

How to Create a Data Analysis Plan: A Detailed Guide
by Barche Blaise | Aug 12, 2020 | Writing

If a good research question equates to a story then, a roadmap will be very vita l for good storytelling. We advise every student/researcher to personally write his/her data analysis plan before seeking any advice. In this blog article, we will explore how to create a data analysis plan: the content and structure.
This data analysis plan serves as a roadmap to how data collected will be organised and analysed. It includes the following aspects:
- Clearly states the research objectives and hypothesis
- Identifies the dataset to be used
- Inclusion and exclusion criteria
- Clearly states the research variables
- States statistical test hypotheses and the software for statistical analysis
- Creating shell tables
1. Stating research question(s), objectives and hypotheses:
All research objectives or goals must be clearly stated. They must be Specific, Measurable, Attainable, Realistic and Time-bound (SMART). Hypotheses are theories obtained from personal experience or previous literature and they lay a foundation for the statistical methods that will be applied to extrapolate results to the entire population.
2. The dataset:
The dataset that will be used for statistical analysis must be described and important aspects of the dataset outlined. These include; owner of the dataset, how to get access to the dataset, how the dataset was checked for quality control and in what program is the dataset stored (Excel, Epi Info, SQL, Microsoft access etc.).
3. The inclusion and exclusion criteria :
They guide the aspects of the dataset that will be used for data analysis. These criteria will also guide the choice of variables included in the main analysis.
4. Variables:
Every variable collected in the study should be clearly stated. They should be presented based on the level of measurement (ordinal/nominal or ratio/interval levels), or the role the variable plays in the study (independent/predictors or dependent/outcome variables). The variable types should also be outlined. The variable type in conjunction with the research hypothesis forms the basis for selecting the appropriate statistical tests for inferential statistics. A good data analysis plan should summarize the variables as demonstrated in Figure 1 below.

5. Statistical software
There are tons of software packages for data analysis, some common examples are SPSS, Epi Info, SAS, STATA, Microsoft Excel. Include the version number, year of release and author/manufacturer. Beginners have the tendency to try different software and finally not master any. It is rather good to select one and master it because almost all statistical software have the same performance for basic and the majority of advance analysis needed for a student thesis. This is what we recommend to all our students at CRENC before they begin writing their results section .
6. Selecting the appropriate statistical method to test hypotheses
Depending on the research question, hypothesis and type of variable, several statistical methods can be used to answer the research question appropriately. This aspect of the data analysis plan outlines clearly why each statistical method will be used to test hypotheses. The level of statistical significance (p-value) which is often but not always <0.05 should also be written. Presented in figures 2a and 2b are decision trees for some common statistical tests based on the variable type and research question
A good analysis plan should clearly describe how missing data will be analysed.

7. Creating shell tables
Data analysis involves three levels of analysis; univariable, bivariable and multivariable analysis with increasing order of complexity. Shell tables should be created in anticipation for the results that will be obtained from these different levels of analysis. Read our blog article on how to present tables and figures for more details. Suppose you carry out a study to investigate the prevalence and associated factors of a certain disease “X” in a population, then the shell tables can be represented as in Tables 1, Table 2 and Table 3 below.
Table 1: Example of a shell table from univariate analysis

Table 2: Example of a shell table from bivariate analysis

Table 3: Example of a shell table from multivariate analysis

aOR = adjusted odds ratio
Now that you have learned how to create a data analysis plan, these are the takeaway points. It should clearly state the:
- Research question, objectives, and hypotheses
- Dataset to be used
- Variable types and their role
- Statistical software and statistical methods
- Shell tables for univariate, bivariate and multivariate analysis
Further readings
Creating a Data Analysis Plan: What to Consider When Choosing Statistics for a Study https://www.ncbi.nlm.nih.gov/pmc/articles/PMC4552232/pdf/cjhp-68-311.pdf
Creating an Analysis Plan: https://www.cdc.gov/globalhealth/healthprotection/fetp/training_modules/9/creating-analysis-plan_pw_final_09242013.pdf
Data Analysis Plan: https://www.statisticssolutions.com/dissertation-consulting-services/data-analysis-plan-2/
Photo created by freepik – www.freepik.com

Dr Barche is a physician and holds a Masters in Public Health. He is a senior fellow at CRENC with interests in Data Science and Data Analysis.
Post Navigation
16 comments.
Thanks. Quite informative.
Educative write-up. Thanks.
Easy to understand. Thanks Dr
Very explicit Dr. Thanks
I will always remember how you help me conceptualize and understand data science in a simple way. I can only hope that someday I’ll be in a position to repay you, my dear friend.
Plan d’analyse

This is interesting, Thanks
Very understandable and informative. Thank you..
love the figures.
Nice, and informative
This is so much educative and good for beginners, I would love to recommend that you create and share a video because some people are able to grasp when there is an instructor. Lots of love
Thank you Doctor very helpful.
Educative and clearly written. Thanks
Well said doctor,thank you.But when do you present in tables ,bars,pie chart etc?
Very informative guide!
Submit a Comment Cancel Reply
Your email address will not be published. Required fields are marked *
Notify me of follow-up comments by email.
Notify me of new posts by email.
Submit Comment
Receive updates on new courses and blog posts
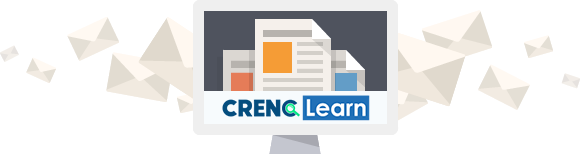
Never Miss a Thing!
Subscribe to our mailing list to receive the latest news and updates on our webinars, articles and courses.
You have Successfully Subscribed!
- Statistical Analysis Plan: What is it & How to Write One

- Data Collection
Statistics give meaning to data collected during research and make it simple to extract actionable insights from the data. As a result, it’s important to have a guide for analyzing data, which is where a statistical analysis plan (SAP) comes in.
A statistical analysis plan provides a framework for collecting data , simplifying and interpreting it , and assessing its reliability and validity.
Here’s a guide on what a statistical analysis plan is and how to write one.
What Is a Statistical Analysis Plan?
A statistical analysis plan (SAP) is a document that specifies the statistical analysis that will be performed on a given dataset. It serves as a comprehensive guide for the analysis, presenting a clear and organized approach to data analysis that ensures the reliability and validity of the results.
SAPs are most widely used in research, data science, and statistics. They are a necessary tool for clearly communicating the goals and methods of analysis, as well as documenting the decisions made during the analysis process.
SAPs typically outline the steps needed to prepare data for analysis, the methods to use, and how details such as sample size, data sources, and any assumptions or limitations of the analysis.
The first step in creating a statistical analysis plan is to identify the research question or hypothesis you’re testing.
Next, choose the appropriate statistical techniques for analyzing the data and specify the analysis details, such as sample size and data sources. It should also include the strategy for presenting and interpreting the results.
How to Develop a Statistical Analysis Plan
Here are the steps for creating a successful statistical analysis plan (SAP):
Identify the Research Question or Hypothesis
This is the main goal of the analysis, and it will guide the rest of the SAP. Here are the steps to identifying research questions or hypotheses:
Define the Analysis’s Goal
The research question or hypothesis should be related to the analysis’s main goal or purpose. If the goal is to evaluate the effectiveness of a content strategy, the research question could be “Is the new strategy more effective than the previous or standard strategy?”
Determine the Variables of Interest
Determine which variables are important to the research question or hypothesis. In the preceding example, the variables could include the effectiveness of the content strategy and its drawbacks.
Formulate the Question or Hypothesis
After identifying the variables, use them to research the question in a clear and precise way. For example, “is the new content strategy more effective than the current one in terms of user acquisition?
Check for Clarity and Specificity
Review the research question or hypothesis for precision and clarity. If a question isn’t well-structured enough to be tested with the data and resources at hand, revise it.
Determine the Sample Size
The main factors that influence the sample size are the type of data being analyzed and the resources available. For example, if the data is continuous, you’ll probably need a large sample size.
Also, your sample size should be tailored to your available resources, time, and budget. You could also calculate the sample size using a sample size formula or software.
Select the Appropriate Statistical Techniques
Choose the most appropriate statistical techniques for the analysis based on the research question, data type, and sample size.
Specify the Details of the Analysis
This includes the data sources, any analysis assumptions or limitations, and any variables that need modifications.
Plan For Presenting and Interpreting the Results
Plan how the results will be interpreted and communicated to your audience. Choose how you want to present the information, such as a report or a presentation.
Identifying the Need for a Statistical Analysis Plan
Here are some real-world examples of where a statistical analysis plan is needed:
Research Studies
Health researchers need SAP to determine the effectiveness of a new drug in treating a specific medical condition. It also outlines the methods and procedures for analyzing the study’s data, including sample size, data sources, and statistical techniques to be used.
Clinic Trials
Clinical trials help to test the safety and efficacy of new medical treatments, which would necessitate gathering a large amount of data on how patients respond to treatment, side effects, and comparisons to existing treatments.
A clinic trial SAP should emphasize the statistical analysis that will be performed on the trial data, such as sample size, data sources, and statistical techniques to be used.
Data-Driven Projects
SAP is used by marketing research firms to outline the statistical analysis that will be performed on market research data. It specifies the sample size, data sources, and statistical techniques that will be used to analyze data and provide insights into consumer behavior.
Government Agencies
When government agencies collect data for new policies such as new tax laws or population censuses, they require a statistical analysis plan outlining how the data will be collected, interpreted, and used. The SAP would specify the sample size, data sources, and statistical techniques that will be used to analyze the data and assess the effectiveness of the policy or program.
Nonprofit Organizations
Nonprofits could also use SAPs to analyze data collected as part of a research study or program evaluation. A non-profit, for example, could gather information about who is likely to donate to their cause and how to contact them to solicit donations.
How Do You Write a Statistical Analysis Plan?
Here are the steps to writing a simple and effective Statistical analysis plan:
Introduction
A statistical analysis plan (SAP) introduction should provide an overview of the research question or hypothesis being tested as well as the goals and objectives of the analysis. It should also provide some context for the topic and the context in which the analysis is being conducted.
This section should describe how the data was collected and prepared for analysis, including sample size, data sources, and any analysis assumptions or limitations.
For example, a clinical trial involving 100 patients with a specific medical condition. The sample will be assigned at random to either the new or current standard treatment.
The SAP will include data on the treatment’s effectiveness in reducing symptoms, which will be collected at the start of the trial and at regular intervals throughout and after it. To avoid common survey bias, data is collected using standardized questionnaires created by researchers.
Next, the data will be cleaned and prepared for analysis by removing any missing or invalid values and ensuring that it is in the correct format. Also, any data collected outside of the specified time frame will be excluded from the analysis.
The small sample size and brief duration of the clinical trial are two of the study’s limitations. These constraints should be considered when interpreting the results of this analysis.
Statistical Techniques
This section should describe the statistical techniques that will be used in the analysis, including any specific software or tools.
Using the preceding example, you can use software such as SPSS or R. They use t-tests and regression analysis to determine the effectiveness of the two treatments.
You can make further investigations using additional statistical techniques such as ANOVA. It enables you to investigate the effects of various variables on treatment efficacy and identify any significant inter-variable interactions.
This section describes how the results will be presented and interpreted, including any plans for visualizing the data or using statistical tests to determine their significance.
Using the clinical trial example, you can visualize the data and find patterns in the data by using graphical representations. Next, interpret the result in light of the research question or hypothesis, as well as any limitations or assumptions of the analysis.
Assess the implications of the clinical trial results and future research on the medical condition’s treatment. Then, develop a summary of the results including any recommendations or conclusions drawn from the research.
The “Conclusion” section should provide a concise summary of the main findings of the analysis as well as any recommendations or implications. It should also highlight any limitations or assumptions of the analysis and discuss the implications of the results for clinical practice and future research.
Information in the Statistical Analysis Plan
1. Statistics on who wrote the SAP, when it was approved, and who signed it.
2. Expected number of participants, and sample size calculation.
3. A detailed explanation of the main and short-term analysis techniques used for analyzing the data. This includes:
- Study goals
- Specify the primary and secondary hypotheses, as well as the parameters you’ll use to assess how well you met the study objectives.
- A detailed description of the study’s sample size.
- A summary of the primary and secondary outcomes of each study. Typically, there should be just one primary outcome.
4. The SAP should also specify how each outcome metric will be assessed. Statistical tests are typically used to examine outcome measures and the method for accounting for missing data.
5. The SAP should also explain the procedures used to analyze and display the study results in detail. This includes:
- The level of statistical significance that will be used, and if one-tailed or two-tailed tests will be used.
- How to deal with missing data.
- Outlier management techniques.
- Protocol variations, noncompliance, and withdrawal procedures.
- Estimation methods for points and intervals.
- How to calculate composite or derived variables, including data-driven definitions and any additional details needed to reduce uncertainties.
- Baseline and covariate data
- Add randomization factors
- Methods for dealing with data from multiple sources
- How to deal with participant interactions
- Multiple comparisons and subgroup analysis methods
- Interim or sequential analyses
- Step-by-step procedure to terminate research and its implications
- Statistical software for analyzing the data
- Validate critical analysis assumptions and sensitivity analyses.
- Visual representation of the research data
- Define the safe population
6. Alternative models for data analysis if the data does not fit the chosen statistical model
Making Modifications to Statistical Analysis Plan
It is not unusual for a statistical analysis plan (SAP) to undergo adjustments during the project’s life cycle. Here’s why you may need to modify your SAP:
- Research question or hypothesis change : As the project progresses, the research question or hypothesis may evolve or change, requiring changes to the SAP.
- New data : As new data is collected or becomes available, it may be necessary to modify the SAP to include the new information.
- Unpredicted challenges : Unexpected challenges may arise during the project, requiring SAP alteration. For example, the data may not be of the expected quality, or the sample size may need to be adjusted.
- Improved Data Understanding : The researcher may gain a better understanding of the data as the analysis progresses and may need to modify the SAP to reflect this enhanced understanding.
Make sure to document the changes made to the SAP, as well as the reasons for them. This ensures the analysis’s reliability and accuracy.
You could also work with a statistician or research expert to ensure that the SAP changes are appropriate and do not jeopardize the results’ reliability and validity.
A statistical analysis plan (SAP) is a step-by-step plan that highlights the methods and techniques to be used in data analysis for a research project. SAPs ensure the reliability and validity of the results and provide a clear roadmap for the analysis.
You have to include the research question or hypothesis, sample size, data sources, statistical techniques, variables, and guidelines for interpreting and presenting the results to have an effective SAP.
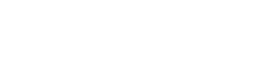
Connect to Formplus, Get Started Now - It's Free!
- data analysis
- statistical analysis
- statistical analysis plan
- Moradeke Owa
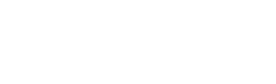
You may also like:
What is Field Research: Meaning, Examples, Pros & Cons
Introduction Field research is a method of research that deals with understanding and interpreting the social interactions of groups of...

