary in relation to feasibility (Joint Committee on Standards for Educational Evaluation, 1994). In general, to date, studies have assumed prior achievement measures, ethnicity, gender, and SES, are acceptable variables on which to match samples or on which to make statistical adjustments. But there are often other variables in need of such control in such evaluations including opportunity to learn, teacher effectiveness, and implementation (see #4 below).
The selection of a unit of analysis is of critical importance to the design. To the extent possible, it is useful to randomly assign the unit for the different curricula. The number of units of analysis necessary for the study to establish statistical significance depends not on the number of students, but on this unit of analysis. It appears that classrooms and schools are the most likely units of analysis. In addition, the development of increasingly sophisticated means of conducting studies that recognize that the level of the educational system in which experimentation occurs affects research designs.
It is essential to examine the implementation components through a set of variables that include the extent to which the materials are implemented, teaching methods, the use of supplemental materials, professional development resources, teacher background variables, and teacher effects. Gathering these data to gauge the level of implementation fidelity is essential for evaluators to ensure adequate implementation. Studies could also include nested designs to support analysis of variation by implementation components.
Outcome data should include a variety of measures of the highest quality. These measures should vary by question type (open ended, multiple choice), by type of test (international, national, local) and by relation of testing to everyday practice (formative, summative, high stakes), and ensure curricular validity of measures and assess curricular alignment with systemic factors. The use of comparisons among total tests, fair tests, and
conservative tests, as done in the evaluations of UCSMP, permits one to gain insight into teacher effects and to contrast test results by items included. Tests should also include content strands to aid disaggregation, at a level of major content strands (see Figure 5-11 ) and content-specific items relevant to the experimental curricula.
Statistical analysis should be conducted on the appropriate unit of analysis and should include more sophisticated methods of analysis such as ANOVA, ANCOVA, MACOVA, linear regression, and multiple regression analysis as appropriate.
Reports should include clear statements of the limitations to generalization of the study. These should include indications of limitations in populations sampled, sample size, unique population inclusions or exclusions, and levels of use or attrition. Data should also be disaggregated by gender, race/ethnicity, SES, and performance levels to permit readers to see comparative gains across subgroups both between and within studies.
It is useful to report effect sizes. It is also useful to present item-level data across treatment program and show when performances between the two groups are within the 10 percent confidence interval of each other. These two extremes document how crucial it is for curricula developers to garner both precise and generalizable information to inform their revisions.
Careful attention should also be given to the selection of samples of populations for participation. These samples should be representative of the populations to whom one wants to generalize the results. Studies should be clear if they are generalizing to groups who have already selected the materials (prior users) or to populations who might be interested in using the materials (demographically representative).
The control group should use an identified comparative curriculum or curricula to avoid comparisons to unstructured instruction.
In addition to these prototypical decisions to be made in the conduct of comparative studies, the committee suggests that it would be ideal for future studies to consider some of the overall effects of these curricula and to test more directly and rigorously some of the findings and alternative hypotheses. Toward this end, the committee reported the tentative findings of these studies by program type. Although these results are subject to revision, based on the potential weaknesses in design of many of the studies summarized, the form of analysis demonstrated in this chapter provides clear guidance about the kinds of knowledge claims and the level of detail that we need to be able to judge effectiveness. Until we are able to achieve an array of comparative studies that provide valid and reliable information on these issues, we will be vulnerable to decision making based excessively on opinion, limited experience, and preconceptions.
This book reviews the evaluation research literature that has accumulated around 19 K-12 mathematics curricula and breaks new ground in framing an ambitious and rigorous approach to curriculum evaluation that has relevance beyond mathematics. The committee that produced this book consisted of mathematicians, mathematics educators, and methodologists who began with the following charge:
- Evaluate the quality of the evaluations of the thirteen National Science Foundation (NSF)-supported and six commercially generated mathematics curriculum materials;
- Determine whether the available data are sufficient for evaluating the efficacy of these materials, and if not;
- Develop recommendations about the design of a project that could result in the generation of more reliable and valid data for evaluating such materials.
The committee collected, reviewed, and classified almost 700 studies, solicited expert testimony during two workshops, developed an evaluation framework, established dimensions/criteria for three methodologies (content analyses, comparative studies, and case studies), drew conclusions on the corpus of studies, and made recommendations for future research.
READ FREE ONLINE
Welcome to OpenBook!
You're looking at OpenBook, NAP.edu's online reading room since 1999. Based on feedback from you, our users, we've made some improvements that make it easier than ever to read thousands of publications on our website.
Do you want to take a quick tour of the OpenBook's features?
Show this book's table of contents , where you can jump to any chapter by name.
...or use these buttons to go back to the previous chapter or skip to the next one.
Jump up to the previous page or down to the next one. Also, you can type in a page number and press Enter to go directly to that page in the book.
Switch between the Original Pages , where you can read the report as it appeared in print, and Text Pages for the web version, where you can highlight and search the text.
To search the entire text of this book, type in your search term here and press Enter .
Share a link to this book page on your preferred social network or via email.
View our suggested citation for this chapter.
Ready to take your reading offline? Click here to buy this book in print or download it as a free PDF, if available.
Get Email Updates
Do you enjoy reading reports from the Academies online for free ? Sign up for email notifications and we'll let you know about new publications in your areas of interest when they're released.

An official website of the United States government
The .gov means it’s official. Federal government websites often end in .gov or .mil. Before sharing sensitive information, make sure you’re on a federal government site.
The site is secure. The https:// ensures that you are connecting to the official website and that any information you provide is encrypted and transmitted securely.
- Publications
- Account settings
Preview improvements coming to the PMC website in October 2024. Learn More or Try it out now .
- Advanced Search
- Journal List
- Int J Health Policy Manag
- v.7(9); 2018 Sep
The Qualitative Descriptive Approach in International Comparative Studies: Using Online Qualitative Surveys
Brayan v. seixas.
1 School of Population and Public Health, Faculty of Medicine, University of British Columbia, Vancouver, BC, Canada.
Neale Smith
2 Centre for Clinical Epidemiology & Evaluation, Vancouver Coastal Health Research Institute, University of British Columbia, Vancouver, BC, Canada.
Craig Mitton
International comparative studies constitute a highly valuable contribution to public policy research. Analysing different policy designs offers not only a mean of knowing the phenomenon itself but also gives us insightful clues on how to improve existing practices. Although much of the work carried out in this realm relies on quantitative appraisal of the data contained in international databases or collected from institutional websites, countless topics may simply not be studied using this type of methodological design due to, for instance, the lack of reliable databases, sparse or diffuse sources of information, etc. Here then we discuss the use of the qualitative descriptive approach as a methodological tool to obtain data on how policies are structured. We propose the use of online qualitative surveys with key stakeholders from each relevant national context in order to retrieve the fundamental pieces of information on how a certain public policy is addressed there. Starting from Sandelowski’s seminal paper on qualitative descriptive studies, we conduct a theoretical reflection on the current methodological proposition. We argue that a researcher engaged in this endeavour acts like a composite-sketch artist collecting pieces of information from witnesses in order to draw a valid depiction of reality. Furthermore, we discuss the most relevant aspects involving sampling, data collection and data analysis in this context. Overall, this methodological design has a great potential for allowing researchers to expand the international analysis of public policies to topics hitherto little appraised from this perspective.
Introduction
International comparative studies may contribute enormously to public policy research. Understanding the distinct manners in which a certain issue may be tackled provides better comprehension of the problem itself as well as useful insights on the design of institutional responses. For instance, the works of Hall and Lamont, 1 Stuckler and Basu, 2 and Schrecker and Bambra 3 consistently show through cross-national comparisons how certain economic policies have profoundly impacted on population health.
However international comparisons pose demanding data collection challenges. Much of the work performed in such studies relies on existing databases kept by international agencies, third sector organizations or research institutions (eg, World Bank, OECD, WHO, UN). For studies on some topics, however, there is no reliable database from which the necessary information might be retrieved. Thus, it is vital that alternative, methodologically rigorous approaches emerge.
Here we discuss the use of online qualitative surveys as a tool to overcome the difficulties of conducting comparative studies on public policies in different national jurisdictions. The basic idea is that instead of relying on institutional websites, publicly available policy documents or well-established databases to understand how certain policies have been addressed, we could question key stakeholders (such as policy-makers, public servants or researchers involved with the topic in question) from each relevant national context in order to retrieve essential information directly from them.
