Thank you for visiting nature.com. You are using a browser version with limited support for CSS. To obtain the best experience, we recommend you use a more up to date browser (or turn off compatibility mode in Internet Explorer). In the meantime, to ensure continued support, we are displaying the site without styles and JavaScript.
- View all journals
- Explore content
- About the journal
- Publish with us
- Sign up for alerts
- Open access
- Published: 22 May 2020

Assessing the Big Five personality traits using real-life static facial images
- Alexander Kachur ORCID: orcid.org/0000-0003-1165-2672 1 ,
- Evgeny Osin ORCID: orcid.org/0000-0003-3330-5647 2 ,
- Denis Davydov ORCID: orcid.org/0000-0003-3747-7403 3 ,
- Konstantin Shutilov 4 &
- Alexey Novokshonov 4
Scientific Reports volume 10 , Article number: 8487 ( 2020 ) Cite this article
129k Accesses
48 Citations
349 Altmetric
Metrics details
- Computer science
- Human behaviour
There is ample evidence that morphological and social cues in a human face provide signals of human personality and behaviour. Previous studies have discovered associations between the features of artificial composite facial images and attributions of personality traits by human experts. We present new findings demonstrating the statistically significant prediction of a wider set of personality features (all the Big Five personality traits) for both men and women using real-life static facial images. Volunteer participants (N = 12,447) provided their face photographs (31,367 images) and completed a self-report measure of the Big Five traits. We trained a cascade of artificial neural networks (ANNs) on a large labelled dataset to predict self-reported Big Five scores. The highest correlations between observed and predicted personality scores were found for conscientiousness (0.360 for men and 0.335 for women) and the mean effect size was 0.243, exceeding the results obtained in prior studies using ‘selfies’. The findings strongly support the possibility of predicting multidimensional personality profiles from static facial images using ANNs trained on large labelled datasets. Future research could investigate the relative contribution of morphological features of the face and other characteristics of facial images to predicting personality.
Similar content being viewed by others
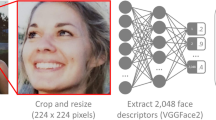
Facial recognition technology can expose political orientation from naturalistic facial images
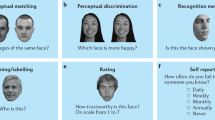
Individual differences and the multidimensional nature of face perception
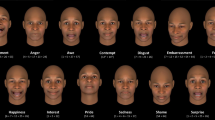
Professional actors demonstrate variability, not stereotypical expressions, when portraying emotional states in photographs
Introduction.
A growing number of studies have linked facial images to personality. It has been established that humans are able to perceive certain personality traits from each other’s faces with some degree of accuracy 1 , 2 , 3 , 4 . In addition to emotional expressions and other nonverbal behaviours conveying information about one’s psychological processes through the face, research has found that valid inferences about personality characteristics can even be made based on static images of the face with a neutral expression 5 , 6 , 7 . These findings suggest that people may use signals from each other’s faces to adjust the ways they communicate, depending on the emotional reactions and perceived personality of the interlocutor. Such signals must be fairly informative and sufficiently repetitive for recipients to take advantage of the information being conveyed 8 .
Studies focusing on the objective characteristics of human faces have found some associations between facial morphology and personality features. For instance, facial symmetry predicts extraversion 9 . Another widely studied indicator is the facial width to height ratio (fWHR), which has been linked to various traits, such as achievement striving 10 , deception 11 , dominance 12 , aggressiveness 13 , 14 , 15 , 16 , and risk-taking 17 . The fWHR can be detected with high reliability irrespective of facial hair. The accuracy of fWHR-based judgements suggests that the human perceptual system may have evolved to be sensitive to static facial features, such as the relative face width 18 .
There are several theoretical reasons to expect associations between facial images and personality. First, genetic background contributes to both face and personality. Genetic correlates of craniofacial characteristics have been discovered both in clinical contexts 19 , 20 and in non-clinical populations 21 . In addition to shaping the face, genes also play a role in the development of various personality traits, such as risky behaviour 22 , 23 , 24 , and the contribution of genes to some traits exceeds the contribution of environmental factors 25 . For the Big Five traits, heritability coefficients reflecting the proportion of variance that can be attributed to genetic factors typically lie in the 0.30–0.60 range 26 , 27 . From an evolutionary perspective, these associations can be expected to have emerged by means of sexual selection. Recent studies have argued that some static facial features, such as the supraorbital region, may have evolved as a means of social communication 28 and that facial attractiveness signalling valuable personality characteristics is associated with mating success 29 .
Second, there is some evidence showing that pre- and postnatal hormones affect both facial shape and personality. For instance, the face is a visible indicator of the levels of sex hormones, such as testosterone and oestrogen, which affect the formation of skull bones and the fWHR 30 , 31 , 32 . Given that prenatal and postnatal sex hormone levels do influence behaviour, facial features may correlate with hormonally driven personality characteristics, such as aggressiveness 33 , competitiveness, and dominance, at least for men 34 , 35 . Thus, in addition to genes, the associations of facial features with behavioural tendencies may also be explained by androgens and potentially other hormones affecting both face and behaviour.
Third, the perception of one’s facial features by oneself and by others influences one’s subsequent behaviour and personality 36 . Just as the perceived ‘cleverness’ of an individual may lead to higher educational attainment 37 , prejudice associated with the shape of one’s face may lead to the development of maladaptive personality characteristics (i.e., the ‘Quasimodo complex’ 38 ). The associations between appearance and personality over the lifespan have been explored in longitudinal observational studies, providing evidence of ‘self-fulfilling prophecy’-type and ‘self-defeating prophecy’-type effects 39 .
Fourth and finally, some personality traits are associated with habitual patterns of emotionally expressive behaviour. Habitual emotional expressions may shape the static features of the face, leading to the formation of wrinkles and/or the development of facial muscles.
Existing studies have revealed the links between objective facial picture cues and general personality traits based on the Five-Factor Model or the Big Five (BF) model of personality 40 . However, a quick glance at the sizes of the effects found in these studies (summarized in Table 1 ) reveals much controversy. The results appear to be inconsistent across studies and hardly replicable 41 . These inconsistencies may result from the use of small samples of stimulus faces, as well as from the vast differences in methodologies. Stronger effect sizes are typically found in studies using composite facial images derived from groups of individuals with high and low scores on each of the Big Five dimensions 6 , 7 , 8 . Naturally, the task of identifying traits using artificial images comprised of contrasting pairs with all other individual features eliminated or held constant appears to be relatively easy. This is in contrast to realistic situations, where faces of individuals reflect a full range of continuous personality characteristics embedded in a variety of individual facial features.
Studies relying on photographic images of individual faces, either artificially manipulated 2 , 42 or realistic, tend to yield more modest effects. It appears that studies using realistic photographs made in controlled conditions (neutral expression, looking straight at the camera, consistent posture, lighting, and distance to the camera, no glasses, no jewellery, no make-up, etc.) produce stronger effects than studies using ‘selfies’ 25 . Unfortunately, differences in the methodologies make it hard to hypothesize whether the diversity of these findings is explained by variance in image quality, image background, or the prediction models used.
Research into the links between facial picture cues and personality traits faces several challenges. First, the number of specific facial features is very large, and some of them are hard to quantify. Second, the effects of isolated facial features are generally weak and only become statistically noticeable in large samples. Third, the associations between objective facial features and personality traits might be interactive and nonlinear. Finally, studies using real-life photographs confront an additional challenge in that the very characteristics of the images (e.g., the angle of the head, facial expression, makeup, hairstyle, facial hair style, etc.) are based on the subjects’ choices, which are potentially influenced by personality; after all, one of the principal reasons why people make and share their photographs is to signal to others what kind of person they are. The task of isolating the contribution of each variable out of the multitude of these individual variables appears to be hardly feasible. Instead, recent studies in the field have tended to rely on a holistic approach, investigating the subjective perception of personality based on integral facial images.
The holistic approach aims to mimic the mechanisms of human perception of the face and the ways in which people make judgements about each other’s personality. This approach is supported by studies of human face perception, showing that faces are perceived and encoded in a holistic manner by the human brain 43 , 44 , 45 , 46 . Put differently, when people identify others, they consider individual facial features (such as a person’s eyes, nose, and mouth) in concert as a single entity rather than as independent pieces of information 47 , 48 , 49 , 50 . Similar to facial identification, personality judgements involve the extraction of invariant facial markers associated with relatively stable characteristics of an individual’s behaviour. Existing evidence suggests that various social judgements might be based on a common visual representational system involving the holistic processing of visual information 51 , 52 . Thus, even though the associations between isolated facial features and personality characteristics sought by ancient physiognomists have emerged to be weak, contradictory or even non-existent, the holistic approach to understanding the face-personality links appears to be more promising.
An additional challenge faced by studies seeking to reveal the face-personality links is constituted by the inconsistency of the evaluations of personality traits by human raters. As a result, a fairly large number of human raters is required to obtain reliable estimates of personality traits for each photograph. In contrast, recent attempts at using machine learning algorithms have suggested that artificial intelligence may outperform individual human raters. For instance, S. Hu and colleagues 40 used the composite partial least squares component approach to analyse dense 3D facial images obtained in controlled conditions and found significant associations with personality traits (stronger for men than for women).
A similar approach can be implemented using advanced machine learning algorithms, such as artificial neural networks (ANNs), which can extract and process significant features in a holistic manner. The recent applications of ANNs to the analysis of human faces, body postures, and behaviours with the purpose of inferring apparent personality traits 53 , 54 indicate that this approach leads to a higher accuracy of prediction compared to individual human raters. The main difficulty of the ANN approach is the need for large labelled training datasets that are difficult to obtain in laboratory settings. However, ANNs do not require high-quality photographs taken in controlled conditions and can potentially be trained using real-life photographs provided that the dataset is large enough. The interpretation of findings in such studies needs to acknowledge that a real-life photograph, especially one chosen by a study participant, can be viewed as a holistic behavioural act, which may potentially contain other cues to the subjects’ personality in addition to static facial features (e.g., lighting, hairstyle, head angle, picture quality, etc.).
The purpose of the current study was to investigate the associations of facial picture cues with self-reported Big Five personality traits by training a cascade of ANNs to predict personality traits from static facial images. The general hypothesis is that a real-life photograph contains cues about personality that can be extracted using machine learning. Due to the vast diversity of findings concerning the prediction accuracy of different traits across previous studies, we did not set a priori hypotheses about differences in prediction accuracy across traits.
Prediction accuracy
We used data from the test dataset containing predicted scores for 3,137 images associated with 1,245 individuals. To determine whether the variance in the predicted scores was associated with differences across images or across individuals, we calculated the intraclass correlation coefficients (ICCs) presented in Table 2 . The between-individual proportion of variance in the predicted scores ranged from 79 to 88% for different traits, indicating a general consistency of predicted scores for different photographs of the same individual. We derived the individual scores used in all subsequent analyses as the simple averages of the predicted scores for all images provided by each participant.
The correlation coefficients between the self-report test scores and the scores predicted by the ANN ranged from 0.14 to 0.36. The associations were strongest for conscientiousness and weakest for openness. Extraversion and neuroticism were significantly better predicted for women than for men (based on the z test). We also compared the prediction accuracy within each gender using Steiger’s test for dependent sample correlation coefficients. For men, conscientiousness was predicted more accurately than the other four traits (the differences among the latter were not statistically significant). For women, conscientiousness was predicted more accurately, and openness was predicted less accurately compared to the three other traits.
The mean absolute error (MAE) of prediction ranged between 0.89 and 1.04 standard deviations. We did not find any associations between the number of photographs and prediction error.
Trait intercorrelations
The structure of the correlations between the scales was generally similar for the observed test scores and the predicted values, but some coefficients differed significantly (based on the z test) (see Table 3 ). Most notably, predicted openness was more strongly associated with conscientiousness (negatively) and extraversion (positively), whereas its association with agreeableness was negative rather than positive. The associations of predicted agreeableness with conscientiousness and neuroticism were stronger than those between the respective observed scores. In women, predicted neuroticism demonstrated a stronger inverse association with conscientiousness and a stronger positive association with openness. In men, predicted neuroticism was less strongly associated with extraversion than its observed counterpart.
To illustrate the findings, we created composite images using Abrosoft FantaMorph 5 by averaging the uploaded images across contrast groups of 100 individuals with the highest and the lowest test scores on each trait. The resulting morphed images in which individual features are eliminated are presented in Fig. 1 .
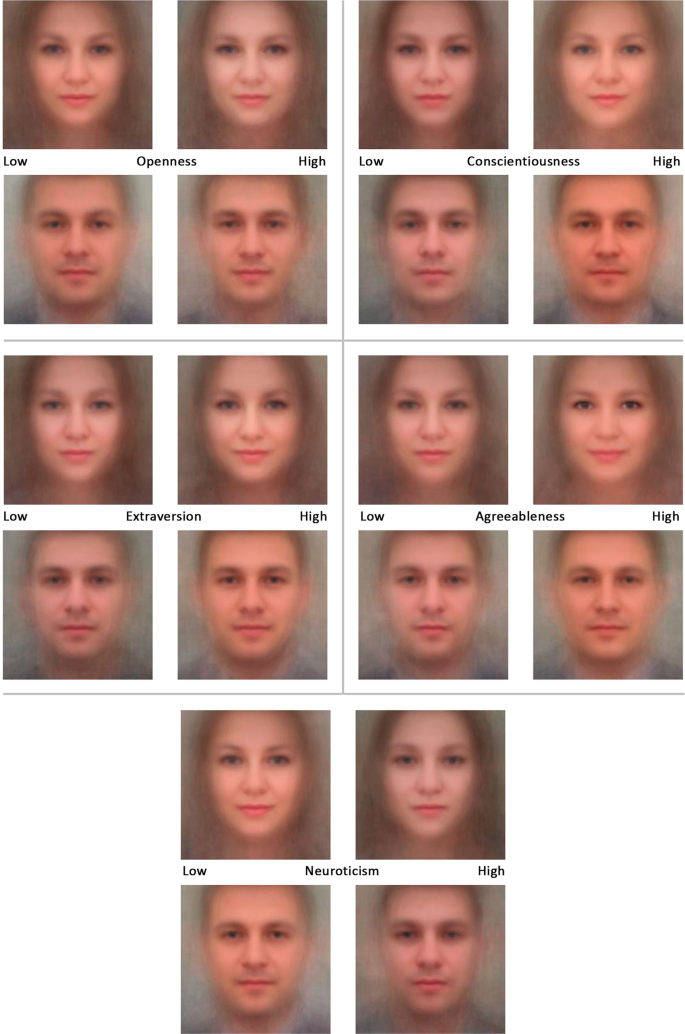
Composite facial images morphed across contrast groups of 100 individuals for each Big Five trait.
This study presents new evidence confirming that human personality is related to individual facial appearance. We expected that machine learning (in our case, artificial neural networks) could reveal multidimensional personality profiles based on static morphological facial features. We circumvented the reliability limitations of human raters by developing a neural network and training it on a large dataset labelled with self-reported Big Five traits.
We expected that personality traits would be reflected in the whole facial image rather than in its isolated features. Based on this expectation, we developed a novel two-tier machine learning algorithm to encode the invariant facial features as a vector in a 128-dimensional space that was used to predict the BF traits by means of a multilayer perceptron. Although studies using real-life photographs do not require strict experimental conditions, we had to undertake a series of additional organizational and technological steps to ensure consistent facial image characteristics and quality.
Our results demonstrate that real-life photographs taken in uncontrolled conditions can be used to predict personality traits using complex computer vision algorithms. This finding is in contrast to previous studies that mostly relied on high-quality facial images taken in controlled settings. The accuracy of prediction that we obtained exceeds that in the findings of prior studies that used realistic individual photographs taken in uncontrolled conditions (e.g., selfies 55 ). The advantage of our methodology is that it is relatively simple (e.g., it does not rely on 3D scanners or 3D facial landmark maps) and can be easily implemented using a desktop computer with a stock graphics accelerator.
In the present study, conscientiousness emerged to be more easily recognizable than the other four traits, which is consistent with some of the existing findings 7 , 40 . The weaker effects for extraversion and neuroticism found in our sample may be because these traits are associated with positive and negative emotional experiences, whereas we only aimed to use images with neutral or close to neutral emotional expressions. Finally, this appears to be the first study to achieve a significant prediction of openness to experience. Predictions of personality based on female faces appeared to be more reliable than those for male faces in our sample, in contrast to some previous studies 40 .
The BF factors are known to be non-orthogonal, and we paid attention to their intercorrelations in our study 56 , 57 . Various models have attempted to explain the BF using higher-order dimensions, such as stability and plasticity 58 or a single general factor of personality (GFP) 59 . We discovered that the intercorrelations of predicted factors tend to be stronger than the intercorrelations of self-report questionnaire scales used to train the model. This finding suggests a potential biological basis of GFP. However, the stronger intercorrelations of the predicted scores can be explained by consistent differences in picture quality (just as the correlations between the self-report scales can be explained by social desirability effects and other varieties of response bias 60 ). Clearly, additional research is needed to understand the context of this finding.
We believe that the present study, which did not involve any subjective human raters, constitutes solid evidence that all the Big Five traits are associated with facial cues that can be extracted using machine learning algorithms. However, despite having taken reasonable organizational and technical steps to exclude the potential confounds and focus on static facial features, we are still unable to claim that morphological features of the face explain all the personality-related image variance captured by the ANNs. Rather, we propose to see facial photographs taken by subjects themselves as complex behavioural acts that can be evaluated holistically and that may contain various other subtle personality cues in addition to static facial features.
The correlations reported above with a mean r = 0.243 can be viewed as modest; indeed, facial image-based personality assessment can hardly replace traditional personality measures. However, this effect size indicates that an ANN can make a correct guess about the relative standing of two randomly chosen individuals on a personality dimension in 58% of cases (as opposed to the 50% expected by chance) 61 . The effect sizes we observed are comparable with the meta-analytic estimates of correlations between self-reported and observer ratings of personality traits: the associations range from 0.30 to 0.49 when one’s personality is rated by close relatives or colleagues, but only from −0.01 to 0.29 when rated by strangers 62 . Thus, an artificial neural network relying on static facial images outperforms an average human rater who meets the target in person without any prior acquaintance. Given that partner personality and match between two personalities predict friendship formation 63 , long-term relationship satisfaction 64 , and the outcomes of dyadic interaction in unstructured settings 65 , the aid of artificial intelligence in making partner choices could help individuals to achieve more satisfying interaction outcomes.
There are a vast number of potential applications to be explored. The recognition of personality from real-life photos can be applied in a wide range of scenarios, complementing the traditional approaches to personality assessment in settings where speed is more important than accuracy. Applications may include suggesting best-fitting products or services to customers, proposing to individuals a best match in dyadic interaction settings (such as business negotiations, online teaching, etc.) or personalizing the human-computer interaction. Given that the practical value of any selection method is proportional to the number of decisions made and the size and variability of the pool of potential choices 66 , we believe that the applied potential of this technology can be easily revealed at a large scale, given its speed and low cost. Because the reliability and validity of self-report personality measures is not perfect, prediction could be further improved by supplementing these measures with peer ratings and objective behavioural indicators of personality traits.
The fact that conscientiousness was predicted better than the other traits for both men and women emerges as an interesting finding. From an evolutionary perspective, one would expect the traits most relevant for cooperation (conscientiousness and agreeableness) and social interaction (certain facets of extraversion and neuroticism, such as sociability, dominance, or hostility) to be reflected more readily in the human face. The results are generally in line with this idea, but they need to be replicated and extended by incorporating trait facets in future studies to provide support for this hypothesis.
Finally, although we tried to control the potential sources of confounds and errors by instructing the participants and by screening the photographs (based on angles, facial expressions, makeup, etc.), the present study is not without limitations. First, the real-life photographs we used could still carry a variety of subtle cues, such as makeup, angle, light facial expressions, and information related to all the other choices people make when they take and share their own photographs. These additional cues could say something about their personality, and the effects of all these variables are inseparable from those of static facial features, making it hard to draw any fundamental conclusions from the findings. However, studies using real-life photographs may have higher ecological validity compared to laboratory studies; our results are more likely to generalize to real-life situations where users of various services are asked to share self-pictures of their choice.
Another limitation pertains to a geographically bounded sample of individuals; our participants were mostly Caucasian and represented one cultural and age group (Russian-speaking adults). Future studies could replicate the effects using populations representing a more diverse variety of ethnic, cultural, and age groups. Studies relying on other sources of personality data (e.g., peer ratings or expert ratings), as well as wider sets of personality traits, could complement and extend the present findings.
Sample and procedure
The study was carried out in the Russian language. The participants were anonymous volunteers recruited through social network advertisements. They did not receive any financial remuneration but were provided with a free report on their Big Five personality traits. The data were collected online using a dedicated research website and a mobile application. The participants provided their informed consent, completed the questionnaires, reported their age and gender and were asked to upload their photographs. They were instructed to take or upload several photographs of their face looking directly at the camera with enough lighting, a neutral facial expression and no other people in the picture and without makeup.
Our goal was to obtain an out-of-sample validation dataset of 616 respondents of each gender to achieve 80% power for a minimum effect we considered to be of practical significance ( r = 0.10 at p < 0.05), requiring a total of 6,160 participants of each gender in the combined dataset comprising the training and validation datasets. However, we aimed to gather more data because we expected that some online respondents might provide low-quality or non-genuine photographs and/or invalid questionnaire responses.
The initial sample included 25,202 participants who completed the questionnaire and uploaded a total of 77,346 photographs. The final combined dataset comprised 12,447 valid questionnaires and 31,367 associated photographs after the data screening procedures (below). The participants ranged in age from 18 to 60 (59.4% women, M = 27.61, SD = 12.73, and 40.6% men, M = 32.60, SD = 11.85). The dataset was split randomly into a training dataset (90%) and a test dataset (10%) used to validate the prediction model. The validation dataset included the responses of 505 men who provided 1224 facial images and 740 women who provided 1913 images. Due to the sexually dimorphic nature of facial features and certain personality traits (particularly extraversion 1 , 67 , 68 ), all the predictive models were trained and validated separately for male and female faces.
Ethical approval
The research was carried out in accordance with the Declaration of Helsinki. The study protocol was approved by the Research Ethics Committee of the Open University for the Humanities and Economics. We obtained the participants’ informed consent to use their data and photographs for research purposes and to publish generalized findings. The morphed group average images presented in the paper do not allow the identification of individuals. No information or images that could lead to the identification of study participants have been published.
Data screening
We excluded incomplete questionnaires (N = 3,035) and used indices of response consistency to screen out random responders 69 . To detect systematic careless responses, we used the modal response category count, maximum longstring (maximum number of identical responses given in sequence by participant), and inter-item standard deviation for each questionnaire. At this stage, we screened out the answers of individuals with zero standard deviations (N = 329) and a maximum longstring above 10 (N = 1,416). To detect random responses, we calculated the following person-fit indices: the person-total response profile correlation, the consistency of response profiles for the first and the second half of the questionnaire, the consistency of response profiles obtained based on equivalent groups of items, the number of polytomous Guttman errors, and the intraclass correlation of item responses within facets.
Next, we conducted a simulation by generating random sets of integers in the 1–5 range based on a normal distribution (µ = 3, σ = 1) and on the uniform distribution and calculating the same person-fit indices. For each distribution, we generated a training dataset and a test dataset, each comprised of 1,000 simulated responses and 1,000 real responses drawn randomly from the sample. Next, we ran a logistic regression model using simulated vs real responses as the outcome variable and chose an optimal cutoff point to minimize the misclassification error (using the R package optcutoff). The sensitivity value was 0.991 for the uniform distribution and 0.960 for the normal distribution, and the specificity values were 0.923 and 0.980, respectively. Finally, we applied the trained model to the full dataset and identified observations predicted as likely to be simulated based on either distribution (N = 1,618). The remaining sample of responses (N = 18,804) was used in the subsequent analyses.
Big Five measure
We used a modified Russian version of the 5PFQ questionnaire 70 , which is a 75-item measure of the Big Five model, with 15 items per trait grouped into five three-item facets. To confirm the structural validity of the questionnaire, we tested an exploratory structural equation (ESEM) model with target rotation in Mplus 8.2. The items were treated as ordered categorical variables using the WLSMV estimator, and facet variance was modelled by introducing correlated uniqueness values for the items comprising each facet.
The theoretical model showed a good fit to the data (χ 2 = 147854.68, df = 2335, p < 0.001; CFI = 0.931; RMSEA = 0.040 [90% CI: 0.040, 0.041]; SRMR = 0.024). All the items showed statistically significant loadings on their theoretically expected scales (λ ranged from 0.14 to 0.87, M = 0.51, SD = 0.17), and the absolute cross-loadings were reasonably low (M = 0.11, SD = 0.11). The distributions of the resulting scales were approximately normal (with skewness and kurtosis values within the [−1; 1] range). To assess the reliability of the scales, we calculated two internal consistency indices, namely, robust omega (using the R package coefficientalpha) and algebraic greatest lower bound (GLB) reliability (using the R package psych) 71 (see Table 4 ).
Image screening and pre-processing
The images (photographs and video frames) were subjected to a three-step screening procedure aimed at removing fake and low-quality images. First, images with no human faces or with more than one human face were detected by our computer vision (CV) algorithms and automatically removed. Second, celebrity images were identified and removed by means of a dedicated neural network trained on a celebrity photo dataset (CelebFaces Attributes Dataset (CelebA), N > 200,000) 72 that was additionally enriched with pictures of Russian celebrities. The model showed a 98.4% detection accuracy. Third, we performed a manual moderation of the remaining images to remove images with partially covered faces, those that were evidently photoshopped or any other fake images not detected by CV.
The images retained for subsequent processing were converted to single-channel 8-bit greyscale format using the OpenCV framework (opencv.org). Head position (pitch, yaw, roll) was measured using our own dedicated neural network (multilayer perceptron) trained on a sample of 8 000 images labelled by our team. The mean absolute error achieved on the test sample of 800 images was 2.78° for roll, 1.67° for pitch, and 2.34° for yaw. We used the head position data to retain the images with yaw and roll within the −30° to 30° range and pitch within the −15° to 15° range.
Next, we assessed emotional neutrality using the Microsoft Cognitive Services API on the Azure platform (score range: 0 to 1) and used 0.50 as a threshold criterion to remove emotionally expressive images. Finally, we applied the face and eye detection, alignment, resize, and crop functions available within the Dlib (dlib.net) open-source toolkit to arrive at a set of standardized 224 × 224 pixel images with eye pupils aligned to a standard position with an accuracy of 1 px. Images with low resolution that contained less than 60 pixels between the eyes, were excluded in the process.
The final photoset comprised 41,835 images. After the screened questionnaire responses and images were joined, we obtained a set of 12,447 valid Big Five questionnaires associated with 31,367 validated images (an average of 2.59 images per person for women and 2.42 for men).
Neural network architecture
First, we developed a computer vision neural network (NNCV) aiming to determine the invariant features of static facial images that distinguish one face from another but remain constant across different images of the same person. We aimed to choose a neural network architecture with a good feature space and resource-efficient learning, considering the limited hardware available to our research team. We chose a residual network architecture based on ResNet 73 (see Fig. 2 ).