What Are Research Repositories?
A research repository is a database that helps organizations to manage, share, and gain access to research data to make product and...
Unit of Analysis: Definition, Types & Examples
Introduction A unit of analysis is the smallest level of analysis for a research project. It’s important to choose the right unit of...
Statistical Analysis Software: A Guide For Social Researchers
Introduction Social research is a complex endeavor. It takes a lot of time, energy, and resources to gather data, analyze and present...
Formplus - For Seamless Data Collection
Collect data the right way with a versatile data collection tool. try formplus and transform your work productivity today..
Customize Your Path
Filters Applied
Customize Your Experience.
Utilize the "Customize Your Path" feature to refine the information displayed in myRESEARCHpath based on your role, project inclusions, sponsor or funding, and management center.
Design the analysis plan
Need assistance with analysis planning?
Get help with analysis planning.
Contact the Biostatistics, Epidemiology, and Research Design (BERD) Methods Core:
- Submit a help request
Writing the Data Analysis Plan
- First Online: 01 January 2010
Cite this chapter
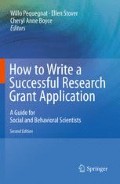
- A. T. Panter 4
5777 Accesses
3 Altmetric
You and your project statistician have one major goal for your data analysis plan: You need to convince all the reviewers reading your proposal that you would know what to do with your data once your project is funded and your data are in hand. The data analytic plan is a signal to the reviewers about your ability to score, describe, and thoughtfully synthesize a large number of variables into appropriately-selected quantitative models once the data are collected. Reviewers respond very well to plans with a clear elucidation of the data analysis steps – in an appropriate order, with an appropriate level of detail and reference to relevant literatures, and with statistical models and methods for that map well into your proposed aims. A successful data analysis plan produces reviews that either include no comments about the data analysis plan or better yet, compliments it for being comprehensive and logical given your aims. This chapter offers practical advice about developing and writing a compelling, “bullet-proof” data analytic plan for your grant application.
This is a preview of subscription content, log in via an institution to check access.
Access this chapter
- Available as PDF
- Read on any device
- Instant download
- Own it forever
- Available as EPUB and PDF
- Compact, lightweight edition
- Dispatched in 3 to 5 business days
- Free shipping worldwide - see info
Tax calculation will be finalised at checkout
Purchases are for personal use only
Institutional subscriptions
Aiken, L. S. & West, S. G. (1991). Multiple regression: testing and interpreting interactions . Newbury Park, CA: Sage.
Google Scholar
Aiken, L. S., West, S. G., & Millsap, R. E. (2008). Doctoral training in statistics, measurement, and methodology in psychology: Replication and extension of Aiken, West, Sechrest and Reno’s (1990) survey of PhD programs in North America. American Psychologist , 63 , 32–50.
Article PubMed Google Scholar
Allison, P. D. (2003). Missing data techniques for structural equation modeling. Journal of Abnormal Psychology , 112 , 545–557.
American Psychological Association (APA) Task Force to Increase the Quantitative Pipeline (2009). Report of the task force to increase the quantitative pipeline . Washington, DC: American Psychological Association.
Bauer, D. & Curran, P. J. (2004). The integration of continuous and discrete latent variables: Potential problems and promising opportunities. Psychological Methods , 9 , 3–29.
Bollen, K. A. (1989). Structural equations with latent variables . New York: Wiley.
Bollen, K. A. & Curran, P. J. (2007). Latent curve models: A structural equation modeling approach . New York: Wiley.
Cohen, J., Cohen, P., West, S. G., & Aiken, L. S. (2003). Multiple correlation/regression for the behavioral sciences (3rd ed.). Mahwah, NJ: Erlbaum.
Curran, P. J., Bauer, D. J., & Willoughby, M. T. (2004). Testing main effects and interactions in hierarchical linear growth models. Psychological Methods , 9 , 220–237.
Embretson, S. E. & Reise, S. P. (2000). Item response theory for psychologists . Mahwah, NJ: Erlbaum.
Enders, C. K. (2006). Analyzing structural equation models with missing data. In G. R. Hancock & R. O. Mueller (Eds.), Structural equation modeling: A second course (pp. 313–342). Greenwich, CT: Information Age.
Hosmer, D. & Lemeshow, S. (1989). Applied logistic regression . New York: Wiley.
Hoyle, R. H. & Panter, A. T. (1995). Writing about structural equation models. In R. H. Hoyle (Ed.), Structural equation modeling: Concepts, issues, and applications (pp. 158–176). Thousand Oaks: Sage.
Kaplan, D. & Elliott, P. R. (1997). A didactic example of multilevel structural equation modeling applicable to the study of organizations. Structural Equation Modeling , 4 , 1–23.
Article Google Scholar
Lanza, S. T., Collins, L. M., Schafer, J. L., & Flaherty, B. P. (2005). Using data augmentation to obtain standard errors and conduct hypothesis tests in latent class and latent transition analysis. Psychological Methods , 10 , 84–100.
MacKinnon, D. P. (2008). Introduction to statistical mediation analysis . Mahwah, NJ: Erlbaum.
Maxwell, S. E. (2004). The persistence of underpowered studies in psychological research: Causes, consequences, and remedies. Psychological Methods , 9 , 147–163.
McCullagh, P. & Nelder, J. (1989). Generalized linear models . London: Chapman and Hall.
McDonald, R. P. & Ho, M. R. (2002). Principles and practices in reporting structural equation modeling analyses. Psychological Methods , 7 , 64–82.
Messick, S. (1989). Validity. In R. L. Linn (Ed.), Educational measurement (3rd ed., pp. 13–103). New York: Macmillan.
Muthén, B. O. (1994). Multilevel covariance structure analysis. Sociological Methods & Research , 22 , 376–398.
Muthén, B. (2008). Latent variable hybrids: overview of old and new models. In G. R. Hancock & K. M. Samuelsen (Eds.), Advances in latent variable mixture models (pp. 1–24). Charlotte, NC: Information Age.
Muthén, B. & Masyn, K. (2004). Discrete-time survival mixture analysis. Journal of Educational and Behavioral Statistics , 30 , 27–58.
Muthén, L. K. & Muthén, B. O. (2004). Mplus, statistical analysis with latent variables: User’s guide . Los Angeles, CA: Muthén &Muthén.
Peugh, J. L. & Enders, C. K. (2004). Missing data in educational research: a review of reporting practices and suggestions for improvement. Review of Educational Research , 74 , 525–556.
Preacher, K. J., Curran, P. J., & Bauer, D. J. (2006). Computational tools for probing interaction effects in multiple linear regression, multilevel modeling, and latent curve analysis. Journal of Educational and Behavioral Statistics , 31 , 437–448.
Preacher, K. J., Curran, P. J., & Bauer, D. J. (2003, September). Probing interactions in multiple linear regression, latent curve analysis, and hierarchical linear modeling: Interactive calculation tools for establishing simple intercepts, simple slopes, and regions of significance [Computer software]. Available from http://www.quantpsy.org .
Preacher, K. J., Rucker, D. D., & Hayes, A. F. (2007). Addressing moderated mediation hypotheses: Theory, methods, and prescriptions. Multivariate Behavioral Research , 42 , 185–227.
Raudenbush, S. W. & Bryk, A. S. (2002). Hierarchical linear models: Applications and data analysis methods (2nd ed.). Thousand Oaks, CA: Sage.
Radloff, L. (1977). The CES-D scale: A self-report depression scale for research in the general population. Applied Psychological Measurement , 1 , 385–401.
Rosenberg, M. (1965). Society and the adolescent self-image . Princeton, NJ: Princeton University Press.
Schafer. J. L. & Graham, J. W. (2002). Missing data: Our view of the state of the art. Psychological Methods , 7 , 147–177.
Schumacker, R. E. (2002). Latent variable interaction modeling. Structural Equation Modeling , 9 , 40–54.
Schumacker, R. E. & Lomax, R. G. (2004). A beginner’s guide to structural equation modeling . Mahwah, NJ: Erlbaum.
Selig, J. P. & Preacher, K. J. (2008, June). Monte Carlo method for assessing mediation: An interactive tool for creating confidence intervals for indirect effects [Computer software]. Available from http://www.quantpsy.org .
Singer, J. D. & Willett, J. B. (1991). Modeling the days of our lives: Using survival analysis when designing and analyzing longitudinal studies of duration and the timing of events. Psychological Bulletin , 110 , 268–290.
Singer, J. D. & Willett, J. B. (1993). It’s about time: Using discrete-time survival analysis to study duration and the timing of events. Journal of Educational Statistics , 18 , 155–195.
Singer, J. D. & Willett, J. B. (2003). Applied longitudinal data analysis: Modeling change and event occurrence . New York: Oxford University.
Book Google Scholar
Vandenberg, R. J. & Lance, C. E. (2000). A review and synthesis of the measurement invariance literature: Suggestions, practices, and recommendations for organizational research. Organizational Research Methods , 3 , 4–69.
Wirth, R. J. & Edwards, M. C. (2007). Item factor analysis: Current approaches and future directions. Psychological Methods , 12 , 58–79.
Article PubMed CAS Google Scholar
Download references
Author information
Authors and affiliations.
L. L. Thurstone Psychometric Laboratory, Department of Psychology, University of North Carolina, Chapel Hill, NC, USA
A. T. Panter
You can also search for this author in PubMed Google Scholar
Corresponding author
Correspondence to A. T. Panter .
Editor information
Editors and affiliations.
National Institute of Mental Health, Executive Blvd. 6001, Bethesda, 20892-9641, Maryland, USA
Willo Pequegnat
Ellen Stover
Delafield Place, N.W. 1413, Washington, 20011, District of Columbia, USA
Cheryl Anne Boyce
Rights and permissions
Reprints and permissions
Copyright information
© 2010 Springer Science+Business Media, LLC
About this chapter
Panter, A.T. (2010). Writing the Data Analysis Plan. In: Pequegnat, W., Stover, E., Boyce, C. (eds) How to Write a Successful Research Grant Application. Springer, Boston, MA. https://doi.org/10.1007/978-1-4419-1454-5_22
Download citation
DOI : https://doi.org/10.1007/978-1-4419-1454-5_22
Published : 20 August 2010
Publisher Name : Springer, Boston, MA
Print ISBN : 978-1-4419-1453-8
Online ISBN : 978-1-4419-1454-5
eBook Packages : Medicine Medicine (R0)

Share this chapter
Anyone you share the following link with will be able to read this content:
Sorry, a shareable link is not currently available for this article.
Provided by the Springer Nature SharedIt content-sharing initiative
- Publish with us
Policies and ethics
- Find a journal
- Track your research

An official website of the United States government
The .gov means it’s official. Federal government websites often end in .gov or .mil. Before sharing sensitive information, make sure you’re on a federal government site.
The site is secure. The https:// ensures that you are connecting to the official website and that any information you provide is encrypted and transmitted securely.
- Publications
- Account settings
Preview improvements coming to the PMC website in October 2024. Learn More or Try it out now .
- Advanced Search
- Journal List
- HCA Healthc J Med
- v.1(2); 2020
- PMC10324782