Given that the diversity of responses can be totally unanticipated by researchers, it is necessary to have a tool that is open enough to allow any type of information to be captured, for which purpose qualitative methodologies are highly appropriate. However, taking into account that the main objective of this type of research is to obtain comparable information from a potentially large number of different countries, the methodology needs also to pre-structure responses to a sufficient extent to allow for viable and efficient data reduction and analysis. And for this particularity, a qualitative description approach through the use of online qualitative surveys, ie, structured questionnaires with open-ended questions, seems to be an interesting solution.
Thus, this paper aims to provide a discussion of the theoretical reasoning underlying the qualitative description approach – presenting as an adequate solution to the tensions noted above – as well as practical insights on the development of such work within the realm of international comparative studies on public policies.
Theoretical Reasoning
According to Sandelowski, 4 basic or fundamental qualitative description differs from other types of qualitative research, such as grounded theory, ethnography, phenomenology or narrative analysis, in the sense that it is — as the label suggests — essentially descriptive rather than interpretive in focus. This does not mean that a qualitative descriptive approach lacks interpretive efforts or that it intends a supposedly neutral depiction of reality. Qualitative description represents the methodological category that has the least level of inference among the qualitative methods, one that allows “ the reading of lines, as opposed to reading into, between, over or beyond the lines. ” 5 However, it should not be understood as a low-quality approach or solely as an entry-point to really deep research. “ There is nothing trivial or easy about getting the facts, and the meanings participants give to those facts, right and then conveying them in a coherent and useful manner. ” 4 Such qualitative description must be viewed as a valuable end-product in itself, and not simply as an entry-point.
We propose the use of on-line surveys as a way of operationalizing qualitative description in international comparative studies, allowing the retrieval of information on governmental/institutional efforts to develop, implement and evaluate public policies based on the reports of involved stakeholders who draw upon their own situated experience and knowledge. Within this context, we offer a reflection emerging from Kvale’s metaphor 6 on the role of a researcher. Kvale presents two ideal types: the researcher as a miner and the researcher as a traveller. For the former, the reality is out there waiting to be discovered. The job of a miner is then to find the precious stones, the gems, ie, the pieces of reality that have value in a given social setting. Thus, the miner-researcher operates under a predominantly positivist framework. On the contrary, the traveller is experiencing the reality herself. There is no separation of what a traveller has to tell us about the reality from the actual reality. Therefore, the traveller-researcher is not a collector of pieces of information, but rather she/he is the proper constructor of the pieces. This type of researcher marches mainly under a socio-constructivist paradigm.
Kvale’s metaphor is indeed incredibly insightful to reflect on the role of the qualitative researcher in general, which should not be understood as either miner or traveller, but as an enterprise with a predominance of one or the other role. Yet for qualitative descriptive studies particularly, we suggest that another metaphorical representation can be even more powerful. Here we propose that the role of a researcher involved in qualitative descriptive efforts is that of a composite sketch artist . The underlying idea is that this artist has the role of depicting a ‘reality’ based on the reports of the witnesses. In other words, the artist has the duty of drawing a picture that is in accordance to the memories of the witnesses, rather than substituting his/her own speculation in its stead. The artist inevitably has her/his own images in mind, but the aim is to capture the understanding of the other—a picture that the witnesses would agree represents the reality they experienced. Contextualizing this for the field of policy research, the role of the researcher conducting qualitative descriptive study is to retrieve information from stakeholders about their own experiences with the institutions in order to reconstruct the actual governmental designs of public policies or organizational management systems. Thus, the method employed has to faithfully draw the picture upon which most of the interviewees from a given setting will agree.
This metaphor leads us to reflect on the concepts of descriptive and interpretive validity, as elaborated by Maxwell. 7 For Maxwell, descriptive validity refers to the accurate, ‘correct’ or faithful use of the factual aspects of data. It is predominantly related to the elements “pertaining to physical and behavioral events that are, in principle, observable.” 7 For example, policy content and the means by which policy is enacted within given political jurisdictions. Thus, the large majority of the work conducted in qualitative descriptions are almost exclusively circumscribed to this level of interpretation and validity. In other words, descriptive validity deals with how the composite-sketch artist treats the information provided by the witness. It does not mean that the researcher would actually work as a copier or a mere reproducer – impossible as he/she is not within the head of the observer and does not share the experience in question. The important thing to note here is that qualitative description is not an inference-free approach, but rather the methodological work of least inference among categories of qualitative work. In the context of international comparative studies, the researcher has to ‘keep close to the surface’ of the information provided by the stakeholders in order to appropriately describe the local systems of management or public policies.
Albeit the fundamental concern of qualitative descriptive studies is to provide a sort of report of events, institutional structures, and commonly observable behaviors, it is also important that researchers account for the meaning of these things for the people studied. It does not signify that qualitative description will dive deeply into the web of meanings in which subjects are constantly moving, but there has to be at least a conscious movement of acknowledging this phenomenon in order to obtain a valid drawing of the reality. This is what Maxwell calls interpretive validity. 7 Thinking in terms of our proposed metaphor, the composite-sketch artist needs to take into consideration what pieces of information provided by witnesses actually can mean to them. So, the witness may say that the crime perpetrator has big green eyes, but although this constitutes factual information, this is not enough in order to draw the actual eyes that would be recognized by the witness. The understood meaning of ‘big’ emerges on paper through the efforts of the sketch artist. It is necessary to take account some level of interpretive data, though just as long as it indeed helps the ‘reconstruction of reality.’
The next section will focus on the more practical details of developing an online survey as a qualitative descriptive endeavour for international comparative studies.
Methodological Issues
As Sandelowski 5 points out, qualitative description is a distributed residual category and, as such, it makes visible the “ porous lines between qualitative and quantitative description (…) and between the erosion and re-invention of method ” (p. 82). In other words, this category of inquiry may incorporate elements from quantitative and qualitative methodologies and, thus, serve as an innovative research tool. In the particular case of obtaining information from different national contexts, a qualitative description approach allows collection of data that will be analysed not only from the perspective of traditionally qualitative methodologies, but also from a more quantitative lens, making possible a quasi-statistical analysis of content, providing an overall summary of the findings.
For this type of research, we propose a combination of purposeful sampling strategies – here we rely on the classification system developed by Patton. 8 At the initial level of sampling, ie, the country-level, it is important to ensure comparability among the selected nations. This is extremely important for the validity of the quantitizing stage of data analysis, which should report a numerical summary of the data and observed patterns. For this level of sampling, hence, it is important to combine two strategies: homogeneity sampling and criterion sampling. For instance, we could select countries by the number of inhabitants or we could decide to include countries only above or below a given value of gross domestic product (GDP) per capita.
Subsequently, sampling may focus on using strategies to guarantee that there is meaningful variation within the sample and that politically important cases are not missing. For example, it may be appropriate to include cases with distinct institutional models, such as countries with parliamentary and presidential systems, or countries with centralized and decentralized responsibilities for a given public service.
Once the countries to be included in an international comparative study are determined, researchers need to identify individual informants. While an explicit sampling frame (eg, a directory of government department heads) may sometimes be available, strategies such as snowball sampling and convenience sampling may be required in order to make the study viable. Figure depicts this whole process of sampling within the context of using a survey as a qualitative descriptive tool to study public policies across countries.

Purposeful Sampling Strategies for Qualitative Description in International Comparative Studies.
Data Collection
As aforementioned, the main objective of data collection within this context is to obtain information about the institutional design of public policies. For this, researchers will rely on the reports of participants to reconstruct the ‘reality’ of each national scenario.
It is precisely at this point that the survey is the basic tool for collecting data. Respondents located in each national context would be invited to participate in an online qualitative survey. Researchers will have to circulate a structured survey instrument that allows participants to express their ideas on their own terms, but, at the same time, within a format that facilitates or guides the process of data analysis. Considering that qualitative description aims to record the fact, or in other words, to describe the things upon which most people would readily agree, the research team needs to develop an effective survey to engage participants in the description of the essential policy elements, without narrowing their possibilities of responses.
Data Analysis
For Sandelowski, 4 the analytic strategy of choice in qualitative description is Qualitative Content Analysis . This is a dynamic analytical tool intended to depict the informational content of the data. 9 Although similar to quantitative content analysis, this is different because the codes are commonly generated from the data (ie, derived inductively) in the course of the study. In addition, in qualitative content analysis, the quantitizing phase (the stage when the coded data elements can be numerically organized) allows the researcher to go beyond the mere summarization of the manifest data (the information readily retrievable from the raw dataset). Inferring associations, depicting tendencies and making predictions provide insight into the latent content of data obtained (ie, the type of information that requires a deeper analytic effort to be revealed).