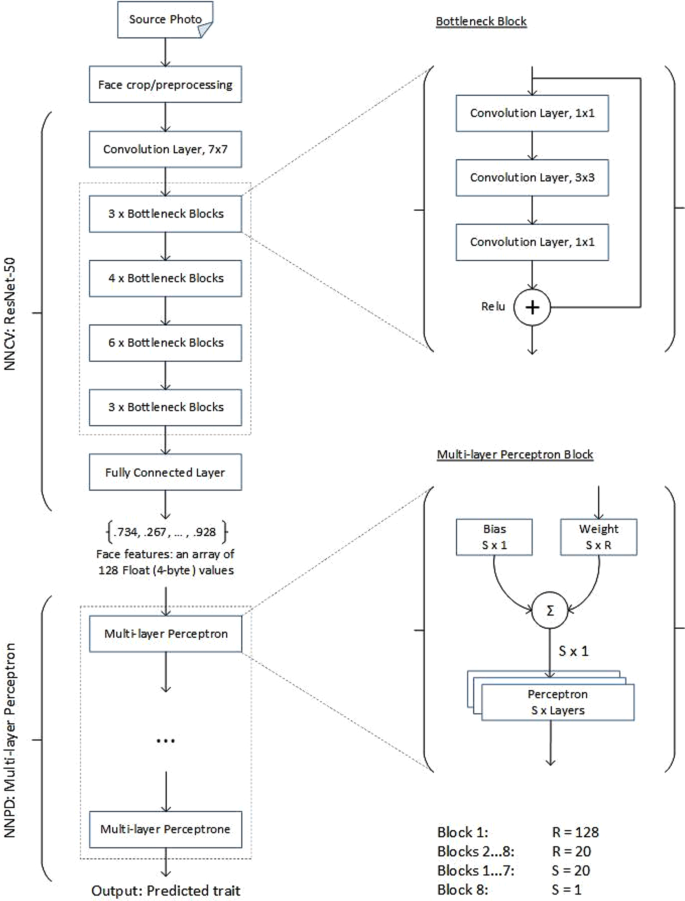
Layer architecture of the computer vision neural network (NNCV) and the personality diagnostics neural network (NNPD).
This type of neural network was originally developed for image classification. We dropped the final layer from the original architecture and obtained a NNCV that takes a static monochrome image (224 × 224 pixels in size) and generates a vector of 128 32-bit dimensions describing unique facial features in the source image. As a measure of success, we calculated the Euclidean distance between the vectors generated from different images.
Using Internet search engines, we collected a training dataset of approximately 2 million openly available unlabelled real-life photos taken in uncontrolled conditions stratified by race, age and gender (using search engine queries such as ‘face photo’, ‘face pictures’, etc.). The training was conducted on a server equipped with four NVidia Titan accelerators. The trained neural network was validated on a dataset of 40,000 images belonging to 800 people, which was an out-of-sample part of the original dataset. The Euclidean distance threshold for the vectors belonging to the same person was 0.40 after the training was complete.
Finally, we trained a personality diagnostics neural network (NNPD), which was implemented as a multilayer perceptron (see Fig. 2 ). For that purpose, we used a training dataset (90% of the final sample) containing the questionnaire scores of 11,202 respondents and a total of 28,230 associated photographs. The NNPD takes the vector of the invariants obtained from NNCV as an input and predicts the Big Five personality traits as the output. The network was trained using the same hardware, and the training process took 9 days. The whole process was performed for male and female faces separately.
Data availability
The set of photographs is not made available because we did not solicit the consent of the study participants to publish the individual photographs. The test dataset with the observed and predicted Big Five scores is available from the openICPSR repository: https://doi.org/10.3886/E109082V1 .
Kramer, R. S. S., King, J. E. & Ward, R. Identifying personality from the static, nonexpressive face in humans and chimpanzees: Evidence of a shared system for signaling personality. Evol. Hum. Behav . https://doi.org/10.1016/j.evolhumbehav.2010.10.005 (2011).
Walker, M. & Vetter, T. Changing the personality of a face: Perceived big two and big five personality factors modeled in real photographs. J. Pers. Soc. Psychol. 110 , 609–624 (2016).
Article Google Scholar
Naumann, L. P., Vazire, S., Rentfrow, P. J. & Gosling, S. D. Personality Judgments Based on Physical Appearance. Personal. Soc. Psychol. Bull. 35 , 1661–1671 (2009).
Borkenau, P., Brecke, S., Möttig, C. & Paelecke, M. Extraversion is accurately perceived after a 50-ms exposure to a face. J. Res. Pers. 43 , 703–706 (2009).
Shevlin, M., Walker, S., Davies, M. N. O., Banyard, P. & Lewis, C. A. Can you judge a book by its cover? Evidence of self-stranger agreement on personality at zero acquaintance. Pers. Individ. Dif . https://doi.org/10.1016/S0191-8869(02)00356-2 (2003).
Penton-Voak, I. S., Pound, N., Little, A. C. & Perrett, D. I. Personality Judgments from Natural and Composite Facial Images: More Evidence For A “Kernel Of Truth” In Social Perception. Soc. Cogn. 24 , 607–640 (2006).
Little, A. C. & Perrett, D. I. Using composite images to assess accuracy in personality attribution to faces. Br. J. Psychol. 98 , 111–126 (2007).
Kramer, R. S. S. & Ward, R. Internal Facial Features are Signals of Personality and Health. Q. J. Exp. Psychol. 63 , 2273–2287 (2010).
Pound, N., Penton-Voak, I. S. & Brown, W. M. Facial symmetry is positively associated with self-reported extraversion. Pers. Individ. Dif. 43 , 1572–1582 (2007).
Lewis, G. J., Lefevre, C. E. & Bates, T. Facial width-to-height ratio predicts achievement drive in US presidents. Pers. Individ. Dif. 52 , 855–857 (2012).
Haselhuhn, M. P. & Wong, E. M. Bad to the bone: facial structure predicts unethical behaviour. Proc. R. Soc. B Biol. Sci. 279 , 571 LP–576 (2012).
Valentine, K. A., Li, N. P., Penke, L. & Perrett, D. I. Judging a Man by the Width of His Face: The Role of Facial Ratios and Dominance in Mate Choice at Speed-Dating Events. Psychol. Sci . 25 , (2014).
Carre, J. M. & McCormick, C. M. In your face: facial metrics predict aggressive behaviour in the laboratory and in varsity and professional hockey players. Proc. R. Soc. B Biol. Sci. 275 , 2651–2656 (2008).
Carré, J. M., McCormick, C. M. & Mondloch, C. J. Facial structure is a reliable cue of aggressive behavior: Research report. Psychol. Sci . https://doi.org/10.1111/j.1467-9280.2009.02423.x (2009).
Haselhuhn, M. P., Ormiston, M. E. & Wong, E. M. Men’s Facial Width-to-Height Ratio Predicts Aggression: A Meta-Analysis. PLoS One 10 , e0122637 (2015).
Lefevre, C. E., Etchells, P. J., Howell, E. C., Clark, A. P. & Penton-Voak, I. S. Facial width-to-height ratio predicts self-reported dominance and aggression in males and females, but a measure of masculinity does not. Biol. Lett . 10 , (2014).
Welker, K. M., Goetz, S. M. M. & Carré, J. M. Perceived and experimentally manipulated status moderates the relationship between facial structure and risk-taking. Evol. Hum. Behav . https://doi.org/10.1016/j.evolhumbehav.2015.03.006 (2015).
Geniole, S. N. & McCormick, C. M. Facing our ancestors: judgements of aggression are consistent and related to the facial width-to-height ratio in men irrespective of beards. Evol. Hum. Behav. 36 , 279–285 (2015).
Valentine, M. et al . Computer-Aided Recognition of Facial Attributes for Fetal Alcohol Spectrum Disorders. Pediatrics 140 , (2017).
Ferry, Q. et al . Diagnostically relevant facial gestalt information from ordinary photos. Elife 1–22 https://doi.org/10.7554/eLife.02020.001 (2014).
Claes, P. et al . Modeling 3D Facial Shape from DNA. PLoS Genet. 10 , e1004224 (2014).
Carpenter, J. P., Garcia, J. R. & Lum, J. K. Dopamine receptor genes predict risk preferences, time preferences, and related economic choices. J. Risk Uncertain. 42 , 233–261 (2011).
Dreber, A. et al . The 7R polymorphism in the dopamine receptor D4 gene (<em>DRD4</em>) is associated with financial risk taking in men. Evol. Hum. Behav. 30 , 85–92 (2009).
Bouchard, T. J. et al . Sources of human psychological differences: the Minnesota Study of Twins Reared Apart. Science (80-.). 250 , 223 LP–228 (1990).
Article ADS Google Scholar
Livesley, W. J., Jang, K. L. & Vernon, P. A. Phenotypic and genetic structure of traits delineating personality disorder. Arch. Gen. Psychiatry https://doi.org/10.1001/archpsyc.55.10.941 (1998).
Bouchard, T. J. & Loehlin, J. C. Genes, evolution, and personality. Behavior Genetics https://doi.org/10.1023/A:1012294324713 (2001).
Vukasović, T. & Bratko, D. Heritability of personality: A meta-analysis of behavior genetic studies. Psychol. Bull. 141 , 769–785 (2015).
Godinho, R. M., Spikins, P. & O’Higgins, P. Supraorbital morphology and social dynamics in human evolution. Nat. Ecol. Evol . https://doi.org/10.1038/s41559-018-0528-0 (2018).
Rhodes, G., Simmons, L. W. & Peters, M. Attractiveness and sexual behavior: Does attractiveness enhance mating success? Evol. Hum. Behav . https://doi.org/10.1016/j.evolhumbehav.2004.08.014 (2005).
Lefevre, C. E., Lewis, G. J., Perrett, D. I. & Penke, L. Telling facial metrics: Facial width is associated with testosterone levels in men. Evol. Hum. Behav. 34 , 273–279 (2013).
Whitehouse, A. J. O. et al . Prenatal testosterone exposure is related to sexually dimorphic facial morphology in adulthood. Proceedings. Biol. Sci. 282 , 20151351 (2015).
Penton-Voak, I. S. & Chen, J. Y. High salivary testosterone is linked to masculine male facial appearance in humans. Evol. Hum. Behav . https://doi.org/10.1016/j.evolhumbehav.2004.04.003 (2004).
Carré, J. M. & Archer, J. Testosterone and human behavior: the role of individual and contextual variables. Curr. Opin. Psychol. 19 , 149–153 (2018).
Swaddle, J. P. & Reierson, G. W. Testosterone increases perceived dominance but not attractiveness in human males. Proc. R. Soc. B Biol. Sci . https://doi.org/10.1098/rspb.2002.2165 (2002).
Eisenegger, C., Kumsta, R., Naef, M., Gromoll, J. & Heinrichs, M. Testosterone and androgen receptor gene polymorphism are associated with confidence and competitiveness in men. Horm. Behav. 92 , 93–102 (2017).
Article CAS Google Scholar
Kaplan, H. B. Social Psychology of Self-Referent Behavior . https://doi.org/10.1007/978-1-4899-2233-5 . (Springer US, 1986).
Rosenthal, R. & Jacobson, L. Pygmalion in the classroom. Urban Rev . https://doi.org/10.1007/BF02322211 (1968).
Masters, F. W. & Greaves, D. C. The Quasimodo complex. Br. J. Plast. Surg . 204–210 (1967).
Zebrowitz, L. A., Collins, M. A. & Dutta, R. The Relationship between Appearance and Personality Across the Life Span. Personal. Soc. Psychol. Bull. 24 , 736–749 (1998).
Hu, S. et al . Signatures of personality on dense 3D facial images. Sci. Rep. 7 , 73 (2017).
Kosinski, M. Facial Width-to-Height Ratio Does Not Predict Self-Reported Behavioral Tendencies. Psychol. Sci. 28 , 1675–1682 (2017).
Walker, M., Schönborn, S., Greifeneder, R. & Vetter, T. The basel face database: A validated set of photographs reflecting systematic differences in big two and big five personality dimensions. PLoS One 13 , (2018).
Goffaux, V. & Rossion, B. Faces are ‘spatial’ - Holistic face perception is supported by low spatial frequencies. J. Exp. Psychol. Hum. Percept. Perform . https://doi.org/10.1037/0096-1523.32.4.1023 (2006).
Schiltz, C. & Rossion, B. Faces are represented holistically in the human occipito-temporal cortex. Neuroimage https://doi.org/10.1016/j.neuroimage.2006.05.037 (2006).
Van Belle, G., De Graef, P., Verfaillie, K., Busigny, T. & Rossion, B. Whole not hole: Expert face recognition requires holistic perception. Neuropsychologia https://doi.org/10.1016/j.neuropsychologia.2010.04.034 (2010).
Quadflieg, S., Todorov, A., Laguesse, R. & Rossion, B. Normal face-based judgements of social characteristics despite severely impaired holistic face processing. Vis. cogn. 20 , 865–882 (2012).
McKone, E. Isolating the Special Component of Face Recognition: Peripheral Identification and a Mooney Face. J. Exp. Psychol. Learn. Mem. Cogn . https://doi.org/10.1037/0278-7393.30.1.181 (2004).
Sergent, J. An investigation into component and configural processes underlying face perception. Br. J. Psychol . https://doi.org/10.1111/j.2044-8295.1984.tb01895.x (1984).
Tanaka, J. W. & Farah, M. J. Parts and Wholes in Face Recognition. Q. J. Exp. Psychol. Sect. A https://doi.org/10.1080/14640749308401045 (1993).
Young, A. W., Hellawell, D. & Hay, D. C. Configurational information in face perception. Perception https://doi.org/10.1068/p160747n (2013).
Calder, A. J. & Young, A. W. Understanding the recognition of facial identity and facial expression. Nature Reviews Neuroscience https://doi.org/10.1038/nrn1724 (2005).
Todorov, A., Loehr, V. & Oosterhof, N. N. The obligatory nature of holistic processing of faces in social judgments. Perception https://doi.org/10.1068/p6501 (2010).
Junior, J. C. S. J. et al . First Impressions: A Survey on Computer Vision-Based Apparent Personality Trait Analysis. (2018).
Wang, Y. & Kosinski, M. Deep neural networks are more accurate than humans at detecting sexual orientation from facial images. J. Pers. Soc. Psychol. 114 , 246–257 (2018).
Qiu, L., Lu, J., Yang, S., Qu, W. & Zhu, T. What does your selfie say about you? Comput. Human Behav. 52 , 443–449 (2015).
Digman, J. M. Higher order factors of the Big Five. J.Pers.Soc.Psychol . https://doi.org/10.1037/0022-3514.73.6.1246 (1997).
Musek, J. A general factor of personality: Evidence for the Big One in the five-factor model. J. Res. Pers . https://doi.org/10.1016/j.jrp.2007.02.003 (2007).
DeYoung, C. G. Higher-order factors of the Big Five in a multi-informant sample. J. Pers. Soc. Psychol. 91 , 1138–1151 (2006).
Rushton, J. P. & Irwing, P. A General Factor of Personality (GFP) from two meta-analyses of the Big Five: Digman (1997) and Mount, Barrick, Scullen, and Rounds (2005). Pers. Individ. Dif. 45 , 679–683 (2008).
Wood, D., Gardner, M. H. & Harms, P. D. How functionalist and process approaches to behavior can explain trait covariation. Psychol. Rev. 122 , 84–111 (2015).
Dunlap, W. P. Generalizing the Common Language Effect Size indicator to bivariate normal correlations. Psych. Bull. 116 , 509–511 (1994).
Connolly, J. J., Kavanagh, E. J. & Viswesvaran, C. The convergent validity between self and observer ratings of personality: A meta-analytic review. Int. J. of Selection and Assessment. 15 , 110–117 (2007).
Harris, K. & Vazire, S. On friendship development and the Big Five personality traits. Soc. and Pers. Psychol. Compass. 10 , 647–667 (2016).
Weidmann, R., Schönbrodt, F. D., Ledermann, T. & Grob, A. Concurrent and longitudinal dyadic polynomial regression analyses of Big Five traits and relationship satisfaction: Does similarity matter? J. Res. in Personality. 70 , 6–15 (2017).
Cuperman, R. & Ickes, W. Big Five predictors of behavior and perceptions in initial dyadic interactions: Personality similarity helps extraverts and introverts, but hurts “disagreeables”. J. of Pers. and Soc. Psychol. 97 , 667–684 (2009).
Schmidt, F. L. & Hunter, J. E. The validity and utility of selection methods in personnel psychology: Practical and theoretical implications of 85 years of research findings. Psychol. Bull. 124 , 262–274 (1998).
Brown, M. & Sacco, D. F. Unrestricted sociosexuality predicts preferences for extraverted male faces. Pers. Individ. Dif. 108 , 123–127 (2017).
Lukaszewski, A. W. & Roney, J. R. The origins of extraversion: joint effects of facultative calibration and genetic polymorphism. Pers. Soc. Psychol. Bull. 37 , 409–21 (2011).
Curran, P. G. Methods for the detection of carelessly invalid responses in survey data. J. Exp. Soc. Psychol. 66 , 4–19 (2016).
Khromov, A. B. The five-factor questionnaire of personality [Pjatifaktornyj oprosnik lichnosti]. In Rus. (Kurgan State University, 2000).
Trizano-Hermosilla, I. & Alvarado, J. M. Best alternatives to Cronbach’s alpha reliability in realistic conditions: Congeneric and asymmetrical measurements. Front. Psychol . https://doi.org/10.3389/fpsyg.2016.00769 (2016).
Liu, Z., Luo, P., Wang, X. & Tang, X. Deep Learning Face Attributes in the Wild. in 2015 IEEE International Conference on Computer Vision (ICCV) 3730–3738 https://doi.org/10.1109/ICCV.2015.425 (IEEE, 2015).
He, K., Zhang, X., Ren, S. & Sun, J. Deep Residual Learning for Image Recognition. in 2016 IEEE Conference on Computer Vision and Pattern Recognition (CVPR) 770–778 https://doi.org/10.1109/CVPR.2016.90 (IEEE, 2016).
Download references
Acknowledgements
We appreciate the assistance of Oleg Poznyakov, who organized the data collection, and we are grateful to the anonymous peer reviewers for their detailed and insightful feedback.
Contributions
A.K., E.O., D.D. and A.N. designed the study. K.S. and A.K. designed the ML algorithms and trained the ANN. A.N. contributed to the data collection. A.K., K.S. and D.D. contributed to data pre-processing. E.O., D.D. and A.K. analysed the data, contributed to the main body of the manuscript, and revised the text. A.K. prepared Figs. 1 and 2. All the authors contributed to the final version of the manuscript.
Corresponding authors
Correspondence to Alexander Kachur or Evgeny Osin .
Ethics declarations
Competing interests.
A.K., K.S. and A.N. were employed by the company that provided the datasets for the research. E.O. and D.D. declare no competing interests.
Additional information
Publisher’s note Springer Nature remains neutral with regard to jurisdictional claims in published maps and institutional affiliations.
Supplementary information
Supplementary information., rights and permissions.
Open Access This article is licensed under a Creative Commons Attribution 4.0 International License, which permits use, sharing, adaptation, distribution and reproduction in any medium or format, as long as you give appropriate credit to the original author(s) and the source, provide a link to the Creative Commons license, and indicate if changes were made. The images or other third party material in this article are included in the article’s Creative Commons license, unless indicated otherwise in a credit line to the material. If material is not included in the article’s Creative Commons license and your intended use is not permitted by statutory regulation or exceeds the permitted use, you will need to obtain permission directly from the copyright holder. To view a copy of this license, visit http://creativecommons.org/licenses/by/4.0/ .
Reprints and permissions
About this article
Cite this article.
Kachur, A., Osin, E., Davydov, D. et al. Assessing the Big Five personality traits using real-life static facial images. Sci Rep 10 , 8487 (2020). https://doi.org/10.1038/s41598-020-65358-6
Download citation
Received : 12 April 2019
Accepted : 28 April 2020
Published : 22 May 2020
DOI : https://doi.org/10.1038/s41598-020-65358-6
Share this article
Anyone you share the following link with will be able to read this content:
Sorry, a shareable link is not currently available for this article.
Provided by the Springer Nature SharedIt content-sharing initiative
This article is cited by
Personality of organizational social media accounts and its relationship with characteristics of their photos: analyses of startups’ instagram photos.
- Yunhwan Kim
BMC Psychology (2024)
Seeing the darkness: identifying the Dark Triad from emotionally neutral faces
- Danielle Haroun
- Yaarit Amram
- Joseph Glicksohn
Current Psychology (2024)
A Richer Vocabulary of Chinese Personality Traits: Leveraging Word Embedding Technology for Mining Personality Descriptors
- Yigang Ding
- Feijun Zheng
Journal of Psycholinguistic Research (2024)
Unravelling the many facets of human cooperation in an experimental study
- Victoria V. Rostovtseva
- Mikael Puurtinen
- Franz J. Weissing
Scientific Reports (2023)
Using deep learning to predict ideology from facial photographs: expressions, beauty, and extra-facial information
- Stig Hebbelstrup Rye Rasmussen
- Steven G. Ludeke
- Robert Klemmensen
By submitting a comment you agree to abide by our Terms and Community Guidelines . If you find something abusive or that does not comply with our terms or guidelines please flag it as inappropriate.
Quick links
- Explore articles by subject
- Guide to authors
- Editorial policies
Sign up for the Nature Briefing: AI and Robotics newsletter — what matters in AI and robotics research, free to your inbox weekly.

- Research article
- Open access
- Published: 05 May 2020
Personality traits, emotional intelligence and decision-making styles in Lebanese universities medical students
- Radwan El Othman 1 ,
- Rola El Othman 2 ,
- Rabih Hallit 1 , 3 , 4 na1 ,
- Sahar Obeid 5 , 6 , 7 na1 &
- Souheil Hallit 1 , 5 , 7 na1
BMC Psychology volume 8 , Article number: 46 ( 2020 ) Cite this article
59k Accesses
34 Citations
16 Altmetric
Metrics details
This study aims to assess the impact of personality traits on emotional intelligence (EI) and decision-making among medical students in Lebanese Universities and to evaluate the potential mediating role-played by emotional intelligence between personality traits and decision-making styles in this population.
This cross-sectional study was conducted between June and December 2019 on 296 general medicine students.
Higher extroversion was associated with lower rational decision-making style, whereas higher agreeableness and conscientiousness were significantly associated with a higher rational decision-making style. More extroversion and openness to experience were significantly associated with a higher intuitive style, whereas higher agreeableness and conscientiousness were significantly associated with lower intuitive style. More agreeableness and conscientiousness were significantly associated with a higher dependent decision-making style, whereas more openness to experience was significantly associated with less dependent decision-making style. More agreeableness, conscientiousness, and neuroticism were significantly associated with less spontaneous decision-making style. None of the personality traits was significantly associated with the avoidant decision-making style. Emotional intelligence seemed to fully mediate the association between conscientiousness and intuitive decision-making style by 38% and partially mediate the association between extroversion and openness to experience with intuitive decision-making style by 49.82 and 57.93% respectively.
Our study suggests an association between personality traits and decision-making styles. The results suggest that EI showed a significant positive effect on intuitive decision-making style and a negative effect on avoidant and dependent decision-making styles. Additionally, our study underlined the role of emotional intelligence as a mediator factor between personality traits (namely conscientiousness, openness, and extroversion) and decision-making styles.
Peer Review reports
Decision-making is a central part of daily interactions; it was defined by Scott and Bruce in 1995 as «the learned habitual response pattern exhibited by an individual when confronted with a decision situation. It is not a personality trait, but a habit-based propensity to react in a certain way in a specific decision context» [ 1 ]. Understanding how people make decisions within the moral domain is of great importance theoretically and practically. Its theoretical value is related to the importance of understanding the moral mind to further deepen our knowledge on how the mind works, thus understanding the role of moral considerations in our cognitive life. Practically, this understanding is important because we are highly influenced by the moral decisions of people around us [ 2 ]. According to Scott and Bruce (1995), there are five distinct decision-making styles (dependent, avoidant, spontaneous, rational, intuitive) [ 1 ] and each individuals’ decision-making style has traits from these different styles with one dominant style [ 3 ].
The dependent decision-making style can be regarded as requiring support, advice, and guidance from others when making decisions. Avoidant style is characterized by its tendency to procrastinate and postpone decisions if possible. On the other hand, spontaneous decision-making style is hallmarked by making snap and impulsive decisions as a way to quickly bypass the decision-making process. In other words, spontaneous decision-makers are characterized by the feeling of immediacy favoring to bypass the decision-making process rapidly without employing much effort in considering their options analytically or relying on their instinct. Rational decision-making style is characterized by the use of a structured rational approach to analyze information and options to make decision [ 1 ]. In contrast, intuitive style is highly dependent upon premonitions, instinct, and feelings when it comes to making decisions driving focus toward the flow of information rather than systematic procession and analysis of information, thus relying on hunches and gut feelings. Several studies have evaluated the factors that would influence an individual’s intuition and judgment. Rand et al. (2016) discussed the social heuristics theory and showed that women and not men tend to internalize altruism _ the selfless concern for the well-being of others_ in their intuition and thus in their intuitive decision-making process [ 4 ]. Additionally, intuitive behavior honesty is influenced by the degree of social relationships with individuals affected by the outcome of our decision: when dishonesty harms abstract others, intuition promotion causes more dishonesty. On the contrary, when dishonesty harms concrete others, intuition promotion has no significant effect on dishonesty. Hence, the intuitive appeal of pro-sociality may cancel out the intuitive selfish appeal of dishonesty [ 5 ]. Moreover, the decision-making process and styles have been largely evaluated in previous literature. Greene et al. (2008) and Rand (2016) showed that utilitarian moral judgments aiming to minimize cost and maximize benefits across concerned individuals are driven by controlled cognitive process (i.e. rational); whereas, deontological moral judgments _where rights and duties supersede utilitarian considerations_ are dictated by an automatic emotional response (e.g. spontaneous decision-making) [ 6 , 7 ]. Trémolière et al. (2012) found that mortality salience makes people less utilitarian [ 8 ].
Another valuable element influencing our relationships and career success [ 9 ] is emotional intelligence (EI) a cardinal factor to positive patient experience in the medical field [ 10 ]. EI was defined by Goleman as «the capacity of recognizing our feelings and those of others, for motivating ourselves, and for managing emotions both in us and in our relationships» [ 11 ]. Hence, an important part of our success in life nowadays is dependent on our ability to develop and preserve social relationships, depict ourselves positively, and control the way people descry us rather than our cognitive abilities and traditional intelligence measured by IQ tests [ 12 ]. In other words, emotional intelligence is a subtype of social intelligence involving observation and analyses of emotions to guide thoughts and actions. Communication is a pillar of modern medicine; thus, emotional intelligence should be a cornerstone in the education and evaluation of medical students’ communication and interpersonal skills.