Introduction to Research Statistical Analysis: An Overview of the Basics
Christian vandever.
1 HCA Healthcare Graduate Medical Education
Description
This article covers many statistical ideas essential to research statistical analysis. Sample size is explained through the concepts of statistical significance level and power. Variable types and definitions are included to clarify necessities for how the analysis will be interpreted. Categorical and quantitative variable types are defined, as well as response and predictor variables. Statistical tests described include t-tests, ANOVA and chi-square tests. Multiple regression is also explored for both logistic and linear regression. Finally, the most common statistics produced by these methods are explored.
Introduction
Statistical analysis is necessary for any research project seeking to make quantitative conclusions. The following is a primer for research-based statistical analysis. It is intended to be a high-level overview of appropriate statistical testing, while not diving too deep into any specific methodology. Some of the information is more applicable to retrospective projects, where analysis is performed on data that has already been collected, but most of it will be suitable to any type of research. This primer will help the reader understand research results in coordination with a statistician, not to perform the actual analysis. Analysis is commonly performed using statistical programming software such as R, SAS or SPSS. These allow for analysis to be replicated while minimizing the risk for an error. Resources are listed later for those working on analysis without a statistician.
After coming up with a hypothesis for a study, including any variables to be used, one of the first steps is to think about the patient population to apply the question. Results are only relevant to the population that the underlying data represents. Since it is impractical to include everyone with a certain condition, a subset of the population of interest should be taken. This subset should be large enough to have power, which means there is enough data to deliver significant results and accurately reflect the study’s population.
The first statistics of interest are related to significance level and power, alpha and beta. Alpha (α) is the significance level and probability of a type I error, the rejection of the null hypothesis when it is true. The null hypothesis is generally that there is no difference between the groups compared. A type I error is also known as a false positive. An example would be an analysis that finds one medication statistically better than another, when in reality there is no difference in efficacy between the two. Beta (β) is the probability of a type II error, the failure to reject the null hypothesis when it is actually false. A type II error is also known as a false negative. This occurs when the analysis finds there is no difference in two medications when in reality one works better than the other. Power is defined as 1-β and should be calculated prior to running any sort of statistical testing. Ideally, alpha should be as small as possible while power should be as large as possible. Power generally increases with a larger sample size, but so does cost and the effect of any bias in the study design. Additionally, as the sample size gets bigger, the chance for a statistically significant result goes up even though these results can be small differences that do not matter practically. Power calculators include the magnitude of the effect in order to combat the potential for exaggeration and only give significant results that have an actual impact. The calculators take inputs like the mean, effect size and desired power, and output the required minimum sample size for analysis. Effect size is calculated using statistical information on the variables of interest. If that information is not available, most tests have commonly used values for small, medium or large effect sizes.
When the desired patient population is decided, the next step is to define the variables previously chosen to be included. Variables come in different types that determine which statistical methods are appropriate and useful. One way variables can be split is into categorical and quantitative variables. ( Table 1 ) Categorical variables place patients into groups, such as gender, race and smoking status. Quantitative variables measure or count some quantity of interest. Common quantitative variables in research include age and weight. An important note is that there can often be a choice for whether to treat a variable as quantitative or categorical. For example, in a study looking at body mass index (BMI), BMI could be defined as a quantitative variable or as a categorical variable, with each patient’s BMI listed as a category (underweight, normal, overweight, and obese) rather than the discrete value. The decision whether a variable is quantitative or categorical will affect what conclusions can be made when interpreting results from statistical tests. Keep in mind that since quantitative variables are treated on a continuous scale it would be inappropriate to transform a variable like which medication was given into a quantitative variable with values 1, 2 and 3.
Categorical vs. Quantitative Variables
Both of these types of variables can also be split into response and predictor variables. ( Table 2 ) Predictor variables are explanatory, or independent, variables that help explain changes in a response variable. Conversely, response variables are outcome, or dependent, variables whose changes can be partially explained by the predictor variables.
Response vs. Predictor Variables
Choosing the correct statistical test depends on the types of variables defined and the question being answered. The appropriate test is determined by the variables being compared. Some common statistical tests include t-tests, ANOVA and chi-square tests.
T-tests compare whether there are differences in a quantitative variable between two values of a categorical variable. For example, a t-test could be useful to compare the length of stay for knee replacement surgery patients between those that took apixaban and those that took rivaroxaban. A t-test could examine whether there is a statistically significant difference in the length of stay between the two groups. The t-test will output a p-value, a number between zero and one, which represents the probability that the two groups could be as different as they are in the data, if they were actually the same. A value closer to zero suggests that the difference, in this case for length of stay, is more statistically significant than a number closer to one. Prior to collecting the data, set a significance level, the previously defined alpha. Alpha is typically set at 0.05, but is commonly reduced in order to limit the chance of a type I error, or false positive. Going back to the example above, if alpha is set at 0.05 and the analysis gives a p-value of 0.039, then a statistically significant difference in length of stay is observed between apixaban and rivaroxaban patients. If the analysis gives a p-value of 0.91, then there was no statistical evidence of a difference in length of stay between the two medications. Other statistical summaries or methods examine how big of a difference that might be. These other summaries are known as post-hoc analysis since they are performed after the original test to provide additional context to the results.
Analysis of variance, or ANOVA, tests can observe mean differences in a quantitative variable between values of a categorical variable, typically with three or more values to distinguish from a t-test. ANOVA could add patients given dabigatran to the previous population and evaluate whether the length of stay was significantly different across the three medications. If the p-value is lower than the designated significance level then the hypothesis that length of stay was the same across the three medications is rejected. Summaries and post-hoc tests also could be performed to look at the differences between length of stay and which individual medications may have observed statistically significant differences in length of stay from the other medications. A chi-square test examines the association between two categorical variables. An example would be to consider whether the rate of having a post-operative bleed is the same across patients provided with apixaban, rivaroxaban and dabigatran. A chi-square test can compute a p-value determining whether the bleeding rates were significantly different or not. Post-hoc tests could then give the bleeding rate for each medication, as well as a breakdown as to which specific medications may have a significantly different bleeding rate from each other.
A slightly more advanced way of examining a question can come through multiple regression. Regression allows more predictor variables to be analyzed and can act as a control when looking at associations between variables. Common control variables are age, sex and any comorbidities likely to affect the outcome variable that are not closely related to the other explanatory variables. Control variables can be especially important in reducing the effect of bias in a retrospective population. Since retrospective data was not built with the research question in mind, it is important to eliminate threats to the validity of the analysis. Testing that controls for confounding variables, such as regression, is often more valuable with retrospective data because it can ease these concerns. The two main types of regression are linear and logistic. Linear regression is used to predict differences in a quantitative, continuous response variable, such as length of stay. Logistic regression predicts differences in a dichotomous, categorical response variable, such as 90-day readmission. So whether the outcome variable is categorical or quantitative, regression can be appropriate. An example for each of these types could be found in two similar cases. For both examples define the predictor variables as age, gender and anticoagulant usage. In the first, use the predictor variables in a linear regression to evaluate their individual effects on length of stay, a quantitative variable. For the second, use the same predictor variables in a logistic regression to evaluate their individual effects on whether the patient had a 90-day readmission, a dichotomous categorical variable. Analysis can compute a p-value for each included predictor variable to determine whether they are significantly associated. The statistical tests in this article generate an associated test statistic which determines the probability the results could be acquired given that there is no association between the compared variables. These results often come with coefficients which can give the degree of the association and the degree to which one variable changes with another. Most tests, including all listed in this article, also have confidence intervals, which give a range for the correlation with a specified level of confidence. Even if these tests do not give statistically significant results, the results are still important. Not reporting statistically insignificant findings creates a bias in research. Ideas can be repeated enough times that eventually statistically significant results are reached, even though there is no true significance. In some cases with very large sample sizes, p-values will almost always be significant. In this case the effect size is critical as even the smallest, meaningless differences can be found to be statistically significant.
These variables and tests are just some things to keep in mind before, during and after the analysis process in order to make sure that the statistical reports are supporting the questions being answered. The patient population, types of variables and statistical tests are all important things to consider in the process of statistical analysis. Any results are only as useful as the process used to obtain them. This primer can be used as a reference to help ensure appropriate statistical analysis.
Funding Statement
This research was supported (in whole or in part) by HCA Healthcare and/or an HCA Healthcare affiliated entity.
Conflicts of Interest
The author declares he has no conflicts of interest.
Christian Vandever is an employee of HCA Healthcare Graduate Medical Education, an organization affiliated with the journal’s publisher.
This research was supported (in whole or in part) by HCA Healthcare and/or an HCA Healthcare affiliated entity. The views expressed in this publication represent those of the author(s) and do not necessarily represent the official views of HCA Healthcare or any of its affiliated entities.
What Is Statistical Analysis?