In the context of international comparative studies, other data analysis strategies may also prove fruitful. For instance, the gaps between qualitative and quantitative analyses can be bridged using Ragin’s Qualitative Comparative Analysis. 10 This may be a valuable tool to investigate the generalizability of findings as well as the causal complexity of the variables encountered in the coded data.
By conducting qualitative descriptive studies with decision-makers, public servants and/or the local research community, it is possible for international comparative studies to use participants’ contextually-situated knowledge to depict the realities of different public policy contexts. The metaphor of the composite sketch artist can be a powerful device to guide methodological reflections, such as the notions of descriptive validity (the depiction of events as perceived by observers in their apparent sequence) and interpretive validity (the appropriate elicitation of the meanings attributed by the agents to those events). It illuminates the researcher’s role in presenting a picture of the topic investigated that most observers would likely agree with.
We argue that qualitative descriptive efforts are neither thick nor thin descriptions, in the sense used by Clifford Geertz. 11 By his account, a thick description is an instrument that unveils the web of significance allowing the researcher to differentiate among ‘the conspiratorial winking,’ ‘the involuntary twitch,’ ‘the parodic-fake winking,’ and ‘the rehearsing winking,’ whereas the thin description is the mere report of people rapidly contracting the eyelids. A qualitative description is something else. A fundamental or basic qualitative descriptive endeavour would seek to describe, for instance, who are the ones supposedly winking, how many they are, how many times they wink, which other gestures are being done, where these people are situated, who else is present, etc. Basic or fundamental qualitative descriptive studies thus cannot be properly understood within this Geertzian dualistic epistemological framework. Its virtue as a qualitative category of inquiry that stand per se (despite its residual nature) is only acknowledged by inscribing it within other framings. A qualitative description could be understood as a comprehensive description, one that seeks to provide a detailed description of the findings more likely to generate consensus among observers.
In the voluminous literature on qualitative research methods, there is no comprehensive study with a systematic reflection about the use of online qualitative surveys in international comparative studies on public policies. Therefore, our current endeavour may provide a valuable contribution to the research community as a potential approach available to researchers for investigating comparative topics in a methodologically rigorous manner.
Ethical issues
Not applicable.
Competing interests
Authors declare that they have no competing interests.
Authors’ contributions
BVS developed the paper’s central reasoning and crafted the first draft; NS provided extensive support in reviewing the text and offering fundamental contributions; CM supervised the whole work and provided meaningful contributions up to its final version.
Authors’ affiliations
1 School of Population and Public Health, Faculty of Medicine, University of British Columbia, Vancouver, BC, Canada. 2 Centre for Clinical Epidemiology & Evaluation, Vancouver Coastal Health Research Institute, University of British Columbia, Vancouver, BC, Canada.
Citation: Seixas BV, Smith N, Mitton C. The qualitative descriptive approach in international comparative studies: using online qualitative surveys. Int J Health Policy Manag. 2018;7(9):778–781. doi:10.15171/ijhpm.2017.142

Research Title
Ai generator.
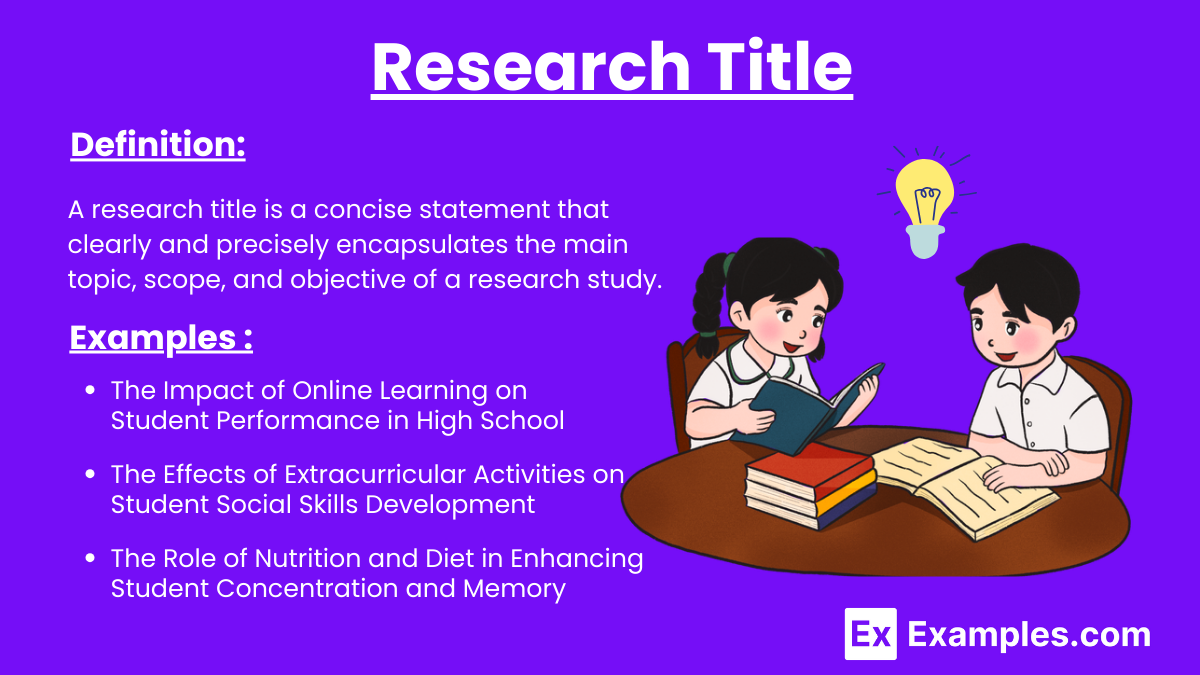
A research title is a succinct, informative phrase that encapsulates a study’s essence. It gives readers a clear indication of the research’s focus, scope, and significance. An effective research title is concise, specific, and engaging, incorporating key terms related to the primary subject matter. Crafting a well-thought-out research title is crucial as it influences first impressions and impacts the study’s visibility and accessibility. Additionally, a strong research title enhances the title page and ensures the research paper cover letter accurately reflects the study’s content.
What is Research Title?
A research title is a concise statement that clearly and precisely encapsulates the main topic, scope, and objective of a research study. It serves as the first point of contact for readers and should effectively communicate the essence of the research in a way that is both engaging and informative. A well-crafted research title is specific, descriptive, and reflective of the study’s core focus, helping to attract interest and provide a clear understanding of the research subject at a glance.