An important predictor of EI is personality [ 13 ] defined as individual differences in characteristic patterns of thinking, feeling and behaving [ 14 ]. An important property of personality traits is being stable across time [ 15 ] and situations [ 16 ], which makes it characteristic of each individual. One of the most widely used assessment tools for personality traits is the Five-Factor model referring to «extroversion, openness to experience, agreeableness, conscientiousness, neuroticism». In fact, personality traits have an important impact on individuals’ life, students’ academic performance [ 17 ] and decision-making [ 18 ].
Extroversion is characterized by higher levels of self-confidence, positive emotions, enthusiasm, energy, excitement seeking, and social interactions. Openness to experience individuals are creative, imaginative, intellectually curious, impulsive, and original, open to new experiences and ideas [ 19 ]. Agreeableness is characterized by cooperation, morality, sympathy, low self-confidence, high levels of trust in others, and tend to be happy and satisfied because of their close interrelationships [ 19 ]. Conscientiousness is characterized by competence, hard work, self-discipline, organization, strive for achievement and goal orientation [ 20 ] with a high level of deliberation making conscientious individuals capable of analyzing the pros and cons of a given situation [ 21 ]. Neuroticism is characterized by anxiety, anger, insecurity, impulsiveness, self-consciousness,and vulnerability [ 20 ]. High neurotic individuals have higher levels of negative affect, are easily irritated, and more likely to turn to inappropriate coping responses, such as interpersonal hostility [ 22 ].
Multiple studies have evaluated the impact of personality traits on decision-making styles. Narooi and Karazee (2015) studied personality traits, attitude to life, and decision-making styles among university students in Iran [ 23 ]. They deduced the presence of a strong relationship between personality traits and decision-making styles [ 23 ]. Riaz and Batool (2012) evaluated the relationship between personality traits and decision-making among a group of university students (Fig. 1 ). They concluded that «15.4 to 28.1% variance in decision-making styles is related to personality traits» [ 24 ]. Similarly, Bajwa et al. (2016) studied the relationship between personality traits and decision-making among students. They concluded that conscientiousness personality trait is associated with rational decision-making style [ 25 ]. Bayram and Aydemir (2017) studied the relationship between personality traits and decision-making styles among a group of university students in Turkey [ 26 ]. Their work yielded to multiple conclusion namely a significant association between rational and intuitive decision-making styles and extroversion, openness to experience, conscientiousness, and agreeableness personality traits [ 26 ]. The dependent decision-making style had a positive relation with both neuroticism and agreeableness. The spontaneous style had a positive relation with neuroticism and significant negative relation with agreeableness and conscientiousness. Extroversion personality traits had a positive effect on spontaneous style. Agreeableness personality had a positive effect on the intuitive and dependent decision-making style. Conscientiousness personality had a negative effect on avoidant and spontaneous decision-making style and a positive effect on rational style. Neuroticism trait had a positive effect on intuitive, dependent and spontaneous decision-making style. Openness to experience personality traits had a positive effect on rational style [ 26 ].
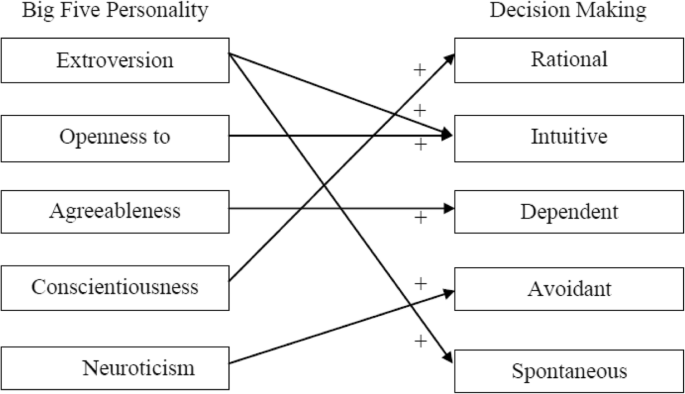
Schematic representation of the effect of the big five personality types on decision-making styles [ 24 ]
Furthermore, several studies have evaluated the relationship between personality traits and emotional intelligence. Dawda and Hart (2000) found a significant relationship between emotional intelligence and all Big Five personality traits [ 27 ]. Day and al. (2005) found a high correlation between emotional intelligence and extroversion and conscientiousness personality traits [ 28 ]. A study realized by Avsec and al. (2009) revealed that emotional intelligence is a predictor of the Big Five personality traits [ 29 ]. Alghamdi and al. (2017) investigated the predictive role of EI on personality traits among university advisors in Saudi Arabia. They found that extroversion, agreeableness, and openness to experience emerged as significant predictors of EI. The study also concluded that conscientiousness and neuroticism have no impact on EI [ 13 ].
Nonetheless, decision-making is highly influenced by emotion making it an emotional process. The degree of emotional involvement in a decision may influence our choices [ 30 ] especially that emotions serve as a motivational process for decision-making [ 31 ]. For instance, patients suffering from bilateral lesions of the ventromedial prefrontal cortex (interfering with normal processing of emotional signals) develop severe impairments in personal and social decision-making despite normal cognitive capabilities (intelligence and creativity); highlighting the guidance role played by emotions in the decision-making process [ 32 ]. Furthermore, EI affects attention, memory, and cognitive intelligence [ 33 , 34 ] with higher levels of EI indicating a more efficient decision-making [ 33 ]. In one study, Khan and al. concluded that EI had a significant positive effect on rational and intuitive decision-making styles and negative effect on dependent and spontaneous decision-making styles among a group of university students in Pakistan [ 35 ].
This study aims to assess the impact of personality traits on both emotional intelligence and decision-making among medical students in Lebanese Universities and to test the potential mediating role played by emotional intelligence between personality and decision-making styles in this yet unstudied population to our knowledge. The goal of the present research is to evaluate the usefulness of implementing such tools in the selection process of future physicians. It also aimed at assessing the need for developing targeted measures, aiming to ameliorate the psychosocial profile of Lebanese medical students, in order to have a positive impact on patients experience and on medical students’ career success.
Study design
This cross-sectional study was conducted between June and December 2019. A total of 296 participants were recruited from all the 7 faculties of medicine in Lebanon. Data collection was done through filling an anonymous online or paper-based self-administered English questionnaire upon the participant choice. All participants were aware of the purpose of the study, the quality of data collected and gave prior informed consent. Participation in this study was voluntary and no incentive was given to the participants. All participants were General medicine students registered as full-time students in one of the 7 national schools of medicine aged 18 years and above regardless of their nationality. The questionnaire was only available in English since the 7 faculties of medicine in Lebanon require a minimum level of good English knowledge in their admission criteria. A pilot test was conducted on 15 students to check the clarity of the questionnaire. To note that these 15 questionnaires related data was not entered in the final database. The methodology used in similar to the one used in a previous paper [ 36 ]
Questionnaire and variables
The questionnaire assessed demographic and health characteristics of participants, including age, gender, region, university, current year in medical education, academic performance (assessed using the current cumulative GPA), parental highest level of education, and health questions regarding the personal history of somatic, and psychiatric illnesses.
The personality traits were evaluated using the Big Five Personality Test, a commonly used test in clinical psychology. Since its creation by John, Donahue, and Kentle (1991) [ 37 ], the five factor model was widely used in different countries including Lebanon [ 38 ]; it describes personality in terms of five board factors: extroversion, openness to experience, agreeableness, conscientiousness and neuroticism according to an individual’s response to a set of 50 questions on a 5-point Likert scale: 1 (disagree) to 5 (agree). A score for each personality trait is calculated in order to determine the major trait(s) in an individual personality (i.e. the trait with the highest score). The Cronbach’s alpha values were as follows: total scale (0.885), extroversion (0.880), openness to experience (0.718), agreeableness (0.668), conscientiousness (0.640), and neuroticism (0.761).
Emotional intelligence was assessed using the Quick Emotional Intelligence Self-Assessment scale [ 38 ]. The scale is divided into four domains: «emotional alertness, emotional control, social-emotional awareness, and relationship management». Each domain is composed of 10 questions, with answers measured on a 5-point Likert scale: 0 (never) to 4 (always). Higher scores indicate higher emotional intelligence [ 38 ] (α Cronbach = 0.950).
The decision-making style was assessed using the Scott and Bruce General Decision-Making Style Inventory commonly used worldwide since its creation in 1995 for this purpose [ 1 ]. The inventory consists of 25 questions answered according to a 5-point Likert scale: 1 (strongly disagree) to 5 (strongly agree) intended to evaluate the importance of each decision-making style among the 5 styles proposed by Scott and Bruce: dependent, avoidant, spontaneous, rational and intuitive. The score for each decision-making style is computed in order to determine the major style for each responder (α Cronbach total scale = 0.744; α Cronbach dependent style = 0.925; α Cronbach avoidant style = 0.927; α Cronbach spontaneous style = 0.935; α Cronbach rational style = 0.933; α Cronbach intuitive style = 0.919).
Sample size calculation
The Epi info program (Centers for Disease Control and Prevention (CDC), Epi Info™) was employed for the calculation of the minimal sample size needed for our study, with an acceptable margin of error of 5% and an expected variance of decision-making styles that is related to personality types estimated by 15.4 to 28.1% [ 24 ] for 5531 general medicine student in Lebanon [ 39 ]. The result showed that 294 participants are needed.
Statistical analysis
Statistical Package for Social Science (SPSS) version 23 was used for the statistical analysis. The Student t-test and ANOVA test were used to assess the association between each continuous independent variable (decision-making style scores) and dichotomous and categorical variables respectively. The Pearson correlation test was used to evaluate the association between two continuous variables. Reliability of all scales and subscales was assessed using Cronbach’s alpha.
Mediation analysis
The PROCESS SPSS Macro version 3.4, model four [ 40 ] was used to calculate five pathways (Fig. 2 ). Pathway A determined the regression coefficient for the effect of each personality trait on emotional intelligence, Pathway B examined the association between EI and each decision-making style, independent of the personality trait, and Pathway C′ estimated the total and direct effect of each personality trait on each decision-making style respectively. Pathway AB calculated the indirect intervention effects. To test the significance of the indirect effect, the macro generated bias-corrected bootstrapped 95% confidence intervals (CI) [ 40 ]. A significant mediation was determined if the CI around the indirect effect did not include zero [ 40 ]. The covariates that were included in the mediation model were those that showed significant associations with each decision-making style in the bivariate analysis.
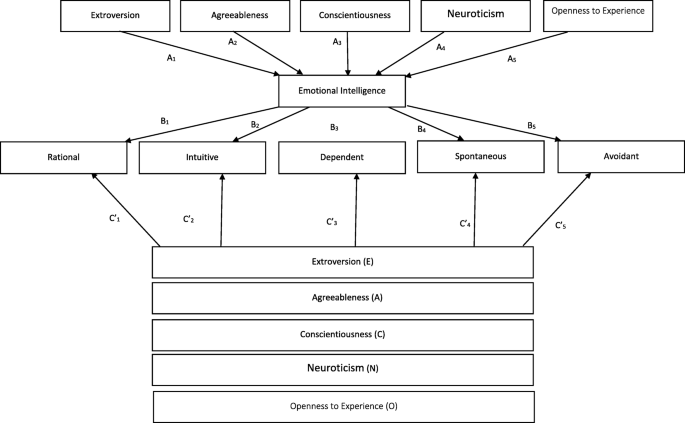
Summary of the pathways followed during the mediation analysis
Sociodemographic and other characteristics of the participants
The mean age of the participants was 22.41 ± 2.20 years, with 166 (56.1%) females. The mean scores of the scales used were as follows: emotional intelligence (108.27 ± 24.90), decision-making: rationale style (13.07 ± 3.17), intuitive style (16.04 ± 3.94), dependent style (15.53 ± 4.26), spontaneous style (13.52 ± 4.22), avoidant style (12.44 ± 4.11), personality trait: extroversion (21.18 ± 8.96), agreeableness (28.01 ± 7.48), conscientiousness (25.20 ± 7.06), neuroticism (19.29 ± 8.94) and openness (27.36 ± 7.81). Other characteristics of the participants are summarized in Table 1 .
Bivariate analysis
Males vs females, having chronic pain compared to not, originating from South Lebanon compared to other governorates, having an intermediate income compared to other categories, those whose mothers had a primary/complementary education level and those whose fathers had an undergraduate diploma vs all other categories had higher mean rationale style scores. Those fathers, who had a postgraduate diploma, had a higher mean intuitive style scores compared to all other education levels. Those who have chronic pain compared to not and living in South Lebanon compared to other governorates had higher dependent style scores. Those who have chronic pain compared to not, those who take medications for a mental illness whose mothers had a primary/complementary education level vs all other categories and those whose fathers had a postgraduate diploma vs all other categories had higher spontaneous style scores (Table 2 ).
Higher agreeableness and conscientiousness scores were significantly associated with higher rational style scores, whereas higher extroversion and neuroticism scores were significantly associated with lower rational style scores. Higher extroversion, openness and emotional intelligence scores were significantly associated with higher intuitive scores, whereas higher agreeableness, conscientiousness and neuroticism scores were significantly associated with lower intuitive style scores. Higher agreeableness and conscientiousness were associated with higher dependent style scores, whereas higher openness and emotional intelligence scores were significantly associated with lower dependent styles scores. Higher agreeableness, conscientiousness, neuroticism, and emotional intelligence scores were significantly associated with lower spontaneous style scores. Finally, higher extroversion, neuroticism and emotional intelligence scores were significantly associated with lower avoidant style scores (Table 3 ).
Post hoc analysis: rationale style: governorate (Beirut vs Mount Lebanon p = 0.022; Beirut vs South p < 0.001; Mount Lebanon vs South p = 0.004; South vs North p = 0.001; South vs Bekaa p = 0.047); monthly income (intermediate vs high p = 0.024); mother’s educational level (high school vs undergraduate diploma p = 0.048); father’s education level (undergraduate vs graduate diploma p = 0.01).
Intuitive style: father’s education level (high school vs postgraduate diploma p = 0.046).
Dependent style: governorate (Beirut vs Mount Lebanon p = 0.006; Beirut vs South p = 0.003);
Avoidant style: mother’s educational level (high school vs undergraduate diploma p = 0.008; undergraduate vs graduate diploma p = 0.004; undergraduate vs postgraduate diploma p = 0.001).
Mediation analysis was run to check if emotional intelligence would have a mediating role between each personality trait and each decision-making style, after adjusting overall covariates that showed a p < 0.05 with each decision-making style in the bivariate analysis.
Rational decision-making style (Table 4 , model 1)
Higher extroversion was significantly associated with higher EI, b = 0.91, 95% BCa CI [0.60, 1.23], t = 5.71, p < 0.001 (R2 = 0.31). Higher extroversion was significantly associated with lower rational decision-making even with EI in the model, b = − 0.06, 95% BCa CI [− 0.11, − 0.02], t = − 2.81, p = 0.003; EI was not significantly associated with rational decision-making, b = 0.02, 95% BCa CI [− 0.0003, 0.03], t = 1.93, p = 0.054 (R2 = 0.29). When EI was not in the model, higher extroversion was significantly associated with lower rational decision-making, b = − 0.05, 95% BCa CI [− 0.09, − 0.01], t = − 2.43, p = 0.015 (R2 = 0.28). The mediating effect of EI was 21.22%.
Higher agreeableness was not significantly associated with EI, b = − 0.05, 95% BCa CI [− 0.40, 0.31], t = − 0.26, p = 0.798 (R2 = 0.31). Higher agreeableness was significantly associated with higher rational decision-making style even with EI in the model, b = 0.07, 95% BCa CI [0.02, 0.11], t = 2.89, p = 0.004; EI was not significantly associated with the rational decision-making, b = 0.01, 95% BCa CI [− 0.0003, 0.03], t = 1.92, p = 0.055 (R2 = 0.29). When EI was not in the model, higher agreeableness was significantly associated with higher rational decision-making, b = 0.07, 95% BCa CI [0.02, 0.11], t = 2.86, p = 0.004 (R2 = 0.28). The mediating effect of EI was 0.10%.
Higher conscientiousness was significantly associated with higher EI, b = 1.40, 95% BCa CI [1.04, 1.76], t = 7.62, p < 0.001 (R2 = 0.31). Higher conscientiousness was significantly associated with the rational decision-making style even with EI in the model, b = 0.09, 95% BCa CI [0.04, 0.14], t = 3.55, p < 0.001; EI was not significantly associated with the rational decision-making, b = 0.01, 95% BCa CI [− 0.0003, 0.03], t = 1.93, p = 0.055 (R2 = 0.29). When EI was not in the model, conscientiousness was significantly associated with the rational decision-making style, b = 0.11, 95% BCa CI [0.07, 0.16], t = 4.76, p < 0.001 (R2 = 0.28). The mediating effect of EI was 22.47%.
Higher neuroticism was significantly associated with lower EI, b = − 0.50, 95% BCa CI [− 0.80, − 0.20], t = − 3.26, p = 0.001 (R2 = 0.31). Neuroticism was not significantly associated with rational decision-making style with EI in the model, b = − 0.09, 95% BCa CI [− 0.05, 0.03], t = − 0.43, p = 0.668; EI was not significantly associated with rational decision-making, b = 0.01, 95% BCa CI [− 0.0003, 0.03], t = 1.93, p = 0.055 (R2 = 0.29). When EI was not in the model, neuroticism was not significantly associated with the rational decision-making style, b = − 0.02, 95% BCa CI [− 0.06, 0.02], t = − 0.81, p = 0.418 (R2 = 0.28).
No calculations were done for the openness to experience personality traits since it was not significantly associated with the rational decision-making style in the bivariate analysis.
Intuitive decision-making style (Table 4 , model 2)
Higher extroversion was significantly associated with higher EI, b = 0.86, 95% BCa CI [0.59, 1.13], t = 6.28, p < 0.001 (R2 = 0.41). Higher extroversion was significantly associated with higher intuitive decision-making even with EI in the model, b = 0.05, 95% BCa CI [0.002, 0.11], t = 2.03, p = 0.043; EI was significantly associated with intuitive decision-making style, b = 0.03, 95% BCa CI [0.01, 0.05], t = 2.91, p = 0.003 (R2 = 0.21). When EI was not in the model, higher extroversion was significantly associated with higher intuitive decision-making, b = 0.08, 95% BCa CI [0.03, 0.13], t = 3.21, p = 0.001 (R2 = 0.18). The mediating effect of EI was 49.82%.
Higher agreeableness was significantly associated with EI, b = − 0.33, 95% BCa CI [− 0.65, − 0.02], t = − 2.06, p = 0.039 (R2 = 0.41). Higher agreeableness was significantly associated with lower intuitive decision-making style even with EI in the model, b = − 0.15, 95% BCa CI [− 0.21, − 0.10], t = − 5.16, p < 0.001; higher EI was significantly associated with higher intuitive decision-making, b = 0.03, 95% BCa CI [0.01, 0.05], t = 2.91, p = 0.004 (R2 = 0.21). When EI was not in the model, higher agreeableness was significantly associated with lower intuitive decision-making, b = − 0.17, 95% BCa CI [− 0.22, − 0.11], t = − 5.48, p < 0.001 (R2 = 0.18). The mediating effect of EI was 6.80%.
Higher conscientiousness was significantly associated with higher EI, b = 1.18, 95% BCa CI [0.85, 1.51], t = 7.06, p < 0.001 (R2 = 0.41). Higher conscientiousness was significantly associated with lower intuitive decision-making style even with EI in the model, b = − 0.10, 95% BCa CI [− 0.16, − 0.03], t = − 2.95, p = 0.003; higher EI was also significantly associated with higher intuitive decision-making, b = 0.03, 95% BCa CI [0.01, 0.05], t = 2.91, p = 0.004 (R2 = 0.21). When EI was not in the model, conscientiousness was not significantly associated with the intuitive decision-making style, b = − 0.06, 95% BCa CI [− 0.12, 0.0004], t = − 1.95, p = 0.051 (R2 = 0.18). The mediating effect of EI was 38%.
Higher openness to experience was significantly associated with higher EI, b = 1.44, 95% BCa CI [1.13, 1.75], t = 9.11, p < 0.001 (R2 = 0.41). Higher openness to experience was significantly associated with higher intuitive decision-making style with EI in the model, b = 0.08, 95% BCa CI [0.01, 0.14], t = 2.38, p = 0.017; higher EI was also significantly associated with intuitive decision-making style, b = 0.03, 95% BCa CI [0.01, 0.05], t = 2.91, p = 0.004 (R2 = 0.21). When EI was not in the model, higher openness to experience was significantly associated with intuitive decision-making style, b = 0.12, 95% BCa CI [0.06, 0.18], t = 4.22, p < 0.001 (R2 = 0.18). The mediating effect of EI was 57.93%.
No calculations were done for neuroticism personality trait since it was not significantly associated with the intuitive decision-making style in the bivariate analysis.
Dependent decision-making style (Table 4 , model 3)
Agreeableness was not significantly associated with EI, b = − 0.15, 95% BCa CI [− 0.49, 0.17], t = − 0.94, p = 0.345 (R2 = 0.32). Higher agreeableness was significantly associated with higher dependent decision-making style even with EI in the model, b = 0.29, 95% BCa CI [0.23, 0.34], t = 10.51, p < 0.001; higher EI was significantly associated with lower dependent decision-making, b = − 0.04, 95% BCa CI [− 0.06, − 0.02], t = − 4.50, p < 0.001 (R2 = 0.40). When EI was not in the model, higher agreeableness was significantly associated with higher dependent decision-making, b = 0.29, 95% BCa CI [0.24, 0.35], t = 10.44, p < 0.001 (R2 = 0.18). The mediating effect of EI was 2.38%.
Higher conscientiousness was significantly associated with higher EI, b = 1.04, 95% BCa CI [0.69, 1.38], t = 5.93, p < 0.001 (R2 = 0.32). Higher conscientiousness was significantly associated with higher dependent decision-making style even with EI in the model, b = 0.15, 95% BCa CI [0.09, 0.20], t = 4.88, p < 0.001; higher EI was also significantly associated with lower dependent decision-making, b = − 0.04, 95% BCa CI [− 0.06, − 0.02], t = − 4.50, p < 0.001 (R2 = 0.40). When EI was not in the model, higher conscientiousness was significantly associated with a higher dependent decision-making style, b = 0.10, 95% BCa CI [0.04, 0.16], t = 3.49, p < 0.001 (R2 = 0.36). The mediating effect of EI was 30.25%.
Higher openness to experience was significantly associated with higher EI, b = 1.37, 95% BCa CI [1.05, 1.69], t = 8.41, p < 0.001 (R2 = 0.32). Higher openness to experience was significantly associated with lower dependent decision-making style even with EI in the model, b = − 0.13, 95% BCa CI [− 0.19, − 0.08], t = − 4.55, p < 0.001; higher EI was also significantly associated with dependent decision-making style, b = − 0.04, 95% BCa CI [− 0.19, − 0.08], t = − 4.50, p < 0.001 (R2 = 0.40). When EI was not in the model, higher openness to experience was significantly associated with lower dependent decision-making style, b = − 0.19, 95% BCa CI [− 0.24, − 0.14], t = − 7.06, p < 0.001 (R2 = 0.36). The mediating effect of EI was 43.69%.
No calculations were done for neuroticism and extroversion personality traits since they were not significantly associated with the dependent decision-making style in the bivariate analysis.
Spontaneous decision-making style (Table 4 , model 4)
Agreeableness was not significantly associated with EI, b = 0.17, 95% BCa CI [− 0.19, 0.53], t = 0.91, p = 0.364 (R2 = 0.17). Higher agreeableness was significantly associated with lower spontaneous decision-making style even with EI in the model, b = − 0.10, 95% BCa CI [− 0.16, − 0.03], t = − 3.07, p = 0.002; EI was not significantly associated with spontaneous decision-making, b = − 0.01, 95% BCa CI [− 0.03, 0.01], t = − 0.71, p = 0.476 (R2 = 0.15). When EI was not in the model, higher agreeableness was significantly associated with lower spontaneous decision-making, b = − 0.10, 95% BCa CI [− 0.16, − 0.04], t = − 3.11, p = 0.002 (R2 = 0.15). The mediating effect of EI was 1.25%.
Higher conscientiousness was significantly associated with higher EI, b = 1.26, 95% BCa CI [0.88, 1.64], t = 6.56, p < 0.001 (R2 = 0.17). Higher conscientiousness was significantly associated with lower spontaneous decision-making style even with EI in the model, b = − 0.16, 95% BCa CI [− 0.23, − 0.09], t = − 4.51, p < 0.001; EI was not significantly associated with spontaneous decision-making style, b = − 0.01, 95% BCa CI [− 0.03, 0.01], t = − 0.71, p = 0.476 (R2 = 0.15). When EI was not in the model, higher conscientiousness was significantly associated with lower spontaneous decision-making style, b = − 0.17, 95% BCa CI [− 0.23, − 0.10], t = − 5.11, p < 0.001 (R2 = 0.15). The mediating effect of EI was 5.64%.
Neuroticism was not significantly associated with EI, b = − 0.22, 95% BCa CI [− 0.53, 0.08], t = − 1.43, p = 0.153 (R2 = 0.17). Higher neuroticism was significantly associated with lower spontaneous decision-making style even with EI in the model, b = − 0.11, 95% BCa CI [− 0.16, − 0.06], t = − 4.05, p < 0.001; EI was not significantly associated with spontaneous decision-making style, b = − 0.01, 95% BCa CI [− 0.03, 0.01], t = − 0.71, p = 0.476 (R2 = 0.15). When EI was not in the model, higher neuroticism was significantly associated with lower spontaneous decision-making style, b = − 0.11, 95% BCa CI [− 0.16, − 0.05], t = − 4.01, p < 0.001 (R2 = 0.15). The mediating effect of EI was 1.49%.
No calculations were done for openness to experience and extroversion personality traits since they were not significantly associated with the spontaneous decision-making style in the bivariate analysis .
Avoidant decision-making style (Table 4 , model 5)
Higher extroversion was significantly associated with higher EI, b = 0.88, 95% BCa CI [0.54, 1.21], t = 5.18, p < 0.001 (R2 = 0.15). Extroversion was not significantly associated with avoidant decision-making style even with EI in the model, b = − 0.01, 95% BCa CI [− 0.06, 0.05], t = − 0.27, p = 0.790; higher EI was significantly associated with avoidant decision-making style, b = − 0.04, 95% BCa CI [− 0.06, 0.03], t = − 4.79, p < 0.001 (R2 = 0.25). When EI was not in the model, extroversion was not significantly associated with avoidant decision-making style, b = − 0.05, 95% BCa CI [− 0.1, 0.08], t = − 1.69, p = 0.092 (R2 = 0.19).
Higher neuroticism was significantly associated with lower EI, b = − 0.59, 95% BCa CI [− 0.91, − 0.27], t = − 3.60, p < 0.001 (R2 = 0.15). Neuroticism was not significantly associated with avoidant decision-making style even with EI in the model, b = − 0.03, 95% BCa CI [− 0.09, 0.02], t = − 1.34, p = 0.182; higher EI was significantly associated with lower avoidant decision-making style, b = − 0.04, 95% BCa CI [− 0.06, − 0.03], t = − 4.79, p < 0.001 (R2 = 0.25). When EI was not in the model, neuroticism was not significantly associated with avoidant decision-making style, b = − 0.09, 95% BCa CI [− 0.06, 0.04], t = − 0.33, p = 0.739 (R2 = 0.19).