Statistical analysis is a technique we use to find patterns in data and make inferences about those patterns to describe variability in the results of a data set or an experiment.
In its simplest form, statistical analysis answers questions about:
- Quantification — how big/small/tall/wide is it?
- Variability — growth, increase, decline
- The confidence level of these variabilities
What Are the 2 Types of Statistical Analysis?
- Descriptive Statistics: Descriptive statistical analysis describes the quality of the data by summarizing large data sets into single measures.
- Inferential Statistics: Inferential statistical analysis allows you to draw conclusions from your sample data set and make predictions about a population using statistical tests.
What’s the Purpose of Statistical Analysis?
Using statistical analysis, you can determine trends in the data by calculating your data set’s mean or median. You can also analyze the variation between different data points from the mean to get the standard deviation . Furthermore, to test the validity of your statistical analysis conclusions, you can use hypothesis testing techniques, like P-value, to determine the likelihood that the observed variability could have occurred by chance.
More From Abdishakur Hassan The 7 Best Thematic Map Types for Geospatial Data
Statistical Analysis Methods
There are two major types of statistical data analysis: descriptive and inferential.
Descriptive Statistical Analysis
Descriptive statistical analysis describes the quality of the data by summarizing large data sets into single measures.
Within the descriptive analysis branch, there are two main types: measures of central tendency (i.e. mean, median and mode) and measures of dispersion or variation (i.e. variance , standard deviation and range).
For example, you can calculate the average exam results in a class using central tendency or, in particular, the mean. In that case, you’d sum all student results and divide by the number of tests. You can also calculate the data set’s spread by calculating the variance. To calculate the variance, subtract each exam result in the data set from the mean, square the answer, add everything together and divide by the number of tests.
Inferential Statistics
On the other hand, inferential statistical analysis allows you to draw conclusions from your sample data set and make predictions about a population using statistical tests.
There are two main types of inferential statistical analysis: hypothesis testing and regression analysis. We use hypothesis testing to test and validate assumptions in order to draw conclusions about a population from the sample data. Popular tests include Z-test, F-Test, ANOVA test and confidence intervals . On the other hand, regression analysis primarily estimates the relationship between a dependent variable and one or more independent variables. There are numerous types of regression analysis but the most popular ones include linear and logistic regression .
Statistical Analysis Steps
In the era of big data and data science, there is a rising demand for a more problem-driven approach. As a result, we must approach statistical analysis holistically. We may divide the entire process into five different and significant stages by using the well-known PPDAC model of statistics: Problem, Plan, Data, Analysis and Conclusion.
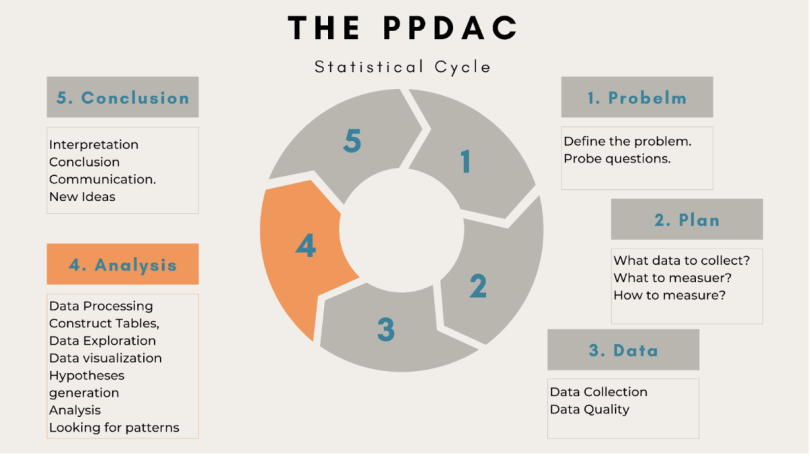
In the first stage, you define the problem you want to tackle and explore questions about the problem.
Next is the planning phase. You can check whether data is available or if you need to collect data for your problem. You also determine what to measure and how to measure it.
The third stage involves data collection, understanding the data and checking its quality.
4. Analysis
Statistical data analysis is the fourth stage. Here you process and explore the data with the help of tables, graphs and other data visualizations. You also develop and scrutinize your hypothesis in this stage of analysis.
5. Conclusion
The final step involves interpretations and conclusions from your analysis. It also covers generating new ideas for the next iteration. Thus, statistical analysis is not a one-time event but an iterative process.
Statistical Analysis Uses
Statistical analysis is useful for research and decision making because it allows us to understand the world around us and draw conclusions by testing our assumptions. Statistical analysis is important for various applications, including:
- Statistical quality control and analysis in product development
- Clinical trials
- Customer satisfaction surveys and customer experience research
- Marketing operations management
- Process improvement and optimization
- Training needs
More on Statistical Analysis From Built In Experts Intro to Descriptive Statistics for Machine Learning
Benefits of Statistical Analysis
Here are some of the reasons why statistical analysis is widespread in many applications and why it’s necessary:
Understand Data
Statistical analysis gives you a better understanding of the data and what they mean. These types of analyses provide information that would otherwise be difficult to obtain by merely looking at the numbers without considering their relationship.
Find Causal Relationships
Statistical analysis can help you investigate causation or establish the precise meaning of an experiment, like when you’re looking for a relationship between two variables.
Make Data-Informed Decisions
Businesses are constantly looking to find ways to improve their services and products . Statistical analysis allows you to make data-informed decisions about your business or future actions by helping you identify trends in your data, whether positive or negative.
Determine Probability
Statistical analysis is an approach to understanding how the probability of certain events affects the outcome of an experiment. It helps scientists and engineers decide how much confidence they can have in the results of their research, how to interpret their data and what questions they can feasibly answer.
You’ve Got Questions. Our Experts Have Answers. Confidence Intervals, Explained!
What Are the Risks of Statistical Analysis?
Statistical analysis can be valuable and effective, but it’s an imperfect approach. Even if the analyst or researcher performs a thorough statistical analysis, there may still be known or unknown problems that can affect the results. Therefore, statistical analysis is not a one-size-fits-all process. If you want to get good results, you need to know what you’re doing. It can take a lot of time to figure out which type of statistical analysis will work best for your situation .
Thus, you should remember that our conclusions drawn from statistical analysis don’t always guarantee correct results. This can be dangerous when making business decisions. In marketing , for example, we may come to the wrong conclusion about a product . Therefore, the conclusions we draw from statistical data analysis are often approximated; testing for all factors affecting an observation is impossible.
Built In’s expert contributor network publishes thoughtful, solutions-oriented stories written by innovative tech professionals. It is the tech industry’s definitive destination for sharing compelling, first-person accounts of problem-solving on the road to innovation.
Great Companies Need Great People. That's Where We Come In.
- Introduction
- Conclusions
- Article Information
eTable 1. Identification of Existing Guidance on the Content of Statistical Analysis Plans.
eTable 2. Consensus Criteria.
eTable 3. Consensus Meeting Contributors and the Areas of Representation.
eTable 4. Items That While Important When Implementing a SAP Do Not Necessarily Need to be Included.
eAppendix 1. Survey of UK Clinical Research Collaborative Registered Clinical Trials Units.
eAppendix 2. Explanation and Elaboration of Essential Items.
eReferences.
- Guidelines for Statistical Analysis Plans JAMA Editorial December 19, 2017 David L. DeMets, PhD; Thomas D. Cook, PhD; Kevin A. Buhr, PhD
- Statistical Analysis Plans for Clinical Trials JAMA Comment & Response May 8, 2018 Bruno Mario Cesana, MD
- Statistical Analysis Plans for Clinical Trials—Reply JAMA Comment & Response May 8, 2018 Carrol Gamble, PhD; Steff Lewis, PhD; Stephen Senn, PhD
See More About
Select your interests.
Customize your JAMA Network experience by selecting one or more topics from the list below.
- Academic Medicine
- Acid Base, Electrolytes, Fluids
- Allergy and Clinical Immunology
- American Indian or Alaska Natives
- Anesthesiology
- Anticoagulation
- Art and Images in Psychiatry
- Artificial Intelligence
- Assisted Reproduction
- Bleeding and Transfusion
- Caring for the Critically Ill Patient
- Challenges in Clinical Electrocardiography
- Climate and Health
- Climate Change
- Clinical Challenge
- Clinical Decision Support
- Clinical Implications of Basic Neuroscience
- Clinical Pharmacy and Pharmacology
- Complementary and Alternative Medicine
- Consensus Statements
- Coronavirus (COVID-19)
- Critical Care Medicine
- Cultural Competency
- Dental Medicine
- Dermatology
- Diabetes and Endocrinology
- Diagnostic Test Interpretation
- Drug Development
- Electronic Health Records
- Emergency Medicine
- End of Life, Hospice, Palliative Care
- Environmental Health
- Equity, Diversity, and Inclusion
- Facial Plastic Surgery
- Gastroenterology and Hepatology
- Genetics and Genomics
- Genomics and Precision Health
- Global Health
- Guide to Statistics and Methods
- Hair Disorders
- Health Care Delivery Models
- Health Care Economics, Insurance, Payment
- Health Care Quality
- Health Care Reform
- Health Care Safety
- Health Care Workforce
- Health Disparities
- Health Inequities
- Health Policy
- Health Systems Science
- History of Medicine
- Hypertension
- Images in Neurology
- Implementation Science
- Infectious Diseases
- Innovations in Health Care Delivery
- JAMA Infographic
- Law and Medicine
- Leading Change
- Less is More
- LGBTQIA Medicine
- Lifestyle Behaviors
- Medical Coding
- Medical Devices and Equipment
- Medical Education
- Medical Education and Training
- Medical Journals and Publishing
- Mobile Health and Telemedicine
- Narrative Medicine
- Neuroscience and Psychiatry
- Notable Notes
- Nutrition, Obesity, Exercise
- Obstetrics and Gynecology
- Occupational Health
- Ophthalmology
- Orthopedics
- Otolaryngology
- Pain Medicine
- Palliative Care
- Pathology and Laboratory Medicine
- Patient Care
- Patient Information
- Performance Improvement
- Performance Measures
- Perioperative Care and Consultation
- Pharmacoeconomics
- Pharmacoepidemiology
- Pharmacogenetics
- Pharmacy and Clinical Pharmacology
- Physical Medicine and Rehabilitation
- Physical Therapy
- Physician Leadership
- Population Health
- Primary Care
- Professional Well-being
- Professionalism
- Psychiatry and Behavioral Health
- Public Health
- Pulmonary Medicine
- Regulatory Agencies
- Reproductive Health
- Research, Methods, Statistics
- Resuscitation
- Rheumatology
- Risk Management
- Scientific Discovery and the Future of Medicine
- Shared Decision Making and Communication
- Sleep Medicine
- Sports Medicine
- Stem Cell Transplantation
- Substance Use and Addiction Medicine
- Surgical Innovation
- Surgical Pearls
- Teachable Moment
- Technology and Finance
- The Art of JAMA
- The Arts and Medicine
- The Rational Clinical Examination
- Tobacco and e-Cigarettes
- Translational Medicine
- Trauma and Injury
- Treatment Adherence
- Ultrasonography
- Users' Guide to the Medical Literature
- Vaccination
- Venous Thromboembolism
- Veterans Health
- Women's Health
- Workflow and Process
- Wound Care, Infection, Healing
Others Also Liked
- Download PDF
- X Facebook More LinkedIn
Gamble C , Krishan A , Stocken D, et al. Guidelines for the Content of Statistical Analysis Plans in Clinical Trials. JAMA. 2017;318(23):2337–2343. doi:10.1001/jama.2017.18556
Manage citations:
© 2024
- Permissions
Guidelines for the Content of Statistical Analysis Plans in Clinical Trials
- 1 Biostatistics Department, University of Liverpool, Liverpool, England
- 2 Clinical Trials Research Centre, University of Liverpool, Liverpool, England
- 3 Newcastle University, Newcastle, England
- 4 Currently with Leeds Institute of Clinical Trials Research, University of Leeds, Leeds, England
- 5 Edinburgh University, Edinburgh, Scotland
- 6 University of Oxford, Oxford, England
- 7 UCL Comprehensive Clinical Trials Unit, London, England
- 8 Centre for Statistics in Medicine, University of Oxford, Oxford, England
- 9 University of Nottingham, Nottingham, England
- 10 Janssen Research & Development LLC, Raritan, New Jersey
- 11 Johnson & Johnson, Titusville, New Jersey
- 12 Luxembourg Institute of Health, Strassen, Luxembourg
- 13 Clinical Trials Consulting & Training Limited, Buckingham, England
- 14 Medicines and Healthcare Products Regulatory Agency, London, England
- 15 BMJ , London, England
- Editorial Guidelines for Statistical Analysis Plans David L. DeMets, PhD; Thomas D. Cook, PhD; Kevin A. Buhr, PhD JAMA
- Comment & Response Statistical Analysis Plans for Clinical Trials Bruno Mario Cesana, MD JAMA
- Comment & Response Statistical Analysis Plans for Clinical Trials—Reply Carrol Gamble, PhD; Steff Lewis, PhD; Stephen Senn, PhD JAMA
Importance While guidance on statistical principles for clinical trials exists, there is an absence of guidance covering the required content of statistical analysis plans (SAPs) to support transparency and reproducibility.
Objective To develop recommendations for a minimum set of items that should be addressed in SAPs for clinical trials, developed with input from statisticians, previous guideline authors, journal editors, regulators, and funders.
Design Funders and regulators (n = 39) of randomized trials were contacted and the literature was searched to identify existing guidance; a survey of current practice was conducted across the network of UK Clinical Research Collaboration–registered trial units (n = 46, 1 unit had 2 responders) and a Delphi survey (n = 73 invited participants) was conducted to establish consensus on SAPs. The Delphi survey was sent to statisticians in trial units who completed the survey of current practice (n = 46), CONSORT (Consolidated Standards of Reporting Trials) and SPIRIT (Standard Protocol Items: Recommendations for Interventional Trials) guideline authors (n = 16), pharmaceutical industry statisticians (n = 3), journal editors (n = 9), and regulators (n = 2) (3 participants were included in 2 groups each), culminating in a consensus meeting attended by experts (N = 12) with representatives from each group. The guidance subsequently underwent critical review by statisticians from the surveyed trial units and members of the expert panel of the consensus meeting (N = 51), followed by piloting of the guidance document in the SAPs of 5 trials.
Findings No existing guidance was identified. The registered trials unit survey (46 responses) highlighted diversity in current practice and confirmed support for developing guidance. The Delphi survey (54 of 73, 74% participants completing both rounds) reached consensus on 42% (n = 46) of 110 items. The expert panel (N = 12) agreed that 63 items should be included in the guidance, with an additional 17 items identified as important but may be referenced elsewhere. Following critical review and piloting, some overlapping items were combined, leaving 55 items.
Conclusions and Relevance Recommendations are provided for a minimum set of items that should be addressed and included in SAPs for clinical trials. Trial registration, protocols, and statistical analysis plans are critically important in ensuring appropriate reporting of clinical trials.
Transparency has been described as a fundamental value of society and initiatives to increase transparency in relation to clinical trial data have been launched. 1 Given the influence of statistical decisions on trial conclusions, well-documented and transparent statistical conduct is essential. This is relevant given concerns regarding research reproducibility. 2
Quiz Ref ID The contribution of the statistician to the design and analysis of clinical trials is acknowledged to be essential. 3 Guidance on statistical principles for clinical trials (International Conference for Harmonisation of Technical Requirements for Pharmaceuticals for Human Use [ICH] E9) 4 state that “the principal features of the eventual statistical analysis of the data should be described in the statistical section of the protocol.” However, ICH E9 4 and SPIRIT (Standard Protocol Items: Recommendations for Interventional Trials) 5 guidelines refer to a separate statistical analysis plan (SAP). The level of detail appropriate for a SAP exceeds that of a protocol. According to ICH E9, 4 a SAP “contains a more technical and detailed elaboration of the principal features of the analysis described in the protocol, and includes detailed procedures for executing the statistical analysis of the primary and secondary variables and other data.” While guidance exists on the content of clinical trial protocols 5 and reporting standards for clinical trials, 6 both of which require a summary of the statistical analyses, there is no guidance on SAP content. Consequently, there is marked variation in practice.
This Special Communication provides recommendations for a minimum set of items that should be addressed and describes the methods used to develop this list. The recommendations are intended to aid the drafting of SAPs for clinical trials and improve their completeness.
The need to develop guidance on SAPs was raised during discussion by statisticians attending a UK Clinical Research Collaboration (UKCRC) Registered CTU (Clinical Trials Unit) Statisticians’ Operational Group meeting in November 2012. This group included 46 senior statisticians, each representing their CTU within the network. This wider group was engaged throughout the development process as well as user-testing and piloting. The members of the CTU network, based in the United Kingdom, conduct clinical trials funded by governmental agencies, foundations, and pharmaceutical companies under the remit of the European Medicines Agency, the UK Medicines and Healthcare Products Regulatory Agency (MHRA), and the US Food and Drug Administration. An application for funding was developed and submitted to the Medical Research Council Network of Hubs for Trials Methodology Research in December 2013 and the project started in May 2014. The SAP guidance document was developed with the primary intention of being applicable to the final analyses of later-phase randomized clinical trials addressing the minimum recommended content of a SAP within the context of the following assumptions:
The SAP is not a standalone document and should be read in conjunction with the clinical trial protocol;
The clinical trial protocol should be consistent with the principles of the SPIRIT 2013 Statement 5 ; and
The SAP is to be applied to a clean or validated data set for analysis.
This guidance document summarizes the findings of a comprehensive search to identify existing SAP guidance; a survey of current practice of statisticians within UKCRC-registered CTUs; and a Delphi survey to establish consensus. Consistent with advice received from the Central Office of Research Ethics, the UK Health Research Authority Decision Tool 7 indicated ethical approval was not required for the surveys and consent to take part was indicated by survey participation.
Major randomized clinical trial funding bodies and regulators were identified from responses to a previous survey, 8 which had generated a list of funders actively supporting clinical trials across at least 2 CTUs within the last 5 years. The full list is contained in eTable 1 in the Supplement and includes the European and Developing Countries Clinical Trials Partnership, FP7 Health Research, Medical Council of Canada, National Cancer Institute of Canada Clinical Trials Group, European Organisation for Research and Treatment for Cancer, National Institutes of Health, and the National Institute for Health Research. Quiz Ref ID The list, which was reviewed by the project team (May 2014), was extended to include regulators (US Food and Drug Administration, European Medicines Agency, and MHRA).
All funders and regulators were contacted by email (June 2014). If a response was not received, up to 2 further reminder emails were sent. If no response was received, the organization was contacted by telephone and the study team discussed whether alternative contacts within the organization could be approached to participate.
Journals were contacted in parallel to funders and regulators, and included JAMA , BMJ , the New England Journal of Medicine , and the Lancet as the leading medical journals publishing clinical trials. Journals identified via a PubMed search (June 2014) publishing SAPs as standalone publications were also contacted ( Trials , Critical Care and Resuscitation , and International Journal of Stroke ). The goal was to identify whether the journals had any internal guidance or recommendations on SAPs, if they followed any externally available guidance on SAPs, whether and how they used SAPs within the peer-review process, and any policies on the publications of SAPs. Each journal website was searched for information relating to SAPs within their support for authors and reviewers prior to contacting a journal editor.
The aim of the survey was to identify current practice and opinions about SAPs. A list of the 45 registered CTUs was accessed from the UKCRC website (June 2014). One CTU reported being split across 2 sites, with each using separate standard operating procedures, and requested that each site complete the survey separately. The survey was developed by A.K., C.G., and D.S. and adapted in response to comments from the project team. To reduce the number of survey questions, copies of standard operational procedures for SAPs and templates or examples of SAPs were also requested. In addition, the survey was piloted during July 2014 by statisticians from the CTUs of the study project team prior to distribution.
A senior statistician at each CTU, identified as the network’s nominated statistics contact, was asked to complete the survey to reflect practices and majority opinion within the statistician’s CTU (August 2014). For networks in which there was no nominated statistics contact, the survey was sent to the CTU director who was asked to delegate completion on behalf of the unit. Two reminder emails were sent to encourage responses. Survey completion was highlighted at network events at which nonresponders were approached to discuss completion. A copy of the survey and the participating CTUs is provided in eAppendix 1 in the Supplement .
The aim of the Delphi survey was to establish consensus among a broad range of stakeholders. The initial list of participants was sent to the project team for review and amendment (January 2015). The UKCRC-registered CTU participants were identified from the survey of current practice (n = 46). CONSORT and SPIRIT guideline authors were identified from relevant publications and websites (n = 16). Pharmaceutical industry contributors were selected from recommendations from the project team and aimed to have both industry and academic experience (n = 5). The journal editors contacted to identify existing guidance were also contacted to participate in the Delphi survey (n = 7). Regulators from the European Medicines Agency and the MHRA were included (n = 2). Contacts with the US Food and Drug Administration were unsuccessful in identifying a participant for the Delphi survey.
A comprehensive list of items that should or could be included within a SAP was derived after reviewing suggested guidance identified from contacting funders and regulators, considering the responses to the survey of current practice, and reviewing copies of standard operational procedures for SAPs and examples of SAPs provided with the survey responses or identified in the literature search. Items were listed individually but grouped under relevant domains.
The list was reviewed by the project team for completeness, comprehension, and suitability of the domains (January 2015). The Delphi survey was completed during February 2015, with each round lasting 2 weeks. During round 1, Delphi participants could suggest additional items for inclusion in round 2. Round 2 included all items from round 1 as well as the additional items suggested by participants. Suggestions were reviewed by the project team and checked for duplication prior to inclusion in round 2.
Participants were asked to score the importance of each item when writing, following, or reviewing a SAP. The scale was presented with 1 to 3 labeled “not important,” 4 to 6 labeled “important but not critical,” and 7 to 9 labeled “critical.” 9
All individual participants who completed round 1 were emailed and asked to complete round 2. In round 2, for each item, participants were presented with the number and percentage of participants who chose each score. Participants were shown their score from round 1 and provided with an option to revise their score for each of the items or keep it the same as their score in round 1.
The definition of consensus was predefined and is presented in eTable 2 in the Supplement . Items were determined to be in (consensus-in) if 70% or more of participants scored the item as critical and less than 15% of participants scored the item as not important. Items were deleted (consensus-out) if 70% or more of participants scored it as not important and less than 15% of participants scored it as critical.
Following round 2 of the Delphi process, a consensus meeting was held (March 2015) with expert representation from each group: CTU senior statisticians, regulators (MHRA), statisticians in the pharmaceutical industry, and journal editors. The 12 expert panel members are listed in eTable 3 in the Supplement .
All items included in the Delphi survey were reviewed at the consensus meeting. Items on which consensus had been reached were highlighted but not discussed further. The expert panel members were asked to discuss each item for which consensus had not been reached and, following discussion, to make a recommendation regarding its inclusion with consensus-in items within the minimum set of items that should be addressed and included in SAPs for clinical trials.
The aim of the critical review and piloting was to ensure the guidance produced was fit for purpose, appropriate to the needs of statisticians authoring and implementing SAPs, and to identify any items requiring clarification. The first draft of the guidance underwent critical review by attendees at the UKCRC Registered CTU Statisticians’ Operational Group meeting in April 2015. Meeting attendees were able to provide additional comments based on further discussions with the statistics team within their CTU until September 2015. Following incorporation of comments, the guidance was sent to the expert panel involved in the Delphi consensus meeting prior to being piloted by senior statisticians across 5 trials in January 2016.
Of the 39 funding bodies or regulators that were contacted and asked about their requirements or guidance for SAPs, 28 responded (72%). Four responders referred to ICH E9, 4 3 to the UK Medical Research Council website or ICH Good Clinical Practice guidance, 3 and 21 indicated an absence of guidance or recommendations relevant to SAPs. A comprehensive search of the literature and references of published SAPs did not identify any publications relevant to the content of SAPs.
The survey to establish current practice was distributed by email to each of the 45 UKCRC-registered CTUs (46 respondents), with a 100% response rate. Responses demonstrated variability in current practice around the processes of producing SAPs and their content. The production of guidance on SAP content was supported by 85% (n = 39) of responders.
Of the 73 invited participants in the Delphi process, 56 (77%) completed round 1 and 54 (73%), round 2. Those completing round 2 included CTU statisticians (40/46; 87%), editors (3/7; 43%), guideline authors (8/16; 50%), industry (5/5; 100%), and a regulator (1/2; 50%) (3 responders contributed to 2 groups each). Thirty percent of the responders were from outside the United Kingdom and included Canada, Germany, Ireland, Denmark, Australia, and the United States.
Round 1 contained 89 items, consensus for items to remain in was reached on 28 items, and an additional 21 items were suggested by responders. Round 2 contained 110 items (89 prepopulated items from round 1 and the 21 suggested items) and at the end of round 2, consensus was reached that 46 items should remain in with 1 item deleted (consensus-out).
At the end of the consensus meeting, there were 63 items in (consensus-in), 30 items deleted (consensus-out), and 17 items that the expert panel felt are important but do not necessarily need to be included (eTable 4 in the Supplement ). These 17 items may be found in other trial documents but the SAP should incorporate references to where details of these items can be found.
The critical review meeting, held in London, was attended by 51 statisticians from 37 CTUs (April 2015). Participants were asked to consider the ordering and clarity of the descriptions of each of the 63 items and to highlight any concerns. To ensure discussion and complete coverage of the items within the meeting, attendees were split into groups, with each group allocated 1 of the 6 sections to review and provide feedback on as a priority. Meeting attendees were also encouraged to discuss the draft guidance with other statisticians within their CTUs and return any additional collective responses. Additional responses were received from 8 CTUs.
Two issues were raised: the first was whether the sample size calculation should be replicated from the protocol in full or referenced and the second was concerning the use of a 2-stage analysis in which the assumptions of the analysis approach are tested and then the analysis determined by whether the assumptions are met or not. The sample size statement was amended to support an individual statistician’s preference to replicate or reference the protocol. The issue surrounding the 2-stage analysis was more controversial and in response to discussions, the guidance was amended to ensure that this was highlighted in the discussion of that item. During critical review of the 63 items, some items were found to overlap and were combined, leaving 55. The Table displays the essential items and their subitems. There are 6 sections: Title and Trial Registration (11 items/subitems); Introduction (2 items); Study Methods (9 items/subitems); Statistical Principles (8 items/subitems); Trial Population (8 items/subitems); and Analysis (17 items/subitems).
An open request for 5 volunteers to undertake piloting of the recommendations in the guidance document was made at the critical review meeting. Twelve statisticians expressed an interest and were invited to participate; 5 were selected to cover CTUs with varying experience in Wales, England, and Scotland, each of whom applied the guidance document to trials in adults and children, and included pharmaceutical and nonpharmaceutical interventions including devices and physiotherapy. The piloting feedback did not require any changes to the guidance and the comments received supported its content and usability.
An elaboration and explanation of each item is included within eAppendix 2 in the Supplement . Examples are provided to illustrate each item, along with an explanation of the rationale and detailed description of the issues to be addressed. Examples for each item are based on real SAPs either published in journals, provided by responders to the CTU survey, or contained within National Institute for Health Research’s Health Technology Assessment monographs.
Quiz Ref ID It is important that every clinical trial has a clear and comprehensive SAP to support reproducibility. Leading organizations and funding bodies openly support data sharing as best practice for clinical trials. 11 Such support will undoubtedly increase the availability of data from original research, resulting in an increase of attempts to replicate results. To support the reproducibility of research and allay concerns of misconduct and fraud in clinical research, a clear comprehensive and transparent account of preplanned statistical analyses must be available. 12 The aim of this guidance is to establish the minimum set of essential items required for a SAP for a clinical trial. It is intended to lead to improvements in the integrity of trial conduct and reporting by facilitating critical appraisal, execution, replication, and identification of any deviations from the prespecified methods.
This SAP guidance was developed following established transparent methods and involving a diverse range of stakeholders involved in the design, funding, conduct, review, and publication of clinical trials. Although the guidance was developed with a focus on the regulatory requirements of trials of medicinal products, and in particular later-phase trials, many aspects are transferable to studies of other types of interventions, phases, and designs.
Quiz Ref ID This guidance document does not cover when a SAP should be written, but early authoring of SAPs—before any data have been collected or analyzed—is the best approach. The final opportunity to amend the SAP should be in response to blind review, defined as the checking and assessment of data during the period between trial completion and the breaking of the blind, the act of unveiling each participant’s random allocation. 4 Following this point, deviations from the SAP and additional analyses should be clearly indicated as such within all reports and publications. 4 In the United Kingdom, the Health Research Authority has developed a protocol template 13 to improve consistency in the way that the items covered by SPIRIT are included within a protocol and a similar template may be beneficial for SAPs.
This guidance assumes that the SAP is not a standalone document, and therefore, it is not necessary to replicate large portions of the protocol, which should instead be clearly referenced. The SAP should contain a statement that it is consistent with the principal features of the statistical methods described in the protocol or a section detailing which analyses are different to those planned in the protocol and why. Any abbreviations used should be spelled out in full.
SAPs should be made publicly available. 14 A major step toward public availability of SAPs is the requirements of the US National Institutes of Health Final Rule for Clinical Trials Registration and Results Information Submission, 15 which in addition to posting of results within ClinicalTrials.gov also requires posting of the SAP if not contained within the protocol. In the discussion of public comments relating to the Final Rule, 15 it was noted that many of the benefits of the protocol that were cited by commenters were derived from the information regarding the statistical analyses. Quiz Ref ID This represents acknowledgment that SAPs have an important role in reducing the occurrence of, and facilitating the detection of, bias particularly in relation to selective analysis and reporting. 16 , 17 Some journals, including JAMA , require the SAP to be submitted alongside the report of a clinical trial for use within the peer-review process. The SAP may be made available as supplementary material or published as a standalone article. While this is encouraging, and increases public availability of SAPs, there is no guidance on how the SAP should be used or evaluated. Similar to protocols, the ability of a SAP to provide transparency is dependent on its content.
Any guidance needs to be responsive to relevant information from future projects and initiatives, as well as changes in legislation. Key initiatives that may influence SAP content include the addendum to ICH E9 on estimands and sensitivity analyses, 18 data-sharing initiatives, 19 and mandatory requirements to post clinical trial results in the European Clinical Trials Database and ClinicalTrials.gov. 15 , 20 , 21 Future revisions of this document will be made available periodically and extensions to other study designs, including observational studies 22 and studies with adaptive designs and Bayesian analyses, should be considered.
Recommendations are provided for a minimum set of items that should be addressed and included in SAPs for clinical trials. Trial registration, protocols, and statistical analysis plans are critically important in ensuring appropriate reporting of clinical trials.
Accepted for Publication: November 7, 2017.
Corresponding Author: Carrol Gamble, PhD, Biostatistics Department, Block F Waterhouse Building, 1-5 Brownlow St, University of Liverpool, Liverpool L69 3GL, England ( [email protected] ).
Author Contributions: Dr Gamble and Ms Krishan had full access to all of the data in the study and take responsibility for the integrity of the data and the accuracy of the data analysis.
Concept and design: Gamble, Stocken, Lewis, Dore, Williamson, Montgomery, Lim, Berlin, Senn.