Research Title Format
A well-crafted research title follows a specific format to ensure clarity and precision. Here’s a structured approach:
[Main Topic]: [Specific Aspect or Focus]
Example: “The Impact of Social Media on Teen Mental Health: A Comprehensive Analysis of Behavioral Changes”
Examples of Research Titles
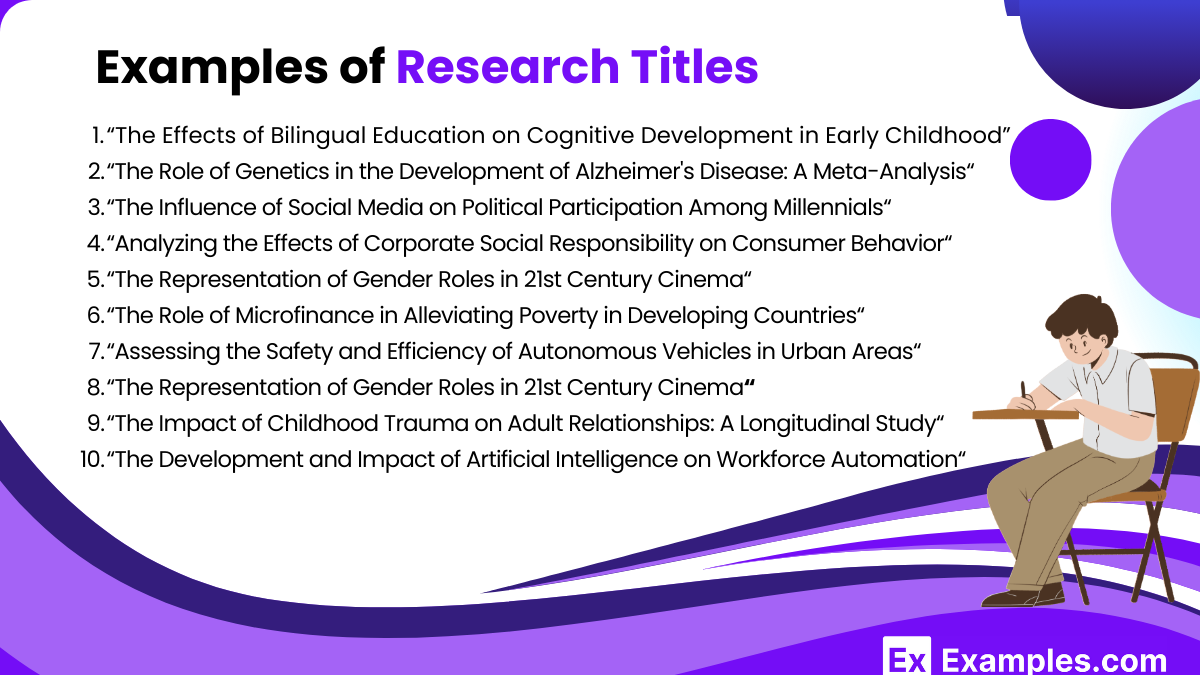
Here are some examples of well-crafted research titles across various fields:
- “The Effects of Bilingual Education on Cognitive Development in Early Childhood”
- “Assessing the Impact of Technology Integration on Student Engagement in High School Classrooms”
- “The Role of Genetics in the Development of Alzheimer’s Disease: A Meta-Analysis”
- “Evaluating the Efficacy of Telemedicine in Managing Chronic Diseases During the COVID-19 Pandemic”
- “The Impact of Urbanization on Local Wildlife Populations: A Case Study of Central Park”
- “Assessing the Effectiveness of Renewable Energy Policies in Reducing Carbon Emissions”
- “The Influence of Social Media on Political Participation Among Millennials”
- “Exploring the Relationship Between Socioeconomic Status and Academic Achievement in Urban Schools”
- “Analyzing the Effects of Corporate Social Responsibility on Consumer Behavior”
- “The Role of Microfinance in Alleviating Poverty in Developing Countries”
- “The Development and Impact of Artificial Intelligence on Workforce Automation”
- “Assessing the Safety and Efficiency of Autonomous Vehicles in Urban Areas”
- “The Representation of Gender Roles in 21st Century Cinema”
- “Exploring the Influence of Renaissance Art on Modern Aesthetic Values”
- “The Impact of Childhood Trauma on Adult Relationships: A Longitudinal Study”
- “Exploring the Effectiveness of Cognitive Behavioral Therapy in Treating Anxiety Disorders”
- “The Effectiveness of Vaccination Campaigns in Reducing the Spread of Infectious Diseases: A Global Perspective”
- “Sustainable Farming Practices and Their Impact on Soil Health: A Comparative Study of Organic and Conventional Methods”
Research Titles for Students
- The Impact of Online Learning on Student Performance in High School
- Exploring the Relationship Between Sleep Patterns and Academic Achievement Among College Students
- The Effects of Extracurricular Activities on Student Social Skills Development
- The Influence of Peer Pressure on High School Students’ Academic Choices
- Assessing the Benefits of Early Childhood Education Programs on Later Academic Success
- The Role of Nutrition and Diet in Enhancing Student Concentration and Memory
- Examining the Effectiveness of Study Groups in Improving Academic Performance in University Settings
- The Impact of Part-Time Employment on High School Students’ Academic Achievement and Time Management
- Exploring the Relationship Between Physical Activity and Mental Health Among College Students
- The Effects of School Uniform Policies on Student Behavior and Academic Outcomes
Qualitative Research Titles
- Exploring Student Perceptions of Remote Learning During the COVID-19 Pandemic
- The Lived Experiences of First-Generation College Students: Challenges and Triumphs
- Understanding Teacher Attitudes Towards Inclusive Education in Mainstream Classrooms
- The Impact of Parental Involvement on Student Motivation and Academic Success
- Exploring the Cultural Adaptation Experiences of International Students in American Universities
- The Role of Peer Support in Coping with Academic Stress Among High School Students
- Investigating the Influence of School Climate on Teacher Job Satisfaction and Retention
- The Effects of Community-Based Learning on Student Engagement and Civic Responsibility
- Understanding the Barriers to STEM Education for Female Students in Rural Areas
- Exploring the Experiences of Students with Learning Disabilities in Higher Education
- The Impact of School Leadership Styles on Teacher Morale and Performance
- The Role of Mentorship Programs in Supporting Minority Students in STEM Fields
- Exploring the Emotional and Social Impacts of Bullying on Middle School Students
- The Influence of Extracurricular Activities on Identity Development in Adolescents
- Understanding the Perspectives of Parents on Bilingual Education Programs
Quantitative Research Titles
- The Impact of Class Size on Student Academic Achievement in Elementary Schools
- Analyzing the Correlation Between Homework Frequency and Student Performance in Mathematics
- The Effects of School Funding on Standardized Test Scores in Public Schools
- Assessing the Relationship Between Attendance Rates and Graduation Rates in High Schools
- Evaluating the Effectiveness of Flipped Classrooms on Student Learning Outcomes
- The Influence of Parental Education Levels on Children’s Academic Success
- The Impact of Early Childhood Education on Literacy Rates in Primary School Students
- Comparing Academic Performance Between Students in Single-Sex and Coeducational Schools
- The Role of Technology in Enhancing Student Engagement in STEM Subjects
- Analyzing the Impact of Nutrition Programs on Student Health and Academic Performance
- The Relationship Between Physical Activity and Academic Achievement in High School Students
- Evaluating the Success of Mentorship Programs on College Retention Rates
- The Effects of Sleep Patterns on Academic Performance Among University Students
- Assessing the Impact of Socioeconomic Status on Access to Higher Education
- The Influence of Teacher Qualifications on Student Achievement in Science
Importance of a Research Title
A research title is a critical component of any research study or academic paper. It serves multiple important functions that contribute to the overall success and impact of the research. Here are key reasons why a research title is important:
1. First Impression
The research title is often the first element a reader encounters. A well-crafted title can create a strong first impression, attracting the reader’s attention and encouraging them to explore the study further.
2. Clarity and Focus
A good research title clearly and succinctly communicates the main topic and scope of the study. It helps the reader quickly understand what the research is about and what specific aspect is being addressed.
3. Guidance
The title provides guidance to the reader about the content and direction of the research. It sets expectations and helps readers decide if the paper is relevant to their interests or research needs.
4. Searchability
In the digital age, research titles are crucial for searchability. A precise and descriptive title improves the chances of the paper being found in online searches, databases, and academic journals, increasing its visibility and accessibility.
5. Academic and Professional Recognition
A well-formulated research title contributes to the academic and professional recognition of the work. It reflects the researcher’s ability to clearly define and articulate their study, which can enhance credibility and reputation within the academic community.
Characteristics of a Good Research Title
A good research title is essential for effectively communicating the main focus and scope of your study. Here are the key characteristics that make a research title effective:
- Clear and Understandable : The title should be easily understood by a broad audience, avoiding jargon or overly complex language.
- Direct : It should convey the main topic and scope of the research without ambiguity.
2. Conciseness
- Brevity : A good title is concise and to the point, typically no longer than 10-15 words.
- Essential Information : It includes only the most relevant information, omitting unnecessary words.
3. Specificity
- Focused : The title should clearly reflect the specific aspect or focus of the research.
- Detailed : It provides enough detail to give a clear sense of what the study entails.
4. Descriptiveness
- Informative : It accurately describes the content and scope of the study.
- Comprehensive : The title should give readers a good understanding of the research without needing to read the entire paper.
5. Keywords
- Relevant Keywords : Including key terms that are central to the research topic helps with searchability and indexing.
- SEO-Friendly : Using keywords that align with what potential readers might search for increases the paper’s visibility.
6. Engagement
- Interest : The title should be engaging and interesting, encouraging readers to want to learn more about the study.
- Appeal : It should appeal to the target audience, whether they are academics, practitioners, or the general public.
How to Write a Research Title?
A well-crafted research title is crucial as it provides the first impression of your study. It should be concise, informative, and engaging to capture the reader’s attention while conveying the essence of your research. Here’s a step-by-step guide on how to write an effective research title.
1. Understand the Purpose of the Title
The title should:
- Summarize the main topic of the research.
- Indicate the scope and focus of the study.
- Reflect the methodology used (if applicable).
- Attract the target audience’s interest.