No calculations were done for openness to experience, agreeableness, and conscientiousness personality traits since they were not significantly associated with the avoidant decision-making style in the bivariate analysis.
This study examined the relationship between personality traits and decision-making styles, and the mediation role of emotional intelligence in a sample of general medicine students from different medical schools in Lebanon.
Agreeableness is characterized by cooperation, morality, sympathy, low self-confidence, high levels of trust in others and agreeable individuals tend to be happy and satisfied because of their close interrelationships [ 19 , 20 ]. Likewise, dependent decision-making style is characterized by extreme dependence on others when it comes to making decisions [ 1 ]. Our study confirmed this relationship similarly to Wood (2012) [ 41 ] and Bayram and Aydemir (2017) [ 26 ] findings of a positive relationship between dependent decision-making style and agreeableness personality trait and a negative correlation between this same personality trait and spontaneous decision-making style. In fact, this negative correlation can be explained by the reliance and trust accorded by agreeable individuals to their surroundings, making them highly influenced by others opinions when it comes to making a decision; hence, avoiding making rapid and snap decisions on the spur of the moment (i.e. spontaneous decision-making style); in order to explore the point of view of their surrounding before deciding on their own.
Conscientiousness is characterized by competence, hard work, self-discipline, organization, strive for achievement, and goal orientation [ 20 ]. Besides, conscientious individuals have a high level of deliberation making them capable of analyzing the pros and cons of a given situation [ 21 ]. Similarly, rational decision-makers strive for achievements by searching for information and logically evaluating alternatives before making decisions; making them high achievement-oriented [ 20 , 42 ]. This positive relationship between rational decision-making style and conscientiousness was established by Nygren and White (2005) [ 43 ] and Bajwa et al. (2016) [ 25 ]; thus, solidifying our current findings. Furthermore, we found that conscientiousness was positively associated with dependent decision-making; this relationship was not described in previous literature to our knowledge and remained statistically significant after adding EI to the analysis model. This relationship may be explained by the fact that conscientious individuals tend to take into consideration the opinions of their surrounding in their efforts to analyze the pros and cons of a situation. Further investigations in similar populations should be conducted in order to confirm this association. Moreover, we found a positive relationship between conscientiousness and intuitive decision-making that lost significance when EI was removed from the model. Thus, solidifying evidence of the mediating role played by EI between personality trait and decision-making style with an estimated mediation effect of 38%.
Extroversion is characterized by higher levels of self-confidence, positive emotions, enthusiasm, energy, excitement seeking, and social interactions. Similarly, intuitive decision-making is highly influenced by emotions and instinct. The positive relationship between extroversion and intuitive decision-making style was supported by Wood (2012) [ 41 ], Riaz et al. (2012) [ 24 ] and Narooi and Karazee (2015) [ 23 ] findings and by our present study.
Neuroticism is characterized by anxiety, anger, self-consciousness, and vulnerability [ 20 ]. High neurotic individuals have higher levels of negative affect, depression, are easily irritated, and more likely to turn to inappropriate coping responses, such as interpersonal hostility [ 22 ]. Our study results showed a negative relationship between neuroticism and spontaneous decision-making style.
Openness to experience individuals are creative, imaginative, intellectually curious, impulsive and original, open to new experiences and ideas [ 19 , 20 ]. One important characteristic of intuitive decision-making style is tolerance for ambiguity and the ability to picture the problem and its potential solution [ 44 ]. The positive relationship between openness to experience and intuitive decision-making style was established by Riaz and Batool (2012) [ 24 ] and came in concordance with our study findings. Additionally, our results suggest that openness personality trait is negatively associated with dependent decision-making style similar to previous findings [ 23 ]. Openness to experience individuals are impulsive and continuously seek intellectual pursuits and new experiences; hence, they tend to depend to a lesser extent on others’ opinions when making decisions since they consider the decision-making process a way to uncover new experiences and opportunities.
Our study results showed that EI had a significant positive effect on intuitive decision-making style. Intuition can be regarded as an interplay between cognitive and affective processes highly influenced by tactic knowledge [ 45 ]; hence, intuitive decision-making style is the result of personal and environmental awareness [ 46 , 47 , 48 ] in which individuals rely on the overall context without much concentration on details. In other words, they depend on premonitions, instinct, and predications of possibilities focusing on designing the overall plan [ 49 ] and take responsibility for their decisions [ 46 ]. Our study finding supports the results of Khan and al. (2016) who concluded that EI and intuitive decision-making had a positive relationship [ 35 ]. On the other hand, our study showed a negative relationship between EI and avoidant and dependent decision-making styles. Avoidant decision-making style is defined as a continuous attempt to avoid decision-making when possible [ 1 ] since they find it difficult to act upon their intentions and lack personal and environmental awareness [ 50 ]. Similarly to our findings, Khan and al. (2016) found that avoidant style is negatively influenced by EI [ 35 ]. The dependent decision-making style can be regarded as requiring support, advice, and guidance from others when making decisions. In other words, it can be described as an avoidance of responsibility and adherence to cultural norms; thus, dependent decision-makers tend to be less influenced by their EI in the decision-making process. Our conclusion supports Avsec’s (2012) findings [ 51 ] on the negative relationship between EI and dependent decision-making style.
Practical implications
The present study helps in determining which sort of decision is made by which type of people. This study also represents a valuable contribution to the Lebanese medical society in order to implement such variables in the selection methods of future physicians thus recruiting individuals with positively evaluated decision-making styles and higher levels of emotional intelligence; implying better communication skills and positively impacting patients’ experience. Also, the present study may serve as a valuable tool for the medical school administration to develop targeted measures to improve students’ interpersonal skills.
Limitations
Even though the current study is an important tool in order to understand the complex relationship between personality traits, decision-making styles and emotional intelligence among medical students; however, it still carries some limitations. This study is a descriptive cross-sectional study thus having a lower internal validity in comparison with experimental studies. The Scott and Bruce General Decision-Making Style Inventory has been widely used internationally for assessing decision-making styles since 1995 but has not been previously validated in the Lebanese population. In addition, the questionnaire was only available in English taking into consideration the mandatory good English knowledge in all the Lebanese medical schools; however, translation, and cross-language validation should be conducted in other categories of Lebanese population. Furthermore, self-reported measures were employed in the present research where participants self-reported themselves on personality types, decision-making styles and emotional intelligence. Although, all used scales are intended to be self-administered; however, this caries risk of common method variance; hence, cross-ratings may be employed in the future researches in order to limit this variance.
The results suggest that EI showed a significant positive effect on intuitive decision-making style and a negative effect on avoidant and dependent decision-making styles. In addition, our study showed a positive relationship between agreeableness and dependent decision-making style and a negative correlation with spontaneous decision-making style. Furthermore, conscientiousness had a positive relationship with rational and dependent decision-making style and extroversion showed a positive relationship with intuitive decision-making style. Neuroticism had a negative relationship with spontaneous style and openness to experience showed a positive relationship with intuitive decision-making style and a negative relationship with dependent style. Additionally, our study underlined the role of emotional intelligence as a mediation factor between personality traits and decision-making styles namely openness to experience, extroversion, and conscientiousness personality traits with intuitive decision-making style. Personality traits are universal [ 20 ]; beginning in adulthood and remaining stable with time [ 52 ]. Comparably, decision-making styles are stable across situations [ 1 ]. The present findings further solidify a previously established relationship between personality traits and decision-making and describes the effect of emotional intelligence on this relationship.
Availability of data and materials
All data generated or analyzed during this study are not publicly available to maintain the privacy of the individuals’ identities. The dataset supporting the conclusions is available upon request to the corresponding author.
Scott SG, Bruce RA. Decision-making style: the development and assessment of a new measure. Educ Psychol Meas. 1995;55(5):818–31.
Article Google Scholar
Capraro V, Everett JA, Earp BD. Priming intuition disfavors instrumental harm but not impartial beneficence. J Exp Soc Psychol. 2019;83:142–9.
Allwood CM, Salo I. Decision-making styles and stress. Int J Stress Manag. 2012;19(1):34.
Rand DG, Brescoll VL, Everett JA, Capraro V, Barcelo H. Social heuristics and social roles: intuition favors altruism for women but not for men. J Exp Psychol Gen. 2016;145(4):389–96.
Article PubMed Google Scholar
Kobis NC, Verschuere B, Bereby-Meyer Y, Rand D, Shalvi S. Intuitive honesty versus dishonesty: meta-analytic evidence. Perspect Psychol Sci. 2019;14(5):778–96.
Greene JD, Morelli SA, Lowenberg K, Nystrom LE, Cohen JD. Cognitive load selectively interferes with utilitarian moral judgment. Cognition. 2008;107(3):1144–54.
Rand DG. Cooperation, fast and slow: meta-analytic evidence for a theory of social heuristics and self-interested deliberation. Psychol Sci. 2016;27(9):1192–206.
Tremoliere B, Neys WD, Bonnefon JF. Mortality salience and morality: thinking about death makes people less utilitarian. Cognition. 2012;124(3):379–84.
Brown C, George-Curran R, Smith ML. The role of emotional intelligence in the career commitment and decision-making process. J Career Assess. 2003;11(4):379–92.
Clancy C. The importance of emotional intelligence. Nursing Management (2014+). 2014;21(8):15.
Goleman D. Working with emotional intelligence. New York: Bantam; 1998.
Google Scholar
Cotruş A, Stanciu C, Bulborea AA. EQ vs. IQ which is Most important in the success or failure of a student? Procedia Soc Behav Sci. 2012;46:5211–3.
Alghamdi NG, Aslam M, Khan K. Personality traits as predictor of emotional intelligence among the university teachers as advisors. Educ Res Int. 2017;2017:1–6 https://doi.org/10.1155/2017/9282565 .
Kazdin AE. Encyclopedia of psychology, vol. Vol 8. Washington, DC: American Psychological Association; 2000.
Bleidorn W, Klimstra TA, Denissen JJ, Rentfrow PJ, Potter J, Gosling SD. Personality maturation around the world: a cross-cultural examination of social-investment theory. Psychol Sci. 2013;24(12):2530–40.
Borkenau P, Riemann R, Spinath FM, Angleitner A. Genetic and environmental influences on person× situation profiles. J Pers. 2006;74(5):1451–80.
Hazrati-Viari A, Rad AT, Torabi SS. The effect of personality traits on academic performance: the mediating role of academic motivation. Procedia Soc Behav Sci. 2012;32:367–71.
Proceedings of the international conference on multiple academic disciplines. HanoiISBN: 978–1–943579-61-7 Hai Phong - . . Paper ID: VM714. 18–19, August: Vietnam (MAD17Vietnam Conference); 2017.
McCrae RR, John OP. An introduction to the five-factor model and its applications. J Pers. 1992;60(2):175–215.
McCrae R, Costa P Jr. Personality in adulthood. In: O envelhecimento. Uma abordagem psicológica . New York: Guilford; 1990. p. 2.
Johnson JA. Units of analysis for the description and explanation of personality. In R. Hogan, J. Johnson, & S. Briggs (Eds.) Handbook of personality psychology : Elsevier; 1997. p. 73–93.
Camps J, Stouten J, Euwema M. The relation between supervisors’ big five personality traits and employees’ experiences of abusive supervision. Front Psychol. 2016;7:112.
Article PubMed PubMed Central Google Scholar
Narooi ZS, Karazee F. Investigating the relationship among personality traits, decision-making styles, and attitude to life (Zahedan branch of Islamic Azad University as case study in Iran). Mediterr J Soc Sci. 2015;6(6 S6):311.
Riaz MN, Riaz MA, Batool N. Personality types as predictors of decision making styles. J Behav Sci. 2012;22(2):100–14.
Bajwa RS, Batool I, Asma M, Ali H, Ajmal A. Personality traits and decision making styles among university students (Pakistan). Pakistan J Life Soc Sci. 2016;14(1):38–41.
Bayram N, Aydemir M. Decision-making styles and personality traits. Int J Recent Adv Organizational Behaviour Decision Sci. 2017;3:905–15.
Dawda D, Hart SD. Assessing emotional intelligence: reliability and validity of the Bar-on emotional quotient inventory (EQ-i) in university students. Personal Individ Differ. 2000;28(4):797–812.
Day AL, Therrien DL, Carroll SA. Predicting psychological health: assessing the incremental validity of emotional intelligence beyond personality, type a behaviour, and daily hassles. Eur J Personality. 2005;19(6):519–36.
Avsec A, Takšić V, Mohorić T. The relationship of trait emotional intelligence with the big five in Croatian and Slovene university student samples. Psihološka obzorja/Horizons Psychol. 2009;18(3):99–110.
Spicer DP, Sadler-Smith E. An examination of the general decision making style questionnaire in two UK samples. J Manag Psychol. 2005;20(2):137–49.
Zeelenberg M, Pieters R. Feeling is for doing: a pragmatic approach to the study of emotions in decision-makingManuscript under review; 2005.
Bechara A, Damasio AR. The somatic marker hypothesis: a neural theory of economic decision. Games Econ Behavior. 2005;52(2):336–72.
Kustubayeva A, Bagayeva E. Emotional intelligence and decision making. RUDN J Psychol Pedagogics. 2011;S5:104–9.
Jaušovec N, Jaušovec K. Sex differences in brain activity related to general and emotional intelligence. Brain Cogn. 2005;59(3):277–86.
Khan E, Riaz M, Batool N, Riaz M. Emotional intelligence as a predictor of decision making styles among university students. J Appl Environ Biol Sci. 2016;6(4S):93–9.
Sarkis A. Hallit S. Hajj A. Lebanese students’ motivation in medical school: does it change throughout the years? A cross-sectional study. BMC Med Educ 20, 94 (2020). https://doi.org/10.1186/s12909-020-02011-w .
John OP, Donahue EM, Kentle RL. The big five inventory—versions 4a and 54. Berkeley: University of California, Berkeley, Institute of Personality; 1991.
Mohapel P. The quick emotional intelligence self-assessment: San Diego City College MESA Program; 2015.
Soueid M, Ghanem S, Hariri Z, Yamout N, Nehme R. Analysis of Lebanon's education sector: BankMed Market & Economic Research Divisionupdated Nov 10; cited 2015 Apr 6; 2014. Available from: http://www.bankmed.com.lb/BOMedia/subservices/categories/News/20150515170635891.pdf . Accessed 17 Jan 2020.
Hayes AF. Introduction to Mediation, Moderation, and Conditional Process Analysis: A Regression‐Based Approach. New York, NY: The Guilford Press.
Thomas E. Nygren, Rebecca J. White; 2005. Relating Decision Making Styles to Predicting Selfefficacy and a Generalized Expectationof Success and Failure. Article in Human Factors andErgonomics Society Annual Meeting Proceedings 49(3):432–34.
Baiocco R, Laghi F, D'Alessio M. Decision-making style among adolescents: relationship with sensation seeking and locus of control. J Adolesc. 2009;32(4):963–76.
Nygren TE, White RJ. Relating decision making styles to predicting selfefficacy and a generalized expectation of success and failure. Paper presented at: Proceedings of the Human Factors and Ergonomics Society Annual Meeting. 2005.
Bergstrand B. Situating the estimate: naturalistic decision-making as an alternative to analytical decision making in the Canadian forcesunpublished article. n. pag. http://www.cfcsc.dnd.ca/irc/nh/nh9798/0021.html ; 2001. p. 24.
Sinclair M, Ashkanasy NM. Intuition: myth or a decision-making tool? Manag Learn. 2005;36(3):353–70.
Harren VA. A model of career decision making for college students. J Vocat Behav. 1979;14(2):119–33.
Hablemitoglu S, Yildirim F. The relationship between perception of risk and decision making styles of Turkish university students: a descriptive study of individual differences. World Appl Sci J. 2008;4(2):214–24.
Singh R, Greenhaus JH. The relation between career decision-making strategies and person–job fit: a study of job changers. J Vocat Behav. 2004;64(1):198–221.
Miller CC, Ireland RD. Intuition in strategic decision making: friend or foe in the fast-paced 21st century? Acad Manag Perspect. 2005;19(1):19–30.
Phillips SD, Pazienza NJ, Ferrin HH. Decision-making styles and problem-solving appraisal. J Couns Psychol. 1984;31(4):497.
Avsec A. Do emotionally intelligent individuals use more adaptive decision-making styles? Stud Psychol. 2012;54(3):209.
Soldz S, Vaillant GE. The big five personality traits and the life course: a 45-year longitudinal study. J Res Pers. 1999;33(2):208–32.
Download references
Acknowledgements
We would like to thank all students who agreed to participate in this study.
Author information
Rabih Hallit, Sahar Obeid and Souheil Hallit are last co-authors.
Authors and Affiliations
Faculty of Medicine and Medical Sciences, Holy Spirit University of Kaslik (USEK), Jounieh, Lebanon
Radwan El Othman, Rabih Hallit & Souheil Hallit
Department of Pediatrics, Bahman Hospital, Beirut, Lebanon
Rola El Othman
Department of Infectious Disease, Bellevue Medical Center, Mansourieh, Lebanon
Rabih Hallit
Department of Infectious Disease, Notre Dame des Secours University Hospital Center, Byblos, Lebanon
Research and Psychology departments, Psychiatric Hospital of the Cross, P.O. Box 60096, Jal Eddib, Lebanon
Sahar Obeid & Souheil Hallit
Faculty of Arts and Sciences, Holy Spirit University of Kaslik (USEK), Jounieh, Lebanon
Sahar Obeid
INSPECT-LB: Institut National de Santé Publique, Epidémiologie Clinique et Toxicologie – Liban, Beirut, Lebanon
You can also search for this author in PubMed Google Scholar
Contributions
REO and REO were responsible for the data collection and entry and drafted the manuscript. SH and SO designed the study; SH carried out the analysis and interpreted the results; RH assisted in drafting and reviewing the manuscript; All authors reviewed the final manuscript and gave their consent; SO, SH and RH were the project supervisors.
Corresponding authors
Correspondence to Sahar Obeid or Souheil Hallit .
Ethics declarations
Ethics approval and consent to participate.
The Holy Spirit University of Kaslik – School of Medicine and Medical Sciences ethics committee approved the study protocol. A written consent was obtained from each student.
Consent for publication
Not applicable.
Competing interests
The authors have nothing to disclose.
Additional information
Publisher’s note.
Springer Nature remains neutral with regard to jurisdictional claims in published maps and institutional affiliations.
Rights and permissions
Open Access This article is licensed under a Creative Commons Attribution 4.0 International License, which permits use, sharing, adaptation, distribution and reproduction in any medium or format, as long as you give appropriate credit to the original author(s) and the source, provide a link to the Creative Commons licence, and indicate if changes were made. The images or other third party material in this article are included in the article's Creative Commons licence, unless indicated otherwise in a credit line to the material. If material is not included in the article's Creative Commons licence and your intended use is not permitted by statutory regulation or exceeds the permitted use, you will need to obtain permission directly from the copyright holder. To view a copy of this licence, visit http://creativecommons.org/licenses/by/4.0/ . The Creative Commons Public Domain Dedication waiver ( http://creativecommons.org/publicdomain/zero/1.0/ ) applies to the data made available in this article, unless otherwise stated in a credit line to the data.
Reprints and permissions
About this article
Cite this article.
El Othman, R., El Othman, R., Hallit, R. et al. Personality traits, emotional intelligence and decision-making styles in Lebanese universities medical students. BMC Psychol 8 , 46 (2020). https://doi.org/10.1186/s40359-020-00406-4
Download citation
Received : 27 January 2020
Accepted : 12 April 2020
Published : 05 May 2020
DOI : https://doi.org/10.1186/s40359-020-00406-4
Share this article
Anyone you share the following link with will be able to read this content:
Sorry, a shareable link is not currently available for this article.
Provided by the Springer Nature SharedIt content-sharing initiative
- Personality traits
- Decision-making
- Decision-making style
- Emotional intelligence
- Medical students
BMC Psychology
ISSN: 2050-7283
- General enquiries: [email protected]
Exploring the role of dysfunctional attitudes and unhelpful thoughts in the relationship between personality traits and psychological distress in Australian University students
- Open access
- Published: 28 June 2024
Cite this article
You have full access to this open access article
- Ronan J. Galloghly 1 ,
- Pragalathan Apputhurai 2 &
- Simon R. Knowles ORCID: orcid.org/0000-0001-8000-1000 1
135 Accesses
Explore all metrics
The impact of dysfunctional attitudes and unhelpful thinking upon clients presenting with personality-related psychological distress is an important clinical area of investigation as it informs psychological interventions. Despite this, there is limited research in this area. Thus, this study had two main aims: (1) examine the interrelationships between maladaptive personality traits, dysfunctional attitudes, unhelpful thinking, and psychological distress; and (2) explore the potential mediating roles of dysfunctional attitudes and unhelpful thinking on the relationship between maladaptive personality traits and psychological distress. A convenience sample of 728 undergraduate psychology students (mean age: 31.57 years; 76% female) completed an online questionnaire for course credit. The results supported the first hypothesis that after controlling for gender and age, there would be significant positive correlations among maladaptive personality traits, dysfunctional attitudes, and psychological distress. A structural equation model with an excellent fit (CMIN/df = 2.23, p = .063, TLI = 0.98, CFI = 0.99, SRMR, = 0.01, and RMSEA = 0.04) provided partial support for the second hypothesis in that dysfunctional attitudes and unhelpful thoughts mediated the relationship between maladaptive personality traits and psychological distress. Specifically, negative affectivity and detachment’s relationship with psychological distress were partially mediated via dysfunction attitudes and unhelpful thoughts, and dysfunctional attitudes respectively. These findings suggest that while dysfunctional attitudes and unhelpful thinking contribute to the relationship between personality traits and psychological distress, identification of other factors are required to improve theoretical understanding and subsequently psychological interventions.
Similar content being viewed by others
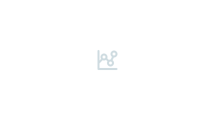
Dysfunctional Beliefs and Personality Traits

Comparing Conceptualizations of Narcissism in Predicting Negative Thinking Styles
The persian version of the personality beliefs questionnaire-short-form (pbq-sf): a psychometric evaluation.
Avoid common mistakes on your manuscript.
Introduction
Psychological distress is transdiagnostic, in that it features in all psychiatric disorders, and is defined as a combination of stress, anxiety and depressive symptoms in response to daily or chronic stressors (Lovibond & Lovibond, 1995 ; Barry et al., 2020 ). Gender and age represent the main biological predictors of psychological distress, with literature suggesting females experience significantly more psychological distress than males (Bracken & Reintjes, 2010 ; Mirowsky & Ross, 2017 ; Watkins & Johnson, 2018 ). Further, the Australian Institute of Health and Welfare (AIHW; 2018 ) found that - irrespective of gender - psychological distress increased throughout adulthood peaking at middle age (~ 50 years), before gradually decreasing. In addition to the biological contributors, two important psychological factors found to influence psychological distress are dysfunctional attitudes and personality (Buschmann et al., 2018 ; Jourdy & Petot, 2017 ).
Dysfunctional attitudes/beliefs reflect the attention, encoding, and interpreting of information in a negatively based format (Beck, 1976 ). Examples of these include rigid ‘must’ statements (e.g., “Everyone must love me’) and extreme “if-then” rules such as “If I do not pass this exam, then this confirms I am stupid”. A meta-analysis by Vîslă et al. ( 2016 ) found that across 83 primary studies including both clinical and community samples, dysfunctional attitudes predicted 20% of psychological distress variance independent of age, gender, income, educational, occupational, and marital status. Dysfunctional attitudes have also been identified to precede and perpetuate unhelpful thoughts (also known as thinking errors, negative automatic thoughts, or cognitive distortions) such as filtering, polarized thinking, overgeneralizing, and catastrophising (McKay et al., 2011 ). Understandably, like dysfunctional attitudes, unhelpful thoughts have been found to be related to, or associated with, changes in depression and anxiety (DeRubeis et al., 2008 ; Ross et al., 1986 ). Due to their significant role in underpinning and perpetuating psychological distress, dysfunctional attitudes and/or unhelpful thoughts are often primary targets for clinicians using psychological interventions such as cognitive behaviour therapy (Beck & Clark, 1997 ; Bowler et al., 2012 ; DeRubeis et al., 2008 ).
Along with dysfunctional attitudes and unhelpful thinking patterns, another primary contributor linked to psychological distress is personality traits (i.e., enduring patterns of perception, relation and thinking of the environment and oneself that are expressed in a wide variety of social and personal contexts; American Psychiatric Association [APA], 2013 ). Although the current approach for classifying personality disorders is based on the much-criticised categorial approach (e.g., Bach & Sellbom, 2016 ; Widiger & Gore, 2014 ), the fifth edition of the Diagnostic and Statistical Manual (DSM-5; APA, 2013 ) took tentative steps to move towards a dimensional approach to personality-based psychopathology (referred to as the alternative model of personality disorders; AMPD). According to the AMPD, personality disorders are dichotomous, and thus evaluated by both the level of personality dysfunction, and the specific personality traits (i.e., negative affectivity, detachment, antagonism, disinhibition, and psychoticism) which mirror the dysfunction. Recent literature supports the AMPD’s validity and usability across multiple mental health disorders including psychological distress (Chauhan et al., 2023 ; Clark & Watson, 2022 ; Nysaeter et al., 2023 ; Uliaszek et al., 2023 ; Vittengl et al., 2023 ).
Research to date provides evidence that personality traits, such as those based on the Big Five personality model (i.e., openness, conscientiousness, extraversion, agreeableness, and neuroticism) account for approximately one third of variance in depression symptoms, with the trait neuroticism (the tendency to experience negative emotions) being the most frequent and strongest contributor (Strickhouser et al., 2017 ; Kotov et al., 2010 ). More broadly, neuroticism has been identified to both predict and perpetuate psychological distress (Pollak et al., 2020 ; Widiger & Oltmanns, 2017 ).
Given personality traits, unhelpful thoughts, and dysfunctional attitudes independently predict psychological distress, it may be unsurprising the three variables are strongly interrelated. Research indicates that neuroticism is particularly correlated with dysfunctional attitudes and unhelpful thoughts (Blau et al., 2006 ; Hopwood et al., 2013 ; Samar et al., 2013 ). However, less is known about the role dysfunction attitudes and unhelpful thoughts play in the relationship between personality traits and psychological distress. Specifically, there is a lack of research examining whether dysfunctional attitudes and/or unhelpful thoughts mediate the relationship between personality traits and psychological distress.
Using a sample comprised of undergraduate psychology students, McDermut et al. ( 2019 ) found dysfunctional attitudes significantly and partially mediated the relationship between personality traits and psychological distress, and concluded that personality traits predicted psychological distress via the mechanism of dysfunctional attitudes. However, findings from McDermut et al. ( 2019 ) were limited by a relatively small sample size ( n = 167), using just one personality trait (neuroticism) in the mediation model, and not investigating potential mediating role of unhelpful thoughts in personality-related psychological distress.