Acquisition, analysis, or interpretation of data: Gamble, Krishan, Stocken, Juszczak, Dore, Williamson, Altman, Montgomery, Lim, Day, Barbachano, Loder.
Drafting of the manuscript: Gamble, Krishan, Stocken, Dore, Altman, Lim.
Critical revision of the manuscript for important intellectual content: Gamble, Stocken, Lewis, Juszczak, Dore, Williamson, Montgomery, Lim, Berlin, Senn, Day, Barbachano, Loder.
Statistical analysis: Gamble, Krishan, Stocken, Williamson, Senn.
Obtained funding: Gamble, Stocken, Lewis, Juszczak, Dore, Williamson, Montgomery.
Administrative, technical, or material support: Williamson, Lim.
Supervision: Gamble, Stocken.
Conflict of Interest Disclosures: All authors have completed and submitted the ICMJE Form for Disclosure of Potential Conflicts of Interest. Dr Berlin is a full-time employee of Johnson & Johnson. Dr Loder is head of research for BMJ . No other disclosures were reported.
Funding/Support: This work was funded by grant MR/L004933/1-R44 from the UK Medical Research Council Network of Hubs for Trials Methodology Research and supported and endorsed by the UK Clinical Research Collaboration Registered Clinical Trials Unit Network.
Role of the Funder/Sponsor: The funders/sponsors had no role in the design and conduct of the study; collection, management, analysis and interpretation of the data; preparation review, or approval of the manuscript; and decision to submit the manuscript for publication.
- Register for email alerts with links to free full-text articles
- Access PDFs of free articles
- Manage your interests
- Save searches and receive search alerts
How to Develop a Statistical Analysis Plan (SAP) For Clinical Trials
Table of Contents
Kolabtree freelance biostatistician consultant , Rudra Patel provides a comprehensive guide on how to develop a Statistical Analysis Plan (SAP) for Clinical Trials .
1. Statistical Analysis Plan (SAP) in a Clinical Trial (CT)
A well-written and complete Statistical Analysis Plan (SAP) is important to increase the quality of clinical trials and make it more valid and generalized.
SAP is a defined outline of the planned statistical basic/advance methods for the analyses for a clinical trial and is written in study protocol as well as separately. SAP is crucial and is one of the key Regulatory confidential documents in the development of a clinical trial. An SAP is a more challenging task in a clinical trial protocol development that requires a strong command on statistical methodology , medical terminology and visualization power. It provides explicit guidance on statistical programming and the presentation of results for clinical trial. The following four important types of SAP are used in a clinical trial (Figure 1).
- Data monitoring
- Interim statistical analysis
- Integrated statistical analysis plan
- Statistical analysis plan for clinical study
Figure 1: Four important types of SAP are used in a CT
The SAP is mostly written as a separate document or it is included in CT study protocol as a standard operating procedure for dealing with the statistical part of the clinical study. A team medical statistician /biostatistician is in-charge of developing the SAP in coordination with the principal investigator of the CT study. The document should be reviewed by Senior Biostatistician and finalized before to submission to the review board and regulatory authorities. If any protocol amendments are done, then the SAP is amended as well.
The SAP must properly explain following the aims and primary objectives, secondary objective, exploratory objectives, primary/secondary/exploratory endpoints, trial population, design of the trial, sample size calculations with justifications/assumptions, and the randomization methods. Additionally, an SAP must describe in detail the statistical methodology i.e. efficacy analysis, safety data analysis , reporting conventions, etc. Figure 2 shows the most important points that need to be considering when developing SAP in the clinical trial study protocol.
Figure 2: Detailed important points considering in when developing SAP in CT protocol.
The analysis plan developed should be reviewed special attention and approved by senior blinded biostatistician before database lock by authorities. Ahrweiler et al. 2011 conference paper published online explained the importance of review statistical analysis plan. The following detailed important points considering in when developing SAP in CT protocol,
- Detailed of the planned statistical analysis
- Elaborating on the principal features of the technical analysis.
- Trial objectives
- Data sources
- Population studied
- Study endpoints
- Statistical methodology
- Sensitivity analysis and missing data
The clinical trial SAP should be developing with an in-depth discussion between the study the principle investigators and statistician. Following are statistician role and responsibility,
- To write a research statement or hypothesis of the clinical trial study.
- Determine the primary endpoints and secondary endpoints.
- To find out and develop a strategy to reduce bias and sample size selection for clinical trial
- To define all appropriate statistical methods for clinical trial data analysis
In the development of SAP in the clinical trial need to explain in-depth key highlights points. Yuan et al.2019 published a special interest article on “ Guide to the statistical analysis plan ” [Figure 2]. The article provides in-depth the SAP of an actual clinical trial research study is to provide a practical detailed guide on writing an effective SAP. Additionally in the same paper discussed where what, why of an SAP, when and who, and highlight the key contents of the SAP. The need for clinical trial research study to well written and documented SAPs, particularly for regulatory studies.
2. Importance of the Statistical Analysis Plan in Clinical Trials
CT is conducted on all new drug/medicine development process and medical devices. Since last one decade, increasing the rate patients recruited into clinical trials for drug/medicine developments have been from Europe and the US as well as developing countries.
In clinical studies, SAP is one of the critically important documents. It ensures that the analyses to evaluate all pre-planned study hypotheses are conducted in a scientifically valid manner and that all decisions are documented. It also provides in-depth detail on how the results will be presented and reported in CT.
Clinical trials are used to assess the additional benefits and improve interventions in medical health care . The more important thing to consider while conducting a clinical trial is to execute the trial with minimum bias. Therefore, each clinical trial to have a clear and detailed SAP to its support to reproducibility. For the best practice of CT scientific research studies, reproducibility of research, and to avoid concerns of misuse of clinical research, a clear detailed and very transparent SAP much be needed, to improve trial conduct and reporting. Following are three essential roles of SAP needs to maintain in conducting CT.
- Transparency: Transparency concerning how the analysis will proceed by specifying in advances the methodology that will be applied
- Communication: Clear communication to everyone involved in the study on how to proceed
- Replication: Facilitates replication so that a future research team can follow the same steps to confirm the results on the same or a new sample.
As per standard guidelines with best practice, it’s important to the clinical trial project statistician/biostatistician prepares a study SAP before clinical trial start, detailing all the planned analyses, study parameters, including analysis set definitions and basic/ advance statistical methodology.
Additionally, some other important considerations relating to SAP in CT include:
- One way of minimizing bias is to blind the Biostatistician.
- The SAP should be documented in such a way that all the data manipulations and analyses performed can be replicated.
- A Trial Master File is required to be maintained with all the relevant documentation at trial completion by the Biostatistician.
The systematically arranged analysis plan helps the clinical trial team to be together on the same page and adds another layer of specificity to the CT. It describes the systematic planned statistical methodology of a clinical trial research study. As compare to the protocol of clinical trial the SAP is an in-depth technical document in which detailed statistical techniques for study designing and analyzing clinical trials data. While writing SAP we generally follow ICH E3 and E9 guidelines. This gives us an idea of the body content of individual sections of SAP. But E3 and E9 do not specify specific statistical techniques.
To improve reproducibility, transparency, and validity among clinical trials. National Institutes of Health ( NIH ) published “Rules for clinical trials studies registration and results information submission”, in that mandates trial registration, posting of clinical trial ongoing recruitment or results within ClinicalTrials.gov , and submission of the separate original document statistical analysis plan (SAP) along with the clinical trial research study protocol.
The big contribution of the medical statistician/biostatistician apart from developing a standard SAP is to the designing, monitoring and analyzing of clinical trial data.
3. Detailed checklist/guidelines for SAPs in clinical trials
In developing SAP of CT, we need to take into consideration all detailed checklist/standard guidelines. Important guidelines used in development in SAP are ICH E9 (International Conference for Harmonisation of Technical Requirements for Pharmaceuticals for Human Use) and SPIRIT (Standard Protocol Items: Recommendations for Interventional Trials).
Transparency and reproducibility have been a fundamental term adding value in clinical trial data. However, the influence of statistical methodology directly affects on decisions making of clinical trial, well-documented, maintained confidentiality and transparent statistical conduct is essential. Expert medical statistician/biostatisticians can help develop SAPs in accordance with standard guidelines.
As per ICH E9 SAP usually known as reporting and analysis plans may also be known as Data Analysis Plans (DAP) or Statistical Analysis Plans (SAP) in other organizations. ICH E9 guidelines state that “the principal features of the eventual SAP of the data should be described in the statistical section of the protocol.” However, SPIRIT (Standard Protocol Items: Recommendations for Interventional Trials) guidelines refer to a separate SAP.
The SAP is a most essential document in CT which needs report to regulatory authorities (E.g. Food and Drug Administration ( FDA ), European Medicines Agency ( EMA )). Standard guidelines suggest that SAP needs to stored in the confidentially clinical trial master file and it is used during regulatory authorizes audits to check if statistical documents followed exactly by standard guidelines.
The SAP is the most commonly used documents to guide statisticians. In general, the followings should be included in an SAP (Figure 3).
The statistician should be referred to The CONSORT Statement (and any extensions) and also ICH E9 Statistical Principles for Clinical Trials (PDF, 325 KB) .
- Trial Planning & Design station
- The EQUATOR Network – A resource centre for good reporting of health research studies.
- The CONSORT website
- In 2017 the published guidelines by Gamble et al .2017, the guidelines of these articles recommend a minimum of 55 important items that should be considered when developing an SAP following.
- Title and registration
- Introduction
- Study methods
- Statistical principles
- Trial population
Figure 3: Gamble et al . 2017, the guidelines are divided into 6 major sections.
4. What does an SAP consist of?
A checklist of 32 detailed checklists for developing the Statistical Analysis Plan (SAP) Checklist (Word) . The primary intention of being more applicable to the final analyses of CTs as well as later-phase randomized CT. The most important guidelines developed by the FDA’s Guidance for Industry: Statistical Principles for Clinical Trials .
The following guidelines and recommendation for the content of an SAP:
- SAP is not a standalone document and should be read in conjunction with the clinical trial protocol.
- The clinical trial protocol should be consistent with the principles of the SPIRIT 2013 Statement .
- The SAP is to be applied to a clean or validated data set for analysis.
Detailed guidelines developed through funders, regulatory authorities, journals, industry representatives and UK Clinical Research Collaboration registered Clinical Trial Units (UKCRC CTUs). The Guidelines for the Content of Statistical Analysis Plans in Clinical Trials in-depth details describe in JAMA . However, a more in-depth detailed explanation of each checklist per item can be found in the elaboration document . The SAP statement also included is included in the Equator Network and MRC-NIHR Trials Methodology Research Partnership (TMRP) . Following are key documents and key links used in developing SAP in clinical trial (Figure 4).
- Elaboration
- Equator Network
- MRC-NIHR Trials Methodology Research Partnership (TMRP)
- UK Clinical Research Collaboration registered Clinical Trial Units
Figure 4: Key documents and key links used in developing SAP in clinical trial
5. Hiring a freelance clinical statistician for help with SAPs
Developing an SAP often requires the support of a freelance clinical statistician. With the help of an experienced biostatistician, you can develop a thorough and error-free SAP, that will improve the quality of your clinical trials.
Browse clinical trial consultants on Kolabtree now and get in touch with an expert directly.
Unlock Corporate Benefits • Secure Payment Assistance • Onboarding Support • Dedicated Account Manager
Sign up with your professional email to avail special advances offered against purchase orders, seamless multi-channel payments, and extended support for agreements.
About Author
Ramya Sriram manages digital content and communications at Kolabtree (kolabtree.com), the world's largest freelancing platform for scientists. She has over a decade of experience in publishing, advertising and digital content creation.
Related Posts
Three ways on-demand medical writers can help your business , big data in biotech: free kolabtree whitepaper, hire a data scientist – the complete guide, leave a reply cancel reply.
Save my name, email, and website in this browser for the next time I comment.
Automated page speed optimizations for fast site performance
Guidelines for the Content of Statistical Analysis Plans in Clinical Trials
Affiliations.
- 1 Biostatistics Department, University of Liverpool, Liverpool, England.
- 2 Clinical Trials Research Centre, University of Liverpool, Liverpool, England.
- 3 Newcastle University, Newcastle, England.
- 4 Currently with Leeds Institute of Clinical Trials Research, University of Leeds, Leeds, England.
- 5 Edinburgh University, Edinburgh, Scotland.
- 6 University of Oxford, Oxford, England.
- 7 UCL Comprehensive Clinical Trials Unit, London, England.
- 8 Centre for Statistics in Medicine, University of Oxford, Oxford, England.
- 9 University of Nottingham, Nottingham, England.
- 10 Janssen Research & Development LLC, Raritan, New Jersey.
- 11 Johnson & Johnson, Titusville, New Jersey.
- 12 Luxembourg Institute of Health, Strassen, Luxembourg.
- 13 Clinical Trials Consulting & Training Limited, Buckingham, England.
- 14 Medicines and Healthcare Products Regulatory Agency, London, England.
- 15 , London, England.
- PMID: 29260229
- DOI: 10.1001/jama.2017.18556
Importance: While guidance on statistical principles for clinical trials exists, there is an absence of guidance covering the required content of statistical analysis plans (SAPs) to support transparency and reproducibility.
Objective: To develop recommendations for a minimum set of items that should be addressed in SAPs for clinical trials, developed with input from statisticians, previous guideline authors, journal editors, regulators, and funders.
Design: Funders and regulators (n = 39) of randomized trials were contacted and the literature was searched to identify existing guidance; a survey of current practice was conducted across the network of UK Clinical Research Collaboration-registered trial units (n = 46, 1 unit had 2 responders) and a Delphi survey (n = 73 invited participants) was conducted to establish consensus on SAPs. The Delphi survey was sent to statisticians in trial units who completed the survey of current practice (n = 46), CONSORT (Consolidated Standards of Reporting Trials) and SPIRIT (Standard Protocol Items: Recommendations for Interventional Trials) guideline authors (n = 16), pharmaceutical industry statisticians (n = 3), journal editors (n = 9), and regulators (n = 2) (3 participants were included in 2 groups each), culminating in a consensus meeting attended by experts (N = 12) with representatives from each group. The guidance subsequently underwent critical review by statisticians from the surveyed trial units and members of the expert panel of the consensus meeting (N = 51), followed by piloting of the guidance document in the SAPs of 5 trials.
Findings: No existing guidance was identified. The registered trials unit survey (46 responses) highlighted diversity in current practice and confirmed support for developing guidance. The Delphi survey (54 of 73, 74% participants completing both rounds) reached consensus on 42% (n = 46) of 110 items. The expert panel (N = 12) agreed that 63 items should be included in the guidance, with an additional 17 items identified as important but may be referenced elsewhere. Following critical review and piloting, some overlapping items were combined, leaving 55 items.
Conclusions and relevance: Recommendations are provided for a minimum set of items that should be addressed and included in SAPs for clinical trials. Trial registration, protocols, and statistical analysis plans are critically important in ensuring appropriate reporting of clinical trials.
Publication types
- Consensus Development Conference
- Clinical Trials as Topic / standards*
- Data Interpretation, Statistical*
- Delphi Technique
- Statistics as Topic / standards*
Grants and funding
- MR/L004933/1/MRC_/Medical Research Council/United Kingdom
- MR/L004933/2/MRC_/Medical Research Council/United Kingdom
- 16895/CRUK_/Cancer Research UK/United Kingdom
- MR/K025635/1/MRC_/Medical Research Council/United Kingdom
- MC_UU_12023/21/MRC_/Medical Research Council/United Kingdom
- Skip to main content
- Skip to primary sidebar
- Skip to footer
- QuestionPro