2. Identify the Key Components
To create a comprehensive title, identify the following components of your research:
- Main topic : The primary subject or focus.
- Variables : Key elements or factors studied.
- Population/sample : The group or sample studied.
- Methodology : The approach or techniques used in the research.
3. Be Clear and Specific
Avoid vague and ambiguous terms. Be precise in describing your research. For example, instead of “Study of Education Methods,” use “Effectiveness of Interactive Learning Techniques in High School Biology.”
4. Keep It Concise
A good title is typically between 10 to 15 words. It should be long enough to include essential information but short enough to be easily readable.
5. Use Descriptive Words
Use words that describe the content and aim of your research effectively. Descriptive words help in making the title informative and engaging. Examples include “effects,” “analysis,” “evaluation,” “comparison,” etc.
6. Avoid Jargon and Abbreviations
Ensure that your title is accessible to a broad audience by avoiding technical jargon and abbreviations that might not be widely understood.
7. Consider the Audience
Think about who will be reading your research. Tailor your title to meet the expectations and interests of your target audience, whether they are academic peers, professionals, or the general public.
8. Reflect the Type of Study
Indicate whether the research is a review, case study, experiment, or theoretical analysis. This helps set the context for the reader. For example, “A Case Study on Renewable Energy Adoption in Urban Areas.”
9. Include Keywords
Incorporate relevant keywords that reflect the main themes of your research. This not only helps in search engine optimization but also makes your research easily discoverable.
10. Revise and Refine
Review your title for clarity, conciseness, and accuracy. Ask for feedback from peers or mentors to ensure it effectively represents your research.
FAQ’s
How should a research title be structured.
A research title should be clear, concise, and informative, often including the main variables, methods, and context of the study.
What are the key elements of a good research title?
Key elements include relevance, clarity, specificity, and the inclusion of main keywords related to the research topic.
Can a research title be a question?
Yes, a research title can be a question if it effectively conveys the research’s focus and intrigues the reader.
How long should a research title be?
A research title should be brief but descriptive, typically between 10 to 15 words, avoiding unnecessary jargon or overly complex terms.
Should a research title include keywords?
Yes, including keywords helps in indexing and searching, making it easier for others to find your research.
Can a research title change during the research process?
Yes, it can be refined or adjusted as the research progresses to better reflect the study’s findings and scope.
Should the research title reflect the research methodology?
It can, especially if the methodology is central to the study’s uniqueness or understanding, but it’s not always necessary.
How specific should a research title be?
A research title should be specific enough to give a clear idea of the study’s focus but not so detailed that it becomes cumbersome.
What makes a research title catchy?
A catchy research title is engaging, piques curiosity, and uses intriguing language while still being clear and informative.
Can humor be used in a research title?
Humor can be used if appropriate for the subject matter and audience, but it should not compromise clarity or professionalism.
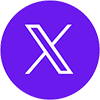
Text prompt
- Instructive
- Professional
10 Examples of Public speaking
20 Examples of Gas lighting
- Skip to main content
- Skip to primary sidebar
- Skip to footer
- QuestionPro

- Solutions Industries Gaming Automotive Sports and events Education Government Travel & Hospitality Financial Services Healthcare Cannabis Technology Use Case NPS+ Communities Audience Contactless surveys Mobile LivePolls Member Experience GDPR Positive People Science 360 Feedback Surveys
- Resources Blog eBooks Survey Templates Case Studies Training Help center

Home Market Research Research Tools and Apps
Causal Comparative Research: Definition, Types & Benefits
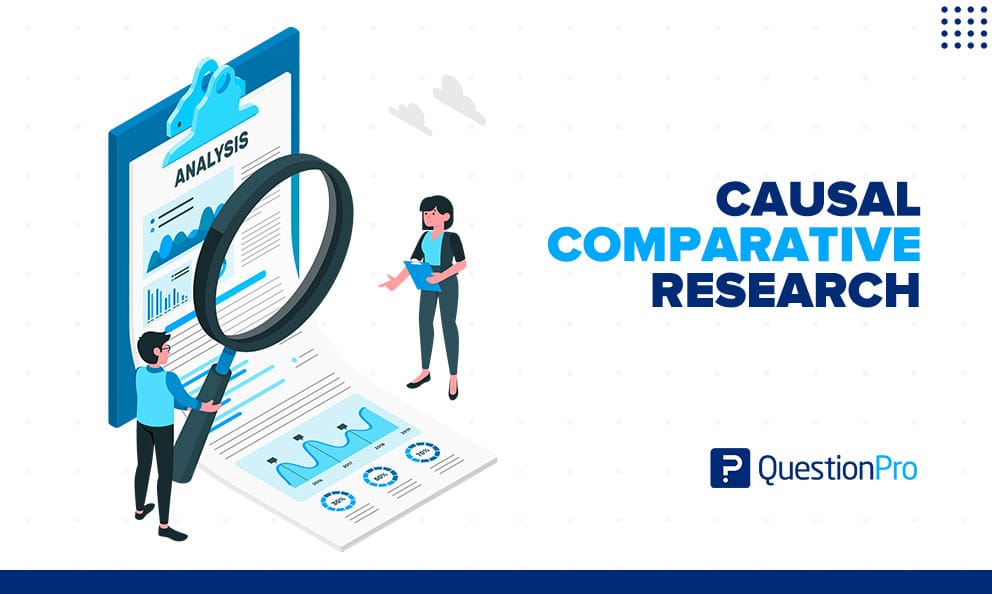
Within the field of research, there are multiple methodologies and ways to find answers to your needs, in this article we will address everything you need to know about Causal Comparative Research, a methodology with many advantages and applications.
What Is Causal Comparative Research?
Causal-comparative research is a methodology used to identify cause-effect relationships between independent and dependent variables.
Researchers can study cause and effect in retrospect. This can help determine the consequences or causes of differences already existing among or between different groups of people.
When you think of Casual Comparative Research, it will almost always consist of the following:
- A method or set of methods to identify cause/effect relationships
- A set of individuals (or entities) that are NOT selected randomly – they were intended to participate in this specific study
- Variables are represented in two or more groups (cannot be less than two, otherwise there is no differentiation between them)
- Non-manipulated independent variables – *typically, it’s a suggested relationship (since we can’t control the independent variable completely)
Types of Casual Comparative Research
Casual Comparative Research is broken down into two types:
- Retrospective Comparative Research
- Prospective Comparative Research
Retrospective Comparative Research: Involves investigating a particular question…. after the effects have occurred. As an attempt to see if a specific variable does influence another variable.
Prospective Comparative Research: This type of Casual Comparative Research is characterized by being initiated by the researcher and starting with the causes and determined to analyze the effects of a given condition. This type of investigation is much less common than the Retrospective type of investigation.
LEARN ABOUT: Quasi-experimental Research
Causal Comparative Research vs Correlation Research
The universal rule of statistics… correlation is NOT causation!
Casual Comparative Research does not rely on relationships. Instead, they’re comparing two groups to find out whether the independent variable affected the outcome of the dependent variable
When running a Causal Comparative Research, none of the variables can be influenced, and a cause-effect relationship has to be established with a persuasive, logical argument; otherwise, it’s a correlation.
Another significant difference between both methodologies is their analysis of the data collected. In the case of Causal Comparative Research, the results are usually analyzed using cross-break tables and comparing the averages obtained. At the same time, in Causal Comparative Research, Correlation Analysis typically uses scatter charts and correlation coefficients.
Advantages and Disadvantages of Causal Comparative Research
Like any research methodology, causal comparative research has a specific use and limitations to consider when considering them in your next project. Below we list some of the main advantages and disadvantages.
- It is more efficient since it allows you to save human and economic resources and to do it relatively quickly.
- Identifying causes of certain occurrences (or non-occurrences)
- Thus, descriptive analysis rather than experimental
Disadvantages
- You’re not fully able to manipulate/control an independent variable as well as the lack of randomization
- Like other methodologies, it tends to be prone to some research bias , the most common type of research is subject- selection bias , so special care must be taken to avoid it so as not to compromise the validity of this type of research.
- The loss of subjects/location influences / poor attitude of subjects/testing threats….are always a possibility
Finally, it is important to remember that the results of this type of causal research should be interpreted with caution since a common mistake is to think that although there is a relationship between the two variables analyzed, this does not necessarily guarantee that the variable influences or is the main factor to influence in the second variable.
LEARN ABOUT: ANOVA testing
QuestionPro can be your ally in your next Causal Comparative Research
QuestionPro is one of the platforms most used by the world’s leading research agencies, thanks to its diverse functions and versatility when collecting and analyzing data.