Given the limited research to date, this study aimed to examine the role of dysfunctional attitudes and/or unhelpful thoughts in personality traits and psychological distress. The study will also seek to address the limited research using Personality Inventory for DSM-5 brief form (PID-5-BF) which assesses the five personality traits (i.e., negative affectivity, detachment, antagonism, disinhibition, and psychoticism) underpinning the AMPD. It was hypothesised that after controlling for gender and age, there would be significant positive correlations among personality traits, unhelpful thoughts, dysfunctional attitudes, and psychological distress. It was also hypothesised that dysfunctional attitudes and unhelpful thoughts would mediate the relationship between personality traits and psychological distress.
Participants and design
This study was based on an observational cross-design design using a convenience sampling method. Seven hundred and twenty-eight first year undergraduate psychology students enrolled at a medium-sized Australian university completed an online survey. The sample was predominantly female (76%), married/defacto (48.8%), and aged ranged between 18 and 74 years ( M = 31.57, SD = 20.84).
Depression anxiety and stress scale (DASS-21; Lovibond & Lovibond, 1995 )
The DASS-21 is a 21-item self-report questionnaire which assesses psychological distress via three subscales each containing seven items: depression, anxiety, and stress. Participants were requested to report the degree to which each item (e.g., ‘I felt I had nothing to look forward to’) applied to them during the preceding week. Each item is measured on a four-point Likert-type scale ranging from 0 (‘never’) to 3 (‘almost always’). As the DASS-21 is a short-form version of the original 42-item DASS, the final score was multiplied by two. Thus, total scores range from 0 to 126, with higher scores reflecting higher levels of psychological distress. The DASS-21 is reliable and valid amongst undergraduate student populations (Lemma et al., 2012 ; Osman et al., 2012 ), whilst demonstrating excellent internal consistency in this sample (Cronbach’s α = 0.94).
Dysfunctional attitude scale-short-form 1 (DAS-SF1: Beevers et al., 2007 )
The DAS-SF1 assesses dysfunctional attitudes across multiple domains including the need for approval from others (e.g., “My value as a person depends greatly on what others think of me”). Each item is scored on a four-point Likert-type scale ranging from 1 (‘totally disagree’) to 4 (‘totally agree). Total scores range from 9–36 with higher scores indicating more severe dysfunctional attitudes. In this study, the short-form version of the DAS (i.e., DAS-SF1) was used to decrease participant burden. The DAS-SF1 has displayed sound psychometrics amongst undergraduate student populations (McDermut et al., 2019 ) and good internal consistency in this sample (Cronbach’s α = 0.87).

The brief unhelpful thinking scale (BUTS; Knowles et al., 2017 )
The BUTS is an 11-item questionnaire examines unhelpful thoughts across several domains such as polarised thinking (e.g., “Things are either black or white, good or bad”). Each item is scored on a six-point Likert-type scale ranging from 0 (‘strongly disagree’) to 5 (‘strongly agree). Total scores range from 0–55 with higher scores indicating greater tendency toward unhelpful thinking. The BUTS demonstrated good internal consistency in this sample (Cronbach’s α = 0.82).
The PID-5 – Brief Form (PID-5-BF; Krueger et al., 2013 )
The PID-5-BF comprises 25 items with five items for five personality traits: Negative affectivity, Detachment, Disinhibition, Antagonism, and Psychoticism. Each item asked respondents whether they felt a statement accurately described them (e.g., ‘I often have to deal with people who are less important than me’). Items were measured on a Likert-type scale ranging from 0 (‘Very False or Often False’) to 3 (‘Very True or Often True’). Each trait domain score ranged from 0 to 15, with higher scores indicating greater personality trait dysfunction. The PID-5-BF is a reliable and valid instrument among university students which its brevity was designed to reduce participant burden (Anderson et al., 2018 ). The PID-5-BF demonstrated excellent internal consistency in this study (Cronbach’s α = 0.90).
Participants were provided a link to the study via course research experience program. Prior to commencing, students were informed that their survey was intended for respondents aged 18 years old and above, their participation was voluntary, they had a right to withdraw any time without reasons, and their responses were anonymous. Agreeing to begin the survey implied informed consent. Following completion of the questionnaire, information was provided explaining the study’s purpose, and contact information for support was provided in the unlikely event that completing the survey caused psychological distress. Course credit was given in compensation for completing the study survey. Ethical approval for the study was obtained by the university human research ethics committee. Pre-testing of the online (Qualtrics) questionnaire was tested by the members of the research team. The questionnaire took approximately 30 min to complete with all questions requiring a response to minimise missing data.
Data analysis strategy
All analyses were performed with SPSS (version 27) and AMOS (version 27) Data were screened, and all assumptions were tested prior to analyses. Bivariate correlations and Multivariate Analysis of Variance (MANOVA) were performed to examine the relationships of the demographic variables age and gender with study variables, evaluating the first hypothesis. The mediation model employing structural equation modeling was developed to evaluate the second hypothesis and the model fit being evaluated using the following criteria recommended by Hu and Bentler ( 1999 ): CMIN/Chi-square goodness of fit test [χ²] p > .05; Normed Chi-square [χ2/df] = 1–3, Tucker–Lewis index [TLI] > 0.95, Steiger-Lind Root Mean Square Error of Approximation [RMSEA] < 0.08, and Standardized Root Mean Square Residual [SRMR] < 0.06. The final model was derived by a stepwise approach of adding or removing paths based on theoretical and AMOS reported modification indices.
There were significant positive correlations among psychological distress, dysfunctional attitudes, unhelpful thoughts, and all five PID-5 personality traits (See Table 1 ). Psychological distress, dysfunctional attitudes, and the PID-5 personality traits were negatively correlated with unhelpful thinking. Negative affectivity produced the strongest correlations with psychological distress ( r = .59), unhelpful thinking ( r = .59), and dysfunctional attitudes ( r = .54). Detachment produced moderate correlations with psychological distress ( r = .45), unhelpful thinking ( r = .42), and dysfunctional attitudes ( r = .43). Unhelpful thoughts were also strongly correlated with dysfunctional attitudes ( r = .59) and psychological distress ( r = .52). Age was found to be a significantly related to negative affectivity ( r = −.29, p < .001), detachment ( r = − .10, p = .01), disinhibition ( r = − .22, p < .001), psychoticism ( r = − .26, p < .001), antagonism ( r = − .27, p < .001), Unhelpful thinking ( r = .27, p < .001), Dysfunctional attitudes ( r = - .21, p < .001), and psychological distress ( r = − .21, p < .001), and therefore age was controlled for in the subsequent analysis. A MANOVA (IV: gender; DVs: study variables) identified result was significant for gender, Pillai’s Trace = 0.01, F (14,1428) = 5.42, p < .001. A subsequent, univariate F test indicated that females had a significantly higher mean psychoticism compared to males ( F (2,719) = 3.35, p = .036). No other gender-based difference across the study variables was found.
In relation to the second hypothesis, a final model was developed based on an iterative approach where nonsignificant paths were removed from a saturated mediation model. Initially the model controlled for age and gender, however its inclusion produced a poorer fit and was therefore removed from the model. Similarly, psychoticism found to be a poor predictor and was also removed from the model. Overall, the final model (see Fig. 1 ) had an excellent fit (CMIN/df = 2.23, p = .063, TLI = 0.98, CFI = 0.99, SRMR, = 0.01, and RMSEA = 0.04). The total amount of variance accounted for in each of the variables was 33% of dysfunctional attitudes, 40% of unhelpful thinking, and 44% of psychological distress. As shown in Fig. 1 , Disinhibition had a significant direct influence on psychological distress and the relationship between Antagonism and psychological distress is fully mediated via BUTS and DAS-SF. The relationship between Negative affectivity and psychological distress is partially mediated via BUTS and DAS-SF while the relationship between Detachment and psychological distress is partially mediated via DAS-SF. The configural model fit indices indicated an adequate fit (χ2(36) = 1.65, p = .009, TLI = 0.98, CFI = 0.99, SRMR = 0.05, RMSEA = 0.02, Akaike information criterion (AIC) = 155.28), and the measurement weights model, with equal factor loadings on males and female groups, fit indices were also acceptable (χ2(46) = 1.42, p = .031, TLI = 0.99, CFI = 0.99, SRMR = 0.05, RMSEA = 0.02, AIC = 144.47). Comparisons across models showed that the increase in chi-squared values (Δχ2(10) = 6.19, p = .799) was not statistically significant and changes in other model fit indices were small, indicating robust measurement consistency across gender.
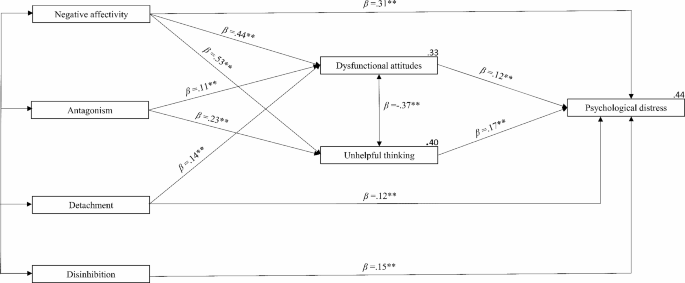
Final mediation model (** = p < .001)
The results supported the first hypothesis in that after controlling for gender and age, there would be significant positive correlations among PID-5 personality traits, dysfunctional attitudes, and psychological distress. These findings were consistent with the cognitive theory model linking these variables (Góngora & Castro Solano, 2017 ; Hopwood et al., 2013 ; Kotov et al., 2010 ; Pretzer & Beck, 2005 ). Also consistent with past research were the findings that Negative affectivity and Detachment produced the strongest correlations with psychological distress, dysfunctional attitudes, and the significant positive correlations between all five PID-5 personality traits and dysfunctional attitudes (Hakulinen et al., 2015 ; Hopwood et al., 2013 ; McDermut et al., 2019 ; Thimm et al., 2016 ). Additionally, there were no significant differences between male and female for individuals concerning dysfunctional attitudes, unhelpful thoughts, psychological distress, and personality traits, except for psychoticism.
The results also partially supported the second hypothesis in that dysfunctional attitudes and unhelpful thoughts would mediate the relationship between PID-5 personality traits and psychological distress. Specifically, Negative affectivity and Detachment’s relationship with psychological distress were partially mediated via dysfunction attitudes and unhelpful thoughts, and dysfunctional attitudes respectively. Further, supporting the second hypothesis was the finding that Antagonism’s effect on psychological distress was fully mediated by both dysfunctional attitudes and unhelpful thoughts. Not supporting the second hypothesis were the findings that neither dysfunctional attitudes nor unhelpful thoughts had any impact on Disinhibition’s effect on psychological distress.
The partial mediation of dysfunctional attitudes and unhelpful thoughts upon Negative affectivity’s relationship with psychological distress was consistent with McDermut and colleagues’ ( 2019 ) findings, and subsequently indicates that Negative affectivity predicts psychological distress, at least partially, by operating through these two cognitive mediators. Moreover, the novel findings that dysfunctional attitudes and unhelpful thoughts fully explained the relationship between Antagonism and psychological distress suggests they are the primary cognitive processes inherent within this relationship. Conversely, neither dysfunctional attitudes nor unhelpful thoughts had any impact upon Disinhibition’s relationship with psychological distress which indicates that this relationship exists outside of these cognitive models linking personality and psychological distress (Beck et al., 2015 ).
Clinical implications
The findings suggest that dysfunctional attitudes and unhelpful thoughts play a role in explaining the relationship between psychological distress and the AMPD PID-5 personality traits Negative affectivity, Detachment, and Antagonism. Subsequently, clients who present with psychological distress and score highly on these three scales may benefit from therapeutic interventions which focus on clients’ attitudes and unhelpful thinking patterns via well-established approaches such as cognitive behavioural therapy. Antagonism specifically, may be efficaciously treated by interpersonal therapy (IPT) given that it’s key feature interpersonal problems (Vize et al., 2022 ; Wright, 2019 ). Conversely, this approach may not be as efficacious for clients scoring high on Disinhibition, as this trait’s characteristics include irresponsibility, impulsivity, and risk taking; all of which infer potential treatment-interfering behaviours including missed appointments, difficulty focusing in-session, and nonadherence to homework tasks (APA, 2013 ). The utilisation of an intervention to such specific personality traits may be more efficacious, rather than focusing on attitudes and thoughts which may be symptoms of the trait. For instance, Conrod et al. ( 2013 ) created personality-specific interventions which included psychoeducation of personality traits and goalsetting to enhance motivation to change. The authors found that the intervention significantly reduce disinhibition-related behaviours (i.e., substance use) among adolescents. However, more research is required to determine the efficacy of this approach across wider community and clinical populations.
Limitations and future studies
This study has several limitations. The cross-section design lacks the robustness of a longitudinal approach from which casual inferences (true mediation) could be attained. The study also utilised a largely homogeneous non-clinical convenience sample of university students thus limiting the generalisability of findings, including age, cultural, socioeconomic, and educational diversity. It is also important to recognise the potential impact and limitations associated with social desirability and selection and response bias given the student-based convenience sample. Future research should investigate if these results can be replicated using community and clinical populations. Additionally, this paper utilised dysfunctional attitudes and unhelpful thoughts broadly. A more judicious approach might be to explore which specific dysfunctional attitudes and unhelpful thoughts are associated with specific personality traits. This could improve theory and potentially offer a more targeted approach to treating personality-related psychological distress.
In addition to addressing the above limitations, future studies should also look to explore and address other potential processes or confounders which may influence the identified relationships. These include coping strategies, comorbid psychological and/or physical conditions, and psychosocial stressors (e.g., relationship, employment, educational, financial). For instance, Huang et al. ( 2021 ) found that other psychological processes such as self-efficacy, coping styles, and psychological resilience mediated this relationship between personality traits and psychological distress among Chinese nurses. Similarly, a recent paper by Kestler-Peleg and colleagues ( 2023 ) found intolerance to uncertainty mediated the relationship between personality traits and a form on adjustment disorder-associated psychological distress.
In conclusion, this study’s findings indicate that three AMPD PID-5 personality traits (i.e., Negative affectivity, Detachment, and Antagonism) predicted psychological distress via the dysfunctional attitudes and unhelpful thoughts. This study is the first to extend the limited research to date by exploring the potential mediating role of dysfunctional attitudes and unhelpful thoughts on the relationship between personality and psychological distress. The findings provide evidence for the mediating role of dysfunctional attitudes and unhelpful thoughts. While limited by the cross-sectional design and evidence for true causal mediation, the findings highlight the relevance and importance of targeting dysfunctional attitudes and unhelpful thoughts in therapy.
Data availability
The datasets generated during and/or analysed during the current study are available from the corresponding author on reasonable request and after relevant ethical approval.
American Psychiatric Association (2013). Diagnostic and statistical manual of mental disorders (5th ed.). https://doi.org/10.1176/appi.books.9780890425596
Anderson, J. L., Sellbom, M., & Salekin, R. T. (2018). Utility of the personality inventory for DSM-5-Brief form (PID-5-BF) in the measurement of maladaptive personality and psychopathology. Assessment , 25 (5), 596–607. https://doi.org/10.1177/1073191116676889
Article PubMed Google Scholar
Australian Institute of Health and Welfare (2018). Australia’s Health 2018. (Cat. No. AUS 221; Australia’s Health Series No. 16) , 570. https://doi.org/10.25816/5ec1e56f25480
Bach, B., & Sellbom, M. (2016). Continuity between DSM-5 categorical criteria and traits criteria for borderline personality disorder. The Canadian Journal of Psychiatry, 61 (8), 489–494. https://doi.org/10.1177/0706743716640756
Barry, V., Stout, M. E., Lynch, M. E., Mattis, S., Tran, D. Q., Antun, A., Ribeiro, M. J., Stein, S. F., & Kempton, C. L. (2020). The effect of psychological distress on health outcomes: A systematic review and meta-analysis of prospective studies. Journal of Health Psychology , 25 (2), 227–239. https://doi.org/10.1177/1359105319842931
Beck, A. T. (1976). Cognitive Therapy and the Emotional Disorders New York, New American Library.
Beck, A. T., & Clark, D. A. (1997). An information processing model of anxiety: Automatic and strategic processes. Behaviour Research and Therapy , 35 (1), 49–58. https://doi.org/10.1016/S0005-7967(96)00069-1
Beck, A. T., Freeman, A., & Davis, D. D. (2015). Cognitive therapy of personality disorders 3rd edition / New York, The Guilford Press.
Beevers, C. G., Strong, D. R., Meyer, B., Pilkonis, P. A., & Miller, I. R. (2007). Efficiently assessing negative cognition in depression: An item response theory analysis of the dysfunctional attitude scale. Psychological Assessment , 19 (2), 199–209. https://doi.org/10.1037/1040-3590.19.2.199
Blau, S., Fuller, J. R., & Vaccaro, T. P. (2006). Rational-emotive disputing and the five-factor model: Personality dimensions of the Ellis emotional efficiency inventory. Journal of Rational-Emotive and Cognitive-Behavior Therapy , 24 (2), 87–100. https://doi.org/10.1007/s10942-005-0020-z
Article Google Scholar
Bowler, J. O., Mackintosh, B., Dunn, B. D., Mathews, A., Dalgleish, T., & Hoppitt, L. (2012). A comparison of cognitive bias modification for interpretation and computerized cognitive behavior therapy: Effects on anxiety, depression, attentional control, and interpretive bias. Journal of Consulting and Clinical Psychology , 80 (6), 1021–1033. https://doi.org/10.1037/a0029932
Article PubMed PubMed Central Google Scholar
Bracken, B. A., & Reintjes, C. (2010). Age, race, and gender differences in depressive symptoms: A lifespan developmental investigation. Journal of Psychoeducational Assessment , 28 (1), 40–53. https://doi.org/10.1177/0734282909336081
Buschmann, T., Horn, R. A., Blankenship, V. R., Garcia, Y. E., & Bohan, K. B. (2018). The relationship between automatic thoughts and irrational beliefs predicting anxiety and depression. Journal of Rational-Emotive & Cognitive-Behavior Therapy , 36 (2), 137–162. https://doi.org/10.1007/s10942-017-0278-y
Chauhan, K., Donahue, J., & Thompson, R. (2023). The predictive validity of the DSM-5 alternative model for borderline personality disorder: Associations with coping strategies, general distress, rumination, and suicidal ideation across one year. Personality and Mental Health , 17 (3), 272–281. https://doi.org/10.1002/pmh.1580
Clark, L. A., & Watson, D. (2022). The trait model of the DSM-5 alternative model of personality disorder (AMPD): A structural review. Personality Disorders , 13 (4), 328–336. https://doi.org/10.1037/per0000568
Conrod, P. J., O’Leary-Barrett, M., Newton, N., Topper, L., Castellanos-Ryan, N., Mackie, C., et al. (2013). Effectiveness of a selective, personality-targeted prevention program for adolescent alcohol use and misuse: A cluster randomized controlled trial. JAMA Psychiatry , 70 (3), 334–342. https://doi.org/10.1001/jamapsychiatry.2013.651
DeRubeis, R. J., Siegle, G. J., & Hollon, S. D. (2008). Cognitive therapy versus medication for depression: Treatment outcomes and neural mechanisms. Nature Reviews Neuroscience , 9 (10), 788–796. https://doi.org/10.1038/nrn2345
Góngora, V. C., & Castro Solano, A. (2017). Pathological personality traits (DSM-5), risk factors, and Mental Health. SAGE Open , 7 (3), 2158244017725129. https://doi.org/10.1177/2158244017725129
Hakulinen, C., Elovainio, M., Pulkki-Råback, L., Virtanen, M., Kivimäki, M., & Jokela, M. (2015). Depression and Anxiety , 32(7), 461–470. https://doi.org/10.1002/da.22376
Hopwood, C. J., Schade, N., Krueger, R. F., Wright, A. G., & Markon, K. E. (2013). Connecting DSM-5personality traits and pathological beliefs: Toward a unifying model. Journal of Psychopathology and Behavioral Assessment , 35 (2), 162–172. https://doi.org/10.1007/s10862-012-9332-3
Hu, L., & Bentler, P. M. (1999). Cutoff criteria for fit indexes in covariance structure analysis: Conventional criteria versus new alternatives. Structural Equation Modeling: A Multidisciplinary Journal , 6 (1), 1–55. https://doi.org/10.1080/10705519909540118
Huang, W., Cai, S., Zhou, Y., Huang, J., Sun, X., Su, Y., Dai, M., & Lan, Y. (2021). Personality profiles and personal factors Associated with psychological distress in Chinese nurses. Psychology Research and Behavior Management , 14 , 1567–1579. https://doi.org/10.2147/PRBM.S329036
Jourdy, R., & Petot, J. M. (2017). Relationships between personality traits and depression in the light of the big five and their different facets. L’Évolution Psychiatrique , 82 (4), e27–e37. https://doi.org/10.1016/j.evopsy.2017.08.002
Kestler-Peleg, M., Mahat-Shamir, M., Pitcho-Prelorentzos, S., & Kagan, M. (2023). Intolerance to uncertainty and self-efficacy as mediators between personality traits and adjustment disorder in the face of the COVID-19 pandemic. Current Psychology (New Brunswick N J) , 42 (10), 8504–8514. https://doi.org/10.1007/s12144-023-04465-9
Knowles, S. R., Apputhurai, P., & Bates, G. (2017). Development and validation of the brief unhelpful thoughts scale (BUTs). Journal of Psychology & Psychotherapy , 4 , 61–70. https://savvysciencepublisher.com/index.php/jppr
Kotov, R., Gamez, W., Schmidt, F., & Watson, D. (2010). Linking big personality traits to anxiety, depressive, and substance use disorders: A meta-analysis. Psychological Bulletin , 136 (5), 768–821. https://doi.org/10.1037/a0020327
Krueger, R., Derringer, J., Markon, K., Watson, D., & Skodol, A. (2013). The personality inventory for DSM-5—brief form (PID-5-BF)—adult . American Psychiatric Association.
Google Scholar
Lemma, S., Gelaye, B., Berhane, Y., Worku, A., & Williams, M. A. (2012). Sleep quality and its psychological correlates among university students in Ethiopia: A cross-sectional study. Bmc Psychiatry , 12 (1), 1–7. https://doi.org/10.1186/1471-244X-12-237
Lovibond, P. F., & Lovibond, S. H. (1995). The structure of negative emotional states: Comparison of the Depression anxiety stress scales (DASS) with the Beck Depression and anxiety inventories. Behaviour Research and Therapy , 33 (3), 335–343. https://doi.org/10.1016/0005-7967(94)00075-u
McDermut, W., Pantoja, G., & Amrami, Y. (2019). Dysfunctional beliefs and personality traits. Journal of Rational-Emotive & Cognitive-Behavior Therapy , 37 (4), 338–357. https://doi.org/10.1007/s10942-019-00315-5
McKay, M., Davis, M., & Fanning, P. (2011). Thoughts and feelings: Taking control of your moods and your life . New Harbinger.
Mirowsky, J., & Ross, C. E. (2017). Social causes of psychological distress (2nd ed.). Routledge.
Book Google Scholar
Nysaeter, T. E., Hummelen, B., Christensen, T. B., Eikenaes, I. U., Selvik, S. G., Pedersen, G., Bender, D. S., Skodol, A. E., & Paap, M. C. S. (2023). The Incremental Utility of Criteria A and B of the DSM-5 Alternative Model for Personality disorders for Predicting DSM-IV/DSM-5 section II personality disorders. Journal of Personality Assessment , 105 (1), 111–120. https://doi.org/10.1080/00223891.2022.2039166
Osman, A., Wong, J. L., Bagge, C. L., Freedenthal, S., Gutierrez, P. M., & Lozano, G. (2012). The Depression anxiety stress Scales-21 (DASS-21): Further examination of dimensions, scale reliability, and correlates. Journal of Clinical Psychology , 68 (12), 1322–1338. https://doi.org/10.1002/jclp.21908
Pollak, A. J., Dobrowolska, M., Timofiejczuk, A., & Paliga, M. M. (2020). The Effects of the Big Five Personality Traits on Stress among Robot Programming Students. Sustainability, 12 , 1–11. https://doi.org/10.3390/su12125196
Pretzer, J. L., & Beck, A. T. (2005). A cognitive theory of personality disorders. In M. F. Lenzen- weger & J. F. Clarkin (Eds.), Major Theories of Personality Disorder (2nd ed., pp. 43–113). New York, The Guilford Press.
Ross, S. M., Gottfredson, D. K., Christensen, P., & Weaver, R. (1986). Cognitive self-statements in depression: Findings across clinical populations. Cognitive Therapy and Research , 10 (2), 159–165. https://doi.org/10.1007/BF01173722
Samar, S. M., Walton, K. E., & McDermut, W. (2013). Personality traits predict irrational beliefs. Journal of Rational-Emotive & Cognitive-Behavior Therapy , 31 (4), 231–242. https://doi.org/10.1007/s10942-013-0172-1
Strickhouser, J. E., Zell, E., & Krizan, Z. (2017). Does personality predict health and well-being? A metasynthesis. Health Psychology , 36 (8), 797–810. https://doi.org/10.1037/hea0000475
Thimm, J. C., Jordan, S., & Bach, B. (2016). The personality inventory for DSM-5 short form (PID-5-SF): Psychometric properties and association with big five traits and pathological beliefs in a Norwegian population. BMC Psychol , 4 (1), 61. https://doi.org/10.1186/s40359-016-0169-5
Uliaszek, A. A., Amestoy, M. E., Fournier, M. A., & Al-Dajani, N. (2023). Criterion a of the alternative model of personality disorders: Structure and validity in a community sample. Psychological Assessment, 35(5), 453–461. https://doi.org/10.1037/pas0001225
Vittengl, J. R., Jarrett, R. B., Ro, E., & Clark, L. A. (2023). How can the DSM-5 alternative model of personality disorders advance understanding of depression?. Journal of Affective Disorders, 320, 254–262. https://doi.org/10.1016/j.jad.2022.09.146
Vîslă, A., Flückiger, C., Holtforth, G., M., & David, D. (2016). Irrational beliefs and psychological distress: A meta-analysis. Psychotherapy and Psychosomatics , 85 (1), 8–15. https://doi.org/10.1159/000441231
Vize, C. E., Ringwald, W. R., Edershile, E. A., & Wright, A. G. C. (2022). Antagonism in Daily Life: An exploratory ecological momentary Assessment Study. Clinical Psychological Science , 10 (1), 90–108. https://doi.org/10.1177/21677026211013507
Watkins, D. C., & Johnson, N. C. (2018). Age and gender differences in psychological distress among African americans and whites: Findings from the 2016 National Health interview survey. Healthcare (Basel Switzerland) , 6 (1), 6. https://doi.org/10.3390/healthcare6010006
Widiger, T. A., & Gore, W. L. (2014). Dimensional versus categorical models of psychopathology. The Encyclopedia of Clinical Psychology , 1–12. https://doi.org/10.1002/9781118625392.wbecp108
Widiger, T. A., & Oltmanns, J. R. (2017). Neuroticism is a fundamental domain of personality with enormous public health implications. World Psychiatry , 16 (2), 144–145. https://doi.org/10.1002/wps.20411
Wright, A. G. C. (2019). Antagonism from the perspective of interpersonal theory. In J. D. Miller, & D. R. Lynam (Eds.), The handbook of antagonism: Conceptualizations, assessment, consequences, and treatment of the low end of agreeableness (pp. 155–170). Elsevier Academic. https://doi.org/10.1016/B978-0-12-814627-9.00011-6
Chapter Google Scholar
Download references
Acknowledgements
The authors would like to thank all the individuals who participated in this research.