- Solutions Industries Gaming Automotive Sports and events Education Government Travel & Hospitality Financial Services Healthcare Cannabis Technology Use Case NPS+ Communities Audience Contactless surveys Mobile LivePolls Member Experience GDPR Positive People Science 360 Feedback Surveys
- Resources Blog eBooks Survey Templates Case Studies Training Help center

Home Market Research
Data Analysis in Research: Types & Methods
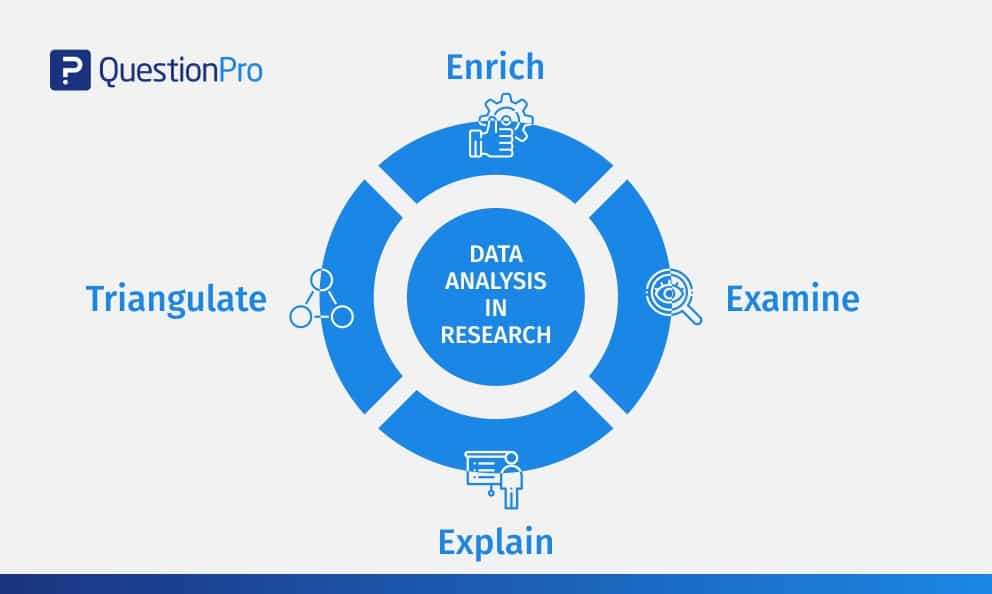
Content Index
Why analyze data in research?
Types of data in research, finding patterns in the qualitative data, methods used for data analysis in qualitative research, preparing data for analysis, methods used for data analysis in quantitative research, considerations in research data analysis, what is data analysis in research.
Definition of research in data analysis: According to LeCompte and Schensul, research data analysis is a process used by researchers to reduce data to a story and interpret it to derive insights. The data analysis process helps reduce a large chunk of data into smaller fragments, which makes sense.
Three essential things occur during the data analysis process — the first is data organization . Summarization and categorization together contribute to becoming the second known method used for data reduction. It helps find patterns and themes in the data for easy identification and linking. The third and last way is data analysis – researchers do it in both top-down and bottom-up fashion.
LEARN ABOUT: Research Process Steps
On the other hand, Marshall and Rossman describe data analysis as a messy, ambiguous, and time-consuming but creative and fascinating process through which a mass of collected data is brought to order, structure and meaning.
We can say that “the data analysis and data interpretation is a process representing the application of deductive and inductive logic to the research and data analysis.”
Researchers rely heavily on data as they have a story to tell or research problems to solve. It starts with a question, and data is nothing but an answer to that question. But, what if there is no question to ask? Well! It is possible to explore data even without a problem – we call it ‘Data Mining’, which often reveals some interesting patterns within the data that are worth exploring.
Irrelevant to the type of data researchers explore, their mission and audiences’ vision guide them to find the patterns to shape the story they want to tell. One of the essential things expected from researchers while analyzing data is to stay open and remain unbiased toward unexpected patterns, expressions, and results. Remember, sometimes, data analysis tells the most unforeseen yet exciting stories that were not expected when initiating data analysis. Therefore, rely on the data you have at hand and enjoy the journey of exploratory research.
Create a Free Account
Every kind of data has a rare quality of describing things after assigning a specific value to it. For analysis, you need to organize these values, processed and presented in a given context, to make it useful. Data can be in different forms; here are the primary data types.
- Qualitative data: When the data presented has words and descriptions, then we call it qualitative data . Although you can observe this data, it is subjective and harder to analyze data in research, especially for comparison. Example: Quality data represents everything describing taste, experience, texture, or an opinion that is considered quality data. This type of data is usually collected through focus groups, personal qualitative interviews , qualitative observation or using open-ended questions in surveys.
- Quantitative data: Any data expressed in numbers of numerical figures are called quantitative data . This type of data can be distinguished into categories, grouped, measured, calculated, or ranked. Example: questions such as age, rank, cost, length, weight, scores, etc. everything comes under this type of data. You can present such data in graphical format, charts, or apply statistical analysis methods to this data. The (Outcomes Measurement Systems) OMS questionnaires in surveys are a significant source of collecting numeric data.
- Categorical data: It is data presented in groups. However, an item included in the categorical data cannot belong to more than one group. Example: A person responding to a survey by telling his living style, marital status, smoking habit, or drinking habit comes under the categorical data. A chi-square test is a standard method used to analyze this data.
Learn More : Examples of Qualitative Data in Education
Data analysis in qualitative research
Data analysis and qualitative data research work a little differently from the numerical data as the quality data is made up of words, descriptions, images, objects, and sometimes symbols. Getting insight from such complicated information is a complicated process. Hence it is typically used for exploratory research and data analysis .
Although there are several ways to find patterns in the textual information, a word-based method is the most relied and widely used global technique for research and data analysis. Notably, the data analysis process in qualitative research is manual. Here the researchers usually read the available data and find repetitive or commonly used words.
For example, while studying data collected from African countries to understand the most pressing issues people face, researchers might find “food” and “hunger” are the most commonly used words and will highlight them for further analysis.
LEARN ABOUT: Level of Analysis
The keyword context is another widely used word-based technique. In this method, the researcher tries to understand the concept by analyzing the context in which the participants use a particular keyword.
For example , researchers conducting research and data analysis for studying the concept of ‘diabetes’ amongst respondents might analyze the context of when and how the respondent has used or referred to the word ‘diabetes.’
The scrutiny-based technique is also one of the highly recommended text analysis methods used to identify a quality data pattern. Compare and contrast is the widely used method under this technique to differentiate how a specific text is similar or different from each other.
For example: To find out the “importance of resident doctor in a company,” the collected data is divided into people who think it is necessary to hire a resident doctor and those who think it is unnecessary. Compare and contrast is the best method that can be used to analyze the polls having single-answer questions types .
Metaphors can be used to reduce the data pile and find patterns in it so that it becomes easier to connect data with theory.
Variable Partitioning is another technique used to split variables so that researchers can find more coherent descriptions and explanations from the enormous data.
LEARN ABOUT: Qualitative Research Questions and Questionnaires
There are several techniques to analyze the data in qualitative research, but here are some commonly used methods,
- Content Analysis: It is widely accepted and the most frequently employed technique for data analysis in research methodology. It can be used to analyze the documented information from text, images, and sometimes from the physical items. It depends on the research questions to predict when and where to use this method.
- Narrative Analysis: This method is used to analyze content gathered from various sources such as personal interviews, field observation, and surveys . The majority of times, stories, or opinions shared by people are focused on finding answers to the research questions.
- Discourse Analysis: Similar to narrative analysis, discourse analysis is used to analyze the interactions with people. Nevertheless, this particular method considers the social context under which or within which the communication between the researcher and respondent takes place. In addition to that, discourse analysis also focuses on the lifestyle and day-to-day environment while deriving any conclusion.
- Grounded Theory: When you want to explain why a particular phenomenon happened, then using grounded theory for analyzing quality data is the best resort. Grounded theory is applied to study data about the host of similar cases occurring in different settings. When researchers are using this method, they might alter explanations or produce new ones until they arrive at some conclusion.
LEARN ABOUT: 12 Best Tools for Researchers
Data analysis in quantitative research
The first stage in research and data analysis is to make it for the analysis so that the nominal data can be converted into something meaningful. Data preparation consists of the below phases.
Phase I: Data Validation
Data validation is done to understand if the collected data sample is per the pre-set standards, or it is a biased data sample again divided into four different stages
- Fraud: To ensure an actual human being records each response to the survey or the questionnaire
- Screening: To make sure each participant or respondent is selected or chosen in compliance with the research criteria
- Procedure: To ensure ethical standards were maintained while collecting the data sample
- Completeness: To ensure that the respondent has answered all the questions in an online survey. Else, the interviewer had asked all the questions devised in the questionnaire.
Phase II: Data Editing
More often, an extensive research data sample comes loaded with errors. Respondents sometimes fill in some fields incorrectly or sometimes skip them accidentally. Data editing is a process wherein the researchers have to confirm that the provided data is free of such errors. They need to conduct necessary checks and outlier checks to edit the raw edit and make it ready for analysis.
Phase III: Data Coding
Out of all three, this is the most critical phase of data preparation associated with grouping and assigning values to the survey responses . If a survey is completed with a 1000 sample size, the researcher will create an age bracket to distinguish the respondents based on their age. Thus, it becomes easier to analyze small data buckets rather than deal with the massive data pile.
LEARN ABOUT: Steps in Qualitative Research
After the data is prepared for analysis, researchers are open to using different research and data analysis methods to derive meaningful insights. For sure, statistical analysis plans are the most favored to analyze numerical data. In statistical analysis, distinguishing between categorical data and numerical data is essential, as categorical data involves distinct categories or labels, while numerical data consists of measurable quantities. The method is again classified into two groups. First, ‘Descriptive Statistics’ used to describe data. Second, ‘Inferential statistics’ that helps in comparing the data .
Descriptive statistics
This method is used to describe the basic features of versatile types of data in research. It presents the data in such a meaningful way that pattern in the data starts making sense. Nevertheless, the descriptive analysis does not go beyond making conclusions. The conclusions are again based on the hypothesis researchers have formulated so far. Here are a few major types of descriptive analysis methods.
Measures of Frequency
- Count, Percent, Frequency
- It is used to denote home often a particular event occurs.
- Researchers use it when they want to showcase how often a response is given.
Measures of Central Tendency
- Mean, Median, Mode
- The method is widely used to demonstrate distribution by various points.
- Researchers use this method when they want to showcase the most commonly or averagely indicated response.
Measures of Dispersion or Variation
- Range, Variance, Standard deviation
- Here the field equals high/low points.
- Variance standard deviation = difference between the observed score and mean
- It is used to identify the spread of scores by stating intervals.
- Researchers use this method to showcase data spread out. It helps them identify the depth until which the data is spread out that it directly affects the mean.
Measures of Position
- Percentile ranks, Quartile ranks
- It relies on standardized scores helping researchers to identify the relationship between different scores.
- It is often used when researchers want to compare scores with the average count.
For quantitative research use of descriptive analysis often give absolute numbers, but the in-depth analysis is never sufficient to demonstrate the rationale behind those numbers. Nevertheless, it is necessary to think of the best method for research and data analysis suiting your survey questionnaire and what story researchers want to tell. For example, the mean is the best way to demonstrate the students’ average scores in schools. It is better to rely on the descriptive statistics when the researchers intend to keep the research or outcome limited to the provided sample without generalizing it. For example, when you want to compare average voting done in two different cities, differential statistics are enough.
Descriptive analysis is also called a ‘univariate analysis’ since it is commonly used to analyze a single variable.
Inferential statistics
Inferential statistics are used to make predictions about a larger population after research and data analysis of the representing population’s collected sample. For example, you can ask some odd 100 audiences at a movie theater if they like the movie they are watching. Researchers then use inferential statistics on the collected sample to reason that about 80-90% of people like the movie.
Here are two significant areas of inferential statistics.
- Estimating parameters: It takes statistics from the sample research data and demonstrates something about the population parameter.
- Hypothesis test: I t’s about sampling research data to answer the survey research questions. For example, researchers might be interested to understand if the new shade of lipstick recently launched is good or not, or if the multivitamin capsules help children to perform better at games.
These are sophisticated analysis methods used to showcase the relationship between different variables instead of describing a single variable. It is often used when researchers want something beyond absolute numbers to understand the relationship between variables.
Here are some of the commonly used methods for data analysis in research.
- Correlation: When researchers are not conducting experimental research or quasi-experimental research wherein the researchers are interested to understand the relationship between two or more variables, they opt for correlational research methods.
- Cross-tabulation: Also called contingency tables, cross-tabulation is used to analyze the relationship between multiple variables. Suppose provided data has age and gender categories presented in rows and columns. A two-dimensional cross-tabulation helps for seamless data analysis and research by showing the number of males and females in each age category.
- Regression analysis: For understanding the strong relationship between two variables, researchers do not look beyond the primary and commonly used regression analysis method, which is also a type of predictive analysis used. In this method, you have an essential factor called the dependent variable. You also have multiple independent variables in regression analysis. You undertake efforts to find out the impact of independent variables on the dependent variable. The values of both independent and dependent variables are assumed as being ascertained in an error-free random manner.
- Frequency tables: The statistical procedure is used for testing the degree to which two or more vary or differ in an experiment. A considerable degree of variation means research findings were significant. In many contexts, ANOVA testing and variance analysis are similar.
- Analysis of variance: The statistical procedure is used for testing the degree to which two or more vary or differ in an experiment. A considerable degree of variation means research findings were significant. In many contexts, ANOVA testing and variance analysis are similar.
- Researchers must have the necessary research skills to analyze and manipulation the data , Getting trained to demonstrate a high standard of research practice. Ideally, researchers must possess more than a basic understanding of the rationale of selecting one statistical method over the other to obtain better data insights.
- Usually, research and data analytics projects differ by scientific discipline; therefore, getting statistical advice at the beginning of analysis helps design a survey questionnaire, select data collection methods , and choose samples.
LEARN ABOUT: Best Data Collection Tools
- The primary aim of data research and analysis is to derive ultimate insights that are unbiased. Any mistake in or keeping a biased mind to collect data, selecting an analysis method, or choosing audience sample il to draw a biased inference.
- Irrelevant to the sophistication used in research data and analysis is enough to rectify the poorly defined objective outcome measurements. It does not matter if the design is at fault or intentions are not clear, but lack of clarity might mislead readers, so avoid the practice.
- The motive behind data analysis in research is to present accurate and reliable data. As far as possible, avoid statistical errors, and find a way to deal with everyday challenges like outliers, missing data, data altering, data mining , or developing graphical representation.
LEARN MORE: Descriptive Research vs Correlational Research The sheer amount of data generated daily is frightening. Especially when data analysis has taken center stage. in 2018. In last year, the total data supply amounted to 2.8 trillion gigabytes. Hence, it is clear that the enterprises willing to survive in the hypercompetitive world must possess an excellent capability to analyze complex research data, derive actionable insights, and adapt to the new market needs.
LEARN ABOUT: Average Order Value
QuestionPro is an online survey platform that empowers organizations in data analysis and research and provides them a medium to collect data by creating appealing surveys.
MORE LIKE THIS
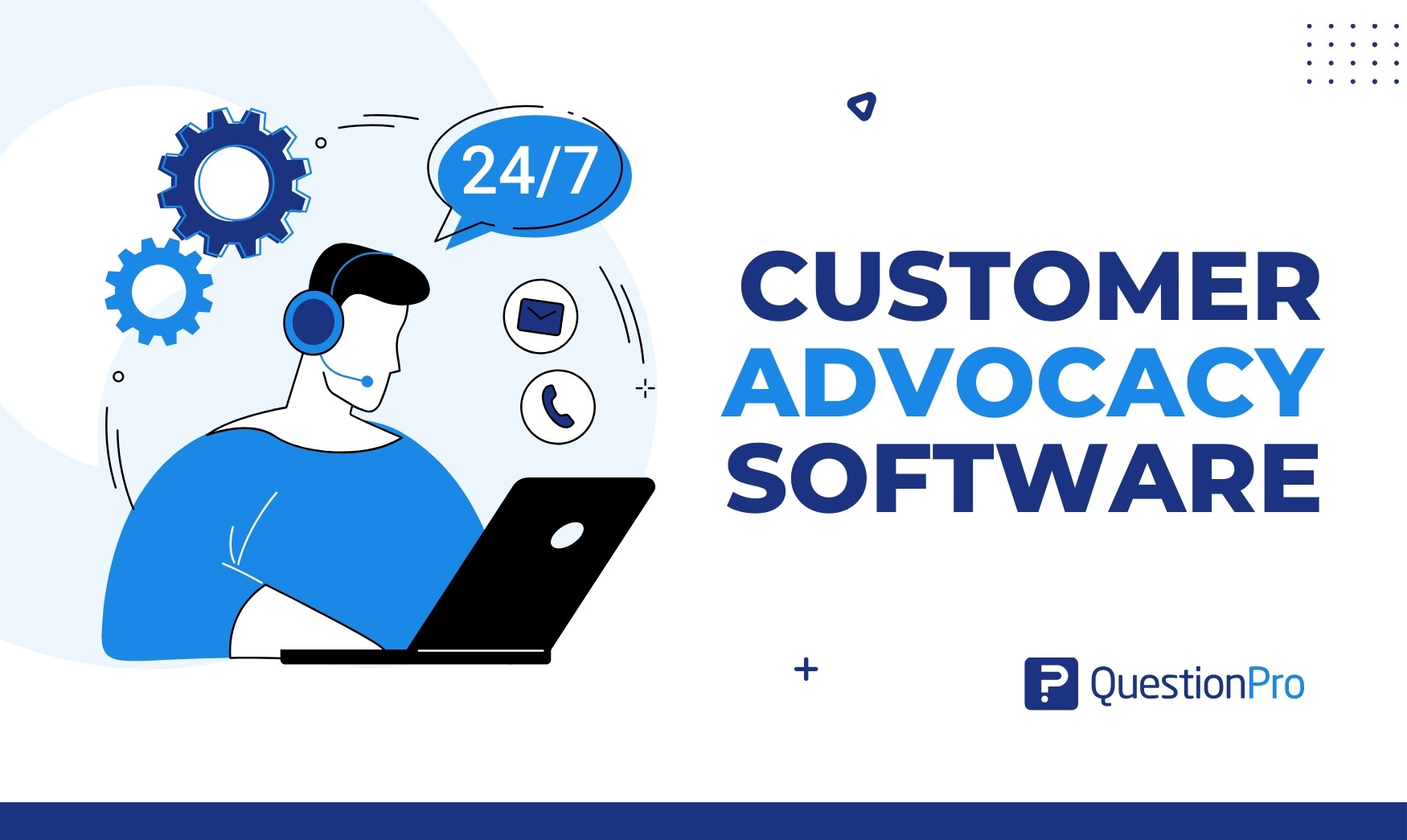
21 Best Customer Advocacy Software for Customers in 2024
Apr 19, 2024
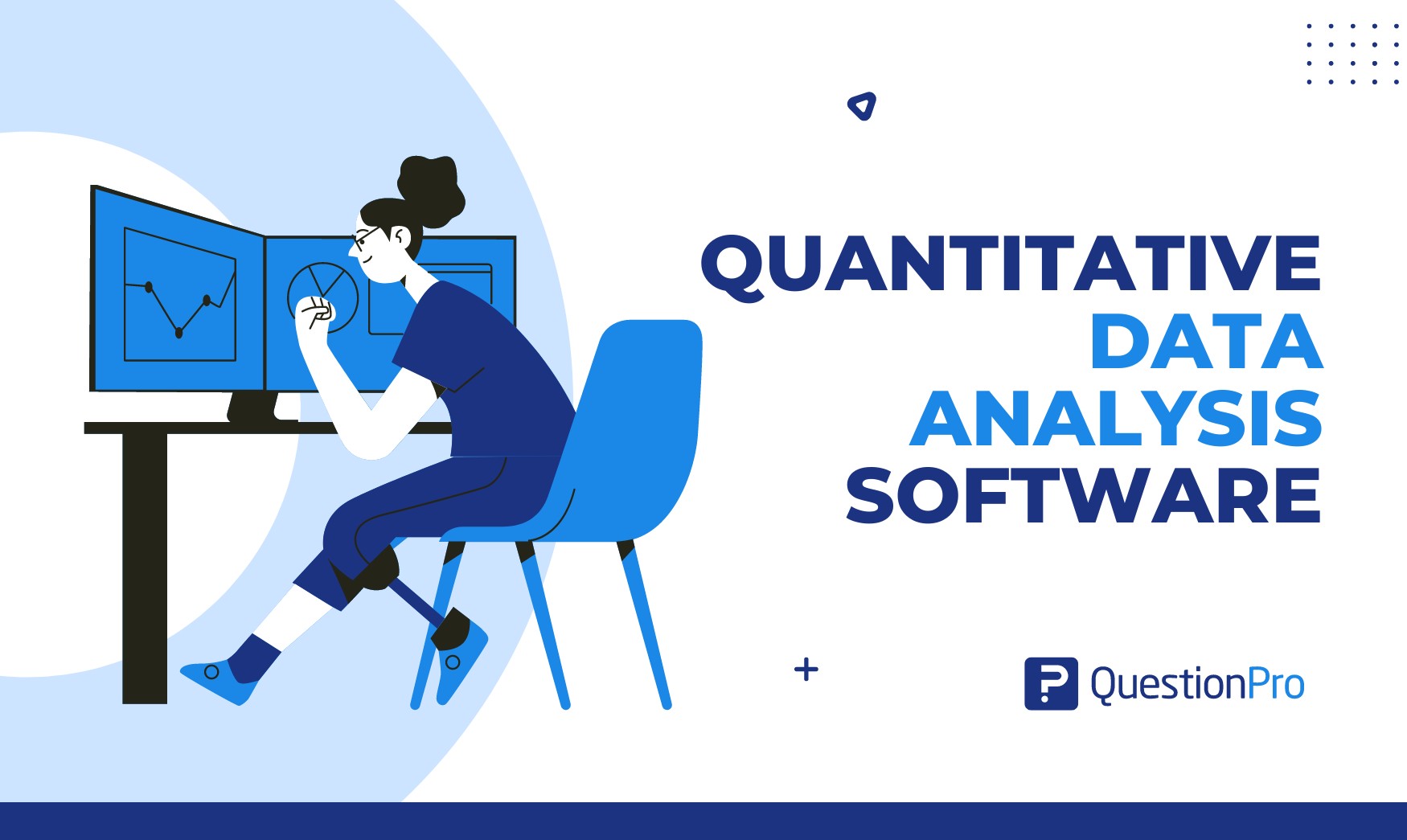
10 Quantitative Data Analysis Software for Every Data Scientist
Apr 18, 2024
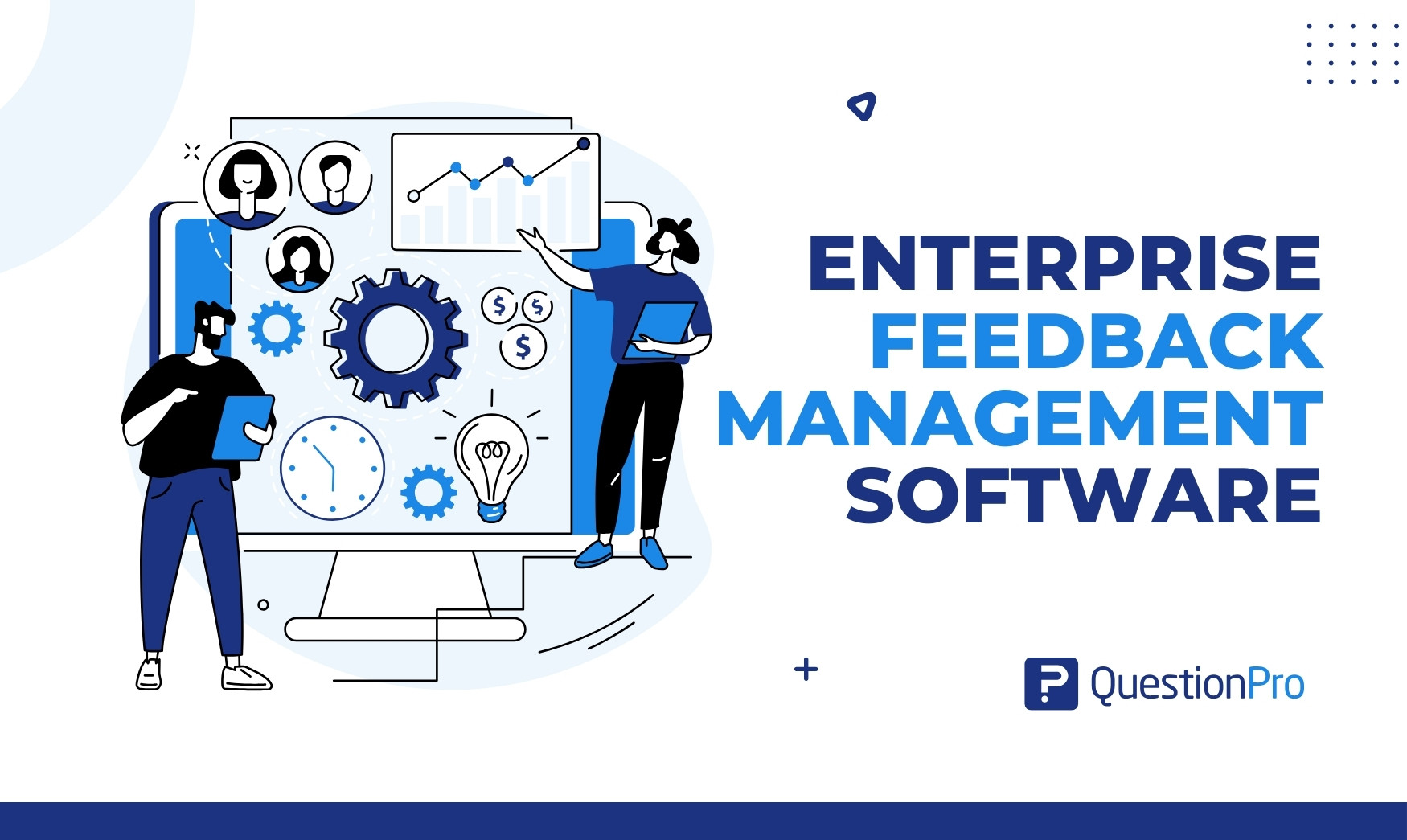
11 Best Enterprise Feedback Management Software in 2024
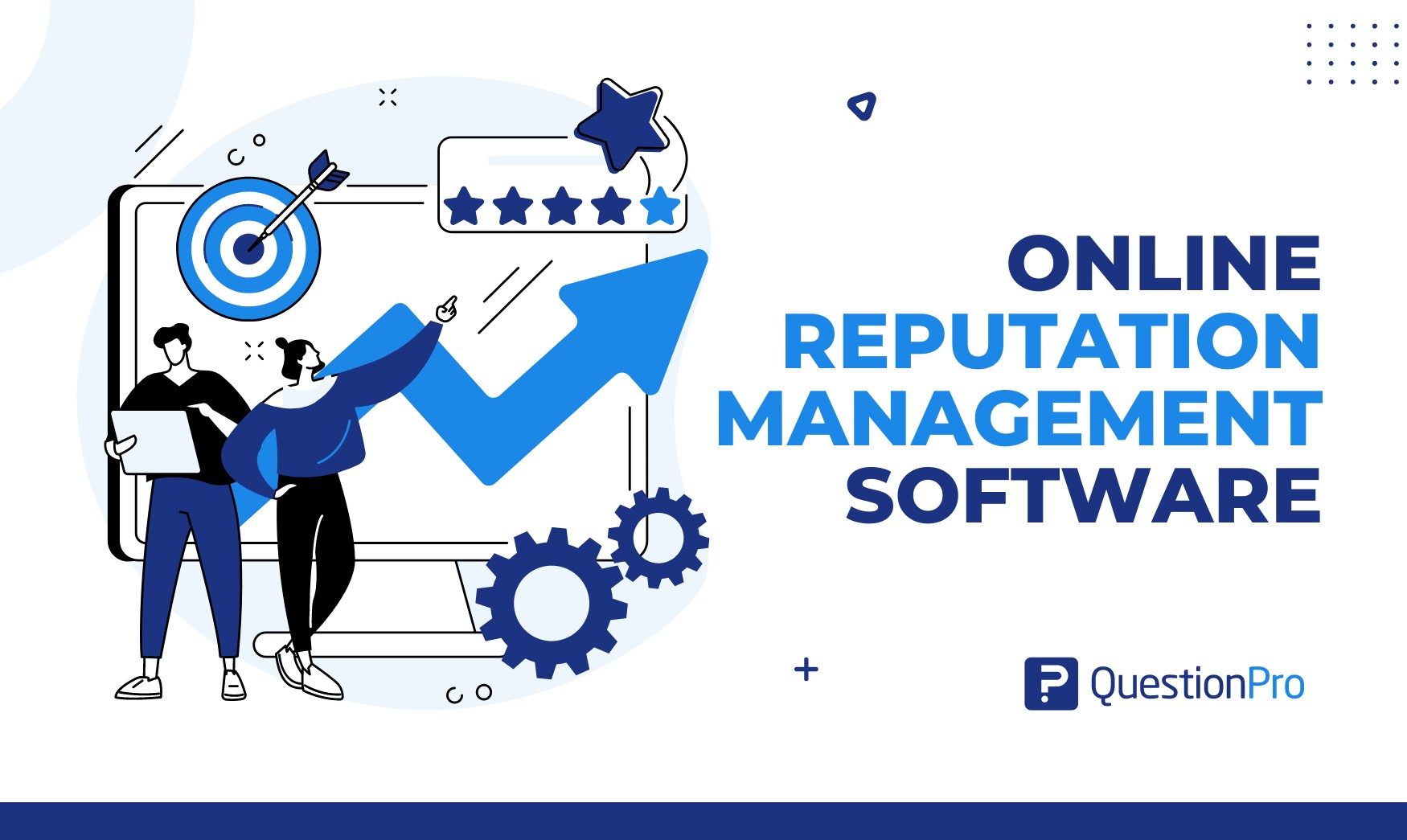
17 Best Online Reputation Management Software in 2024
Apr 17, 2024
Other categories
- Academic Research
- Artificial Intelligence
- Assessments
- Brand Awareness
- Case Studies
- Communities
- Consumer Insights
- Customer effort score
- Customer Engagement
- Customer Experience
- Customer Loyalty
- Customer Research
- Customer Satisfaction
- Employee Benefits
- Employee Engagement
- Employee Retention
- Friday Five
- General Data Protection Regulation
- Insights Hub
- Life@QuestionPro
- Market Research
- Mobile diaries
- Mobile Surveys
- New Features
- Online Communities
- Question Types
- Questionnaire
- QuestionPro Products
- Release Notes
- Research Tools and Apps
- Revenue at Risk
- Survey Templates
- Training Tips
- Uncategorized
- Video Learning Series
- What’s Coming Up
- Workforce Intelligence