With QuestionPro you will not only be able to collect the necessary data to carry out your causal comparative research, you will also have access to a series of advanced reports and analyses to obtain valuable insights for your research project.
We invite you to learn more about our Research Suite, schedule a free demo of our main features today, and clarify all your doubts about our solutions.
LEARN MORE SIGN UP FREE
Author : John Oppenhimer
MORE LIKE THIS
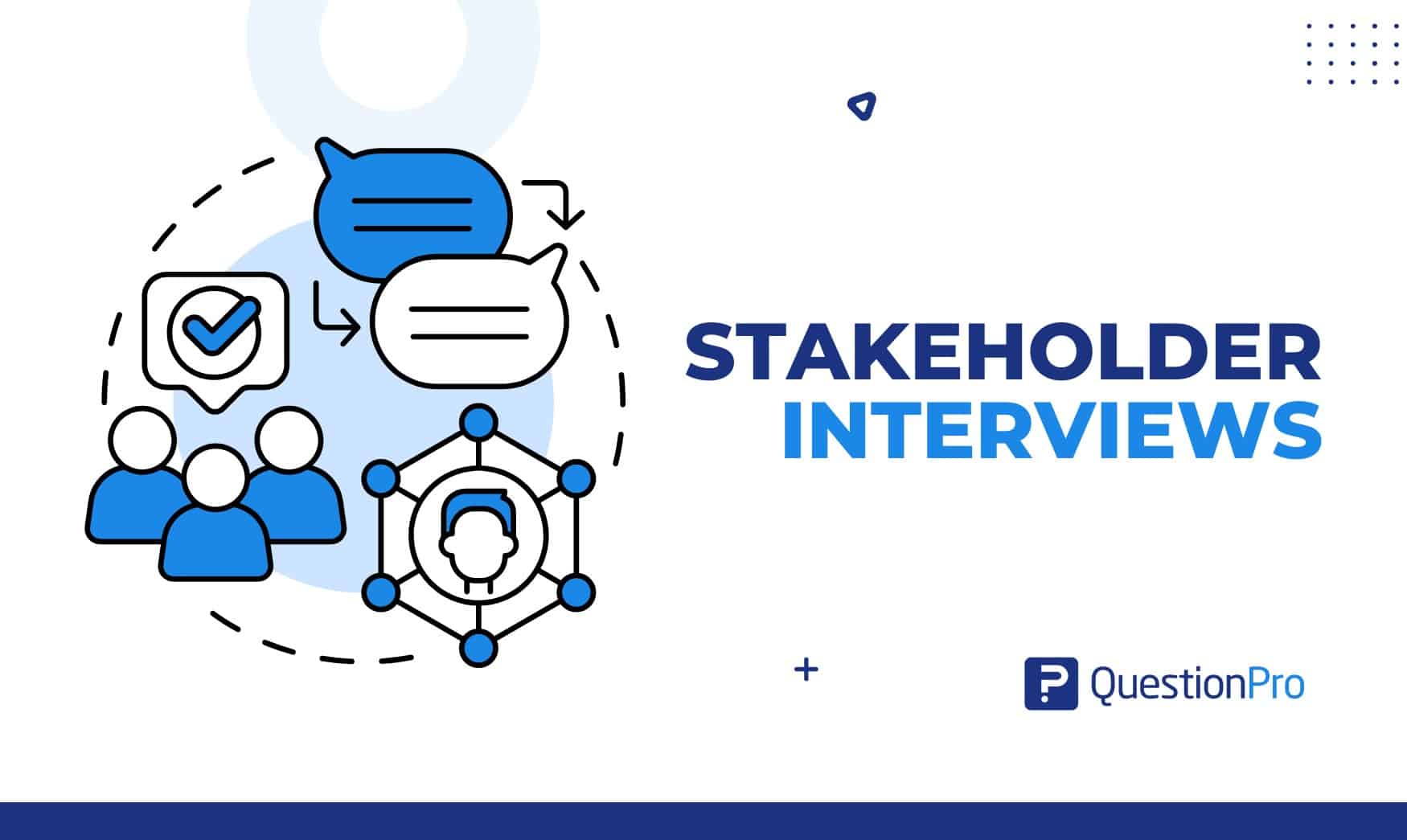
Stakeholder Interviews: A Guide to Effective Engagement
Jul 2, 2024
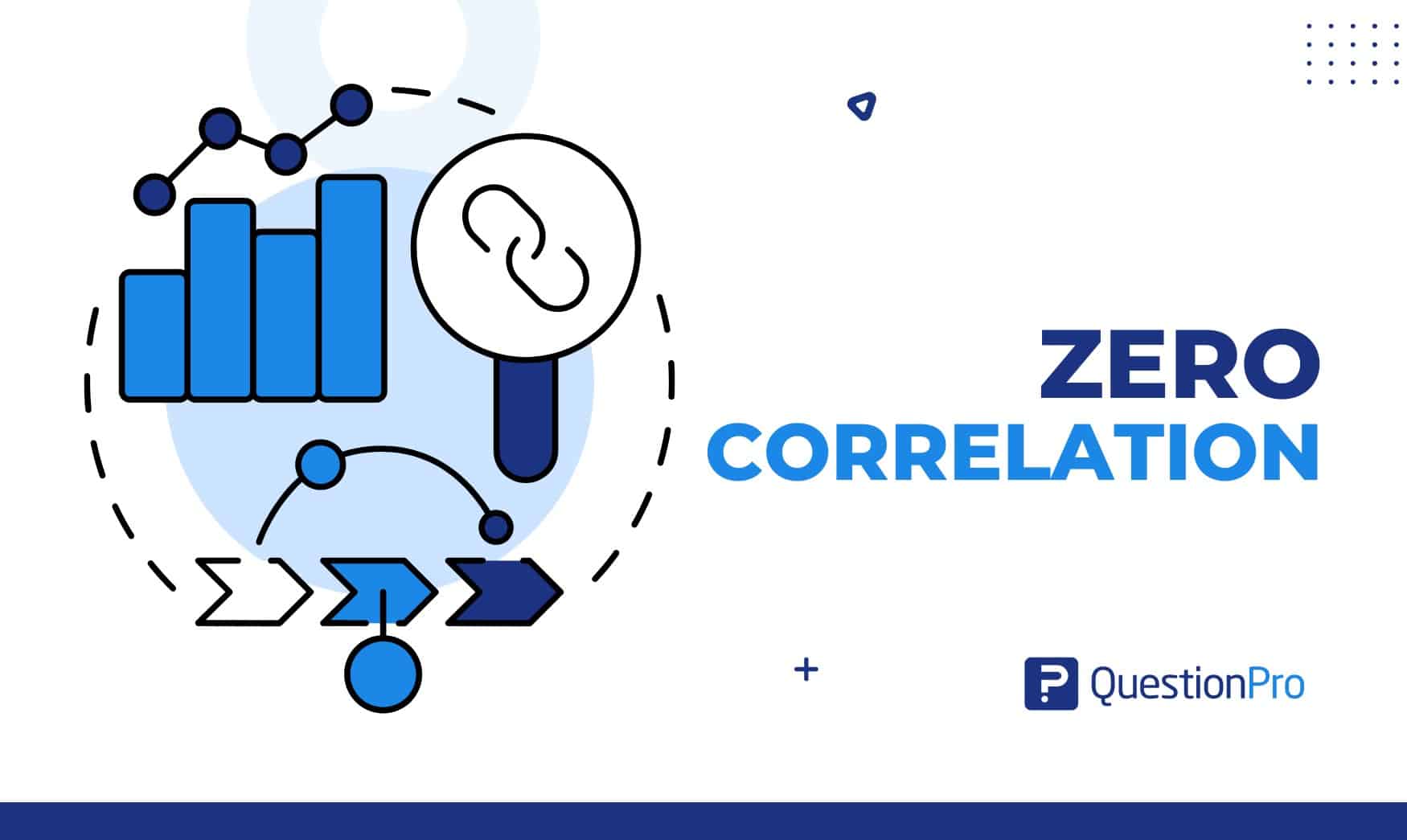
Zero Correlation: Definition, Examples + How to Determine It
Jul 1, 2024
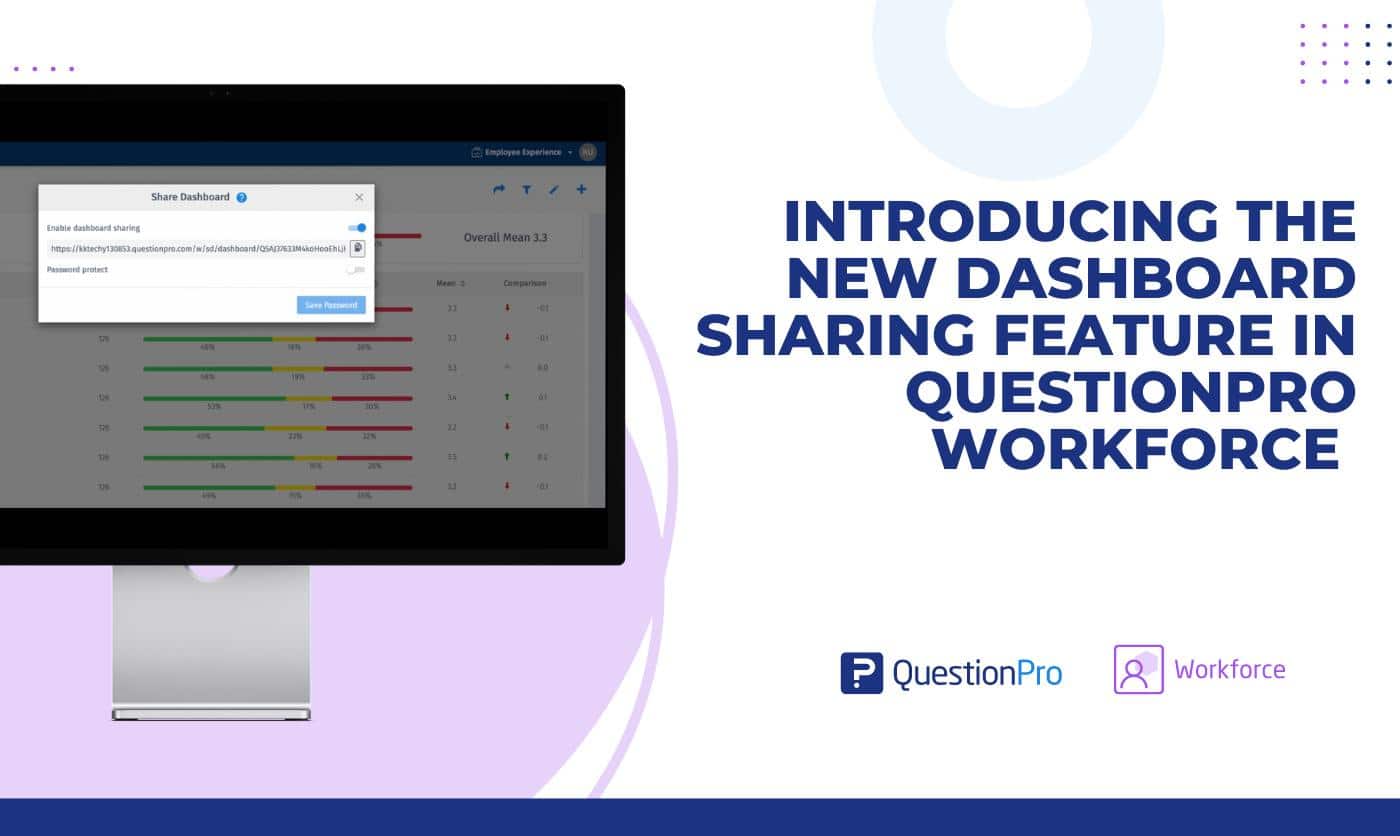
When You Have Something Important to Say, You want to Shout it From the Rooftops
Jun 28, 2024
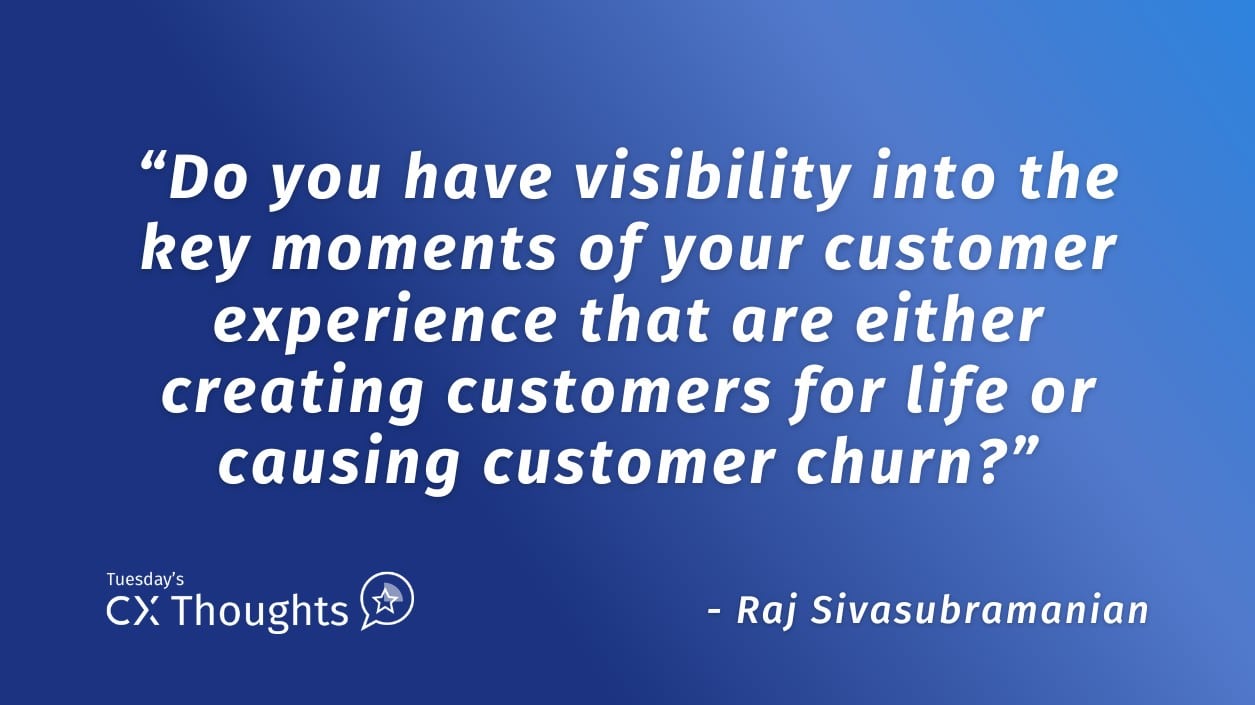
The Item I Failed to Leave Behind — Tuesday CX Thoughts
Jun 25, 2024
Other categories
- Academic Research
- Artificial Intelligence
- Assessments
- Brand Awareness
- Case Studies
- Communities
- Consumer Insights
- Customer effort score
- Customer Engagement
- Customer Experience
- Customer Loyalty
- Customer Research
- Customer Satisfaction
- Employee Benefits
- Employee Engagement
- Employee Retention
- Friday Five
- General Data Protection Regulation
- Insights Hub
- Life@QuestionPro
- Market Research
- Mobile diaries
- Mobile Surveys
- New Features
- Online Communities
- Question Types
- Questionnaire
- QuestionPro Products
- Release Notes
- Research Tools and Apps
- Revenue at Risk
- Survey Templates
- Training Tips
- Tuesday CX Thoughts (TCXT)
- Uncategorized
- Video Learning Series
- What’s Coming Up
- Workforce Intelligence

Causal Comparative Research: Methods And Examples
Ritu was in charge of marketing a new protein drink about to be launched. The client wanted a causal-comparative study…

Ritu was in charge of marketing a new protein drink about to be launched. The client wanted a causal-comparative study highlighting the drink’s benefits. They demanded that comparative analysis be made the main campaign design strategy. After carefully analyzing the project requirements, Ritu decided to follow a causal-comparative research design. She realized that causal-comparative research emphasizing physical development in different groups of people would lay a good foundation to establish the product.
What Is Causal Comparative Research?
Examples of causal comparative research variables.
Causal-comparative research is a method used to identify the cause–effect relationship between a dependent and independent variable. This relationship is usually a suggested relationship because we can’t control an independent variable completely. Unlike correlation research, this doesn’t rely on relationships. In a causal-comparative research design, the researcher compares two groups to find out whether the independent variable affected the outcome or the dependent variable.