Provenance and peer review
Not commissioned, externally peer reviewed.
Open Access funding enabled and organized by CAUL and its Member Institutions. None.
Author information
Authors and affiliations.
Department of Psychological Sciences, Swinburne University of Technology, John St, Hawthorn, Melbourne, VIC, 3122, Australia
Ronan J. Galloghly & Simon R. Knowles
Department of Health Science and Biostatistics, School of Health Sciences, Swinburne University of Technology, Melbourne, Australia
Pragalathan Apputhurai
You can also search for this author in PubMed Google Scholar
Corresponding author
Correspondence to Simon R. Knowles .
Ethics declarations
Ethical approval.
All procedures performed in studies involving human participants were in accordance with the ethical standards of the institutional and/or national research committee and with the 1964 Helsinki declaration and its later amendments or comparable ethical standards.
Consent to participate
Informed consent was obtained from all individual participants included in the study.
Consent to publish
Informed consent to publish the study findings was obtained from all individual participants included in the study.
Conflict of interest
The authors have no conflict of interests in relation to this study.
Additional information
Publisher’s note.
Springer Nature remains neutral with regard to jurisdictional claims in published maps and institutional affiliations.
Rights and permissions
Open Access This article is licensed under a Creative Commons Attribution 4.0 International License, which permits use, sharing, adaptation, distribution and reproduction in any medium or format, as long as you give appropriate credit to the original author(s) and the source, provide a link to the Creative Commons licence, and indicate if changes were made. The images or other third party material in this article are included in the article’s Creative Commons licence, unless indicated otherwise in a credit line to the material. If material is not included in the article’s Creative Commons licence and your intended use is not permitted by statutory regulation or exceeds the permitted use, you will need to obtain permission directly from the copyright holder. To view a copy of this licence, visit http://creativecommons.org/licenses/by/4.0/ .
Reprints and permissions
About this article
Galloghly, R.J., Apputhurai, P. & Knowles, S.R. Exploring the role of dysfunctional attitudes and unhelpful thoughts in the relationship between personality traits and psychological distress in Australian University students. Curr Psychol (2024). https://doi.org/10.1007/s12144-024-06239-3
Download citation
Accepted : 05 June 2024
Published : 28 June 2024
DOI : https://doi.org/10.1007/s12144-024-06239-3
Share this article
Anyone you share the following link with will be able to read this content:
Sorry, a shareable link is not currently available for this article.
Provided by the Springer Nature SharedIt content-sharing initiative
- Australian university students
- Dysfunctional attitudes
- Personality traits
- Psychological distress
- Unhelpful thinking
- Find a journal
- Publish with us
- Track your research
- Open access
- Published: 10 July 2018
Predicting the Big Five personality traits from handwriting
- Mihai Gavrilescu ORCID: orcid.org/0000-0002-4616-7362 1 &
- Nicolae Vizireanu 1
EURASIP Journal on Image and Video Processing volume 2018 , Article number: 57 ( 2018 ) Cite this article
29k Accesses
43 Citations
13 Altmetric
Metrics details
We propose the first non-invasive three-layer architecture in literature based on neural networks that aims to determine the Big Five personality traits of an individual by analyzing offline handwriting. We also present the first database in literature that links the Big Five personality type with the handwriting features collected from 128 subjects containing both predefined and random texts. Testing our novel architecture on this database, we show that the predefined texts add more value if enforced on writers in the training stage, offering accuracies of 84.4% in intra-subject tests and 80.5% in inter-subject tests when the random dataset is used for testing purposes, up to 7% higher than when random datasets are used in the training phase. We obtain the highest prediction accuracy for Openness to Experience, Extraversion, and Neuroticism (over 84%), while for Conscientiousness and Agreeableness, the prediction accuracy is around 77%. Overall, our approach offers the highest accuracy compared with other state-of-the-art methods and results are computed in maximum 90 s, making the approach faster than the questionnaire or psychological interviews currently used for determining the Big Five personality traits. Our research also shows there are relationships between specific handwriting features and prediction with high accuracy of specific personality traits and this can be further exploited for improving, even more, the prediction accuracy of the proposed architecture.
1 Introduction
Handwriting has been used for centuries as a way of communication and expression for humans, but only recently its links to the brain activity and the psychological aspects of humans have been studied. The psychological study of handwriting with the purpose of determining the personality traits, psychological states, temperament, or the behavior of the writer is called graphology and is still a debatable domain as it lacks a standard, most of the handwriting interpretations being done subjectively by trained graphologists.
However, there have been various research papers showing the link between handwriting and neurological aspects of humans, one such study being the one of Plamondon [ 1 ], where it was shown that the brain forms characters based on habits of writers and each neurological brain pattern forms a distinctive neuromuscular movement which is similar for individuals with the same type of personality. Therefore, handwriting is, from this perspective, an accurate mirror of people’s brain.
Graphologists currently analyze multiple handwriting features in order to assess the psychological aspects of the writer, such as the weights of strokes [ 2 ], the trajectory of writing [ 3 ], the way the letter “t” or “y” are written [ 4 ], as well as other features related to how letters or words are written or how the text is positioned on the page.
In the current paper, we aim to build the first architecture in literature that is able to automatically analyze a set of handwriting features and evaluate the personality of the writer using the Five-Factor Model (FFM). To test this architecture, we propose the first database that links the FMM personality traits to handwriting features, which is a novel aspect of this research paper. The proposed system offers an attractive alternative to the standard FMM questionnaire or psychological interviews that are currently used for evaluating personality, because it is easier to use, it involves less effort, and is faster as well as removes the subjectivity from both subject’s (as usually the subject is asked to self-report on a specific questionnaire) as well as clinician’s sides (as typically psychologists are reviewing the questionnaire results and share opinions regarding the personality of the individual, opinions which can sometimes be prone to bias such that different psychologists might provide different evaluations). We show that our proposed system offers the highest accuracy compared to other state-of-the-art methods as well as share our findings regarding the relationship between several handwriting features and specific personality traits that can be further exploited to improve, even more, the accuracy of such a system.
In the following section, we present the state-of-the-art in the area of handwriting analysis, focusing on papers related to predicting the psychological traits of individuals. We continue in the subsequent section with describing the two models used (FMM and graphology analysis) followed by a detailed presentation of the three-layer architecture, as well as the classifiers and the structure of the neural network used. Finally, we detail the experimental results and share our findings and conclusions on the results obtained.
2 Related work
As mentioned previously, currently, there is no standard developed in predicting behavior based on handwriting, the majority of graphological analysis being done by specialized graphologists. However, research was conducted in the area of computer science which aimed to create such systems in order to recognize the behavior from handwriting in an easier way and also to standardize the graphological analysis. In the next paragraphs, we present the state-of-the-art in this area as well as several studies which made use of handwriting to determine the psychological traits or mental status of individuals.
Behnam Fallah and Hassan Khotanlou describe in [ 5 ] a research with a similar purpose as the one conducted in this paper, aiming to determine the personality of an individual by studying handwriting. The Minnesota Multiphasic Personality Inventory (MMPI) is used for training their system and a Hidden Markov Model (HMM) is employed for classifying the properties related to the target writer, while a neural network (NN) approach is used for classifying the properties which are not writer-related. The handwriting image is analyzed by these classifiers and compared with the patterns from the database, the output being provided in the form of the personality of the writer on the MMPI scale. Their system offers over 70% accuracy at this task. Similarly, in [ 4 ], an instrument for behavioral analysis is described with the task of predicting personality traits from handwriting. The approach takes into account the following handwriting features: letter “t,” lower loop of the letter “y,” the pen pressure, and the slant of writing. A rule-based classifier is used to assess the personality trait of the writer on the Myers-Briggs Type Indicator (MBTI) scale with also over 70% accuracy. The work of Chen and Tao [ 6 ] also provides an interesting exploratory study where they use combinations of Support Vector Machine (SVM), AdaBoost, and k-nearest neighbors (k-NN) classifiers for each of the seven personality dimensions in order to analyze a unique set of handwriting features. Their results are promising with accuracies ranging from 62.5 to 83.9%.
Although not aiming for personality traits, Siddiqi et al. [ 7 ] present a system that is able to predict the gender of individuals from scanned images containing their handwriting. A set of features is extracted from their writing samples, and artificial neural networks (ANNs) and Support Vector Machines (SVMs) are used to discriminate between the writing of a male and that of a female. The handwriting features employed are slant, curvature, texture, and legibility, computed in both local and global features. Evaluated on two databases under a number of scenarios, the system is able to predict with over 80% accuracy the gender of the writer. Similarly, in [ 8 ], it is proposed a way to describe handwritings based on geometric features which are combined using random forest algorithms and kernel discriminant analysis. The system is able to predict gender with 75.05%, age with 55.76%, and nationality with 53.66% when all the writers were asked to write the same text, and 73.59% for gender prediction, 60.62% for age prediction, and 47.98% for nationality prediction when each subject wrote a different text.
Another interesting research is the one conducted by Gil Luria and Sara Rosenblum [ 9 ] which uses handwriting behavior in order to determine the characteristics of both low and high mental workloads. They asked 56 participants to write three arithmetic progressions of different difficulties on a digitizer, and differences are seen in temporal, spatial as well as angular velocity spaces, but less in the pressure space. Using data reduction, they identify three clusters of handwriting types and conclude that handwriting behavior is affected by the mental workload. Zaarour et al. [ 10 ] show another interesting research where handwriting is employed to improve the performance of pupils through a system which takes as input different drawings and writings and, by means of a Bayesian network-based model, they can determine the writing style of the child which can be further analyzed by a child psychologist in order to advise parents on how to improve their child education. Similarly, Sudirman et al. [ 11 ] present a system that studies the behavior of children based on their handwriting, starting from the assumption that children are the best subjects to be analyzed in the context of handwriting as they are less influenced by cultural background and their cognition rate is evolving very fast. Therefore an automatic system is built which aims to determine the developmental disorders that the children might be suffering from, with accuracies of over 78%, making the approach attractive for both teachers as well as therapists for patients’ monitoring. Researchers in [ 12 ] present a system tasked with decreasing the time for job candidate selection in the pre-employment stage using automatic personality screening based on visual, audio, and lexical cues. The system extracts a set of relevant features which are used by a chain of machine learning techniques in order to predict candidates’ scores on the Five-Factor Model scale and a classifier is used to combine the prediction results from all the three cues. The experimental results show promising results in terms of performance on first impression database.
Another direction for many studies involving handwriting analysis is the detection of deceit. Luria et al. [ 13 ] show such research where a non-intrusive system analyzes the handwriting in the context of healthcare with the purpose of detecting the false information that patients provide about their health. As current ways of determining deception are invasive and do not comply with a clinician-patient relationship, such an approach of using the handwriting as a tool is attractive from research perspectives. Subjects participating in the experiment were asked to write true/false statements about their medical condition on a paper linked to a digitizer. After this first step, the deceptive and truthful writings of all the subjects are compared and used to divide the subjects into three groups according to their handwriting profiles. It is found that the deceptive writing takes longer to write and is broader and the two types of writings show significant differences in both spatial and temporal vectors. In [ 14 ], similar research is conducted, based on the same assumption that for people it is easier to tell the truth than to lie; hence, we need to see changes in both velocity and temporal spaces when analyzing the handwriting features. Conducted in 11 languages, this research demonstrates the same point as in [ 13 ], with the specific purpose of helping managers pinpoint sudden emotional changes and decode handwritten messages to reveal the true meaning of those messages as well as detect lies.
Besides detecting deceit, the handwriting is also used for predicting physical diseases. Researchers in [ 15 ] present a study where diabetics’ disease can be predicted with over 80% accuracy from handwriting. Similarly, in [ 16 ], the handwriting is used to predict micrographia (the decrease in the size of letters as well as the velocity and acceleration of writing) that is commonly associated with Parkinson’s disease (PD). The system, tested on PD-diagnosed patients, offers over 80% accuracy on 75 tested subjects. The study described in [ 17 ] is another research analyzing the link between the handwriting and children with autism spectrum disorder (ASD), knowing the fact that children with ASD have several weaknesses in handwriting. Boys aged 8–12 years and diagnosed with ASD were asked to take a digitized task in order to determine the handwriting performance using advanced descriptive methods. The study shows moderate to large links between handwriting performance and attention, ASD symptoms and motor proficiency, providing a relationship between handwriting and the ASD symptoms in terms of severity, attention, and motor behaviors.
Since handwriting analysis is a complex task requiring multiple techniques in order to analyze the multitude of handwriting features, there is a wide range of methods typically employed. For offline handwriting analysis, the normalization of the handwritten sample is the first step in order to ensure any possible noise is filtered out. As part of normalization phase, methods for removing the background noise (morphological approaches or Boolean filters are typically used [ 18 ]), sharpening (Laplace filters, Gradient masking or unsharp masking [ 19 ]), and contrast enhancement (unsharp mask filters [ 20 ]) are essential for ensuring the analysis of the handwriting is done with high accuracy. Also, as the contour of the written letters is essential for this task, methods for contour smoothing also need to be used, the most common ones being the local weighted averaging methods [ 21 ]. After all these processing steps are applied to the handwritten sample, the image needs to be compressed and converted to greyscale and different types of thresholding techniques can be employed for this step [ 22 ]. Post-compression, the written text needs to be delimited through page segmentation methods where techniques for examining the foreground and background regions are employed, the most common one being the white space rectangles segmentation [ 23 ]. One of the most challenging tasks is the one of segmenting the handwritten image into text lines and words. For this, the Vertical Projection Profile [ 24 ] method has shown the most promising results and this is the one that we use in this paper for both row and word segmentation. Regarding feature classification, different classifiers are used successfully for each of the handwriting features. For example, for lowercase letters “t” and “f,” the most common method used is template matching, for writing pressure gray-level thresholding methods are employed [ 22 ], while for connecting strokes the Stroke Width Transform (SWT) has shown the best classification accuracy compared to other state-of-the-art methods. In the following sections, we present in detail the classifiers used for each of the handwriting features analyzed in the current paper.
With all these in mind, the current research proposes a novel non-invasive neural network-based architecture for predicting the Big Five personality traits of a subject by only analyzing handwriting. This system would serve as an attractive alternative to the extensive questionnaire typically used to assess the FMM personality traits and which is usually cumbersome and non-practical, as well as avoid the use of invasive sensors. We focus our attention on handwriting because it is an activity familiar to almost everyone and can be acquired fast and often.
In the next section, we present the theoretical model and the architecture of our system.
3.1 Theoretical model
As mentioned in the previous section, our research is proposing a novel non-invasive neural network-based architecture for predicting the Big Five personality traits of an individual solely based on handwriting. Therefore, our study is based on two psychological tools: Big Five (Five-Factor Model—FMM) [ 17 ] and graphological analysis. We detail both these instruments in the next subsections.
3.1.1 Big Five (Five-Factor Model)
Big Five (Five-Factor Model) [ 25 ] is a well-known model for describing the personality of an individual. It is based on five basic personality traits which are grouped in sub-factors, as follows:
Openness to Experience : refers to people who can easily express their emotions and have a desire for adventure, appreciation for art, and out-of-the-box ideas. Typically, on this scale, people are rated based on the dichotomy: consistent vs. curious ;
Conscientiousness : refers to people who are dependable, have a predilection towards behaviors which are carefully planned, and are oriented towards results and achievements. On this scale, people are rated based on the dichotomy: organized vs. careless ;
Extraversion : refers to people who easily express positive emotions, like other’s people company, are assertive, and talkative. On this scale, people are rated on the dichotomy: outgoing vs. solitary ;
Agreeableness : refers to people who have a tendency to be compassionate instead of suspicious, as well as helpful, and tempered. On this scale, people are rated based on the dichotomy: compassionate vs. detached ;
Neuroticism: refers to people who lack emotional stability and control and tend to experience negative emotions easily, such as anger and anxiety, as well as a vulnerability to depression. On this scale, people are rated based on the dichotomy: nervous vs. confident.
FMM is successfully used on a wide variety of tasks. The research conducted in [ 26 ] shows that compared to other methods for assessing the personality of an individual, FMM offers more stability over time, the Big Five personality types reaching their stability peak 4 years after starting work. FMM has also proved to be useful in determining personality disorders, such as depression or anxiety, and even substance use, and was shown to be an indicator for different physical diseases, such as heart problems, cancer, diabetes or respiratory issues [ 27 ]. It is also successfully used in the area of career development and counseling as well as team performance, but also for improving learning styles and the academic performances of students [ 28 ]. Because of its extensive use and broad perspective of applications we employ it in our current study.
3.1.2 Graphological analysis
Typically, when analyzing the handwriting of an individual, graphologists are looking for a specific set of features, each of them conveying a specific message [ 29 ]. The main handwriting features used and the ones that we explore in the current paper are the following: baseline, word slant, writing pressure, connecting strokes, space between lines, lowercase letter “t,” and lowercase letter “f.” Examples of each of these features and their types as explained in [ 30 ] can be observed in Table 1 .
The baseline of the handwriting refers to the line on which the written words flow. It is further divided into ascending baseline (associated with optimistic people), descending baseline (associated with pessimistic people and over-thinkers), and leveled (associated with people with high levels of self-control and reasoning).
The word slant refers to how the words are written in terms of inclination/slant. Possible slant types are the following: vertical slant (associated with people who can easily control their emotions), moderate left slant (associated with people who find it hard to express emotions), extreme left slant (associated with people who want to be in permanent control and suffer from self-rejection), moderate right slant (associated with people who can easily exteriorize their emotions and opinions), and extreme right slant (associated with people who are impulsive and lack self-control).
The writing pressure refers to the amount of pressure that is applied to the pen on the paper: light writer (refers to people who hardly get affected by traumas), medium writer (refers to people who are usually affected by pain or traumas), and heavy writer (refers to people who are deeply affected by traumas and emotions).
Connecting strokes refer to how the letters composing words are connected to each other. These are dichotomized into not connected (refers to people that can hardly adapt to change), medium connectivity (refers to people who can adapt to change as well as like changing environments), and connected letters (refers to people who can quickly adapt to change).
Lowercase letter “t” typically refers to how the t-bar on the letter “t” is written. If it is written very low , it is an indication of low self-esteem, if it is written very high it is an indicator of high self-esteem.
Lowercase letter “f” refers to how the letter “f” is written. If it has an angular point , the person can be easily revolted, if it has an angular loop , the person has a strong reaction to obstacles, if it has a narrow upper loop it is usually associated with narrow-minded people, if it is cross-like it is associated with an increased level of concentration, and if it is balanced it is an indicator of leadership abilities.
Spaces between lines refer to the space left by the writer between two consecutive lines. We can have lines separated, evenly spaced (associated with people who can organize work and have clear thoughts) or lines crowded together with overlapping loops (associated with people with confused thinking and poor organizational skills).
3.2 Proposed architecture
We design the architecture on three layers as follows: a base layer where the handwriting sample is normalized and the handwriting features are acquired, an intermediary layer where a Handwriting Map is built based on the handwriting features provided by the base layer, and a top layer where a neural network is used in order to determine the Big Five personality type of the writer. In the following subsections, we present each of these layers in detail.
3.2.1 Base layer
The base layer has the primary purpose of converting the scanned handwriting in the set of handwriting features mentioned in previous sections. A flowchart of the central processing blocks of this layer can be observed in Fig. 1 .
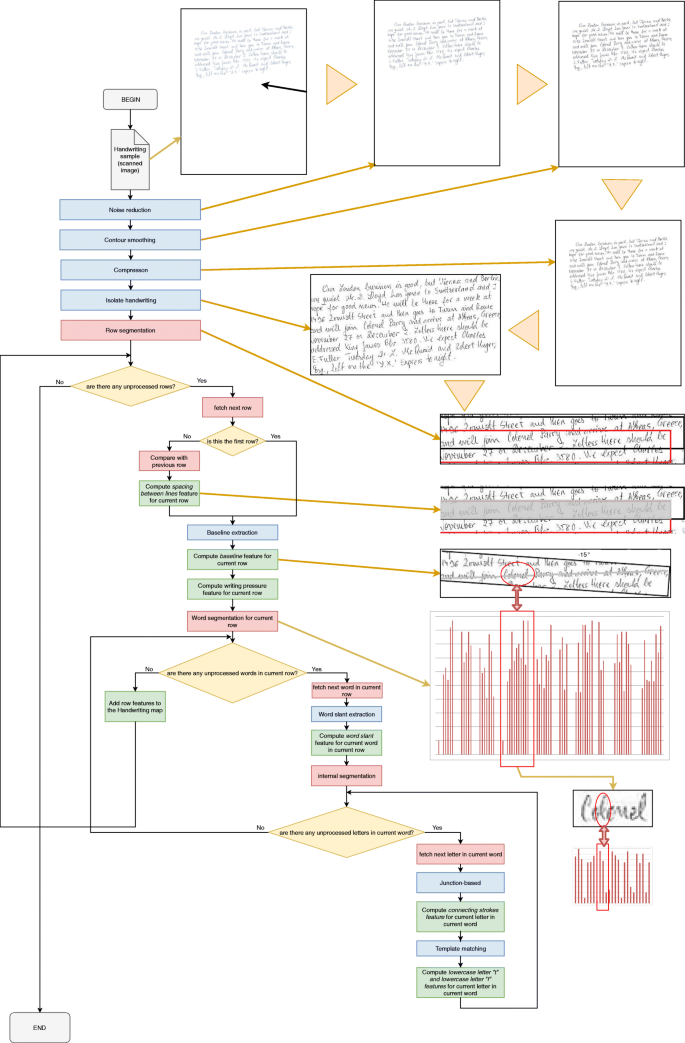
Flowchart of the base layer and handwriting features extraction
The main steps are detailed below:
Normalization:
◦ Noise reduction : in order to remove the noise added by the scanning device or the writing instrument which typically cause distortion, disconnected strokes or unwanted lines or points, we use three filters. Boolean filters are used for removing the textured background as they were shown to outperform other morphological methods for cases when the text is written on highly texturized backgrounds both in terms of accuracy and processing time [ 18 ]. For sharpening, we use the ramp width reduction filter as it is known as the most effective algorithm for ramp edge sharpening [ 19 ]. Adaptive unsharp masking is employed for adjusting the contrast [ 20 ] which is widely used as an effective method for contrast enhancement.
◦ Contour smoothing : in order to reduce the possible errors that appear due to unwanted movement of writer’s hand during writing we use an optimal local weighted averaging method [ 21 ] ensuring that these glitches are filtered out and only the strokes relevant for our analysis are kept. We opted for this algorithm as opposed to other less complex local weighted averaging methods because this method is known to provide more accurate estimations of contour point positions, tangent slopes, or deviation angles which are essential for our handwriting analysis task.
◦ Compression : we used global thresholding in order to convert the color images to binary. We used the histogram modified by integral ratio [ 22 ] in order to determine the global threshold value as it was shown to provide better performance compared to other compression techniques.
◦ Isolation of handwriting in the page : in order to only keep the handwritten text for the next steps of our handwriting analysis task, we use the white space thinning method [ 23 ] as it is a simple and fast method for this task; hence, we cut the page recursively on the two dimensions until only the handwritten text is delimited.
Row segmentation : For row segmentation, we use the Vertical Projection Profile (VPP) method [ 24 ] as it was showed to provide the best classification accuracy compared to other row and word segmentation methods. We, therefore, analyze the sum of pixels for each row in the image and determine as row boundaries those with a sum lower than 8% of the highest pixel sum in the text sample. The threshold of 8% was chosen through trial-and-error after conducting tests on 100 handwriting samples using a leave-one-out approach and the average accuracy for correct row segmentation was 98.5%. Following this step, every row in the handwritten text has a corresponding bounding rectangle.
◦ Spacing between lines feature : based on the bounding rectangles delimiting each row from handwriting, we determine the amount of overlap between two consecutive rows. If the overlap is higher than 15% of the sum of both row bounding rectangles’ surfaces, we consider that the rows are crowded together , otherwise, they are considered evenly spaced . The 15% threshold was determined to be optimal for ensuring over 98% accurate classification of this handwriting feature.
◦ Baseline feature : in order to determine the baseline features for each row, we use the method depicted in [ 31 ] where we study the pixel density of each segmented row rectangle and we rotate the rectangle within the − 30° and + 30° angle thresholds until the highest pixel density is horizontally centered. This method is broadly used for baseline feature extraction offering higher classification accuracy and faster convergence compared to other state-of-the-art methods. If the rotation needed to align the highest pixel density horizontally is within [− 5°; + 5°], we consider that we have a leveled baseline , if it is within [− 30°; − 5°], an ascending baseline, and within [+ 5°; + 30°] a descending baseline .
◦ Writing pressure feature : we use the standard gray-level thresholding method that is widely used for the task of writing pressure classification [ 32 ] with high accuracy and fast convergence. We analyze the grayscale values for the segmented rectangle containing the row and we calculate the average for the segmented row. The result is classified as light writer for a value within 25 and 50%, medium writer for a value within 10 and 25%, and heavy writer for a value within 0% (absolute black) and 10%.
Word segmentation : In order to further segment the words in a row, we use the same VPP method [ 24 ] that we employed for row segmentation as it was shown to provide better classification results than other state-of-the-art methods. We compute the height of the row first and use it for comparison purposes in order to determine whether a space between two strokes is indeed an inter-word space or not. We generate a vertical projection profile where we determine the pixel density for each vertical column and we determine the columns with low density, which are considered candidates for spaces between words. As there are cases when such gaps might not correspond to actual word separation spaces, we consider them spaces only if the number of consecutive columns with low density is not lower than 10% of the row height. The 10% threshold was determined through trial-and-error after testing the algorithm on 100 handwritten samples and obtaining the highest word segmentation accuracy of 98.2%. The segmented words are bounded by rectangles similarly as in the row segmentation case.
◦ Word slant feature : in order to determine the word slant feature, we use the same technique described in [ 33 ]. We calculate the vertical pixel density histogram for each angle within [− 20°; + 20°] and for each column in the histogram we determine the number of pixels and divide it with the highest and lowest pixel in the analyzed word segment. The values from all columns are then summed and the angle where the computed sum is the highest is considered to be the slant of the writing. We then classify the word slant as follows: if the angle is within [− 2.5°; + 2.5°], it is a vertical slant ; if it is within [− 7.5°; − 2.5°], it is a moderate left slant ; if it is lower than − 7.5°, it is an extreme left slant ; if it is within [+ 2.5°; + 7.5°], it is a moderate right slant ; and if it is higher than + 7.5°, an extreme right slant .