IMAGES
VIDEO
COMMENTS
INTRODUCTION. Statistics represent an essential part of a study because, regardless of the study design, investigators need to summarize the collected information for interpretation and presentation to others. It is therefore important for us to heed Mr Twain's concern when creating the data analysis plan. In fact, even before data collection ...
Step 1: Write your hypotheses and plan your research design. To collect valid data for statistical analysis, you first need to specify your hypotheses and plan out your research design. Writing statistical hypotheses. The goal of research is often to investigate a relationship between variables within a population. You start with a prediction ...
A Data Analysis Plan (DAP) is about putting thoughts into a plan of action. Research questions are often framed broadly and need to be clarified and funnelled down into testable hypotheses and action steps. The DAP provides an opportunity for input from collaborators and provides a platform for training. Having a clear plan of action is also ...
A good data analysis plan should summarize the variables as demonstrated in Figure 1 below. Figure 1. Presentation of variables in a data analysis plan. 5. Statistical software. There are tons of software packages for data analysis, some common examples are SPSS, Epi Info, SAS, STATA, Microsoft Excel.
To improve reproducibility, transparency, and validity among clinical trials, the National Institute of Health recently updated its grant application requirements, which mandates registration of clinical trials and submission of the original statistical analysis plan (SAP) along with the research protocol. Many leading journals also require the ...
The SAP (statistical analysis plan) will direct us from the beginning to the conclusion, help us summarize and describe the data, and test our hypotheses. The statistical analysis plan (SAP) describes the intended clinical trial analysis. The SAP is a technical document that describes the statistical methods of research analysis, as opposed to ...
An analysis plan is a description of the steps of the analyses that will be used to understand study objectives (Yuan et al., 2019). The analysis plan is a part of the collaborative process ...
The Statistical Analysis Plan (SAP) is a key document that complements the study protocol in randomized controlled trials (RCT). SAPs are a vital component of transparent, objective, rigorous, reproducible research.
A statistical analysis plan (SAP) is a document that specifies the statistical analysis that will be performed on a given dataset. It serves as a comprehensive guide for the analysis, presenting a clear and organized approach to data analysis that ensures the reliability and validity of the results. SAPs are most widely used in research, data ...
All clinical trials need a statistical analysis plan that guides the analyses processes and sets up the rules to promote research integrity. The plan is initiated and led by the biostatistical team in collaboration with the principal investigator and other key members of the research team. This chapter presents an overview of the contents of ...
Guidelines for Statistical Analysis Plans. The emergence of the randomized clinical trial as the gold standard for the evaluation of new clinical interventions has been met by the emergence of a host of guidelines for the design, conduct, monitoring, analysis, 1 - 3 and reporting 4 of randomized clinical trials including guidance from ...
Get Help. Designing an analysis plan ensures data collection methods meet the needs of the research question, and that the study is accurately powered to produce meaningful results. Based on investigator affiliations and the type of analysis, consultative services are available to discuss statistical methods, analysis software, or potential ...
22.1 Writing the Data Analysis Plan. Congratulations! You have now arrived at one of the most creative and straightforward, sections of your grant proposal. You and your project statistician have one major goal for your data analysis plan: You need to convince all the reviewers reading your proposal that you would know what to do with your data ...
Introduction. Statistical analysis is necessary for any research project seeking to make quantitative conclusions. The following is a primer for research-based statistical analysis. It is intended to be a high-level overview of appropriate statistical testing, while not diving too deep into any specific methodology.
Analysis Plan and Manage Data. The main tasks are as follows: 1. Create an analysis plan • Identify research questions and/or hypotheses. • Select and access a dataset. • List inclusion/exclusion criteria. • Review the data to determine the variables to be used in the main analysis. • Select the appropriate statistical methods and ...
Statistical analysis is useful for research and decision making because it allows us to understand the world around us and draw conclusions by testing our assumptions. Statistical analysis is important for various applications, including: Statistical quality control and analysis in product development. Clinical trials.
Importance While guidance on statistical principles for clinical trials exists, there is an absence of guidance covering the required content of statistical analysis plans (SAPs) to support transparency and reproducibility.. Objective To develop recommendations for a minimum set of items that should be addressed in SAPs for clinical trials, developed with input from statisticians, previous ...
•Data are random numbers. Plan accordingly. • Statistical analysis is the language of scientific inference. Expand your vocabulary. • Statistical analysis is harder than it looks. • Get help now, before you start writing. • Get help while you are writing. • Budget help for later. • When in doubt, call statistician. • When not in doubt, call statistician.
5. Hiring a freelance clinical statistician for help with SAPs. Developing an SAP often requires the support of a freelance clinical statistician. With the help of an experienced biostatistician, you can develop a thorough and error-free SAP, that will improve the quality of your clinical trials.
Importance: While guidance on statistical principles for clinical trials exists, there is an absence of guidance covering the required content of statistical analysis plans (SAPs) to support transparency and reproducibility. Objective: To develop recommendations for a minimum set of items that should be addressed in SAPs for clinical trials, developed with input from statisticians, previous ...
How to Use This Statistical Analysis Plan Template This Statistical Analysis Plan (SAP) template has been created by the Ottawa Methods Centre (OMC), drawing on the recommendations presented in the Guidelines for the Content of Statistical Analysis Plans in Clinical Trials (Gamble C, Krishan A, Stocken D, et al.). Users of
Abstract. Biomedical research has been struck with the problem of study findings that are not reproducible. With the advent of large databases and powerful statistical software, it has become easier to find associations and form conclusions from data without forming an a-priori hypothesis. This approach may yield associations without clinical ...
The Statistical Analysis Plan (SAP) Sample Template for Clinical Trials is a technical document that describes the planned statistical analysis of a clinical trial as outlined in the protocol. ... Michigan Institute for Clinical & Health Research (MICHR) 1600 Huron Parkway, Building 400 Ann Arbor, MI 48109 ...
Definition of research in data analysis: According to LeCompte and Schensul, research data analysis is a process used by researchers to reduce data to a story and interpret it to derive insights. The data analysis process helps reduce a large chunk of data into smaller fragments, which makes sense. Three essential things occur during the data ...
This Statistical Analysis Plan (SAP) describes the planned analyses for the Medication Focused Outpatient Care for Underutilization of Secondary Prevention (MEDFOCUS) study [National Heart, ... Each visit will include a research blood pressure measurement, surveys, phlebotomy for a lipid panel, and HgbA1c. For intervention site study ...