A causal-comparative method determines whether one variable has a direct influence on the other and why. It identifies the causes of certain occurrences (or non-occurrences). It makes a study descriptive rather than experimental by scrutinizing the relationships among different variables in which the independent variable has already occurred. Variables can’t be manipulated sometimes, but a link between dependent and independent variables is established and the implications of possible causes are used to draw conclusions.
In a causal-comparative design, researchers study cause and effect in retrospect and determine consequences or causes of differences already existing among or between groups of people.
Let’s look at some characteristics of causal-comparative research:
- This method tries to identify cause and effect relationships.
- Two or more groups are included as variables.
- Individuals aren’t selected randomly.
- Independent variables can’t be manipulated.
- It helps save time and money.
The main purpose of a causal-comparative study is to explore effects, consequences and causes. There are two types of causal-comparative research design. They are:
Retrospective Causal Comparative Research
For this type of research, a researcher has to investigate a particular question after the effects have occurred. They attempt to determine whether or not a variable influences another variable.
Prospective Causal Comparative Research
The researcher initiates a study, beginning with the causes and determined to analyze the effects of a given condition. This is not as common as retrospective causal-comparative research.
Usually, it’s easier to compare a variable with the known than the unknown.
Researchers use causal-comparative research to achieve research goals by comparing two variables that represent two groups. This data can include differences in opportunities, privileges exclusive to certain groups or developments with respect to gender, race, nationality or ability.
For example, to find out the difference in wages between men and women, researchers have to make a comparative study of wages earned by both genders across various professions, hierarchies and locations. None of the variables can be influenced and cause-effect relationship has to be established with a persuasive logical argument. Some common variables investigated in this type of research are:
- Achievement and other ability variables
- Family-related variables
- Organismic variables such as age, sex and ethnicity
- Variables related to schools
- Personality variables
While raw test scores, assessments and other measures (such as grade point averages) are used as data in this research, sources, standardized tests, structured interviews and surveys are popular research tools.
However, there are drawbacks of causal-comparative research too, such as its inability to manipulate or control an independent variable and the lack of randomization. Subject-selection bias always remains a possibility and poses a threat to the internal validity of a study. Researchers can control it with statistical matching or by creating identical subgroups. Executives have to look out for loss of subjects, location influences, poor attitude of subjects and testing threats to produce a valid research study.
Harappa’s Thinking Critically program is for managers who want to learn how to think effectively before making critical decisions. Learn how leaders articulate the reasons behind and implications of their decisions. Become a growth-driven manager looking to select the right strategies to outperform targets. It’s packed with problem-solving and effective-thinking tools that are essential for skill development. What more? It offers live learning support and the opportunity to progress at your own pace. Ask for your free demo today!
Explore Harappa Diaries to learn more about topics such as Objectives Of Research Methodology , Types Of Thinking , What Is Visualisation and Effective Learning Methods to upgrade your knowledge and skills.
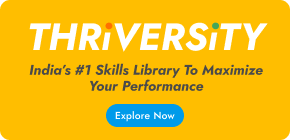

IMAGES
VIDEO
COMMENTS
Descriptive-comparative; In descriptive-comparative research, the researcher considers 2 variables that are not manipulated, and establish a formal procedure to conclude that one is better than the other. For example, an examination body wants to determine the better method of conducting tests between paper-based and computer-based tests.
As discussed earlier, common data analysis methods for descriptive research include descriptive statistics, cross-tabulation, content analysis, qualitative coding, visualization, and comparative analysis. I nterpret results: Interpret your findings in light of your research question and objectives.
Types of Research Designs Compared | Guide & Examples. Published on June 20, 2019 by Shona McCombes.Revised on June 22, 2023. When you start planning a research project, developing research questions and creating a research design, you will have to make various decisions about the type of research you want to do.. There are many ways to categorize different types of research.
Descriptive research aims to accurately and systematically describe a population, situation or phenomenon. It can answer what, where, when and how questions, but not why questions. A descriptive research design can use a wide variety of research methods to investigate one or more variables. Unlike in experimental research, the researcher does ...
Descriptive research is an exploratory research method.It enables researchers to precisely and methodically describe a population, circumstance, or phenomenon.. As the name suggests, descriptive research describes the characteristics of the group, situation, or phenomenon being studied without manipulating variables or testing hypotheses.This can be reported using surveys, observational ...
A descriptive-comparative research design is intended to describe the differences among groups in a population without manipulating the independent variable (Cantrell, 2011). In this study, the ...
For example, suppose you are a website beta testing an app feature. In that case, descriptive research invites users to try the feature, tracking their behavior and then asking their opinions. Can be applied to many research methods and areas. Examples include healthcare, SaaS, psychology, political studies, education, and pop culture.
INTRODUCTION. In our previous article in this series, [ 1] we introduced the concept of "study designs"- as "the set of methods and procedures used to collect and analyze data on variables specified in a particular research question.". Study designs are primarily of two types - observational and interventional, with the former being ...
Descriptive research aims to accurately and systematically describe a population, situation or phenomenon. It can answer what, where, when, and how questions, but not why questions. A descriptive research design can use a wide variety of research methods to investigate one or more variables. Unlike in experimental research, the researcher does ...
Comparative research in communication and media studies is conventionally understood as the contrast among different macro-level units, such as world regions, countries, sub-national regions, social milieus, language areas and cultural thickenings, at one point or more points in time. ... In the latter case, descriptive comparative analysis ...
What makes a study comparative is not the particular techniques employed but the theoretical orientation and the sources of data. All the tools of the social scientist, including historical analysis, fieldwork, surveys, and aggregate data analysis, can be used to achieve the goals of comparative research. So, there is plenty of room for the ...
6. Technological Advances in Healthcare (Healthcare): This research describes and categorizes different technological advances (such as telemedicine, AI-enabled tools, digital collaboration) in healthcare without testing or modifying any parameters, making it an example of descriptive research. 7.
Descriptive research is a methodological approach that seeks to depict the characteristics of a phenomenon or subject under investigation. In scientific inquiry, it serves as a foundational tool for researchers aiming to observe, record, and analyze the intricate details of a particular topic. This method provides a rich and detailed account ...
Descriptive research examples show the thorough research involved in such a study. ... Descriptive Comparative. Comparing two variables can show if one is better than the other. Doing this through tests or surveys can reveal all the advantages and disadvantages associated with the two. For example, this technique can be used to find out if ...
There are two main types of nonexperimental research designs: comparative design and correlational design. In comparative research, the researcher examines the differences between two or more groups on the phenomenon that is being studied. For example, studying gender difference in learning mathematics is a comparative research.
A descriptive correlation study was a research method that observes and characterizes the behavior of participants from a scientific standpoint in relation to factors in a setting. It seeks to ...
In nearly all studies in the comparative group, the titles of experimental curricula were explicitly identified. The only exception to this was the ARC Implementation Center study (Sconiers et al., 2002), where three NSF-supported elementary curricula were examined, but in the results, their effects were pooled. ... but this is descriptive at ...
In eHealth evaluation, comparative studies aim to find out whether group differences in eHealth system adoption make a difference in important outcomes. These groups may differ in their composition, the type of system in use, and the setting where they work over a given time duration. The comparisons are to determine whether significant differences exist for some predefined measures between ...
A comparative study is a kind of method that analyzes phenomena and then put them together. to find the points of differentiation and similarity (MokhtarianPour, 2016). A comparative perspective ...
The goal of comparative research is drawing a solution out of the similarities and differences between the focused variables. Through non-experimental or qualitative research, you can include this type of research method in your comparative research design. 13+ Comparative Research Examples. Know more about comparative research by going over ...
Theoretical Reasoning. According to Sandelowski, 4 basic or fundamental qualitative description differs from other types of qualitative research, such as grounded theory, ethnography, phenomenology or narrative analysis, in the sense that it is — as the label suggests — essentially descriptive rather than interpretive in focus. This does not mean that a qualitative descriptive approach ...
A research title is a critical component of any research study or academic paper. It serves multiple important functions that contribute to the overall success and impact of the research. Here are key reasons why a research title is important: 1. First Impression. The research title is often the first element a reader encounters.
Causal-comparative research is a methodology used to identify cause-effect relationships between independent and dependent variables. Researchers can study cause and effect in retrospect. This can help determine the consequences or causes of differences already existing among or between different groups of people.
In a causal-comparative research design, the researcher compares two groups to find out whether the independent variable affected the outcome or the dependent variable. A causal-comparative method determines whether one variable has a direct influence on the other and why. It identifies the causes of certain occurrences (or non-occurrences).