Letter segmentation : for segmenting the letters from each delimited word segment, we use the stroke width transform (SWT) [ 34 ] method for determining the average stroke width of the word. We use this operator because it is local and data dependent, making it faster and more robust than other methods that need multi-scale computations. We then create a projected profile for the word segment and determine the columns where the projection value is lower than 8% than the highest projected value in the word. For the identified strokes, we determine their width and compare it with the word’s average stroke width. If it is lower than 50%, we create a bounding box surrounding the character and we crop out the bounding box from the word segment. The 50% threshold was determined to be optimal after testing the method on 100 handwritten samples and obtaining 98.2% accuracy for letter segmentation. With the remaining part of the word segment, the process is repeated until all letters are identified.
◦ Connecting strokes feature : in order to compute the connecting strokes feature, we use the letter segmentation algorithm previously described and we compare each stroke width connecting two consecutive letter bounding boxes with the average stroke width of the word. If the stroke width is below 10% of the average stroke width of the word, we consider it as not connected ; if it is above 30%, we consider it connected ; and if it is between 10 and 30%, it is considered as having medium connectivity.
◦ Lowercase letter “t” feature : as letters are now delimited in corresponding bounding boxes, we use template matching to compare each letter to a set of predefined templates of letter “t” from the Modified National Institute of Standards and Technology (MNIST) database [ 35 ]. The templates were previously divided into the two categories of letter “t” ( very low “t” bar and very high “t” bar ), and we use Euclidean similarity to measure the letter matching to the chosen MNIST prototypes. The threshold matching determined as optimal through trial-and-error is 0.88 and the accuracy for detecting the right letter “t,” tested on 100 handwriting samples with a leave-one-out approach, is 98.2%.
◦ Lowercase letter “f” feature : we use the same method depicted for letter “t” with the difference that the letter “f” templates from the MNIST database are divided into five categories corresponding to the ones analyzed (angular point, angular loop, narrow upper loop, cross-like and balanced). The threshold, in this case, is 0.92 corresponding to an accuracy of 97.5%.
3.2.2 Intermediary layer (Handwriting Map)
As we previously mentioned, the base layer offers as inputs to the intermediary layer the handwriting feature types for each letter in the exemplar. These are coded in the Handwriting Map (HM) using a binary code. Therefore if, for example, connecting strokes have medium connectivity, the code for this is 010 (0—connected, 1—medium connectivity, 0—not strongly connected). Typically, for each analyzed letter, we have the following possible codes associated with each of the seven handwriting features that all compose one row in the HM:
Baseline : position 1 to 3 : possible values are 100—ascending, 010—descending, 001—leveled;
Connecting strokes : position 4 to 6 ; possible values are 100—not connected, 010—medium connectivity, 001—strongly connected;
Word slant : position 7 to 11 ; possible values are 10000—vertical slant, 01000—moderate left slant, 00100—extreme left slant, 00010—moderate right slant, 00001—extreme right slant;
Writing pressure : position 12 to 14 ; possible values are 100—light writer, 010—medium writer, 001—heavy writer;
Lowercase letter “t” : position 15 to 16 ; possible values are 10—very high; 01—very low; 00—not a lowercase letter “t”;
Lowercase letter “f” : position 17 to 21 ; possible values are 10000—cross-like, 01000—angular loop, 00100—angular point, 00010—narrow upper loop, 00001—balanced; 00000—not a lowercase letter “f”;
Space between the lines : position 22 to 23 ; possible values are 10—evenly spaced, 01—crowded together.
Therefore any row entry in the map has the following structure: [100][010][00010][100][00][00010][10] (which means ascending baseline —100, medium strokes connectivity —010, moderate right slant —00010, light writer —100, not a lowercase letter “t” —00, Narrow Upper Loop on lowercase letter “f” —00010, evenly spaced lines —10).
Two observations should be made about the above-constructed mapping:
For baseline , we might have the same code for all letters;
For space between the lines , we might have the same code for all letters that are associated with a row in the handwritten sample.
Therefore, each letter in the handwriting sample generates a row in the HM in the form of a binary code which is then used in the top layer in a pattern recognition task in order to determine the Big Five personality traits.
3.2.3 Top layer
As we have detailed earlier, we have an HM that contains for each letter its handwriting features in the form of a binary code. Therefore, the HM is a matrix containing all the letters in the handwriting exemplar together with their coded features and based on this the system should be able to determine the Big Five personality trait of the writer.
As the task is a pattern recognition task and also considering that our architecture is bottom-up with no feedback loops, we use a feed-forward neural network. Also, with the same premises in mind, the training method used is backpropagation , which has proven to be very effective and offers fast learning in similar cases [ 36 ].
We define only one neural network that is called the Five-Factor Model–Neural Network (FFM-NN). In order to avoid overfitting it by fetching all the letters from the exemplar, we fetch them by rows and we consider that we do not have more than 70 letters on each row. If a row in the handwritten sample has more than 70 letters, only the first 70 are analyzed. More than this, this approach offers the ability to have multiple tests done on the neural network and we can average the results in order to reach more conclusive ones. As we have 23 entries for each row in the HM, in total we have 1610 input nodes in FFM-NN.
The output layer contains five nodes for each of the five dimensions of FMM. Each node computes a 0 if the subject is found on the lower side of the analyzed dimension, and 1 if it is found on the higher side of the dimension (e.g., a 1 for Openness to Experience means that the subject is more curious than consistent, while a 0 for Neuroticism means that the subject is more inclined towards being nervous than confident).
If we consider N in the number of input training vectors and an N-dimensional set of input vectors for the FFM-NN neural network X FFM − NN = { x FFM − NN n }, n = 1, 2… N in , so that x FFM − NN = [ x FFM − NN 1 , x FFM − NN 2 … x FFM − NN N ] T , and a K out the number of output vectors and K-dimensional set of output vectors Y FMM − NN = { y FMM − NN k }, k = 1, 2… K out so that y FFM − NN = [ y FFM − NN 1 , y FFM − NN 2 , …, y FFM − NN K ] T , and if we denote the matrix of weights between input and hidden nodes, W FFM − NNH , the matrix of weights between the hidden nodes and the output nodes W FFM − NNO with L the number of hidden nodes, and f FMM − NN 1 a and f FMM − NN 2 a the activation functions, the expression form for the output vectors can be written as follows:
The input features for each letter on a row is fetched to the input nodes which then distributes the information to the hidden nodes and computes the weighted sum of inputs sending the result to the output layer through the activation function. In backpropagation stage, the Average Absolute Relative Error (AARE) (2) is calculated as the difference between what is expected ( y FMM − NN e ) and what is determined ( y FMM − NN p with p = 1, 2… N in ) and W FMM − NNH and W FMM − NNO weight matrices are calibrated in order to minimize the AARE FMM − NN :
With the purpose of +/− balance in the hidden layer, the activation function chosen for the input layer is tanh, also considering it offers fast convergence and has a stronger gradient than the sigmoid function. Because the final task of the neural network is a predictive one, we use sigmoid as activation function for the hidden layer, taking into account its non-linearity and that its output is in the range of [0,1]. Conducting various tests, through trial-and-error, we determined that the optimal number of hidden nodes in order to avoid overfitting is 1850. The optimal learning rate is determined as 0.02, the optimal momentum is 0.4, and 200,000 training epochs are needed to train the system in an average of 8 h on an Intel i7 processor computer. We use Gradient Descent to learn the weights and biases of the neural network until AARE is minimized and, in order to ensure an even spread of the initial weights, we use the Nguyen-Widrow weight initialization. The structure of the neural network can be observed in Fig. 2 .
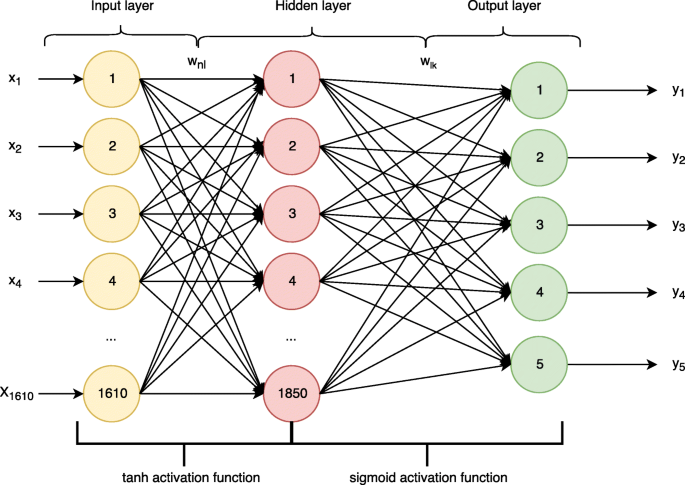
FMM—neural network structure
3.3 Overall architecture
3.3.1 training database and handwriting text samples.
For testing the above-described architecture, we create our database containing both handwritten exemplars as well as the FMM personality trait of the writer. In collecting this, we involved 128 individuals, out of which 64 were males and 64 females, with ages between 18 and 35, all of them participating to this experiment in accordance and aware of the Helsinki Ethical Declaration.
Each of the 128 subjects was asked to take the FMM questionnaire as well as provide six handwriting samples. The FMM questionnaire results were analyzed by specialized psychologists to assess their results on the five personality dimensions. In what it concerns the six handwriting samples, two of them are a predefined text representing the London Letter [ 32 ], a standard exemplar broadly used by graphologists for handwriting analysis, while the others are minimum 300 words texts that subjects could write freely and randomly. All text samples are collected in the English language.
To summarize, for each subject involved in training we have their corresponding FMM personality dimensions results as well as six handwriting samples, out of which two are the London Letter.
In Fig. 3 we can observe an example of the London Letter collected from one of the subjects. The London Letter is chosen because of the handwriting features that we are collecting, such that lowercase letter “t” is assessed at the beginning (e.g., “ t o”, “ t hen”, “ t onight”), middle (e.g., “Swi t zerland”, “Le tt ers”), and end (e.g., “quie t ”, “expec t ”) of words, lowercase letter “f” is analyzed at the beginning of words (e.g., “ f or”) or intercalated (e.g., “le f t”) as well as other situations that pose difficulties to writers and help us better discriminate between other handwriting features, such as: words starting with uppercase (e.g., Zermott Street), group of longer words (e.g., “Athens, Greece, November”), words containing doubled letters (e.g., “Greece”, “Zermott”), use of letters that need additional strokes (such as x, z, i, j; e.g., “E x press”, “Swit z erland”, “V i enna”, “ j o i n”), and intercalating numbers and/or punctuation (e.g., “King James Blv. 3580.”).
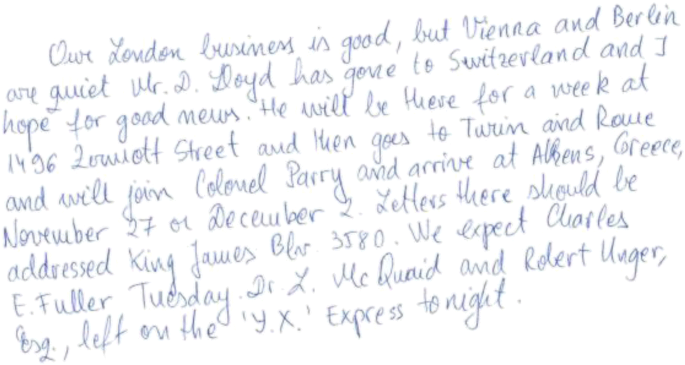
Handwritten sample of The London Letter
In the following section, we present the training as well as testing stages and how they use the above-described database.
3.3.2 Training and testing phases
The proposed architecture is built using 55,000 code lines in Scala programming with Spark Library. The testbed is functioning on an i7 processor with 8GB of RAM and it is designed to work in two stages: training and testing. The overall architecture can be seen in Fig. 4 .
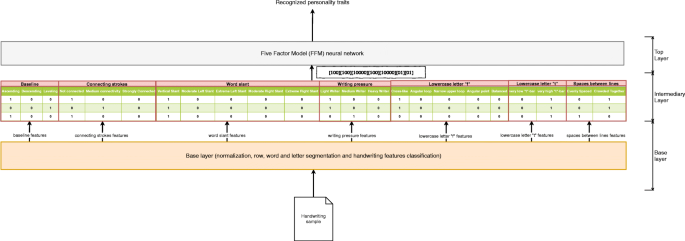
Proposed architecture—overview
In the training stage , the FMM-NN needs to be trained to learn the handwriting patterns and compute the right values for the five personality dimensions. We, therefore, use a set of handwriting samples as training samples that are fetched to the base layer. The handwriting samples are first normalized, then the words are split into letters and the handwriting features for each letter are extracted and sent to the intermediary layer. In the intermediary layer, the HM is built which contains a row for each letter from the handwritten sample in the form of binary codes as previously presented. Every time we have handwritten features collected for 70 new letters, these are fetched to the FMM-NN which is trained via backpropagation so that its output is the one obtained from the FMM questionnaire. When AARE is low enough and the training samples are finished the system is considered trained and can be tested.
In the testing stage , the analyzed handwriting exemplar is also normalized and split into letters in the base layer. The letters are then analyzed and their features are determined and sent to the intermediary layer which computes the HM. When 70 new letters are computed in HM, these are sent to the FMM-NN which provides an output representing its predicted FMM personality dimensions in the form of five binary codes, as previously explained. When on five consecutive rows (five sets of 70 letters) we have the same binary codes, the system considers that those are the personality dimensions of the writer and outputs the final result. If there are no five consecutive rows generating the same binary output (meaning that different personality traits are detected in any five consecutive rows), the result is flagged as Undefined. We chose five consecutive rows as they correspond to an average sized word (of five letters) and we determined that reducing or increasing this threshold results in lower system accuracies.
In the next section, we show the experimental results after testing the architecture as well as a comparison with state of the art.
4 Experimental results and discussion
As we described previously, due to the lack of a publicly available database that would relate the handwriting features with FMM, we built our database to support this study. The database contains handwritings collected from 128 subjects (64 females and 64 males), with ages between 18 and 35 years old as well as their results after filling in the FMM questionnaire which was subsequently analyzed by specialized psychologists to ensure the FMM personality traits are evaluated correctly. For testing the degree of generalization of the proposed approach when dealing with random handwritten text and the influence of the predefined handwritten text in both training and test phases, the database is divided into two main datasets: controlled dataset (consisting of handwriting samples where subjects were asked to write a predefined text—the London letter), and the random dataset (consisting of handwriting samples where subjects wrote a minimum 300 words text freely). Also for testing purposes, in order to determine the ability of the proposed approach to recognize the FMM features of a writer that was not involved in training, we divide the database in writer-specific datasets which contain handwritings only from one specific writer. Each sample from the database is therefore tagged with both the type of dataset to which it pertains (controlled or random) as well as a unique code specifying the writer. The tests conducted in both the intra-subject and inter-subject methodologies are presented in the following sections.
4.1 Own database tests
4.1.1 intra-subject methodology.
Intra-subject methodology refers to training and testing the system on handwriting samples coming from the same writer. We, therefore, use n -fold cross-validation for each writer-specific dataset taking also into account the handwriting type (controlled or random). For example, for determining the accuracy of the method when the controlled dataset is used both in test and training phases, since we have only two samples for each writer, we use leave-one-out cross-validation where one of the samples is involved in training and the other is used for testing and vice-versa. Similarly, for determining the accuracy of the method when the random dataset is used for training and the controlled dataset of testing, we train the system on the writer-specific random dataset (containing four samples) and we test it on the writer-specific controlled dataset (containing two samples) via n -fold cross-validation. The tests are repeated for all 128 users and the results are averaged and are detailed in Table 2 .
We observe the highest prediction accuracy when the system is trained and tested on the controlled dataset reaching 85.3% prediction accuracy, however when we use the same controlled dataset for training and we test the proposed approach on samples from the random dataset the accuracy does not decrease by much, reaching 84.4%. This is an important observation as it shows that the need for predefined handwritten texts is only for training purposes, while for testing we can use random texts which perform roughly similar to the predefined one. Similarly, when the controlled dataset is used for training, the cases where the personality type is flagged as Undefined is the lowest (0.2%), also sustaining the idea that the controlled dataset adds more value to the prediction accuracy when used in training stage as opposed to the random one. This indicates that if the text exemplar used for training handwriting samples is adequately chosen in order to train the neural network on all the analyzed features, using such an application we do not need a standard text for testing and we can ask the subject to write any text they like, making the approach more flexible and easy to use.
The highest prediction accuracies are obtained for Openness to Experience (88.3%—when the system was trained on the controlled dataset and tested on the random dataset), followed by Extraversion (87.4%), Neuroticism (85.3%), while for Conscientiousness and Agreeableness the results are lower, around 80%.
The average number of rows needed to compute the FMM personality types is 9 for the case where the controlled dataset is used in training and the random one for testing and maximum 14 when the random dataset is used for training. Typically, for a row to be computed it takes an average of 5 s, hence the system provides the FMM personality type in no more than 45 s when the controlled dataset is used in training, making the approach fast and attractive for clinicians as an alternative to the FMM questionnaire or psychological interviews.
4.1.2 Inter-subject methodology
In inter-subject methodology, we train the system with handwriting samples coming from different writers than those used for testing in order to determine the ability of the proposed approach to extrapolate the trained data to new writers. We used n -fold cross-validation, keeping the database division in controlled and random datasets, and ensuring that handwritings from the writer tested have not been used for training. For example, for training the accuracy of the system when trained on handwritings containing a predefined text (controlled dataset) and testing on handwritings with random text in inter-subject methodology, we use the controlled handwritings from all subjects except the one used for training (2 controlled samples/subject × 127 subjects used in test = 254 samples), and we test using n -fold cross-validation on random handwriting samples from the remaining subject (four samples). The tests are repeated until all subjects and all their samples are used in the testing phase and the averaged results are detailed in Table 3 . To note that we also conduct several tests where we decreased the number of subjects involved in training in order to analyze the change in accuracy when the number of subjects is increased.
Similarly to the intra-subject methodology, the highest prediction accuracy is obtained when the controlled dataset is used for both training and testing and when the system is trained on the highest number of subjects. In this case, the overall prediction accuracy is 84.5%. It is interesting to observe that reducing the number of subjects involved in training does not result in many decreases in terms of prediction accuracy, such that if we use only 96 subjects in training the prediction accuracy is 1.8% lower and when we use 64 subjects in training it decreases with about 1.6% more. This little decrease as well as the fact that high accuracies are obtained when the controlled dataset is used for training and the random dataset for test (78.6%) compared to when the random datasets are used for both training and testing (when the prediction accuracy was 6% lower) provides the same conclusion as in the intra-subject methodology, that the controlled dataset adds more value to the performance of the system if used in the training stage, helping the system learn better the handwriting features. Once learned, for testing purposes random texts can be used in the handwriting sample, providing only 5% lower accuracy, but making the system more practical (in the sense that the subject can write freely whatever text he/she wants). Similarly as in the case for intra-subject methodology, the fact that the number of cases where the personality type is flagged as Undefined is lower when the controlled dataset is used for training, with a maximum of just 0.7%, is another indicator that using the controlled dataset in the training stage improves the prediction accuracy by improving system’s ability to discriminate between different FMM personality types.
As in the intra-subject tests, in inter-subject ones, the highest prediction accuracy is obtained for Openness to Experience (88.6%), Extraversion (87.1%), and Neuroticism (86.3%), while lower accuracies are obtained for Consciousness and Agreeableness, roughly around 80%. When controlled datasets are used for training, the average number of rows needed to determine the personality types is 12 taking around 60 s which supports the idea that the proposed approach is fast and can be an attractive alternative to the FMM questionnaire or psychological interviews commonly used for evaluating the FMM personality types.
4.1.3 Relationship between the handwriting features and FMM
We conduct the next experiment in order to see which handwriting feature is associated with each of the five personality traits in FMM. In order to accomplish this, we create a background application that checks the HM and counts each occurrence of all the handwriting feature classifications against each of the five personality traits. This is acquired with the system trained on controlled datasets for 127 subjects and tested on the random datasets for the remaining subject with n -fold cross-validation, averaging the results. The results obtained are highlighted in Table 4 .
It can be observed that there are several links between the five personality types and the handwriting features, such that extreme left word slant, descending baseline, and cross-like lowercase letter “f” are associated with Conscientiousness, while medium connected strokes, medium right word slant, and balanced lowercase letter “f” are associated with Openness to Experience. These findings are significant as they can be used to optimize the proposed architecture such that the neural network is trained and tested only on the handwriting features that have relevant information about the personality traits that are investigated, the others being filtered out.
4.2 Comparison with state-of-the-art
As currently there is no standard public database that is broadly used for testing and comparing different architectures and methods for evaluating personality evaluation based on handwriting, we test the most common methods for assessing personality from handwriting on our database and compare the results with those obtained from our proposed approach. As it can be observed, our approach offers 84.4% accuracy for intra-subject tests and 80.5% accuracy for inter-subject tests, surpassing the rule-based classifier approach of Champa and AnandaKumar [ 4 ] with 12.5%, as well as the SVM, k-NN, and Ada-Boost combination of classifiers employed by Chen and Lin in [ 6 ], with 7.2%, respectively. Similarly, the proposed approach performs slightly better at the task of determining the FMM personality traits based on handwriting compared with the HMM-NN combination employed by Fallah and Khotanlou [ 5 ]. The results are detailed in Table 5 .
5 Conclusions
We described the first non-invasive three-layer architecture in literature that aims to determine the Big Five personality type of individuals solely by analyzing their handwriting. This novel architecture has a base layer where the handwritten sample in the form of a scanned image is normalized, segmented in rows, words, and letters and based on the computed segments the handwriting features are determined; an intermediary layer where a Handwriting Map (HM) is computed by binary coding the handwriting feature type of each letter; and a top layer where a feed-forward neural network is trained via backpropagation to learn the patterns from the HM map and compute the FMM personality traits.
In order to train and test this novel architecture, due to lack of any database that would link the FMM personality traits with handwriting samples, we create the first such database containing the FMM personality traits of 128 subjects and six handwriting samples from each of them with both predefined text (referred to as controlled dataset) as well as random text freely chosen by subjects (referred to as random dataset). We test our novel architecture on this database in both intra-subject and inter-subject methodologies and we obtain the highest prediction accuracies when the controlled dataset is used in the training stage, which shows that choosing a predefined text to be used for training the system is an important point in order to reach high accuracies, while testing can be done on random texts with no essential need for predefined texts to be used. This is an essential finding for real applications of such a systems, as it provides flexibility to the end-user, such that he/she will not have to write a predefined text every time, instead writing it only at the beginning in order to train the system, and then, to evaluate his/her personality traits at any given moment of time, he/she can use any random text he/she wants. In intra-subject tests, when the controlled dataset is used for training and random dataset for testing, we obtain an overall accuracy of 84.4%, while in inter-subject tests with a similar test-case we obtain an overall prediction accuracy of 80.5%. The highest prediction accuracies are obtained for Openess to Experience, Neuroticism, and Extraversion, reaching above 84%, while for Agreeableness and Conscientiousness we only obtained roughly around 77%. Overall, the prediction accuracy of the system is higher than that of any other state-of-the-art method tested on the same database. Another significant finding is that we determined several relationships between the prediction with high accuracy of specific FMM personality traits and the handwriting features analyzed which can be further exploited to improve the accuracy of the system. The accuracy of the system can also be further improved either by analyzing other handwriting features together with the seven ones already analyzed in our study or grouping these features based on the relevant information they offer in this task and filter out the irrelevant ones for each of the five personality traits. This will be the direction of our future research.
The proposed system computes the results in no more than 90 s which makes it faster than the current ways of determining personality traits through extensive self-report questionnaires, usually more cumbersome and time-consuming to fill in and involving more effort from both subject’s and psychologist’s side which will have to post-process the questionnaire results and evaluate the five personality traits; this shows that our current approach could be used as an attractive, faster, and easier to use alternative to these commonly used personality evaluation techniques.
Abbreviations
Average Absolute Relative Error
Artificial neural networks
Autism spectrum disorder
Controlled Dataset
Five-Factor Model–Neural Network
Five-Factor Model
Handwriting Map
Hidden Markov Model
K-nearest neighbors
Myers-Briggs Type Indicator
Minnesota Multiphasic Personality Inventory
Modified National Institute of Standards and Technology
Neural network
Parkinson’s disease
Random Dataset
Random Access Memory
Support Vector Machines
Stroke Width Transform
Vertical Projection Profile
R Plamondon, Neuromuscular studies of handwriting generation and representation. International Conference on Frontiers in Handwriting Recognition (ICFHR), 261 (2010) Kolkata, November 2010
Y Tang, X Wu, W Bu, Offline text-independent writer identification using stroke fragment and contour-based features. 2013 IEEE International Conference on Biometrics (ICB), 1–6 (June 2013)
M Naghibolhosseini, F Bahrami, A behavioral model of writing. International Conference on Electrical and Computer engineering (ICECE), 970–973 (December 2008)
HN Champa, KR Anandakumar, Automated human behavior prediction through handwriting analysis. 2010 First International Conference on Integrated Intelligent Computing (ICIIC), 160–165 (August 2010)
B Fallah, H Khotanlou, in Artificial Intelligence and Robotics (IRANOPEN) . Identify human personality parameters based on handwriting using neural networks (April 2016)
Google Scholar
Z Chen, T Lin, Automatic personality identification using writing behaviors:an exploratory study. Behav Inform Technol 36 (8), 839–845 (2017)
Article Google Scholar
I Siddiqi, C Djeddi, A Raza, L Souici-Meslati, Automatic analysis of handwriting for gender classification. Pattern. Anal. Applic. 18 (4), 887–899 (November 2015)
Article MathSciNet Google Scholar
S Maadeed, A Hassaine, Automatic prediction of age, gender, and nationality in offline handwriting. EURASIP Journal on Image and Video Processing 2014 , 10 (December 2014)
G Luria, S Rosenblum, A computerized multidimensional measurement of mental workload via handwriting analysis. Behav. Res. Methods 44 (2), 575–586 (June 2012)
I Zaarour, L Heutte, P Leray, J Labiche, B Eter, D Mellier, Clustering and Bayesian network approaches for discovering handwriting strategies of primary school children. Int. J. Pattern Recognit. Artif. Intell. 18 (7), 1233–1251 (2004)
R Sudirman, N Tabatabaey-Mashadi, I Ariffin, Aspects of a standardized automated system for screening children’s handwriting. First international conference on Informatics and Computational Intelligence (ICI) , 48–54 (December 2011)
J Gorbova, I Lusi, A Litvin, G Anbarjafari, Automated screening of job candidate based on multimodal video processing. Computer Vision and Pattern Recognition Workshops (CVPRW) (2017) IEEE Conference on , July 2017
G Luria, A Kahana, S Rosenblum, Detection of deception via handwriting behaviors using a computerized tool: Toward an evaluation of malingering. Cogn. Comput. 6 (4), 849–855 (December 2014)
TLP Tang, Detecting honest People’s lies in handwriting. J. Bus. Ethics 106 (4), 389–400 (April 2012)
SB Bhaskoro, SH Supangkat, An extraction of medical information based on human handwritings. 2014 International Conference on Information Technology Systems and Innovation (ICITSI), 253–258 (November 2014)
Drotar, P., Mekyska, J., Smekal, Z., Rektorova, I., Prediction potential of different handwriting tasks for diagnosis of Parkinson’s, 2013 E-Health and Bioengineering Conference, Pages 1–4, November 2013.
N Grace, PG Enticott, BP Johnson, NJ Rinehart, Do handwriting difficulties correlated with core symptomology, motor proficiency and attentional behaviors. Journal of Autism and Developmental Disorders , 1–12 (January 2017)
WL Lee, K-C Fan, Document image preprocessing based on optimal Boolean filters. Signal Process. 80 (1), 45–55 (2000)
Article MATH Google Scholar
JG Leu, Edge sharpening through ramp width reduction. Image Vis. Comput. 18 (6), 501–514 (2000)
SCF Lin et al., Intensity and edge based adaptive unsharp masking filter for color image enhancement. Optik - International Journal for Light and Electron Optics 127 (1), 407–414 (2016)
R Legault, CY Suen, Optimal local weighted averaging methods in contour smoothing. IEEE Trans. Pattern Anal. Mach. Intell. 18 , 690–706 (July 1997)
Y Solihin, CG Leedham, Integral ratio: a new class of global thresholding techniques for handwriting images. IEEE Trans. Pattern Anal. Mach. Intell. 21 , 761–768 (Aug. 1999)
Kai Chen, Fei Yin, Cheng-Lin Liu, Hybrid page segmentation with efficient whitespace rectangles extraction and grouping, Document Analysis and Recognition (ICDAR) 2013 12th International Conference on , pp. 958–962, 2013.
V Papavassiliou, T Stafylakis, V Katsouro, G Carayannis, Handwritten document image segmentation into text lines and words. Pattern Recogn. 43 , 369–377 (2010)
Costa, P.T. Jr., McCrae, R.R., Revised NEO Personality Inventory (NEO-PI-R) and NEO Five-Factor Inventory (NEO-FFI) manual, Psychol. Assess. Resources, Odessa, FL, 1992.
BW Roberts, D Mroczek, Personality trait change in adulthood. Curr. Dir. Psychol. Sci. 17 (1), 31–35 (2008)
M Jokela, C Hakulinen, A Singh-Manoux, M Kivimaki, Personality change associated with chronic diseases: pooled analysis of four perspective cohort studies. Psychol. Med. 44 , 2629–2640 (2014)
SJ Karau, RR Schmeck, AA Avdic, The big five personality traits, learning styles, and academic achievement. Journal on Personality and Individual Differences 51 (4), 472–477 (September 2011)
Morris, R. N., Forensic Handwriting Identification: Fundamental Concepts and Principles, 2000.
K Amend et al., Handwriting Analysis: The Complete Basic Book (Borgo Press, San Bernardino, California, 1981)
MB Menhaj, F Razzazi, A new fuzzy character segmentation algorithm for Persian/Arabic typed texts. International Conference on Computational Intelligence, Fuzzy Days 1999 : .Computational Intelligence, 151–158 (1999)
R Coll, A Fornes, J Llados, Graphological analysis of handwritten text documents for human resources recruitment. 12th International Conference on Document Analysis and Recognition, 1081–1085 (July 2009)
EM Hicham, H Akram, S Khalid, Using features of local densities, statistics and HMM toolkit (HTK) for offline Arabic handwriting text recognition. Journal of Electrical Systems and Information Technology 4 (3), 387–396 (2017)
B Epshtein, E Ofek, Y Wexler, Detecting text in natural scenes with stroke width transform. Computer Vision and Pattern Recognition (CVPR), 2010 IEEE Conference on (August 2010)
L Deng, The MNIST database of handwritten digit images for machine learning research [best of the web]. IEEE Signal Process. Mag. 29 (6), 141–142 (November 2012)
L Xiaoyuang, Q Bin, W Lu, A new improved BP neural network algorithm. Second International Conference on Intelligent Computation Technology and Automation, 19–22 (October 2009)
Download references
Availability of data and materials
Data is not shared publicly. Please contact the author for data requests.
Author information
Authors and affiliations.
Department of Telecommunications, University “Politehnica” of Bucharest, 1-3 Iuliu Maniu Blvd, 06107, Bucharest 6, Romania
Mihai Gavrilescu & Nicolae Vizireanu
You can also search for this author in PubMed Google Scholar
Contributions
MG contributed to the state-of-the-art research, implementation of the neural network-based testbed and methods employed in Scala using Spark library, testing the proposed architecture and discussion around the results and conclusions. NV contributed to the discussion around the results obtained and conclusions. Both authors read and approved the final manuscript.
Corresponding author
Correspondence to Mihai Gavrilescu .
Ethics declarations
Competing interests.
The authors declare that they have no competing interests.
Publisher’s Note
Springer Nature remains neutral with regard to jurisdictional claims in published maps and institutional affiliations.
Rights and permissions
Open Access This article is distributed under the terms of the Creative Commons Attribution 4.0 International License ( http://creativecommons.org/licenses/by/4.0/ ), which permits unrestricted use, distribution, and reproduction in any medium, provided you give appropriate credit to the original author(s) and the source, provide a link to the Creative Commons license, and indicate if changes were made.
Reprints and permissions
About this article
Cite this article.
Gavrilescu, M., Vizireanu, N. Predicting the Big Five personality traits from handwriting. J Image Video Proc. 2018 , 57 (2018). https://doi.org/10.1186/s13640-018-0297-3
Download citation
Received : 20 April 2018
Accepted : 25 June 2018
Published : 10 July 2018
DOI : https://doi.org/10.1186/s13640-018-0297-3
Share this article
Anyone you share the following link with will be able to read this content:
Sorry, a shareable link is not currently available for this article.
Provided by the Springer Nature SharedIt content-sharing initiative
- Neural networks
- Handwriting analysis
- Personality classification
- Feature classification
Academia.edu no longer supports Internet Explorer.
To browse Academia.edu and the wider internet faster and more securely, please take a few seconds to upgrade your browser .
- We're Hiring!
- Help Center
Personality Traits
- Most Cited Papers
- Most Downloaded Papers
- Newest Papers
- Last »
- Maritime Education Follow Following
- Seafaring Follow Following
- Research Methods for Business Students Follow Following
- School Climate(Pupil and Teacher Discipline) Follow Following
- Behavior Follow Following
- Psychological distress Follow Following
- Big Five Personality Traits Follow Following
- Conceptual Modelling Follow Following
- Parenting Style Follow Following
- Case Studies Follow Following
Enter the email address you signed up with and we'll email you a reset link.
- Academia.edu Publishing
- We're Hiring!
- Help Center
- Find new research papers in:
- Health Sciences
- Earth Sciences
- Cognitive Science
- Mathematics
- Computer Science
- Academia ©2024
Help | Advanced Search
Computer Science > Computation and Language
Title: inclusivity in large language models: personality traits and gender bias in scientific abstracts.
Abstract: Large language models (LLMs) are increasingly utilized to assist in scientific and academic writing, helping authors enhance the coherence of their articles. Previous studies have highlighted stereotypes and biases present in LLM outputs, emphasizing the need to evaluate these models for their alignment with human narrative styles and potential gender biases. In this study, we assess the alignment of three prominent LLMs - Claude 3 Opus, Mistral AI Large, and Gemini 1.5 Flash - by analyzing their performance on benchmark text-generation tasks for scientific abstracts. We employ the Linguistic Inquiry and Word Count (LIWC) framework to extract lexical, psychological, and social features from the generated texts. Our findings indicate that, while these models generally produce text closely resembling human authored content, variations in stylistic features suggest significant gender biases. This research highlights the importance of developing LLMs that maintain a diversity of writing styles to promote inclusivity in academic discourse.
Subjects: | Computation and Language (cs.CL); Artificial Intelligence (cs.AI) |
Cite as: | [cs.CL] |
(or [cs.CL] for this version) |
Submission history
Access paper:.
- HTML (experimental)
- Other Formats

References & Citations
- Google Scholar
- Semantic Scholar
BibTeX formatted citation

Bibliographic and Citation Tools
Code, data and media associated with this article, recommenders and search tools.
- Institution
arXivLabs: experimental projects with community collaborators
arXivLabs is a framework that allows collaborators to develop and share new arXiv features directly on our website.
Both individuals and organizations that work with arXivLabs have embraced and accepted our values of openness, community, excellence, and user data privacy. arXiv is committed to these values and only works with partners that adhere to them.
Have an idea for a project that will add value for arXiv's community? Learn more about arXivLabs .
- DOI: 10.54097/ijeh.v8i3.8328
- Corpus ID: 259463831
Analysis of the Characters' Personalities in Everyday Use from the Perspective of Violating the Principle of Cooperation
- Wenlong Liu , Yanying Wang
- Published in International Journal of… 17 May 2023
- Education, Linguistics, Psychology
5 References
Related papers.
Showing 1 through 3 of 0 Related Papers
Kellogg School of Management at Northwestern University
Marketing Jul 1, 2024
Gen ai can tailor ads to our personalities—and they’re pretty persuasive, “the effects are probably only likely to get stronger as time persists.”.
Sandra Matz
Jacob D. Teeny
Sumer S. Vaid
Heinrich Peters
Think of your favorite commercial. Maybe it makes you laugh out loud or tugs at your heart strings.
Now, imagine that every ad you see online speaks to you like this. It’s almost as if the advertisers know you, your personality, and the things that matter to you.
Welcome to personalized advertising in the age of generative artificial intelligence (gen AI). Advances in gen AI and our expanding digital footprints are not only making highly personalized ads possible, they’re making them more likely, says Jacob Teeny , an assistant professor of marketing at the Kellogg School of Management.
“This specific influence tactic, personalized persuasion, is now going to be more scalable and widely deployed than ever before,” Teeny says.
By using free applications like ChatGPT—along with personal information about our browsing behavior, social-media posts, smartphone use, and more—advertisers can create highly personalized and therefore influential marketing appeals.
To learn just how effective these customized advertisements can be, Teeny teamed up with a group of researchers including Sandra Matz, Heinrich Peters, and Moran Cerf of Columbia Business School; and Sumer Vaid and Gabriella Harari of Stanford University.
The researchers set up a robust series of studies that gauged how consumers reacted to personalized pitches written by ChatGPT. Overall, they found that this gen AI was able to write messages tailored to people’s complex psychological profiles, and these messages were more persuasive than non-tailored or generic messages.
Personality traits influence ad preference
In an initial study, the researchers showed 120 participants pairs of messages advertising an iPhone and asked them which message they preferred. One message was designed by ChatGPT to appeal to someone who scored very high on a given personality trait, and a second message appealed to someone who scored very low on that same trait.
For instance, an excerpt from the ad for extroverts read: “If you’re the life of the party, always up for a good time, and enjoy being surrounded by people, then this is the phone for you!” Meanwhile, an excerpt from the ad for introverts read: “If you’re looking for a phone that won’t draw attention to itself, the iPhone is the perfect choice.”
“We’ve seen a bit of cultural shifting in terms of the acceptability of being persuaded.” — Jacob Teeny
Participants then rated which message was more persuasive and which made them want to get an iPhone. They then took a 30-question survey to identify their own personality traits.
The researchers found that for three of the four personality traits tested, the personalized ad designed by ChatGPT (as determined by whether participants scored high or low for a given trait on the personality survey) was more persuasive than the non-personalized ad.
Consumers don’t mind being targeted
But how would people respond if they knew the message had been personalized to them using gen AI?
For the next study, 471 participants completed the same tasks described above but with a wrinkle. One group of participants served as a baseline group, while another group was told that gen AI had created the ad for them based on their personality traits.
Again, the researchers found that the personalized ads were effective overall, replicating the original finding. But they also found that the participants knowing the ads had been generated by gen AI to appeal to them specifically didn’t change the ads’ effectiveness.
That’s notable because prior research has shown that people try to resist being swayed when they know someone is trying to persuade them, Teeny says.
“We’ve seen a bit of cultural shifting in terms of the acceptability of being persuaded. When we go online, we know we’re getting targeted ads. And because that’s just become the norm so much, I think people are more OK with it,” Teeny said.
Simulating how advertisers use AI
Finally, the researchers wanted to use what they had learned and put it into action—much in the same way that advertisers would use consumer data. So, they invited 297 of their earlier participants back for another study more than half a year later.
For each participant, they relied on their previous data to identify their most salient personality trait (out of extroversion, openness, and conscientiousness) and then used that to have gen AI create new personalized ads, this time promoting a Rome getaway or a pair of Nike sneakers.
Participants were shown both ChatGPT’s personalized and generic ads for each offering and rated the persuasiveness of the ads as well as how much they would be willing to pay for them.
This time, using messages that were personalized to each participant’s personality from data collected half a year ago, the researchers continued to find that the personalized ads from gen AI were more effective than the generic ads. This was particularly true for the trip to Rome relative to the sneakers.
This makes sense, Teeny says, since someone’s personality might be more at play when selecting experiences (which can speak to many of a person’s traits) than a consumer product (which may speak to fewer). After all, extroverts and introverts both wear shoes, but will likely have varying preferences for how they’d like to spend their free time.
“The more that there’s an opportunity to tailor the service or the product to a person’s predisposition, the more that generative AI or even a human author should be able to tailor a message to appeal, and thereby influence, your interests and behavior,” he says.
Don’t believe everything you read online
All of this sends a clear message to marketers: personalizing messages to consumers is greatly effective, and free AI tools are making it easier than ever.
For consumers, the flipside is that this new frontier of messaging requires everyone to be even more mindful of the content they read online, Teeny says.
“We’re going to be inundated with things that naturally feel like they appeal to us. So we might have to take a second step to really investigate the source or the veracity of the message—whether that’s in regard to a consumer product or in regard to a political news article,” he says.
Not taking that extra beat to stop and think might mean buying a product you really don’t need or voting for a politician who never endorsed the messages attributed to him or her, he says.
From a policy standpoint, the research suggests that social platforms may need to do more than simply flag AI-generated content to protect users from being persuaded by misinformation. “Meta is implementing a new policy where they have to say if an image was created or an ad was created with AI. I think that’s a great first step, but as we see in this paper, that doesn’t necessarily resolve the issue,” he says.
As gen AI continues to improve and evolve, it will create even more persuasive content, Teeny says. The study was done using ChatGPT-3, which is already outdated, and it’s likely that newer versions will get better at incorporating more facets of someone’s personality in a single ad.
“The effects are probably only likely to get stronger as time persists,” he says.
Assistant Professor of Marketing
Previously a member of the Marketing Department faculty at Kellogg
Amy Hoak is a freelance writer in Chicago.
Matz, Sandra, Jacob D. Teeny, Sumer S. Vaid, Heinrich Peters, Gabriella Harari, and Moran Cerf. 2024. "The Potential of Generative AI for Personalized Persuasion at Scale. Scientific Reports. 14 No. 4692.
Read the original
We’ll send you one email a week with content you actually want to read, curated by the Insight team.
Predicting the Big Five Personality Traits in Chinese Counselling Dialogues Using Large Language Models
- Ma, Jingsong
- Lan, Zhenzhong
Accurate assessment of personality traits is crucial for effective psycho-counseling, yet traditional methods like self-report questionnaires are time-consuming and biased. This study exams whether Large Language Models (LLMs) can predict the Big Five personality traits directly from counseling dialogues and introduces an innovative framework to perform the task. Our framework applies role-play and questionnaire-based prompting to condition LLMs on counseling sessions, simulating client responses to the Big Five Inventory. We evaluated our framework on 853 real-world counseling sessions, finding a significant correlation between LLM-predicted and actual Big Five traits, proving the validity of framework. Moreover, ablation studies highlight the importance of role-play simulations and task simplification via questionnaires in enhancing prediction accuracy. Meanwhile, our fine-tuned Llama3-8B model, utilizing Direct Preference Optimization with Supervised Fine-Tuning, achieves a 130.95\% improvement, surpassing the state-of-the-art Qwen1.5-110B by 36.94\% in personality prediction validity. In conclusion, LLMs can predict personality based on counseling dialogues. Our code and model are publicly available at \url{https://github.com/kuri-leo/BigFive-LLM-Predictor}, providing a valuable tool for future research in computational psychometrics.
- Computer Science - Computation and Language;
- Computer Science - Artificial Intelligence
Information
- Author Services
Initiatives
You are accessing a machine-readable page. In order to be human-readable, please install an RSS reader.
All articles published by MDPI are made immediately available worldwide under an open access license. No special permission is required to reuse all or part of the article published by MDPI, including figures and tables. For articles published under an open access Creative Common CC BY license, any part of the article may be reused without permission provided that the original article is clearly cited. For more information, please refer to https://www.mdpi.com/openaccess .
Feature papers represent the most advanced research with significant potential for high impact in the field. A Feature Paper should be a substantial original Article that involves several techniques or approaches, provides an outlook for future research directions and describes possible research applications.
Feature papers are submitted upon individual invitation or recommendation by the scientific editors and must receive positive feedback from the reviewers.
Editor’s Choice articles are based on recommendations by the scientific editors of MDPI journals from around the world. Editors select a small number of articles recently published in the journal that they believe will be particularly interesting to readers, or important in the respective research area. The aim is to provide a snapshot of some of the most exciting work published in the various research areas of the journal.
Original Submission Date Received: .
- Active Journals
- Find a Journal
- Proceedings Series
- For Authors
- For Reviewers
- For Editors
- For Librarians
- For Publishers
- For Societies
- For Conference Organizers
- Open Access Policy
- Institutional Open Access Program
- Special Issues Guidelines
- Editorial Process
- Research and Publication Ethics
- Article Processing Charges
- Testimonials
- Preprints.org
- SciProfiles
- Encyclopedia
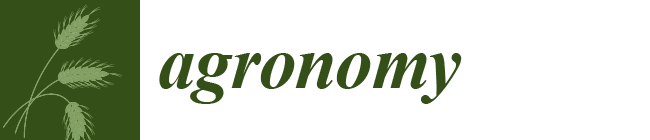
Article Menu
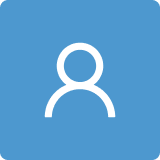
- Subscribe SciFeed
- Recommended Articles
- Google Scholar
- on Google Scholar
- Table of Contents
Find support for a specific problem in the support section of our website.
Please let us know what you think of our products and services.
Visit our dedicated information section to learn more about MDPI.
JSmol Viewer
Water management and hydrological characteristics of paddy-rice fields under alternate wetting and drying irrigation practice as climate smart practice: a review.
Share and Cite
Bwire, D.; Saito, H.; Sidle, R.C.; Nishiwaki, J. Water Management and Hydrological Characteristics of Paddy-Rice Fields under Alternate Wetting and Drying Irrigation Practice as Climate Smart Practice: A Review. Agronomy 2024 , 14 , 1421. https://doi.org/10.3390/agronomy14071421
Bwire D, Saito H, Sidle RC, Nishiwaki J. Water Management and Hydrological Characteristics of Paddy-Rice Fields under Alternate Wetting and Drying Irrigation Practice as Climate Smart Practice: A Review. Agronomy . 2024; 14(7):1421. https://doi.org/10.3390/agronomy14071421
Bwire, Denis, Hirotaka Saito, Roy C. Sidle, and Junko Nishiwaki. 2024. "Water Management and Hydrological Characteristics of Paddy-Rice Fields under Alternate Wetting and Drying Irrigation Practice as Climate Smart Practice: A Review" Agronomy 14, no. 7: 1421. https://doi.org/10.3390/agronomy14071421
Article Metrics
Article access statistics, further information, mdpi initiatives, follow mdpi.
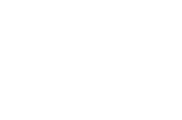
Subscribe to receive issue release notifications and newsletters from MDPI journals

COMMENTS
Abstract. This review paper on the current status of the studies on personality traits, aimed at summarizing the progress achieved in the study of personality traits and examining the evidences ...
1. Current conceptualization of personality. The Five Factor Model (FFM) of personality has guided research and theory building for almost three decades (John, Naumann, & Soto, 2008).FFM, also known as the Big Five model, contends that the construct of personality includes Basic Tendencies or traits that are biologically-based, as well as Characteristic Adaptations that result from dynamic ...
The Big Five—Extraversion, Agreeableness, Conscientiousness, Neuroticism and Openness to Experience— are a set of five. broad, bipolar trait dimensions that constitute the most widely used ...
Personality traits can be defined as broad patterns of thoughts, feelings, and behaviors (Lucas & Donnellan, 2011).Early empirical research on personality mainly focused on the structure, measurement, and consequences of traits (e.g., Digman, 1990).Stability and change in traits were less common topics, largely because traits were regarded as highly stable once people reach adulthood (McCrae ...
In this paper, we review the current state of scientific evidence regarding the nature, sources, and processes of personality trait stability and change. We revisit past disputes over the relative ...
Personality traits were measured using 50 items from the IPIP when participants were 67-71 years old in 2006 and then three times more in 2008, 2012, and 2016 for a ... For the duration of this paper, 'baseline' refers to the initial assessment of personality for each study. ... Journal of Research in Personality, 70, 174-186. 10.1016/j ...
Personality psychologists generally believe that there are five major dimensions that can be used to categorize a wide range of possible personality traits. These dimensions are referred to as the Big Five, and they include Extraversion, Neuroticism, Agreeableness, Conscientiousness, and Openness to experience 12.
Another widely studied indicator is the facial width to height ratio (fWHR), which has been linked to various traits, such as achievement striving 10, deception 11, dominance 12, aggressiveness 13 ...
About the journal. Emphasizing experimental and descriptive research, the Journal of Research in Personality presents articles that examine important issues in the field of personality and in related fields basic to the understanding of personality. The subject matter includes treatments of genetic, physiological, …. View full aims & scope.
Personality traits and personal values are important psychological characteristics, serving as important predictors of many outcomes. ... Journal of Research in Personality, 30, 23-41. Crossref. ISI. Google Scholar. ... Paper presented at the Annual Meeting for the Society for Industrial and Organizational Psychology, Dallas, TX. ...
However, there is sparse research available in the literature that explains how does personality traits affect innovativeness among individuals and satisfaction with life perceptions (subjective wellbeing). The current study proposes and empirically examines a conceptual model that addresses this important gap in the body of knowledge.
The Big Five personality traits are the most widely used and recognized model as a comprehensive taxonomy of individual differences in human personality (John & Srivastava, Citation 1999). Stake and Eisele ( Citation 2010 ), considered the Big Five to provide a comprehensive map of universal personality traits.
important theori es o f per sonality (Ewen. 2003), and the big five traits (BFT) repre-. sent the heart of the theory of personality. traits to descript, interpret, and predict hu-. man behavior ...
This study aims to assess the impact of personality traits on emotional intelligence (EI) and decision-making among medical students in Lebanese Universities and to evaluate the potential mediating role-played by emotional intelligence between personality traits and decision-making styles in this population. This cross-sectional study was conducted between June and December 2019 on 296 general ...
The findings of research on the role of personality traits on education have been mixed, with most effects insignificant or very small (Poropat, 2009). ... While the approach taken in this paper may not please some clinical psychologists, the intention is to identify a path forward for integrating personality traits into mainstream social ...
Introduction. In a seminal study, Borghans et al. [] propose a conceptualization of personality traits as skills that make an independent contribution to the map of individuals' preferences, choices and behaviours compared with the conventional measures of mental or cognitive skills adopted by economists.While foremost economic literature has thoroughly examined the role of cognitive ...
The impact of dysfunctional attitudes and unhelpful thinking upon clients presenting with personality-related psychological distress is an important clinical area of investigation as it informs psychological interventions. Despite this, there is limited research in this area. Thus, this study had two main aims: (1) examine the interrelationships between maladaptive personality traits ...
We propose the first non-invasive three-layer architecture in literature based on neural networks that aims to determine the Big Five personality traits of an individual by analyzing offline handwriting. We also present the first database in literature that links the Big Five personality type with the handwriting features collected from 128 subjects containing both predefined and random texts.
Personality traits and personal values: a conceptual and empirical integration. Independent advances in the study of personality traits and personal values have clarified their foundations, antecedents, content, structure, and measurement. Accumulating evidence shows that personality traits are largely endogenous... more. Download. by Jim Olver.
This research highlights the importance of developing LLMs that maintain a diversity of writing styles to promote inclusivity in academic discourse. Subjects: ... View a PDF of the paper titled Inclusivity in Large Language Models: Personality Traits and Gender Bias in Scientific Abstracts, by Naseela Pervez and 1 other authors. View PDF;
This study examines the personality traits of characters in Everyday Use through the lens of violating the cooperative principle. The purpose is to analyze how characters' communication behaviors reflect their individual personalities. The methods include identifying examples of violating the cooperative principle in the text and analyzing their implications for character traits. The findings ...
The Big Five Personality T raits. Personality traits include relatively stable patterns of cognitions, beliefs, and behaviors. The Big Five model has functioned as the powerful theoretical ...
This paper arises from the question of the correlation between specific personality traits and academic performance, since it is of crucial importance to consider variables other to students' grades that also affect this phenomenon. ... The purpose of this research was to correlate the personality structure and academic performance of ...
They then took a 30-question survey to identify their own personality traits. The researchers found that for three of the four personality traits tested, the personalized ad designed by ChatGPT (as determined by whether participants scored high or low for a given trait on the personality survey) was more persuasive than the non-personalized ad.
Accurate assessment of personality traits is crucial for effective psycho-counseling, yet traditional methods like self-report questionnaires are time-consuming and biased. This study exams whether Large Language Models (LLMs) can predict the Big Five personality traits directly from counseling dialogues and introduces an innovative framework to perform the task.
The continuities, consistencies and stabilities of personality traits and dispositions over time define personality development (Larsen & Buss, 2008). Discover the world's research 25+ million members
The X-type strike-slip fault system and weathering crust karst fracture-cave and channel reservoirs were developed in the Halahatang area of the northern Tarim Basin. However, the relationship between the reservoir and the strike-slip fault remains controversial. Based on the core data, and taking an NE-striking strike-slip fault as an example, this paper dissects the karst reservoir from ...
In contrast, research shows that personality traits continue to change in adulthood (e.g., Roberts, Walton, ... Preparation of this paper was supported by National Institute of Aging Grants AG19414 and AG20048; National Institute of Mental Health Grants MH49414, MH45070, MH49227; United Kingdom Medical Research Council Grant G0100527; and by ...
2.1. Inclusion and Exclusion Criteria. Studies that were included in this review are (i) full-text articles; (ii) articles published in Sage, Web of Science, APA PsycNet, Wiley Online Library, and PubMed; (iii) research with at least 20 respondents (to reduce the bias associated with a small sample size; (iv) studies that examine the link between personality traits and criminal behaviour; and ...
A Feature Paper should be a substantial original Article that involves several techniques or approaches, provides an outlook for future research directions and describes possible research applications. Feature papers are submitted upon individual invitation or recommendation by the scientific editors and must receive positive feedback from the ...